DOI:
10.1039/D2RA03782C
(Paper)
RSC Adv., 2022,
12, 25269-25278
Optimization of uric acid detection with Au nanorod-decorated graphene oxide (GO/AuNR) using response surface methodology
Received
20th June 2022
, Accepted 23rd August 2022
First published on 7th September 2022
Abstract
A modified glassy carbon electrode (GCE) was developed based on a synthesized graphene oxide (GO) gold nanorod (AuNR) decorated composite (GO/AuNR) for sensitive electrochemical sensing of uric acid (UA). The electrochemical performance of GO/AuNR/GCE for UA detection was investigated employing the differential pulse voltammetry (DPV) technique. Central composite design (CCD) was applied to obtain the optimum composition of the GO and AuNR composite, which provide the highest possible UA oxidation peak current. The optimum composition was obtained at a GO concentration of 5 mg mL−1 and AuNR volume of 10 mL. Under the optimum conditions, GO/AuNR/GCE showed acceptable analytical performance for UA detection with good linearity (concentration range of 10–90 μM) and both a low detection limit (0.4 μM) and quantitation limit (1.0 μM). Furthermore, the proposed sensor exhibits superior stability, reproducibility, and selectivity using ascorbic acid (AA), dopamine (DA), urea, glucose, and magnesium as interferents. Finally, practical use of GO/AuNR/GCE was demonstrated by successfully determining the content of UA in human urine samples with the standard addition approach.
1. Introduction
Uric acid (C5H4N4O3) is a biological substance found in body fluids as the end product of purine metabolism in the human body.1 Generally, uric acid (UA) concentrations in blood or urine are indicative of a person's physical state.2 Normal UA levels in healthy adults range from 1.5–6.0 mg dL−1 for women and 2.5–7.0 mg dL−1 for men.3 UA has limited solubility in water, and if its concentration exceeds normal levels, it can lead to the formation of monosodium urate crystals.4 Humans cannot convert UA to a more soluble substance, allantoin, due to the lack of a uricase enzyme.3 Maintaining UA levels at normal levels becomes important, as higher or lower UA levels can trigger various diseases, such as hyperuricemia,5 gout,6 kidney stones,7 and diabetes.8 As an essential biological substance, UA can be accurately detected in clinical monitoring and diagnosis.
Various analytical methods have been reported for UA, including fluorometry,9 liquid chromatography,10 colorimetry,11 and UV-Vis spectrophotometry.12 Although these studies had success in detecting UA, there are several obstacles to their application, such as the need for various chemical reagents and trained analysts to operate the instrument. In contrast, electrochemical sensors are a suitable alternative, as they yield high sensitivity with low detection limits, simple sample preparation, and shorter analysis time.13
The essential component for the development of electrochemical sensors is the electrode. A glassy carbon electrode (GCE) is widely used to develop an electrochemical sensor, as it provides a wide electrochemical potential window.14 Various organic or inorganic materials are used to modify GCE to improve its performance. The surface of a working electrode can be modified using a carbon allotrope, such as graphene oxide (GO), which consists of single or several layers of 2D graphite arranged hexagonally and containing carboxyl, hydroxyl, and epoxy functional groups.15 GO is an attractive material for the development of sensor devices owing to its high surface area and ability to hold conductive nanoparticles, while preventing them from leaching.16 Conductive nanoparticles, such as the Au nanorod (AuNR) increase the sensitivity of the working electrode17 and act as an electrocatalyst.18 The respective advantages of GO and AuNR, when mixed into composites, can provide a large electrochemically active surface area for biomolecule adsorption and accelerate the electron transfer from the analyte solution to the electrode.19
GO and its composites in the UA's electrochemical sensor have been previously documented in the literature. Sohouli and co-workers reported that GCE modified with MC-GO-Fe3O4 coating has a larger surface area and provides a finer-grained media to promote electron transport during the reaction between the analyte and electrode.20 Imran et al. reported that the UA, DA, and AA oxidation peaks were well-separated in their simultaneous determination using GO/AuNP-modified GCE.19 Metal nanoparticles, such as AuNR, likewise increased electrocatalytic properties of modified electrodes for sensing applications.21 The composite of GO and AuNR has been used in a few previous electrochemical sensors for tartrazine,22 DNA23 and indomethacin.24 However, has not been previously used in the context of UA electrochemical sensing.
In this study, we developed a GO/AuNR composite modified GCE for a sensitive non-enzymatic UA sensor. Response surface methodology (RSM), namely central composite design (CCD), was applied as the experimental design for optimizing the composition of the GO and AuNR. It was chosen as it can be applied to various chemical reactions with several variables and only require a minimal quantity of experimental data.25 Furthermore, CCD is among the most significant RSMs for designing an experiment with the ability to display data on variable interactions and the potential to predict optimal conditions to achieve a satisfactory performance of the sensor.26
2. Experimental methods
2.1 Materials
All chemicals were analytical grade and used as received without further purification. Graphite powder, H2SO4, gold(III) chloride hydrate (HAuCl4·xH2O), hexadecyltrimethylammonium bromide (CTAB), NaBH4, AgNO3, L-(+)-ascorbic acid, and UA were purchased from Sigma Aldrich. Meanwhile, NaNO3, KMnO4, and H2O2 30% were obtained from Merck (Darmstadt, Germany) and KCl was obtained from HiMedia. Deionized water was used throughout all experiments.
2.2 Synthesis of graphene oxide
Graphene oxide was prepared according to Hummers' method with some modifications.27 For this purpose, 1.0 g of graphite powder mixed with 0.5 g NaNO3 and dissolved in 25 mL H2SO4 and subsequently stirred in an ice bath at 0 °C for one hour. Then, 3.0 g KMnO4 was slowly added into the mixed solution and vigorously stirred for one hour, while keeping its temperature below 20 °C. This mixture was slowly warmed to room temperature for 30 min, and 50 mL water was added, thus producing an exothermic reaction at a temperature of 90–95 °C. This obtained mixture was stirred for one hour and left to rest for 15 min. A volume of 50 mL H2O2 (30%) was added to the mixture solution to terminate the reaction and reduce the residual permanganate anions into soluble manganese ions. In the last step of the synthesis, the mixture was washed with deionized water, filtered, and dried in an oven at 80 °C for six hours.
