DOI:
10.1039/D2RA01496C
(Paper)
RSC Adv., 2022,
12, 13003-13013
In silico screening for oligopeptides useful as capture and reporting probes for interleukin-6 biosensing†
Received
7th March 2022
, Accepted 24th April 2022
First published on 28th April 2022
Abstract
IL-6 is an important interleukin associated with inflammation and several diseases such as cancer. Evaluation of its levels in human blood sera is a critical step for an accurate diagnosis of the diseases. Our goal is to design peptides that can selectively bind in different poses with good affinities to IL-6. For this purpose, we started from the crystal structures of different IL-6/protein complexes available in the Protein Data Bank (PDB) to select short peptides in the interaction zones, in which we intentionally introduced point mutations to increase their stability and affinity. To examine their usefulness as capture and reporting probes for the IL-6 biosensing, the five peptides and their interaction with IL-6 were studied in saline aqueous solution. Molecular docking, MD, and MM-PBSA were used to investigate the affinity and stability of these complexes. The conformational changes, the distance between the mass centers, the gyration radii, and the numbers of hydrogen bonds were analyzed to select the most suitable candidates. Three peptides, namely CTE17, CAY15 and CSE25, have the highest affinities presenting significant numbers of residues that have contact frequencies greater than 50% of simulation run time and are the most promising candidates. CTE17 and CSE25 showed they can form a stable sandwich with the target protein. For sake of comparison, we examined the previously known peptides (FND20, INL19 and CEK17) having affinity to IL-6 and the affinity of the lead i.e. CSE25 to two other interleukin family members (IL-4 and to IL-10).
1. Introduction
Interleukin-6 (IL-6) is an important cytokine with major implications in immune inflammatory diseases, such as psoriasis,1,2 rheumatoid arthritis,3 systemic lupus erythematosus,4 systemic juvenile idiopathic arthritis,5 Crohn's disease,6 and tumorigenesis.7 In the human body, IL-6 is produced by T and B lymphotactin, fibroblasts, monocytes, keratinocytes, and endothelial and tumoral cells.8 In blood sera, the normal physiological concentrations of IL-6 are low (1–5 pg mL−1), but drastically increase by 11- to 17-fold in case of infection, inflammation, and autoimmune diseases.9,10 Therefore, IL-6 acts as a pro- and anti-inflammatory protein and is considered as a good biomarker for several diseases.11
Peptides are stable, specific, cost-effective, easy to synthetize by standardized protocols and to modify to meet tailored applications.12–14 They can be selected from combinatorial libraries can interact with various surfaces such as graphene to form nanocomposites or can be functionalized and immobilized to substrate surface to create ordered receptor layers.15–17 Very recently, we witnessed the selection of several peptides that are useful to the diagnosis of SARS-CoV-2 or to design vaccines for it,18 by blocking the association of the virus with the angiotensin-converting enzyme 2 (ACE2) receptor on the host cells.19–21 Ranganath et al. recently reported the selection of a peptide (PN-2921) to antagonize IL-6 induced signaling and associated physiopathology.22 A computer-aided selection of a peptide (KCF18) derived from cytokine receptors that can bind to pro-inflammatory cytokines such as IL-6 and TNF-α was recently reported by Jiang et al.23
Peptide-based biosensors are relatively less abundant than those based on whole proteins or aptamers due the difficulty to select peptides. They have been recently reviewed and their uses as recognition elements in the building of biosensors were examined.24–27 Indeed, peptides are suitable to detect proteins,28 nucleic acids,29 bacteria,30 metallic ions31 using fluorescent or nanoparticle tags for the signal transducing. For instance, Oh et al. designed an excimer-based beacon for the sensing of an anti-HIV antibody.32 The beacon unfolds in presence of low concentrations of the anti-P17 antibody to emit light at the pyrene emission wavelength, which differs from that of the π-stacked pyrenes. A sensitive electrogenerated chemiluminescence sandwich-based biosensor using two peptides for the capture and the reporting was designed for the sensing of troponin I protein.33 Very recently, Soamalala et al. reported the design of fluorescent peptide-based biosensing platform that can probe the CDK6 kinase activity in various cancer cells.34 Li et al. used a ferrocene-tagged 9-mers peptide-modified gold electrode to electrochemically detect the presence of amyloid β 1–42 soluble oligomer based on the conformational change of the oligopeptide probe following the recognition of the target.35
In the present work, we investigated the binding of several peptides designed from crystal structures of IL-6/protein complexes to examine their usefulness as capture and reporting probes for the IL-6 sensing. Molecular docking was carried out to provide the interfaces and poses of connections of each peptide with IL-6. To deepen the study, we resort to MD to model the natural progression of IL-6/peptide binding in saline aqueous solution to study and to improve their differences and viability. CTE17, CAY15, and SCE25 have the highest affinities and have a contact frequency greater than 50% of the simulation time, which make them the most promising candidates to design biosensors for IL-6. Finally, we used CTE17 and CSE25 to probe their usefulness to form a stable sandwich with IL-6. We compared their affinity of the peptides from literature known to antagonize IL-6. The selectivity was assessed by examining the affinity of the most promising lead CSE25 to IL-4 and to IL-10 interleukins.
2. Methods
2.1. Selection of the peptides
The structural data for IL-4 (PBD ID: 2B8U36), IL-6 (PBD ID: 1IL637) and IL-10 (PBD ID: 2ILK38) proteins were available from RCSB PDB databank. Peptides were selected from the binding areas of IL-6/IL-6 receptor or antibody complexes. This structural analysis was carried out with PyMOL,39 which enabled us to identify the possible linking sites to the IL-6. Following identification of the interaction site, we selected some amino acids for the point mutation to increase the stability and affinity of these peptides. The modeling of the variant peptides were performed using PEP-FOLD to generate their 3D structures.40 The energy of each peptide was minimized for 120 ps with NAMD.41 The snapshot of secondary structure of each peptide obtained in the end of minimization are shown in Fig. S1 (from the ESI†). All models were validated by SAVES v6.0 (https://saves.mbi.ucla.edu/).56 Pycontact42 software was used to determine the hydrophobic interactions and the salt bridges present in the different peptide/protein complexes. The number of atoms in contact between the peptides and the protein was calculated using MDAnalysis43 software.
The first peptide (called CTE17) was extracted from the structure of IL-6 in complex with a camelid Fab fragment (PBD ID: 4O9H44) from VAL29 to PRO34 and GLY89 to GLU99, with the mutation of VAL29 with CYS, GLY89 with PRO, and ALA90 with ASN. Form the same complex, we designed a second peptide (called SGC20), from SER25 to ARG33 and ALA97 to TYR107 with the mutation of ALA97 with PRO and TYR107 with CYS. The sequence of third peptide (called CAY15) was selected from the crystal structure of the Fab protein of olokizumab in complex with IL-6 (PBD ID: 4CNI45) from VAL42 to TYR62 with the mutation of VAL42 with CYS. From the structural mimicry of receptor interaction by antagonist IL-6 antibodies (PDB ID: 4ZS744) the fourth peptide (called CGF24) was extracted, from ALA23 to VAL35 and ALA91 to PHE101, with the mutation of ALA23 with CYS, VAL35 with PRO, and ALA91 with ASN. Finally, the last peptide called CSE25 was extracted from crystal structure of the hexameric human IL-6/IL-6 alpha receptor/gp130 complex (PDB ID: 1P9M22). Peptides FND20,22 INL19
46 and CEK17,47 reported in literature, to associate with IL-6 were tested for the sake of selectivity using docking and molecular dynamics in the same conditions as for the studied peptides.
