DOI:
10.1039/D2SC02728C
(Edge Article)
Chem. Sci., 2022,
13, 14052-14062
Peptide nano ‘bead-grafting’ for SDT-facilitated immune checkpoints blocking†
Received
17th May 2022
, Accepted 13th November 2022
First published on 23rd November 2022
Abstract
Combination therapies based on immune checkpoint blockade (ICB) are currently the mainstay of cancer treatment, in which the synergetic delivery of multiple drugs is the essential step. Although nanoparticle drugs (NPDs) show satisfactory anticancer effects, the promotion of active co-delivery of NPDs is premature, since the processes are usually difficult to predict and control. Targeting peptide self-assemblies have been widely used as carriers for small-molecular drugs, but remain elusive for NPDs. We describe here peptide-based nano ‘bead-grafting’ for the active delivery of quantum-dot NPDs through a co-assembly method. Based on a ‘de novo’ design, we used a ‘one-bead-one-compound (OBOC)’ combinatorial chemical screening method to select a peptide RT with high affinity for the immune checkpoint CD47, which could also form biocompatible nanofibers and efficiently trap Ag2S quantum dots along the self-assembly path. This system can combine ICB therapy and sonodynamic therapy (SDT) to effectively inhibit tumor growth. Moreover, the tumor antigen produced by SDT can activate the adaptive immune system, which enhances the anti-tumor immune response of the ICB and shows efficient inhibition of both primary and distant tumors. This study provides a new strategy for the active control and delivery of NPDs and a new option for ICB therapy with immune checkpoints that are highly susceptible to systemic side effects.
Introduction
As a ‘don't eat me’ signal, CD47 (Cluster of differentiation 47) is closely associated with the development and progression of cancers.1,2 Through binding to SIRPα (signal regulatory protein α) it can inhibit macrophage phagocytosis and thus cause immune escape.3 Blocking the CD47/SIRPα axis enhances the phagocytosis of macrophages and the cross-presentation of dendritic cells.4 In recent years, various efforts have been made to block CD47/SIRPα interactions, in particular in antibody development.5,6 Unfortunately, severe hemolytic reactions caused by antibodies are a major barrier to achieving clinical translation, which result from CD47 also being expressed on normal red blood cells.7,8 Besides possessing recognition properties similar to antibodies, targeting peptides also have the advantage of low immunogenicity, minimizing the risk of systemic toxicity though rational design.9,10 Therefore, targeting peptides are the most promising alternatives to antibodies for immune checkpoint blockade (ICB) in the CD47/SIRPα axis.11,12 However, current targeting peptides are mostly derived from the modification of natural peptides and it is difficult to achieve breakthroughs in their performance, so there is an urgent need to develop new targeting peptides. In addition, ICB has shown remarkable success in the treatment of patients with advanced disease, but clinical results show that the majority of patients do not benefit from it.13,14 To address this dilemma, the combination of ICB with other therapies (chemotherapy, photodynamic therapy, etc.) is the mainstay of current treatment and has shown excellent anti-tumor effects.15,16
The assemblies formed by targeting peptides can both recognize immune checkpoints and act as drug carriers. Therefore, they possess unique advantages for combination therapy against tumors. Among various types of peptides, surfactant-like peptides (SLPs) have a structure similar to that of natural surfactants. The assemblies they form have hydrophilic residues displayed on the surface and hydrophobic residues hidden in the core of the assembly.17 Based on this feature, a variety of different SLPs have been designed and their applications in biomineralization, as antimicrobial agents and in drug delivery have been reported.18–20 For example, the surfactant-like structural domains of SLPs can bind to membrane proteins, thereby enhancing their stabilization in aqueous media.21 The dense peptide epitopes formed on the surface of SLP assemblies can be used for tissue regeneration.22 The hydrophobic regions formed by the assembly of SLPs can accommodate hydrophobic drug molecules, such as paclitaxel, doxorubicin and etomidate, for delivery into tumor cells.23–25 In addition, as an emerging cancer treatment strategy, sonodynamic therapy (SDT) uses ultrasound (US) as a radiation source to excite sonosensitizers for the production of reactive oxygen species (ROS), which directly kill or induce apoptosis of tumor cells.26–29 Compared with photodynamic therapy (PDT), it has high tissue penetration capability, non-ionizing properties, controllability and low cost.30 Most importantly, research has shown that SDT has the potential to act as a cancer vaccine to promote increased levels of infiltrating CD4+ and CD8+ T lymphocytes in tumor tissue, promote dendritic cell (DC) maturation and reverse immunosuppression.29,31–33 This is certainly the icing on the cake for enhancing the anti-tumor immune response. Regrettably, many sonosensitizers have poor pharmacokinetic behaviors and may be cleared from the vasculature, thus greatly reducing their exposure at the tumor tissue.34 The development of efficient and biocompatible carriers for SDT is undoubtedly crucial.35
In fact, we can see scenarios in which various drug carriers are explored.36,37 The holy grail in this field is the development of tools that specifically reach the site of the lesion.38 To date, different strategies have been developed, such as chemical conjugation and physical encapsulation. Among them, physical encapsulation is currently the preferred strategy due to its simplicity and high drug loading capacity.39–41 However, this approach mostly relies on hydrophobic cavities or surface adsorption to load the drugs and enrich them in the lesion site by an enhanced penetration and retention (EPR) effect, which is susceptible to off-site release during circulation. In addition, this strategy mainly focuses on small hydrophobic molecular drugs, and remains elusive for nanoparticle-based drugs (e.g., QDs).42 The active delivery of QDs is known to be notoriously difficult at present. Due to their large size and rigid property, delivery is currently achieved mainly by modifying targeted peptides or antibodies on their surface.43 However, this approach needs complex protocols, including chemical modification.44 These processes are usually difficult to predict and control. On the other hand, in combination therapy, surface modifications often lead to difficulties in synergy between QDs and modified units.
In this context, physically encapsulated carriers with active targeting and stably encapsulated nanoparticle-based QD capabilities are urgently needed. Nanofibers formed by the self-assembly of peptides have been widely used as physical encapsulation carriers due to their stability, safety, long retention time and their ability to act as a drug themselves.45,46 However, most lack active targeting capabilities and are powerless to deliver nanoparticle-based QDs. Therefore, it is of great interest to design new peptide nanofibers with active delivery whilst stably encapsulating QDs.
In this study, based on the analysis of peptide nanofiber assembly pathways, we have attempted to construct integral peptide nanofibers that both controllably deliver QD sonosensitizers and block the CD47/SIRPα axis. Unlike conventional peptide nanofibers that adsorb nanoparticles on their surface, this system traps QDs into the inner-fiber during the orientated growth and maturation of the protofibrils, ultimately forming an integral peptide–sonosensitizer ‘bead-grafting’ structure (Scheme 1a). We explain its QD control and delivery mechanism and demonstrate that it could combine ICB and SDT to achieve efficient tumor inhibition. Furthermore, we demonstrate that SDT-treated tumor cells can release tumor antigens to activate the adaptive immune system, and that the combination with ICB not only inhibits the primary tumor but also effectively kills distant tumors (Scheme 1b).
