DOI:
10.1039/D2RA00966H
(Paper)
RSC Adv., 2022,
12, 13896-13916
Quality by design approach for green HPLC method development for simultaneous analysis of two thalassemia drugs in biological fluid with pharmacokinetic study†
Received
14th February 2022
, Accepted 21st April 2022
First published on 9th May 2022
Abstract
This work implements a combined experimental approach of analytical quality-by-design (AQbD) and green analytical chemistry (GAC) to develop an HPLC method for simultaneous determination of the two thalassemia drugs, deferasirox (DFX) and deferiprone (DFP), in biological fluid for the first time. This integration was designed to maximize efficiency and minimize environmental impacts, as well as energy and solvent consumption. To accomplish this goal, an analytical quality-by-design approach was performed, beginning with quality risk assessment and scouting analysis, followed by Placket–Burman design screening for five chromatographic parameters. Critical method parameters were thoroughly recognized and then optimized by using a two levels-three factors custom experimental design to evaluate the optimum conditions that achieved the highest resolution with acceptable peak symmetry within the shortest run time. The desirability function was used to define the optimal chromatographic conditions, and the optimal separation was achieved using an XBridge® HPLC RP-C18 (4.6 × 250 mm, 5 μm) column with ethanol
:
acidic water at pH 3.0 adjusted by phosphoric acid in the ratio of (70
:
30, v/v) as the mobile phase at a flow rate of 1 mL min−1 with UV detection at 225 nm at a temperature of 25 °C. Linearity was obtained over the concentration range of 0.30–20.00 μg mL−1 and 0.20–20.00 μg mL−1 for DFX and DFP, respectively, using 20.00 μg mL−1 ibuprofen (IBF) as an internal standard. The established method's greenness profile was evaluated and measured using various assessment tools, and the developed method was green. For the validation of the developed method, FDA recommendations were followed, and all the results obtained met the acceptance criteria. The suggested method was successfully used to study the pharmacokinetic parameters of DFX and DFP in rat plasma. Due to the substantial increase in bioavailability of the two iron chelating drugs, the results from this study strongly recommend their co-administration.
1. Introduction
Thalassemia is an inherited blood disorder in which the body produces an aberrant form or an insufficient amount of an oxygen-carrying component of red blood cells (hemoglobin). Anemia is caused by this disorder due to the large number of red blood cells destroyed. To improve the anemia and to suppress the ineffective erythropoiesis, blood transfusion is indicated to prevent most of the serious growth, skeletal, and neurological complications of thalassemia.1 Iron overload can occur in people who have a lot of blood transfusions. Because red blood cells contain a lot of iron, the iron from all of the transfusions can accumulate in the body over time. When iron builds up in organs like the heart, liver, and brain, it can make it difficult for these systems to function effectively. To avoid the serious consequences of iron overload, labile plasma iron (LPI) must be suppressed and excess iron must be removed from the bloodstream. Iron chelation therapy may be required for people with thalassemia to avoid iron accumulation, and it is necessary to prevent organ failure and decrease mortality.2 An iron chelating agent binds ferric ions, reducing their reactivity dramatically. The ultimate complex is water soluble and can easily enter the bloodstream and excrete it without causing harm.3 The two main oral bioavailable iron chelating drugs which are frequently used for the treatment of iron overload and thalassemia conditions are deferasirox and deferiprone.4 Deferasirox (DFX), 4-[3,5-bis(2-hydroxyphenyl)-1H-1,2,4-triazol-1-yl]-benzoic acid,5 Fig. 1S, ESI,† It is a tridentate oral iron chelator that works by binding to ferric iron and releasing stored iron. It eliminates cellular (cardiac and hepatic) and serum iron from the body by excreting it in the faeces.6 On the other hand, deferiprone (DFP), (3-hydroxy-1,2-dimethylpyridin-4-one),5 Fig. 1S, ESI,† is an oral iron chelator with a 3
:
1 molar ratio of iron binding. It has the ability to inhibit the accumulation of iron in tissues and serum. It is mostly eliminated through the kidneys after being absorbed in the upper gastrointestinal tract.7
Many studies have shown that co-administering DFX and DFP in severe thalassemia has an additive effect that reduces serum ferritin levels considerably in patients with severe iron overload, and the two combined drugs were tolerated well without any serious adverse effects. This oral combination was determined to be safe, effective, low-cost, and a reasonable option for patients who did not respond optimally to monotherapy.8,9 By surveying the literature review of these drugs, it was found that numerous methods have been reported for the detection of DFX alone in bulk or in its pharmaceutical formulation or in biological fluids, including spectrophotometric10–12 and HPLC13–24 methods. Additionally, DFP was determined alone either in bulk or in human plasma by different methods like spectrophotometric,25–27 spectrofluorimetric,28 HPLC,29–34 capillary zone electrophoresis,34 and electrochemical35,36 methods. There were only two published voltammetric methods for determining the two proposed drugs simultaneously.37,38
The aim of this work is to establish a green and robust HPLC method for analysis of the two oral chelating drugs in biological fluid by using a combined approach of GAC and AQbD for the first time. AQbD is a pre-validation methodology that aims to establish a comprehensive framework of method variables and incorporate robustness into methods during development rather than its assessment in the final stage. As a result, it conserves valuable resources and time while facilitating method transfer without the requirement for re-validation.39 It is predicated on statistical principles and experimental designs for risk assessment, parameter screening, and optimization.40 HPLC separation is controlled by a large number of variables, including mobile phase pH, column temperature, buffer concentration, flow rate, percentage of organic modifiers, detecting wavelength, etc. HPLC method development strategies were extended for a long time by trial and error, aided by the analyst's expertise and knowledge. This traditional one factor at a time (OFAT) technique takes time, effort, and funds while producing results that are only partially justified.41 On the other hand, the design of experiments (DOE) technique employs the fewest possible experiments to determine the influence of factors and their interactions on measured responses in order to obtain the desired outcome. A step-by-step description of the AQbD methodology was tracked where critical method parameters were identified and optimized by experimental design. Custom design is a highly adaptable experimental approach that is matched to the available budget and desired output. It enables analysts to define factor constraints and evaluate the major effects and interactions using the fewest possible experiments.41–43
Green chemistry ideas are widely employed in global industrial management, government policy, educational practice, and technology development. The circular economy's fundamental goal is to strike a balance between economic growth, resource sustainability, and environmental protection. Green analytical chemistry (GAC), which results in a shift in attitudes and behavior in the chemical industry, can be seen as a critical tool for achieving sustainability.44 The correct application of GAC provides many advantages in different aspects of sustainability. However, we have to believe that GAC is a journey and not a destination. It is a multi-step approach, starting from selecting or modifying an appropriate analytical method that should meet the specified performance criteria of being a direct analysis method, performing real-time analysis, and being a multi-analyte or multi-parameters method, then using less toxic or hazardous solvents and reagents in sample pretreatment and analytical measurements, passing by depending on renewable feedstocks or substrates and avoiding derivatization to minimize solvent use. Additionally, resorting to in situ measurements on the basis of (in or on-line) analysis decreases sample size and sample number, saving time, cost, and energy consumption. An analytical waste management approach should be applied to protect the environment from pollutants. Finally, keep the analytical operator safe by conducting all the safety precautions and restrictions that provide eco-friendly analytical methods and maintain sustainability development. These steps align with the twelve principles stated concerning GAC.45
Thus, the integration between GAC principles and the AQbD approach acts as a synergistic platform for developing environmentally benign methods with high efficiency and operational flexibility.46 Therefore, to achieve this integration, a green and selective HPLC method was developed using a custom experimental design with minimal trials and maximum efficiency. The greenness profile of the proposed method was evaluated by using eight greenness tools: national environmental methods index (NEMI), modified NEMI, analytical method volume intensity (AMVI), analytical eco-scale, analytical method greenness score (AMGS), environmental assessment tool (HPLC-EAT), green analytical procedure index (GAPI), and analytical greenness (AGREE) tools. After making a comparison between the developed and the reported methods, the developed HPLC method exhibited more green performance than the reported ones.19,31 In rat plasma, the pharmacokinetic characteristics of DFX and DFP were carefully calculated, and the results were effectively confirmed according to FDA guidelines.47 The proposed HPLC method was effectively applied to DFX and DFP pharmacokinetic studies due to its being simple, affordable, precise, accurate, and sensitive procedures with a short analytical time.
2. Experimental
2.1. Instruments
2.1.1 For HPLC method. The Dionex Ultimate 3000 UHPLC was employed. It had a quaternary solvent delivery pump, an autosampler, and a diode array detector (Massachusetts, USA). Developing and quantification were carried out using an XBridge® HPLC RP-C18 reversed phase stationary phase column (4.6 × 250 mm, 5 μm) (California, USA). A 0.45 μm millipore membrane filter, and nylon membrane filters and 0.22 μm MS® disposable syringe filters were used to filter the mobile phase and samples, respectively. Mobile phase pH adjustment was conducted during the work using a Jenway pH-meter (model 3510, Staffordshire, UK). A digital balance (Sartorius, German), Sonix TV SS-series ultrasonicator (South Carolina, USA) were used.
2.1.2 For the preparation of plasma samples. For sample preparation, a vortex mixer with 250 VM (Hwashin, Seoul, Korea) and a rongtai variable volume micropipette device with 0.1–100.0 μL volume (Mainland, Shanghai, China) were utilized. For sampling centrifugation, an 80–2C low-speed electric centrifuge with 1968 (g) (Zjmzym, China) with a capacity of 12 × 20 mL and a power source of 110 V/220 V was employed.
2.2. Software programmes
Placket–Burman, custom experimental designs, and data analysis calculations were accomplished using the JMP® Pro software version 16.0.0. SAS Institute Inc., Cary, North Carolina, USA software, copyright 2021. The output signal of the HPLC chromatogram was monitored and analyzed using Chromeleon software.
2.3. Chemicals and reagents
2.3.1. Pure sample. Deferasirox and deferiprone were purchased from Ambeed, Inc. for chemicals (Arlington Heights, USA). Their purities were estimated using the reported HPLC procedures19,31 and were confirmed to be 99.33 ± 0.74 and 99.71 ± 0.89, respectively. While ibuprofen (IBF) that was utilized as internal standard material (IS), was generously gifted by Hikma Pharmaceuticals (6th of October City, Egypt) with a purity of 99.66 ± 1.06 according to the manufacturer's certifications of analysis.
2.3.2. Pharmaceutical formulation. Exjade® tablets and Kelfer® capsules are branded to contain 250 mg of DFX and DFP, respectively. They are manufactured by Novartis Pharmaceuticals (Basel, Switzerland) and Cipla Limited (Mumbai, India), respectively. They were obtained from the local Egyptian market.