2.2. Docking
The peptides were used for docking with the protein IL-6 to check the stability and determine the binding energy of each complex. The molecular docking between the protein and the different peptides was conducted using Smina,48 using Vinardo scoring function.49 For the docking, polar hydrogen atoms were added to IL-6 and its nonpolar hydrogen atoms were merged using AutoDock Tools.50 A grid box with a dimension of 54 × 52 × 58 Å3 was used to cover the full protein for the docking. For the peptides, all bonds were set as rotatable. Molecular interactions between the protein and the peptides such as the hydrogen bonds (h-bonds) and the bond lengths were analyzed using PyMOL, BIOVIA Discovery Studio Visualizer,51 and VMD (ver. 1.9.4).52
2.3. Molecular dynamics
Initially, the protein–peptide structures were minimized and equilibrated using MD for 10
000 cycles. Detailed MD simulations using the complex structures were conducted with the CHARMM36 force field.53 Each complex was solvated in a cubic box and keeping 1 nm between the complex and the edge of the solvated box. Sodium and chloride ions (C = 0.1 M) were added to neutralize the whole box. The simulation details for the IL-6/peptide in solvent environment are shown in Table S1.†
The system charge and the energy were harmonized for 30
000 cycles. In all simulations, the conditions were set at room temperature (310 K) and the atmospheric pressure (1 bar) to closely mimic the general experiment wet lab conditions. Subsequently, the fully temperature and pressure of the equilibrated system was then used as the initial configuration for the MD production analysis. All simulations were conducted using a 2 fs time step. To verify the robustness of the results multiple simulations of the protein–peptide complex combinations were conducted for a minimum of 110 ns following the same MD procedure. The results were then analyzed using common functions such as root mean square deviation (RMSD), radius of gyration (RoG) and contact frequency in VMD, while the formation of h-bonds implanted in VMD to investigate the conformational changes and the stability of the peptides, the protein, and the peptide/IL-6 complexes. H-bond occupancy for each complex was calculated using h-bond occupancy function in VMD.
Finally, starting from the final poses of CTE17/IL-6 and CSE25/IL-6 complexes, we run a simulation for CTE17/IL-6/CSE25 ternary complex for 50 ns. MD simulations were run for 40 ns to examine the CSE25 affinity to IL-6 as compared to two other interleukin family members (IL-4 and IL-10).
2.4. Binding free energy
Binding free energy using the MM-PBSA method of each complex was calculated using VMD CaFE plugin.54 Finally, the binding free energy was calculated with a total of 100 snapshots extracted from the trajectories between 70 and 110 ns simulation time (when the systems converged). In this method, the binding free energy is decomposed into the relative free energy of the solvated receptor–ligand complex (ΔGcomplex,slvd) and the separated, solvated ligand (ΔGligand,slvd) and receptor (ΔGreceptor,slvd), according to eqn (1). |
ΔGbinding,slvd = ΔGcompex,slvd − ΔGcompex,slvd − ΔGcompex,slvd
| (1) |
Each free energy change in eqn (1) is comprised of the sum of several terms, according to eqn (2).
|
ΔGslvd = ΔEMM + ΔGpol + ΔGnonpol + ΔGdisp − TΔS
| (2) |
where, Δ
EMM is the molecular mechanics energy, Δ
Gpol is the polar solvation free energy estimated from the Poisson–Boltzmann or generalized Born models, Δ
Gnonpol is the nonpolar solvation energy obtained as a function of the solvent accessible surface area, Δ
Gdisp is a cavity dispersion term, and Δ
S is an estimate of the solute entropy. Typically, the solute entropy term is neglected, as its inclusion is more sensitive to incomplete sampling errors than other terms. The Δ
EMM is further decomposed into an electrostatic component (Δ
Eelec) and a van der Waals contribution (Δ
Evdw).
3. Results and discussion
3.1. Docking
First, we looked for all potential engagement among the peptides and IL-6 protein using Smina, with Vinardo scoring function, which gives a score to each IL-6/peptide complex based on the evaluation of the binding free energy and the number of physical interactions. The Smina scores for the different IL-6/peptide complexes are summarized in Table 1. For sake of comparison, we also determined the docking score, RMSD values and the residues involved in the interaction of IL-6 and FND20, INL19 and CEK17. The latter are reported literature as having high affinities to IL-6 protein.
Table 1 Sequences of the peptides, their docking score, and RMSD values after docking. The RMSD values were determined by aligning before and after docking structure using PyMOL. Hydrogen bond interactions within 4 Å distance between each peptide and IL-6
Peptides |
Sequences |
Docking scores (kcal mol−1) |
RMSD (nm) |
Residues involved in h-bonds (within 4 Å) |
The value between parenthesis indicates the number h-bonds of a given amino acid with the protein. These peptides were studied in literature and were run for sake of comparison. |
CTE17 |
1CTASNYPPNLDIGDITE17 |
−4.4 |
0.57 |
THR2, ALA3, SER4, TYR6, ILE12, ASP14, THR16 |
CAY15 |
1CAQMRNKNYQYGTYY15 |
−9.3 |
0.73 |
GLN3, ARG5 (6)a, ASN6, LYS7, ASN8, TYR9 (2), TYR15 |
CSE25 |
1CSWQSDLDIHLLFLKTEWERDKNEE25 |
−5.9 |
0.74 |
CYS1 (3), TRP3 (2), GLN4 (2), HSD10, TRP18, GLU19, ASN23, GLU25 |
CGF24 |
1CGANNDIGTYAVPNSYRNFNNAVF24 |
−5.6 |
0.82 |
CYS1 (3), ASN4 (4), SER15, TYR16, PHE24 (2) |
SGC20 |
1SGFTFSSYRPNRAGWGMGDC20 |
−5.4 |
0.75 |
SER1 (2), GLY2, PHE3, PHE5 |
FND20b |
1FNMQQRFYLHPNENAKKSRD20 |
−4.8 |
0.60 |
HIS10, PRO11, ASN14 (2) |
INL19b |
1INTLLSEINSILLDIISLL19 |
−5.4 |
0.72 |
SER6 (2), ASN9, SER10 (2), ASP14, SER17 (2), LEU19 (2) |
CEK17b |
1CESSKEALAENNLNLPK17 |
−5.3 |
0.74 |
SER3, SER4 (3), GLU10 |
3.1.1. Short range interactions. Docking results represented in Table 1 show that CTE17 has the lowest docking score energy with the target protein. A further analysis, with Discovery software, shows that the peptide forms seven h-bonds with LYS110 (2), SER03, LEU115 and GLN109 (3) from IL-6 with less than 4 Å. Fig. 1 displays using color code all the mentioned interactions. CTE17 also makes one salt-bridge and an electrostatic interaction. On the contrary, peptide CAY15 exhibits the highest docking score (−9.3 kcal mol−1) and shows the greatest number of interactions making it the most stable complex. Indeed, CAY15 forms 13 h-bonds with CYS32, GLU37, ALA38 (2), ALA40, ASN43 (2), LEU46, GLU51, CYS55, ARG150, SER151, GLU154, and two electrostatic bonds of π-cation type with ARG161 and π-anion type with GLU154. Two hydrophobic bonds of π–sigma and π–alkyl types with ARG161 and π–π stacking interaction with PHE56 are also formed.