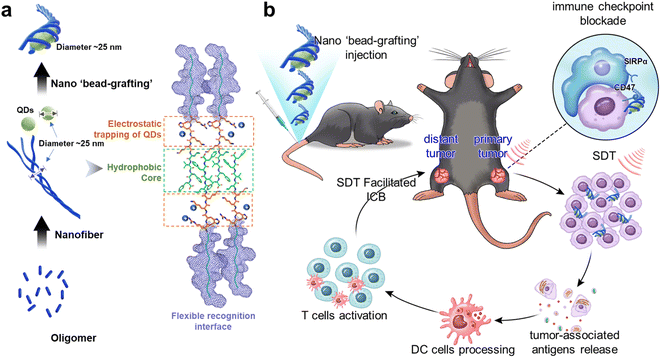 |
| Scheme 1 The formation process of ‘bead-grafting’ nanostructures and the mechanisms of ICB combined with SDT to elicit an anti-tumor immune response. RT nanofibers carry QDs for active transport to tumor tissue. On the one hand, the system blocks the CD47/SIRPα axis to achieve immune checkpoint blockade; on the other hand, the ROS produced by SDT kill tumor cells and release tumor antigens. The tumor antigens are taken up and processed by DC cells, further activating the adaptive immune system. This powerful anti-tumor immune response has a significant therapeutic effect on both primary and post-metastatic distant tumors. | |
Results and discussion
Design and screening of CD47-targeting peptides
We designed surfactant-like peptides (SLPs) to achieve both the active delivery of carboxyl-modified Ag2S quantum dots (QDs) and the targeting of CD47. On the one hand, SLPs consist of hydrophilic head groups and hydrophobic tails. The self-assembly into nanofibers can rely on the hydrophobic collapse of the tail to drive rapid aggregation of the peptides, while the hydrophilic or charged head group tends to be exposed to the surface.17,24 Therefore, negatively charged QDs have the potential to be trapped by positively charged fibers. On the other hand, since the CD47/SIRPα binding interface is mostly dominated by hydrophilic residues,47 the enrichment of hydrophilic residues in the SLP head group has the potential to increase the affinity of the peptide for CD47. Based on the above analysis, in order to obtain a head group sequence with high affinity for CD47, we first performed ‘one-bead-one-compound (OBOC)’ combinatorial chemical screening of the head group sequence.48 The length of the peptide chain was set to 10 residues and the general formula can be expressed as NH2–H1X2X3X4X5X6X7X8X9X10–COOH, where the candidates at the different sites are shown in Fig. S1.† To enable negatively charged QDs to be trapped in the fiber, X10 and X9 at the C terminal were set as positively charged residues (R, K, H). In order to ensure that the targeting peptide could specifically bind tumor cells rather than red blood cells (RBCs), X1 was set as H for the hydrogen bonding formation toward the free Asn residues on the tumor-expressed-CD47 loops. These Asn residues were reported to be glycosylated on RBC-expressed-CD47.49 Based on the library design, we obtained a leading peptide (sequence: HFEYWEERHK) according to the previous screening protocol.50,51 Then, the hydrophobic motif ‘LVFF’ was attached to its C-termina. This approach allows the positively charged region to be concentrated at the hydrophilic and hydrophobic interface. We named the final sequence RT (sequence: HFEYWEERHKKLVFF) (Fig. 1a). Fmoc solid-phase synthesis was used to prepare the peptide and its purity was determined (Fig. S2†). We confirmed the targeting and selectivity of RT at both the molecular and cellular levels. Molecular docking was first used to predict the potential binding sites of RT in CD47. As shown in Fig. 1b, RT is capable of binding to CD47 with major binding sites, including His1–Asn55 (hydrogen bonding) and Trp5–Val70 (hydrophobic interactions), among which the Asn55 of CD47 is the critical glycosylation site on red blood cells. Surface plasmon resonance imaging (SPRi) results showed that the dissociation constant (KD) of RT was 3.18 × 10−7 M (Fig. 1c) toward CD47, which is higher than that of their natural ligands.52 Next, confocal microscope imaging was used to verify the targeting and specificity of RT at the cellular level. Mouse colon cancer cell line CT26 with CD47 high expression were selected as a positive model and embryonic kidney cell line 293T with CD47 low expression were used as a negative cell model. Both cells were incubated with FITC-labeled RT, respectively. As shown in Fig. 1d, there was bright fluorescence on the membranes of CT26 cells (green channel), while little fluorescence appeared on the 293T cells (Fig. 1e). In addition, CT26 cells were transfected with CD47-siRNA. As shown in Fig. 1f, compared with the untreated cells, fluorescence was substantially lost on the CT26 cells. The above results demonstrated that RT has selective binding ability. Finally, a competitive binding assay was used to further demonstrate the ability of RT to block the binding between CD47 and its natural ligand SIRPα. RT and Cy5-labeled SIRPα were first mixed and then incubated with CT26 cells. As shown in Fig. 1g, SIRPα incubated with CT26 showed bright fluorescence on the cell membrane (red channel), whereas when RT was co-incubated with SIRPα, the fluorescence on the cell membrane almost disappeared, indicating that RT was able to efficiently block the CD47/SIRPα axis and so shows potential as an inhibitor.