2.3.3. Solvents. All the solvents used were of analytical grade and they were consumed without any additional purification. Acetonitrile and ethanol were of HPLC grade (Fisher, Loughborough, UK). Phosphoric acid was given by (El-Nasr Pharmaceutical Chemical Co., Abu Zabaal, Cairo, Egypt). Deionized water was gotten from SEDICO Pharmaceuticals Co. (6th October City, Egypt).
2.4. Solutions
Standard stock solutions of DFX, DFP and IBF with concentrations of 1.00 mg mL−1 were prepared in ethanol. Each drug was weighed into three separate 100 mL volumetric flasks, dissolved in 50.0 mL of ethanol, and finished to the mark with ethanol before being stored at 2 °C for future use.
Ten Exjade® tablets and Kelfer® capsules that contained 250 mg of DFX and DFP, respectively, were separately grounded to an even consistency. An amount equivalent to 50 mg of DFX and DFP was accurately weighed and transferred into two separate 50 mL volumetric flasks, and the volume was completed with ethanol to obtain concentrations of 1.00 mg mL−1 of pharmaceutical formulation stock solutions. To obtain appropriate working solutions of DFX, DFP, and IBF (IS) (0.10 mg mL−1), the stock solutions were extra diluted with the mobile phase mixture for further application.
3. Procedure
3.1. Experimental design
JMP® software, version 16.0.0 Copyright 2021, by SAS Institute Inc., Cary, North Carolina, USA, was used to create a two-level-three-factor custom experimental with a single center point. Using the Placket–Burman design and five variables with two levels, an initial screening analysis was conducted to identify the essential parameters impacting chromatographic separation. For screening, the independent variables of the flow rate, temperature, percentage of organic solvent in mobile phase, pH in mobile phase, and detection wavelength were chosen. For each run, the run time, DFX and DFP tailing factors, and DFX and DFP resolution were recorded and used as dependent variables (responses). The standard least square method was used to compute the second-order polynomial equations that describe the quadratic models and indicate the interaction between the components. The data was statistically examined, and the desirability function was used to accomplish the final optimization. The run time and tailing factors were tuned to be as short as possible, while the resolution was set to be as high as possible.
3.2. Animals
Adult female Wister Albino rats were taken from the Nahda Animals Facility at Nahda University (Beni-Suef, Egypt) and fed a standard laboratory rat diet and water until they reached weights of 180–220 g. Rats were acclimatized in the laboratory for a week prior to the studies and housed in separate cages in animal facilities at 22–25 °C, with a 12 hour light/dark cycle and 50% relative humidity, and with free access to standard food and water. Rats were given free access to water and were fasted for 12 hours before the studies. All rat housing and handling was carried out in accordance with the testing guidelines established by the Animal Care Committee of the National Research Center (Cairo, Egypt), which followed the recommendations of the National Institutes of Health Guidelines for the Care and Use of Laboratory Animals.48
3.3. Animal ethical statement
The study was approved by the Ethical Committee of the National Research Center (NRC) (Cairo, Egypt), as long as the animals did not suffer at any stage during the experiment and were cared for in accordance with the guide for the Care and Use of Laboratory Animals (ethical approval no: 17-5-11-2021).
3.4. Chromatographic conditions
HPLC separation was performed using isocratic elution mode on an XBridge® C18 column (250 × 4.6 mm, 5 μm) with a mobile phase consisting of ethanol and acidic water at pH 3.0 adjusted with phosphoric acid in the ratio (70
:
30, % v/v) at a flow rate of 1.00 mL min−1 utilizing IBF as an IS. The run took 6 minutes in total. Each sample was injected in triplicate with 20.0 μL. UV detection was carried out at a wavelength of 225.0 nm and the temperature was set to 25 °C.
3.5. Construction of calibration curves and quality control samples (QCs)
DFX and DFP were made in a series of 10 mL volumetric flasks at concentrations ranging from 0.30–20.00 μg mL−1 and 0.20–20.00 μg mL−1, respectively, using their working solution (0.10 mg mL−1). Each flask was filled with one mL of blank plasma, followed by 2.0 mL of IBF (IS) stock solution (0.10 mg mL−1), and the volume was then adjusted to the mark using ethanol. After vortex mixing for one minute, the produced solutions were centrifuged at 1968 (g) for 30 minutes. The filtrate was completely shifted to a new set of 10 mL volumetric flasks, evaporated to dryness, and then reconstituted in 2.0 mL of ethanol before being topped off with the mobile phase. 20.0 μL of each solution was automatically injected in triplicates using an autosampler to achieve concentrations of 0.30, 0.70, 1.00, 3.00, 5.00, 10.00, 12.00, 15.00, 18.00, and 20.00 μg mL−1 for DFX and 0.20, 1.00, 2.00, 5.00, 7.00, 10.00, 12.00, 15.00, 18.00, and 20.00 μg mL−1 for DFP using IBF (IS) at a concentration of 20.00 μg mL−1. QCs containing DFX at 1.00, 10.00, and 15.00 μg mL−1 and DFP at 1.00, 10.00, and 15.00 μg mL−1 were formulated and analyzed similarly to calibration standards.
3.6. Preparation of the rat plasma samples
Twenty-eight female albino rats were randomly allocated into four groups (n = 7 per group). The first group consisted of rats from a control group that did not receive any medications. However, the second group of rats got a dose of DFX of 185.0 mg kg−1 through extraction of 277.5 mg of Exjade® tablets and dilution to a volume of 6 mL with distilled water. The third group received 465.0 mg of DFP by dissolving 700 mg of Kelfer® capsules in 6 mL of distilled water, and the fourth group received a combination of 92.5 mg kg per DFX and 232.5 mg kg per DFP (half doses of each iron chelating drugs), due to the additive effect of DFX and DFP according to previous studies.49–51 The computed doses of DFX and DFP were then given orally to each rat. Two minutes prior to drug delivery, the animals were sedated with isoflurane. A 0.50 mL blood sample was then taken from each rat by retro-orbital puncture at various time intervals (0.5, 1, 1.5, 2, 4, 6, 12, and 24 hours) post-dosing into a heparinized polythene tube, then directly centrifuged at 1968 (g) for 30 min. Each sample's supernatant layer was isolated and stored at 20 °C for further analysis.
After thawing frozen plasma samples collected from rats to room temperature, 100.0 μL of the plasma samples were accurately transferred into a 1.5 mL centrifuge tube, followed by the addition of 20.0 μL of IBF from their respective stock solutions (1.00 mg mL−1). Following that, the volume was adjusted to 1.0 mL using acetonitrile. After one minute of vortex mixing, samples were centrifuged for 20 minutes at 1968 (g); the clear supernatant from each sample was totally removed and evaporated to dryness. After the samples' dryness, they were reconstituted with a 1.0 mL mobile phase mixture. After that, 20.0 μL of each produced sample was administered in triplicate.
4. Results and discussion
There is a great intersection between the development of eco-friendly, hazardous-free, and less environmentally impacting analytical methods with minimum trials and optimum outcomes. The majority of analytical procedures have either health or environmental concerns, necessitating constant revalidation. As a result, new trends have evolved for incorporating GAC and AQbD concepts into a single technique in order to increase its sustainability and robustness. As a result, chemists worldwide strive to strike a compromise between developing valid, sensitive, and selective analytical procedures and substituting more ecological and energy-efficient technologies. When it comes to green, robust, and sustainable analytical procedures, GAC and AQbD are two sides of the same coin. Fig. 1 illustrates the interference between GAC and the AQbD approach. Optimization of an HPLC method is a complex procedure that requires simultaneous adjustment of various variables in order to achieve the desired separation. Utilizing an experimental design throughout the optimization process has a number of advantages. Among these benefits are improved separation quality, a reduction in the number of experiments, and a reduction in the requirement for laboratory resources and trained labor.52 This article provides a step-by-step explanation of AQbD in accordance with the GAC principles for developing analytical methods.
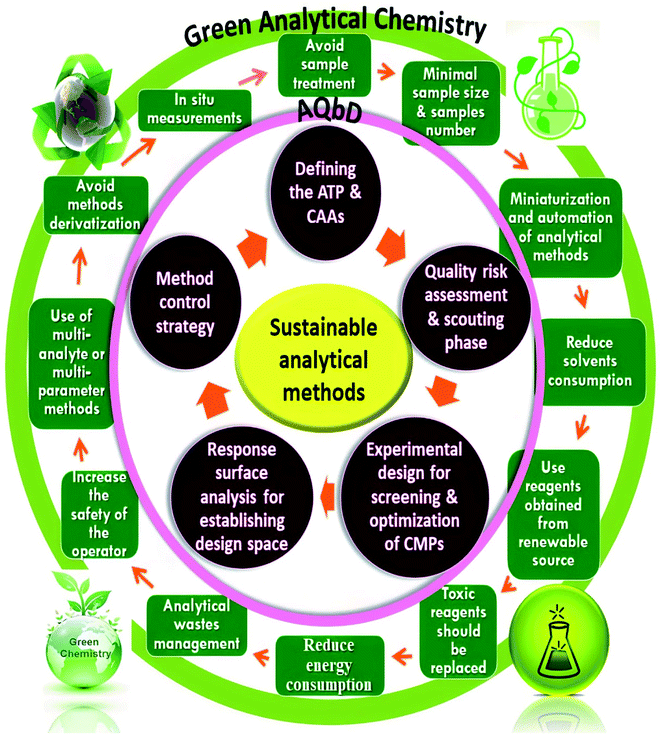 |
| Fig. 1 Integration of the green analytical chemistry (GAC) principles and Quality-by-Design (QbD) steps for sustainable analytical methods. | |
4.1. Defining ATP and CAAs
The first stage in establishing an analytical method is to precisely define the analytical target profile, or ATP, which summarizes the method's primary objectives and anticipated outcomes. It is a statement that specifies the objective of a method and serves as the driving force behind method selection, design, and development activities. ATP is a critical metric in AQbD because it enables the ongoing improvement of analytical methods and their selection following regulatory approval of the ATP statement.38,49 The ATP is used to determine an analytical procedure's fitness during the development phase and throughout its analytical lifecycle. Herein, the ATP in this approach was to develop an accurate, precise, simple, sensitive, and robust analytical method. On the other hand, critical analytical attributes (CAA) are the measurable variables (responses) that reflect method performance. According to preliminary trials, the most significant CAAs were peak resolution (Rs), tailing factor (TF), and run time, with ideal values of Rs ≥ 2, TF between 0.8 and 1.5, and shorter run time.
