DOI:
10.1039/D2RA03451D
(Paper)
RSC Adv., 2022,
12, 21452-21467
Molecular docking and dynamics based approach for the identification of kinase inhibitors targeting PI3Kα against non-small cell lung cancer: a computational study†
Received
3rd June 2022
, Accepted 23rd June 2022
First published on 3rd August 2022
Abstract
Non-small cell lung cancer (NSCLC) is an obscure disease whose incidence is increasing worldwide day by day, and PI3Kα is one of the major targets for cell proliferation due to the mutation. Since PI3K is a class of kinase enzyme, and no in silico research has been performed on the inhibition of PI3Kα mutation by small molecules, we have selected the protein kinase inhibitor database and performed the energy minimization process by ligand preparation. The key objective of this research is to identify the potential hits from the protein kinase inhibitor library and further to perform lead optimization by a molecular docking and dynamics approach. And so, the protein was selected (PDB ID: 4JPS), having a unique inhibitor and a specific binding pocket with amino acid residue for the inhibition of kinase activity. After the docking protocol validation, structure-based virtual screening by molecular docking and MMGBSA binding affinity calculations were performed and a total of ten hits were reported. Detailed analysis of the best scoring molecules was performed with ADMET analysis, induced fit docking (IFD) and molecular dynamics (MD) simulation. Two molecules – 6943 and 34100 – were considered lead molecules and showed better results than the PI3K inhibitor Copanlisib in the docking assessment, ADMET analysis, and molecular dynamics simulation. Furthermore, the synthetic accessibility of the two compounds – 6943 and 34100 – was investigated using SwissADME, and the two lead molecules are easier to synthesize than the PI3K inhibitor Copanlisib. Computational drug discovery tools were used for identification of kinase inhibitors as anti-cancer agents for NSCLC in the present research.
1 Introduction
The discovery of lead molecules by computerized searching of databases by targeting a specific protein is the trending method of drug discovery with the development of AI (artificial intelligence) and ML (machine learning).1 And, the preliminary study of drug discovery begins with the selection of the disease. Recent developments in pharmacology have led the way for a better understanding of the molecular basis of diseases at the cellular level.2 Hence, most pharmaceutical companies and university research initiatives start with appropriate target identification in the body by going through a detailed study of the signaling pathways, and the development of medication to interact with the target. The study of the structural and functional properties of the target along with the mechanism by which it interacts with drug molecules is important for this approach.3
Cancer is the appearance of aberrant cells which grow abnormally and also infect the neighboring cells of the body at any age and in both men and women.4 There are more than 200 types of cancer and the disease process begins at different locations and the causes are diverse.4 Among these, squamous cell carcinoma in non-small cell lung cancer (NSCLC) is caused by abnormalities in the PI3K/Akt pathway of cell proliferation.5 The primary symptoms begin with a persistent cough, coughing up blood, and breathing problems. Several treatments with chemotherapy and radiation therapy have been employed but due to the growth of resistance and intolerance of the adverse effects of chemotherapy and radiation therapy, there is a need for identification of enzyme inhibitors.4,5 Most cancers share a common signaling pathway of PI3K-Akt, EGFR, STAT, MAPK, etc. for the growth factor receptor.6
Hence, to inhibit squamous cell carcinoma in NSCLC, novel molecules are needed because mutation on the biological target results in the growth of drug resistance. Among various targets of the disease, phosphoinositide 3-kinases (PI3Ks), also known as phosphatidylinositol 3-kinases, are a family of enzymes involved in cellular functions such as cell growth, proliferation, and differentiation.7 There are four types of PI3K: class I, class II, class III, and class IV. They’re divided into groups depending on their primary sequence, management, and lipid substrate selectivity in in vitro studies.8,9 In multicellular creatures, PI3K signaling has remained constant over time. The PDGF receptor (PDGFR) and epidermal growth factor receptor (EGFR),10 both of which promote proliferation and invasion, the insulin-like growth factor receptor (IGFR), which fosters survival and reproduction, and the insulin receptor (INSR), which modulates metabolic homeostasis, all activate PI3K signaling in mammals.8 Any unwanted mutation in this biomarker can cause excessive proliferation of cells and leads to the activation of oncogenes. The oncogenes PIK3CA, PIK3CB, and PIK3CD, and the tumor suppressor gene PTEN are also involved. As most p110α mutations constitutively activate its kinase activity, PI3Kα appears to be an ideal target for drug development. Modification of the kinase enzyme begins with phosphorylation, which results in the functional dysregulation of the target protein PI3Ks, and changes enzyme activity.8,9
Numerous researchers already use computational approaches to investigate PI3K inhibitors, particularly from natural products, and for specific malignancies by targeting PI3Kα in previous studies;11–14 however, there is no research on the identification of kinase inhibitors against PI3Kα by structure-based virtual screening approaches and comparison with the PI3K pan-inhibitor Copanlisib. PI3Kα is a kinase enzyme, and so kinase inhibitors are chosen because they inhibit the auto-phosphorylation of the tyrosine residues of the protein. Hence, we have found a research gap, in that there has been no research performed by structure-based approaches on the identification of kinase inhibitors against PI3Kα by molecular docking and dynamics studies, compared with the standard PI3K pan-inhibitor Copanlisib for the treatment of NSCLC. A schematic representation of the biological target with its inhibition by the pan-inhibitor is shown in Fig. 1.8
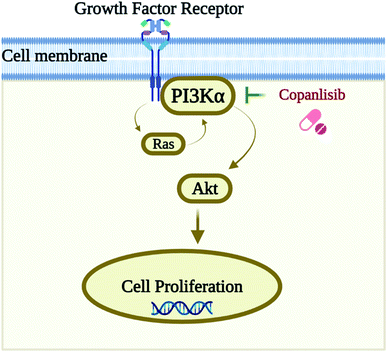 |
| Fig. 1 Schematic representation of the biological target PI3Kα and its inhibition by the pan-inhibitor Copanlisib. | |
In this research work, a specific biological target PI3Kα, which has a pan and a selective inhibitor, was selected and a kinase inhibitor database of 36
324 molecules from ChemDiv was chosen for the structure-based virtual screening to obtain the top ten hits, for further MMGBSA free binding energy analysis, ADMET predictions, IFD (induced fit docking) analysis and MD (molecular dynamics) simulations for the optimization of the kinase inhibitors for pan and selective inhibition of PI3Kα in the treatment of NSCLC squamous carcinoma.
2 Materials and methodology
The computational study was executed using the Maestro interface in the Schrodinger suite on an HP desktop system integrated with Ubuntu OS, along with an Intel® CORE® i3-5160 CPU and an integrated NVidia GPU. Fig. 2 depicts a schematic illustration of the in silico methodology.
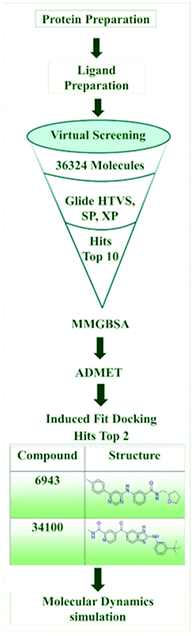 |
| Fig. 2 Schematic representation of the in silico methodology. | |
2.1. Protein preparation, receptor grid generation, and validation of docking protocol
An X-ray crystal structure of PI3Kα with a pan and selective isoform inhibitor has been selected having two macromolecular structures, phosphatidylinositol-4,5-bisphosphate 3-kinase catalytic subunit α which has single chain A of sequence length 1074 with two mutations in the PIK3CA gene (EC: 2.7.1.153, 2.7.11.1) and α regulatory subunit of phosphatidylinositol 3-kinase having single chain B with a sequence length of 293 without any mutations, and was downloaded from the RCSB PDB (Research Collaboratory for Structural Bioinformatics Protein Data Bank) having PDB code 4JPS with a resolution of 2.20 Å and an observed R-value of 0.206 which is in an acceptable range. A unique co-crystal ligand NVP-BYL719 is linked N + H with the protein with amino acid residue Val851.9
The selection of a particular protein from Uniprot (https://www.uniprot.org/uniprot/P42336) requires several filters, such as a resolution of 2.20 Å, the presence of a small molecule inhibitor (co-crystal ligand), the mutations in the PIK3CA gene and the most important requirement – the organism of the protein is Homo sapiens.
