DOI:
10.1039/D1SC04471K
(Perspective)
Chem. Sci., 2022,
13, 1526-1546
Natural product drug discovery in the artificial intelligence era
Received
13th August 2021
, Accepted 10th December 2021
First published on 13th December 2021
Abstract
Natural products (NPs) are primarily recognized as privileged structures to interact with protein drug targets. Their unique characteristics and structural diversity continue to marvel scientists for developing NP-inspired medicines, even though the pharmaceutical industry has largely given up. High-performance computer hardware, extensive storage, accessible software and affordable online education have democratized the use of artificial intelligence (AI) in many sectors and research areas. The last decades have introduced natural language processing and machine learning algorithms, two subfields of AI, to tackle NP drug discovery challenges and open up opportunities. In this article, we review and discuss the rational applications of AI approaches developed to assist in discovering bioactive NPs and capturing the molecular “patterns” of these privileged structures for combinatorial design or target selectivity.
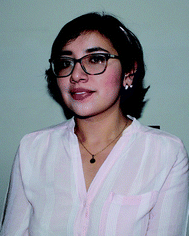 F. I. Saldívar-González | Fernanda I. Saldivar-González received her BSc degree in Chemistry Pharmacy and Biology (2017) from the National Autonomous University of Mexico (UNAM). She received the Master's degree in Chemical Sciences in 2019 under the supervision of Prof. José Luis Medina, after spending a research period in the group of Prof. Andrea Trabocchi at the University of Florence, Italy. She is currently a PhD student in Chemistry in the area of pharmacy where she develops her project focused on the design of virtual chemical libraries of antidiabetic compounds. |
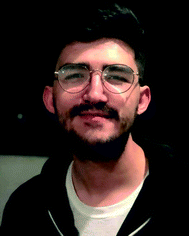 V. D. Aldas-Bulos | Victor D. Aldas-Bulos is a MSc student in Integrative Biology from Centre for Research and Advanced Studies of the National Polytechnic Institute (CINVESTAV-IPN) Advanced Genomics Unit (LANGEBIO) under the supervision of Dr Fabien Plisson since 2020. He received a BSc degree in Biotechnology from the Autonomous University of the State of Mexico (UAEMex) in 2021. His current research interests are in the development and discovery of antimicrobial peptides using artificial intelligence. |
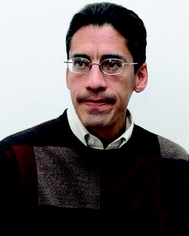 J. L. Medina-Franco | José L. Medina-Franco received his Ph.D. degree from the National Autonomous University of Mexico (UNAM). He was a postdoctoral fellow at the University of Arizona and joined the Torrey Pines Institute for Molecular Studies in Florida in 2007. In 2013, he moved to the Mayo Clinic and later joined UNAM as Full Time Research Professor. He currently leads the DIFACQUIM research group. In 2017 he was named Fellow of the Royal Society of Chemistry. His research interests include development and application of chemoinformatics and molecular modeling methods for bioactive compounds with emphasis on drug discovery. |
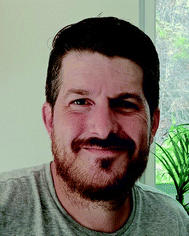 F. Plisson | Fabien Plisson obtained his Ph.D. degree from the University of Queensland (UQ), Australia, combining marine natural product chemistry, kinase drug discovery and chemoinformatics in partnership with biopharmaceutical company Noscira, Spain. In 2012, he carried out postdoctoral studies in peptide drug design at UQ, in collaborations with Pfizer and Protagonist Therapeutics. In late 2017, he moved to Mexico and joined the Centre for Research and Advanced Studies of the National Polytechnic Institute (CINVESTAV-IPN) Advanced Genomics Unit (LANGEBIO) as Assistant Research Professor. His research foci combine peptide drug design, drug discovery and artificial intelligence. |
Introduction
Artificial intelligence (AI) refers to the abilities demonstrated by computer machines (and human judgement) to ingest, process and recognise large and complex information patterns. AI has moved from theoretical studies to real-world applications, thanks to the revolution in high-performance computer hardware, extensive storage and accessible software. Machine learning (ML) is a subfield of AI, which englobes the ensemble of mathematical formulas and advanced statistics that humans apply through algorithms to treat such problems. ML algorithms can be executed at very large scales in the cloud at affordable costs and with ease. The digitization of data types (imaging, textual information, soundwaves, biometrics) from sensors or wearables into online public and proprietary databases have inundated the Internet, often referred to as “data deluge”.1 Those databases and scattered online information have been crucial for building practical predictive applications such as recommendation systems. Open-source toolkits, massive online courses, and educational videos on social media platforms have democratized the use of AI applications to many sectors, including finance, law, cybersecurity, transportation, manufacturing, entertainment, robotics, education, health, and services.2
Machine learning algorithms have steadily gained attraction within the pharmaceutical industry, we are seeing numerous supervised and unsupervised learning approaches being applied to the different stages of the drug discovery pipeline. For example, clustering methods have segmented cell type imaging, predicted protein target druggability, and supported de novo molecular design. Supervised learning techniques, i.e., regressions and classifications, identified possible targets for Huntington's disease. They speculated over the biological activities and absorption, distribution, metabolism, excretion, and toxicity (ADME/Tox) properties for drug design and many more applications.3 Lastly, generative algorithms are now supporting the molecular design of new chemical entities in medicinal chemistry.4–6 In 2019, the American company Insilico Medicine developed an AI system named GENTRL (for Generative Tensorial Reinforcement Learning) that successfully invented six kinase inhibitors of discoidin domain receptor 1 linked to lung fibrosis, in just 46 days.7
Natural products (NPs) are primarily recognized as privileged structures to interact with therapeutically relevant protein targets. Their structural diversity and biological activities still inspire the development of small molecules8 and macrocyclic drugs.9 NPs have dominated the sources of novel human therapeutics in the pharmaceutical drug pipeline in the mid-1970s. Two-thirds of the drugs originated from unaltered NPs (5%), NP analogues (28%), or contained NP pharmacophores (35%) between the 1980s and the 2010s.10 Despite being a proven source for modern small-molecule drug discovery, natural product research has declined at most major pharmaceutical companies. The main arguments are the time-consuming dereplication process, complex syntheses and high-throughput screening-unfriendly extracts.11,12 Moreover, many NPs present ADME and physicochemical properties, e.g., high degrees of stereochemistry, fused ring systems or rotatable bonds, that are beyond the current drug-like chemical space.13,14
The uniqueness of NPs continues to marvel laboratory and computer scientists alike. Not surprisingly, researchers have developed and adopted several computational methods throughout the drug pipeline; (1) to assist in the discovery and structural elucidation of bioactive NPs15,16 and (2) to capture the molecular patterns of these privileged structures for combinatorial design or target selectivity (Fig. 1).17–19 Over the years, chemoinformatic, bioinformatic, and other informatics-related disciplines have largely contributed to NP-based drug discovery. Their successful applications and limitations have recently been reviewed.20–23 Computational strategies involving artificial intelligence and machine learning algorithms have slowly made their ways into natural product research, a proven source of modern small molecule drug discovery. For example, in the early 2000s, AI applications mostly included the digitization of organic molecules, and dimensionality reduction techniques (i.e. principal component analysis, self-organizing maps) to map the NP chemical space. The following decade led to the development of ML binary classifiers to predict their biological functions. Recently, scientists have started to implement neural network architectures for genome mining, molecular design. Herein, this perspective article discusses the recent contributions of AI and ML algorithms to assist in the discovery of bioactive NPs and the design of NP-inspired drugs, and their future development.
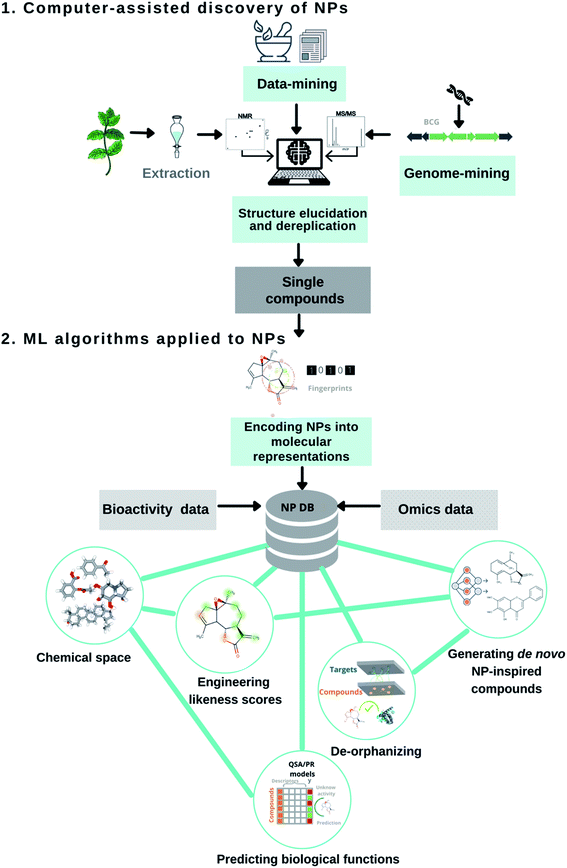 |
| Fig. 1 Overview of ML/AI algorithms that are implemented across the different stages of the natural product drug discovery pipeline. The pipeline presents two sections: (1) computer-assisted discovery of NPs (data-mining into traditional medicines and peer-reviewed articles, genome mining & structural elucidation and dereplication) and (2) machine learning algorithms applied to NPs (encoding into molecular representations, molecular descriptors, likeness scores, chemical space, predicting biological functions, de-orphanizing and generating de novo NP-inspired compounds). | |
Computer-assisted discovery of natural products
Data-mining into traditional medicines and peer-reviewed articles
Scientific compendium has long been documented into codices, dissertations, publications, patents, reports or laboratory notebooks. With an estimated 10
000 chemistry-related articles published every year, retrieving chemical information exceeds human readability, and many findings remain hidden. Machine-readable contents are critically needed. The recent transition from printed hard copies to digitized documents and restricted geographical locations to the World Wide Web has kick-started data-mining technologies. In the field of chemistry alone, many text-mining approaches monitor the 20
000 new compounds published in medicinal and biological chemistry journals every year.24 In 2020, Rajan and co-workers developed DECIMER, the ultimate Optical Chemical Entity Recognition software system using deep learning (DL) that recognized chemical structures from journal articles.25 Deep learning refers to a subfield of machine learning using neural network architectures with 3 or more layers. In the year prior, Tshitoyan and co-workers reported the discovery of “forgotten” thermoelectric materials from peer-reviewed articles published between 1922 and 2018. The authors first curated a corpus of 1.5 million abstracts from which they established semantic relationships using Word2vec, a technique for natural language processing (NLP). They built a ML model with word embeddings (vector representations of words) to predict the thermoelectric property for 1820 known and 7663 candidate materials.26 NLP is a branch of AI focused on understanding the interactions between computers and human languages. NLP methodologies extract, categorize, analyse words or sentences to get insights (e.g., knowledge graphs27) from unstructured documents. Beyond textual information in natural languages (i.e., English), NLP algorithms must process the many molecular representations associated with biomedical research, a domain referred to as BioNLP.28,29 To date, subfields of drug discovery; i.e., protein docking,30,31 protein–protein interactions32,33 or protein-disease associations34 have too benefitted from applying biomedical text mining. In contrast, NLP has shown limited applications to the discovery of bioactive NPs.
So far, BioNLP methodologies have predominantly deciphered ancient texts from disappearing traditional medicines to identify bioactive plants. Traditional medicine has stimulated the search for bioactive NPs from various sources and novel drugs throughout history. Early evidence referred to plants' medicinal use on clay tablets written in cuneiforms.35 Traditional Chinese medicine (TCM) has gained attention worldwide due to its role in discovering treatments for malaria36 and rheumatoid arthritis.37 In 2014, May and co-workers developed an algorithm that could screen, extract, select, classify and score information from ancient texts.38 Equipped with that technology, the authors monitored changes in the terminology used for specific diseases over time. They identified common treatments, and they eased the discovery of NPs in both Zhong Hua Yi Dian (ZHYD; Encyclopaedia of Traditional Chinese Medicine), the most comprehensive encyclopedia of TCM books, and Zhong Yi Fang Ji Da Ci Dian (Great Compendium of Chinese Medical Formulae), the largest compendium of herbal formulas.38 In 2015, Shergis and co-workers conducted a text-mining search within TCM codices, searching natural products as potential treatments for chronic cough, a by-product of cancer, obstructive pulmonary disease, tuberculosis, and asthma.39 Employing the keywords jiǔ hāi, jiǔ sòu, and jiǔ késòu for chronic/prolonged cough and keeping their terminological authenticity, the authors identified 331 compounds in the ZHYD including; 250 from herbal sources, 47 from animals and 34 from minerals.
Despite their originality, these semantic approaches carry many flaws and remain rudimentary. One of the main drawbacks in mining ancient texts is the changing paradigm and concept of medicine over the centuries. For example, the diagnosis and treatment systems in TCM originated from ancient philosophies such as the Qi theory (or the yin-yang theory) and the five elements theory. TCM practitioners call a syndrome a set of patient symptoms that may result from different diseases and be caused by different mechanisms, hampering the identification of treatments.40 Like traditional medicine, ethnobotanical explorations have contributed to discovering countless NPs.41 In 2014, Sharma and co-workers explored the Palau and Pohnpei Primary Health Care Manuals, traditional botanical accounts from Micronesian islands, aiming to pinpoint medicinal plants and their therapeutic usages.35 The authors first digitized the manuals with the Biodiversity Heritage Library to establish individual plants and therapeutic annotations. The extracted information was crossed with contemporary biomedical terminology using MetaMap42 (https://metamap.nlm.nih.gov/). This BioNLP tool employs computational linguistic techniques to identify equivalent terms in the Unified Medical Language System. The team discovered 129 unique plant species and over 700 treatment indications from the Primary Health Care Manuals. Biodiversity Heritage Library commonly reported 72 plant species; ten displayed comparative symptoms (i.e. diarrhea, pain, rash) resulting from venomous stings or pathogen infections.
Predicting chemical structures from microbial genomes
Rapid fractionations, hyphenated chromatography techniques, and bioassay screenings of natural sources such as plants, marine invertebrates or microbes have traditionally guided the discovery of bioactive NPs.43 The recent advances in genome sequencing have revealed the biosynthetic logic and genetic basis behind NPs of microbial origin and beyond.12,44,45 Enzymatic complexes such as polyketide synthases (PKSs)46 and nonribosomal peptide synthetases (NRPSs),47 or the ribosomally synthesized and post-translationally modified peptides (RiPPs)48 are behind the production of these secondary/specialized metabolites. Microbial genomes encode these multi-domain pieces of machinery as biosynthetic gene clusters (BGCs). Over the last decades, considerable efforts in bioinformatics, commonly referred to as the umbrella term “Genome mining”, recently reviewed,49–52 have enabled the discovery of cryptic BGCs within microbial genomes and the experimental characterization of novel NPs. ML algorithms and pattern-recognition approaches have partaken the genome-mining tools into two areas; (1) scrutinizing novel BGCs and (2) predicting chemical structures.
Biosynthetic gene clusters are traditionally discovered through a rule-based selection process, except for novel RiPPs. These peptides are typically identified from a limited set of known RiPP tailoring enzymes and precursor peptides (PPs). In 2017, Tietz and co-workers developed a multi-layer predictor enabling the discovery of lasso peptides.53 The same year, Mohanty's group created RiPPMiner, a multi-label Support Vector Machine (SVM) classifier that distinguishes between a dozen PP classes.54 Both approaches limited their training to specific RiPP classes. In 2019, de los Santos complemented the ML-guided discovery of novel RiPPs with NeuRiPP; the neural network architectures could identify known PPs and new PP-like sequences.55 The following year, three additional methods pitched the automated discovery of new ribosomal peptides. First, Merwin and co-workers created DeepRiPP, the tripartite pipeline employed natural language processing to capture a wider diversity of RiPPs independently from genomic context (NLPPrecursor). Their other components included Basic Alignment of Ribosomal Encoded Products Locally (BARLEY) and Computational Library for Analysis of Mass Spectra (CLAMS) that indexed biosynthetic loci to candidate RiPPs within a database of thousands of microbial extracts from genomic and metabolomic information.56 The authors applied DeepRiPP to analyze 65,421 sequenced bacterial genomes and identify 19,498 unique unknown RiPPs. Later, Kloosterman and co-workers reported two new bioinformatics tools to support the discovery of novel RiPPs; DecRiPPter (Data-driven Exploratory Class-independent RiPP TrackER)57 and RRE-finder (RRE stands for RiPP recognition element).58 The former applied an SVM with a custom database of RiPP-specific BGCs and PPs to prioritize genomic regions. The latter focused on finding RREs, BGC elements that participate in the RiPP biosynthesis encoding for discrete proteins or fused protein domains. Unlike DecRiPPter, RRE-finder capitalized on sequence similarity and protein homology; the tool either detected known RiPP classes using 35 custom profile Hidden Markov Models (pHMMs) (precision mode) or predicted novel RiPP classes with a modified version of the HHpred pipeline59 (exploratory mode). Both tools confidently identified novel RiPPs; thus, DecRiPPter discovered 42 new RiPP families, including the novel subclass V of lanthipeptides from 1295 Streptomyces genomes.