4.2. Quality risk assessment and scouting phase
A risk-based strategy is necessary as a crucial component of QbD, according to ICH Q8 and Q9.50,51 The Ishikawa fishbone diagram, a science-based benchmark aimed at brainstorming all conceivable analysis parameters and pointing out the likely risk ones based on prior experimentation and knowledge, can be used to monitor quality risk assessment. This tool assists in identifying and prioritizing potential method risks produced by a range of conditions, such as tiny inadvertent changes to method parameters and changes that may occur across laboratories, analysts, instruments, reagents, and the time period in which the method is run. The development of HPLC methods is a sophisticated process in which numerous parameters can influence separation performance, necessitating meticulous monitoring. Fig. 2 depicts the suggested Ishikawa fishbone diagram for the HPLC analysis.52,53
 |
| Fig. 2 Ishikawa diagram to review the effect of all the possible factors on CAAs. | |
Regarding the Ishikawa fishbone diagram, many parameters were investigated for the determination of CAAs. Concerning the organic phase and with respect to GAC principles, ethanol was used as an organic modifier instead of other available solvents. Microextraction was used to extract rat plasma samples, which were then filtered using 0.22 μm disposal membrane filters, which reduce solvent and energy consumption. Isocratic elution mode was run due to having several advantages over gradient elution, including greater simplicity; lower cost, easier instrumentation, and the elimination of the requirement for column reequilibration between injections. Various stationary phases with varying packing materials, lengths, and particle sizes were evaluated, including Hypersil Gold® C18 (2.1 × 50 mm, 1.9 μm), C18 (4.6 × 150 mm, 3 μm), XBridge® HPLC RP-C18 (4.6 × 250 mm, 5 μm), C8 (2.1 × 50 mm, 1.9 μm), and CN (4.6 × 150 mm, 3 μm) columns. Preliminary results indicated that the XBridge® HPLC RP-C18 (4.6 × 250 mm, 5 μm) column adequately retained and separated the studied compounds. The detection wavelength was chosen based on the DAD spectra of the two drugs studied, with 225 nm providing the best sensitivity with minimum noise. The injection flow rate was adjusted by trials to 1 mL min−1 as an optimum flow rate to compromise between good separation and faster elution with short analysis run time.
In contrast, pH scouting studies were done to determine the drug's behavior over the manufacturer-specified pH range (2.5–8.0) for the used C18 column. Additionally, the oven temperature was tuned to provide the best resolution and peak shape. The pH and temperature scouting investigations indicate that the acquired data for the two examined drugs has a high resolution and a low tailing factor. They were obtained at pH values ranging from 3.0 to 7.0 and temperatures ranging from 25 to 35 °C. These pH and temperature ranges (3.0–7.0) and (25–35 °C) were then subjected to screen procedures to get additional insight into their effects. The other parameters in the proposed fishbone diagram have been left at their default values due to their little effect on method performance.
4.3. Experimental design (DoE)
4.3.1. Screening studies via Placket–Burman design. Following a risk assessment of the quality of the product and preliminary scouting trials, experimental designs were developed to first screen the effects of the five selected parameters on CCAs, namely organic solvent percent, pH, temperature, flow rate, and the detection wavelength.Screening studies were performed using the Placket–Burman experimental design matrix with the low (−1) and high (+1) levels of each parameter, and using the Rs, TF, and run time as responses. The design analysis revealed that the percentage of organic solvent, pH, and temperature were the significant factors affecting the measurable responses (CAAs). These variables were identified as critical method parameters (CMPs) and then subjected to method optimization analysis. The remaining two parameters, on the other hand, had such a negligible effect on the CCAs that they were kept constant in subsequent experiments; the flow rate was set to 1 mL min−1 with a detection wavelength of 225 nm.
4.3.2. Method optimization and desirability function. The three CMPs were submitted to multivariate custom experimental analysis design in order to get the most optimum conditions, and then their design space was established for robust analysis. Based on preliminary investigations, levels of each parameter were assigned to attain the desired values of the selected CAAs. Although the high ethanol percentage (>70%) and mobile phase pH (>7.0) make the peaks sharper, they had a negative impact on resolution. Furthermore, reasonable resolution was obtained at low ethanol concentrations (60%) and pH levels (3.0–5.0). On the other hand, oven temperature had an effect on both resolution and run time, with the temperature at 25 °C providing high resolution with the limitation of increasing run time, whilst the temperature at 35 °C shortened the run time but generated poor resolution. As a result, the experiments were conducted at three distinct levels of organic solvent concentration (60, 65, and 70%), at different pH (3.0, 5.0, and 7.0), and at temperatures of (25, 30, and 35 °C).A custom design of sixteen experiments was created using the JMP® software version 16.0.0.43 To measure the curvature, the design included four experiments at the center point (zero values for the three factors). The trials were carried out, and the assigned responses were recorded in order to feed the model (Table 1). For each crucial response, prediction models were built. Each main effect, interaction, and quadratic term is assigned a coefficient by the models, Fig. 3. The latter specified the non-linear (polynomial) interactions, whereas the coefficients determined the direction and strength of each influence on the corresponding response. ANOVA statistical analyses were carried out, and P-values were computed to demonstrate the importance of each term at a significance level of 0.05. Table 2 highlights significant terms, lack of fit, non-significance besides the acceptable values of R2, R2-adjusted and predicted root mean square error (RMSE) as revealed in Table 3, as the created model is used to forecast the responses. These were plotted against the experimentally established responses. The estimated R2 for the five responses reached unity, suggesting that the expected and experimental responses were perfectly fit. Furthermore, the modest difference between R2 and R2-adjusted supported the model's good fit. The root mean square error of predictions (RMSEP) was minimal, indicating that the prediction model was accurate. On the other hand, the calculated residuals presented in Fig. 2S, ESI,† looked to be approximately normally distributed (with a mean of zero) and independent of one another over time. As a result, residual analysis confirmed that the models adequately characterized the data.
Table 1 Levels, factors, and responses of the proposed experimental design
Factors |
Responses |
Experiment no. |
Temperature |
% of organic solvent |
pH |
RSDFX & DFP |
RSDFP & IS |
TFDFX |
TFDFP |
Run time |
1 |
25 |
60 |
5 |
5.05 |
2.99 |
1.23 |
1.32 |
10.00 |
2 |
25 |
70 |
3 |
2.21 |
2.25 |
1.11 |
1.02 |
6.00 |
3 |
35 |
70 |
3 |
1.01 |
1.99 |
1.24 |
1.20 |
6.41 |
4 |
25 |
70 |
7 |
2.14 |
3.05 |
1.44 |
1.33 |
6.10 |
5 |
25 |
60 |
7 |
3.55 |
3.03 |
1.32 |
1.29 |
10.20 |
6 |
25 |
65 |
3 |
3.25 |
2.88 |
1.23 |
1.16 |
7.22 |
7 |
30 |
65 |
7 |
1.55 |
2.53 |
1.42 |
1.40 |
7.51 |
8 |
30 |
70 |
5 |
1.65 |
1.95 |
1.28 |
1.32 |
6.00 |
9 |
35 |
70 |
7 |
0.89 |
1.03 |
1.34 |
1.29 |
5.45 |
10 |
25 |
70 |
7 |
2.08 |
2.98 |
1.40 |
1.35 |
6.11 |
11 |
30 |
65 |
5 |
1.70 |
2.63 |
1.33 |
1.30 |
7.06 |
12 |
35 |
65 |
5 |
2.14 |
2.49 |
1.34 |
1.48 |
6.38 |
13 |
25 |
60 |
3 |
5.14 |
3.10 |
1.15 |
1.25 |
10.00 |
14 |
30 |
60 |
3 |
4.54 |
2.88 |
1.31 |
1.51 |
9.44 |
15 |
35 |
60 |
3 |
2.87 |
2.99 |
1.22 |
1.08 |
8.89 |
16 |
35 |
60 |
7 |
2.10 |
3.71 |
1.45 |
1.32 |
8.68 |
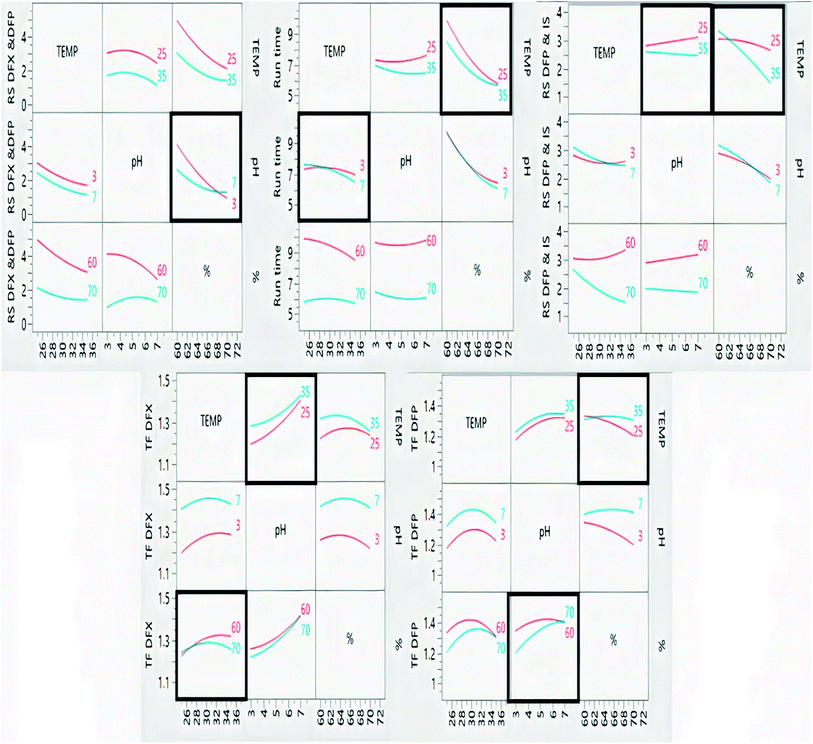 |
| Fig. 3 Interaction plots of the studied factors and their effects on the measured response. | |
Table 2 The calculated coefficients of the prediction models and P-values obtained from the ANOVA test results a
Term |
RSDFX & DFP |
RSDFP & IS |
TFDFX |
TFDFP |
Run time |
Coef. |
p-value |
Coef. |
p-value |
Coef. |
p-value |
Coef. |
p-value |
Coef. |
p-value |
Highlighted values (bold) indicate significant effect on the corresponding response. Coef. = coefficient (slope), I = intercept, X = temperature, Y = pH, Z = % of organic solvent, Rs = resolution and TF = tailing factor. |
I |
2.2582 |
0.0004* |
2.5758 |
<0.0001* |
1.3392 |
<0.0001* |
1.4079 |
<0.0001* |
7.0995 |
<0.0001* |
X |
−0.6521 |
0.0035* |
−0.2156 |
0.0002* |
0.0274 |
0.0793 |
0.0189 |
0.6373 |
−0.3714 |
0.0007* |
Y |
−0.2845 |
0.0748 |
0.0383 |
0.1502 |
0.0873 |
0.0010* |
0.0655 |
0.1456 |
−0.0510 |
0.3653 |
Z |
−1.1108 |
0.0003* |
−0.5558 |
<0.0001* |
−0.0110 |
0.4218 |
−0.0327 |
0.4289 |
−1.7230 |
<0.0001* |
XY |
0.00345 |
0.9815 |
−0.1088 |
0.0075* |
−0.0156 |
0.3195 |
−0.0073 |
0.8706 |
−0.1928 |
0.0197* |
XZ |
0.30036 |
0.0868 |
−0.3592 |
<0.0001* |
−0.0189 |
0.2373 |
0.0306 |
0.5025 |
0.3170 |
0.0026* |
YZ |
0.4524 |
0.0233* |
−0.1058 |
0.0082* |
0.0084 |
0.5737 |
0.0388 |
0.3988 |
−0.1304 |
0.0696 |
XYZ |
−0.2328 |
0.1703 |
−0.3147 |
<0.0001* |
−0.0344 |
0.0628 |
−0.0555 |
0.2590 |
−0.0788 |
0.2371 |
X2 |
0.21398 |
0.4686 |
0.1648 |
0.0194* |
−0.0385 |
0.2150 |
−0.0966 |
0.2912 |
−0.2631 |
0.0626 |
Y2 |
−0.4120 |
0.1964 |
0.00303 |
0.9533 |
0.0279 |
0.3576 |
−0.0457 |
0.6054 |
0.2456 |
0.0792 |
Z2 |
0.38952 |
0.2179 |
−0.1068 |
0.0823 |
−0.0405 |
0.2009 |
−0.0246 |
0.7786 |
0.6257 |
0.0025* |
Table 3 Fitting results of the predicted against the found responses of the developed modela
Term |
RSDFX & DFP |
RSDFP & IS |
TFDFX |
TFDFP |
Run time |
P-value define the model significance for prediction of the corresponding response. |
R2 |
0.9250 |
0.9817 |
0.7399 |
0.6176 |
0.9795 |
R2-adjusted |
0.8875 |
0.9658 |
0.6999 |
0.5903 |
0.9721 |
Predicted root mean square error |
0.4249 |
0.0756 |
0.0423 |
0.1274 |
0.1716 |
P-valuea |
0.0038 |
<0.0001 |
0.0171 |
0.5213 |
<0.0001 |
The impacts of CMPs on each CAA were studied using graphical data interpretation using response surface methodology (RSM) like contour and surface plots,54 Fig. 4, and 3S, ESI.† When multiple responses are present, the desirability function technique provides a simple, quick, and accurate tool for optimizing them. The desirability function is the recommended way for multiresponse optimization because it contains more subjective parameters than any other optimization method.55 The response optimizer tool was used to establish the best separation parameters, Fig. 5. Additionally, the “maximize desirability” option was chosen to maximize desirability. The optimal chromatographic condition was ethanol and acidic water at pH 3.0, adjusted by phosphoric acid in the ratio of (70
:
30, v/v) at 25 °C as acceptable resolution, symmetric peaks, and suitable run time were obtained, as shown in Fig. 6.