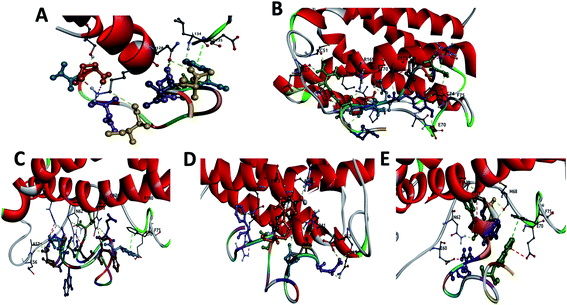 |
| Fig. 1 Display of the interactions of: (A) CTE17, (B) CAY15, (C) CSE25, (D) CGF24 and (E) SGC20 with IL-6 protein showing the hydrogen bonds (yellow), salt bridges (magenta), electrostatic interactions (red) and vdW interactions (green). | |
The three complexes formed by CSE25, CGF24 and SGC20 have close interaction energy values. Among the 3 complexes, CSE25 has the highest number of hydrogen bonds interacting with ARG22 (2), GLU37, ALA38 (2), ASN43, GLU51, ARG150 (3), GLU154, GLN157 and 4 salt bridges. It is worthy to note that ARG22 and ARG150 from the IL-6 protein form 5 h-bonds and 4 salt bridges with 4 residues from the above-mentioned peptide. CGF24 also shows a high number of hydrogen bond interactions (11 h-bonds) and one salt bridge. Finally, SGC20 has the lowest number of hydrogen bonds (5 h-bonds) and one salt bridge, but it has the highest number hydrophobic interactions with LEU46, PRO47 (2), PHE56 and one electrostatic interaction with GLU51.
The docking scores for the three reference peptides are in the same range as CTE17, CSE25, CGF24 and SGC20 peptides and are lower than that of CAY15. Except INL19 which established 10 h-bonds with IL-6, FND20 and CEK17 have only 4 and 5 h-bonds with the target protein, which less than those made by the peptides examined in this study.
3.1.2. RMSD analysis of the peptides. With respect to their initial structures, the RMSD docking values for all peptides, ranges from 0.57 to 0.82 nm, as presented in Table 1. The whole bonds in the peptide backbone were set to be rotatable. Each atom will be capable to turn freely to be engaged with the receptor surface following the force field algorithm in the docking software. For this reason, the RMSD values show a high flexibility of the peptides, relatively to the protein. Comparison of the conformation before and after docking demonstrated that CAY15 and CSE25 have a high RMSD (<0.82 nm), as they changed their conformation from an α-helix to a coil (Fig. S1†). The general impact of a such modification may be identified with the reorientation of a few residues and torsional modifications in the principal chain (helix-coil transition) by breaking the helix internal h-bonds and establishing new interactions with IL-6.55 CTE17 has the lowest RMSD, which can be explained by the minor structural changes before and after the docking. Furthermore, the RMSD values for FND20, INL19 and CEK17 vary from 0.60 and 0.74 nm, which is the same range as for the studied peptides, denoting the similarities.
3.1.3. Analysis of the interaction poses. The structure of IL-6 is constituted of four-helix arranged in an up–up–down–down with a long loop connecting the helices. The interface analysis revealed that the peptides are interacting in different regions within the protein. Fig. 2 shows that peptides CAY15, CSE25, CGF24 and SGC20 interact mainly with helix 4 and loop 1, and they share only the amino acids GLU37, ASN43, ALA58 in loop 1 LYS143, ARG150 and GLU154 in helix 4. CTE17 interacts with the IL-6 protein in a different section in respect to the others, it interacts with helix 1, helix 3 and loop 2. The reference peptides FND20, INL19 and CEK17 interact with loop 1 and loop 2 (FND20) and INL19 and CEK17 bind to the same area ranging from the loop 2, helix 2 and helix 3. This area is the opposite side than CSE25 with make possible for IL-6 to simultaneously bind to the two peptides. All the peptides form with the target protein at least 5 h-bonds and one salt bridge with other electrostatic and hydrophobic interactions. This diversity of zones of interaction between the peptides and the protein, and the important number of interactions should be verified with MD simulation.
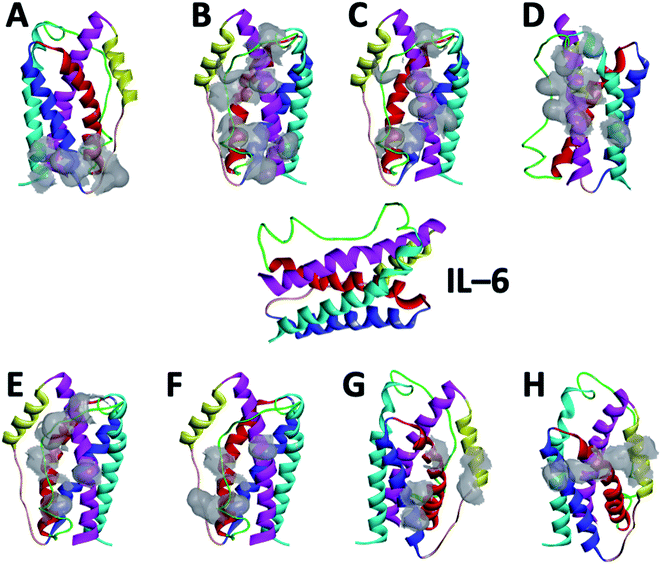 |
| Fig. 2 Amino acid residues of IL-6 participating in the interaction with the different peptides: (A) CTE17, (B) CAY15, (C) CSE25, (D) CGF24, (E) SGC20, (F) FND20, (G) INL19 and (H) CEK17. IL-6 color coded components: helix 1, helix 2, helix 3, and helix 4, loop 1, and loop 2. | |
3.2. Molecular dynamics
MD simulation is an attractive approach to examine the real-time dynamics and conformational stability of a protein upon binding to a given ligand. For this purpose, we carried out MD simulation for 110 ns. To understand the affinity between these peptides and IL-6 and the rigidity for the complexes, several points such as the peptide stability and its average RMSD (Fig. 3A), hydrogen bonding capacity (Fig. 3B), distance between IL-6 and peptides (Fig. 3C) and contact frequency (Fig. 3D) were investigated. The RMSD, hydrogen bonding capacity and contact frequency for INL19 and CEK17 are given the ESI.†
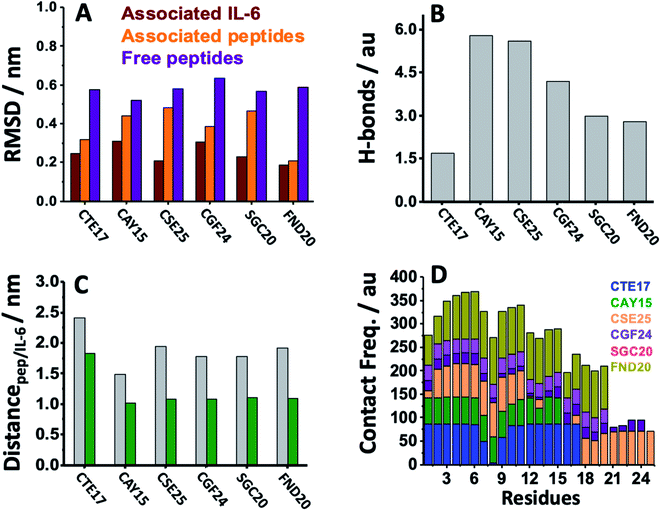 |
| Fig. 3 (A) Average RMSD of the free peptides, the associated peptides and IL-6 in complex systems studied in aqueous NaCl solution. (B) Average number of hydrogen bonds established between the peptides and IL-6 for the different peptide/IL-6 systems. (C) Average distance separating the centers of masses of the protein/peptide (grey) and protein/surface of contacts (green). (D) Contact frequency between the residues of each peptide and IL-6 calculated for the different complexes. | |