2.3 Synthesis of Au nanorods
AuNR synthesis was carried out according to the seed-mediated method reported by Nikoobakht and El-Sayed.28 The Au seeds solution was prepared by adding 1.2 mL of 10 mM NaBH4 into a mixture containing 10 mL of 0.5 mM HAuCl4·xH2O and 10 mL of 0.1 M CTAB solution, followed by vigorous mixing for 10 min with a magnetic stirrer. Meanwhile, the growth solution was prepared under continuous stirring of 10 mL of 0.25 mM HAuCl4·xH2O and 10 mL of 0.1 M CTAB for 10 min. Next, in the sequence of 0.6 mL of 4 mM AgNO3, 140 μL of 0.078 M ascorbic acid and 0.3 mL of 1.0 M HCl were added to the growth solution under a vigorous stirring. To prepare the final AuNRs, 24 μL of the acquired seed solution was pipetted and transferred to the growth solution. The final mixture was allowed to sit at room temperature overnight and then centrifuged in distilled water at 6000 rpm for 20 min.
2.4 Characterizations and electroanalytical study
Raman spectra of GO were obtained using HORIBA HR Evolution Raman Microscopes with laser excitation at 514 nm. The optical absorption spectra of AuNR were assessed using a Genesys 10s UV-Vis Spectrophotometer. TEM images of the GO and AuNR were acquired using TECNAI G2 Spirit Twin HR-TEM. SEM-EDS images were obtained using the FEI Quanta 650 Scanning Electron Microscope equipped with Energy Dispersive Spectrometer OXFORD. The diffraction pattern was acquired by XRD (PANanalytical AERIS). Furthermore, all electrochemical experiments were carried out using the Palmsens Emstat3 (ES316U669) potentiostat in a three-electrode cell with a glassy carbon electrode (GCE, 3 mm diameter), an Ag/AgCl electrode, and a platinum wire electrode as the working, reference, and auxiliary electrodes, respectively.
2.5 Experimental design
The experimental design was employed to optimize the GO/AuNR composite formula before the deposition step onto an electrode surface. The entire step, including design, mathematical modelling, statistical analysis, and optimization, was performed with Design-Expert software (version 13, Inc., Minneapolis, MN, USA). The interaction phenomena of two variables (GO and AuNR) and their influence on the peak intensity of UA oxidation currents from DPV measurement were investigated using a central composite design (CCD) method. The CCD method was chosen among other experimental designs owing to its capacity to construct the surface responses with fewer required runs.25 It comprises a two-level factorial design augmented with center and axial points to fit quadratic models.29 The variable of two independent inputs was varied on a five-level scale: high level (1), center (0), lower level (−1), and two outer (axial) points. The outer points represent each variable's extreme values (maximum and minimum). For a rotatable design having n variables, the value of α = 2n/4. In our design n = 2, and α = 22/4 = 1.414. Table 1 shows the coded and actual levels of each variable.
Table 1 Experimental levels of variables
Variables |
Codes |
Levels |
−α (lowest) |
−1 (lower) |
0 (center) |
+1 (higher) |
+α (highest) |
GO (mg mL−1) |
A |
1.98223 |
2.5 |
3.75 |
5 |
5.51777 |
AuNR (mL) |
B |
3.9645 |
5 |
7.5 |
10 |
11.0355 |
The selection of GO concentration was based on a previous scientific report. The low level (−1) and high level (+1) concentrations of GO were 2.5 and 5.0 mg mL−1, respectively, which provides excellent electrochemical properties and flexibility.30,31 In addition, the high and low levels of AuNR were 5.0 and 10.0 mL, following a scientific report32 for the low level and a randomly chosen high level. The total number of required runs is calculated as 2n +2n + nc. The values 2n, 2n, and nc represent factorial, axial, and center runs, respectively. The recommended number of replicates at the center point for two variables is five.33 Consequently, a total of 13 runs are performed. The runs were executed in a randomized order to eliminate systematic bias. The results of these experiments are shown in Table 2.
Table 2 Central composite design for two independent variables and observed responses
Run |
Coded value |
Uncoded value |
I (μA) |
Predicted accuracy |
A |
B |
GO (mg mL−1) |
AuNR (mL) |
Predicted |
Experimental |
1 |
0 |
−1.414 |
3.75 |
3.96447 |
4.426 |
4.510 |
98.14% |
2 |
−1.414 |
0 |
1.98223 |
7.5 |
5.789 |
5.900 |
98.12% |
3 |
1.414 |
0 |
5.51777 |
7.5 |
4.413 |
4.447 |
99.24% |
4 |
−1 |
1 |
2.5 |
10 |
6.571 |
6.480 |
98.60% |
5 |
−1 |
−1 |
2.5 |
5 |
4.802 |
4.695 |
97.72% |
6 |
0 |
0 |
3.75 |
7.5 |
5.739 |
5.687 |
99.09% |
7 |
0 |
0 |
3.75 |
7.5 |
5.739 |
5.787 |
99.17% |
8 |
0 |
1.414 |
3.75 |
11.0355 |
6.173 |
6.235 |
99.01% |
9 |
1 |
1 |
5 |
10 |
5.065 |
5.028 |
99.26% |
10 |
0 |
0 |
3.75 |
7.5 |
5.739 |
5.785 |
99.20% |
11 |
1 |
−1 |
5 |
5 |
4.363 |
4.310 |
98.77% |
12 |
0 |
0 |
3.75 |
7.5 |
5.739 |
5.649 |
98.41% |
13 |
0 |
0 |
3.75 |
7.5 |
5.739 |
5.791 |
99.10% |
2.6 Preparation of GO/AuNR composite modified GCE
To prepare the GO/AuNR composite, GO and AuNR were mixed based on the formula suggested by CCD (Table 2). The concentration of GO was varied in the ranges of 1.982–5.517 mg mL−1 and mixed with AuNR in the ranges of 3.964–11.035 mL, followed by dilution with deionized water to obtain a total volume of 20 mL. The mixture was then ultrasonicated for 30 minutes. The amount of 4 μL GO/AuNR composite was drop-cast onto the GCE surface. The electrode was then dried in an oven at 80 °C for 4 min and labelled as GO/AuNR/GCE. For comparison, AuNR/GCE and GO/GCE electrodes were also prepared by a similar method.