Finding novel chemical entities early on is critical to the drug discovery process. It could alleviate the costs and the experimental time associated with the dereplication of natural crude extracts (NCEs). Chemical novelty is also inherent to the intellectual property (i.e., patents) of pharmaceutical and biotechnology companies competing to develop new drugs in similar target/disease landscapes.60,61 In 2019, Hannigan and co-workers created DeepBGC, the deep learning framework utilized recurrent neural networks (RNN) to identify novel BGC classes followed by random forest (RF) classifiers to predict their biological activities (i.e., antibacterial, cytotoxic, inhibitor or antifungal). DeepBGC identified adequately many NP classes from predicted BGCs but the algorithm predicted poorly their biological activities, due to the lack of training examples.62 The following year, Skinnider and co-workers63 presented the fourth version of PRISM (stands for PRediction Informatics for Secondary Metabolomes, available at http://prism.adapsyn.com) that could predict the chemical structures for 16 classes of NPs from bacterial BGCs, including aminoglycosides, nucleosides, β-lactams, alkaloids, and lincosamides. PRISM4 employed 1772 HMMs and 618 tailoring reactions, reaching a high degree of chemical similarity between predicted structures and the authentic products of known BGCs. With cryptic BGCs, the tool predicted structural features of known NPs. The authors further set a library of 1281 BGCs and trained moderate SVM classifiers to predict the probability for a BGC to display one or more biological activities (i.e., antibacterial, antifungal, antiviral, antitumor, or immunomodulatory activity). The same year, Agrawal and Mohanty developed two RF classifiers that predicted macrocyclization patterns for PKs and NRPs.64 The first model predicted the capacity of a linear precursor to (or not to) adopt a macrocyclic structure, based on a training dataset made of 196 empirically known macrocyclic PK/NRP compounds and 162 linear chemical entities. The second classifier identified the accurate macrocyclic structure of a PK/NRP compound given its linear precursor, using the 196 macrocyclic compounds previously mentioned and all its theoretically possible macrocyclic structures. Finally, in 2021, Walker and Clardy revisited ML classifiers to predict the antibacterial or antifungal activity based on BGC-derived features. Alongside the development of moderate classifiers (i.e., 57–79% accuracy), which outperformed DeepBGC, the authors uncovered activity-associated BGC domains.65
Besides finding novel chemical entities, microbes have flourished as biofactories for the development of exogenous metabolites, peptides and proteins through recombinant DNA technology.66 As the connections between microbial BGCs and NPs grow, we can foresee that the future steps in metabolite engineering would involve hacking directly the genetic information of biosynthetic powerhouses like streptomyces67 to develop novel and complex NPs, hardly synthesizable and in more sustainable manner.
Automating natural product dereplication process
Early-stage discovery of NPs from organisms of all kingdoms is characterized by the repetitive extractions, subsequent chromatography/spectrometry-guided fractionations and purifications leading to single metabolites (or mixtures thereof) – the process is known as dereplication. One or more biological assays often guide the process to screen and prioritize the extracts, fractions and isolates containing the bioactive substances. Dereplication is lengthy, tedious and might face problems that would hinder the expected return on investments (time, equipment, human resources), such as discovering already known NPs, purifying supposedly novel structures in insufficient amounts, or screening natural crude extracts (NCEs) with high-throughput robotics.11,12,43 Scientists have strategized different approaches to reduce redundant NCEs with the early chemical profiling of (un-)targeted NPs.68,69 They have notably prioritized NCEs using state-of-the-art analytical chemistry techniques, i.e., gas/liquid chromatography (GC/LC), nuclear magnetic resonance (NMR) spectroscopy, mass spectrometry (MS), and combinations thereof.
The increasing data digitization has enabled the implementation of mathematical and statistical methods. The field of chemometrics has leveraged the multivariate statistical analysis of data from the aforementioned studies and from the optical radiation (i.e., infrared, visible and ultraviolet) for the rapid identification of known and unknown bioactive NPs from NCEs. In 2019, Cornejo-Baez and co-workers documented the most common statistical techniques used to study NPs.70 The list included both unsupervised (i.e., hierarchical cluster analysis, principal component analysis and discriminant analysis) and supervised ML algorithms (i.e., partial least squares, orthogonal projection to latent structures). Beyond NCEs, scientists have capitalized on ML algorithms to extract information from metabolomic data and generate new biological insights. In particular, supervised ML algorithms such as random forest, support vector machine (SVM), artificial neural network, and genetic algorithms have shown great potential in metabolomics research due to the ability to provide quantitative predictions.71 The implementation of these algorithms has facilitated analytical data processing, integrated omics data, and stimulated biological applications. For example, ML algorithms are used to integrate chromatogram peaks,72 predict retention time,73–75 or amputate missing data.76
With the growing volume of MS data, several metabolomics platforms arose such as the software MetaboAnalyst 5.0 (https://www.metaboanalyst.ca/).77 In 2016, Wang and co-workers presented the Global Natural Products Social Molecular Networking (GNPS, http://gnps.ucsd.edu).78 The platform organizes vast tandem MS datasets into visual molecular networks. Molecular networking (MN) uses nodes to display the high-resolution spectra, and edges to characterize the spectrum-to-spectrum alignments. The initiative is gaining attraction in NP dereplication79 as well as other applications related to the study of NPs.80 Overlapping or neighbouring nodes are synonymous with NCE replicates or NCEs with shared fragmentation ions. However, in absence of reference spectra in molecular databases, tandem MS datasets cannot be aligned and molecules cannot be identified. Alternatively, scientists have developed tools such as CSI:FingerID,81 MS2LDA82 and SIRIUS 4 (ref. 83) for small molecules, and VarQuest84 for peptides, that coupled tandem MS spectra to specialised molecular databases in order to identify NP substructures. Of the aforementioned bioinformatic tools, three utilised ML algorithms to match fragmentation ions with molecular substructures. First, CSI:FingerID81 (www.csi-fingerid.org) computed fragmentation trees from MS spectra and applied ML algorithms (multiple kernel learning, SVM) to predict the presence or absence of 1415 molecular fingerprints in unknown compounds. Each molecular fingerprint is scored and ranked using Platt probabilities. In result, CSI:FingerID matched NPs and NP substructures from a molecular structure database such as PubChem to the submitted spectra or fragmentation trees from either Agilent (N = 2055) or GNPS (N = 3868). The platform SIRIUS 4 derived from CSI:FingerID.83 The discovery platform MS2LDA82 (http://ms2lda.org/) implemented latent Dirichlet allocation (“-LDA”), an unsupervised method, originally used for text mining, to decompose tandem MS data (“MS2-”) into sets of co-occurring fragments or neutral losses (called Mass2Motifs). Those motifs were matched to a set of biochemical features (i.e., amino acids, nucleotides, conjugated acids, polyamines, carbohydrates) to deduce molecular ((sub)structures).
Tandem MS data alone remain insufficient to fully elucidate chemical structures, the last half-century has seen the elaboration of computer-assisted structural elucidation (CASE) expert systems. Two recent reviews provide a comprehensive and historical overview of these systems.85,86 CASE expert systems support the identification of an unknown chemical compound by matching its similar spectral properties to a list of potential candidates. Such systems were primarily based on one-dimensional (1D) and two-dimensional (2D) NMR spectra to elucidate the structures of NPs and organic compounds. In 2020, Reher and co-workers87 reported the first ML-driven tool called ‘Small Molecule Accurate Recognition Technology’ or SMART 2.0, for the rapid characterization of NPs from NMR spectra of NCEs. At the core of SMART 2.0, the team trained a convolutional neural network on a set of 53
076 2D-NMR spectra (i.e., HSQC – Heteronuclear Single Quantum Coherence spectroscopy) from NPs of the JEOL database and ACD Labs Predictor reduced to a 180-dimensional embedding space. The authors validated their application by discovering and fully characterizing a novel cytotoxic swinholide NP named symplocolide A from the NMR-based SMART mixture analysis of the filamentous marine cyanobacterium Symploca sp. Besides NMR experiments, several non-spectroscopic techniques, i.e., atomic force microscopy,88 “crystalline sponge” X-ray analysis,89 and micro-electron diffraction90 are starting to provide structural insights in combination with CASE expert systems.
Machine learning applied to natural products
Encoding natural products into molecular representations
Modelling and predicting the properties and bioactivities of NPs (or any chemical structure per se) primarily pass through their translation into computer-readable format(s), the so-called molecular representations (Fig. 2). Most representations encode chemical information for a specific use. Original generic and IUPAC names retrieve chemical compounds that share nomenclatures. Matching chemical structures based on their bidimensional molecular graph depictions was computationally demanding. Early molecular representations were designed for light-weight storage space of chemical information or efficient structural search. The simplified input line entry system (SMILES),91 SMILES arbitrary target specification (SMARTS, Daylight CIS and OpenEye Scientific Software) and international chemical identifier (InChI)92 were created to store and retrieve molecular information as well as identifying shared molecular features or substructures from databases. Novel molecular representations like DeepSMILES93 and SELFIES94 recently arose for their practical use in ML algorithms.
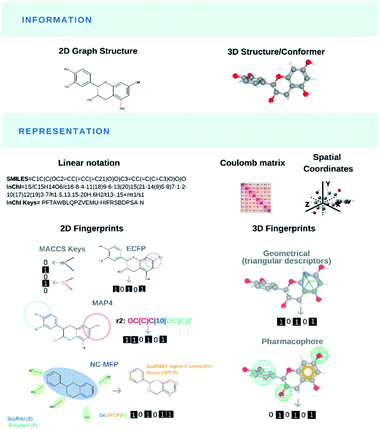 |
| Fig. 2 Molecular representations frequently used in NPs. | |
Chemical and biomolecular databases are central to many AI applications and they are commonly found across informatic-related disciplines.95 Chemical databases96 played an important role to improve the dereplication process of NPs using preassembled NP libraries and chemical fingerprinting (spectroscopic/chromatographic data, calculated physical properties). In the mid-1990s, public and private research entities have initiated several commercial databases from curated literature review including the generalist Chemical Abstracts Service by the American Chemical Society or the highly specialised MarinLit by the University of Canterbury, New Zealand, and now under the British Royal Society of Chemistry. However, the elevated costs and limited access to commercial databases have pushed numerous academic researchers to consider free and open-access options like ChemSpider. Few years ago, the number of NP databases in the public domain was very limited. But the renewed interest in NP research plus rapid advances in informatics and data sharing boosted the generation, and publication of NP collections in the public domain. In 2020, Soronika and Steinbeck reviewed 123 open-access and commercial databases, industrial catalogues, books and collections for NP information, that were still cited in the scientific literature after 2000.97 Many NP databases, commercial and open-access alike, are sporadically maintained by their creators. Less than half of these databases offered substructure searching using at least one of the aforementioned molecular representations, and many lacked stereochemical information. In result, the authors built the largest collection of open-access natural products named COCONUT (https://coconut.naturalproducts.net/) that compiled the structures and related information of over 400
000 non-redundant NPs. The same research group has continued developing and curating COCONUT and has released an improved version called LOTUS.98
The need for efficient substructure searching in growing chemical databases and reduced storage space have also led to the development of molecular fingerprints. Initial bitstring fingerprints denoted the presence (1) or the absence (0) of substructures as binary vectors.99,100 Subsequent topological fingerprints based on atom pairs,101 local circular neighborhoods and Extended Connectivity fingerprints (ECFP),102 Molecular ACCess System (MACCS) keys,103 to name a few, were specifically designed for bioactivity prediction and similarity analysis. Bidimensional fingerprints were implemented to compare the molecular similarity of NPs against synthetic chemical libraries. The similarity of any two molecules is measured using one of many distance metrics such as the Tanimoto coefficient.104–106 In their 1999 seminal paper, Henzel and co-workers107 converted 78
318 entries from the Database of Natural Products, 29
432 entries from the Bioactive Natural Product Database, 182
822 chemical compounds from the Available Chemicals Directory, 14
596 drugs and some synthetic compounds from Bayer AG into MACCS- and UNITY-format. NPs presented larger molecular weights and distinct heteroatom distributions, and over 40% of NP-derived pharmacophores were not represented in the synthetic libraries. In the two decades that followed, pharmaceutical companies and academic institutions have integrated bioactive NP scaffolds to their combinatorial drug design strategies, and they have created diversity-oriented synthesis (DOS), diverted total synthesis (DTS), biology-oriented synthesis (BIOS) and function-oriented synthesis (FOS) of NP-like libraries, as summarized by the many reviews.108–115 In parallel, computational scientists have used the aforementioned fingerprints indifferently between NPs and synthetic molecules to conduct successive structural similarity analyses,107,116–121 novel visual representations of the chemical space (see Mapping NPs in chemical space),121–132 and they generated new metrics, i.e., NP-likeness score or metabolite-likeness score (see Engineering likeness scores),133–141 to monitor the success of that endeavour. In 2020, Seo and co-workers developed NC-MFP as the first natural product molecular fingerprint for NP drug discovery.142 The same year, Capecchi and co-workers created the MinHashed atom-pair fingerprint reaching up to 4 bonds (or MAP4 for short), suitable for both drugs, NPs as well as macromolecules.143 Molecular representations have depicted small and large organic molecules indifferently, natural products and synthetic compounds, with the latest MAP4. The goal is to embrace a universal fingerprint to describe and search chemical space. In contrast, we also see emerging novel fingerprints like NC-MFP that promote the singularity of chemical entities, to possibly classify structures or predict biological activity. Both fingerprints will likely co-exist for specific domain applications.
In the early 2000s, researchers also created 3D fingerprints based on geometric distances144,145 and methods such as the rapid overlay of chemical structures (ROCS)146 to leverage spatial information and shape similarity. Tridimensional fingerprints have predominantly been used to match ligands from virtual screening experiments, based on shape similarity.147,148 Shape-matching has notably been used in scaffold-hopping, a process focused on discovering novel compounds by changing the core of known parent bioactive structures (see Generating de novo NP-inspired compounds).149–151 In 2013, Riniker and Landrum benchmarked several fingerprints for ligand-based virtual screening, where the authors concluded that simpler topological (bidimensional) fingerprints could retrieve structural information, making scaffold-hopping potentially obsolete.152 With respect to bioactive NPs, in 2017, Skinnider and co-workers presented an algorithm named LEMONS for the enumeration of hypothetical modular NPs. The authors leveraged their algorithm to compare different molecular similarity methods with the NP chemical space. Their results suggested that circular fingerprints with a retrosynthetic approach (GRAPE/GARLIC) would outperform the more conventional topological and structural fingerprints.153 In 2020, Chen and co-workers applied ROCS to interrogate the potential macromolecular targets of NPs, a process coined de-orphanizing (see De-orphanizing). They successfully identified the targets for many small molecules, but they struggled to find those of NPs and macrocyclic ligands.154 The overall advantages of 2D over 3D fingerprints remain to be demonstrated.155 However, it is generally accepted that these advantages depend on the application (goal of the study) and the specific types of fingerprints to be compared. More recently, 3D fingerprints have been reported to predict and rank the biological activities from chemical structures, the so-called Quantitative Structure–Activity/Property Relationship (QSA/PR) models.156
Vectorizing natural products with molecular descriptors
Besides fingerprints (frequently used by chemoinformaticians), computational chemists would use molecular representations to compute thousands of features (variables) known as molecular descriptors157 through well-defined algorithms. These descriptors grasp specific molecular features (e.g., atomic properties, size, shape, flexibility, polarity, lipophilicity, pharmacophore) that researchers could easily interpret. Molecular descriptors have been central to the development of predictive QSA/PR modelling. They have been essential to describe the distributions of NPs and synthetic compounds in low-dimensional representations of chemical space(s). At the turn of the 21st century, Lipinski and co-workers devised a set of empirical rules or guidelines, known as rule-of-five (Ro5), based on key molecular descriptors to rapidly identify orally available small molecules from screening campaigns and combinatorial libraries.158 Together with their structural similarity analyses, several computational studies used molecular descriptors to compare and describe the chemical spaces occupied by NPs, combinatorial chemical libraries, synthetic compounds and marketed drugs.121–123,125–132 Natural products and macrocycles,9 which were not initially considered to establish the Ro5 rules, have been found to violate one or more of these rules and yet, they exhibited oral bioavailability. In 2008, Quinn and co-workers attempted to establish a set of rules for NPs only.159 Several research groups puzzled have followed suit establishing a new set of empirical rules based on molecular descriptors, known as ‘beyond the Ro5’ (bRo5) to explain cell permeability and oral bioavailability of macrocycles.160–168
In a recent chapter, Grisoni and co-workers reviewed the impact of molecular descriptors upon chemoinformatic applications.169 The authors primarily introduced molecular representations beyond 3D leading to new features such as conformational flexibility, protonation states or orientations. Once the irrelevant features are removed (missing values, low variance threshold, multicollinearity) and the remaining descriptors are scaled, they are employed for similarity search or QSA/PR modelling. In the former application, the authors warned upon choosing the correct molecular descriptors, and the distance metrics to quantify (dis-)similarity between chemical entities. They advised that optimal molecular descriptors and distance measures could be selected following their quantification through the enrichment factor.170 In the latter application, identifying the best molecular descriptors to predict the biological activity/property of interest relies mainly on the stability, performance and interpretability of the algorithm in use as well as the metrics (e.g., accuracy, root mean squared error) for model evaluation.