3.2.1. RMSD. To find out time-dependent conformational modification and the stability of complexes, RMSD analysis was undertaken. The RMSD of each peptide and the IL-6 domain was performed and are presented in Fig. S3.† The initial energy-minimized structure of each system was taken as the reference structure. The average RMSD values of the peptides were calculated, it can be noted that the highest value does not exceed 0.5 nm denoting a decrease of 15% to 18% for CAY15, CSE25 and SGC20 and more drastic decrease of 40% to 45% CGF24 and CTE17 compared to the RMSD averages of the free peptides. The overall decrease denotes a stabilization of the peptides when associated with the IL-6 protein via noncovalent interactions.As shown in the ESI (Fig. S4†), the associated CTE17 and SGC20 witnessed their RMSD values progressively increasing until 20 ns and then converged with minor fluctuations. The RMSD plot of associated CAY15 recorded a jump of 0.3 nm at a simulation time of 33 ns. Similarly, in the case of CSE25 and CGF24, the RMSD values sharply increased by 0.2 nm at simulation time of 60 ns and 70 ns, respectively, then the two complexes remained stable and nicely converged after this drift. Similarly, FND20 used a reference peptide showed that its RMSD did not have any drift during the MD simulation time, thus confirming its stable conformation during the run. The RMSD value of IL-6 associated with this peptide (1.9 nm) is close to that of IL-6/CTE17 complex and lower than that of IL-6/CSE25 (2.5 nm).
The average RMSD of the associated IL-6 did not exceed 0.31 nm and due to it high molecular weight compared to the short peptides. It is worthy noting that IL-6 associated with CSE25 have the lowest average RMSD.
3.2.2. Hydrogen bonding. Hydrogen bonding of the complexes turns out to verify affinity of each peptide to IL-6 as estimated by the docking simulation. For this purpose, a further analysis based on two methods was applied. Firstly, the average number of h-bonds in the complexes was calculated. Based on these results, CTE17 has the lowest hydrogen bonding capacity (less than 02 h-bonds) with IL-6, in contrast CAY15 and CSE25 have an average of 6 h-bonds. These results are close those found by molecular docking, showing CAY15 and CSE25 having almost twice hydrogen bonds as CTE17. Likewise, FND20 have the same number of h-bonds as CSE25. The ARG6, ARG19, ASP20 residues from FND20 established hydrogen bonding with GLU51, LYS52 amino acids from the IL-6 protein.We further computed the occupancy of h-bonds in interaction between the peptides and IL-6. Some of the active binding residues can form hydrogen bonds by becoming the electron donor through their main and side chains. Also, CAY15 and CSE25 have the most active binding residues that give more than 50% occupancy of dynamic intermolecular hydrogen bonds, namely GLU37, GLU51, GLU154, and ARG161 of the receptor protein with CAY15, also ARG22, ARG150, ARG162, and ARG164 with CSE25 (Table S2†).
3.2.3. Distance IL-6/peptides. The mass centers between the protein and the peptides were also calculated. The results showed that CTE17 is the most distant from IL-6, this may be explained by the interaction poses since it interacts with amino acids far from the mass center, as shown in Fig. 3C. On the other hand, the distance between the mass center of CAY15 and IL-6 is shorter by 40% than that between CTE17 and IL-6. The three other peptides lay a distance shorter by 20% to 30% compared to that observed for CTE17, since they all interact with the lateral face of the protein, which is close to the mass center.The distance separating about 1.9 nm is close to that found for the studied peptides such as CSE25, CGF25 and SGC20, but is longer that found for CTE17 and CAY15.
Based on the contact frequency, the peptides CTE17, CAY15, and CSE25 are promising candidates because they have a significant number of residues that have a contact frequency greater than 50% in the last 40 ns. Indeed, CTE17 has 15 residues out of 17, CAY15 has 13 residues out of 15, while SGC20 has 18 residues out of 25 in contact with IL-6, respectively.
3.2.4. Gyration radii. Finally, we analyzed the gyration radii which can provide information related to the folding and unfolding of the protein structure upon binding of the ligands. High RoG values would explain less compactness with high conformational entropy while low values denote high compactness and more stability of the structure. As evident from Fig. S5,† all the systems have projected the gyration averages between 0.76 to 1.02 nm. In the case of CTE17, the average of RoG was found to be 0.76 nm while CAY15 and CGF24 showed averages of 0.85 and 0.92 nm, respectively. Similarly, in case peptide SGC20 and CSE25 bound to the target protein the RoG averages was found to be 0.94 and 1.02 nm, respectively. The data reveal that all the systems were compact throughout the simulation, which indicate that the systems are well converged.
3.2.5. Contacts, salt bridges and hydrophobic interactions. The total number of contacts for CTE17, CAY15, CSE25, CGF24 and SGC20 are given in Fig. 4. The averages for the different peptides are 73, 131, 105, 88 and 120 points of contact, respectively. These results do not corroborate with the data from thermodynamic calculations. While the same peptides have 5 (2 hydrophobic), 12 (5 hydrophobic), 28 (0 hydrophobic), 9 (0 hydrophobic) and 1 (3 hydrophobic) salt bridges of the mean occupancy of frame for time cutoff of 50%. These results are in good agreement with the high affinity of CTE17, CAY15, CSE25 toward IL-6 protein (Table S3†).