2.7 Sensing evaluation
2.7.1 Linearity, LOD, and LOQ. The linearity of the proposed UA sensor was evaluated by preparing UA solutions at various concentrations ranging from 10 to 90 μM in 0.1 M KCl as a supporting electrolyte. Each UA concentration was then scanned in triplicate measurements using the DPV technique at a potential window of +0.2 to +1.0 V, a scan rate of 50 mV s−1, potential step of 5 mV, potential pulse of 25 mV, and a pulse time of 0.01 s. Linearity was examined from the relationship between the concentration of UA solution and the response of anodic peak current from UA measurements. Meanwhile, the signal-to-noise ratio (SNR) approach was employed to determine the limit of detection (LOD) and limit of quantitation (LOQ). The LOD value was determined by gradually lowering the concentration of UA until SNR reached 3
:
1. Meanwhile, the LOQ value was estimated from the ratio of UA concentration to blank solution, as SNR typically yielded 10
:
1.
2.7.2 Reproducibility and stability. The reproducibility of the proposed sensor was examined by preparing five different electrodes of the GCE-modified GO/AuNR composite. The reproducibility was evaluated by utilizing these five electrodes to measure 80 μM of UA solution. Meanwhile, the stability of the proposed UA sensor was examined by measuring 80 μM of UA solution for five days using a similar electrode. All electrochemical UA measurements were performed using the DPV technique under optimum experimental conditions.
2.7.3 Selectivity. The selectivity of the proposed sensor was investigated by measuring 80 μM of UA solution in the presence of several probable interfering compounds. Ascorbic acid (AA), dopamine (DA), glucose (G), urea (UR), and magnesium (Mg) are some of the candidate interferents. The DPV technique was utilized at a potential window from −0.2 to +1.0 V, scan rate 50 mV s−1, potential step of 5 mV, potential pulse of 25 mV, and a pulse time of 0.01 s.
2.8 Real sample analysis
The performance of the proposed sensor in real sample analysis was evaluated on a human urine sample collected from a healthy volunteer. The sample was first diluted 50-fold. Then, 5 mL of diluted urine was spiked with UA standard solution, followed by 0.1 M KCl dilution to obtain a final concentration of spiked UA in the range of 5–50 μM. The spiked sample was then measured using the DPV technique in the potential range of +0.2 to +1.0 V, at a scan rate of 50 mV s−1, a potential step of 5 mV, a potential pulse of 25 mV, and a pulse time of 0.01 s. The real concentration of UA in human urine was estimated based on the x-intercept from the calibration curve of the standard addition method, using the equation below.
where Ca denotes the spike concentration, V0 is the initial volume of urine sample before spiking, and Vf is the final volume of urine sample after spiking.
3. Results and discussions
3.1 Characterization of GO and AuNR
D, G, and 2D are the most important bands to consider in the Raman spectrum of carbon-based materials. Carbon structural defects or vacancies and the presence of sp3 carbons are correlated with the D band. In-plane vibrations of sp2-bonded carbon atoms are associated with the G band. Meanwhile, 2D confirms the number of graphene layers.34 Fig. 1(a) shows the graphite spectrum with three distinct bands. Graphite has a strong G band at 1575 cm−1, as it has not yet been oxidized, a low-intensity D band at 1347 cm−1, due to the small number of defects and vacancies, and a medium intensity 2D band at 2707 cm−1 attributed to the considerable number of layers.35 Meanwhile, in the GO spectrum, the D band has a higher intensity than the graphite due to the formation of defects, vacancies, and distortions during oxidation. The G band of GO was observed at 1593 cm−1, with a lower intensity than those observed in graphite. When graphite is oxidized, the introduction of functional groups causes disruption of its structure. The p orbitals' reaction transforms sp2 bonds into sp3 bonds, leading to functionalization. The value obtained is a qualitative tool for evaluating the material's structural defects.27 The ID/IG ratio intensity indicates the degree of disorder in graphene. Because of the insertion of some functional groups, ID/IG increases from 0.14 (graphite) to 1.08 (GO), indicating a decrease in the degree of crystallinity. The low intensity of the 2D peak in the GO spectrum typically means that GO has a three-dimensional structure with a limited number of layers.36
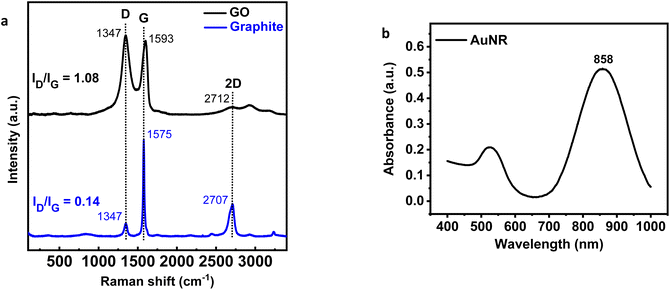 |
| Fig. 1 (a) Raman spectrum of graphene oxide (GO) and graphite, (b) UV-VIS absorption spectrum of Au nanorod (AuNR). | |
The appearance of visible light absorption due to the SPR effect in the UV-Vis spectrum was used to confirm the formation of AuNR. As shown in Fig. 1(b), AuNR was found to have two distinct SPR bands at 526 and 858 nm. The formation of these two bands is caused by electron oscillation in separate plasmonic vibration modes.37,38 Electron oscillations could explain the strong SPR band at longer wavelengths along the long axis, also known as the longitudinal band. In contrast, the weak SPR band was formed by transversal electron oscillation at shorter wavelengths.
TEM analysis provides additional evidence to study the GO morphology, as well as the shape and size of AuNR. A thin crumpled sheet-like structure is revealed by TEM images of GO (Fig. 2(a)). The layers of GO are disassembled, revealing a wrinkled structure. Results from TEM images of AuNR (Fig. 2(b)) demonstrated that the as-prepared AuNR was successfully synthesized. The size of 200 particles of AuNR was measured to determine the average aspect ratio. Consequently, nanorods with an average aspect ratio of 3.5 are formed. The average size of AuNR is approximately 50 ± 5 nm in length and 14 ± 1 nm in width. Fig. 2(c) shows a TEM image of the GO–AuNR composite. TEM images indicate that the as-prepared AuNR are successfully combined with GO to form the GO/AuNR composite. Several small gold nanoparticles with rod shapes appeared on the surface of the ultra-thin wrinkled morphology of GO sheets.