Over the past decade, deep learning (DL) algorithms have been widely adopted in domains such as computer vision171 and natural language processing.172 Recently, DL models emerged for their applications to drug discovery and molecular informatics.173–176 At their cores, neural networks handle large datasets and capture the complex relationships between input features (e.g., 2D/3D fingerprints, molecular descriptors) and output decisions (e.g., biological activity, ADME/Tox). Despite their remarkable improvements over traditional ML algorithms, DL models are mostly built from a chosen set of features (i.e., molecular representations) rather than learning from “raw” chemical information. Existing convolutional neural networks (CNNs), used for image classification, are ill-suited for reading 2D graph depictions or 3D structures. Chemical entities such as NPs, synthetic small molecules or drugs could be depicted as molecular graphs of irregular sizes and shapes. Moreover, CNNs conventionally scan images in a specific order; the DL architectures must correctly read the atoms (i.e., nodes/vertices) and chemical bonds (i.e., edges) that molecular graphs are made of. Recent efforts have been achieved with the development of graph convolutional networks (GCNs), setting the state-of-the-art techniques to read the irregular and raw information coming from molecular graphs. So far, Sun and co-workers have reviewed their applications to four domains of the drug discovery pipeline; QSA/PR modelling, drug-target/drug–drug interaction, synthesis planning, and de novo molecular design.177 Several studies were reported to use large and general chemical datasets such as NCI dataset from the National Cancer Institute (https://cactus.nci.nih.gov/download/nci/), three datasets from European Molecular Biology Laboratory (https://www.ebi.ac.uk – SIDER, STITCH, ChEMBL) or the University of California San Diego's BindingDB (https://www.bindingdb.org/bind/index.jsp) to develop GCNs with domain-specific applications. Close to NPs, Sanchez-Lengeling and co-workers reported the odor profiles (138 labels) for some 5030 molecules from GoodScents perfume materials and Leffingwell 2001 PMP databases in 2019.178 On average, each molecule presented 1–15 odor labels. The authors could predict using GCNs all 138 descriptors for all molecules at once due to the observed strong correlations between structures and odor labels. GCNs remain to be explicitly applied to NP databases like COCONUT or LOTUS (vide supra).
Mapping natural products in chemical space
The chemical space is the geometric space defined by all the possible chemical compounds, their structural and functional properties. An NP chemical space refers to the space occupied by a set of known NPs. Visualizing this high-dimensional space through human-readable graphical representations (of one to three dimensions) has been critical to decision-making and advances in the drug discovery process.179,180 One of the most common ways to generate visual representations of the chemical space is using coordinate-based representations that often require reducing the number of dimensions. Transforming high-dimensional data into a smaller set of dimensions to better understand and interpret results is known as dimensionality reduction, a technique familiar to many AI projects.181 Over the last two decades, numerous research groups have implemented different dimensionality-reduction techniques to explore NP chemical space, extensively reviewed elsewhere.128,182–184 Besides mapping chemical spaces, dimensionality-reduction techniques expose structure–activity/property relationships (SA/PRs) between compounds and compound datasets. The increasing amount of data stored in chemical datasets makes the visualization of SA/PRs a challenging endeavour.185 Finally, dimensionality-reduction techniques help define the applicability domain of QSA/PR modelling, a specific region in the underlying chemical space where the model predictions are considered reliable. In that application, dimensionality-reduction techniques identify outliers and generate robust models. Overall, three techniques are commonly employed to either map the chemical space, define its limitations or exhibit SARs/SPRs; principal component analysis (PCA), t-distributed stochastic neighbour embedding (t-SNE), and self-organizing map (SOM).
Early accounts to support the analysis, navigation and comparison of chemical space(s) include the works by Schneider and co-workers introducing SOMs to NPs and drugs with scaffold architecture and pharmacophores.120,122 In 2003, Feher and Schmidt117 compared the property distributions of drugs, NPs, combinatorial libraries using PCA. Larsson and co-workers developed ChemGPS-NP,124 a PCA-like representation to compare NP libraries like WOMBAT121 with their biological activities. Waldmann and co-workers have charted the NP chemical space with SCONP,123 and ScaffoldHunter.126 Both tools explore the relationships between the more and more complex scaffolds and their biological activities through the intuitive hierarchical organization of scaffold libraries arranged in tree-like maps.125,127,129,130,132 These tree-like arrangements led the authors of this review to develop the ChemMaps.186 In 2020, Reymond's group embedded chemical spaces with very large dimensions into two-dimensional trees named TMAPs187 along with their recently reported fingerprint MAP4 (ref. 143) to analyze the similarity between 25
523 NPs of bacterial or fungal origin.188 Sánchez-Cruz and co-workers also implemented TMAPs to display NP databases, synthetic compound collections, as well as NP-based fragment libraries.189 The same year, Chávez-Hernández and co-workers applied TMAPs to compare the chemical spaces of 382
248 NPs from the database COCONUT (vide supra), molecules from the ‘dark chemical matter’, and other datasets.190 Similarly, the authors compared 52
630 molecular fragments generated from COCONUTs NPs with 14
001 fragments from dark chemical matter. They concluded that the NPs (complete compounds and fragments) largely delineated the chemical space.191 Of note, fragment libraries of NPs can be very useful for the rational fragment-based design of the so-called “pseudo-NPs”.192 With regards to graphically evaluating the predictive reliability of QSA/PR models, Majumdar and Basak used robust PCA to define a reliable predictive space of 508 chemical mutagens in 2016.193 The same year, Aniceto and co-workers introduced reliability-density neighbourhoods.194 Last year, Plisson and co-workers combined unsupervised multivariate outlier detection methods with a t-SNE manifold to delineate the limitations of their hemolytic QSA/PR models. The authors applied their methods to discover novel (non-)hemolytic antimicrobial peptides of natural origin.195
Engineering likeness scores
Natural products adopt numerous shapes and ring systems. They contain several oxygens but fewer nitrogen, sulfur, and halogen atoms than synthetic counterparts. Their structural complexity includes a high fraction of carbon sp3 atoms, stereogenic centres, and multiple hydrogen-bonding functional groups (donors and acceptors).107,116,117,122,196–198 Small NPs are intrinsically rigid198 whereas large NPs (more than 500 Daltons), in particular macrocycles,9 present a higher degree of flexibility, which confer to both sized molecules optimal affinity and specificity to bind to proteins and protein–protein interactions. This optimization process has been strongly attributed to the coevolution or complementary chemical design between NPs and specific protein targets to benefit the producer's survival fitness.199,200 Consequently, NPs and their derived physicochemical properties are regarded as privileged features supporting their total syntheses and the design of bioactive compound libraries.
Computational studies have contributed to the design of focused compound libraries by creating new scoring measures to quantify how similar a compound is to the chemical space. In machine learning, generating novel relevant feature(s) is referred to as feature engineering. In 2008, Ertl and co-workers developed a Bayesian measure named NP-likeness score that quantified the similarity of a compound according to the characteristic structural fragments in NPs.133 The authors compared NPs, synthetic molecules (SMs) and drugs from DrugBank based on the unidimensional distributions of their NP-likeness scores. They could also identify common building blocks to both NPs and drugs. Three years later, Jayaseelan, Ertl and co-workers implemented an open-source, open-data version of the scoring system.201 And in 2019, Soronika and Steinbeck created the NaPLeS web application (http://naples.naturalproducts.net) that computes the NP-likeness score for chemical libraries.202 Alongside Ertl's original NP-likeness score, Yu reported an alternative approach to quantify NP-likeness, based on Extended Connectivity Fingerprints (ECFP).203 Recently, Chen and co-workers developed and validated new ML models for the discrimination of NPs and SMs for the quantification of NP-likeness.204 NP-scout is the web application derived from this work that computes the probability of a molecule to be a NP based on its physicochemical properties, Morgan2 fingerprints, and MACCS keys. In addition, NP-scout allows visualizing atoms in molecules that make decisive contributions to the assignment of compounds to any class by integrating similarity maps.
Similarly to the NP-likeness score, ML applications have contributed to rationalization of alternative scoring systems to narrow large chemical compound libraries with metabolite-likeness,135,137,138,141,205,206 lead-likeness,207,208 or drug-likeness209,210 profiles. Such concepts in more sophisticated abstract terms have allowed the elaboration of models that could be applied for the identification of drug-like NPs beyond the application of empirical rules. Recently, Marshall and co-workers introduced the first intrinsic measure of molecular complexity, called the molecular assembly index (MA).211 The team developed the MA index to easily track complex molecules in abundance using mass spectrometry, and to support the existence of living producers within our cryptic terrestrial ecosystems or beyond alien exoplanets. To illustrate an application of the molecular complexity index, the authors retrieved 2.5 million small molecules (less than 600 Daltons) from Reaxys® database (https://www.elsevier.com/solutions/reaxys), and compared the MA distribution (1–25) between four libraries; NPs, industrial compounds, metabolites, and pharmaceuticals. Their results showed that the MA index is mostly constrained by mass, and all libraries exhibited a wide range of values. Moreover, the index estimated well the fragmentation complexity in MS/MS spectra from different biological samples. Beyond its application to identify life in outer space, one might divert the original purpose of the molecular complexity index as a fitness function to optimize the design of NP-inspired drugs.
Predicting biological functions
Bioactive NPs are present in small amounts in natural crude extracts (NCEs, μg to few mg), sometimes they are insufficient to conduct biological evaluations in multiple and successive phenotypic or target-based assays. Traditional bioassay-guided fractionation looks at a handful of biological responses at times, the fractions that do not respond to the assay(s) are considered inactive, the NPs within are left out, and their biological profile(s) remain unresolved. Atanasov and co-workers recently discussed the roles of genome mining, metabolite engineering and cultivation systems to tackle that recurrent issue.12 On the ground, research laboratories stock their purified NPs and NCEs in freezers (diluted at different concentrations or lyophilized) to maximize their future screening campaigns. Some countries have created national chemical repositories, such as France's Chimiothèque Nationale (https://chembiofrance.cn.cnrs.fr) and Compound Australia (https://www.griffith.edu.au/griffith-sciences/compounds-australia), to facilitate the interactions between chemical and biological laboratories. In silico, structure-based approaches (i.e., docking and virtual screening) and ligand-based approaches (i.e., QSA/PR modelling) predict the biological or ADME/Tox profiles of untapped chemical structures. Quantitative Structure–Activity/Property Relationship (QSA/PR) models use ML algorithms, mainly regressors and classifiers, to link the biological activity or physicochemical property of interest with changes in chemical moieties. In the past half-century, QSA/PR modelling has risen from a niche area of computational/theoretical chemistry to one of the major strategies to monitor large chemical libraries with applications in drug design, quantum mechanics, materials, and nanomaterials science, regenerative medicine and environmental toxicity.212
The application of ML algorithms to predict the biological activities of NPs is new; most models were developed in the last 5–10 years.213,214 Binary classification models predominate the list of ML algorithms for NP biological activity prediction (as active or inactive). Early classifiers include the development of a linear discriminant analysis (LDA) model using topological descriptors for the search of new anti-inflammatory NPs from MicroSource,215–217 and two RF classifiers using CDK descriptors218 to discover antimicrobial and anticancer agents among 1194 marine and microbial NPs from AntiMarin database.219,220 In 2016, Dai and co-workers predicted the anticancer properties for 5278 out of 21
334 plant-derived NPs from TCM.221 The authors used their in-house web server CDRUG222 based on a method coined relative-frequency weighted fingerprints (RFW_FP) and a hybrid score to compare molecular similarity. Between 2017 and 2020, Rayan and co-workers introduced the iterative stochastic elimination (ISE) optimization for the discovery of bioactive NPs; they reported NPs for anticancer,223 antidiabetic,224 anti-inflammatory,225 antibacterial,226 and antifungal227 activities. In all examples mentioned above, the authors constructed binary classifiers using sets of approved drugs with the biological activity of interest as the active class, and 2892 NPs as the inactive class. The ISE algorithm scores iteratively the variables (i.e., physicochemical descriptors) and combinations thereof with the biological activity. The common NPs were not inactive per se; rather, their biological activity was either ignored or unknown. Rayan and co-workers assumed these false negatives in the training set might have a minor effect.223 Noteworthy, the authors did not consider the clear physicochemical differences between NPs and drugs as detrimental factors for their good model performances. In 2018, Egieyeh and co-workers trained several binary classifiers from a dataset of NPs with in vitro antimalarial activity and applied their best models (RF and Sequential Minimization Optimization (SMO) with 82.8% and 85.9% accuracy, respectively) against 450 NPs from InterBioScreen chemical library.228 The same year, Onguéné and co-workers profiled in silico toxicity of 806 African plant-derived NPs from three databases curated for their antimalarial and anti-HIV properties (p-ANAPL, AfroMalariaDb, and Afro-HIV).229 The team implemented the knowledge-based predictive software Derek230 and the Cambridge University small-molecule pharmacokinetics prediction (pkCSM) web server.231 The former detected toxic sub-structures in small molecules, and the latter assessed the ADME/Tox profiles from graph-based structural signatures. The pkCSM predictions used RF and Logistic Regression algorithms for classification tasks, GP and Model Tree for regression tasks.231 The following year, Dias and co-workers illustrated the emergence of computational multi-target drug design232 with the development of two QSA/PR models to discover novel antibiotics against methicillin-resistant Staphylococcus aureus (MRSA).233 The first model was a modest regression model trained from 6645 anti-MRSA compounds with molecular descriptors to predict the negative decimal logarithm of minimum inhibitory concentration (pMIC) value against MRSA.