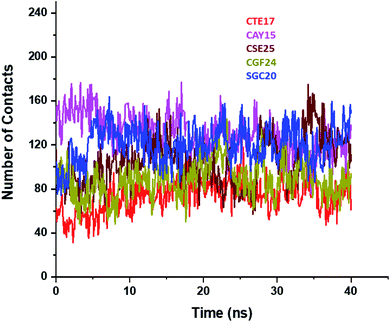 |
| Fig. 4 Plots of number of contacts between IL-6 and the peptides during the last 40 ns of the simulation time. | |
3.3. Binding free energy
Energy calculation was carried out for all five complexes to define their binding affinities using MM-PBSA method. To evaluate the binding free energy, each time 100 conformations were extracted from the MD production run when the complexes stabilized after 70 ns. The calculated free energies for the solvated systems formed by the target protein and the peptides (CTE17, CAY15, CSE25, CGF24 and SGF20) were found to be −64.9 kcal mol−1, −74.1 kcal mol−1, −92.7 kcal mol−1, −21.9 kcal mol−1, −39.4 kcal mol−1, respectively. One can see that peptides CAY15 and CSE25 bound more strongly to the IL-6 than others, these results were coherent with Smina scores that indicated that CAY15 and CSE25 have the lowest energy scores (cf. Table 1). To grasp the effect of the different energy contribution terms in this method, total free energy decomposition into van der Waals (ΔEvdw) and electrostatic (ΔEelec) contributions, and polar solvation energy and apolar solvation energy components are summarized in Table 2.
Table 2 Free energies for the solvated systems and details of the energy contributions for the different complexes
Complexes |
ΔG(binding,slvd) (kcal mol−1) |
ΔEvdw (kcal mol−1) |
ΔEelec (kcal mol−1) |
ΔGpol (kcal mol−1) |
ΔGnonpol (kcal mol−1) |
CTE17/IL-6 |
−64.9 |
−29.9 |
−324.1 |
294.1 |
−5.0 |
CAY15/IL-6 |
−74.1 |
−33.6 |
−207.8 |
173.0 |
−5.7 |
CSE25/IL-6 |
−92.7 |
−17.3 |
−469.3 |
399.1 |
−5.1 |
CGF24/IL-6 |
−21.9 |
−12.0 |
−57.0 |
49.8 |
−2.6 |
SGF20/IL-6 |
−39.4 |
−21.2 |
−139.8 |
125.2 |
−3.5 |
The results indicated that the major favorable contributors come from the electrostatic (ΔEelec) and van der Waals interactions (ΔEvdw) while the polar component of solvation (ΔGpol) contributed unfavorably to the binding of all five complexes. The nonpolar solvation energy has a minor favorable effect, which is one sixth to one third of the electrostatic one.
3.4. CTE17/IL-6/CSE25 sandwich system
Based on the results of MD simulations and the free binding energies, it appears that CAY15 and CSE25 are the most attractive candidates for the IL-6 protein recognition. They showed the lowest free binding energy and have the highest stability and affinity with the target protein. CTE 17 is also a suitable candidate, however it has lesser hydrogen binding capacity. CTE17 has 15 of 17 residues that have a contact frequency greater than 50%, and it recorded the lowest RMSD and gyration radius averages. The lower averages values of RoG and RMSD for CTE17 is an indication of a stronger interaction and greater stability established with the target protein.
3.4.1. Selecting the peptides. To verify the interaction poses between the peptides and IL-6, we started the last frames displayed by VMD and the contact frequency in the last 10 ns from the MD simulations of each complex. The visual analysis showed that CTE17 and CSE25 interact in different positions with the target protein (Fig. S7†), while the CAY15 and CSE25 compete for the same region and share the ARG150 to GLN165 from IL-6 helix 4 (Fig. 5). Obviously, CAY15 and CSE25 can't be used to form a sandwich with the protein since they will compete to occupy the same positions, while CTE17 and CSE25 can be used.
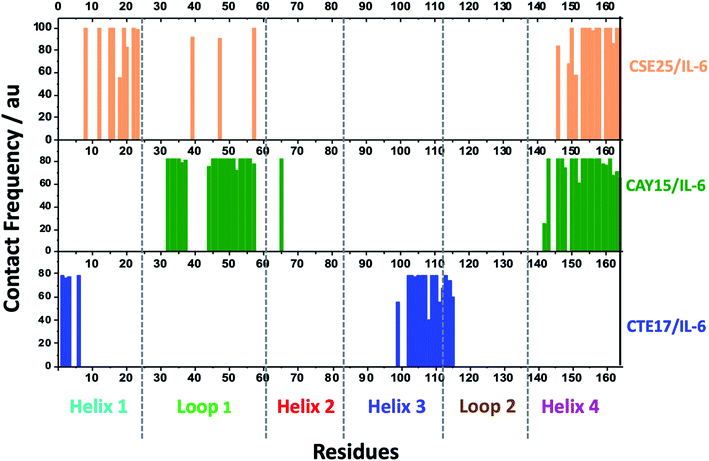 |
| Fig. 5 Contact frequency of IL-6 residues in the last 10 ns of the MD simulation run time of CTE17, CAY15 and CSE25 with the target protein. | |
3.4.2. RMSD analysis. For this purpose, another MD simulation for 50 ns in the same conditions was run using two selected peptides (i.e. CTE17 and CSE25) associated with IL-6 to evidence the possibility to make a stable sandwich system. Analysis of RMSD and gyration radius, given in Fig. S8,† shows the sandwich system (CTE17/IL-6/CSE25) rapidly converged and stabilized after 10 ns. The new CTE17/IL-6/CSE25 complex is more stable and rigid than the two starting complexes (Fig. 6). Compared with CTE17/IL-6 and CSE25/IL-6, CTE17/IL-6/CSE25 witnesses a decrease of its RMSD average (Fig. 6A). For the CTE17, the average RMSD decrease was 13% compared to that of CTE17/IL-6 and a more drastic decrease of 52% in respect to free peptide. CSE25 witnessed a decrease of its RMSD average respectively by 42% and 35% when compared with its free and associated forms.
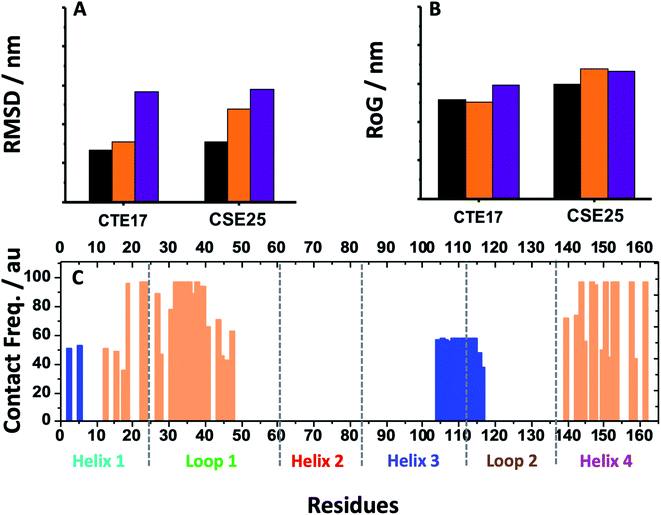 |
| Fig. 6 (A) Average RMSD values and (B) RoG values for free CTE17 and CSE25 (purple), associated with IL-6 (orange) and in sandwich IL-6 system (black). (C) Contact frequency of IL-6 residues in the last 10 ns with CTE17 (blue) and CSE25 (orange) in sandwich IL-6 system. | |
Furthermore, the RMSD average of IL-6 sandwich for was found to be 0.21 nm, which is at least 33% lower that of the different IL-6/peptides. These RMSD value decreases are indicative of a strong interaction establishment between the two peptides and the target protein, which reduces the fluctuation of the different components.