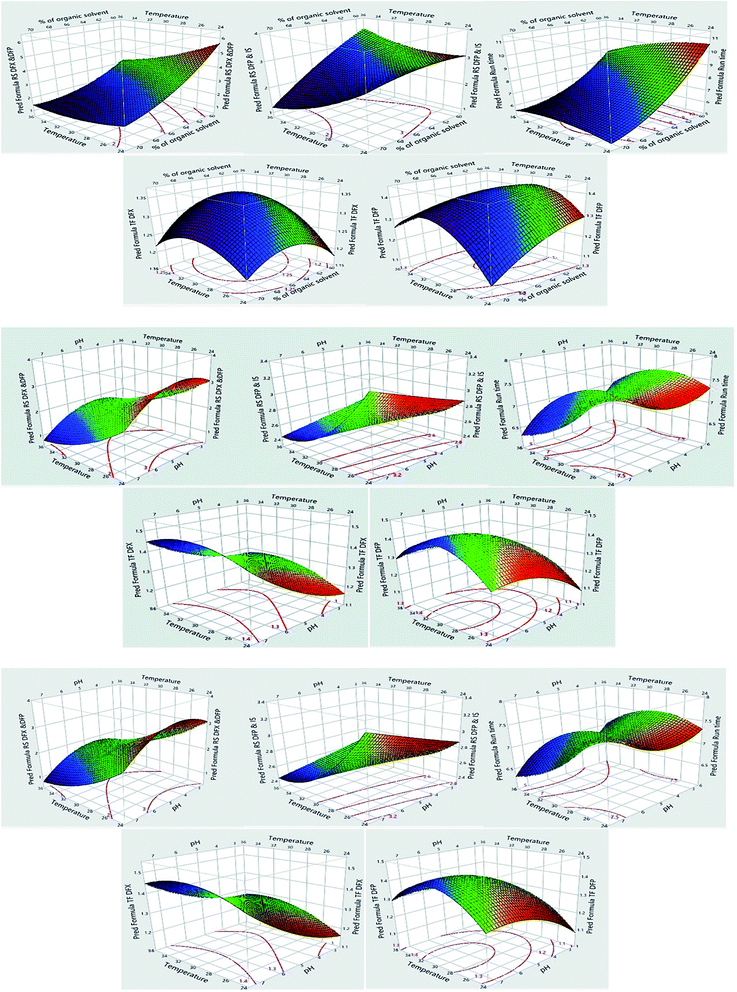 |
| Fig. 4 Surface plots for the measured responses. | |
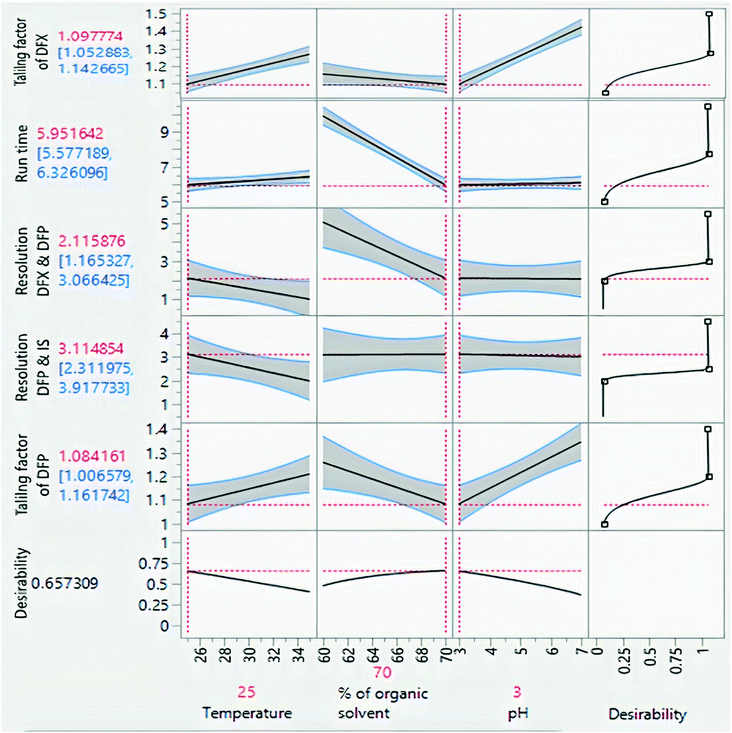 |
| Fig. 5 Prediction profiler of the experimental design. | |
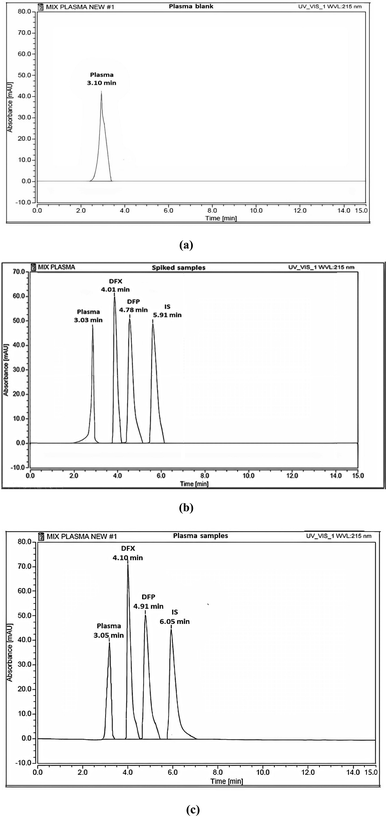 |
| Fig. 6 HPLC chromatograms of (a) blank plasma, (b) blank plasma spiked with standard solutions of DFX and DFP (10 μg mL−1) and (c) rat plasma samples from I. V administration. | |
4.4. Establishing design space
The design space is a multidimensional configuration and interaction of input variables and process parameters that has been shown to provide quality assurance.49 It is used to demonstrate the link between process parameters and CAAs. It was designed for each step of the approach and then integrated to provide design space for the entire method. It is critical to establish design space to ensure that changes in method parameters do not impair the quality of the analysis and that the resulting responses remain within the method's pre-established desired limits, as well as to find a simpler empirical model that adequately predicts the method's behavior over limited ranges of the factors. The design space for the proposed experimental design is depicted in Fig. 4S, ESI,† by yellow zones, in which the ethanol concentration, pH, and temperature can be varied flexibly between 65.8-68.8%, 3.0–6.0, and 25.0–29.6 °C, respectively, to obtain the desired Rs, TF, and run time values.
4.5. Control strategy and methods evaluation
The predicted experimental conditions were validated experimentally and evaluated using the calculated system suitability parameters, which were found to fall within the desired limits, Table 1 S, ESI.†
4.6. Method validation
The proposed method was validated to determine its suitability for routine laboratory work. Numerous factors such as specificity, linearity, selectivity, accuracy, precision, and robustness have been evaluated against the acceptance criteria specified in the FDA-approved “Guidance for Industry-Bioanalytical Method Validation”.47
4.6.1. Linearity of the calibration curves. The linearity of each drug was determined by analyzing ten concentrations in triplicate. The calibration curves were linear over the 0.3–20 and 0.2–20 μg mL−1 concentration sets of DFX and DFP, respectively, with a range of 19.7 μg mL−1 for DFX, and 19.8 μg mL−1 for DFP, and with correlation coefficients (r) of 0.9999 and 0.9998 for DFX and DFP, respectively. Table 4 contains the calculated regression parameters, including the slopes and intercepts.