The second model predicted the antibacterial activity using the 1D-NMR data (1H and 13C) from marine samples (crude extracts, fractions and pure compounds) with 77% accuracy. In 2020, Yoo and co-workers developed the first DL-driven multi-classification algorithm to identify the medicinal uses of NPs for 15 diseases.234 The authors trained their model from a heterogeneous dataset of 4507 NPs and 2882 drugs, and 686 variables derived from PubMed text mining, molecular interactions features and physicochemical descriptors. The algorithm predicted 31 NPs and their possible uses in 15 phenotypes, including neurological disorders (Alzheimer's disease, Parkinson's disease, pain, stroke), heart problems (failure, myocardial infarction), infectious diseases (bacterial, urinary tract, skin), and autoimmune diseases (rheumatoid arthritis). Finally, in 2021, Liu and co-workers reported a DL algorithm called pretrained self-attentive message passing neural network (P-SAMPNN) to discover novel and potent anti-osteoclastogenic NPs.235
Most QSA/PR models employed (binary) classification algorithms to predict phenotypic responses such as anticancer or anti-inflammatory activity. Dias and co-workers created the first regression model to predict a phenotypic response, the pMIC value of a compound with antibacterial activity against MRSA.233 Very few research groups have ventured into developing models, regressors and classifiers, to predict the biological activity against specific protein targets, which is primarily due to the heterogeneity of biological information (e.g., MIC, IC50, EC50, Ki, % inhibition) in databases, and the variety of biological assay conditions these results came from. All the following models were developed to prioritize the virtual screening of sizable chemical libraries. The earliest target-based QSA/PR model could be attributed to Rupp and co-workers for discovering NP-derived Peroxisome Proliferator-Activating Receptor γ (PPARγ) activators for type 2 diabetes mellitus.236 The authors trained Gaussian process (GP) regression models with 144 PPARγ synthetic ligands and their pKd values. The compounds were encoded using molecular descriptors (i.e., 2D properties, topological pharmacophores, fragment counts), structure graphs (i.e., bond types, pharmacophores types) and combinations thereof, leading to 16 different GP models. Besides classical performance metrics, the authors evaluated their models using the so-called fraction of inactive among top-20-ranked compounds or FI20. They selected the 30 top-ranked compounds from the Asinex and Platinum collections (http://www.asinex.com), including ten compounds from the model with the best FI20 score. A total of eight displayed moderate-high and selective agonistic activity towards PPARα or PPARγ activation assays. One of their hits, the moderate yet selective PPARγ agonist compound 8 is a derivative of truxillic acid.236 In 2016, Sun and co-workers constructed five inductive logic programming (ILP) models to predict NPs that inhibit Sirtuin 1 (SIRT1), a promising target to treat type 2 diabetes and cancer.237 The first “inhibitor” model used 179 SIRT1/2 inhibitors (IC50 < 50 μM), whereas the “activator” model was made from 51 activators with EC50 < 2.15 μM. A third “differential” model compared SIRT1/2 inhibitors and activators. Two additional models estimated inhibitor binding energy and inhibitor affinity to SIRT1. The inhibitor models prioritised the virtual screening of 1.4M TCM compounds, leading to twelve candidates for AutoDock Vina software. In 2018, Pang and co-workers developed two classifiers (Naive Bayesian – NB, Recursive Partitioning – RP) to identify NPs with agonistic activity against estrogen receptor α (ERα), a protein target for breast cancer.238 The authors employed 9075 ERα agonists (IC50 < 10 μM) from the BindingDB and DUD-E databases and a suite of 2–3D physicochemical descriptors. The original dataset was divided into a 60:40 train/test split (6556:2519) with an imbalanced representation of active (2075) and inactive (7000) compounds. Both NB and RF classifiers showed good performances with a clear bias towards the inactive class in both training and testing sets. The authors applied their two best models to a library of 13
166 NPs; 393 NB-derived candidates, 193 RP-derived candidates, 162 NPs were commonly found in both sets. All candidates were docked against ERα (PDB ID: 3ERT) using the docking programs LibDock and CDOCKER, leading to the discovery and biological evaluations of eight NPs with antiestrogenic effects.
Besides their cost-effectiveness and time economy, many ML models have inconveniently incorporated drugs and synthetic compounds into their training sets, affecting the performances and the applicability domains of NP bioactivity predictors. For example, in 2017, Zhang and co-workers developed blood–brain barrier (BBB) permeability models that were applied to Traditional Chinese Medicine (TCM).239 Their preliminary models, based on a synthetic chemical library, performed poorly. Their subsequent models (SVM, RF, Naïve Bayes, and probabilistic neural network), integrating an NP dataset, showed an overall 90% accuracy. Additional in vitro evaluation further validated the BBB permeability predictions for 25 out of 32 TCM molecules. Alternatively, in 2019, Plisson and Piggott created good BBB permeability models based on ensemble classifiers built from 448 disclosed small molecules,240 that they applied to 471 marine NPs exhibiting kinase inhibition.241 The authors further implemented univariate Mahalanobis distance measures to define the applicability domain of their models, leading to the discovery of 13 marine-derived kinase inhibitors with appropriate physicochemical characteristics for BBB permeability. These strategies are mainly due to the lack of experimental data on the activity of NPs and the difficulty of representing compounds of natural origin in linear notations. Inadequate molecular representations could impact the performances of ML models as well.169,214 To date, the same representations define any organic molecule, drug and NP alike. Current proposals to address these issues include integrating experimental NP data to training sets, harmonizing NP bioassay results in public databases, applying ML algorithms that deal with small and imbalanced datasets, and creating NP-specific molecular representations.213 Finally, the democratization of AI/ML algorithms without proper training and expertise has also led to a surge of malpractices in ML modelling and chemoinformatics, with numerous non-reproducible QSA/PR models. Computational experts remind the scientific community about the best practices to adopt in QSA/PR and ML modelling.242–244
De-orphanizing
We rarely know the native binding targets of NPs. Moreover, most bioactive NPs are discovered through phenotypic assays, where their (protein) drug targets remain elusive. Recent advances in screening technologies245 and novel laboratory strategies help to identify their plausible modes of action,246–248 a process known as “target fishing.” In parallel, growing computational efforts for ligand-based target fishing include developing ML models and web servers to analyze the medicinal potential of the many NPs annotated in public chemical databases.249 These tools represent an opportunity to “de-orphanize” NPs by predicting their macromolecular (protein) targets. De-orphanizing predictors of NP drug targets typically employ supervised or semi-supervised ML algorithms trained with combinations of labelled and unlabelled features such as structural representations and types of interactions important for NP pharmacological effects.250
Several examples of web servers recently developed with ML methods for ligand-based target fishing are described in Table 1. The majority of these servers rely on chemical similarity searches. The PASS (Prediction of Activity Spectra for Substances) software is one of the earliest attempts to predict thousands of biological activities from two-dimensional chemical structures using molecular fragment descriptors.251 Applications of the PASS web server in the study of NPs have been extensively described.252 Recently, PASS was implemented in the SistematX Web Portal to profile a large NP database of Brazil.253 In a similar context, the SEA (similarity ensemble approach) server compares the structural similarity of a query molecule to a group of compounds of a potential target and has evaluated the statistical significance of the resulting similarity score.254 This server has been validated with NPs such as miconidine acetate (main metabolite of the Brazilian plant Eugenia hiemalis),255 and the physalins A, D, F and G.256
Table 1 All different ML algorithms/tools used to predict molecular targets of NPsa
Tool |
Algorithm(s) |
Application(s) |
Ref |
kNN: k-nearest neighbors; LoR: logistic regression; MLP: multilayer perceptron; MST: minimum spanning tree; NB: naive Bayes; RF: random forest; SOM: self-organizing map.
|
PASS (prediction of biological activity for substances) |
NB |
It predicts over 3500 pharmacotherapeutic effects, mechanisms of action, interaction with the metabolic system, and specific toxicity for drug-like molecules on the basis of their structural formula |
251
|
SEA (similarity ensemble approach) |
Kruskal algorithm of MST |
It relates proteins based on the set-wise chemical similarity among their ligands |
254
|
SPiDER (self-organizing map-based prediction of drug equivalence relationships) |
SOMs |
Useful to identify innovative compounds in chemical biology, and help investigate the potential side effects of drugs and their repurposing options |
257
|
TiGER (target inference GEneratoR) |
Multiple SOMs |
It performs qualitative predictions of up to 331 targets |
258
|
DEcRyPT (drug–target relationship predictor) |
RF |
It deconvolves phenotypic hit targets and accurately predicts affinities |
258
|
STarFish |
kNN, RF, MLP and LoR |
It considers small molecule binding to 1907 targets and its performance on natural products target prediction is explicitly considered |
259
|
However, these tools might not predict the biological targets of structurally intricate NPs because of their somewhat different molecular constitution compared with that of synthetic drugs. In 2014, Reker and co-workers presented SPiDER (self-organizing map-based prediction of drug equivalence relationships) to tackle this problem. This approach relies on self-organizing maps (SOMs), a clustering approach that uses pharmacophore correlations and physicochemical properties to map the relationships between chemical compounds.257 SPiDER was adapted to predict the targets of natural products with more complex and challenging structures, such as the macrocyclic archazolid A (ArcA),258 resiniferatoxin,259 (−)-englerin A260 and doliculide.261 The authors demonstrated that by deconvoluting the macrocyclic structures into fragments, assuming that the bioactivity fingerprint could be partly stored into those fragments and subsequently used them as surrogate structures for processing SPiDER, natural product-derived fragments (NPDFs) may help for the prediction of macromolecular targets of their corresponding parent NPs. In 2016, Keum and co-workers created good ML classifiers to predict the interactions between orphan herbal compounds and several protein targets (i.e., GPCRs, ion channels, transporters, receptors, enzymes).262 In 2017, Schneider and Schneider presented TIGER (Target Inference GEneratoR) in subsequent development, a chemocentric computational method for target prediction that leverages a consensus of two SOMs with slightly modified descriptors.263 TIGER scores each target unlike previous approaches, where higher score values suggest greater confidence in the prediction. TIGER was validated for the target prediction of resveratrol,263 (±)-marinopyrrole A264 and (−)-galantamine.265 In 2018, Rodrigues and co-workers developed an orthogonal ML workflow called DEcRyPT (Drug–Target Relationship Predictor) based on RF regression to deconvolve phenotypic hit targets and accurately predict affinities. DEcRyPT was used successfully to identify β-lapachone as an allosteric modulator of 5-lipoxygenase.266 The following year, the team reported the alternate method DEcRyPT 2.0, including y-randomization,267 that predicted with more robustness the biological target(s) of celastrol.268 In 2019, Cockroft and co-workers developed the online target prediction tool named STarFish, which was trained with a synthetic composite dataset consisting of 107
190 pairs of compound-targets (88
728 unique compounds and 1907 unique targets) and tested on an NP dataset containing 5589 pairs of compound-targets.269 STarFish uses a stacking approach, where logistic regression is taken as a meta-classifier that combines model predictions and can produce better predictions than individual models. Furthermore, a multilabel classification approach is taken to emphasize the consideration of polypharmacology during training. Beyond fishing the biological targets of NPs, de-orphanizing ML approaches provide new opportunities for drug repurposing/repositioning.270,271
Generating de novo natural product-inspired compounds
Natural products contain privileged features to interact with (protein) drug targets that have supported their uses as starting, intermediate or final products for the design of synthetic compound libraries. Despite these advantages, most NPs do not fulfil the drug discovery paradigm in terms of toxicity, selectivity, lipophilicity and bioavailability, and require medicinal chemistry interventions (e.g., 92% of NP-inspired drugs were altered between 1980 and 2014 (ref. 10)). Their complex structures (i.e., stereogenic centres, heteroatom-containing functional groups, fused rings) have often handicapped the synthetic routes to analogues and their structure–activity/property relationship (SA/PR) studies. Moreover, patenting bioactive NPs in their original form might not be authorised where the compounds were discovered.272 Consequently, multiple synthetic strategies have led to designing lead structures that would preserve the NP privileges.192,273,274 The first strategy is the biology-oriented synthesis (BIOS), where NPs are taken as templates to generate synthetically accessible derivatives and mimetics.275,276 The diversity-oriented or diverted total synthesis (DOS/DTS) focuses on populating the underexplored chemical space by creating new chemical structures with NP-like pharmacophores.277–279 The complexity-to-diversity strategy (CtD) synthetically mimics enzymatic processes by chemically functionalizing and distorting NPs to structurally diverse compound collections.280,281 Finally, the function-oriented synthesis or FOS refines the BIOS concept to recapitulate or fine-tuning the function of a biologically active lead structure to obtain simpler scaffolds, increase their ease of synthesis, and achieve synthetic innovation.282,283 Waldmann and co-workers have recently introduced a set of principles to guide the generation of the “pseudo-NPs”, small molecule compound libraries that combine two or more NP-derived fragments (NPDFs), leading to unprecedented scaffolds. Their chemoinformatic analyses suggested that pseudo-NPs shared more characteristics (sizes, shapes, lipophilicity) with drugs than other libraries such as BIOS and ChEMBL NPs.192,284,285
Computer-aided de novo design tools286,287 have appeared alongside the synthetic strategies over the last 20 years and have recently started to generate NP-like compounds. One FOS-inspired approach automatically morphs NPs into synthetically accessible and isofunctional compounds. First, several chemical candidates are produced in silico using a generative algorithm. The compound generation is steered by optimizing the topological similarity between the candidates and a NP template. Subsequently, the computational prediction of the biological target is carried out. In 2016, Friedrich and co-workers reported the computational de novo design of the natural anticancer agent (−)-englerin A, the NP and its mimetics were all identified as potent TRPM8 agonists (TRPM8 stands for transient receptor potential calcium channel subfamily M (melastatin) member 8).288 In 2018, Merk and co-workers successfully applied two generative algorithms to design fatty acid mimetics as new modulators of retinoid X receptor (RXR) and peroxisome proliferator-activated receptor (PPAR). The authors first used their in-house de novo design algorithm named DOGS289 (Design Of Genuine Structures) to generate and test NP mimetics from dehydroabietic acid, isopimaric acid and valerenic acid, three known RXR agonists.290 In 2021, Friedrich and co-workers revisited the computational de novo DOGS design by generating (±)-marinopyrrole A mimetics as moderate-high inhibitors of cyclooxygenases COX-1 and COX-2.291
In the last five years, scientists have started to design de novo organic chemical entities for material science and drug discovery applications using generative AI.4–6,292,293 Early applications of DL algorithms to produce new molecules include the use of recurrent neural networks (RNN) with long-short term memory (LSTM),294 autoencoders,295,296 generative adversarial networks297 and reinforcement learning.298 In 2018, Merk and co-workers implemented LSTM-RNN to produce new RXR and PPAR agonists inspired by 25 fatty acid mimetics.299,300 The same year, Mȕller and co-workers adapted LSTM-RNNs to generate novel peptide sequences inspired by natural antimicrobial peptides, exempted from repetitive cysteine and proline residues.301 In 2019, Zheng and co-workers developed a de novo molecular generator to make quasi-biogenic compounds named QBMG.302 The authors used RNN with a gated recurrent unit (GRU) and trained the generator with 153
733 biogenic compounds from the ZINC15 library.303 In 2021, Bung and co-workers applied transfer learning and reinforcement learning to create novel small molecule inhibitors of the protease 3CL from the severe acute respiratory syndrome virus 2 (SARS-CoV-2). The team pre-trained the deep neural network architecture with 1.6 million drug-like small molecules from ChEMBL and generated 42
484 molecules. They filtered the generated dataset based on physicochemical descriptors, rule-based criteria, and virtual screening scores resulting in 33 new chemical entities, including two aurantiamide-like compounds.304
Scaffold-hopping comes as an alternative strategy to computer-aided de novo design. The computational process, widely used in medicinal chemistry, aims at identifying chemical compounds with different molecular backbones that share similar activity/property space.149–151 Scaffold-hopping applied to NPs means finding simpler NP mimetics, but their structural differences with synthetic compounds could hamper the computational process. In 2018, Grisoni and co-workers introduced a molecular similarity approach that hopped from complex NP scaffolds to simpler isofunctional synthetic mimetics while retaining their biological functions. The authors hopped from structures to structures using their in-house descriptors called weighted holistic atom localization and entity shape (WHALES) for the computational search. They exemplified their strategy using four natural cannabinoids as queries leading to seven novel biologically active compounds; three compounds became cannabinoid receptor modulators.19 The following year, the team employed WHALES descriptors in conjunction with SPiDER and TIGER tools in a multitarget ligand design approach. Grisoni and coworkers identified eight small molecules inspired by the natural product (−)-galantamine exhibiting multiple target activity profiles against enzymes and protein receptors related to Alzheimer's disease.265
In addition to the generation of simpler NP-inspired compounds, the total syntheses of NPs305 and NP analogues306 are the livelihoods of many chemists and mesmerize the field of organic chemistry, driven by synthetic efficiency, elegance and quality. Training algorithms to support the autonomous synthetic planning of complex NPs has also evolved over half a century since LHASA,307 giving rise to multiple softwares.308 Artificial intelligence has integrated computer-aided synthetic planning (CASP) such as Chematica/Synthia, a hybrid human-AI system.309,310 Artificial intelligence has also informed the fully automated platforms for the synthesis of NPs.311,312 The next paradigm shift aims to combine CASP with ML-driven models for predicting biological activities, biological targets, ADME/Tox properties to automate the discovery of biologically active NPs and the de novo design of NP-inspired drugs.180
Conclusions
Natural products have originated multiple drug discovery success stories, yet the many challenges associated with their discovery or their design – minute amounts, unfriendly extracts, unknown biological functions, missing biological targets, difficult chemical syntheses, complex SA/PR studies, undruggable ADME/Tox properties – led to the decline of NP drug discovery programmes. However, laboratory and computer scientists alike continue to marvel at NPs for their unique privileges to bind biological drug targets specifically for their therapeutic potentials. Artificial intelligence and machine learning algorithms have slowly integrated different stages of NP drug discovery (1) to assist discovering and elucidating bioactive structures and (2) to capture the molecular patterns of these privileged structures for molecular design and target selectivity. About the early discovery of bioactive NPs, natural language processing and text-mining tools have barely deciphered the many bioactive compounds hidden or forgotten in codices of traditional medicines and peer-reviewed articles. In contrast, ML-fuelled applications in genome mining and dereplication processes have reduced the screening of redundant producers or natural crude extracts and accelerated the discovery of novel natural chemical entities such as the subclass V lanthipeptides. With reported NPs, the inclusion of new AI technologies started at the turn of the 21st century with encoding their structures into computer-readable formats (i.e., 1–3D molecular representations, molecular descriptors) and generating chemical space visualization methods to manage and interpret the many naturally occurring compounds present in publicly available databases. The successive application of dimensionality reduction techniques such as PCA, t-SNE, SOM and lately TMAP has provided the means to compare NP privileged features (i.e., physicochemical properties, fragments, likeness scores) with those of drugs and synthetic libraries. In the 2010s, the development of ML models, i.e., regressions and classifications, to predict the biological activity/property of NPs has pushed candidates towards more advanced stages of drug development. It is worth noting that many predictions might inadvertently discard several bioactive NPs due to their striking physicochemical and structural differences with the model training sets (i.e., drugs). The limitations of these predictive models, also known as the applicability domains, are not systematically identified. Future improvements of predictive ML models should include an understanding of the scope and limitations of the available data. Besides biological activities, predictive algorithms and derived web servers have de-orphanized NPs to identify therapeutically relevant protein partners, expanding the realm of applications beyond their natural functions. Finally, deep generative models are re-routing NP-inspired de novo design with the autonomous generation of new drug candidates with simplified structures and inherited biological activities from NPs. Likewise, combining de novo design with de-orphanizing models produces novel isofunctional chemotypes (i.e., NP mimetics) that populate NP-exclusive and uncharted regions of the chemical space. These strategies are improving the synthetic accessibility, potency, and drug-likeness similarity of NP-inspired molecules.