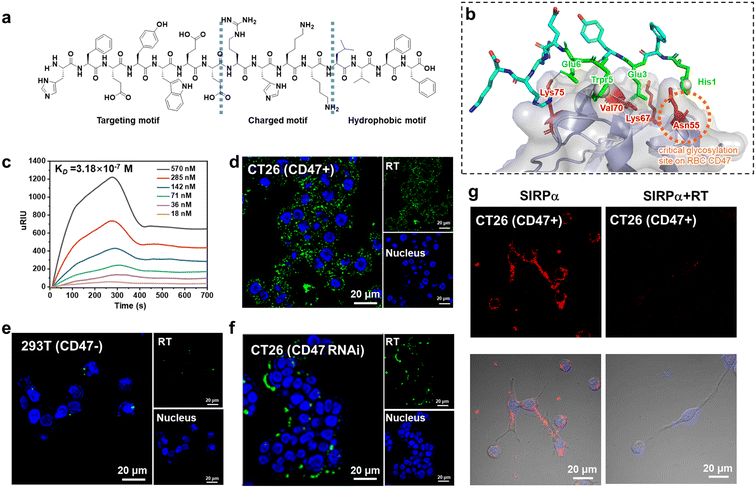 |
| Fig. 1 (a) The chemical structure of the RT molecule and an illustration of the different functional regions. (b) The structure of RT/CD47 predicted with crystal molecular docking. (c) SPRi results between RT and CD47. Confocal images of FITC-RT treated MC38 cells (d) and 293T cells (e). (f) Confocal images of CD47-siRNA-transfected CT26 cells incubated with FITC-RT. (g) Competition experiments of CT26 cells incubated with SIRPα or a mixture of RT and SIRPα. | |
Co-assembly of RT peptide with QDs
The formation of peptide nanofibers is a nucleation-dependent process. In this process, the peptide monomers are first primitively nucleated in solution, then the filaments grow linearly and undergo secondary nucleation to rapidly form protofibrils. Finally, the protofibrils intertwine with each other to form mature nanofibers. This is the conventional pathway for nanofiber formation.53–55 What we were curious about was whether it was possible to intervene with hydrophilic QDs during the maturation of the fibers so that the drug could be trapped by the fibers and form the whole. This structure is expected to be more stable than the usual physical encapsulation and is less likely to be released prematurely in circulation (Fig. 2a). In fact, in our system, it is highly likely that positively charged regions will capture negatively charged QDs during fiber maturation. To verify this notion, we first analyzed the assembly behavior of RT. As shown in Fig. 2b, at 30 min, a large number of oligomers appeared and they further aggregated to form larger structures. From the magnified view these structures were observed to be composed of fibrils. This indicates that RT can undergo nucleation in a short time. With increasing time, a large number of protofibrils could be seen at 6 h and there is a tendency for further entanglement between the protofibrils (magnified view). After 24 h of assembly, numerous mature nanofibers were formed, with their length increasing to microns and a diameter of about 25 nm. In addition, fibrous entangled structures can still be observed, indicating a tendency for further assembly. In summary, the assembly pathway of RT is consistent with the classical nucleation-growth process. Then, we added QDs to the system at the further entanglement stage of the protofilament (6 h), expecting it to trap QDs without affecting its growth. The AFM results for the QDs are shown in Fig. S3,† indicating that the QDs are around 25 nm in diameter. After co-assembly, the morphology shows that the QDs were encapsulated within the peptide nanofibers, forming a ‘bead-grafting-like’ structure (RTQDs). AFM height profiles showed the variation in height along the fiber pathway (Fig. 2c). In addition, Fig. 2d shows the more detailed structure. Element mapping results of RTQDs demonstrated the existence of Ag, S, C, O in the structure (Fig. S4†). Fig. 2e shows the elemental energy dispersive spectroscopy (EDS) mapping performed on the RTQDs. The results show that Ag and S overlapped, indicating that Ag2S was intact in the system. By comparing the distribution areas of Ag, S and O, it was found that Ag and S fell within the range of O, indicating that the QDs were encapsulated in the nanofibers. Circular dichroism (CD) results illustrated that RT nanofibers encapsulating QDs were formed based on a β-sheet secondary structure (Fig. 2f). After co-assembly, we kept them at room temperature for 1 month. Fig. S5† shows that the QDs in the RTQDs appear to sink with the nanofibers compared to the QD solution alone, indicating that the QDs can be stably encapsulated in the nanofibers over time. Then, we verified whether the affinity and specificity of the nanofibers formed by RT were maintained at the cellular level. We used tetraphenylethylene (TPE) conjugated RT with aggregation-induced emission (AIE) effects to confer a fluorescence property on the nanofibers. CT26 cells (CD47+) and 293T cells (CD47−) were incubated with the nanofibers. As shown in Fig. 2g, bright fluorescence was observed on CT26 cells, while fluorescence was very faint on 293T cells, indicating that the assembled RT still exhibits excellent targeting and selectivity properties. Finally, we validated the targeting of the integrated RTQDs. As shown in Fig. 2g and S6,† after incubation with RTQDs, a large amount of fluorescence from QDs appeared on CT26 cells while there was almost none on 293T cells, demonstrating that RTQDs effectively target CD47 positive cells.
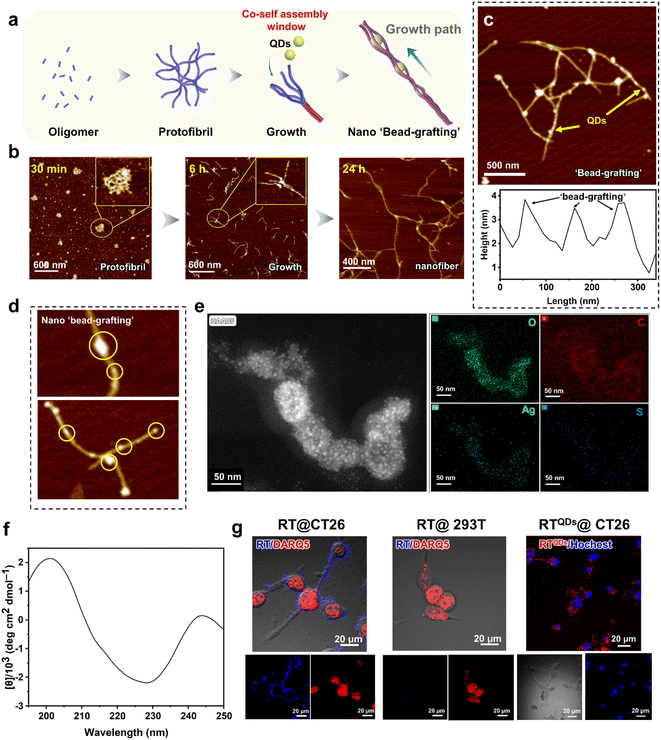 |
| Fig. 2 Characterization of RT and QDs co-assembled into ‘bead-grafting’ structures. (a) The nucleation-growth process and the trapping of QDs in peptide nanofibers. (b) Evolution of RT assembly behavior over time. (c) AFM images and height profile of RTQDs. (d) Details of RTQDs forming ‘bead-grafting’ structures. (e) EDS mapping performed on the RTQDs. (f) CD results for RTQDs. (g) Confocal images of RT nanofibers incubated with CT26 and 293T cells, respectively, and RTQDs incubated with CT26 cells. | |
In vitro sonodynamic therapy (SDT) and biocompatibility
We first examined the production of ROS during sonication of RTQDs with the probe 1,3-diphenylisobenzofuran (DPBF). As shown in Fig. 3a, the absorbance decreased obviously with increasing sonication time, indicating a large amount of ROS production. The ROS at the cellular level was also verified. As shown in Fig. 3b and c, compared with PBS and QDs (−) groups, the fluoresce in QDs (+) and RTQDs (−) groups was enhanced to a certain extent. It is notable that ROS were greatly enhanced in the RTQDs (+) group. Such results indicated that RT-encapsulated QDs could enhance ROS in cells. We then demonstrated the effectiveness of acoustic dynamics in killing tumor cells. A calcein-AM/PI cell-survival assay was conducted to distinguish live cells (green fluorescence) and dead cells (red fluorescence). As shown in Fig. 3d, a portion of the cells died when treated with QDs (−), suggesting the SDT-generated ROS induce cell apoptosis. In the case of RTQDs (−), some of the cells also died, but the cells died almost completely when treated with RTQDs (+), indicating that RT wrapping of QDs significantly enhanced the sonodynamic effect. The killing effect on cells of RT wrapping different concentrations of QDs was evaluated using a CCK-8 assay. As shown in Fig. 3e, without sonication, when the concentration of QDs was increased to 50 μg mL−1, the cells were still retained 68% viability. However, cell viability decreased rapidly when treated with ultrasound, and was only 9.2% when the QD concentration was increased to 50 μg mL−1. These results further suggested that ROS generated by SDT can efficiently kill tumor cells.