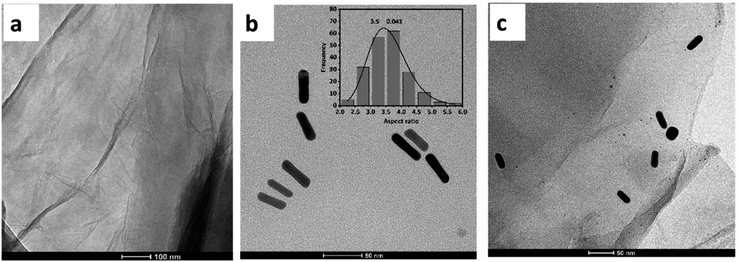 |
| Fig. 2 TEM image of (a) GO, (b) AuNR, and (c) GO/AuNR composite. | |
Fig. 3(a) and (b) show the SEM image and EDS spectra of the electrodes (SPCE) before and after modification with the GO/AuNR composite. The GO/AuNR modified electrode exhibits a rough and corrugated surface. The typical wrinkle-like pattern of GO and its integration with AuNR can be observed in the SEM image (Fig. 3(b)). The SEM-EDS analysis was used to confirm the composition of GO/AuNR composite on the surface of the electrode. The EDS spectra show signals of carbon (C), oxygen (O), and gold (Au) at the GO/AuNR modified electrode (Fig. 3(b)) compared to the unmodified electrode (Fig. 3(a)).
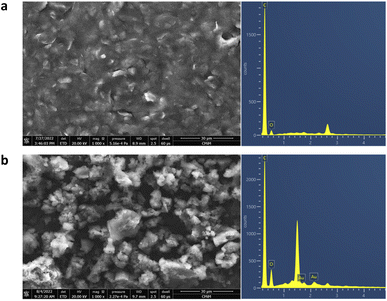 |
| Fig. 3 SEM-EDS image of (a) unmodified electrode and (b) GO/AuNR modified electrode. | |
Fig. 4 depicts the diffraction patterns of the GO/AuNR composite. At 2θ of 10.2, a strong and distinct peak corresponds to the GO characteristic.39 The presence of another peak at 2θ values of 38.2°, 42.1°, and 77.3° in the GO/AuNR composite corresponds to the face-centered cubic crystalline Au pattern (ICDD code 00-002-1095).40 The existence of characteristic Au signals in the composite indicates that both materials maintained their phase and crystallinity, even in the composite form. Furthermore, it demonstrates that AuNR effectively prevents GO layers from re-stacking.
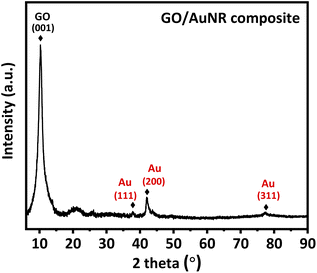 |
| Fig. 4 XRD pattern of GO/AuNR composite. | |
3.2 Optimum formula of GO/AuNR composite by CCD
The GO/AuNR composite optimization was carried out by the experimental design using mathematical and statistical analyses to evaluate the effect of variables (GO and AuNR) on the response (peak current intensity of UA oxidation). The design matrix in the form of variables and their ranges, as well as the resulting response, is shown in Table 2. Experiments were conducted with five replicates at the center to determine reproducibility and experimental error.
The quadratic model for predicting the optimal condition was derived according to the equation:
where
β0 is a constant coefficient;
xi and
xj are independent variables (GO and AuNR in this experiment);
i and
j range from 1 to
n. The coefficients
βi,
βii, and
βij represent the linear, quadratic, and interaction effects, respectively.
n is the number of studied variables, and
ε is the error.
41 In this study, the quadratic relationship between responses (
Ioks) and two variables (GO and AuNR) acquired by the software is expressed in the equation:
Ioks (μA) = −1.90752 + 1.78383GO (mg mL−1) + 1.09484AuNR (mL) − 0.204376GO (mg mL−1) × GO (mg mL−1) − 0.035174AuNR (mL) × AuNR (mL) − 0.085360GO (mg mL−1) × AuNR (mL) |
The analysis of variance (ANOVA) was used to test the feasibility of the model. As displayed in Table 3, the model with a P-value of less than 0.05 implies that the data is statistically significant. All linear terms of GO and AuNR significantly influence the P-value < 0.05. Every square term and interaction also have significant P-values (<0.05). The non-significant P-value of the lack of fit (more than 0.05) confirms that the quadratic model is in agreement with the data. Furthermore, the model's coefficient of determination (R2) and adjusted R2 values of 0.9894 and 0.9818, respectively, are close to 1. The latter agrees with the predicted R2 of 0.9403, implying a good correlation between the actual and predicted values.26,29 The actual and predicted UA oxidation currents are shown in Table 2 for various sets of independent variables (GO and AuNR). The experimental (actual) values are present a satisfactory fit compared to the predicted values. Consequently, the model is judged adequate for prediction within the studied range.