Author contributions
FISG: visualization, investigation, writing – original draft, review & editing. VDAB: investigation, Writing – original draft & review. JLMF: writing – review & editing. FP: conceptualization, visualization, investigation, writing – original draft, review & editing. All authors read and approved the final manuscript.
Conflicts of interest
There are no conflicts to declare.
Acknowledgements
This work was supported by the Mexican research council Consejo Nacional de Ciencia y Tecnología (CONACYT). FISG and VDAB are thankful to CONACYT for their respective granted scholarships numbers 848061, and 772901. JLMF is supported by DGAPA, UNAM, Programa de Apoyo a Proyectos de Investigación e Innovación Tecnológica (PAPIIT), grant IN201321. FP is supported by a Cátedras CONACYT fellowship – 2017-present.
References
-
T. Hey and A. Trefethen, in Wiley Series in Communications Networking & Distributed Systems, John Wiley & Sons, Ltd, Chichester, UK, 2003, pp. 809–824 Search PubMed.
- M. I. Jordan and T. M. Mitchell, Science, 2015, 349, 255–260 CrossRef CAS PubMed.
- J. Vamathevan, D. Clark, P. Czodrowski, I. Dunham, E. Ferran, G. Lee, B. Li, A. Madabhushi, P. Shah, M. Spitzer and S. Zhao, Nat. Rev. Drug Discovery, 2019, 18, 463–477 CrossRef CAS PubMed.
- P. Schneider, W. P. Walters, A. T. Plowright, N. Sieroka, J. Listgarten, R. A. Goodnow Jr, J. Fisher, J. M. Jansen, J. S. Duca, T. S. Rush, M. Zentgraf, J. E. Hill, E. Krutoholow, M. Kohler, J. Blaney, K. Funatsu, C. Luebkemann and G. Schneider, Nat. Rev. Drug Discovery, 2020, 19, 353–364 CrossRef CAS PubMed.
- W. P. Walters and M. Murcko, Nat. Biotechnol., 2020, 38, 143–145 CrossRef CAS PubMed.
- N. Brown, P. Ertl, R. Lewis, T. Luksch, D. Reker and N. Schneider, J. Comput.-Aided Mol. Des., 2020, 34, 709–715 CrossRef CAS PubMed.
- A. Zhavoronkov, Y. A. Ivanenkov, A. Aliper, M. S. Veselov, V. A. Aladinskiy, A. V. Aladinskaya, V. A. Terentiev, D. A. Polykovskiy, M. D. Kuznetsov, A. Asadulaev, Y. Volkov, A. Zholus, R. R. Shayakhmetov, A. Zhebrak, L. I. Minaeva, B. A. Zagribelnyy, L. H. Lee, R. Soll, D. Madge, L. Xing, T. Guo and A. Aspuru-Guzik, Nat. Biotechnol., 2019, 37, 1038–1040 CrossRef CAS PubMed.
- T. Rodrigues, D. Reker, P. Schneider and G. Schneider, Nat. Chem., 2016, 8, 531–541 CrossRef CAS PubMed.
- E. M. Driggers, S. P. Hale, J. Lee and N. K. Terrett, Nat. Rev. Drug Discovery, 2008, 7, 608–624 CrossRef CAS PubMed.
- D. J. Newman and G. M. Cragg, J. Nat. Prod., 2020, 83, 770–803 CrossRef CAS PubMed.
- W. R. Strohl, Drug Discovery Today, 2000, 5, 39–41 CrossRef PubMed.
- A. G. Atanasov, S. B. Zotchev, V. M. Dirsch, International Natural Product Sciences Taskforce and C. T. Supuran, Nat. Rev. Drug Discovery, 2021, 20, 200–216 CrossRef CAS PubMed.
- C. M. Dobson, Nature, 2004, 432, 824–828 CrossRef CAS PubMed.
- Y. Chen, M. Garcia de Lomana, N.-O. Friedrich and J. Kirchmair, J. Chem. Inf. Model., 2018, 58, 1518–1532 CrossRef CAS PubMed.
- C. W. Johnston, M. A. Skinnider, M. A. Wyatt, X. Li, M. R. M. Ranieri, L. Yang, D. L. Zechel, B. Ma and N. A. Magarvey, Nat. Commun., 2015, 6, 8421 CrossRef CAS PubMed.
- A. E. Nugroho and H. Morita, J. Nat. Med., 2019, 73, 687–695 CrossRef PubMed.
- F. Giordanetto and J. Kihlberg, J. Med. Chem., 2014, 57, 278–295 CrossRef CAS PubMed.
- T. Rodrigues, Org. Biomol. Chem., 2017, 15, 9275–9282 RSC.
- F. Grisoni, D. Merk, V. Consonni, J. A. Hiss, S. G. Tagliabue, R. Todeschini and G. Schneider, Commun. Chem., 2018, 1, 1–9 CrossRef CAS.
- F. Pereira and J. Aires-de-Sousa, Mar. Drugs, 2018, 6, 236 CrossRef PubMed.
- J. D. Romano and N. P. Tatonetti, Front. Genet., 2019, 10, 368 CrossRef CAS PubMed.
- Y. Chen and J. Kirchmair, Mol. Inf., 2020, 39, e2000171 CrossRef PubMed.
- J. L. Medina-Franco and F. I. Saldívar-González, Biomolecules, 2020, 10, 1566 CrossRef CAS PubMed.
- M. Krallinger, O. Rabal, A. Lourenço, J. Oyarzabal and A. Valencia, Chem. Rev., 2017, 117, 7673–7761 CrossRef CAS PubMed.
- K. Rajan, A. Zielesny and C. Steinbeck, J. Cheminf., 2020, 12, 65 CAS.
- V. Tshitoyan, J. Dagdelen, L. Weston, A. Dunn, Z. Rong, O. Kononova, K. A. Persson, G. Ceder and A. Jain, Nature, 2019, 571, 95–98 CrossRef CAS PubMed.
- P. Ernst, A. Siu and G. Weikum, BMC Bioinf., 2015, 16, 157 CrossRef PubMed.
- D. Rebholz-Schuhmann, A. Oellrich and R. Hoehndorf, Nat. Rev. Genet., 2012, 13, 829–839 CrossRef CAS PubMed.
- H. Öztürk, A. Özgür, P. Schwaller, T. Laino and E. Ozkirimli, Drug Discovery Today, 2020, 25, 689–705 CrossRef PubMed.
- V. D. Badal, P. J. Kundrotas and I. A. Vakser, PLoS Comput. Biol., 2015, 11, e1004630 CrossRef PubMed.
- V. D. Badal, P. J. Kundrotas and I. A. Vakser, Bioinformatics, 2021, 37, 497–505 CrossRef CAS PubMed.
- F. S. Tsai, Int. J. Comput. Biol. Drug Des., 2011, 4, 239–244 CrossRef CAS PubMed.
- N. Papanikolaou, G. A. Pavlopoulos, T. Theodosiou and I. Iliopoulos, Methods, 2015, 74, 47–53 CrossRef CAS PubMed.
-
M. Krallinger, F. Leitner and A. Valencia, in Bioinformatics Methods in Clinical Research, ed. R. Matthiesen, Humana Press, Totowa, NJ, 2010, pp. 341–382 Search PubMed.
- V. Sharma, W. Law, M. J. Balick and I. N. Sarkar, AMIA Annu. Symp. Proc., 2017, 1537–1546 Search PubMed.
- T. N. C. Wells, Malar. J., 2011, 10(1), S3 CrossRef CAS PubMed.
- X. Xia, B. H. May, A. L. Zhang, X. Guo, C. Lu, C. C. Xue and Q. Huang, Evid. Based Complement. Alternat. Med., 2020, 7531967 Search PubMed.
- B. H. May, A. Zhang, Y. Lu, C. Lu and C. C. L. Xue, J. Altern. Complementary Med., 2014, 20, 937–942 CrossRef PubMed.
- J. L. Shergis, L. Wu, B. H. May, A. L. Zhang, X. Guo, C. Lu and C. C. Xue, Chron. Respir. Dis., 2015, 12, 204–211 CrossRef PubMed.
- X. Zhou, Y. Peng and B. Liu, J. Biomed. Inf., 2010, 43, 650–660 CrossRef PubMed.
- G. Porras, F. Chassagne, J. T. Lyles, L. Marquez, M. Dettweiler, A. M. Salam, T. Samarakoon, S. Shabih, D. R. Farrokhi and C. L. Quave, Chem. Rev., 2021, 121, 3495–3560 CrossRef CAS PubMed.
- A. R. Aronson and F.-M. Lang, J. Am. Med. Inform. Assoc., 2010, 17, 229–236 CrossRef PubMed.
- F. E. Koehn and G. T. Carter, Nat. Rev. Drug Discovery, 2005, 4, 206–220 CrossRef CAS PubMed.
- L. Katz and R. H. Baltz, J. Ind. Microbiol. Biotechnol., 2016, 43, 155–176 CrossRef CAS PubMed.
- M. H. Medema, T. de Rond and B. S. Moore, Nat. Rev. Genet., 2021, 22, 553–571 CrossRef CAS PubMed.
- C. P. Ridley, H. Y. Lee and C. Khosla, Proc. Natl. Acad. Sci. U. S. A., 2008, 105, 4595–4600 CrossRef CAS PubMed.
- B. Behsaz, E. Bode, A. Gurevich, Y.-N. Shi, F. Grundmann, D. Acharya, A. M. Caraballo-Rodríguez, A. Bouslimani, M. Panitchpakdi, A. Linck, C. Guan, J. Oh, P. C. Dorrestein, H. B. Bode, P. A. Pevzner and H. Mohimani, Nat. Commun., 2021, 12, 3225 CrossRef CAS PubMed.
- P. G. Arnison, M. J. Bibb, G. Bierbaum, A. A. Bowers, T. S. Bugni, G. Bulaj, J. A. Camarero, D. J. Campopiano, G. L. Challis, J. Clardy, P. D. Cotter, D. J. Craik, M. Dawson, E. Dittmann, S. Donadio, P. C. Dorrestein, K.-D. Entian, M. A. Fischbach, J. S. Garavelli, U. Göransson, C. W. Gruber, D. H. Haft, T. K. Hemscheidt, C. Hertweck, C. Hill, A. R. Horswill, M. Jaspars, W. L. Kelly, J. P. Klinman, O. P. Kuipers, A. J. Link, W. Liu, M. A. Marahiel, D. A. Mitchell, G. N. Moll, B. S. Moore, R. Müller, S. K. Nair, I. F. Nes, G. E. Norris, B. M. Olivera, H. Onaka, M. L. Patchett, J. Piel, M. J. T. Reaney, S. Rebuffat, R. P. Ross, H.-G. Sahl, E. W. Schmidt, M. E. Selsted, K. Severinov, B. Shen, K. Sivonen, L. Smith, T. Stein, R. D. Süssmuth, J. R. Tagg, G.-L. Tang, A. W. Truman, J. C. Vederas, C. T. Walsh, J. D. Walton, S. C. Wenzel, J. M. Willey and W. A. van der Donk, Nat. Prod. Rep., 2013, 30, 108–160 RSC.
- A. L. Harvey, R. Edrada-Ebel and R. J. Quinn, Nat. Rev. Drug Discovery, 2015, 14, 111–129 CrossRef CAS PubMed.
- N. Ziemert, M. Alanjary and T. Weber, Nat. Prod. Rep., 2016, 33, 988–1005 RSC.
- E. Kalkreuter, G. Pan, A. J. Cepeda and B. Shen, Trends Pharmacol. Sci., 2020, 41, 13–26 CrossRef CAS PubMed.
- M. H. Medema, Nat. Prod. Rep., 2021, 38, 301–306 RSC.
- J. I. Tietz, C. J. Schwalen, P. S. Patel, T. Maxson, P. M. Blair, H.-C. Tai, U. I. Zakai and D. A. Mitchell, Nat. Chem. Biol., 2017, 13, 470–478 CrossRef CAS PubMed.
- P. Agrawal, S. Khater, M. Gupta, N. Sain and D. Mohanty, Nucleic Acids Res., 2017, 45, W80–W88 CrossRef CAS PubMed.
- E. L. C. de Los Santos, Sci. Rep., 2019, 9, 13406 CrossRef PubMed.
- N. J. Merwin, W. K. Mousa, C. A. Dejong, M. A. Skinnider, M. J. Cannon, H. Li, K. Dial, M. Gunabalasingam, C. Johnston and N. A. Magarvey, Proc. Natl. Acad. Sci. U. S. A., 2020, 117, 371–380 CrossRef CAS PubMed.
- A. M. Kloosterman, P. Cimermancic, S. S. Elsayed, C. Du, M. Hadjithomas, M. S. Donia, M. A. Fischbach, G. P. van Wezel and M. H. Medema, bioRxiv, 2020 DOI:10.1101/2020.05.19.104752.
- A. M. Kloosterman, K. E. Shelton, G. P. van Wezel, M. H. Medema and D. A. Mitchell, mSystems, 2020, 5, e00267 CrossRef CAS PubMed.
- J. Söding, A. Biegert and A. N. Lupas, Nucleic Acids Res., 2005, 33, W244–W248 CrossRef PubMed.
- G. V. Paolini, R. H. B. Shapland, W. P. van Hoorn, J. S. Mason and A. L. Hopkins, Nat. Biotechnol., 2006, 24, 805–815 CrossRef CAS PubMed.
- P. Agarwal, P. Sanseau and L. R. Cardon, Nat. Rev. Drug Discovery, 2013, 12, 575–576 CrossRef CAS PubMed.
- G. D. Hannigan, D. Prihoda, A. Palicka, J. Soukup, O. Klempir, L. Rampula, J. Durcak, M. Wurst, J. Kotowski, D. Chang, R. Wang, G. Piizzi, G. Temesi, D. J. Hazuda, C. H. Woelk and D. A. Bitton, Nucleic Acids Res., 2019, 47, e110 CrossRef CAS PubMed.