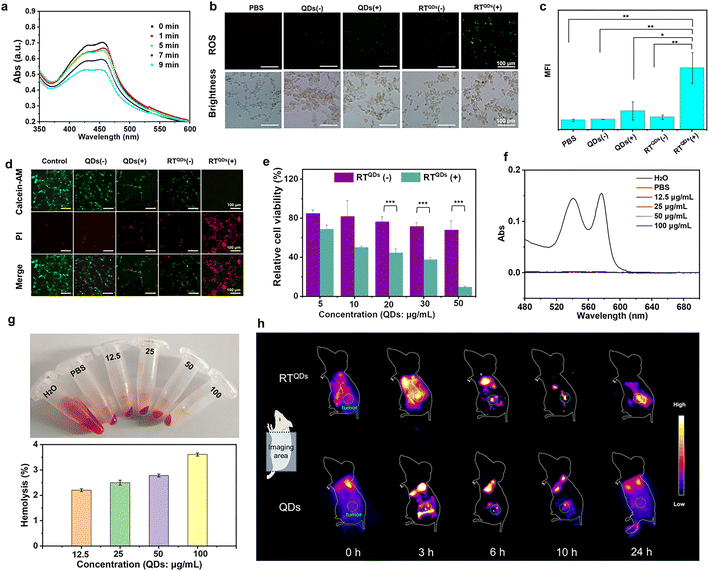 |
| Fig. 3 (a) Detection of ROS generation of RTQDs by the probe DPBF. (b) Detection of ROS production in different treatment groups at the cellular level. (c) Mean fluorescence intensity (MFI) statistics of DCFH-DA in different groups. Data are shown as means ± s.d. (n = 3). (d) Calcein-AM/PI cell-survival assay for the detection of cell viability in different treatment groups. (e) CCK-8 assay to detect the killing effect of RTQDs at different concentrations with and without sonication. Data are shown as means ± s.d. (n = 3). (f) UV-vis spectroscopy of supernatant after treatment of red blood cells with different concentrations of RT. (g) Hemolysis rates and samples after co-assembly of RT (1 mg mL−1) and different concentrations of QDs. (h) In vivo fluorescence imaging of living mice at different time points post injection of QDs and RTQDs. Data are shown as means ± s.d. (n = 3). ***P < 0.001, **P < 0.01, *P < 0.05; P values were determined with one-way analysis of variance (ANOVA) followed by Post Hoc Tukey's test for the indicated comparison. | |
As a specific immune checkpoint, CD47 is also expressed on red blood cells.56 The severe hemolytic effect is a major barrier to the clinical development of antibody-based inhibitors. Therefore, the hemolytic effect is a prerequisite to test whether a drug can be applied in vivo. In our system, the CD47 binding site of RT on blood red cells is covered by glycosylation modifications and therefore theoretically does not have a hemolytic effect. We then verified the hemolytic effect of RT and different concentrations of QDs after co-assembly. As shown in Fig. 3f and g, the supernatant of RT-incubated red blood cells showed a colorless state even at high concentration. The absorbance of the supernatant was measured to demonstrate that RT has almost no hemolytic effect. Finally, to investigate the biodistribution and accumulation of RTQDs in tumor tissues, we carried out an in vivo real-time imaging assay. As shown in Fig. 3h and S19,† effective accumulation was observed in the tumors of the RTQDs-treated group, with a bright fluorescent signal being observed at 6 h after injection and significant fluorescence intensity remained 24 h post injection. After 24 h, all the mice were sacrificed and the major organs were collected. Fig. S7† shows fluorescence images of the biodistribution for the excised organs. It is shown that the fluorescence intensity of the tumors in the RTQDs-treated group was significantly higher than that of the control group. In addition, the fluorescence of the heart, liver, spleen, lung, kidney and tumors was faint. These results demonstrated that tumor-targeted RT could efficiently carry QDs to tumor tissue with low impact on normal tissue.
In vivo tumor therapy by RTQDs toward CD47-positive tumors
Since RTQDs can combine immune checkpoint blockade and SDT to kill tumor cells, we next confirmed its anti-tumor effects at the in vivo level. CT26 tumor bearing C57BL/6 mice were randomly divided into 5 groups (n = 6). The mice were intravenously treated with PBS, QDs (ultrasound, +), RT, RTQDs (without ultrasound, −), or RTQDs (ultrasound, +). The treatment time and frequency are shown in Fig. 4a. Tumor volume and body weight continued to be monitored for 5 days after the treatment. As shown in Fig. 4b and c, the RTQDs (+) treated group was the most significant, with some of the tumors being completely eradicated, indicating that ICB combined with SDT can efficiently kill tumor cells. In contrast, the RT and RTQDs (−) groups, in which only peptides were used for immune checkpoint blockade, were slightly less effective. However, only the QDs (+) treated tumors showed no significant difference with the PBS group, indicating that the delivery of RT nanofiber carriers is essential for the accumulation of QDs in the tumor tissue and to enhance the anti-tumor effect. We next performed weight analysis of the excised tumors (Fig. 4d) and the results were consistent with the tumor volume outcome. The body weights of all the mice were maintained at normal levels during the therapeutic process, indicating its biocompatibility (Fig. S8†). The ROS in tumor tissues were examined and the results showed that the level of ROS in the RTQDs (+) treatment group was significantly higher than in the other groups, indicating that RT can effectively capture QDs to reach the tumor tissue and generate more ROS to achieve tumor killing under the action of ultrasound (Fig. 4e, S9 and S20†). Furthermore, the release of high mobility group box protein 1 (HMGB1) into an extracellular matrix can activate the maturation of dendritic cells and trigger the secretion of pro-inflammatory cytokines. Therefore, we tested the HMGB1 and the results are shown in Fig. 4f, S10 and S21.