The process of energy minimization is a crucial step in the protein preparation where the net inter-atomic force becomes negligible. Hence, the minimization of the protein in molecular modeling provides the best structural conformation of the protein in the OPLS3e force field15 in Maestro.16
The approach for energy minimization of the protein begins after importing the protein by the protein preparation wizard.17 The Prime module18 was used for filling the missing chains and loops and the pH was 7.5 ± 0. Furthermore, with the deletion of the regulatory subunit from the protein, the refining process was implemented. And therefore, optimization of the protein using PROPKA pH 7.5 (ref. 19) and removal of water beyond 3 Å was implemented and minimization was performed using the force field. The minimized protein (PDB: 4JPS) was further processed for grid generation in the receptor grid generation panel, for representing the active binding pocket of the protein to the ligand for Glide docking.20,21 The grid generation in the receptor was executed by selecting the ligand from the prepared protein, so that the ligand was excluded from the calculation of grid generation, and also to exclude that ligand from ligand–receptor docking. Finally, the receptor grid generation was implemented with the default settings of the site, constraining rotatable groups, and excluding volume, by scaling the van der Waals radius, scaling factor 1.0, and cutting off partial charge. After that, the docking protocol was validated using the co-crystal ligand and protein complex, by calculating the RMSD (root mean square deviation) of the docked co-crystal ligand and energy minimized co-crystal ligand.
2.2. Ligand preparation
The protein kinase inhibitor library of 36
324 compounds was downloaded from the ChemDiv database (access date: 1 July 2022) and imported into Maestro for energy optimization and generation of accurate 3D structures using the LigPrep application.22 The most important characteristic features of LigPrep are the elimination of errors in ligands and the generation of optimized structures which can be further processed for Glide20,21 and phase screening as well as in molecular dynamics simulation. Further, the generation of 3D coordinates and energy minimization of 36
324 compounds of the kinase inhibitor library were executed in LigPrep by keeping the ionization state pH at 7.5 ± 0, defining desalt, single tautomer generation, and also retaining specified chiralities, using the Epik module.23 And finally, ligand preparation was executed using the OPLS3e force field.15
The standard drug Copanlisib, a PI3K pan-inhibitor, was selected and imported from Pubchem (https://pubchem.ncbi.nlm.nih.gov/compound/Copanlisib, access date: 1 July, 2022) and ligand optimization in similar settings to the kinase inhibitor library was implemented using the OPLS3e force field.15
2.3. Structure-based virtual screening by molecular docking
Glide (grid-based ligand docking with energetics)20,21 in the Maestro interface16 of the Schrödinger suite is an extremely effective method for identifying ligand hits and helps with lead optimization in structure-based virtual screening by molecular docking. Molecular docking is an approach where the receptor remains rigid and analyzes the behavior of small molecules in the binding pocket of the target protein and uncovers crucial biological processes. For the initial screening of 36
324 molecules, high throughput virtual screening (HTVS) at a rate of 2 seconds per compound was employed, and then the best 1000 molecules were selected for SP (standard precision) docking at a rate of 10 seconds per compound on the basis of docking score. Further, the best 30 molecules were executed for Glide XP (extra precision) docking.20,21 And finally, the top 10 molecules were selected for further analysis on the basis of docking score and molecular interactions with the target protein.
On the other hand, Glide XP docking20,21 of the standard drug Copanlisib was executed and compared with the results of the top ten hits.
2.4. MMGBSA
The MMGBSA (molecular mechanics generalized Born surface area) calculation method provided the relative binding free energy (ΔG bind) of each ligand molecule for determining ligand binding affinity with the receptor using the Prime module.18 The free binding energy calculation of the 10 best molecules (hits) was executed by keeping the solvation model VSGB 2.0 (ref. 24) and OPLS3e force field.15 For the calculation of binding affinity, the equation is as follows:
ΔG (binding affinity) = ΔG (solvation energy) + ΔE (minimized energy) + ΔG (surface area energies) |
ΔG (solvation energy) is the difference between the solvation energy of the GBSA of the PI3Kα–inhibitor complex, and the sum of the solvation energies for unligated PI3Kα and the respective inhibitor. ΔE (minimized energy) is the difference between the energy of the PI3Kα–inhibitor complex and the sum of the energies for unligated PI3Kα and the respective inhibitor. ΔG (surface area energies) is the difference between the surface area energy of the PI3Kα–inhibitor complex and the sum of the energies for unligated PI3Kα and the respective inhibitor.
The binding affinity and energies of optimized free receptors, free ligand, and the ligand–protein complex were calculated by the MMGBSA Prime module.18 The calculation of the strain energy of the ligand was executed by placing it in a solution that was auto-generated by the VSGB24 suite and the energy visualizer in Prime can present the energy visualization.
Binding affinity is free energy, and so it includes both entropy and enthalpy. There were specific limitations in the above equation, such as conformational entropy on ligand binding was neglected in the MM/GBSA calculations since they use the generalized Born approximation, which is an approximate and faster treatment of the Poisson–Boltzmann equation. Generalized Born (GB) is an approximate solution to the PB equation that is faster to compute than the original PB solution while maintaining a respectable level of accuracy compared to the original PB solution. The methodologies of MM/GBSA and MM/PBSA have been successfully applied in the estimation of free energies for binding of small molecules (drug candidates) with proteins, and hence, the limitations for accurate predictions are well known, although sufficient sampling is required for suitable convergence of free energy calculations, and the results strongly depend on the quality of the MM potential.25
2.5. Drug-likeness and ADMET predictions
The ADMET properties were analyzed using the QikProp module in Maestro. The drug-likeness property of the best ten molecules along with the standard PI3K pan-inhibitor Copanlisib was examined and drug-likeness could be analyzed on the basis of Lipinski’s rule of five.7 Qikprop helps to analyze the drug-likeness property along with other ADMET properties, such as molecular weight, hydrogen bond donation and acceptance, predicted octanol/water partition coefficient (QlogPo/w), polar surface area (PSA), and % human oral absorption. The other descriptors of ADMET analysis and their predictions, such as prediction of aqueous solubility, prediction of IC50 value for HERG K+ channel blockage, prediction of apparent Caco-2 cell permeability in nm s−1, etc. were also reported for the top ten hits compared with the PI3K standard Copanlisib.
2.6. Induced fit docking (IFD) analysis
The induced fit docking protocol26,27 predicts the effect of flexible ligand docking on protein structure, moving beyond the rigid ligand–receptor docking, which is typical in structure-based virtual screening. After the analysis of Glide XP docking,20,21 free binding energy calculations, and ADMET analysis, IFD26,27 was executed with the two best molecules selected compared with the standard with the protein – PI3Kα – by scaling the van der Waals energy to 0.50, and maximum pose generation, and refinement by Prime within 5 Å. IFD26,27 used Glide20,21 for the docking and the Prime module18 for refinement in the binding poses, which cannot resemble the biological condition although it provides information on the stability of the molecule in different poses and different frames at a particular binding pocket. In the present research, molecular dynamics simulation28 was executed for the two best molecules, along with the standard drug Copanlisib after IFD analysis,26,27 for a better understanding of the stability of the compounds in biological conditions.