Table 4 Regression and analytical parameters of the proposed method for the determination of deferasirox and deferiprone in spiked rat plasma
Parameters |
DFX |
DFP |
Calibration range (μg mL−1) |
0.3–20 |
0.2–20 |
Slope |
0.1411 |
0.1271 |
Intercept |
0.0154 |
0.1686 |
Correlation coefficient (r) |
0.9999 |
0.9998 |
LLOQ (μg mL−1) |
0.3 |
0.2 |
4.6.2. Accuracy and precision. Accuracy was determined and expressed as recovery percent for each of the three QCs of DFX and DFP. The obtained concentrations were within a range of 100 ± 15% of their nominal concentrations. Excellent recoveries have been obtained, as shown in Table 2 S, ESI.† Additionally, the intraday and interday precisions were confirmed and expressed as a percentage of RSD. The intra-day precision was determined by analysis of six replicates of QCs for each of DFX and DFP within one day. On the other hand, the inter-day precision was evaluated by repeating the analysis of three concentration levels of QCs on three consecutive days by different analysts. They ranged between 0.05 and 1.41% for DFX and 0.06 and 1.43% for DFP, respectively, which are within the FDA standard limits.46 Table 3 S, ESI,† illustrates the intra-day and inter-day precision.
4.6.3. Selectivity and sensitivity. The developed method's selectivity was determined by comparing the chromatograms of different sources of blank plasma to those of plasma samples spiked with DFX, DFP, and IS, as well as rat plasma samples. No significant interference from endogenous plasma constituents was observed in the chromatograms shown in Fig. 6.On the other hand, the sensitivity of the method was evaluated by determining the LLOQ of DFX and DFP. The LLOQ for DFX was 0.3 μg mL−1, whereas the LLOQ for DFP was 0.2 μg mL−1, demonstrating the proposed method's high sensitivity.
4.6.4. Stability. Stability is a critical parameter to consider when evaluating drugs in biological matrices. The stability of DFX and DFP in the endogenous plasma matrix was determined by revealing the QCs to a variety of conditions, including preservation for six hours at room temperature (25 °C) [Bench-Top stability], one day at 25 °C in an autosampler [post preparative stability], long term stability for six weeks, and finally, three freeze–thaw cycles from −80 °C to room temperature (12 hours) (freeze and thaw stability). The studied drugs were thought to be plasma stable. The stability testing results in Table 4 S, ESI,† confirmed that the two drugs are stable in plasma samples under the storage conditions experienced.
4.6.5. System suitability testing parameters. The tailing factor (TF), column efficiency (number of theoretical plates), resolution (Rs), selectivity (α), capacity factor (K0), and height equivalent to a theoretical plate (HETP) were all calculated to determine the system's suitability. All the measured parameters that were presented in Table 1 S, ESI,† were within acceptable ranges,46 indicating that the developed method's reproducibility, selectivity, and resolution were adequate.
4.6.6. Application to pharmacokinetic study. Pharmacokinetic principles are primarily used to manage or cure disease by administering the smallest amount of drug for the shortest time with the fewest side effects. Pharmacokinetics is critical because it allows for the calculation of dosage regimens and drug doses, as well as the determination of the most efficient route of administration, protein binding, drug–drug, food–drug interactions, drug design and development, and formulation design in order to maximize therapeutic benefit and minimize drug toxicity.56–59 These advantages demanded the investigation of pharmacokinetic characteristics such as half-life, Cmax, Tmax, elimination rate constant, clearance, apparent volume of distribution, and area under the curve (AUC).Different parameters were calculated after DFX and DFP were administered orally in single doses and in combination to four groups of rats. Single medication administration was investigated in order to serve as a benchmark against which the effects of combined drug administration could be evaluated. The combined administration of these drugs was investigated to determine whether or not there was any drug–drug interaction between the analyzed drugs, and to determine the possibility of co-administration of these drugs without any adverse effects. The reported studies confirmed the additive effect of this combination in decreasing the serum ferritin level in thalassemia patients. This combination regimen led to a decrease in the prescribed doses, which led to a decrease in the adverse effects with the same efficiency. This hypothesis was proved by monitoring various markers like hepatic iron concentration (HIC), serum ALT, and serum ferritin.49–51 The key pharmacokinetic parameters were computed using non-compartmental analysis (NCA) utilizing Thermo Kinetica software (version 5.0) and PK solver software, and are shown in Table 5. Fig. 7 depicts the mean plasma concentration–time profiles (n = 7) of the investigated drugs. The values of these parameters were statistically compared to determine whether the difference between the single and combination groups of each drug was significant or not. Table 6 displays the results.
Table 5 Pharmacokinetic parameters of deferasirox and deferiprone in rat plasma after I. V administration of 185 mg kg−1 of DFX and 465 mg kg−1 of DFP
Parameters |
DFX |
DFP |
Single administration gp |
Combined administration gp |
Single administration gp |
Combined administration gp |
t1/2 (h) |
7.92 ± 1.14 |
7.15 ± 0.60 |
9.29 ± 1.13 |
9.26 ± 1.14 |
Cmax (μg mL−1) |
38.05 ± 3.08 |
41.28 ± 3.21 |
35.25 ± 3.63 |
36.66 ± 4.11 |
Tmax (h) |
1.50 ± 0.51 |
1.50 ± 0.53 |
1.00 ± 0.21 |
1.00 ± 0.22 |
Elimination rate constant (K) |
0.088 ± 0.05 |
0.097 ± 0.04 |
0.075 ± 0.02 |
0.075 ± 0.03 |
Volume of distribution (Vd) (L) |
9.83 ± 1.91 |
9.38 ± 1.46 |
28.27 ± 2.86 |
27.30 ± 2.70 |
Clearance (cl) (L h−1) |
0.89 ± 0.09 |
0.91 ± 0.23 |
2.11 ± 0.23 |
2.04 ± 0.38 |
AUC 0→t (μg mL−1 h−1) |
12.23 ± 1.89 |
12.96 ± 1.67 |
17.66 ± 2.54 |
16.44 ± 2.98 |
AUC 0→∞ (μg mL h−1) |
275.39 ± 7.70 |
278.26 ± 3.66 |
256.86 ± 6.34 |
304.52 ± 5.71 |
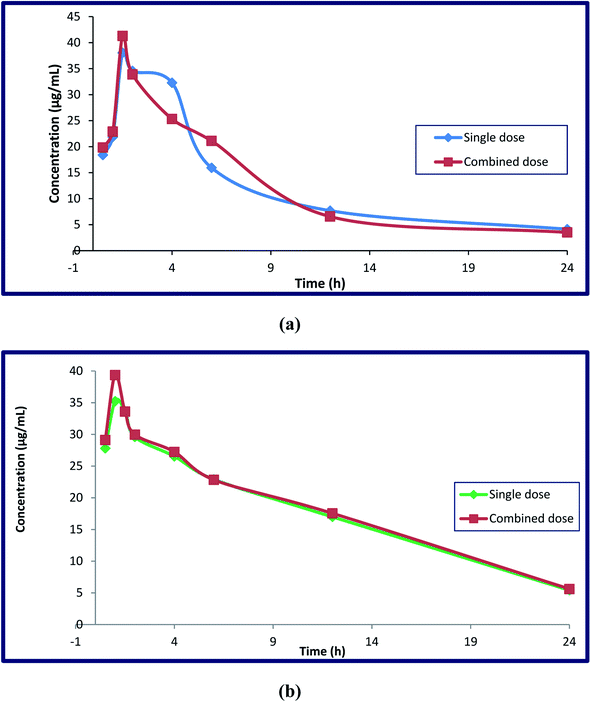 |
| Fig. 7 Mean plasma concentration–time curves (mean ± SD, n = 7) of deferasirox alone after IV administration of 185 mg kg−1 and combined administration of DFP (92.5 mg kg−1) (a), and deferiprone alone after IV administration of 465 mg kg−1 and combined administration of DFX (232.5 mg kg−1) (b) in RP-HPLC method. | |
Table 6 Statistical comparison of the results obtained by pharmacokinetic study of deferasirox and deferiprone between the single and the combined groups in HPLC methoda
Drug |
Parameters |
t1/2 |
Cmax |
Tmax |
K |
Vd |
cl |
AUC0 t |
Single group |
Combined group |
Single group |
Combined group |
Single group |
Combined group |
Single group |
Combined group |
Single group |
Combined group |
Single group |
Combined group |
Single group |
Combined group |
*, **: Degree of significant difference among means. |
DFX |
Mean |
7.92 |
7.15 |
38.05 |
41.28 |
1.50 |
1.50 |
0.088 |
0.097 |
9.83 |
9.38 |
0.89 |
0.91 |
275.39 |
278.26 |
SD |
1.14 |
0.60 |
3.08 |
3.21 |
0.51 |
0.53 |
0.05 |
0.04 |
1.91 |
1.46 |
0.09 |
0.23 |
7.70 |
3.66 |
n |
7 |
P value |
0.07 |
0.46 |
0.46 |
0.38 |
0.27 |
0.06 |
0.03 |
Student's t-test (2.447) |
2.19 |
2.03 |
0.004 |
0.89 |
1.21 |
0.99 |
1.13 |
F-test (4.284) |
3.64 |
1.09 |
1.08 |
1.30 |
1.71 |
3.59 |
4.44 |
Significant diff. among means (P < 0.05) |
No |
No |
No |
No |
No |
No |
Yes (*) |
Drug |
Parameters |
t1/2 |
Cmax |
Tmax |
K |
Vd |
cl |
AUC0 t |
Single group |
Combined group |
Single group |
Combined group |
Single group |
Combined group |
Single group |
Combined group |
Single group |
Combined group |
Single group |
Combined group |
Single group |
Combined group |
DFP |
Mean |
9.29 |
9.26 |
35.25 |
36.66 |
1.00 |
1.00 |
0.075 |
0.075 |
28.27 |
27.30 |
2.11 |
2.04 |
256.86 |
304.52 |
SD |
1.13 |
1.14 |
3.63 |
4.11 |
0.21 |
0.22 |
0.02 |
0.03 |
2.86 |
2.70 |
0.23 |
0.38 |
6.34 |
5.71 |
n |
7 |
P value |
0.50 |
0.014 |
0.45 |
0.34 |
0.44 |
0.13 |
0.04 |
Student's t-test (2.447) |
0.37 |
2.91 |
0.07 |
0.20 |
1.30 |
0.79 |
12.66 |
F-test (4.284) |
1.01 |
1.29 |
1.12 |
1.42 |
1.13 |
2.71 |
1.23 |
Significant diff. among means (P < 0.05) |
No |
Yes (**) |
No |
No |
No |
No |
Yes (*) |
In terms of DFX, it appears that the single DFX group had a significant difference in their area under the curve compared to the drug in the combination group. The rise in DFX AUC was significant, indicating higher drug bioavailability. Despite its volume of distribution (Vd) in the body, there was no significant difference in pharmacokinetic parameters when compared to the DFX in combination with DFP, and the half-life (t1/2) showed a slight decrease, while the drug clearance (cl), elimination rate constant (k), and maximum serum concentration (Cmax) showed slight increases, with the same (Tmax) (1.5 h) for both groups when taken with DFP.