† It can be seen that RTQDs (+) can effectively secrete HMGB1 compared to the other groups. This reveals its effectiveness in increasing immunogenicity and making the treatment effective. We also examined CD80+/CD86+ dendritic cells (DC) in tumor tissue from different treated groups (Fig. 4g, S11 and S22†). The results showed a significant increase in mature DC cells in RTQDs (+) tumors, suggesting that RT transported QDS increased the SDT effect and promoted the maturation of DC cells. Then, organs such as heart, spleen, kidney, liver and lung were extracted after treatment to perform hematoxylin–eosin (H&E) and terminal deoxynucleotidyl transferase dUTP Nick-End labeling (TUNEL) experiments. As shown in Fig. 4h and S12,† compared to the PBS-treated group, the RTQDs (+) treated group suffered severe damage to the tumor tissue while there were no obvious pathological changes to or any other adverse effects on normal organs, which further demonstrated its biocompatibility. In summary, immune checkpoint blockade using RT alone does not achieve optimal tumor inhibition, while RT efficiently transports QDs to enrich them in tumor tissue, which combined with SDT can effectively inhibit tumor growth. Meanwhile, the excellent biocompatibility indicates its potential for clinical application. To provide further insight into the overall molecular mechanisms of inhibition at the biomolecular level, RNA sequencing (RNA-seq) based transcriptomic approaches were used to map the genetic profile of the treated tumors. Tumors in the RTQDs (+) and QDs (+) treated groups were compared with the PBS treated group, respectively. Heatmaps of differentially expressed genes in these groups emerged (Fig. S13†). To identify the number of genes that differed between all groups, a volcano plot was drawn in which upregulated or downregulated genes were marked in red and blue colors (Fig. S14 and S15†). Furthermore, we used Gene Ontology (GO) analysis and the Kyoto Encyclopedia of Genes and Genomes (KEGG) to identify the biological process and molecular pathways that were affected. From GO analysis we can see that the RTQDs (+) treatment group alters pathways related to cytokine secretion, metabolic processes of reactive oxygen species, regulation of the inflammatory response, etc (Fig. 4i). As a comparison, these processes were less altered in the QDs (+) treated group (Fig. 4j), suggesting that RTQDs (+) combined with ICB and SDT markedly modulate the immune response and the production of ROS. In addition, KEGG results suggest that RTQDs (+) alters the TNF (tumor necrosis factor) signaling pathway, cytokine–cytokine receptor interaction and Toll-like receptor signaling pathway (Fig. 4k). These are related to anti-tumor and immunity responses. QDs (+) have less impact on these pathways (Fig. S16†). Finally, detailed CD47-related genetic factors were listed (Fig. 4l), and it can be observed that many positive regulators of CD47 were downregulated in the RTQDs (+) treated group, whereas this was not evident in the QDs group (+).
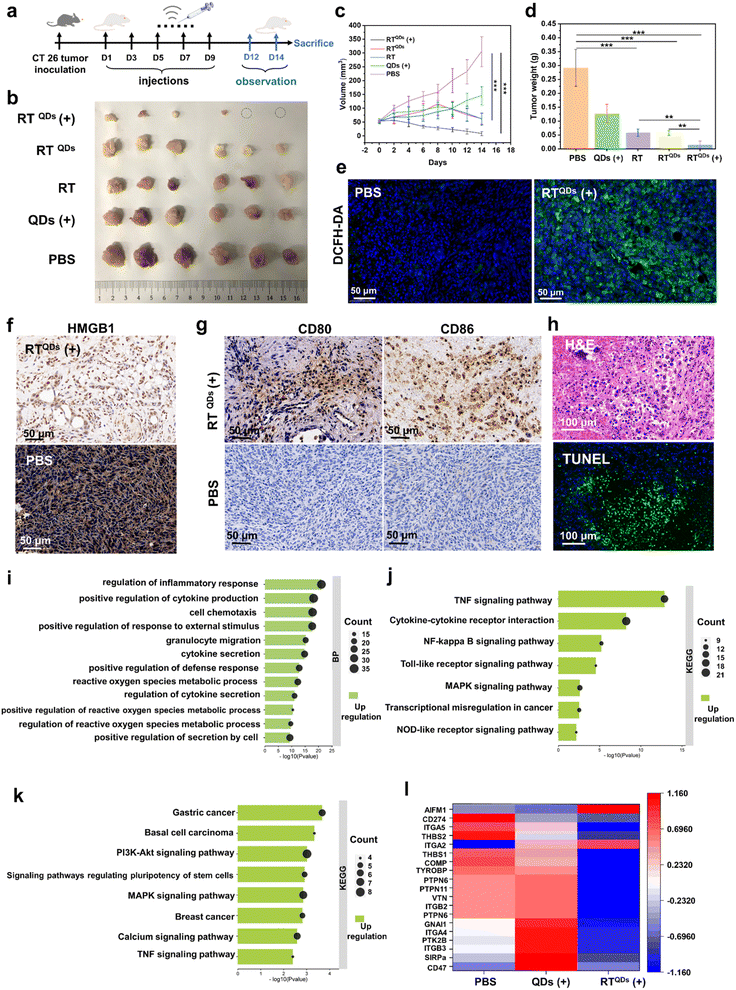 |
| Fig. 4
In vivo therapy of RTQDs in CT26 tumor-bearing mice. (a) Timeline for treatment of C57BL/6N mice with CT26 tumors (n = 6). (b) Images of tumors after treatment in different groups (n = 6). (c) Tumor volume change curves during treatment in different groups (n = 6). (d) Tumor weights of different groups at the end of treatment (n = 6). (e) ROS levels in RTQDs (+) treated and control tumor tissues (n = 3). (f) Immunofluorescence staining results of HMGB1 in RTQDs (+) and control tumor tissues (n = 3). (g) CD80+/CD86+ dendritic cells (DC) in tumor tissue from RTQDs (+) and control groups (n = 3). (h) H&E and TUNEL staining of tumors after receiving RTQDs (+) treatment. BP map for GO analysis (i) and KEGG analysis (j) of altered genes in RTQDs (+) treated group. (k) BP map for GO analysis of altered genes in QDs (+) treated group. (l) Changes in CD47-related factors after RTQDs (+) and QDs (+) treatment. Data are mean ± s.d., and n represents the number of biologically independent samples. Statistical analysis was performed using one-way ANOVA followed by Post Hoc Tukey's test for the indicated comparison; **P < 0.01, ***P < 0.001. | |
Inhibition of primary and distant tumors by RTQDs
To understand the mechanism of the enhanced anti-tumor immune response of RTQDs, the therapeutic effect on distant tumors was assessed using a bilateral tumor model. CD47 positive tumor cells were injected into the left and right flanks of C57BL/6 mice to create a bilateral tumor model. The tumor on the right was designated as the primary tumor for local ultrasound irradiation and the left tumor was designated as the distant tumor. The mice were randomly divided into 4 groups and intravenously injected with PBS, QDs, RT and RTQDs, respectively. Injections were given every 2 days for a total of 4 times, and ultrasound irradiation was performed on the primary tumor after 6 h of injection (Fig. 5a). Fig. 5b–d summarize the treatment outcomes of the