2.7. Molecular dynamics (MD) simulation
The function and dynamics of protein–ligand complexes have long been studied using MD.28 Molecular docking does not perfectly mimic these events, unlike biological procedures that involve dissolving the protein and ligand in water. So, to further understand the stability of the two best detected leads, by comparing with the standard PI3K pan-inhibitor Copanlisib, the non-bonding interaction between the ligand and the protein using Desmond MD simulations28 was run for 100 ns for the protein – PI3Kα. Prior to completing the dynamics, the entire system was immersed in a simple point charge (SPC) solvent model.29 Throughout the system development process, the boundary condition was kept in its orthorhombic box shape. In the System Builder tool, the OPLS3e force field15 was used for preparation and further neutralized by the addition of 0.15 M NaCl to the buffer. Furthermore, the minimization tool was used for minimization. During the MD simulation, around 1000 frames were generated, with the recording interval (ps) for the trajectory set to 100 frames per second. In the MD process, the temperature (K) and pressure (bar) were both maintained at 300 K and 1.01325 bar, respectively, throughout the experiment. After that, the reports were generated with the help of the simulation interaction diagram (SID) tool incorporated in Desmond.28
2.8. Synthetic accessibility analysis
The synthetic accessibility analysis was performed using the SwissADME free online server, by drawing the two best leads in ChemDraw and importing them to SwissADME along with the standard drug Copanlisib. Further the synthetic accessibility score was reported for the two leads while comparing with Copanlisib. The synthetic accessibility (SA) score is an approximation; the SA score was generated as a mixture of two components: the fragment score was created to collect “historical synthetic knowledge” by examining common structural properties in a vast number of previously synthesised molecules, as previously indicated. It was given as 1 (very easy) to 10 (very difficult).30
3 Results and discussion
In the past few years, computer-aided lead identification using a structure-based approach has become a trend in the field of computational chemistry. Hit-to-lead optimization has become easier with the advancement of artificial intelligence (AI) as well as machine learning (ML).31 The virtual screening methodology has also provided several possibilities for drug discovery. Squamous cell carcinoma in NSCLC is a well-known disease to carry research forward, since it has several signaling pathways, genetic mutations, growth factors and space for drug development due to continuous growth of resistance.4 Hence, the initial priority of research is to find an appropriate target, and therefore, we have selected PI3Kα as a potential target by an elaborate study of cancer signaling pathways to inhibit the mutation factor for the proliferation of cells.
The most important characteristic of PI3Ks involves the proliferation of cells, and their motility, apoptosis and cell division. The two catalytic subunits of PI3Ks are class IA and class IB. There are three genes present in the class IA PI3K receptor, – PIK3CA, PIK3CB and PIK3CG, which are also known as p110α, p110β, and p110δ. p110α and p110β are present in most of the tissues, but p110δ is primarily present in white blood cells (WBC). In class IB, only one enzyme, PI3Kc, is present, which is encoded with the PIK3CG gene, also termed as p110c, mostly expressed in WBC. Hence, any mutation or dysregulation of the PI3K signaling pathway can induce cancers like squamous cell carcinoma as a result of kinase activity, and can be a possible cause of NSCLC. The only gene involved in mutation is PIK3CA, also termed as p110α mutation, – PI3Kα has emerged to be a promising therapeutic research target.32 Specifically, the protein was chosen, since it is involved in mutation and the growth of resistance due to squamous cell carcinoma in NSCLC. Various small molecules including PI3K pan-inhibitors and p110α isoform specific inhibitors exhibit anti-cancer activity against PI3Kα mutant squamous cell carcinoma in NSCLC, but in the current research, we are reporting a medicinal and computational chemistry approach for the structure based virtual screening, and hit to lead identification of kinase inhibitors as PI3Kα inhibitors.
3.1. Protein preparation, receptor grid generation and validation of docking protocol
The process of PI3Kα protein preparation was performed using Epik,23 ProtAssign,17 and Impref applications at pH 7.5 ± 0 in the protein preparation wizard17 of the Maestro interface.16 The protein preparation wizard17 helps to bring several tools and integrates the complex procedure of energy minimization into a simple workflow for researchers. Furthermore, the receptor grid generation was implemented in the binding pocket of the co-crystal ligand for further analysis in Glide20,21 based ligand docking using the receptor grid generation workflow mentioned in the materials and methodology.
Further validation of the docking protocol was performed before the structure-based virtual screening using XP docking of the co-crystal ligand using the Glide module20,21 for checking the resemblance between the lowest energy state of the co-crystal ligand predicted by Glide20,21 and the experimental binding mode of the X-ray crystallographic structure. The superimposition between the docked molecule pose and the experimental X-ray crystallographic structure pose is presented in Fig. 3 with an RMSD between the two poses of 1.1327 Å. Hence, the docking protocol was validated since the RMSD value is less than 2.0 Å, which was known from previous studies.
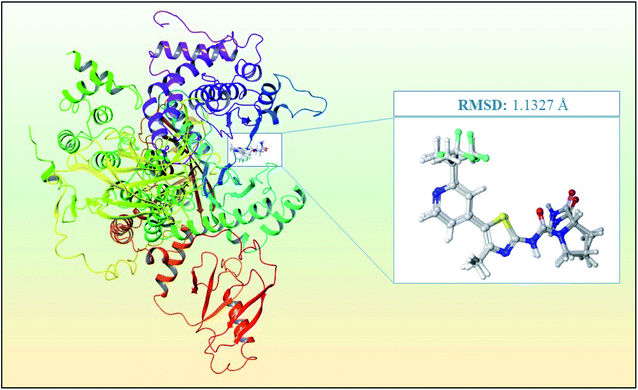 |
| Fig. 3 Superimposition of the docked co-crystal ligand and the co-crystal ligand with RMSD of 1.1327 Å for the PI3Kα inhibitor for the validation of the study. | |
3.2. Structure based virtual screening by molecular docking
Chemical library screening with a large number of compounds is time-consuming as well as expensive, considering both false positive and false negative rates. And therefore, ligand–receptor docking is the apparent computational technique of choice for virtual screening in the process of hit to lead identification. The main advantage of this methodology is that it is rapid and time-saving while requiring minimum investment. Furthermore, ligand preparation of 36
324 molecules of the protein kinase inhibitor library was performed using LigPrep at the same pH of 7.5, like protein preparation.
Structure-based virtual screening was performed for 36
324 molecules using Glide HTVS, and on the basis of docking score, the best 1000 molecules were selected for SP docking, and then the best 30 molecules were selected for XP docking for analysis of accurate binding interactions of the ligand with the receptor in the same grid generated with PDB 4JPS. A brief understanding of energy optimization and ligand–protein affinity could be acknowledged by the ligand docking study. HTVS and SP may provide false-positive results, so we have chosen XP docking, to get a more accurate outcome.
The top ten molecules were selected having compound ID 6943, 34100, 31140, 12500, 14178, 7165, 438, 6450, 19885, and 16021, and are described in Table 1 along with their structures, docking score and free binding energy MMGBSA ΔG score with the protein.
Table 1 The top ten hits from molecular docking along with their structure, docking score and MMGBSA ΔG
Compound |
Structure |
Docking score (kcal mol−1) |
MMGBSA ΔG (kcal mol−1) |
6943 |
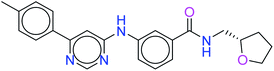 |
−11.973 |
−62.97 |
34100 |
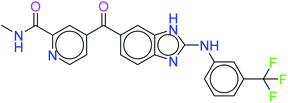 |
−11.312 |
−55.18 |
31140 |
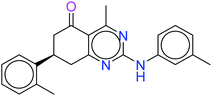 |
−11.079 |
−49.53 |
12500 |
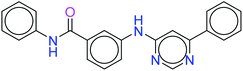 |
−11.060 |
−60.91 |
14178 |
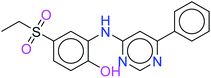 |
−10.822 |
−53.09 |
7165 |
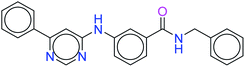 |
−10.927 |
−62.46 |
438 |
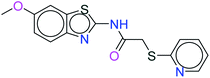 |
−10.846 |
−59.11 |
6450 |
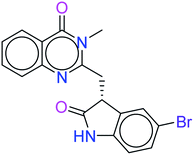 |
−10.830 |
−55.45 |
19885 |
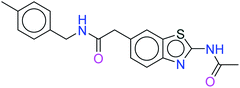 |
−10.823 |
−52.15 |
16021 |
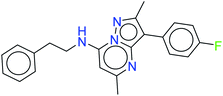 |
−10.522 |
−61.09 |
Copanlisib |
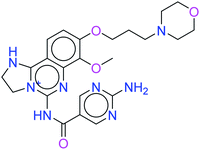 |
−3.941 |
−42.58 |
The best ten molecules show docking scores in the range of −11.973 to −10.522, although the standard PI3K pan-inhibitor Copanlisib shows a docking score of −3.941. And, the MMGBSA binding affinity value ranges between −62.97 and −49.53 kcal mol−1, and the value for the standard drug is −42.58 kcal mol−1, which is comparatively lower than that of the hit molecules. The top 10 molecules expressed significant interactions, compared with all molecules present in the PI3Kα drug database as well as with the PI3K pan-inhibitor Copanlisib.