When DFP is combined with DFX, it appears that the area under the curve (AUC) and maximum serum concentration (Cmax) are greatly increased. This increase was due to the additive effect of this combination, which makes us use the half-daily doses of the proposed drugs, resulting in fewer adverse effects. Other parameters such as clearance, apparent volume of distribution, half-life, Tmax, and elimination rate constant do not differ significantly between single and combination groups. Furthermore, the clearance, elimination rate constant, and half-lives of these components in the combined dose groups may be the same as in the single dose groups, indicating that there was no difference in their absorption, metabolism, and elimination when provided simultaneously. As a result, additional pharmacokinetic and pharmacodynamics investigations on human volunteers are required as the findings obtained from this work strongly encourage the co-administration of the two iron chelating drugs due to the remarkable increase in their bioavailability.
4.7. Statistical analysis
Student's t-tests and F-tests were used to compare the proposed method's results to the reported methods for analysis each of the studied drugs. None of the calculated results exceeded the tabulated values, indicating insignificant differences in the methods' accuracy and precision, Table 5 S, ESI.†
4.8. Comparison to the reported HPLC methods
Due to its capacity to determine DFX and DFP simultaneously, the newly developed approach demonstrated high selectivity and sensitivity due to the fact that it has a lower limit of quantification (LLOQ) (0.3 and 0.2 μg mL−1, for DFX and DFP, respectively) and a broader linearity range (0.3–20 and 0.2–20 μg mL−1, for DFX and DFP, respectively) than the previously reported methods with have LLOQ (0.4 and 0.25 μg mL−1, for DFX and DFP, respectively) and linearity range of (0.4–80 and 0.25–10 μg mL−1, for DFX and DFP, respectively). Comparison between the developed HPLC and the reported ones was illustrated in Table 7. The findings show that the developed method has less time consumption and produces less solvent waste than the reported methods. Additionally, the proposed method is more environmentally friendly than the reported methods. Using an experimental design for technique optimization resulted in a significant savings in both time and money.
Table 7 Comparison between the developed HPLC method and the reported ones
Methods |
Developed HPLC method |
Reported method for DFX |
Reported method for DFP |
Solvent waste: (run time × flow rate). |
Linearity range (μg mL−1) |
0.3–20 for DFX |
0.4–80 |
0.25–10 |
0.2–20 for DFP |
Mobile phase |
Ethanol : acidic water at pH 3.0 adjusted by phosphoric acid in the ratio of (70 : 30, by volume) |
50 mM ammonium acetate and 20 mM tetrabutylammonium hydrogen sulfate buffer (pH 6.3) : acetonitrile : methanol (33 : 22 : 45, by volume) |
Methanol : potassium phosphate buffer (50 mM) containing heptane sulfonic acid (5 mM) adjusted the pH = 3.5 ± 0.05 with phosphoric acid (18 : 82, by volume) |
Stationary phase |
XBridge® HPLC RP-C18 (4.6 × 250 mm, 5 μm) |
PEEK-lined ProteCol-P C18 HQ105-coated column (4.6 × 250 mm, 5 μm) |
Discovery supelco C18 column (4.6 × 250 mm, 5 μm) |
Run time (min) |
6 |
8 |
12 |
Flow rate (mL min−1) |
1 |
1 |
1.2 |
Solvent wastea (g per run) |
6 |
8 |
14.4 |
Wavelength (nm) |
225 |
225 |
280 |
4.9. Greenness assessment
Today's analysts have a strong desire to develop novel, eco-friendly, and green analytical procedures that require them to substitute safer solvents for hazardous and toxic ones, minimize pretreatment steps, sample preparations, energy and solvent consumption. Additionally, conduct on-line or in situ analysis rather than off-line measurements and avoid derivatization of methods. These prerequisites were incorporated into the GAC's 12 principles.60 However, the majority of analytical chemists' suggestions stated that the 12 principles may be used to reduce performance characteristics by reducing sample quantity, reagents, energy, waste, and instrument miniaturization.61 For many years, this claim raised significant concerns about the use of GAC in laboratories and industry. The compromise between increasing the quality of the analysis and improving the environmental aspects of analytical methods has become the most critical obstacle for the future of GAC implementation.62 However, while developing new analytical approaches, they must be evaluated for their green profile to ensure that they are environmentally acceptable.
The greenness of the developed HPLC method was evaluated using eight different greenness tools. The first one was NEMI63 which is one of the oldest evaluation tools for green analytical chemistry. The summarized profile criteria contain four vital terms: persistent bioaccumulative and toxic (PBT), hazardous, corrosive, and waste, depicted in a circular pictogram divided into four quadrants. It offers a simple pictogram with easy visualization and immediate information; by just one fast look at the pictogram will be enough to have information about the environmental impact of a procedure. However, the NEMI pictogram is qualitative, not a quantitative method, the search of each chemical used in the procedure in official lists is time wasting, with an absence of energy, chemicals and reagents consumption evaluation, and an absence of the amount of waste generated.
These limitations were cleared by using the second tool, which named the modified NEMI.64 This tool depends on five criteria: health hazard, safety hazard, environmental hazard, energy, and waste amount. It has the advantages of being a semi-quantitative and descriptive tool that considers energy, reagent consumption, and waste amount while keeping its simplicity in method selection and parameter comparison.
The third tool, analytical method volume intensity (AMVI),65 is a semi-quantitative tool that evaluates solvent consumption by the instrument or during sample preparation by assigning a score; the lower the AMVI value, the more environmentally friendly the procedure. The most major shortcoming of this greenness tool is that the toxicity of the solvents employed in the method is not considered. The fourth used greenness tool is analytical eco-scale,66 the most common used tool for greenness profile.67 It has a maximum eco-score of 100 and is determined by the penalty points (PP) associated with the substances or processes used in an analytical procedure. The volume of reagents, a hazard that comprises, energy, an occupational hazard, and waste are all included while evaluating the eco-scale. It has many benefits of being simple, calculates the amounts of chemicals and wastes semi-quantitatively, offers quantitative information on the environmental impact of analytical procedures, incorporates many aspects of environmental impact into its evaluation, and facilitates comparisons between analytical methods. On the other hand, the lack of additional quantifiers capable of discriminating between method applications at the nano-, micro-, and macro-scale, results that are not informative because of negative environmental impact were the most obvious limitations of the discussed tool.
The analytical method greenness score (AMGS)68 was also used in the greenness assessment because it is a composite of several metrics, including the safety, health, and environmental assessment (SHE) used to calculate solvent safety via geometric mean and the analytical mass volume intensity (AMVI) used to calculate solvent volume waste. It is free software that is available on the ACS green pharmaceutical website. It assigns a score to the proposed analytical method, with a lower number indicating that the method is more green.69 Additionally, high performance liquid chromatography-environmental assessment tools (HPLC-EAT) is one of the utilized green evaluating tools that has three criteria: operator risks, toxicity, and environmental risks. The lower the EAT value, the greener the procedure used. It is simple and easy to use, and its software is freely available.70 But it concerns liquid chromatography only, like AMVI, and it does not provide enough information about the character of risk.
Green analytical procedure index (GAPI) is considered one of the most well-known greenness matrices.71 It is good semi-quantitative tool for laboratory practice and educational purposes. It evaluates and quantifies the environmental impact involved in each step of an analytical method. It have many advantages of being simple method has a pictogram with three levels (red, yellow, and green), assess the green character of an entire analytical methodology, from sample collection to final determination, it also provides qualitative information and the ease of comparison of different analytical procedures.72 The last used greenness tool in this work was analytical greenness metric (AGREE).73 It is a greenness calculator using software that its calculations are based on 12 parameters that correspond to the 12 principles of green analytical chemistry. Each principle or parameter has a score range of 0–1 that is determined based on the hazard to the greenness of that principle.
A comparison between the proposed and reported methods19,31 was conducted regarding greenness profile and is presented in Table 8. From the comparison findings, the eight adopted metrics synergistically emphasized the green superiority of the proposed method, which showed an outstanding environmentally friendly method with more green than the reported methods. The proposed method was the only one having a complete green NEMI pictogram, the lowest AMVI, AMGS, and HPLC-EAT scores (51.0, 163.89, and 50.30, respectively), the highest AGREE score (0.75), excellent eco-scale score (90.0), Table 6 S, ESI,† and with one red zone only and six green zones in the GAPI pictogram.