3.4.3. Gyration radii. The study of RoG for CSE25 and CTE17 showed different behaviors. Indeed, the RoG of CSE25 decreased for the sandwich format in respect to the binary associated formats with IL-6, while the RoG value of sandwiched CTE17 is slightly higher than CTE17/IL-6 binary associated format. This can be attributed to the interaction positions of CTE17 and CSE25 with the IL-6, which loses some interacting amino acids (LEU01, THR02, LYS102, VAL103, LEU104) and the decrease of the time of contact (ILE105 to LEU115) after the association with CSE25 (Fig. 6C).
3.4.4. Binding energy. The Binding free energies indicated in Table 3 show that sandwich system a binding energy slightly lower than the sum of CTE17/IL-6 and CSE25/IL-6 energies. Moreover, the ΔEelec and ΔEvdw have the highest significant difference (ΔΔEelec and ΔΔEvdw) of −121.9 and −11.0 kcal mol−1, respectively. A ΔΔGpol of +138.6 kcal mol−1 is the most disfavoring component, which compensate the van der Waals and electrostatic contributions to give a slightly less stable ternary complex than the sum of the two binary complexes. This can be attributed to the overall changes in the h-bonds, electrostatic interactions, and the time of interactions with the ternary complex compared to the binary ones (Fig. 6C).
Table 3 Free energies for the solvated systems and details of the energy contributions for the different complexes
Complexes/energy |
CTE17/IL-6/CSE25 |
CSE25/IL-6 |
CTE17/IL-6 |
ΔΔGbinding,slvd = ΔGbinding,slvd (CTE17/IL-6/CSE25) − ΔGbinding,slvd (CTE17/IL-6) − ΔGbinding,slvd (CSE25/IL-6). ΔΔEvdw = ΔEvdw (CTE17/IL-6/CSE25) − ΔEvdw (CTE17/IL-6) − ΔEvdw (CSE25/IL-6). ΔΔEelec = ΔEelec (CTE17/IL-6/CSE25) − ΔEelec (CTE17/IL-6) − ΔEelec (CSE25/IL-6). ΔΔGpol = ΔGpol (CTE17/IL-6/CSE25) − ΔGpol (CTE17/IL-6) − ΔGpol (CSE25/IL-6). ΔΔGnonpol = ΔGnonpol (CTE17/IL-6/CSE25) − ΔGnonpol (CTE17/IL-6) − ΔGnonpol (CSE25/IL-6). |
ΔG(binding,slvd) (kcal mol−1) |
−153.1 |
−92.7 |
−64.9 |
ΔEvdw (kcal mol−1) |
−58.2 |
−17.3 |
−29.9 |
ΔEelec (kcal mol−1) |
−915.3 |
−469.3 |
−324.1 |
ΔGpol (kcal mol−1) |
831.8 |
399.1 |
294.1 |
ΔGnonpol (kcal mol−1) |
−11.3 |
−5.1 |
−5.0 |
ΔΔG(binding,slvd)a (kcal mol−1) |
+4.5 |
*** |
*** |
ΔΔEvdwb (kcal mol−1) |
−11.0 |
*** |
*** |
ΔΔEelecc (kcal mol−1) |
−121.9 |
*** |
*** |
ΔΔGpold (kcal mol−1) |
+138.6 |
*** |
*** |
ΔΔGnonpole (kcal mol−1) |
−1.2 |
*** |
*** |
3.5. Selectivity
To study the selectivity, we first examined the affinity of known peptides from literature to IL-6 target protein. As displayed in Fig. 7A, we can see that CSE25 has a slightly lower affinity than FND20 and a higher affinity than both INL19 and CEK17, suggesting that CSE25 is a good candidate to antagonize this protein. Furthermore, FND20 has the highest number of contacts and the number of hydrogen bonds and the lowest RMSD value. This is good agreement with the thermodynamic data showing FND20 has the highest interaction energy.
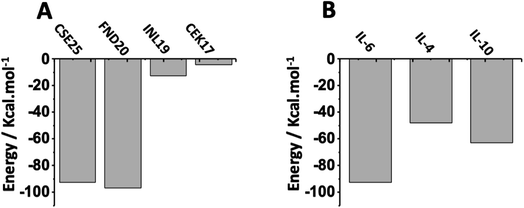 |
| Fig. 7 Interaction energies of (A) CSE25, FND20, INL19 and CEK17 with IL-6 protein and (B) CSE25 with IL-6, IL-4, and IL-10. | |
On another hand, thermodynamic calculations showed that CSE25 to has higher affinity to IL-6 compared to those found with IL-4 and IL-8. Indeed, the peptide demonstrates that it has twice affinity for IL-6 than IL-4 and is more prone to associate with IL-6 by ca. 30% than to IL-10 (Fig. 7B). The results suggest that CSE25 could recognize the target protein with high affinity as experimentally found for FND20 in complex matrix containing other biomolecules.
To further deepen the study, we determined the RMSD values, the number of hydrogen bonds, total number of contacts and the interaction energies, extracted for MD calculations, for CTE17, C1Y15 and CSE25 with IL-4, IL-6 and IL-10 (Table 4). Indeed, the RSMD value for CTE17 with IL-6 is lower than those obtained with IL-4 and IL-10. For CAY15, the values are similar for all the examined three proteins, while that calculated for CSE25 is slightly higher than the RMSD values obtained for the two other proteins, denoting a lower affinity of the peptide to the competitor proteins. These results are contradicted by the value of hydrogen bonds and number of contacts for CTE17, CAY15 and CSE25 which are 2- to 10-fold higher than those obtained with IL-4 and IL-10 proteins, suggesting higher affinity to the target protein.
Table 4 RMSD values, number of hydrogen bonds, total number of contacts and interaction energies for CTE17, C1Y15 and CSE25 with IL-4, IL-6 and IL-10 proteins
Peptides |
Proteins |
RMSD (nm) |
Number of h-bonds |
Number of contacts |
ΔG(binding,slvd) (kcal mol−1) |
CTE17 |
IL-6 |
0.08 |
1.70 |
73 |
−64.9 |
IL-4 |
0.14 |
0.85 |
27 |
−27.4 |
IL-10 |
0.30 |
1.09 |
84 |
−66.6 |
CAY15 |
IL-6 |
0.16 |
5.90 |
131 |
−74.1 |
IL-4 |
0.14 |
1.64 |
35 |
48.1 |
IL-10 |
0.17 |
0.60 |
21 |
−21.5 |
CSE25 |
IL-6 |
0.26 |
5.50 |
105 |
−92.7 |
IL-4 |
0.18 |
1.39 |
58 |
−47.8 |
IL-10 |
0.22 |
0.71 |
84 |
−62.6 |
Overall, the number of contacts established between the three peptides and the different proteins is higher in case of IL-6 than with IL-4 and IL-10. Graphical representation of the number of contacts for all the peptides, number of hydrogen bonds are given ESI (Fig. S7 and S10†). Finally, the interaction energies of CTE17 with IL-6 and IL-10 are close and are twofold higher than that computed for IL-4/CTE17 binding. CAY15 has 31% and 71% more affinity to IL-6 than to IL-4 and to IL-10, respectively. CSE25 binds 32% and 48% more strongly with IL-6 than with IL-4 and with IL-10, respectively. These findings show the potential selectivity of the candidates (CTE17, CAY15 and CSE25) designed in this study, toward IL-6 and may yield selective sensing of the target protein in complex biological matrix such as blood serum.