The molecules of protein kinase inhibitors – compound ID 6943, 34100, 31140, 12500, 14178, 7165, 438, 6450, 19885, and 16021 – expressed significant interactions, comparing all molecules present in the kinase inhibitor database, in the specific binding pocket of PI3Kα (PDB ID: 4JPS). After interpreting the grid based extra precision docking of the top 10 molecules, it can be summarized that amino acid residue Val851 played the most important role in the hydrogen bonding interaction, followed by Ser854 and subsequently Asn853 and Asp933.
Hydrophobic interactions were shown by the Val850, Val851, Tyr836, Ile848, Phe930, Ile932, Trp780, Ile800, Pro778, Met922, and Met772 amino acid residues, and π–π stacking was observed with Tyr836 and subsequently Trp780. Various ligands show π–π stacking interactions with Tyr836 and Trp780, although only one ligand (compound ID: 34100) shows a π–cation interaction with Lys802. The positive charge and negative charge interactions with Lys802 and Arg852, and Glu849 and Asp933 respectively also played a dynamic role in the particular interaction (Table 2).
Table 2 2D interaction diagram of the best ten hits along with their interactions with the protein PI3Kα (PDB ID: 4JPS) compared with the standard drug Copanlisib
Compound |
2D interaction diagram |
Interactions |
6943 |
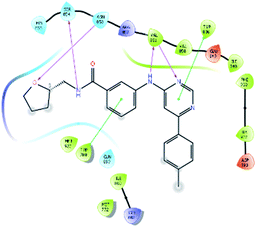 |
H bond: Val851(2), Ser854, Asn853 |
Hydrophobic: Val850, Val851, Tyr836, Ile848, Phe930, Ile932, Met922, Trp780, Ile800, Met772 |
π–π stacking: Tyr836, Trp780 |
Polar: His855, Ser854, Asn853, Gln859 |
Charged (+ve): Lys802, Arg852 |
Charged (−ve): Glu849 |
34100 |
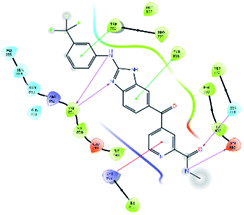 |
H bond: Val851(2), Ser774, Asp933 |
Hydrophobic: Val850, Val851, Tyr836, Ile848, Phe930, Ile932, Met922, Trp780, Ile800, Pro778 Met772 |
π–π stacking: Tyr836, Trp780 |
π–cation: Lys802 |
Polar: His855, Ser854, Asn853, Gln859, Ser774 |
Charged (+ve): Lys802, Arg852 |
Charged (−ve): Glu849, Asp933 |
31140 |
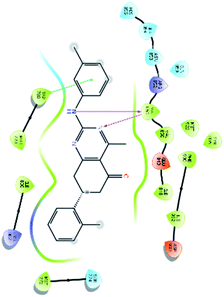 |
H bond: Val851(2) |
Hydrophobic: Val850, Val851, Tyr836, Ile848, Phe930, Ile932, Trp780, Ile800, Pro778, Met922, Met772 |
π–π stacking: Tyr836 |
Polar: His855, Ser854, Asn853, Gln859, Ser774 |
Charged (+ve): Lys802, Arg852 |
Charged (−ve): Glu849, Asp933 |
12500 |
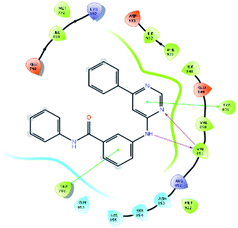 |
H bond: Val851(2) |
Hydrophobic: Val850, Val851, Tyr836, Ile848, Phe930, Ile932, Met922, Trp780, Ile800, Met772 |
π–π stacking: Tyr836, Trp780 |
Polar: His855, Ser854, Asn853, Gln859 |
Charged (+ve): Lys802, Arg852 |
Charged (−ve): Glu849, Asp933, Glu798 |
14178 |
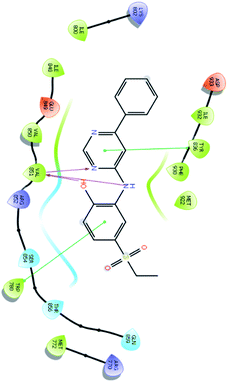 |
H bond: Val851(3) |
Hydrophobic: Val850, Val851, Tyr836, Ile848, Phe930, Ile932, Met922, Trp780, Ile800, Met772 |
π–π stacking: Tyr836, Trp780 |
Polar: Ser854, Thr856, Gln859 |
Charged (+ve): Lys802, Arg852, Arg770 |
Charged (−ve): Glu849, Asp933 |
7165 |
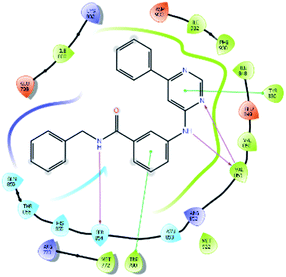 |
H bond: Val851(2), Ser854 |
Hydrophobic: Val850, Val851, Tyr836, Ile848, Phe930, Ile932, Met922, Trp780, Ile800, Met772 |
π–π stacking: Tyr836, Trp780 |
Polar: His855, Ser854, Asn853, Gln859, Thr856 |
Charged (+ve): Lys802, Arg852, Arg770 |
Charged (−ve): Glu849, Asp933, Glu798 |
438 |
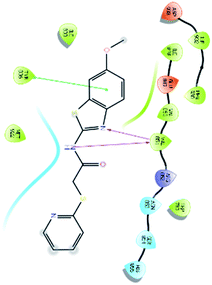 |
H bond: Val851(2) |
Hydrophobic: Val850, Val851, Tyr836, Ile848, Phe930, Ile932, Met922, Trp780, Ile800 |
π–π stacking: Tyr836 |
Polar: His855, Ser854, Asn853 |
Charged (+ve): Arg852 |
Charged (−ve): Glu849, Asp933 |
6450 |
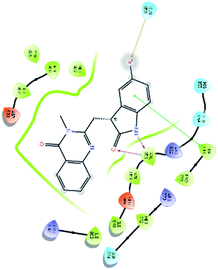 |
H bond: Val851(2) |
Halogen bond: Gln859 |
Hydrophobic: Val850, Val851, Tyr836, Ile848, Phe930, Ile932, Trp780, Ile800, Pro778, Met922, Met772 |
π–π stacking: Trp780 |
Polar: His855, Ser854, Gln859, Ser774 |
Charged (+ve): Lys802, Arg852, Arg770 |
Charged (−ve): Glu849, Asp933 |
19885 |
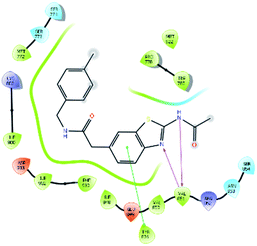 |
H bond: Val851(2) |
Hydrophobic: Val850, Val851, Tyr836, Ile848, Phe930, Ile932, Trp780, Ile800, Pro778, Met922, Met772 |
π–π stacking: Tyr836 |
Polar: Ser854, Asn853 Ser773, Ser774 |
Charged (+ve): Lys802, Arg852 |
Charged (−ve): Glu849, Asp933 |
16021 |
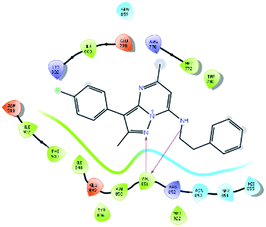 |
H bond: Val851(2) |
Hydrophobic: Val850, Val851, Tyr836, Ile848, Phe930, Ile932, Trp780, Ile800, Met922, Met772 |
Polar: His855, Ser854, Asn853, Gln859 |
Charged (+ve): Lys802, Arg770, Arg852 |
Charged (−ve): Glu798, Glu849, Asp933 |
Copanlisib |
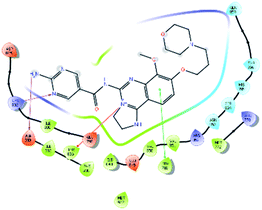 |
H bond: Lys802, Asp933 |
Hydrophobic: Val850, Val851, Tyr836, Ile848, Phe930, Ile932, Trp780, Ile800, Met922, Met772 |
π–π stacking: Trp780 |
π–cation: Tyr836 |
Polar: His855, Ser854, Asn853, Gln859, Thr856 |
Charged (+ve): Lys802, Arg770, Arg852 |
Charged (−ve): Glu798, Glu849, Asp933 |