Table 8 Comparison of the greenness profile assessment of the proposed HPLC method and the reported ones
Green assessment tool |
Developed HPLC method |
Reported method for DFX |
Reported method for DFP |
National environmental methods index (NEMI) |
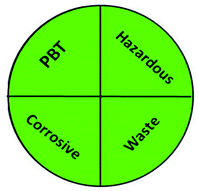 |
 |
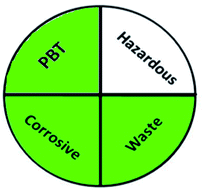 |
Modified NEMI |
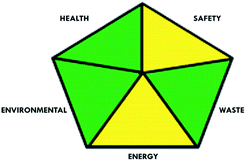 |
 |
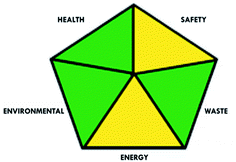 |
Analytical method volume intensity (AMVI) |
51.0 |
145.0 |
89.2 |
Eco-scale |
90.0 |
90.0 |
86.0 |
Analytical method greenness score (AMGS) |
163.89 |
641.37 |
257.44 |
Environmental assessment tool (HPLC-EAT) |
50.30 |
88.69 |
72.53 |
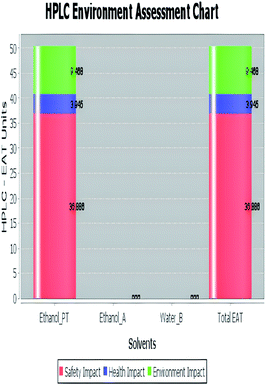 |
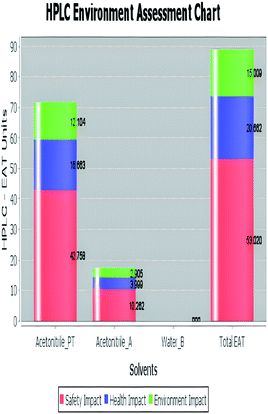 |
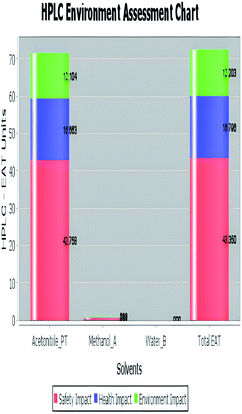 |
Green analytical procedure index (GAPI) |
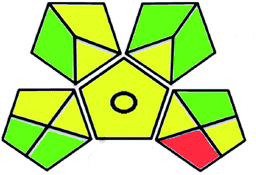 |
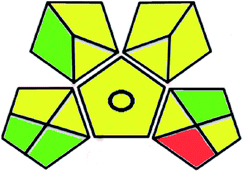 |
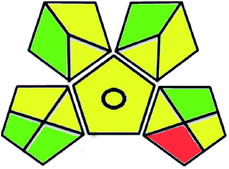 |
Analytical GREEnness (AGREE) |
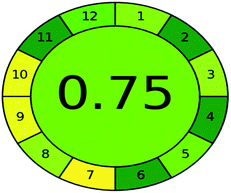 |
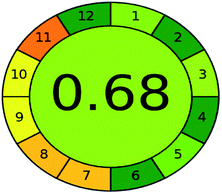 |
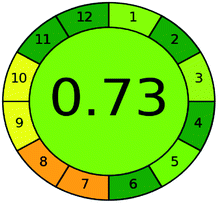 |
5. Conclusion
Numerous individuals with red cell abnormalities and myelodysplasia acquire chronic iron overload as a result of transfusion. Iron overload is connected with significant morbidity and mortality. As a result, it was critical to develop an accurate, eco-friendly, and highly robust HPLC method based on an innovative integration of the AQbD methodology and GAC postulates for the first time. It was successful in analyzing the two drugs under investigation, DFX and DFP, in rat plasma. Statistical screening and optimization studies were conducted using a variety of experimental designs, including Placket–Burman and custom-designed experimental designs. The results of the regression and ANOVA tests allowed for the assessment of the factors' main, quadratic, and interaction impacts on each response. With a small number of trials, an optimal separation with an appropriate peak shape and run time was obtained. Several green evaluation tools argued that the presented method’ greenness profile was superior to those of previously described approaches. The developed method showed an excellent green analytical procedure regarding GAC principles. Additionally, the analysis of two oral chelating agents in rat plasma using pharmacokinetic characteristics enables the use of this technology for drug monitoring and therapeutic applications. Finally, the proposed approach was evaluated in accordance with FDA standards and demonstrated promising results that were within acceptable limits.
Declarations
Availability of data and material
The data are available from the corresponding author upon sensible request.
Ethics approval and consent to participate
Approval of the Ethics Committee of Nahda University number 17-5-11-2021 was obtained. The study conducted in compliance with the International Guidelines for Research Ethics.
Author contributions
Conceptualization: Maha A. Hegazy, Nada S. Abdelwahab; methodology: Michel Y. Fares, Nada S. Abdelwahab; formal analysis and investigation: Ghada M. El-Sayed, Maha M. Abdelrahman; writing – original draft preparation: Michel Y. Fares; writing – review and editing: Maha A. Hegazy, Ghada M. El-Sayed, Nada S. Abdelwahab, Maha M. Abdelrahman; funding acquisition: not applicable; resources: Michel Y. Fares, Ghada M. El-Sayed, Nada S. Abdelwahab; supervision: Maha A. Hegazy. All authors have revised and approved the manuscript.
Funding
This research did not receive any specific grant from funding agencies in the public, commercial, or not-for-profit sectors.
Conflicts of interest
The authors declare that there is no conflict of interest.
References
- J. Porter, V. Viprakasit, and A. Kattamis, Guidelines for the management of transfusion dependent thalassaemia (TDT), Thalassaemia International Federation, Nicosia, 3rd edn, 2014 Search PubMed.
- Centers for Disease Control and Prevention, Thalassemia: complications and treatment, Centers for Disease Control and Prevention (CDC), 2021. [Online]. Available: https://www.cdc.gov/ncbddd/thalassemia/treatment.html Search PubMed.
- A. Malik, S. D. Firke, R. R. Patil, A. A. Shirkhedkar and S. J. Surana, Determination of iron chelating agents by analytical methods: a review, Crit. Rev. Anal. Chem., 2020, 50(3), 254–264 CrossRef CAS PubMed.
- N. Mobarra, et al., A review on iron chelators in treatment of iron overload syndromes, Int. J. Hematol. Oncol. Stem Cell Res., 2016, 10, 239–247 Search PubMed.
- British pharmacopoeia, Medicines and healthcare products regulatory agency, London, 2018.vol. 1 Search PubMed.
- W. T. Lindsey and B. R. Olin, Deferasirox for transfusion-related iron overload: a clinical review, Clin. Therapeut., 2007, 29(10), 2154–2166 CrossRef CAS PubMed.
- R. R. DivakarJose, C. G. Delhikumar and G. Ram Kumar, Efficacy and safety of combined oral chelation with deferiprone and deferasirox on iron overload in transfusion dependent children with thalassemia – A Prospective Observational Study, Indian J. Pediatr., 2021, 88(4), 330–335 CrossRef PubMed.
- S. S. Jamuar and A. H. M. Lai, Safety and efficacy of iron chelation therapy with deferiprone in patients with transfusion-dependent thalassemia, Ther. Adv. Hematol., 2012, 3(5), 299–307 CrossRef CAS PubMed.
- M. Stevens, et al., The deferiprone and deferasirox combination is efficacious in iron overloaded patients with b-thalassemia major: a prospective, single center, open-label study, Pediatr. Blood Cancer, 2008, 50(5), 1018–1025 CrossRef PubMed.
- S. Chaitanya and K. L. Prasanna, Development and validation of new spetrophotometric methods for the estimation of deferasirox in bulk drug and its formulations using 1,10-Phenanthroline and Mbth reagents, Unique Research Journal of Chemistry, 2013, 1(01), 47–51 Search PubMed.
- V. S. Rao Somisetty, P. K. Bichala, C. M. M. Prasada Rao and V. Kirankumar, Development and validation of newer analytical methods for the estimation of deferasirox in bulk and in tablet dosage form by calorimetric method, Int. J. Pharm. Pharm. Sci., 2013, 5(3), 521–525 Search PubMed.
- G. M. Marathe, V. V. Pande, P. H. Patil, R. E. Mutha and S. B. Bari, Quantitative determination of deferasirox in bulk and pharmaceutical formulation by UV spectrophotometric method, Indian Drugs, 2013, 50(2), 27–32 CrossRef.
- E. Chauzit, S. Bouchet, M. Micheau, F. X. Mahon, N. Moore, K. Titier and M. Molimard, A method to measure deferasirox in plasma using HPLC coupled with MS/MS detection and its potential application, Ther. Drug Monit., 2010, 32(4), 476–481 CrossRef CAS PubMed.
- S. Thomas, et al., Identification, characterization and quantification of a new impurity in deferasirox active pharmaceutical ingredient by LC-ESI-QT/MS/MS, J. Pharm. Biomed. Anal., 2012, 63, 112–119 CrossRef CAS PubMed.
- N. C. Desai and R. D. Senta, Simultaneous RP-HPLC determination of salicylamide, salicylic acid and deferasirox in the bulk API dosages forms, J. Taibah Univ. Sci., 2015, 9(2), 245–251 CrossRef.
- N. Padmaja and N. Ramathilagam, Method development and validation of RP-HPLC method for the determination of deferasirox in tablets, Int. J. Pharm. Biol. Sci., 2012, 2(4), 338–343 CAS.
- H. Pligoropoulou, A. Vonaparti and I. Panderi, Hydrophilic interaction liquid chromatography/positive ion electrospray mass spectrometry for the quantification of deferasirox, an oral iron chelator, in human plasma, J. Chromatogr. B: Anal. Technol. Biomed. Life Sci., 2012, 893–894, 114–120 CrossRef CAS PubMed.
- T. Li, et al., A simple LC–MS/MS method for determination of deferasirox in human plasma: troubleshooting of interference from ferric ion in method development and its application, J. Pharm. Biomed. Anal., 2018, 151, 145–150 CrossRef CAS PubMed.
- M. Y. Lu, et al., Simultaneous determination of plasma deferasirox and deferasirox-iron complex using an HPLC-UV system and pharmacokinetics of deferasirox in patients with β-thalassemia major: once-daily versus twice-daily administration, Clin. Therapeut., 2015, 37(8), 1751–1760 CrossRef CAS PubMed.
- M. S. Kalshetti, R. Y. Patil, R. A. Karale and A. A. Kulkarni, Bioanalytical method development for estimation of deferasirox in
human plasma, Int. J. Pharm. Pharm. Sci., 2015, 7(9), 399–402 CAS.
- S. De Francia, et al., A new HPLC-UV validated method for therapeutic monitoring of deferasirox in thalassaemic patients, J. Chromatogr. B: Anal. Technol. Biomed. Life Sci., 2012, 893(894), 127–133 CrossRef PubMed.
- H. Malvia, A. Sharma, P. Sharma and R. Mishra, A micellar liquid chromatographic method for the determination of azosemide in solubilized system, J. Surfactants Deterg., 2017, 20(6), 1411–1418 CrossRef CAS.
- C. B. V. Narasimha Raju, G. Panda and G. Nageswara Rao, Stability indicating LC assay method for the determination of famciclovir in bulk drug and pharmaceutical dosage forms, Chromatographia, 2008, 68(9–10), 837–841 CrossRef CAS.
- S. Saravanan and R. Swetha, Method development and validation for determination of impurities in deferasirox by RP-HPLC technique, J. Drug Deliv. Therapeut., 2012, 2(3), 148–152 Search PubMed.
- M. Prashanthi and G. Venkateshwarlu, Kinetic spectrophotometric determination of drugs based on oxidation by alkaline KMnO4, World J. Pharm. Res., 2016, 5(6), 8–14 Search PubMed.
- H. Barot, D. Shah and D. DilipMaheshwari, Development of stability indicating UV- spectroscopy method for the estimation of deferiprone in pharmaceutical formulation, Am. J. PharmTech Res., 2015, 5(1), 621–632 CAS.
- M. K. Aamir Malik, S. Firke, R. Patil and A. Shirkhedkar, Development and validation of zero and first-order derivative area under curve spectrophotometric methods for the determination of deferiprone in bulk material and capsules, Asian J. Pharm. Anal. Med. Chem., 2019, 9(2), 49–54 Search PubMed.
- J. L. Manzoori, M. Amjadi and J. Soleymani, Determination of deferiprone in urine and serum using a terbium-sensitized luminescence method, Luminescence, 2012, 27, 268–273 CrossRef CAS PubMed.