different groups. Compared to the PBS group, there was little effect from QDs (+) alone. RT can block the CD47/SIRPα pathway, enhancing the phagocytosis of macrophages and the cross-presentation of dendritic cells, so it has shown moderate inhibition of both primary and distant tumors. In contrast, RTQDs (+) obviously inhibited both primary and distant tumors. The above results suggest that RT-mediated ICB inhibits both primary and distant tumors, and this function is further enhanced by the combination of SDT. This phenomenon may occur because the tumor cell death caused by SDT is able to release tumor-associated antigens that trigger the maturation of dendritic cells. Then, mature dendritic cells can deliver antigens to CD4+ (T helper cells) and CD8+ (cytotoxic T cells), and the activated T cells can infiltrate tumor tissue to exert anti-tumor effects. The immunofluorescence images can support this conclusion. As can be seen in Fig. 5e and f, the results showed that CD4 and CD8 expression was markedly increased in primary and distant tumor tissues in the RTQDs (+) treatment group, but nonsignificant in tissues from the other groups (Fig. S17†). In addition, we investigated the effect of macrophages in tumor tissue. The results showed that the expression of CD68 was increased in the RT and RTQDs (+) treated groups, suggesting that blockade of CD47/SIRPα pathway by ICB or combined with SDT could increase macrophage infiltration in tumor tissue. Overall, in the bilateral tumor model, RT-mediated ICB combined with SDT efficiently inhibited both primary and distant tumors. Furthermore, RT combined with SDT resulted in increased levels of infiltrating CD4+ and CD8+ cells, suggesting that SDT-treated tumor cells can act as antigens to activate the adaptive immune system. Finally, we comprehensively validated the biocompatibility of RTQDs. The bodyweights of all the mice remained regular during the therapeutic process (Fig. S18†). After treatment, routine blood examination (Fig. 5g) and blood chemistry (Fig. 5h) results remained at normal levels. These results show that RTQDs have the potential to achieve clinical translation.
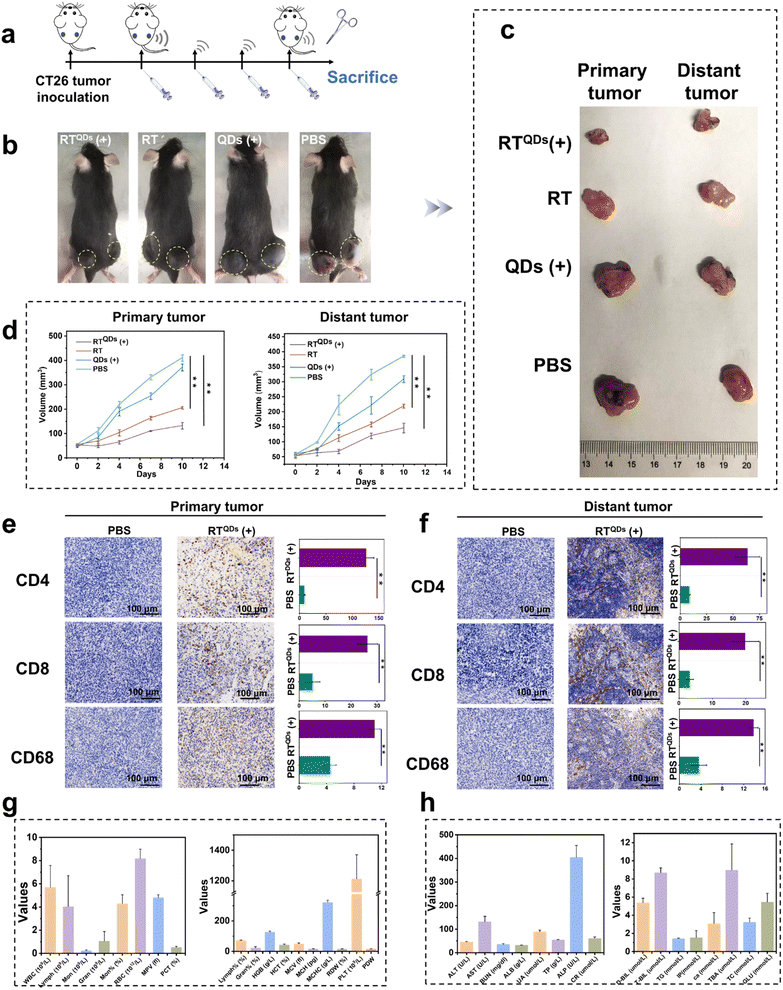 |
| Fig. 5 Treatment of primary and distant tumors. (a) Treatment process for bilateral tumors. (b) Representative images of bilateral tumor-bearing mice in different treatment groups. (c) Representative images of the excised tumor at the end of the treatment. (d) Curves of primary and the distant tumor volume changes during treatment in different groups. Immunofluorescence staining and statistical results of CD8, CD4 and CD68 in primary (e) and distant tumors (f) after treatment with PBS or RTQDs (+). Note: Fig. S17† has the same PBS results. Routine blood examination (g) and blood biochemistry analysis (h) of mice after treatment with RTQDs (+). Data are shown as means ± s.d. (n = 3), and n represents the number of biologically independent samples. Statistical analysis was performed using one-way ANOVA followed by Post Hoc Tukey's test for the indicated comparison; **P < 0.01. | |
Conclusion
Peptide assemblies have been widely used both as drugs and as drug carriers,57 which certainly has inherent advantages for combination therapy. However, achieving the active delivery of nano-scaled drugs is still insufficient. In fact, most targeting peptide assemblies originate from natural sequences, which makes it difficult to improve their performance. Therefore, there is an urgent need for new targeting peptide molecules. In this study, we designed a surfactant-like peptide library and selected a peptide with high affinity for the immune checkpoint CD47 by using a ‘one bead one compound (OBOC)’ combinatorial chemical peptide library screening strategy. The peptide is able to self-assemble into nanofibers and efficiently trap QDs in the self-assembly path. We demonstrated its ability to kill CD47-positive tumor cells while protecting red blood cells from damage. It could efficiently inhibit tumor growth at the in vivo level in combination with ICB and SDT. Notably, we demonstrated that tumor cells killed by SDT can release tumor-associated antigens to activate the adaptive immune system and facilitate ICB for both primary and distant tumors. Overall, through rational peptide molecular design and efficient screening, we have provided a new therapeutic platform with potential clinical applications.
Data availability
All other relevant data are available from the corresponding authors upon reasonable request.
Author contributions
W. Wang supervised the project, and finalized the manuscript. L. Zhang conceived the idea and carried out the experiments. Y. Tian and M. Li analyzed the data. M. Wang and S. Wu assisted in material synthesis and characterization. Z. Jiang and Q. Wang assisted in the analysis of the data. All authors have read, commented and given approval to the final version of the manuscript.