On the other hand, Copanlisib is a standard marketed drug, and shows non-bonding H-bond interactions with Lys802 and Asp933, but not with Val851, which is the key amino acid for PI3Kα inhibition. Hydrophobic interactions with Val850, Val851, Tyr836, Ile848, Phe930, Ile932, Trp780, Ile800, Met922, and Met772, π–π stacking with Trp780, π–cation interaction with Tyr836, polar interactions with His855, Ser854, Asn853, Gln859, and Thr856, and the positive and negative charge interactions are indistinguishable (Table 2). Hence, it can be observed that the top 10 hit molecules may be effective in the treatment of NSCLC by inhibiting the PI3Kα activity. The 3D interactions of the top ten hits are provided in the ESI.†
3.3. Drug likeness predictions and ADMET analysis
The top 10 molecules were selected on the basis of docking score, ligand receptor interactions and MMGBSA binding affinity compared with Copanlisib as the standard drug and a PI3K pan-inhibitor. The ADMET analysis was performed using the QikProp application of Maestro. First, drug likeness properties and rule of five violation were analyzed and are reported in Table 3, and compared with the standard.
Table 3 Evaluation of the ADMET properties of the chosen compounds
Compound |
Molecular weight |
Hydrogen bond donor |
Hydrogen bond acceptor |
PSAa |
Percent human oral absorption |
Rule of five |
PSA: polar surface area. |
6943 |
388.468 |
2 |
6.7 |
76.14 |
100 |
0 |
34100 |
439.396 |
3 |
7.5 |
99.77 |
89.978 |
0 |
31140 |
357.454 |
1 |
4.5 |
54.88 |
100 |
0 |
12500 |
366.421 |
2 |
5 |
66.91 |
100 |
0 |
7165 |
380.448 |
2 |
5 |
66.91 |
100 |
0 |
438 |
331.407 |
1 |
5.75 |
117.65 |
100 |
0 |
6450 |
384.231 |
1 |
6.5 |
61.77 |
94.382 |
0 |
19885 |
353.438 |
2 |
6.5 |
99.33 |
86.122 |
0 |
14178 |
355.411 |
2 |
7.25 |
100.56 |
80.998 |
0 |
16021 |
360.433 |
1 |
2.5 |
42.22 |
100 |
1 |
Copanlisib |
480.525 |
6.5 |
8 |
76.14 |
43.555 |
2 |
Furthermore, the values of other ADMET descriptors are reported in Table 4. It was finally observed that the best two molecules – 6943 and 34100 – of the ten hits showed the best results. Although all ten molecules showed better results than the standard drugs.
Table 4 Evaluation of other ADMET properties of the selected moleculesa
Compound |
QPlogPo/w |
QPlogS |
QPlogHERG |
QPlogBB |
QPPCaco |
QPPMDCK |
QPlogKhsa |
QPlogS: predicted aqueous solubility, QPlogHERG: predicted IC50 value for blockage of HERG K+ channels, QPPCaco: predicted apparent Caco-2 cell permeability in nm s−1, QPlogBB: predicted brain/blood partition coefficient, QPPMDCK: predicted apparent MDCK cell permeability in nm s−1, QPlogKhsa: prediction of binding to human serum albumin. |
6943 |
4.164 |
−6.312 |
−6.915 |
−0.866 |
1246.697 |
627.851 |
0.499 |
34100 |
3.555 |
−6.622 |
−6.992 |
−1.402 |
228.465 |
442.638 |
0.325 |
31140 |
4.784 |
−6.628 |
−6.229 |
−0.288 |
2102.976 |
1104.831 |
0.903 |
12500 |
4.597 |
−6.19 |
−7.915 |
−0.738 |
1326.693 |
671.507 |
0.617 |
7165 |
4.874 |
−6.431 |
−7.848 |
−0.851 |
1210.115 |
607.961 |
0.715 |
438 |
3.125 |
−4.621 |
−6.077 |
−0.537 |
1209.78 |
1544.245 |
0.028 |
6450 |
2.719 |
−4.39 |
−5.248 |
−0.451 |
755.857 |
968.803 |
0.032 |
19885 |
2.48 |
−4.137 |
−4.495 |
−1.01 |
312.473 |
415.155 |
−0.101 |
14178 |
2.001 |
−3.451 |
−5.454 |
−1.36 |
231.929 |
101.947 |
−0.134 |
16021 |
6.093 |
−7.438 |
−6.671 |
0.047 |
4437.132 |
4471.604 |
1.219 |
Copanlisib |
0.586 |
−3.692 |
−6.175 |
−0.523 |
152.836 |
71.858 |
−0.263 |
The two kinase inhibitors – compound IDs 6943 and 34100 – were selected according to the results of docking score and interactions, MMGBSA free energy calculation and ADMET analysis. Further in silico investigation was done by IFD and molecular dynamics simulation.
3.4. Induced fit docking analysis
The induced fit docking (IFD) protocol26,27 was based on Glide docking20,21 and Prime18 is used as the refinement module in the Maestro interface of Schrodinger. It helps in accurate prediction of ligand binding mode in the receptor binding pocket, as well as the concomitant structural changes. It almost generates an accurate binding mode similar to the biological ligand receptor binding, by eradicating false negative bonds. IFD is used to understand the stability of the complex in the particular binding pocket of the receptor to undergo a specific inhibitory effect.
In the present study, IFD was performed for the two best molecules, which are the lead compounds, according to the molecular docking, MMGBSA analysis, and ADMET predictions targeting PI3Kα (PDB ID: 4JPS) and a comparison was made with PI3K pan-inhibitor Copanlisib.
After analysis of IFD interactions, it was observed that compound 6943 had a strong hydrogen bonding interaction with Val851 and an IFD score of −2252.79, which showed maximum inhibitory effect, greater than that of compound 34100 showing a hydrogen bonding interaction with Ser854 and an IFD score of −2245.53, and both compounds showed better interaction and IFD score than the standard PI3K pan inhibitor Copanlisib, showing a hydrogen bonding interaction with Trp780 and an IFD score of −2244.06. The IFD score and 3D interactions are provided in Fig. 4.