Table 3 ANOVA on design experiment quadratic modela
Source |
DF |
Sum of squares |
Mean square |
F-value |
P-value |
DF = degree of freedom. significant. not significant. |
Model |
5 |
6.16559 |
1.23312 |
130.26 |
0.000b |
A |
1 |
1.89331 |
1.89331 |
200.00 |
0.000 |
B |
1 |
3.05356 |
3.05356 |
322.56 |
0.000 |
AB |
1 |
0.70940 |
0.70940 |
74.94 |
0.000 |
A2 |
1 |
0.33620 |
0.33620 |
35.51 |
0.001 |
B2 |
1 |
0.28462 |
0.28462 |
30.07 |
0.001 |
Lack of fit |
3 |
0.04834 |
0.01611 |
3.60 |
0.124c |
Pure error |
4 |
0.01792 |
0.00448 |
|
|
Total |
12 |
6.23186 |
|
|
|
The contour plot shown in Fig. 5(a) informs the effect of the interaction between the two variables. The optimum conditions are depicted by the red area. Experiments conducted within the red zone will yield the optimum response. As shown in Fig. 5(b), the maximum UA oxidation peak current is obtained at a lower GO concentration and higher volume of AuNR. The increasing value of AuNR has a positive effect on the response, which might be related to its conductive properties.42 GO can likewise provide a high surface area for AuNR loading. Nevertheless, its excessive addition can cause stacking of graphene sheets on the GCE surface, thus obstructing electron flows and lowering its conductivity.43
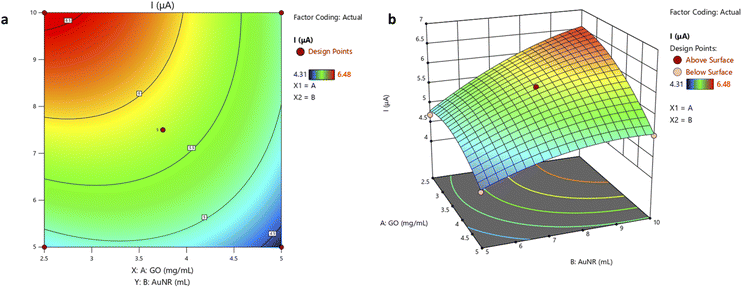 |
| Fig. 5 (a) The contour plot and (b) 3D surface plot exhibit the effect of GO and AuNR variables on uric acid oxidation current. | |
The contour plot comprises three parts: a vertical axis/y value (independent variable 2/AuNR volume), a horizontal axis/x value (independent variable 1/GO concentration), and lines (response values/z values). A contour plot depicts surfaces with similar z-heights (response) on a plane with x and y dimensions. For a given z value, lines are drawn from a point in (x, y) coordinates to the following point with the same z value. Each independent variable is usually restricted to a regular grid of interest. Actual techniques to determine z values usually rely on computer simulations to numerically generate the data.44
Furthermore, a confirmatory run was conducted under the proposed optimum conditions to optimize and validate the mathematical model. The predicted optimization result associated with the maximum UA oxidation peak current of 6.572 μA is reached in the experiment. The average obtained from a triplicate measurement of UA oxidation current (Table 4) using the optimal composite formula is 6.572 μA, which is in good agreement with the predicted response. This confirms that the model is adequate in the studied range. However, the contour plot in this study has not yet achieved the true center of the optimum condition. The level of variables in the experiment must be extended to find the actual optimum condition. The extension of the variable level was not conducted in this study. Overcoming this limitation could open doors to future research opportunities.
Table 4 Uric acid peak current validation at optimum formula of composite GO/AuNR
Repetition |
GO (mg mL−1) |
AuNR (mL) |
I (μA) |
1 |
2.5 |
10 |
6.574 |
2 |
2.5 |
10 |
6.566 |
3 |
2.5 |
10 |
6.578 |
Average |
|
|
6.572 |
3.3 Electrochemical oxidation of UA at GO/AuNR/GCE
The modified electrode was evaluated through UA solution measurement. UA has a pKa1 of 5.5 and a pKa2 of 10.3. In human urine, pKa1 is responsible for converting UA to a more soluble anionic salt.45 The modified GCE was evaluated by cyclic voltammetry (CV) and the DPV technique. CV was applied between the potentials of +0.2 to +1.0 V vs. Ag/AgCl in electrolyte 0.1 M KCl in the presence of 0.1 mM UA at a scanning rate of 100 mV s−1 and a potential step of 10 mV. Meanwhile, DPV was applied between the potentials of +0.2 to +0.9 V vs. Ag/AgCl at a scan rate of 50 mV s−1, a potential step of 5 mV, potential pulse of 25 mV, and pulse time of 0.01 s. Voltammetry requires a conductive solvent to ensure current flow between the electrode and the solution. The addition of a supporting electrolyte increases the solution's conductivity. The supporting electrolyte must be inert and not undergo a redox reaction in the measurement's potential window. Because KCl has a strong ionic field, it can accelerate electron transfer and double-layer capacitance kinetics.46 It does so by reducing the electron transfer barrier at the electrode and electrolyte solution interface.
In this electrochemical study, CV was employed to investigate the material's electrochemical behavior. The current was measured by sweeping the potential back and forth (from positive to negative and vice versa) between the chosen potential window. A cyclic voltammogram reveals the redox peaks and predicts the electrode's capacitive behavior.47 Fig. 6(a) shows the cyclic voltammograms of UA at different electrodes. At bare GCE, a broad UA oxidation peak is obtained at 0.7 V vs. Ag/AgCl. However, at GO/AuNR/GCE, the oxidation peak appears at 0.57 V vs. Ag/AgCl with a significant increase in the current (five times higher than at bare GCE). Furthermore, Fig. 6(b) displays the DPVs voltammogram of UA at different electrodes. The peak related to the oxidation of UA appeared in the potential of 0.49 V vs. Ag/AgCl at GO/AuNR/GCE (shifted to a more negative potential compared to other modified GCE) which indicates that UA is easier to oxidize at GO/AuNR/GCE. In this electrochemical study, DPV was employed owing to its high sensitivity and capability to eliminate capacitive or background current. This can be accomplished by measuring the current twice during the potential scanning process, namely before and at the end of the pulse.
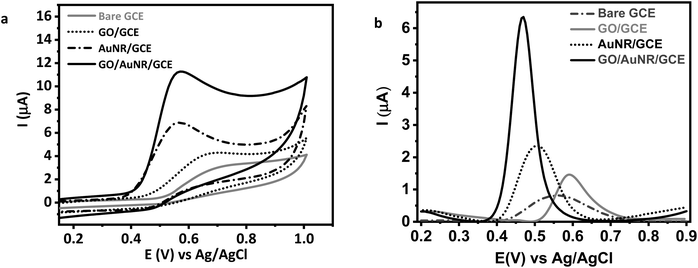 |
| Fig. 6 (a) Cyclic and (b) differential pulse voltammogram of 0.1 mM UA in 0.1 M KCl at bare GCE and modified GCE. | |
The enhancement in response currents and lowering of the anodic potential indicate a catalytic effect of GO/AuNR/GCE for UA oxidation. AuNR may exhibit an electrocatalytic effect for UA oxidation. While GO sheets as a conductive material offer a large surface for electron transfer, UA may form hydrogen bonds or π–π interactions with GO, leading to the adsorption of UA on the GO surface and increase of the electron transfer.48,49 In turn, AuNR also attaches to GO due to electrostatic interactions.50 The AuNR role as a carrier-transfer route enables fast electron transfer ability for the UA.18 AuNR further prevents GO sheets from aggregating, maintaining UA accessibility to the surface of GO. Consequently, the GO/AuNR composite plays an essential role in improving the sensitivity of the UA sensor. Hence, both materials improve the detection signal and lower the detection limit of the sensors.