- K. S. Sireesha, B. S. sai Kiran, K. B. C. Sekhar and S. Muneer, A new RP-HPLC method development and validation of deferiprone in bulk and its pharmaceutical dosage form, Int. J. Adv. Res., 2016, 4(8), 2174–2179 CrossRef.
- T. Song, Y. Hsieh, C. Peng and C. Liu, Development of a fast LC-MS/MS assay for the determination of deferiprone in human plasma and application to pharmacokinetics, Biomed. Chromatogr., 2012, 26, 1575–1581 CrossRef CAS PubMed.
- M. Abbas, R. Nawaz, T. Iqbal, M. Alim and M. R. Asi, Quantitative determination of deferiprone in human plasma by reverse phase high performance liquid chromatography and its application to pharmacokinetic study, Pak. J. Pharm. Sci., 2012, 25(2), 343–348 CAS.
- B. Siva, S. Kiran, S. Raja, A. Pradesh and A. Pradesh, Development and validation of RP-HPLC method for the estimation of deferiprone, J. Pharm. Sci. Innovation, 2018, 7(6), 231–234 Search PubMed.
- P. V. Reddy, V. A. Ranjani, R. C. Sekhar and M. S. Sundar, Stability indicating method development and validation of deferiprone in pharmaceutical dosage form by RP-HPLC, J. Innovations Pharm. Biol. Sci., 2017, 4(2), 53–57 CAS.
- M. Asmari, A. M. Abdel-megied, L. Michalcová, Z. Glatz and S. El, Analytical approaches for the determination of deferiprone and its iron ( III ) complex : investigation of binding affinity based on liquid chromatography- mass spectrometry ( LC-ESI/MS ) and capillary electrophoresis-frontal analysis ( CE/FA ), Microchem. J., 2020, 154, 1–8 CrossRef.
- H. Yadegari, H. Heli and A. A. Moosavi-movahedi, Electro-oxidation and determination of deferiprone on a glassy carbon electrode, Chem. Anal., 2008, 53(5), 5–16 CAS.
- A. Kam and E. Nm, Electrochemical determination of deferiprone using PVC membrane sensors, Austin J. Anal. Pharm. Chem., 2018, 5(1), 1–4 Search PubMed.
- M. Hajjizadeh, A. Jabbari, H. Heli and A. A. Moosavi-movahedi, Electrocatalytic oxidation and determination of deferasirox and deferiprone on a nickel oxyhydroxide-modified electrode, Anal. Biochem., 2008, 373, 337–348 CrossRef CAS PubMed.
- H. Heliab, H. Yadegaric and K. Karimiand, Nanoflakes of cobalt oxide for highly sensitive sensing of two orally iron chelating drugs deferasirox and deferiprone, J. Exp. Nanosci., 2011, 6(5), 488–508 CrossRef.
- C. Hubert, S. Houari, E. Rozet, P. Lebrun and P. Hubert, Towards a full integration of optimization and validation phases: an analytical Quality-by-Design approach, J. Chromatogr. A, 2015, 1395, 88–98 CrossRef CAS PubMed.
- S. Orlandini, S. Pinzauti and S. Furlanetto, Application of Quality-by-Design to the development of analytical separation methods, Anal. Bioanal. Chem., 2013, 405, 443–450 CrossRef CAS PubMed.
- A. Prafulla, K. Sahu and R. N. Rao, An overview of experimental designs in HPLC method development and validation, J. Pharm. Biomed. Anal., 2018, 147, 590–611 CrossRef PubMed.
- E. J. Klein and S. L. Rivera, A Review of criteria functions and response surface methodology for the optimization of analytical scale HPLC separations, J. Liq. Chromatogr. Relat. Technol., 2000, 23(14), 37–41 CrossRef.
- JMP, Design of Experiments (DOE) with JMP®, JMP, 2020. [Online]. Available: https://www.jmp.com/en_us/applications/design-ofexperiments.%0Ahtml#Custom-Designs.
- C. Turner, Sustainable analytical chemistry - more than just being green, Pure Appl. Chem., 2013, 85(12), 2217–2229 CrossRef CAS.
- L. H. Keith, et al., Green analytical methodologies, Chem. Rev., 2007, 107(6), 2695–2708 CrossRef CAS PubMed.
- K. Gaudin, C. Boussès, L. Ferey, E. Vedrines and K. Gaudin, Using an innovative combination of Quality-by-Design and green analytical chemistry approaches for the development of a stability indicating UHPLC method in pharmaceutical products, J. Pharm. Biomed. Anal., 2015, 114–122 Search PubMed.
- FDA, Guidance for Industry Bioanalytical Method Validation. 2013 Search PubMed.
- N. R. Council, Guide for the Care and Use of Laboratory Animals, 8th edn, 2011 Search PubMed.
- E. Poggiali, E. Cassinerio, L. Zanaboni and M. D. Cappellini, An update on iron chelation therapy, J. Blood Transfus., 2012, 10(4), 411–422 Search PubMed.
- M. S. Elalfy, A. M. Adly, Y. Wali, S. Tony, A. Samir and Y. I. Elhenawy, Efficacy and safety of a novel combination of two oral chelators deferasirox/deferiprone over deferoxamine/deferiprone in severely iron overloaded young beta thalassemia major patients, Eur. J. Haematol., 2015, 95(5), 411–420 CrossRef CAS PubMed.
- N. F. Olivieri, A. Sabouhanian and B. L. Gallie, Single-center retrospective study of the effectiveness and toxicity of the oral iron chelating drugs deferiprone and deferasirox, PLoS One, 2019, 14(2), 1–22 CrossRef PubMed.
- Y. Vander Heyden, A. Nijhuis and J. Smeyers-verbeke, Guidance for robustness/ruggedness tests in method validation, J. Pharm. Biomed. Anal., 2001, 24, 723–753 CrossRef CAS.
- R. Peraman, K. Bhadraya and Y. P. Reddy, Analytical quality by design : A tool for regulatory flexibility and robust analytics, Int. J. Anal. Chem., 2015, 9, 2015 Search PubMed.
- A. R. Bagheri, M. Arabi, A. Ostovan, X. Wang, J. Li, and L. Chen, Dummy molecularly imprinted polymers based on a green synthesis strategy for magnetic solid-phase extraction of acrylamide in food samples, Talanta, 2018 Search PubMed.
- C. S. Aksezer, On the sensitivity of desirability functions for multiresponse optimization, J. Ind. Manag. Optim., 2008, 4(4), 685–696 Search PubMed.
- L. Zhao, E. Y. Shang and C. G. Sahajwalla, Application of pharmacokinetics – pharmacodynamics/clinical response modeling and simulation for biologics drug, J. Pharm. Sci., 2012, 1–16 Search PubMed.
- M. Y. Fares, N. S. Abdelwahab, M. M. Abdelrahman and H. M. Abdel-Rahman, Determination of sofosbuvir with two co-administered drugs; Paracetamol and DL-methionine by two chromatographic methods. Application to a pharmacokinetic study, Bioanalysis, 2019, 11(5), 349–364 CrossRef CAS PubMed.
- A. A. Emam, N. M. Habib, H. M. Mahmoud, N. S. Abdelwhab and M. M. Abdelrahman, Ecofriendly chromatographic methods for determination of co-prescribed drugs, olanzapine and metformin, in rat plasma, Bioanalysis, 2020, 12(9), 597–613 CrossRef CAS PubMed.
- M. M. Abdelrahman, N. S. Abdelwahab, M. A. Hegazy, M. Y. Fares and G. M. EL-Sayed, Determination of the abused intravenously self-administered madness drops (Tropicamide) by liquid chromatography in rat plasma; an application to pharmacokinetic study and greenness profile assessment, Microchem. J., 2020, 159, 1–11 CrossRef.
- P. T. Anastas; and J. C. Warner, Green chemistry : theory and practice, Oxford University Press, New York, 1998 Search PubMed.
- P. T. A. Carlos, A. M. Afonso and J. P. G. Crespo, Green Separation Processes, in Book Reviews, vol. 13, 2006, p. 145 Search PubMed.
- M. A. Korany, H. Mahgoub, R. S. Haggag, M. A. A. Ragab and O. A. Elmallah, Green Chemistry : Analytical and Chromatography, J. Liq. Chromatogr. Relat. Technol., 2017, 60(September), 1520–1572 Search PubMed.
- NEMI, National Environmental Methods Index, 2016. [Online]. Available: https://www.nemi.gov/home/ Search PubMed.
- S. A. M. de la Guardia, A green evaluation of existing analytical methods, in, Green Anal. Chem., ed. M. D. La Guardia and S. B. T.-C. A. C. Armenta, no. 11, 2011, ch. 3, vol. 57, pp. 39–57 Search PubMed.
- R. Hartman, R. Helmy, M. Al-Sayah and C. J. Welch, Analytical method volume intensity ( AMVI ): A green chemistry metric for HPLC methodology in the pharmaceutical industry, Green Chem., 2011, 13, 934–939 RSC.
- A. Gałuszka, P. Konieczka and Z. M. Migaszewski, Analytical eco-scale for assessing the greenness of analytical procedures, Trends Anal. Chem., 2012, 37, 61–72 CrossRef.
- K. P. Kannaiah, A. Sugumaran, H. K. Chanduluru and S. Rathinam, Environmental impact of greenness assessment tools in liquid chromatography – A review, Microchem. J., 2021, 170(May), 1–15 Search PubMed.
- M. B. Hicks, et al., Introduction of the analytical method greenness score (AMGS) calculator, Green Chem., 2019, 1–25 CAS.
- A. (n.d.), AMGS, AMGS spreadsheet, ACS. (n.d.). https://www.acsgcipr.org/amgs/ (accessed April 9, 2021), AMGS spreadsheet, 2021.
- Y. Gaber, U. Tornvall, M. A. Kumar, M. Amin and R. Hatti-kaul, HPLC-EAT ( Environmental Assessment Tool): A tool for profiling safety, health and environmental impacts of liquid chromatography methods, Green Chem., 2011, 13, 2021–2025 RSC.
- J. Płotka-Wasylka, A new tool for the evaluation of the analytical procedure: Green Analytical Procedure Index, Talnta, 2017, 181, 204–209 CrossRef PubMed.
- N. S. Abdelwahab and M. M. Abdelrahman, Appraisal of the greenness profile of a chromatographic method for the simultaneous estimation of carbamazepine and oxcarbazepine, along with two potential impurities and three formulation excipients, RSC Adv., 2021, 11(14), 7790–7800 RSC.
- F. Pena-pereira, W. Wojnowski and M. Tobiszewski, AGREE—analytical greenness metric approach and software, Anal. Chem., 2020, 92, 10076–10082 CrossRef CAS PubMed.
|
This journal is © The Royal Society of Chemistry 2022 |
Click here to see how this site uses Cookies. View our privacy policy here.