- M. A. Skinnider, C. W. Johnston, M. Gunabalasingam, N. J. Merwin, A. M. Kieliszek, R. J. MacLellan, H. Li, M. R. M. Ranieri, A. L. H. Webster, M. P. T. Cao, A. Pfeifle, N. Spencer, Q. H. To, D. P. Wallace, C. A. Dejong and N. A. Magarvey, Nat. Commun., 2020, 11, 6058 CrossRef CAS PubMed.
- P. Agrawal and D. Mohanty, Bioinformatics, 2021, 37, 603–611 CrossRef CAS PubMed.
- A. S. Walker and J. Clardy, J. Chem. Inf. Model., 2021, 61, 2560–2571 CrossRef CAS PubMed.
- J. V. Pham, M. A. Yilma, A. Feliz, M. T. Majid, N. Maffetone, J. R. Walker, E. Kim, H. J. Cho, J. M. Reynolds, M. C. Song, S. R. Park and Y. J. Yoon, Front. Microbiol., 2019, 10, 1404 CrossRef PubMed.
- C. M. Whitford, P. Cruz-Morales, J. D. Keasling and T. Weber, Essays Biochem., 2021, 65, 261–275 CrossRef PubMed.
- J. Hubert, J.-M. Nuzillard and J.-H. Renault, Phytochem. Rev., 2017, 16, 55–95 CrossRef CAS.
- J.-L. Wolfender, M. Litaudon, D. Touboul and E. F. Queiroz, Nat. Prod. Rep., 2019, 36, 855–868 RSC.
- A. A. Cornejo-Báez, L. M. Peña-Rodríguez, R. Álvarez-Zapata, M. Vázquez-Hernández and A. Sánchez-Medina, Drug Discovery Today, 2020, 25, 27–37 CrossRef PubMed.
- U. W. Liebal, A. N. T. Phan, M. Sudhakar, K. Raman and L. M. Blank, Metabolites, 2020, 10, 243 CrossRef CAS PubMed.
- A. B. Risum and R. Bro, Talanta, 2019, 204, 255–260 CrossRef CAS PubMed.
- M. Witting and S. Böcker, J. Sep. Sci., 2020, 43, 1746–1754 CrossRef CAS PubMed.
- A. M. Wolfer, S. Lozano, T. Umbdenstock, V. Croixmarie, A. Arrault and P. Vayer, Metabolomics, 2015, 12, 8 CrossRef.
- R. Bouwmeester, L. Martens and S. Degroeve, Anal. Chem., 2019, 91, 3694–3703 CrossRef CAS PubMed.
- M. Kokla, J. Virtanen, M. Kolehmainen, J. Paananen and K. Hanhineva, BMC Bioinf., 2019, 20, 492 CrossRef PubMed.
- Z. Pang, J. Chong, G. Zhou, D. A. de Lima Morais, L. Chang, M. Barrette, C. Gauthier, P.-É. Jacques, S. Li and J. Xia, Nucleic Acids Res., 2021, 49, W388–W396 CrossRef CAS PubMed.
- M. Wang, J. J. Carver, V. V. Phelan, L. M. Sanchez, N. Garg, Y. Peng, D. D. Nguyen, J. Watrous, C. A. Kapono, T. Luzzatto-Knaan, C. Porto, A. Bouslimani, A. V. Melnik, M. J. Meehan, W.-T. Liu, M. Crüsemann, P. D. Boudreau, E. Esquenazi, M. Sandoval-Calderón, R. D. Kersten, L. A. Pace, R. A. Quinn, K. R. Duncan, C.-C. Hsu, D. J. Floros, R. G. Gavilan, K. Kleigrewe, T. Northen, R. J. Dutton, D. Parrot, E. E. Carlson, B. Aigle, C. F. Michelsen, L. Jelsbak, C. Sohlenkamp, P. Pevzner, A. Edlund, J. McLean, J. Piel, B. T. Murphy, L. Gerwick, C.-C. Liaw, Y.-L. Yang, H.-U. Humpf, M. Maansson, R. A. Keyzers, A. C. Sims, A. R. Johnson, A. M. Sidebottom, B. E. Sedio, A. Klitgaard, C. B. Larson, C. A. B. P. D. Torres-Mendoza, D. J. Gonzalez, D. B. Silva, L. M. Marques, D. P. Demarque, E. Pociute, E. C. O'Neill, E. Briand, E. J. N. Helfrich, E. A. Granatosky, E. Glukhov, F. Ryffel, H. Houson, H. Mohimani, J. J. Kharbush, Y. Zeng, J. A. Vorholt, K. L. Kurita, P. Charusanti, K. L. McPhail, K. F. Nielsen, L. Vuong, M. Elfeki, M. F. Traxler, N. Engene, N. Koyama, O. B. Vining, R. Baric, R. R. Silva, S. J. Mascuch, S. Tomasi, S. Jenkins, V. Macherla, T. Hoffman, V. Agarwal, P. G. Williams, J. Dai, R. Neupane, J. Gurr, A. M. C. Rodríguez, A. Lamsa, C. Zhang, K. Dorrestein, B. M. Duggan, J. Almaliti, P.-M. Allard, P. Phapale, L.-F. Nothias, T. Alexandrov, M. Litaudon, J.-L. Wolfender, J. E. Kyle, T. O. Metz, T. Peryea, D.-T. Nguyen, D. VanLeer, P. Shinn, A. Jadhav, R. Müller, K. M. Waters, W. Shi, X. Liu, L. Zhang, R. Knight, P. R. Jensen, B. O. Palsson, K. Pogliano, R. G. Linington, M. Gutiérrez, N. P. Lopes, W. H. Gerwick, B. S. Moore, P. C. Dorrestein and N. Bandeira, Nat. Biotechnol., 2016, 34, 828–837 CrossRef CAS PubMed.
- J. Y. Yang, L. M. Sanchez, C. M. Rath, X. Liu, P. D. Boudreau, N. Bruns, E. Glukhov, A. Wodtke, R. de Felicio, A. Fenner, W. R. Wong, R. G. Linington, L. Zhang, H. M. Debonsi, W. H. Gerwick and P. C. Dorrestein, J. Nat. Prod., 2013, 76, 1686–1699 CrossRef CAS PubMed.
- M. Valli, H. M. Russo, A. C. Pilon, M. E. F. Pinto, N. B. Dias, R. T. Freire, I. Castro-Gamboa and V. da S. Bolzani, Phys. Sci. Rev., 2019, 4, 20180167 Search PubMed.
- K. Dührkop, H. Shen, M. Meusel, J. Rousu and S. Böcker, Proc. Natl. Acad. Sci. U. S. A., 2015, 112, 12580–12585 CrossRef PubMed.
- J. J. J. van der Hooft, J. Wandy, M. P. Barrett, K. E. V. Burgess and S. Rogers, Proc. Natl. Acad. Sci. U. S. A., 2016, 113, 13738–13743 CrossRef PubMed.
- K. Dührkop, M. Fleischauer, M. Ludwig, A. A. Aksenov, A. V. Melnik, M. Meusel, P. C. Dorrestein, J. Rousu and S. Böcker, Nat. Methods, 2019, 16, 299–302 CrossRef PubMed.
- A. Gurevich, A. Mikheenko, A. Shlemov, A. Korobeynikov, H. Mohimani and P. A. Pevzner, Nat. Microbiol., 2018, 3, 319–327 CrossRef CAS PubMed.
- D. C. Burns, E. P. Mazzola and W. F. Reynolds, Nat. Prod. Rep., 2019, 36, 919–933 RSC.
- M. Elyashberg and D. Argyropoulos, Magn. Reson. Chem., 2021, 59, 669–690 CrossRef CAS PubMed.
- R. Reher, H. W. Kim, C. Zhang, H. H. Mao, M. Wang, L.-F. Nothias, A. M. Caraballo-Rodriguez, E. Glukhov, B. Teke, T. Leao, K. L. Alexander, B. M. Duggan, E. L. Van Everbroeck, P. C. Dorrestein, G. W. Cottrell and W. H. Gerwick, J. Am. Chem. Soc., 2020, 142, 4114–4120 CrossRef CAS PubMed.
- L. Gross, F. Mohn, N. Moll, G. Meyer, R. Ebel, W. M. Abdel-Mageed and M. Jaspars, Nat. Chem., 2010, 2, 821–825 CrossRef CAS PubMed.
- Y. Inokuma, S. Yoshioka, J. Ariyoshi, T. Arai, Y. Hitora, K. Takada, S. Matsunaga, K. Rissanen and M. Fujita, Nature, 2013, 495, 461–466 CrossRef CAS PubMed.
- E. Danelius, S. Halaby, W. A. van der Donk and T. Gonen, Nat. Prod. Rep., 2021, 38, 423–431 RSC.
- D. Weininger, J. Chem. Inf. Comput. Sci., 1988, 28, 31–36 CrossRef CAS.
- S. R. Heller, A. McNaught, I. Pletnev, S. Stein and D. Tchekhovskoi, J. Cheminf., 2015, 7, 23 Search PubMed.
- N. O'Boyle and A. Dalke, ChemRxiv, 2018 DOI:10.26434/chemrxiv.7097960.v1.
-
M. Krenn, F. Häse, A. Nigam, P. Friederich and A. Aspuru-Guzik, 2019, arXiv 1905.13741 [cs.LG].
- E. López-López, J. Bajorath and J. L. Medina-Franco, J. Chem. Inf. Model., 2021, 61, 26–35 CrossRef PubMed.
- D. G. Corley and R. C. Durley, J. Nat. Prod., 1994, 57, 1484–1490 CrossRef CAS.
- M. Sorokina, P. Merseburger, K. Rajan, M. A. Yirik and C. Steinbeck, J. Cheminf., 2021, 13, 2 Search PubMed.
- A. Rutz, M. Sorokina, J. Galgonek, D. Mietchen, E. Willighagen, A. Gaudry, J. G. Graham, R. Stephan, R. Page, J. Vondrášek, C. Steinbeck, G. F. Pauli, J.-L. Wolfender, J. Bisson and P.-M. Allard, bioRxiv, 2021 DOI:10.1101/2021.02.28.433265.
-
E. K. F. Ahrens, in Chemical Structures, Springer Berlin Heidelberg, 1988, pp. 97–111 Search PubMed.
- B. D. Christie, B. A. Leland and J. G. Nourse, J. Chem. Inf. Comput. Sci., 1993, 33, 545–547 CrossRef CAS.
- R. E. Carhart, D. H. Smith and R. Venkataraghavan, J. Chem. Inf. Comput. Sci., 1985, 25, 64–73 CrossRef CAS.
- D. Rogers and M. Hahn, J. Chem. Inf. Model., 2010, 50, 742–754 CrossRef CAS PubMed.
- J. L. Durant, B. A. Leland, D. R. Henry and J. G. Nourse, J. Chem. Inf. Comput. Sci., 2002, 42, 1273–1280 CrossRef CAS PubMed.
- X. Chen and C. H. Reynolds, J. Chem. Inf. Comput. Sci., 2002, 42, 1407–1414 CrossRef CAS PubMed.
- R. Todeschini, V. Consonni, H. Xiang, J. Holliday, M. Buscema and P. Willett, J. Chem. Inf. Model., 2012, 52, 2884–2901 CrossRef CAS PubMed.
- D. Bajusz, A. Rácz and K. Héberger, J. Cheminf., 2015, 7, 20 Search PubMed.
- T. Henkel, R. M. Brunne, H. Müller and F. Reichel, Angew. Chem., Int. Ed., 1999, 38, 643–647 CrossRef CAS PubMed.
- A. M. Boldi, Curr. Opin. Chem. Biol., 2004, 8, 281–286 CrossRef CAS PubMed.
- S. Shang and D. S. Tan, Curr. Opin. Chem. Biol., 2005, 9, 248–258 CrossRef CAS PubMed.
- N. Yao, A. Song, X. Wang, S. Dixon and K. S. Lam, J. Comb. Chem., 2007, 9, 668–676 CrossRef CAS PubMed.
- W. R. J. D. Galloway, A. Isidro-Llobet and D. R. Spring, Nat. Commun., 2010, 1, 80 CrossRef PubMed.
- L. Eberhardt, K. Kumar and H. Waldmann, Curr. Drug Targets, 2011, 12, 1531–1546 CrossRef CAS PubMed.
-
M. Dow, F. Marchetti and A. Nelson, in Diversity-Oriented Synthesis, John Wiley & Sons, Inc., Hoboken, NJ, USA, 2013, pp. 289–323 Search PubMed.
- C. Zhao, Z. Ye, Z.-X. Ma, S. A. Wildman, S. A. Blaszczyk, L. Hu, I. A. Guizei and W. Tang, Nat. Commun., 2019, 10, 4015 CrossRef PubMed.
- J. Chauhan, T. Luthra, R. Gundla, A. Ferraro, U. Holzgrabe and S. Sen, Org. Biomol. Chem., 2017, 15, 9108–9120 RSC.
- F. L. Stahura, J. W. Godden, L. Xue and J. Bajorath, J. Chem. Inf. Comput. Sci., 2000, 40, 1245–1252 CrossRef CAS PubMed.
- M. Feher and J. M. Schmidt, J. Chem. Inf. Comput. Sci., 2003, 43, 218–227 CrossRef CAS PubMed.
- J.-Y. Ortholand and A. Ganesan, Curr. Opin. Chem. Biol., 2004, 8, 271–280 CrossRef CAS PubMed.
- G. Kristina and S. Gisbert, Curr. Chem. Biol., 2006, 1, 115–127 Search PubMed.
- K. Grabowski, K.-H. Baringhaus and G. Schneider, Nat. Prod. Rep., 2008, 25, 892–904 RSC.
- J. Rosén, J. Gottfries, S. Muresan, A. Backlund and T. I. Oprea, J. Med. Chem., 2009, 52, 1953–1962 CrossRef PubMed.
- M. L. Lee and G. Schneider, J. Comb. Chem., 2001, 3, 284–289 CrossRef CAS PubMed.
- M. A. Koch, A. Schuffenhauer, M. Scheck, S. Wetzel, M. Casaulta, A. Odermatt, P. Ertl and H. Waldmann, Proc. Natl. Acad. Sci. U. S. A., 2005, 102, 17272–17277 CrossRef CAS PubMed.
- J. Larsson, J. Gottfries, S. Muresan and A. Backlund, J. Nat. Prod., 2007, 70, 789–794 CrossRef CAS PubMed.
- S. Renner, W. A. L. van Otterlo, M. D. Seoane, S. Möcklinghoff, B. Hofmann, S. Wetzel, A. Schuffenhauer, P. Ertl, T. I. Oprea, D. Steinhilber, L. Brunsveld, D. Rauh and H. Waldmann, Nat. Chem. Biol., 2009, 5, 585–592 CrossRef CAS PubMed.
- S. Wetzel, K. Klein, S. Renner, D. Rauh, T. I. Oprea, P. Mutzel and H. Waldmann, Nat. Chem. Biol., 2009, 5, 581–583 CrossRef CAS PubMed.
- R. S. Bon and H. Waldmann, Acc. Chem. Res., 2010, 43, 1103–1114 CrossRef CAS PubMed.
- M. Reutlinger and G. Schneider, J. Mol. Graphics Modell., 2012, 34, 108–117 CrossRef CAS PubMed.
- H. Lachance, S. Wetzel, K. Kumar and H. Waldmann, J. Med. Chem., 2012, 55, 5989–6001 CrossRef CAS PubMed.
- K. Klein, O. Koch, N. Kriege, P. Mutzel and T. Schäfer, Mol. Inf., 2013, 32, 964–975 CrossRef CAS PubMed.
- T. Miyao, D. Reker, P. Schneider, K. Funatsu and G. Schneider, Planta Med., 2015, 81, 429–435 CrossRef CAS PubMed.
- T. Schäfer, N. Kriege, L. Humbeck, K. Klein, O. Koch and P. Mutzel, J. Cheminf., 2017, 9, 1–18 Search PubMed.
- P. Ertl, S. Roggo and A. Schuffenhauer, J. Chem. Inf. Model., 2008, 48, 68–74 CrossRef CAS PubMed.
- M. Krier, G. Bret and D. Rognan, J. Chem. Inf. Model., 2006, 46, 512–524 CrossRef CAS PubMed.