3.4 Electrochemical evaluation of GO/AuNR/GCE for UA detection
3.4.1 Linearity, LOD, and LOQ. The electrochemical performance of GO/AuNR/GCE for UA detection was initially studied in UA solutions at various concentrations (10–90 μM). Fig. 7(a) shows that well-defined peaks increase in intensity as the UA concentration rises from 10 to 90 μM. Potential oxidations of UA appear to be around 0.49 V vs. Ag/AgCl. The equation for linear regression of oxidation current versus varied UA concentrations is I (μA) = 0.0623 CUA − 0.4084 (R2 = 0.9950). Each point represents the average of a triplicate of experimental trials. In this study, the value of detection limit (LOD) (S/N = 3) and quantification limit (LOQ) (S/N = 10) were estimated as 0.4 and 1.0 μM, respectively. The LOD value is lower than the concentration of UA in normal human urine, implying that this sensor can be used to determine UA in a real sample. Furthermore, the concentration range obtained in this study was comparable to that acquired by other UA sensors, as shown in Table 5. The LOD still requires improvement, and the detection range is relatively small compared to other electrochemical methods. Nevertheless, the key advantage of this study is that we performed a voltametric measurement using KCl as the supporting electrolyte. Preparing a KCl solution in water is relatively simple, and will therefore shorten the measurement time. Furthermore, with a detection limit of 0.4 μM, the detection of UA in both blood and urine is possible.
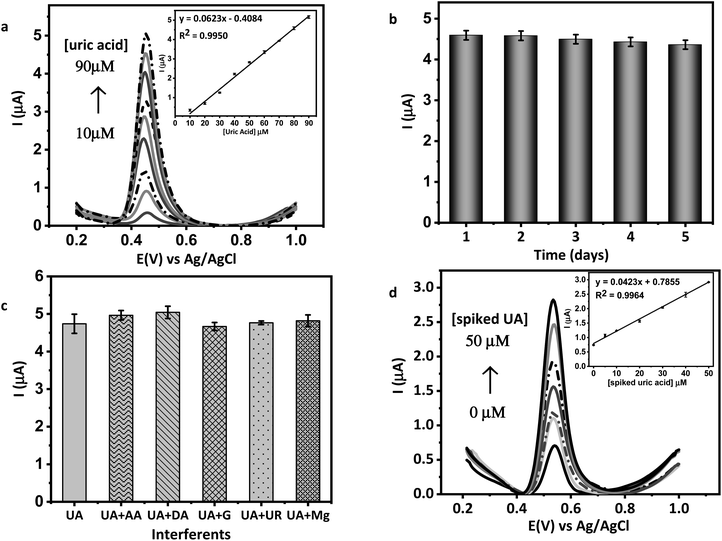 |
| Fig. 7 (a) Differential pulse voltammogram of various concentrations of UA in KCl 0.1 M at GO/AuNR/GCE (inset: the linear correlation between UA concentration and peak current), (b) graph of stability evaluation, (c) graph of selectivity studies, and (d) differential pulse voltammogram of human urine spiked with UA in range of 5–50 μM (inset: resulting calibration plot of human urine sample analysis). | |
Table 5 Comparison of proposed sensor's performance with other UA sensorsa
Electrode |
Technique |
Linear range (μM) |
LOD (μM) |
Ref. |
Methylcellulose/graphene oxide/iron oxide nano hydrogel (MC–GO–Fe3O4), electrochemically reduced graphene oxide (ErGO), poly(3,4-ethylenedioxythiophene) polystyrene sulfonate (PEDOT:PSS), gold nanoparticles decorated polypyrrole/graphene oxide composite on carbon fiber paper (AuNPs@GO/PPy/CFP), 3D-networked nanostructure composed of molibdenum disulfida, reduced graphene oxide and gold nanoparticles (3D-MoS2/rGO/Au), hierarchical nanoporous gold silver alloy (HNP–AuAg), chemical reduced graphene oxide–Au nanocages (CRGO–AuNCs), gold nanoparticles–polyaniline (AuNPs/PANI). |
GCE/MC–GO–Fe3O4 |
DPV |
0.5–140 |
0.17 |
20 |
ErGO/PEDOT:PSS/GCE |
DPV |
10–100 |
1.08 |
55 |
AuNPs@GO/PPy/CFP |
DPV |
2–360 |
1.68 |
56 |
3D-MoS2/rGO/Au/ITO |
DPV |
5–2215 |
0.74 |
57 |
HNP–AuAg/GCE |
Amperometry |
5–425 |
1.00 |
58 |
CRGO–AuNCs/GCE |
DPV |
5–150 |
2.00 |
59 |
AuNPs/PANI/GCE |
LSV |
20–100 |
16.00 |
60 |
GO/AuNR/GCE |
DPV |
10–90 |
0.40 |
This work |
3.4.2 Reproducibility and stability. Five sensors were prepared independently under identical conditions to evaluate the reproducibility of GO/AuNR/GCE for the determination of UA. These electrodes were used for DPV measurements of 80 μM UA in 0.1 M KCl. The DPV response currents using five different electrodes displayed a relative standard deviation (RSD) of 2.758%. This excellent reproducibility with % RSD < 5% demonstrates the reliability of the detection results.51 The resulting sensor stability was investigated by repeating the measurements with 80 μM UA using the same modified electrode for five consecutive days (Fig. 7(b)). The result shows that the day-to-day DPV response for 80 μM UA at GO/AuNR/GCE yields a % RSD value of 2.207%, providing evidence for good sensor stability.
3.4.3 Sensor selectivity. The selectivity of the proposed sensor for UA detection was investigated by measuring the sensor responses in the presence of candidate interfering species. Species with the oxidation peak potential close to the UA potential are commonly employed in selectivity studies. AA, UA, and DA oxidation potentials typically overlap at the non-modified electrode, leading to low selectivity.52,53 Further, species such as magnesium (Mg) ions and molecules like glucose and urea are present in a complex biological sample. Therefore, measuring UA in the presence of these species is required for real application purposes. As shown in Fig. 7(c), the DPV responses for 80 μM UA in the presence of various interferents (80 μM) demonstrates that these interfering substances have only negligible influence on the detection of UA. The % recovery obtained after the addition of each interference ranged from 98.5 to 104.8%. Therefore, the proposed sensor might be used to determine UA in the presence of the investigated several interference substances present in real samples.