Conflicts of interest
The authors declare no competing financial interests.
Acknowledgements
The authors are grateful for financial support from National Natural Science Foundation of China (22074006), Beijing Natural Science Foundation (2222029), Beijing Institute of Technology Research Fund Program for Young Scholars. We thank Analysis and Testing Center in Beijing Institute of Technology on the experimental data acquisition. We thank Prof. Yeteng Zhong and Dr Chenlei Wang from National Center for Nanoscience and Technology of China for their help on in vivo imaging.
References
- J. Theruvath, M. Menard, B. A. H. Smith, M. H. Linde, G. L. Coles, G. N. Dalton, W. Wu, L. Kiru, A. Delaidelli, E. Sotillo, J. L. Silberstein, A. C. Geraghty, A. Banuelos, M. T. Radosevich, S. Dhingra, S. Heitzeneder, A. Tousley, J. Lattin, P. Xu, J. Huang, N. Nasholm, A. He, T. C. Kuo, E. R. B. Sangalang, J. Pons, A. Barkal, R. E. Brewer, K. D. Marjon, J. G. Vilches-Moure, P. L. Marshall, R. Fernandes, M. Monje, J. R. Cochran, P. H. Sorensen, H. E. Daldrup-Link, I. L. Weissman, J. Sage, R. Majeti, C. R. Bertozzi, W. A. Weiss, C. L. Mackall and R. G. Majzner, Nat. Med., 2022, 28, 333–344 CrossRef CAS PubMed.
- E. Eladl, R. Tremblay-LeMay, N. Rastgoo, R. Musani, W. Chen, A. Liu and H. Chang, J. Hematol. Oncol., 2020, 13, 96 CrossRef PubMed.
- Z. Jiang, H. Sun, J. Yu, W. Tian and Y. Song, J. Hematol. Oncol., 2021, 14, 180 CrossRef CAS PubMed.
- R. Majeti, M. P. Chao, A. A. Alizadeh, W. W. Pang, S. Jaiswal, K. D. Gibbs Jr, N. van Rooijen and I. L. Weissman, Cell, 2009, 138, 286–299 CrossRef CAS PubMed.
- W.-B. Yu, Z.-H. Ye, X. Chen, J.-J. Shi and J.-J. Lu, Drug Discovery Today, 2021, 26, 561–568 CrossRef CAS PubMed.
- Y. Pan, J.-P. Volkmer, K. E. Mach, R. V. Rouse, J.-J. Liu, D. Sahoo, T. C. Chang, T. J. Metzner, L. Kang, M. v. d. Rijn, E. C. Skinner, S. S. Gambhir, I. L. Weissman and J. C. Liao, Sci. Transl. Med., 2014, 6, 260ra148 Search PubMed.
- L. Silberstein, Blood, 2002, 99, 3491 CrossRef CAS.
- P.-A. Oldenborg, A. Zheleznyak, Y.-F. Fang, C. F. Lagenaur, H. D. Gresham and F. P. Lindberg, Science, 2000, 288, 2051–2054 CrossRef CAS PubMed.
- C. Zhang, W. Wu, R. Q. Li, W. X. Qiu, Z. N. Zhuang, S. X. Cheng and X. Z. Zhang, Adv. Funct. Mater., 2018, 28, 1804492 CrossRef.
- S. Lv, M. Sylvestre, A. N. Prossnitz, L. F. Yang and S. H. Pun, Chem. Rev., 2021, 121, 11653–11698 CrossRef CAS PubMed.
- H. Wang, H. Yao, C. Li, H. Shi, J. Lan, Z. Li, Y. Zhang, L. Liang, J.-Y. Fang and J. Xu, Nat. Chem. Biol., 2019, 15, 42–50 CrossRef CAS PubMed.
- W.-Y. Xiao, Y. Wang, H.-W. An, D. Hou, M. Mamuti, M.-D. Wang, J. Wang, W. Xu, L. Hu and H. Wang, ACS Appl. Mater. Interfaces, 2020, 12, 40042–40051 CrossRef CAS PubMed.
- J. Kline, J. Godfrey and S. M. Ansell, Blood, 2020, 135, 523–533 CrossRef CAS PubMed.
- M. A. Postow, R. Sidlow and M. D. Hellmann, N. Engl. J. Med., 2018, 378, 158–168 CrossRef CAS PubMed.
- H. Ruan, L. Bu, Q. Hu, H. Cheng, W. Lu and Z. Gu, Adv. Healthcare Mater., 2019, 8, e1801099 Search PubMed.
- S. A. Patel and A. J. Minn, Immunity, 2018, 48, 417–433 CrossRef CAS PubMed.
- J. Li, J. Wang, Y. Zhao, P. Zhou, J. Carter, Z. Li, T. A. Waigh, J. R. Lu and H. Xu, Coord. Chem. Rev., 2020, 421 CAS.
- M. Meier and P. Burkhard, J. Struct. Biol., 2006, 155, 116–129 CrossRef CAS PubMed.
- S. H. Medina, M. S. Michie, S. E. Miller, M. J. Schnermann and J. P. Schneider, Angew. Chem., Int. Ed., 2017, 56, 11404–11408 CrossRef CAS PubMed.
- H. Xu, C. X. Chen, J. Hu, P. Zhou, P. Zeng, C. H. Cao and J. R. Lu, Biomaterials, 2013, 34, 2731–2737 CrossRef CAS PubMed.
- S. Koutsopoulos, L. Kaiser, H. M. Eriksson and S. Zhang, Chem. Soc. Rev., 2012, 41, 1721–1728 RSC.
- R. N. Shah, N. A. Shah, M. M. D. R. Lim, C. Hsieh, G. Nuber and S. I. Stupp, Proc. Natl. Acad. Sci. U. S. A., 2010, 107, 3293–3298 CrossRef CAS PubMed.
- F. Peng, Y. Chen, J. Liu, Z. Xing, J. Fan, W. Zhang and F. Qiu, J. Colloid Interface Sci., 2021, 591, 314–325 CrossRef CAS PubMed.
- J. Zhang, Y. Zhao, S. Han, C. Chen and H. Xu, Sci. China: Chem., 2014, 57, 1634–1645 CrossRef CAS.
- M. J. Sis and M. J. Webber, Trends Pharmacol. Sci., 2019, 40, 747–762 CrossRef CAS PubMed.
- S. Son, J. H. Kim, X. Wang, C. Zhang, S. A. Yoon, J. Shin, A. Sharma, M. H. Lee, L. Cheng, J. Wu and J. S. Kim, Chem. Soc. Rev., 2020, 49, 3244–3261 RSC.