- S. Gupta and J. Aires-de-Sousa, Mol. Diversity, 2007, 11, 23–36 CrossRef CAS PubMed.
- J. Hert, J. J. Irwin, C. Laggner, M. J. Keiser and B. K. Shoichet, Nat. Chem. Biol., 2009, 5, 479–483 CrossRef CAS PubMed.
- P. D. Dobson, Y. Patel and D. B. Kell, Drug Discovery Today, 2009, 14, 31–40 CrossRef CAS PubMed.
- J. E. Peironcely, T. Reijmers, L. Coulier, A. Bender and T. Hankemeier, PLoS One, 2011, 6, e28966 CrossRef CAS PubMed.
- A. B. Yongye, J. Waddell and J. L. Medina-Franco, Chem. Biol. Drug Des., 2012, 80, 717–724 CrossRef CAS PubMed.
- D. Genis, M. Kirpichenok and R. Kombarov, Drug Discovery Today, 2012, 17, 1170–1174 CrossRef CAS PubMed.
- S. O′Hagan, N. Swainston, J. Handl and D. B. Kell, Metabolomics, 2015, 11, 323–339 CrossRef PubMed.
- M. Seo, H. K. Shin, Y. Myung, S. Hwang and K. T. No, J. Cheminf., 2020, 12, 6 CAS.
- A. Capecchi, D. Probst and J.-L. Reymond, J. Cheminf., 2020, 12, 43 CAS.
- R. P. Sheridan, M. D. Miller, D. J. Underwood and S. K. Kearsley, J. Chem. Inf. Comput. Sci., 1996, 36, 128–136 CrossRef CAS.
- G. B. McGaughey, R. P. Sheridan, C. I. Bayly, J. C. Culberson, C. Kreatsoulas, S. Lindsley, V. Maiorov, J.-F. Truchon and W. D. Cornell, J. Chem. Inf. Model., 2007, 47, 1504–1519 CrossRef CAS PubMed.
- T. S. Rush 3rd, J. A. Grant, L. Mosyak and A. Nicholls, J. Med. Chem., 2005, 48, 1489–1495 CrossRef PubMed.
- P. C. D. Hawkins, A. G. Skillman and A. Nicholls, J. Med. Chem., 2007, 50, 74–82 CrossRef CAS PubMed.
- G. Hu, G. Kuang, W. Xiao, W. Li, G. Liu and Y. Tang, J. Chem. Inf. Model., 2012, 52, 1103–1113 CrossRef CAS PubMed.
- H.-J. Böhm, A. Flohr and M. Stahl, Drug Discov. Today Technol., 2004, 1, 217–224 CrossRef PubMed.
- H. Sun, G. Tawa and A. Wallqvist, Drug Discovery Today, 2012, 17, 310–324 CrossRef CAS PubMed.
- J. Bajorath, Future Med. Chem., 2017, 9, 629–631 CrossRef CAS PubMed.
- S. Riniker and G. A. Landrum, J. Cheminf., 2013, 5, 26 CAS.
- M. A. Skinnider, C. A. Dejong, B. C. Franczak, P. D. McNicholas and N. A. Magarvey, J. Cheminf., 2017, 9, 46 Search PubMed.
- Y. Chen, N. Mathai and J. Kirchmair, J. Chem. Inf. Model., 2020, 60, 2858–2875 CrossRef CAS PubMed.
-
G. M. Maggiora, in Foodinformatics: Applications of Chemical Information to Food Chemistry, ed. K. Martinez-Mayorga and J. L. Medina-Franco, Springer International Publishing, Cham, 2014, pp. 1–81 Search PubMed.
- A. Sato, T. Miyao, S. Jasial and K. Funatsu, J. Comput.-Aided Mol. Des., 2021, 35, 179–193 CrossRef CAS PubMed.
-
R. Todeschini and V. Consonni, Molecular Descriptors for Chemoinformatics, Wiley, 2009 Search PubMed.
- C. A. Lipinski, F. Lombardo, B. W. Dominy and P. J. Feeney, Adv. Drug Delivery Rev., 2001, 46, 3–26 CrossRef CAS PubMed.
- R. J. Quinn, A. R. Carroll, N. B. Pham, P. Baron, M. E. Palframan, L. Suraweera, G. K. Pierens and S. Muresan, J. Nat. Prod., 2008, 71, 464–468 CrossRef CAS PubMed.
- D. F. Veber, S. R. Johnson, H.-Y. Cheng, B. R. Smith, K. W. Ward and K. D. Kopple, J. Med. Chem., 2002, 45, 2615–2623 CrossRef CAS PubMed.
- F. Lovering, J. Bikker and C. Humblet, J. Med. Chem., 2009, 52, 6752–6756 CrossRef CAS PubMed.
- M. D. Shultz, J. Med. Chem., 2019, 62, 1701–1714 CrossRef CAS PubMed.
- D. A. DeGoey, H.-J. Chen, P. B. Cox and M. D. Wendt, J. Med. Chem., 2018, 61, 2636–2651 CrossRef CAS PubMed.
- P. D. Leeson and B. Springthorpe, Nat. Rev. Drug Discovery, 2007, 6, 881–890 CrossRef CAS PubMed.
- M. Vieth, M. G. Siegel, R. E. Higgs, I. A. Watson, D. H. Robertson, K. A. Savin, G.
L. Durst and P. A. Hipskind, J. Med. Chem., 2004, 47, 224–232 CrossRef CAS PubMed.
- M. C. Wenlock, R. P. Austin, P. Barton, A. M. Davis and P. D. Leeson, J. Med. Chem., 2003, 46, 1250–1256 CrossRef CAS PubMed.
- N. A. Meanwell, Chem. Res. Toxicol., 2016, 29, 564–616 Search PubMed.
- H. N. Hoang, T. A. Hill and D. P. Fairlie, Angew. Chem. Weinheim Bergstr. Ger., 2021, 133, 8466–8471 Search PubMed.
-
F. Grisoni, V. Consonni and R. Todeschini, in Computational Chemogenomics, ed. J. B. Brown, Springer New York, New York, NY, 2018, pp. 171–209 Search PubMed.
- J.-F. Truchon and C. I. Bayly, J. Chem. Inf. Model., 2007, 47, 488–508 CrossRef CAS PubMed.
- W. Rawat and Z. Wang, Neural Comput, 2017, 29, 2352–2449 CrossRef PubMed.
- T. Young, D. Hazarika, S. Poria and E. Cambria, IEEE Comput. Intell. Mag., 2018, 13, 55–75 Search PubMed.
- E. Gawehn, J. A. Hiss and G. Schneider, Mol. Inf., 2016, 35, 3–14 CrossRef CAS PubMed.
- I. I. Baskin, D. Winkler and I. V. Tetko, Expert Opin. Drug Discovery, 2016, 11, 785–795 CrossRef CAS PubMed.
- H. Chen, O. Engkvist, Y. Wang, M. Olivecrona and T. Blaschke, Drug Discovery Today, 2018, 23, 1241–1250 CrossRef PubMed.
- A. Lavecchia, Drug Discovery Today, 2019, 24, 2017–2032 CrossRef PubMed.
- M. Sun, S. Zhao, C. Gilvary, O. Elemento, J. Zhou and F. Wang, Brief. Bioinform., 2020, 21, 919–935 CrossRef PubMed.
-
B. Sanchez-Lengeling, J. N. Wei, B. K. Lee, R. C. Gerkin, A. Aspuru-Guzik and A. B. Wiltschko, arXiv [stat.ML], 2019.
- D. M. Maniyar, I. T. Nabney, B. S. Williams and A. Sewing, J. Chem. Inf. Model., 2006, 46, 1806–1818 CrossRef CAS PubMed.
- G. Schneider, Nat. Rev. Drug Discovery, 2018, 17, 97–113 CrossRef CAS PubMed.
- J. Arús-Pous, M. Awale, D. Probst and J.-L. Reymond, Chimia, 2019, 73, 1018–1023 CrossRef PubMed.
- F. I. Saldívar-González, B. A. Pilón-Jiménez and J. L. Medina-Franco, Physical Sciences Reviews, 2018, 0, 525 Search PubMed.
- J. L. Medina-Franco, K. Martínez-Mayorga, M. A. Giulianotti, R. A. Houghten and C. Pinilla, Curr. Comput.-Aided Drug Des., 2008, 4, 322–333 CrossRef CAS.
- D. I. Osolodkin, E. V. Radchenko, A. A. Orlov, A. E. Voronkov, V. A. Palyulin and N. S. Zefirov, Expert Opin. Drug Discovery, 2015, 10, 959–973 CrossRef PubMed.
- J. L. Medina-Franco, J. J. Naveja and E. López-López, Drug Discovery Today, 2019, 24, 2162–2169 CrossRef CAS PubMed.
- J. Jesús Naveja and J. L. Medina-Franco, F1000Res., 2017, 6, 1134 Search PubMed.
- D. Probst and J.-L. Reymond, J. Cheminf., 2020, 12, 12 Search PubMed.
- A. Capecchi and J.-L. Reymond, Biomolecules, 2020, 10, 1385 CrossRef CAS PubMed.
- N. Sánchez-Cruz, B. A. Pilón-Jiménez and J. L. Medina-Franco, F1000Res., 2020, 8, 2071 Search PubMed.
- A. L. Chávez-Hernández, N. Sánchez-Cruz and J. L. Medina-Franco, Mol. Inf., 2020, 39, e2000050 CrossRef PubMed.
- A. L. Chávez-Hernández, N. Sánchez-Cruz and J. L. Medina-Franco, Biomolecules, 2020, 10, 1518 CrossRef PubMed.
- G. Karageorgis, D. J. Foley, L. Laraia and H. Waldmann, Nat. Chem., 2020, 12, 227–235 CrossRef CAS PubMed.
- S. Majumdar and S. C. Basak, Curr. Comput.-Aided Drug Des., 2016, 12, 294–301 CrossRef CAS PubMed.
- N. Aniceto, A. A. Freitas, A. Bender and T. Ghafourian, J. Cheminf., 2016, 8, 1–20 Search PubMed.
- F. Plisson, O. Ramírez-Sánchez and C. Martínez-Hernández, Sci. Rep., 2020, 10, 16581 CrossRef CAS PubMed.
- J. Clardy and C. Walsh, Nature, 2004, 432, 829–837 CrossRef CAS PubMed.
- L. A. Wessjohann, E. Ruijter, D. Garcia-Rivera and W. Brandt, Mol. Diversity, 2005, 9, 171–186 CrossRef CAS PubMed.
- A. D. G. Lawson, M. MacCoss and J. P. Heer, J. Med. Chem., 2018, 61, 4283–4289 CrossRef CAS PubMed.
- D. H. Williams, M. J. Stone, P. R. Hauck and S. K. Rahman, J. Nat. Prod., 1989, 52, 1189–1208 CrossRef CAS PubMed.
- M. J. Stone and D. H. Williams, Mol. Microbiol., 1992, 6, 29–34 CrossRef CAS PubMed.
- K. V. Jayaseelan, P. Moreno, A. Truszkowski, P. Ertl and C. Steinbeck, BMC Bioinf., 2012, 13, 106 CrossRef PubMed.
- M. Sorokina and C. Steinbeck, J. Cheminf., 2019, 11, 55 Search PubMed.
- M. J. Yu, J. Chem. Inf. Model., 2011, 51, 541–557 CrossRef CAS PubMed.
- Y. Chen, C. Stork, S. Hirte and J. Kirchmair, Biomolecules, 2019, 9, 43 CrossRef PubMed.
- D. B. Kell, Nat. Rev. Drug Discovery, 2016, 15, 143 CrossRef CAS PubMed.
- Y. H. Lee, H. Choi, S. Park, B. Lee and G.-S. Yi, BMC Bioinf., 2017, 18, 226 CrossRef PubMed.
- G. M. Rishton, Drug Discovery Today, 2003, 8, 86–96 CrossRef CAS PubMed.
- M. M. Hann and T. I. Oprea, Curr. Opin. Chem. Biol., 2004, 8, 255–263 CrossRef CAS PubMed.
- W. P. Walters and M. A. Murcko, Adv. Drug Delivery Rev., 2002, 54, 255–271 CrossRef CAS PubMed.
- T. Wunberg, M. Hendrix, A. Hillisch, M. Lobell, H. Meier, C. Schmeck, H. Wild and B. Hinzen, Drug Discovery Today, 2006, 11, 175–180 CrossRef CAS PubMed.
- S. M. Marshall, C. Mathis, E. Carrick, G. Keenan, G. J. T. Cooper, H. Graham, M. Craven, P. S. Gromski, D. G. Moore, S. I. Walker and L. Cronin, Nat. Commun., 2021, 12, 3033 CrossRef CAS PubMed.
- E. N. Muratov, J. Bajorath, R. P. Sheridan, I. V. Tetko, D. Filimonov, V. Poroikov, T. I. Oprea, I. I. Baskin, A. Varnek, A. Roitberg, O. Isayev, S. Curtarolo, D. Fourches, Y. Cohen, A. Aspuru-Guzik, D. A. Winkler, D. Agrafiotis, A. Cherkasov and A. Tropsha, Chem. Soc. Rev., 2020, 49, 3525–3564 RSC.
- R. Zhang, X. Li, X. Zhang, H. Qin and W. Xiao, Nat. Prod. Rep., 2021, 38, 346–361 RSC.
- J. Jeon, S. Kang and H. U. Kim, Nat. Prod. Rep., 2021, 38, 1954–1966 RSC.
- M. Galvez-Llompart, R. Zanni and R. García-Domenech, Int. J. Mol. Sci., 2011, 12, 9481–9503 CrossRef CAS PubMed.
- M. Galvez-Llompart, M. Del Carmen Recio Iglesias, J. Gálvez and R. García-Domenech, Mol. Diversity, 2013, 17, 573–593 CrossRef CAS PubMed.
- R. García-Domenech, R. Zanni, M. Galvez-Llompart and J. V. de Julián-Ortiz, Comb. Chem. High Throughput Screening, 2013, 16, 628–635 CrossRef PubMed.
- C. Steinbeck, Y. Han, S. Kuhn, O. Horlacher, E. Luttmann and E. Willighagen, J. Chem. Inf. Comput. Sci., 2003, 43, 493–500 CrossRef CAS PubMed.
- F. Pereira, D. A. R. S. Latino and S. P. Gaudêncio, Mar. Drugs, 2014, 12, 757–778 CrossRef PubMed.
- F. Pereira, D. A. R. S. Latino and S. P. Gaudêncio, Molecules, 2015, 20, 4848–4873 CrossRef CAS PubMed.
- S.-X. Dai, W.-X. Li, F.-F. Han, Y.-C. Guo, J.-J. Zheng, J.-Q. Liu, Q. Wang, Y.-D. Gao, G.-H. Li and J.-F. Huang, Sci. Rep., 2016, 6, 25462 CrossRef CAS PubMed.
- G.-H. Li and J.-F. Huang, Bioinformatics, 2012, 28, 3334–3335 CrossRef CAS PubMed.
- A. Rayan, J. Raiyn and M. Falah, PLoS One, 2017, 12, e0187925 CrossRef PubMed.
- M. Zeidan, M. Rayan, N. Zeidan, M. Falah and A. Rayan, Molecules, 2017, 22, 1563 CrossRef PubMed.
- M. Aswad, M. Rayan, S. Abu-Lafi, M. Falah, J. Raiyn, Z. Abdallah and A. Rayan, Inflammation Res., 2018, 67, 67–75 CrossRef CAS PubMed.
- M. Masalha, M. Rayan, A. Adawi, Z. Abdallah and A. Rayan, Mol. Med. Rep., 2018, 18, 763–770 CAS.
- M. Rayan, Z. Abdallah, S. Abu-Lafi, M. Masalha and A. Rayan, Curr. Comput.-Aided Drug Des., 2019, 15, 235–242 CrossRef CAS PubMed.
- S. Egieyeh, J. Syce, S. F. Malan and A. Christoffels, PLoS One, 2018, 13, e0204644 CrossRef PubMed.
- P. A. Onguéné, C. V. Simoben, G. W. Fotso, K. Andrae-Marobela, S. A. Khalid, B. T. Ngadjui, L. M. Mbaze and F. Ntie-Kang, Comput. Biol. Chem., 2018, 72, 136–149 CrossRef PubMed.
- J. E. Ridings, M. D. Barratt, R. Cary, C. G. Earnshaw, C. E. Eggington, M. K. Ellis, P. N. Judson, J. J. Langowski, C. A. Marchant, M. P. Payne, W. P. Watson and T. D. Yih, Toxicology, 1996, 106, 267–279 CrossRef CAS PubMed.