3.5 Analysis of human urine samples
GO/AuNR composite modified GCE was applied to determine UA quantities in real human urine samples. Under normal conditions, UA is eliminated in human urine at a rate of 250–750 mg per day or 1.48–4.46 mmol per day. The concentration of UA in human urine is 0.77–5.58 mM if the urine volume excreted by a healthy human is estimated to be 800–2000 mL. The multiple-point standard addition method was applied to evaluate UA measurements for real samples. Fig. 7(d) shows the DPV voltammograms and the inset resulting calibration plot of human urine sample analysis. The obtained UA concentration in urine samples using the DPV technique was 1.845 mM.
The estimation of UA concentration was also compared using a commercial sensor to evaluate the performance of the proposed modified electrode. The commercial sensor employed is a multipurpose monitoring system of blood glucose, cholesterol, and UA. The commercial sensor read the concentration of UA in the human urine sample at 1.715 mM. The results from developed and commercial sensors were then compared with a two-tailed t test and resulted in tcount < ttable. In conclusion, data obtained from the two methods show no significant differences.54 This result proves that the proposed modified electrode exhibits good accuracy. Therefore, the modified GCE proposed in this study has the potential to be used to determine the UA concentration in a human urine sample.
4. Conclusions
A modified GCE based on the GO–AuNR composite was successfully manufactured. We demonstrated its excellent electrochemical characteristics in the detection of UA. The central composite design yielded the optimum GO/AuNR composite formula to develop the sensor. Results indicate that the proposed sensor has prospective electroanalytical characteristics, including a linear dynamic range with low LOD (0.4 μM) and LOQ (1.0 μM) values. Moreover, satisfactory results were obtained with regard to the selectivity, reproducibility, and stability of the modified electrode and the successful quantitation of UA in real human urine samples. The present study demonstrates the function of the composite GO/AuNR as a promising electrode candidate for constructing sensitive UA sensors.
Conflicts of interest
There are no conflicts to declare.
Acknowledgements
We acknowledge the Ministry of Research and Technology, National Research and Innovation Agency RI for the research funding in scheme PDUPT fiscal year 2022 (Contract No. 3644/IT3.L1/PT.01.03/P/B/2022) and partial support from Hibah PUTI Q1 2022 (Contract No. NKB-480/UN2.RST/HKP.05.00/2022).
References
- K. Jindal, M. Tomarb and V. Gupta, Analyst, 2013, 138, 4353–4362 RSC.
- K. Arora, M. Tomarb and V. Gupta, Analyst, 2014, 139, 837–849 RSC.
- J. Maiuolo, F. Oppedisano, S. Gratteri, C. Muscoli and V. Mollace, Int. J. Cardiol., 2016, 213, 8–14 CrossRef PubMed.
- F. Perez-Ruiz, N. Dalbeth and T. Bardin, Adv. Ther., 2015, 32, 31–41 CrossRef CAS PubMed.
- C. Benn, P. Dua, R. Gurrell, P. Loudon, A. Pike, R. I. Storer and C. Vangjeli, Front. Med., 2018, 160, 1–28 Search PubMed.
- P. K. Tan and J. N. Miner, ADMET & DMPK, 2017, 5, 59–74 Search PubMed.
- E. Paul, P. Sasikumar, S. Gomathi, A. Abhishek and G. S. Selvam, Multifunct. Syst. Comb. Delivery, Biosens. Diagn., 2017, 327–345 CAS.
- Y. Cui, H. Bu, X. Ma, S. Zhao, X. Li and S. Lu, J. Diabetes Res., 2016, 2016, 1–6 CrossRef PubMed.
- Y. Wang, Y. Yang, W. Liu, F. Ding, Q. Zhao, P. Zou, X. Wang and H. Rao, Microchim. Acta, 2018, 185, 1–9 CrossRef PubMed.
- N. Wijemanne, P. Soysa, S. Wijesundara and H. Perera, Int. J. Anal. Chem., 2018, 2018, 1–6 CrossRef PubMed.
- M. Islam, I. Ahmed, M. I. Anik, M. Ferdous and M. S. Khan, Front. Chem., 2018, 6, 496 CrossRef CAS.
- N. Norazmi, Z. A. Rasad, M. Mohamad and H. Manap, IOP Conf. Ser.: Mater. Sci. Eng., 2017, 257, 012031 Search PubMed.
- T. M. Mariano, M. A. Beluomini and N. R. Stradiotto, J. Braz. Chem. Soc., 2021, 32, 249–259 CAS.
- L. Durai and S. Badhulika, Mater. Sci. Eng., C, 2020, 111, 110806 CrossRef CAS.
- A. J. S. Ahammad, T. Islam, Md. M. Hasan, M. N. I. Mozumder, R. Karim, N. Odhikari, P. R. Pal, S. Sarker and D. M. Kim, J. Electrochem. Soc., 2018, 165, B174–B183 CrossRef CAS.
- N. Vafa, M. Kakroudi and M. Asl, Ceram. Int., 2020, 46, 8561–8566 CrossRef.
- F. Shahdost-fard and M. Roushani, Talanta, 2020, 209, 120506 CrossRef CAS.
- A. R. Marlinda, et al., J. Alloys Compd., 2020, 847, 156552 CrossRef CAS.
- H. Imran, P. N. Manikandan and V. Dharuman, RSC Adv., 2015, 5, 63513–63520 RSC.
- E. Sohouli, E. M. Khosrowshahi, P. Radi, E. Naghian, M. Rahimi-Nasrabadi and F. Ahmadi, J. Electroanal. Chem., 2020, 877, 114503 CrossRef CAS.
- S. Jayabal, P. Viswanathan and R. Ramaraj, RSC Adv., 2014, 4, 33541–33548 RSC.
- K. Deng, C. Li, X. Li and H. Huang, J. Electroanal. Chem., 2016, 780, 296–302 CrossRef CAS.