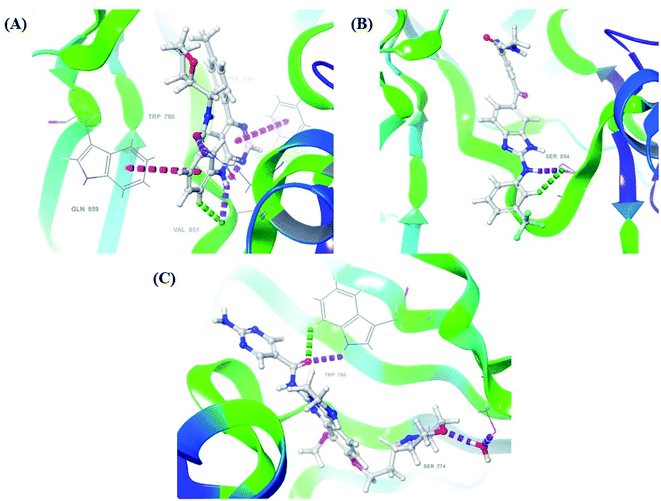 |
| Fig. 4 3D interaction diagram of induced fit docking of (A) 6943 [IFD score: −2252.79], (B) 34100 [IFD score: −2245.53] and (C) Copanlisib [IFD score: −2244.06]. | |
3.5. Molecular dynamics (MD) simulation
An MD simulation study was executed to validate the stability of the receptor–ligand complex, predicted binding mode, and the types of potential interactions, which were studied previously by Glide XP docking.20,21 The primary reason behind using MD simulation is that it can accurately simulate actual biological situations. Although the flexible docking study can be performed by induced fit docking (IFD),26,27 it was unable to mimic the biological conditions. The simulation was carried out using the Desmond28 application in the Maestro interface, as it executes explicit solvent simulation with periodic boundary conditions in a orthorhombic simulation box with a high-resolution dynamic structure of the protein in a water-solvated model similar to the biological system.33 The information on structural changes in the form of conformations and ligand–protein interactions was sufficient. Further MD simulation was performed for the top two ligands and the standard drug for comparison targeting the PI3Kα protein simultaneously.
In the present research, MD simulation was performed for the two best leads – 6943 and 34100 – and compared with the standard Copanlisib–PI3Kα complex. After analysing the data from the simulation interaction diagram, it was observed that the RMSD of the 6943–PI3Kα complex shows fluctuations of 1.2–1.6 Å, which is very stable and in the acceptable range (1–4 Å) in the specific binding pocket of PI3Kα, and the maximum protein–ligand contacts are hydrogen bonding interactions with Val851, Asn853 and Ser854 which are crucial for the inhibitory activity (Fig. 5). Similarly, after the analysis of the 34100–PI3Kα complex, it was observed that the RMSD fluctuation is between 2 and 3 Å [Fig. 6(B)], which is in the acceptable range, but less stable than the 6943–PI3Kα complex, and more stable than the standard Copanlisib–PI3Kα complex with an RMSD of 2–4 Å [Fig. 7(B)].
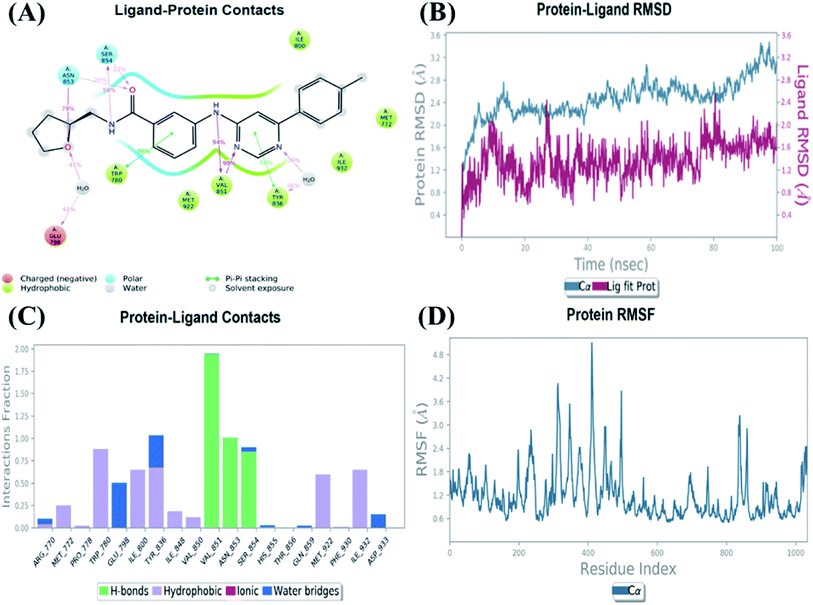 |
| Fig. 5 MD simulation for the 6943–PI3Kα complex (PDB ID: 4JPS), (A) interaction diagram of PI3Kα–6943 after MD simulation, (B) the RMSD plot of the PI3Kα–6943 complex, (C) histogram of the PI3Kα–6943 complex, (D) RMSF of the PI3Kα–6943 complex. | |
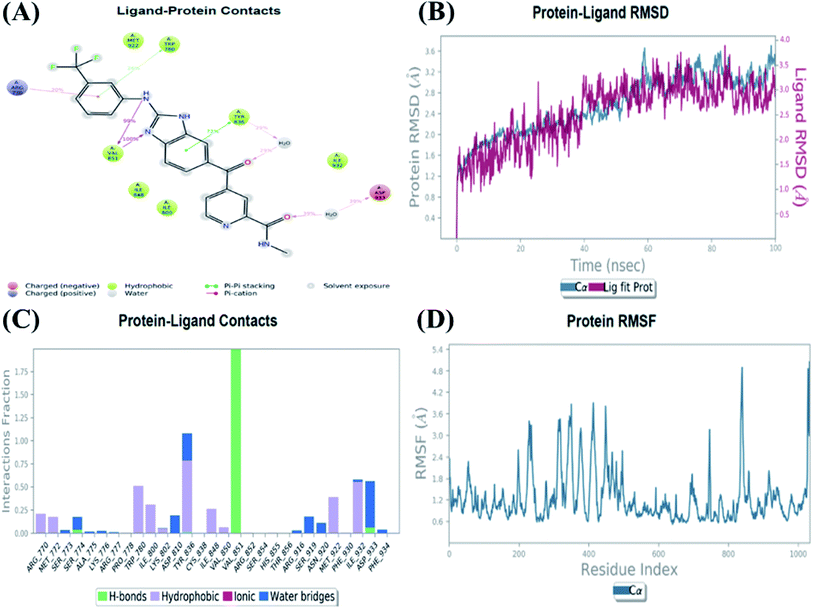 |
| Fig. 6 MD simulation for the 34100–PI3Kα complex (PDB ID: 4JPS), (A) interaction diagram of PI3Kα–34100 after MD simulation, (B) the RMSD plot of the PI3Kα–34100 complex, (C) histogram of the PI3Kα–34100 complex, (D) RMSF of the PI3Kα–34100 complex. | |
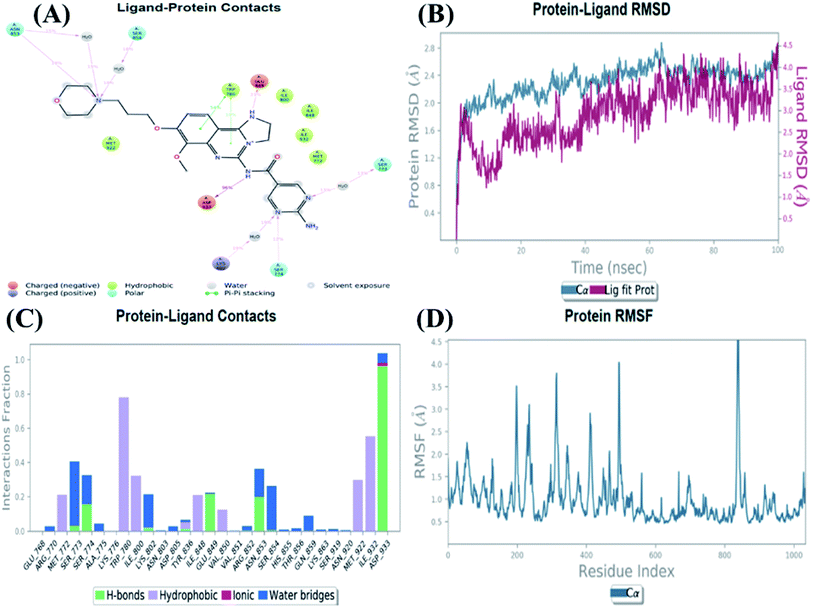 |
| Fig. 7 MD simulation for the Copanlisib–PI3Kα complex (PDB ID: 4JPS), (A) interaction diagram of PI3Kα–Copanlisib after MD simulation, (B) the RMSD plot of the PI3Kα–Copanlisib complex, (C) histogram of the PI3Kα–Copanlisib complex, (D) RMSF of the PI3Kα–Copanlisib complex. | |
The compound 34100–PI3Kα complex shows a hydrogen bonding interaction with Val851 only [Fig. 6(C)], although this is better than the standard Copanlisib–PI3Kα complex which has a hydrogen bonding interaction with Asp933 [Fig. 7(C)].