- D. E. V. Pires, T. L. Blundell and D. B. Ascher, J. Med. Chem., 2015, 58, 4066–4072 CrossRef CAS PubMed.
- W. Zhang, J. Pei and L. Lai, J. Chem. Inf. Model., 2017, 57, 403–412 CrossRef CAS PubMed.
- T. Dias, S. P. Gaudêncio and F. Pereira, Mar. Drugs, 2019, 17, 16 CrossRef CAS PubMed.
- S. Yoo, H. C. Yang, S. Lee, J. Shin, S. Min, E. Lee, M. Song and D. Lee, Front. Pharmacol., 2020, 11, 584875 CrossRef CAS PubMed.
- Z. Liu, D. Huang, S. Zheng, Y. Song, B. Liu, J. Sun, Z. Niu, Q. Gu, J. Xu and L. Xie, Eur. J. Med. Chem., 2021, 210, 112982 CrossRef CAS PubMed.
- M. Rupp, T. Schroeter, R. Steri, H. Zettl, E. Proschak, K. Hansen, O. Rau, O. Schwarz, L. Müller-Kuhrt, M. Schubert-Zsilavecz, K.-R. Müller and G. Schneider, ChemMedChem, 2010, 5, 191–194 CrossRef CAS PubMed.
- Y. Sun, H. Zhou, H. Zhu and S.-W. Leung, Sci. Rep., 2016, 6, 19312 CrossRef CAS PubMed.
- X. Pang, W. Fu, J. Wang, D. Kang, L. Xu, Y. Zhao, A.-L. Liu and G.-H. Du, Oxid. Med. Cell. Longevity, 2018, 2018, 6040149 Search PubMed.
- X. Zhang, T. Liu, X. Fan and N. Ai, J. Mol. Graphics Modell., 2017, 75, 347–354 CrossRef CAS PubMed.
- L. K. Chico, L. J. Van Eldik and D. M. Watterson, Nat. Rev. Drug Discovery, 2009, 8, 892–909 CrossRef CAS PubMed.
- F. Plisson and A. M. Piggott, Mar. Drugs, 2019, 17, 81 CrossRef CAS PubMed.
- A. Tropsha, Mol. Inf., 2010, 29, 476–488 CrossRef CAS PubMed.
- N. Artrith, K. T. Butler, F.-X. Coudert, S. Han, O. Isayev, A. Jain and A. Walsh, Nat. Chem., 2021, 13, 505–508 CrossRef CAS PubMed.
- J. L. Medina-Franco, K. Martinez-Mayorga, E. Fernández-de Gortari, J. Kirchmair and J. Bajorath, F1000Res., 2021, 10, 397 Search PubMed.
- J. G. Moffat, F. Vincent, J. A. Lee, J. Eder and M. Prunotto, Nat. Rev. Drug Discovery, 2017, 16, 531–543 CrossRef CAS PubMed.
- R. Zhuo, H. Liu, N. Liu and Y. Wang, Molecules, 2016, 21, 1516 CrossRef PubMed.
- M. Schirle and J. L. Jenkins, Drug Discovery Today, 2016, 21, 82–89 CrossRef CAS PubMed.
- C. Fellmann, B. G. Gowen, P.-C. Lin, J. A. Doudna and J. E. Corn, Nat. Rev. Drug Discovery, 2017, 16, 89–100 CrossRef CAS PubMed.
-
T. Rodrigues, in Progress in the Chemistry of Organic Natural Products 110: Cheminformatics in Natural Product Research, ed. A. D. Kinghorn, H. Falk, S. Gibbons, J. ’ichi Kobayashi, Y. Asakawa and J.-K. Liu, Springer International Publishing, Cham, 2019, pp. 73–97 Search PubMed.
- A. F. A. Moumbock, J. Li, P. Mishra, M. Gao and S. Günther, Comput. Struct. Biotechnol. J., 2019, 17, 1367–1376 CrossRef CAS PubMed.
- A. Lagunin, A. Stepanchikova, D. Filimonov and V. Poroikov, Bioinformatics, 2000, 16, 747–748 CrossRef CAS PubMed.
- A. Lagunin, D. Filimonov and V. Poroikov, Curr. Pharm. Des., 2010, 16, 1703–1717 CrossRef CAS PubMed.
- R. P. O. Costa, L. F. Lucena, L. M. A. Silva, G. J. Zocolo, C. Herrera-Acevedo, L. Scotti, F. B. Da-Costa, N. Ionov, V. Poroikov, E. N. Muratov and M. T. Scotti, J. Chem. Inf. Model., 2021, 61, 2516–2522 CrossRef CAS PubMed.
- M. J. Keiser, B. L. Roth, B. N. Armbruster, P. Ernsberger, J. J. Irwin and B. K. Shoichet, Nat. Biotechnol., 2007, 25, 197–206 CrossRef CAS PubMed.
- G. A. Zatelli, V. Temml, Z. Kutil, P. Landa, T. Vanek, D. Schuster and M. Falkenberg, Planta Medica Letters, 2016, 3, e17–e19 CrossRef.
- M. S. Sá, M. N. de Menezes, A. U. Krettli, I. M. Ribeiro, T. C. B. Tomassini, R. Ribeiro dos Santos, W. F. de Azevedo Jr and M. B. P. Soares, J. Nat. Prod., 2011, 74, 2269–2272 CrossRef PubMed.
- D. Reker, T. Rodrigues, P. Schneider and G. Schneider, Proc. Natl. Acad. Sci. U. S. A., 2014, 111, 4067–4072 CrossRef CAS PubMed.
- D. Reker, A. M. Perna, T. Rodrigues, P. Schneider, M. Reutlinger, B. Mönch, A. Koeberle, C. Lamers, M. Gabler, H. Steinmetz, R. Müller, M. Schubert-Zsilavecz, O. Werz and G. Schneider, Nat. Chem., 2014, 6, 1072–1078 CrossRef CAS PubMed.
- T. Rodrigues, F. Sieglitz and G. J. L. Bernardes, Chem. Soc. Rev., 2016, 45, 6130–6137 RSC.
- T. Rodrigues, F. Sieglitz, V. J. Somovilla, P. M. S. D. Cal, A. Galione, F. Corzana and G. J. L. Bernardes, Angew. Chem., Int. Ed., 2016, 55, 11077–11081 CrossRef CAS PubMed.
- G. Schneider, D. Reker, T. Chen, K. Hauenstein, P. Schneider and K.-H. Altmann, Angew. Chem., Int. Ed., 2016, 55, 12408–12411 CrossRef CAS PubMed.
- J. Keum, S. Yoo, D. Lee and H. Nam, BMC Bioinf., 2016, 17(6), 219 CrossRef PubMed.
- P. Schneider and G. Schneider, Angew. Chem., Int. Ed., 2017, 56, 11520–11524 CrossRef CAS PubMed.
- P. Schneider and G. Schneider, Chem. Commun., 2017, 53, 2272–2274 RSC.
- F. Grisoni, D. Merk, L. Friedrich and G. Schneider, ChemMedChem, 2019, 14, 1129–1134 CrossRef CAS PubMed.
- T. Rodrigues, M. Werner, J. Roth, E. H. G. da Cruz, M. C. Marques, P. Akkapeddi, S. A. Lobo, A. Koeberle, F. Corzana, E. N. da Silva Júnior, O. Werz and G. J. L. Bernardes, Chem. Sci., 2018, 9, 6899–6903 RSC.
- C. Rücker, G. Rücker and M. Meringer, J. Chem. Inf. Model., 2007, 47, 2345–2357 CrossRef PubMed.
- T. Rodrigues, B. P. de Almeida, N. L. Barbosa-Morais and G. J. L. Bernardes, Chem. Commun., 2019, 55, 6369–6372 RSC.
- N. T. Cockroft, X. Cheng and J. R. Fuchs, J. Chem. Inf. Model., 2019, 59, 4906–4920 CrossRef CAS PubMed.
- D. Parisi, M. F. Adasme, A. Sveshnikova, Y. Moreau and M. Schroeder, bioRxiv, 2019, 715094 Search PubMed.
- N. S. Madhukar, P. K. Khade, L. Huang, K. Gayvert, G. Galletti, M. Stogniew, J. E. Allen, P. Giannakakou and O. Elemento, Nat. Commun., 2019, 10, 5221 CrossRef PubMed.
- C. Harrison, Nat. Biotechnol., 2014, 32, 403–404 CrossRef CAS PubMed.
- E. C. Barnes, R. Kumar and R. A. Davis, Nat. Prod. Rep., 2016, 33, 372–381 RSC.
- E. K. Davison and M. A. Brimble, Curr. Opin. Chem. Biol., 2019, 52, 1–8 CrossRef CAS PubMed.
- R. Breinbauer, I. R. Vetter and H. Waldmann, Angew. Chem., Int. Ed., 2002, 41, 2879–2890 Search PubMed.
-
G. Karageorgis and H. Waldmann, in Chemical and Biological Synthesis, 2018, pp. 45–73 Search PubMed.
- S. L. Schreiber, Science, 2000, 287, 1964–1969 CrossRef CAS PubMed.
- D. S. Tan, Nat. Chem. Biol., 2005, 1, 74–84 CrossRef CAS PubMed.
- S. Yi, B. V. Varun, Y. Choi and S. B. Park, Front. Chem., 2018, 6, 507 CrossRef PubMed.
- R. W. Huigens 3rd, K. C. Morrison, R. W. Hicklin, T. A. Flood Jr, M. F. Richter and P. J. Hergenrother, Nat. Chem., 2013, 5, 195–202 CrossRef PubMed.
- R. J. Rafferty, R. W. Hicklin, K. A. Maloof and P. J. Hergenrother, Angew. Chem. Weinheim Bergstr. Ger., 2014, 126, 224–228 CrossRef.
- P. A. Wender, V. A. Verma, T. J. Paxton and T. H. Pillow, Acc. Chem. Res., 2008, 41, 40–49 CrossRef CAS PubMed.
- P. A. Wender, R. V. Quiroz and M. C. Stevens, Acc. Chem. Res., 2015, 48, 752–760 CrossRef CAS PubMed.
- G. Karageorgis, E. S. Reckzeh, J. Ceballos, M. Schwalfenberg, S. Sievers, C. Ostermann, A. Pahl, S. Ziegler and H. Waldmann, Nat. Chem., 2018, 10, 1103–1111 CrossRef CAS PubMed.
- M. Grigalunas, A. Burhop, A. Christoforow and H. Waldmann, Curr. Opin. Chem. Biol., 2020, 56, 111–118 CrossRef CAS PubMed.
-
M. Hartenfeller and G. Schneider, in Chemoinformatics and Computational Chemical Biology, ed. J. Bajorath, Humana Press, Totowa, NJ, 2011, pp. 299–323 Search PubMed.
- P. Schneider and G. Schneider, J. Med. Chem., 2016, 59, 4077–4086 CrossRef CAS PubMed.
- L. Friedrich, T. Rodrigues, C. S. Neuhaus, P. Schneider and G. Schneider, Angew. Chem., Int. Ed., 2016, 55, 6789–6792 CrossRef CAS PubMed.
- M. Hartenfeller, H. Zettl, M. Walter, M. Rupp, F. Reisen, E. Proschak, S. Weggen, H. Stark and G. Schneider, PLoS Comput. Biol., 2012, 8, e1002380 CrossRef CAS PubMed.
- D. Merk, F. Grisoni, L. Friedrich, E. Gelzinyte and G. Schneider, J. Med. Chem., 2018, 61, 5442–5447 CrossRef CAS PubMed.
- L. Friedrich, G. Cingolani, Y.-H. Ko, M. Iaselli, M. Miciaccia, M. G. Perrone, K. Neukirch, V. Bobinger, D. Merk, R. K. Hofstetter, O. Werz, A. Koeberle, A. Scilimati and G. Schneider, Adv. Sci., 2021, e2100832 CrossRef PubMed.
- B. Sanchez-Lengeling and A. Aspuru-Guzik, Science, 2018, 361, 360–365 CrossRef CAS PubMed.
- J. Meyers, B. Fabian and N. Brown, Drug Discovery Today, 2021, 26(11), 2707–2715 CrossRef CAS PubMed.
- A. Gupta, A. T. Müller, B. J. H. Huisman, J. A. Fuchs, P. Schneider and G. Schneider, Mol. Inf., 2018, 37, 1700111 CrossRef PubMed.
- R. Gómez-Bombarelli, J. N. Wei, D. Duvenaud, J. M. Hernández-Lobato, B. Sánchez-Lengeling, D. Sheberla, J. Aguilera-Iparraguirre, T. D. Hirzel, R. P. Adams and A. Aspuru-Guzik, ACS Cent. Sci., 2018, 4, 268–276 CrossRef PubMed.
- T. Blaschke, M. Olivecrona, O. Engkvist, J. Bajorath and H. Chen, Mol. Inf., 2017, 7, 1700123 Search PubMed.
- A. Kadurin, S. Nikolenko, K. Khrabrov, A. Aliper and A. Zhavoronkov, Mol. Pharm., 2017, 14, 3098–3104 CrossRef CAS PubMed.
- M. Olivecrona, T. Blaschke, O. Engkvist and H. Chen, J. Cheminf., 2017, 9, 48 Search PubMed.
- D. Merk, L. Friedrich, F. Grisoni and G. Schneider, Mol. Inf., 2018, 37, 1700153 CrossRef PubMed.
- D. Merk, F. Grisoni, L. Friedrich and G. Schneider, Commun. Chem., 2018, 1, 1–9 CrossRef.
- A. T. Müller, J. A. Hiss and G. Schneider, J. Chem. Inf. Model., 2018, 58, 472–479 CrossRef PubMed.
- S. Zheng, X. Yan, Q. Gu, Y. Yang, Y. Du, Y. Lu and J. Xu, J. Cheminf., 2019, 11, 5 Search PubMed.
- T. Sterling and J. J. Irwin, J. Chem. Inf. Model., 2015, 55, 2324–2337 CrossRef CAS PubMed.
- N. Bung, S. R. Krishnan, G. Bulusu and A. Roy, Future Med. Chem., 2021, 13, 575–585 CrossRef CAS PubMed.
-
J. J. Li and E. J. Corey, Total Synthesis of Natural Products: At the Frontiers of Organic Chemistry, Springer, Berlin, Heidelberg, 2012 Search PubMed.
- M. E. Maier, Org. Biomol. Chem., 2015, 13, 5302–5343 RSC.
- E. J. Corey and W. T. Wipke, Science, 1969, 166, 178–192 CrossRef CAS PubMed.
- B. Mikulak-Klucznik, P. Gołębiowska, A. A. Bayly, O. Popik, T. Klucznik, S. Szymkuć, E. P. Gajewska, P. Dittwald, O. Staszewska-Krajewska, W. Beker, T. Badowski, K. A. Scheidt, K. Molga, J. Mlynarski, M. Mrksich and B. A. Grzybowski, Nature, 2020, 588, 83–88 CrossRef CAS PubMed.
- S. Szymkuć, E. P. Gajewska, T. Klucznik, K. Molga, P. Dittwald, M. Startek, M. Bajczyk and B. A. Grzybowski, Angew. Chem., Int. Ed., 2016, 55, 5904–5937 CrossRef PubMed.
- E. P. Gajewska, S. Szymkuć, P. Dittwald, M. Startek, O. Popik, J. Mlynarski and B. A. Grzybowski, Chem, 2020, 6, 280–293 CAS.
- J. Li, S. G. Ballmer, E. P. Gillis, S. Fujii, M. J. Schmidt, A. M. E. Palazzolo, J. W. Lehmann, G. F. Morehouse and M. D. Burke, Science, 2015, 347, 1221–1226 CrossRef CAS PubMed.
- C. W. Coley, D. A. Thomas 3rd, J. A. M. Lummiss, J. N. Jaworski, C. P. Breen, V. Schultz, T. Hart, J. S. Fishman, L. Rogers, H. Gao, R. W. Hicklin, P. P. Plehiers, J. Byington, J. S. Piotti, W. H. Green, A. J. Hart, T. F. Jamison and K. F. Jensen, Science, 2019, 365, eaax1566 CrossRef CAS PubMed.
|
This journal is © The Royal Society of Chemistry 2022 |
Click here to see how this site uses Cookies. View our privacy policy here.