4. Conclusion
In this work, we set as a goal to select peptides that can form a sandwich complex with IL-6 protein to construct a sensing device. Starting from the crystal structure of IL-6 complexes available in the PDB bank, we designed five peptides that can bind to IL-6 in different poses. Using docking and MD simulations, we showed that the different peptides are able to recognize different poses on the surface of the target protein. From the analysis of the number of hydrogen bonds and the occupancy, we found that CTE17, CAY15, and CSE25 are the most promising candidates since they have a significant number of residues that have a contact frequency over 50%. Furthermore, MM-PBSA energy calculation confirmed that they have the highest interaction energies coming mostly the electrostatic and van der Waals contributions. We further showed that CTE17, CSE25 and IL-6 form a stable sandwich complex, with a free binding energy approximately equal to the sum of the two binary CTE17/IL-6 and CSE25/IL-6 complexes. Furthermore, we compared the ability of these peptides to antagonize IL-6 with peptides from literature selected for their affinity of the target protein. We also examined the selectivity of CSE25 to IL-6 by examining it affinity to IL-4 and to IL-10 using MD simulations. Based on these computer-aided findings, we can conclude that these are the best candidates to build sandwich-based sensing platforms for IL-6 detection using different transduction techniques.
Conflicts of interest
The authors declare no conflict of interest.
Acknowledgements
Authors wish to acknowledge the financial support from NanoFastResponse and SmartBioSens PRF projects (ref. PRF2017-D4P1 and PRFCOV19-D2P2).
References
- A. Saggini, S. Chimenti and A. Chiricozzi, J. Immunol. Res., 2014, 2014, 964069 CrossRef PubMed
. - A. L. Croxford, S. Karbach, F. C. Kurschus, S. Wortge, A. Nikolaev, N. Yogev, S. Klebow, R. Schuler, S. Reissig, C. Piotrowski, E. Brylla, I. Bechmann, J. Scheller, S. Rose-John, F. Thomas Wunderlich, T. Munzel, E. von Stebut and A. Waisman, J. Invest. Dermatol., 2014, 134, 728–735 CrossRef CAS PubMed
. - Y. Yoshida and T. Tanaka, BioMed Res. Int., 2014, 2014, 698313 Search PubMed
. - K. Ohl and K. Tenbrock, J. Biomed. Biotechnol., 2011, 2011, 432595 Search PubMed
. - S. Yokota, T. Imagawa, M. Mori, T. Miyamae, Y. Aihara, S. Takei, N. Iwata, H. Umebayashi, T. Murata, M. Miyoshi, M. Tomiita, N. Nishimoto and T. Kishimoto, Lancet, 2008, 371, 998–1006 CrossRef CAS
. - V. Gross, T. Andus, I. Caesar, M. Roth and J. Scholmerich, Gastroenterology, 1992, 102, 514–519 CrossRef CAS
. - J. F. Rossi, Z. Y. Lu, M. Jourdan and B. Klein, Clin. Cancer Res., 2015, 21, 1248–1257 CrossRef CAS PubMed
. - P. Ataie-Kachoie, M. H. Pourgholami, D. R. Richardson and D. L. Morris, J. Clin. Pathol., 2014, 67, 932–937 CrossRef CAS PubMed
. - P. Fraunberger, Y. Wang, E. Holler, K. G. Parhofer, D. Nagel, A. K. Walli and D. Seidel, Shock, 2006, 26, 10–12 CrossRef CAS PubMed
. - T. Robak, A. Gladalska, H. Stepien and E. Robak, Mediators Inflammation, 1998, 7, 347–353 CrossRef CAS PubMed
. - C. A. Hunter and S. A. Jones, Nat. Immunol., 2015, 16, 448–457 CrossRef CAS PubMed
. - M. Erak, K. Bellmann-Sickert, S. Els-Heindl and A. G. Beck-Sickinger, Bioorg. Med. Chem., 2018, 26, 2759–2765 CrossRef CAS PubMed
. - M. S. Zambrano-Mila, K. E. S. Blacio and N. S. Vispo, Ther. Innov. Regul. Sci., 2020, 54, 308–317 CrossRef PubMed
. - T. MacCulloch, A. Buchberger and N. Stephanopoulos, Org. Biomol. Chem., 2019, 17, 1668–1682 RSC
. - L. Wang, Y. Zhang, A. Wu and G. Wei, Anal. Chim. Acta, 2017, 985, 24–40 CrossRef CAS PubMed
. - V. Secchi, S. Franchi, M. Santi, M. Dettin, A. Zamuner, G. Iucci and C. Battocchio, J. Phys. Chem. C, 2018, 122, 6236–6239 CrossRef CAS
. - J. P. Piccoli, A. Santos, N. A. Santos-Filho, E. N. Lorenzón, E. M. Cilli and P. R. Bueno, Pept. Sci., 2016, 106, 357–367 CrossRef CAS PubMed
. - V. Kesarwani, R. Gupta, R. R. Vetukuri, S. K. Kushwaha and S. Gandhi, Front. Immunol., 2021, 12, 725240 CrossRef CAS PubMed
. - P. A. Valiente, H. Wen, S. Nim, J. Lee, H. J. Kim, J. Kim, A. Perez-Riba, Y. P. Paudel, I. Hwang, K. D. Kim, S. Kim and P. M. Kim, J. Med. Chem., 2021, 64, 14955–14967 CrossRef CAS PubMed
. - V. K. Priya, S. P. Rath and P. Abraham, PLoS One, 2021, 16, e0251913 CrossRef PubMed
. - T. Li, Q. Kan, J. Ge, Z. Wan, M. Yuan, Y. Huang, Q. Xie, Y. Yang, H. Shao, X. Li, L. Ye, A. Qin, Z. Bu, P. Liu and J. Ye, Cell. Mol. Immunol., 2021, 18, 2563–2565 CrossRef CAS PubMed
. - S. Ranganath, A. Bhandari, N. Avitahl-Curtis, J. McMahon, D. Wachtel, J. Zhang, C. Leitheiser, S. G. Bernier, G. Liu, T. T. Tran, H. Celino, J. Tobin, J. Jung, H. Zhao, K. E. Glen, C. Graul, A. Griffin, W. C. Schairer, C. Higgins, T. L. Reza, E. Mowe, S. Rivers, S. Scott, A. Monreal, C. Shea, G. Bourne, C. Coons, A. Smith, K. Tang, R. A. Mandyam, J. Masferrer, D. Liu, D. V. Patel, A. Fretzen, C. A. Murphy, G. T. Milne, M. L. Smythe and K. E. Carlson, PLoS One, 2015, 10, e0141330 CrossRef PubMed