- X. Han, X. Fang, A. Shi, J. Wang and Y. Zhang, Anal. Biochem., 2013, 443, 117–123 CrossRef CAS PubMed.
- M. Arvand and T. M. Gholizadeh, Sens. Actuators, B, 2013, 186, 622–632 CrossRef CAS.
- S. M. Ghoreishi, M. Behpour and A. Khoobi, Anal. Methods, 2012, 4, 2475–2485 RSC.
- F. Hashemi, A. R. Zanganeh, F. Naeimi and M. Tayebani, Anal. Methods, 2020, 12, 3045–3055 RSC.
- O. Akhavan, K. Bijanzad and A. Mirsepah, RSC Adv., 2014, 4, 20441–20448 RSC.
- B. Nikoobakht and M. A. El-Sayed, Chem. Mater., 2003, 15, 1957–1962 CrossRef CAS.
- T. M. Laid, K. Abdelhamid, L. S. Eddine and B. Abderrhmane, J. Mol. Struct., 2021, 1229, 129497 CrossRef CAS.
- B. Li, et al., ACS Appl. Mater. Interfaces, 2019, 11, 46044–46053 CrossRef CAS PubMed.
- P. Li, C.-A. Tao, B. Wang, J. Huang, T. Li and J. Wang, J. Nanosci. Nanotechnol., 2017, 18, 713–718 CrossRef PubMed.
- Y. Yang, Y. Wang, M. Zhu, Y. Chen, Y. Xiao, Y. Shen and A. Xie, Biomater. Sci., 2017, 5, 990–1000 RSC.
- T. K. Trinh and L. S. Kang, Environ. Eng. Res., 2010, 15, 63–70 CrossRef.
- A. E. F. Oliveira, G. B. Braga, C. R. T. Tarley and A. C. Pereira, J. Mater. Sci., 2018, 53, 12005–12015 CrossRef CAS.
- B. D. Ossonon and D. Bélanger, RSC Adv., 2017, 7, 27224–27234 RSC.
- L. Shahriary and A. Athawale, Int. J. Renew. Energy Env. Eng., 2014, 2, 58–63 Search PubMed.
- M. Khalil, R. I. Pratama, M. Sujak, A. Garry, D. Djuhana, A. Umar, C. W. Lai and B. M. Jan, Mater. Chem. Phys., 2019, 249, 123018 CrossRef.
- T. C. Lebepe, S. Parani and O. S. Oluwafemi, Nanomaterials, 2020, 10, 1–24 CrossRef PubMed.
- F. M. Casallas Caicedo, et al., Diamond Relat. Mater., 2020, 109, 108064 CrossRef CAS.
- H. Naeem, M. Ajmal, S. Muntha, J. Ambreenc and M. Siddiq, RSC Adv., 2018, 8, 3599–3610 RSC.
- P. Mondal and M. K. Purkait, J. Cleaner Prod., 2018, 170, 1111–1123 CrossRef CAS.
- G. Zhang, Nanotechnol. Rev., 2013, 2, 269–288 CAS.
- J. Song, L. Xu, R. Xing, Q. Li, C. Zhou, D. Liu and H. Song, Sci. Rep., 2014, 4, 1–7 Search PubMed.
- L. Hasanah, M. Iryanti, N. D. Ardhi and S. Feranie, Appl. Phys. Res., 2013, 5, 78 Search PubMed.
- W. T. Wahyuni, B. R. Putra, R. Heryanto, E. Rohaeti, D. H. Y. Yanto and A. Fauzi, Int. J. Electrochem. Sci., 2021, 16, 1–14 Search PubMed.
- E. E. Krisnaniningrum, A. Mulyasuryani and H. Sulistyarti, Molekul, 2021, 16, 186–193 CrossRef.
- Y. S. Choudhary, L. Jothi, and G. Nageswaran, Spectroscopic Methods for Nanomaterials Characterization, Elsevier, 2017, pp. 19–54 Search PubMed.
- S. N. Faisal, C. M. Subramaniyam, E. Haque, M. M. Islam, N. Noorbehesht, A. K. Roy, M. S. Islam, H. K. Liu, S. X. Dou, A. T. Harris and A. I. Minett, ACS Appl. Energy Mater., 2018, 1, 5211–5223 CAS.
- Y. Xue, H. Zhao, Z. Wu, X. Li, Y. He and Z. Yuan, Biosens. Bioelectron., 2011, 29, 102–108 CrossRef CAS PubMed.
- T. C. Lebepe, S. Parani and O. S. Oluwafemi, Nanomaterials, 2020, 10, 2149 CrossRef CAS PubMed.
- Z. Fredj, B. Ali and N. Abbas, Anal. Methods, 2020, 12, 3883–3891 RSC.
- N. M. M. A. Edris, J. Abdullah, S. Kamaruzaman and Y. Sulaiman, J. Electrochem. Soc., 2019, 166, B664–B672 CrossRef CAS.
- Y. Li, Y. Li, H. Song, L. Zhang, P. Zuo, B. C. Ye, J. Yao and W. Chen, Biosens. Bioelectron., 2016, 78, 308–314 CrossRef CAS PubMed.
- D. Harvey, Analytical Chemistry 2.1, McGraw-Hill Comp, New York, US, 2016 Search PubMed.
- B. R. Putra, U. Nisa, R. Heryanto, E. Rohaeti, M. Khalil, A. Izzataddini and W. T. Wahyuni, Anal. Sci., 2021, 38, 157–166 Search PubMed.
- C. Tan, J. Zhao, P. Sun, W. Zheng and G. Cui, New J. Chem., 2020, 44, 4916–4926 RSC.
- Y. Zhao, J. Zhou, Z. Jia, D. Huo, Q. Liu, D. Zhong, Y. Hu, M. Yang, M. Bian and C. Hou, Microchim. Acta, 2019, 186, 1–10 CrossRef PubMed.
- J. Hou, C. Xu, D. Zhao and J. Zhou, Sens. Actuators, B, 2016, 225, 241–248 CrossRef CAS.
- W. Li, L. Ma, B. Wu, Y. Zhang and Z. Li, Anal. Methods, 2017, 9, 3819–3824 RSC.
- S. Mahalakshmi and V. Sridevi, Electrocatalysis, 2021, 12, 415–435 CrossRef CAS.
|
This journal is © The Royal Society of Chemistry 2022 |