The Root Mean Square Fluctuations (RMSF) help in characterizing local changes in the protein. These fluctuations were used for determining the residue present that contributes to structural fluctuations in the complex. The fewer the fluctuations, the better the stability, and so, by comparing Fig. 5(D) with Fig. 6(D) and 7(D), it was observed that Fig. 5(D) for the 6943–PI3Kα complex shows fewer fluctuations than Fig. 6(D) for the 34100–PI3Kα complex, and both complexes show fewer fluctuations than Fig. 7(D) for the Copanlisib–PI3Kα complex.
Hence, from the MD simulation it was proved that ligand 6943 shows the best stability with PI3Kα inhibitory activity, compared to the other molecule 34100; although both molecules are more stable and potent inhibitors of PI3Kα than the standard pan-inhibitor Copanlisib.
3.6. Synthetic accessibility (SA) analysis
SA evaluation is a method for determining the ease with which substances can be synthesized.34 A quick method for assessing synthetic accessibility for a large number of chemical compounds is likely to be a new approach for drug discovery, based on 1024 fragmental contributions (FP2) modulated by size and complexity penalties, trained on 12
782
590 molecules and tested on 40 external molecules (r2 = 0.94).35
The two leads after the structure-based virtual screening, ADMET analysis and MD simulation, underwent SwissADME synthetic accessibility score prediction, reported in Table 5. It was observed that the lead compounds – 6943 and 34100 – have SA scores of 3.44 and 2.84 which are lower than that of the standard PI3K pan-inhibitor Copanlisib of 3.84. All the molecules exhibit easy to moderate difficulty for synthesis. Hence, it may be predicted that the lead kinase inhibitors – 6943 and 34100 – are easier to synthesize than the standard PI3K pan-inhibitor Copanlisib as reported in Table 5.
Table 5 Synthetic accessibility of the top two kinase inhibitors, compared with the PI3K pan-inhibitor Copanlisib
Compound |
Structure |
SA score |
6943 |
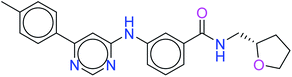 |
3.44 |
34100 |
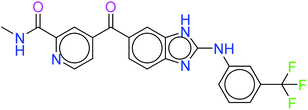 |
2.88 |
Copanlisib |
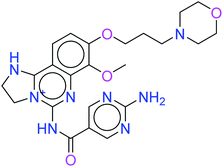 |
3.84 |
4 Conclusion
Mutation in PI3Kα is a continuous process in squamous cell carcinoma in NSCLC. And so, to inhibit the growth and cell proliferation abnormalities, small molecules are needed for targeted therapy. PI3K is a kind of kinase enzyme which readily mutates in squamous cell carcinoma in NSCLC, and therefore, the kinase inhibitor library from ChemDiv was chosen for the virtual screening. The selection of a protein from the Uniprot database was a difficult procedure, and in the present research, a mutated protein of PI3Kα (PDB ID: 4JPS) was first taken and the docking protocol was validated successfully with the co-crystal ligand. After the structure based virtual screening protocol of 36
324 molecules from the kinase inhibitor library, only 10 molecules were chosen as promising hits after going through HTVS, SP and XP docking, and compared with the PI3K pan-inhibitor Copanlisib. Hit to lead optimization was performed using filters like MMGBSA, drug-likeness and ADMET analysis, and IFD. The two best molecules, compound IDs 6943 and 34100, showed promising interaction with the amino acid residue Val851, which produces a better inhibitory effect on the PI3Kα of squamous cell carcinoma in NSCLC than the PI3K pan-inhibitor Copanlisib. In the IFD, the two molecules – 6943 and 34100 – also showed better interactions and binding poses than the standard drug. Furthermore, in the molecular dynamics simulation, the stability of the two lead molecules 6943 and 34100 showed more promising results, in the ligand protein interactions as well as in the RMSD and RMSF, than the standard PI3K pan-inhibitor. Furthermore, the synthetic accessibility of the lead molecules was examined and compared with that of the standard drug Copanlisib to understand the difficulty of the synthetic approach in medicinal chemistry. Thus, the current study demonstrates the association between PI3Kα inhibition in NSCLC and the in silico approach for optimising the best two molecules. Further in vitro and in vivo assays of these molecules can be performed, and these molecules may act as potential anticancer agents in the therapy of NSCLC.
Abbreviations
Arg | Arginine |
Asn | Asparagine |
Asp | Aspartic acid |
AI | Artificial intelligence |
EGFR | Epidermal growth factor receptor |
Glide | Grid-based ligand docking with energetics |
Gln | Glutamate |
Glu | Glutamate |
His | Histidine |
HTVS | High throughput virtual screening |
IGFR | Insulin-like growth factor receptor |
INSR | Insulin receptor |
IFD | Induced fit docking |
Ile | Isoleucine |
Lys | Lysine |
MAPK | Mitogen-activated protein kinase |
MD | Molecular dynamics |
ML | Machine learning |
Met | Methionine |
MMGBSA | Molecular mechanics generalized Born surface area |
NSCLC | Non-small cell lung cancer |
OPLS | Optimized potentials for liquid simulations |
PI3K | Phosphatidylinositol 3-kinase |
Phe | Phenylalanine |
PDGFR | Platelet-derived growth factor receptor |
PTEN | Phosphatase and tensin homolog |
Pro | Proline |
Ras | Rat sarcoma virus |
RCSB PDB | Research Collaboratory for Structural Bioinformatics Protein Data Bank |
RMSD | Root mean square deviation |
Ser | Serine |
STAT | Signal transducers and activators of transcription |
SP | Standard precision |
SPC | Simple point charge |
SID | Simulation interaction diagram |
SA | Synthetic accessibility |
Thr | Threonine |
Trp | Tryptophan |
Tyr | Tyrosine |
VSGB | Surface generalized Born model and variable dielectric |
Val | Valine |
WBC | White blood cell |
XP | Extra precision |
Conflicts of interest
The authors declare no conflict of interest in this article.
Acknowledgements
Author Subham Das is grateful to the Manipal Academy of Higher Education, Manipal, for the Dr TMA Pai Doctoral Fellowship. Furthermore, the authors express gratitude to the Manipal-Schrödinger Centre for Molecular Simulations. The authors would like to thank the Manipal College of Pharmaceutical Sciences for providing the necessary resources for this study. The authors also acknowledge ChemDraw and https://BioRender.com.
References
- D. Paul, G. Sanap, S. Shenoy, D. Kalyane, K. Kalia and R. K. Tekade, Drug Discovery Today, 2021, 26, 80–93 CrossRef CAS PubMed.
- S. Dotolo, C. Cervellera, M. Russo, G. L. Russo and A. Facchiano, Molecules, 2021, 46, 1–13 Search PubMed.
- A. Talevi, Front. Pharmacol., 2015, 6, 1–7 Search PubMed.