. - S. J. Jiang, P. I. Tsai, S. Y. Peng, C. C. Chang, Y. Chung, H. H. Tsao, H. T. Huang, S. Y. Chen and H. J. Hsu, Sci. Rep., 2019, 9, 2317 CrossRef PubMed
. - S. Pavan and F. Berti, Anal. Bioanal. Chem., 2012, 402, 3055–3070 CrossRef CAS PubMed
. - A. J. M. Barbosa, A. R. Oliveira and A. C. A. Roque, Trends Biotechnol., 2018, 36, 1244–1258 CrossRef CAS PubMed
. - M. Puiu and C. Bala, Bioelectrochemistry, 2018, 120, 66–75 CrossRef CAS PubMed
. - Q. Liu, J. Wang and B. J. Boyd, Talanta, 2015, 136, 114–127 CrossRef CAS PubMed
. - S. K. Arya, P. Kongsuphol, C. C. Wong, L. J. Polla and M. K. Park, Sens. Actuators, B, 2014, 194, 127–133 CrossRef CAS
. - K. T. O'Neil, R. H. Hoess and W. F. DeGrado, Science, 1990, 249, 774–778 CrossRef PubMed
. - E. Pardoux, D. Boturyn and Y. Roupioz, Molecules, 2020, 25, 1988 CrossRef PubMed
. - M. R. Contarino, M. Sergi, A. E. Harrington, A. Lazareck, J. Xu and I. Chaiken, J. Mol. Recognit., 2006, 19, 363–371 CrossRef CAS PubMed
. - K. J. Oh, K. J. Cash and K. W. Plaxco, J. Am. Chem. Soc., 2006, 128, 14018–14019 CrossRef CAS PubMed
. - M. Shan, M. Li, X. Qiu, H. Qi, Q. Gao and C. Zhang, Gold Bull., 2014, 47, 57–64 CrossRef CAS
. - J. Soamalala, S. Diot, M. Pellerano, C. Blanquart, M. Galibert, M. Jullian, K. Puget and M. C. Morris, Chembiochem, 2021, 22, 1065–1071 CrossRef CAS PubMed
. - H. Li, Y. Cao, X. Wu, Z. Ye and G. Li, Talanta, 2012, 93, 358–363 CrossRef CAS PubMed
. - M. Kraich, M. Klein, E. Patino, H. Harrer, J. Nickel, W. Sebald and T. D. Mueller, BMC Biol., 2006, 4, 13 CrossRef PubMed
. - G. Y. Xu, H. A. Yu, J. Hong, M. Stahl, T. McDonagh, L. E. Kay and D. A. Cumming, J. Mol. Biol., 1997, 268, 468–481 CrossRef CAS PubMed
. - A. Zdanov, C. Schalk-Hihi and A. Wlodawer, Protein Sci., 1996, 5, 1955–1962 CrossRef CAS PubMed
. - The PyMOL Molecular Graphics System, Version 2.0 Schrödinger, LLC Search PubMed
. - Y. Shen, J. Maupetit, P. Derreumaux and P. Tuffery, J. Chem. Theory Comput., 2014, 10, 4745–4758 CrossRef CAS PubMed
. - J. C. Phillips, D. J. Hardy, J. D. C. Maia, J. E. Stone, J. V. Ribeiro, R. C. Bernardi, R. Buch, G. Fiorin, J. Hénin, W. Jiang, R. McGreevy, M. C. R. Melo, B. K. Radak, R. D. Skeel, A. Singharoy, Y. Wang, B. Roux, A. Aksimentiev, Z. Luthey-Schulten, L. V. Kalé, K. Schulten, C. Chipot and E. Tajkhorshid, J. Chem. Phys., 2020, 153, 044130 CrossRef CAS PubMed
. - M. Scheurer, P. Rodenkirch, M. Siggel, R. C. Bernardi, K. Schulten, E. Tajkhorshid and T. Rudack, Biophys. J., 2018, 114, 577–583 CrossRef CAS PubMed
. - N. Michaud-Agrawal, E. J. Denning, T. B. Woolf and O. Beckstein, J. Comput. Chem., 2011, 32, 2319–2327 CrossRef CAS PubMed
. - C. Blanchetot, N. De Jonge, A. Desmyter, N. Ongenae, E. Hofman, A. Klarenbeek, A. Sadi, A. Hultberg, A. Kretz-Rommel, S. Spinelli, R. Loris, C. Cambillau and H. de Haard, J. Biol. Chem., 2016, 291, 13846–13854 CrossRef CAS PubMed
. - S. Shaw, T. Bourne, C. Meier, B. Carrington, R. Gelinas, A. Henry, A. Popplewell, R. Adams, T. Baker, S. Rapecki, D. Marshall, A. Moore, H. Neale and A. Lawson, mAbs, 2014, 6, 774–782 CrossRef PubMed
. - T. Kobayashi, M. Kakui, T. Shibui and Y. Kitano, Mol. Biotechnol., 2011, 48, 147–155 CrossRef CAS PubMed
. - L. Desallais, C. Bouchez, H. Mouhsine, G. Moreau, R. Ratsimandresy, M. Montes, H. Do, F. Quintin-Colonna and J. F. Zagury, Sci. Rep., 2016, 6, 19549 CrossRef CAS PubMed
. - D. R. Koes, M. P. Baumgartner and C. J. Camacho, J. Chem. Inf. Model., 2013, 53, 1893–1904 CrossRef CAS PubMed
. - R. Quiroga and M. A. Villarreal, PLoS One, 2016, 11, e0155183 CrossRef PubMed
. - G. M. Morris, R. Huey, W. Lindstrom, M. F. Sanner, R. K. Belew, D. S. Goodsell and A. J. Olson, J. Comput. Chem., 2009, 30, 2785–2791 CrossRef CAS PubMed
. - Biovia and Dassault Systèmes, Discovery Studio Visualizer, 2018 Search PubMed
. - W. Humphrey, A. Dalke and K. Schulten, J. Mol. Graphics, 1996, 14(33–38), 27–38 Search PubMed
. - K. Vanommeslaeghe, E. Hatcher, C. Acharya, S. Kundu, S. Zhong, J. Shim, E. Darian, O. Guvench, P. Lopes, I. Vorobyov and A. D. Mackerell Jr, J. Comput. Chem., 2010, 31, 671–690 CAS
. - H. Liu and T. Hou, Bioinformatics, 2016, 32, 2216–2218 CrossRef CAS PubMed
. - R. Nussinov, B. Ma and C. J. Tsai, Biophys. Chem., 2014, 186, 22–30 CrossRef CAS PubMed
. - C. Colovos and T. O. Yeates, Verification of protein structures: patterns of nonbonded atomic interactions, Protein Sci., 1993, 2, 1511–1519 CrossRef CAS PubMed
.
|
This journal is © The Royal Society of Chemistry 2022 |
Click here to see how this site uses Cookies. View our privacy policy here.