- T. Zhang, Y. Sun, J. Cao, J. Luo, J. Wang, Z. Jiang and P. Huang, J. Nanobiotechnol., 2021, 19, 315 CrossRef CAS PubMed.
- J. Li, Y. Luo and K. Pu, Angew. Chem., Int. Ed., 2021, 60, 12682–12705 CrossRef CAS PubMed.
- Z. Zeng, C. Zhang, S. He, J. Li and K. Pu, Adv. Mater., 2022, 34, e2203246 CrossRef PubMed.
- Y. Zhang, X. Zhang, H. Yang, L. Yu, Y. Xu, A. Sharma, P. Yin, X. Li, J. S. Kim and Y. Sun, Chem. Soc. Rev., 2021, 50, 11227–11248 RSC.
- C. Ji, J. Si, Y. Xu, W. Zhang, Y. Yang, X. He, H. Xu, X. Mou, H. Ren and H. Guo, Theranostics, 2021, 11, 8587–8604 CrossRef CAS PubMed.
- W. Yue, L. Chen, L. Yu, B. Zhou, H. Yin, W. Ren, C. Liu, L. Guo, Y. Zhang, L. Sun, K. Zhang, H. Xu and Y. Chen, Nat. Commun., 2019, 10, 2025 CrossRef PubMed.
- C. Zhang, J. Huang, Z. Zeng, S. He, P. Cheng, J. Li and K. Pu, Nat. Commun., 2022, 13, 3468 CrossRef CAS PubMed.
- L. Sun, P. Wang, J. Zhang, Y. Sun, S. Sun, M. Xu, L. Zhang, S. Wang, X. Liang and L. Cui, Biomater. Sci., 2021, 9, 1945–1960 RSC.
- C. Cao, X. Wang, N. Yang, X. Song and X. Dong, Chem. Sci., 2022, 13, 863–889 RSC.
- Y. Lu, Z. Yue, J. Xie, W. Wang, H. Zhu, E. Zhang and Z. Cao, Nat. Biomed. Eng., 2018, 2, 318–325 CrossRef CAS PubMed.
- J.-W. Yoo, D. J. Irvine, D. E. Discher and S. Mitragotri, Nat. Rev. Drug Discovery, 2011, 10, 521–535 CrossRef CAS PubMed.
- H. Zhang, Z. Gao, X. Li, L. Li, S. Ye and B. Tang, Chem. Sci., 2021, 12, 12429–12436 RSC.
- Z.-J. Chen, S.-C. Yang, X.-L. Liu, Y. Gao, X. Dong, X. Lai, M.-H. Zhu, H.-Y. Feng, X.-D. Zhu, Q. Lu, M. Zhao, H.-Z. Chen, J. F. Lovell and C. Fang, Nano Lett., 2020, 20, 4177–4187 CrossRef CAS PubMed.
- S. Choi, Y. j. Choi, M. S. Jang, J. H. Lee, J. H. Jeong and J. Kim, Adv. Funct. Mater., 2017, 27, 1703826 CrossRef.
- J. Li and D. J. Mooney, Nat. Rev. Mater., 2016, 1, 16071 CrossRef CAS PubMed.
- S. Sindhwani, A. M. Syed, J. Ngai, B. R. Kingston, L. Maiorino, J. Rothschild, P. MacMillan, Y. Zhang, N. U. Rajesh, T. Hoang, J. L. Y. Wu, S. Wilhelm, A. Zilman, S. Gadde, A. Sulaiman, B. Ouyang, Z. Lin, L. Wang, M. Egeblad and W. C. W. Chan, Nat. Mater., 2020, 19, 566–575 CrossRef CAS PubMed.
- S. O. Souza, R. B. Lira, C. R. A. Cunha, B. S. Santos, A. Fontes and G. Pereira, Top. Curr. Chem., 2021, 379, 1 CrossRef CAS PubMed.
- N. Rades, K. Achazi, M. Qiu, C. Deng, R. Haag, Z. Zhong and K. Licha, J. Controlled Release, 2019, 300, 13–21 CrossRef CAS PubMed.
- D. Su, L. Gao, F. Gao, X. Zhang and X. Gao, Chem. Sci., 2020, 11, 5614–5629 RSC.
- X. Dong, R. K. Brahma, C. Fang and S. Q. Yao, Chem. Sci., 2022, 13, 4239–4269 RSC.
- B. Huang, Z. Bai, X. Ye, C. Zhou, X. Xie, Y. Zhong, K. Lin and L. Ma, Comput. Struct. Biotechnol. J., 2021, 19, 5494–5503 CrossRef CAS PubMed.
- M. Guo, L. Zhang, Y. Tian, M. Wang and W. Wang, Anal. Chem., 2021, 93, 8035–8044 CrossRef CAS PubMed.
- D. Hatherley, S. C. Graham, J. Turner, K. Harlos, D. I. Stuart and A. N. Barclay, Mol. Cell, 2008, 31, 266–277 CrossRef CAS PubMed.
- Y. Tian, L. Zhang, F. Liu, M. Wang, L. Li, M. Guo, H. Xu, Z. Yu and W. Wang, Biosens. Bioelectron., 2021, 184, 113235 CrossRef CAS PubMed.
- Y. Wang, F. Jia, Z. Wang, Y. Qian, L. Fan, H. Gong, A. Luo, J. Sun, Z. Hu and W. Wang, Anal. Chem., 2019, 91, 7245–7253 CrossRef CAS PubMed.
- K. Weiskopf, Eur. J. Cancer, 2017, 76, 100–109 CrossRef CAS PubMed.
- C. Yuan, A. Levin, W. Chen, R. Xing, Q. Zou, T. W. Herling, P. K. Challa, T. P. J. Knowles and X. Yan, Angew. Chem., Int. Ed., 2019, 58, 18116–18123 CrossRef CAS PubMed.
- H. Cui, A. G. Cheetham, E. T. Pashuck and S. I. Stupp, J. Am. Chem. Soc., 2014, 136, 12461–12468 CrossRef CAS PubMed.
- H. A. Lashuel, S. R. LaBrenz, L. Woo, L. C. Serpell and J. W. Kelly, J. Am. Chem. Soc., 2000, 122, 5262–5277 CrossRef CAS PubMed.
- M. O. Barros, M. Yamamoto, M. S. Figueiredo, E. Y. Kimura and J. O. Bordin, Blood, 2006, 108, 3738 CrossRef.
- M. Muttenthaler, G. F. King, D. J. Adams and P. F. Alewood, Nat. Rev. Drug Discovery, 2021, 20, 309–325 CrossRef CAS PubMed.
Footnote |
† Electronic supplementary information (ESI) available: Detailed experimental materials and methods can be found in the Supplementary Information. See DOI: https://doi.org/10.1039/d2sc02728c |
|
This journal is © The Royal Society of Chemistry 2022 |
Click here to see how this site uses Cookies. View our privacy policy here.