- S. Das, S. Roy, S. B. Rahaman, S. Akbar, B. Ahmed, D. Halder, A. Kunnath Ramachandran and A. Joseph, Curr. Med. Chem., 2022 DOI:10.2174/0929867329666220509112423.
- A. C. Tan, Thorac. Cancer, 2020, 11, 511–518 CrossRef PubMed.
- H. Y. K. Yip and A. Papa, Cells, 2021, 10, 659 CrossRef CAS PubMed.
- K. Haider, K. Ahmad, A. K. Najmi, S. Das, A. Joseph and M. Shahar Yar, Arch. Pharm., 2022 DOI:10.1002/ardp.202200146.
- H. Cheng, S. T. M. Orr, S. Bailey, A. Brooun, P. Chen, J. G. Deal, Y. L. Deng, M. P. Edwards, G. M. Gallego, N. Grodsky, B. Huang, M. Jalaie, S. Kaiser, R. S. Kania, S. E. Kephart, J. Lafontaine, M. A. Ornelas, M. Pairish, S. Planken, H. Shen, S. Sutton, L. Zehnder, C. D. Almaden, S. Bagrodia, M. D. Falk, H. J. Gukasyan, C. Ho, X. Kang, R. E. Kosa, L. Liu, M. E. Spilker, S. Timofeevski, R. Visswanathan, Z. Wang, F. Meng, S. Ren, L. Shao, F. Xu and J. C. Kath, J. Med. Chem., 2021, 64, 644–661 CrossRef CAS PubMed.
- P. Furet, V. Guagnano, R. A. Fairhurst, P. Imbach-Weese, I. Bruce, M. Knapp, C. Fritsch, F. Blasco, J. Blanz, R. Aichholz, J. Hamon, D. Fabbro and G. Caravatti, Bioorg. Med. Chem. Lett., 2013, 23, 3741–3748 CrossRef CAS PubMed.
- K. Haider, S. Das, A. Joseph and M. S. Yar, Drug Dev. Res., 2022, 83, 859–890, DOI:10.1002/ddr.21925.
- G. Madhukar and N. Subbarao, J. Biomol. Struct. Dyn., 2022, 40, 4697–4712, DOI:10.1080/07391102.2020.1861980.
- S. Srivastava, A. Vengamthodi, I. Singh, B. S. Choudhary, M. Sharma and R. Malik, Struct. Chem., 2019, 30, 1761–1778 CrossRef CAS.
- K. Mohankumar, S. Pajaniradje, S. Sridharan, V. K. Singh, L. Ronsard, A. C. Banerjea, C. S. Benson, M. S. Coumar and R. Rajagopalan, Chem.-Biol. Interact., 2014, 210, 51–63 CrossRef CAS PubMed.
- S. Reddy Eda, Bioinformation, 2019, 15, 709–715 CrossRef PubMed.
- K. Roos, C. Wu, W. Damm, M. Reboul, J. M. Stevenson, C. Lu, M. K. Dahlgren, S. Mondal, W. Chen, L. Wang, R. Abel, R. A. Friesner and E. D. Harder, J. Chem. Theory Comput., 2019, 15, 1863–1874 CrossRef CAS PubMed.
- Maestro User Manual, Schrödinger Press, 2015, pp. 1–337 Search PubMed.
- G. Madhavi Sastry, M. Adzhigirey, T. Day, R. Annabhimoju and W. Sherman, J. Comput.-Aided Mol. Des., 2013, 27, 221–234 CrossRef CAS PubMed.
- Prime User Manual, Schrödinger Press, 2015, pp. 1–133 Search PubMed.
- M. H. M. Olsson, C. R. SØndergaard, M. Rostkowski and J. H. Jensen, J. Chem. Theory Comput., 2011, 7, 525–537 CrossRef CAS PubMed.
- T. A. Halgren, R. B. Murphy, R. A. Friesner, H. S. Beard, L. L. Frye, W. T. Pollard and J. L. Banks, J. Med. Chem., 2004, 47, 1750–1759 CrossRef CAS PubMed.
- R. A. Friesner, J. L. Banks, R. B. Murphy, T. A. Halgren, J. J. Klicic, D. T. Mainz, M. P. Repasky, E. H. Knoll, M. Shelley, J. K. Perry, D. E. Shaw, P. Francis and P. S. Shenkin, J. Med. Chem., 2004, 47, 1739–1749 CrossRef CAS PubMed.
- LigPrep User Manual, Schrödinger Press, 2015, pp. 1–79 Search PubMed.
- J. C. Shelley, A. Cholleti, L. L. Frye, J. R. Greenwood, M. R. Timlin and M. Uchimaya, J. Comput.-Aided Mol. Des., 2007, 21, 681–691 CrossRef CAS PubMed.
- J. Li, R. Abel, K. Zhu, Y. Cao, S. Zhao and R. A. Friesner, Proteins: Struct., Funct., Bioinf., 2011, 79, 2794–2812 CrossRef CAS PubMed.
- E. Wang, H. Sun, J. Wang, Z. Wang, H. Liu, J. Z. H. Zhang and T. Hou, Chem. Rev., 2019, 119, 9478–9508 CrossRef CAS PubMed.
- E. B. Miller, R. B. Murphy, D. Sindhikara, K. W. Borrelli, M. J. Grisewood, F. Ranalli, S. L. Dixon, S. Jerome, N. A. Boyles, T. Day, P. Ghanakota, S. Mondal, S. B. Rafi, D. M. Troast, R. Abel and R. A. Friesner, J. Chem. Theory Comput., 2021, 17, 2630–2639 CrossRef CAS PubMed.
- W. Sherman, T. Day, M. P. Jacobson, R. A. Friesner and R. Farid, J. Med. Chem., 2006, 49, 534–553 CrossRef CAS PubMed.
- K. J. Bowers, D. E. Chow, H. Xu, R. O. Dror, M. P. Eastwood, B. A. Gregersen, J. L. Klepeis, I. Kolossvary, M. A. Moraes, F. D. Sacerdoti, J. K. Salmon, Y. Shan and D. E. Shaw, in ACM/IEEE SC 2006 Conference (SC’06), IEEE, 2006, pp. 43–43 Search PubMed.
- H. J. C. Berendsen, J. P. M. Postma, W. F. van Gunsteren and J. Hermans, in Intermolecular Forces, ed. B. Pullman, D. Reidel Publishing Company, 1981, vol. 3, pp. 331–342 Search PubMed.
- P. Ertl and A. Schuffenhauer, J. Cheminf., 2009, 1, 8 Search PubMed.
- K. A. Carpenter and X. Huang, Curr. Pharm. Des., 2018, 24, 3347–3358 CrossRef CAS PubMed.
- T. P. Heffron, R. A. Heald, C. Ndubaku, B. Wei, M. Augistin, S. Do, K. Edgar, C. Eigenbrot, L. Friedman, E. Gancia, P. S. Jackson, G. Jones, A. Kolesnikov, L. B. Lee, J. D. Lesnick, C. Lewis, N. McLean, M. Mörtl, J. Nonomiya, J. Pang, S. Price, W. W. Prior, L. Salphati, S. Sideris, S. T. Staben, S. Steinbacher, V. Tsui, J. Wallin, D. Sampath and A. G. Olivero, J. Med. Chem., 2016, 59, 985–1002 CrossRef CAS PubMed.
- S. Akbar, S. Das, A. Iqubal and B. Ahmed, J. Biomol. Struct. Dyn., 2021, 22, 1–18 CrossRef PubMed.
- G. Xiong, Z. Wu, J. Yi, L. Fu, Z. Yang, C. Hsieh, M. Yin, X. Zeng, C. Wu, A. Lu, X. Chen, T. Hou and D. Cao, Nucleic Acids Res., 2021, 49, W5–W14 CrossRef CAS PubMed.
- A. Daina, O. Michielin and V. Zoete, Sci. Rep., 2017, 7, 1–13 CrossRef PubMed.
|
This journal is © The Royal Society of Chemistry 2022 |
Click here to see how this site uses Cookies. View our privacy policy here.