DOI:
10.1039/D2SC03881A
(Edge Article)
Chem. Sci., 2022,
13, 9701-9705
O-GlcNAcylation mapping of single living cells by in situ quantitative SERS imaging †
Received
12th July 2022
, Accepted 29th July 2022
First published on 1st August 2022
Abstract
O-GlcNAcylation is involved in many biological processes including cancerization. Nevertheless, its in situ quantification in single living cells is still a bottleneck. Here we develop a quantitative SERS imaging strategy for mapping the O-GlcNAcylation distribution of single living cells. O-GlcNAcylated compounds (OGCs) can be quantified through their in situ azide labeling and then a click reaction competing with azide and Raman reporter labeled 15 nm-gold nanoparticles (AuNPs) for linking to dibenzocyclooctyne labeled 40 nm-AuNPs to produce OGC-negatively correlated SERS signals. The calibration curve obtained in vitro can be conveniently used for detecting OGCs in different areas of single living cells due to the negligible effect of cell medium on the click linkage and Raman signal. This method has been successfully applied in mapping O-GlcNAcylation distribution in different cell lines and monitoring O-GlcNAcylation variation during cell cycling, which demonstrate its great practicability and expansibility in glycosylation related analysis.
Introduction
O-GlcNAcylation of proteins is a vital protein post-translational modification, which links single N-acetylglucosamine (GlcNAc) residues to O-linkages (serine or threonine) on proteins.1–4 More than one thousand proteins can be O-GlcNAcylated. Thus O-GlcNAcylated proteins are abundant in the nucleus, cytosol and other cellular compartments, and O-GlcNAcylation plays key roles in the regulation of different cellular processes, including transcription, translation, protein trafficking, and degradation, and has cross talk with phosphorylation of proteins.5,6 An abnormal level of O-GlcNAcylation causes numerous chronic diseases such as diabetes, Alzheimer's disease, and cancer. Especially, O-GlcNAcylation occurs on most of the oncoproteins and hence regulates cancer cell growth, cycle, adhesion and cancer progression.5,6 Thus, the analysis of O-GlcNAcylation in cancer cells is of great importance for revealing O-GlcNAcylation related biological processes and cancer etiology.
Mass spectroscopy is a general technology used to detect O-GlcNAcylation.7 However, the extreme lability of O-GlcNAc in ionization limits its application in O-GlcNAcylation analysis.6,7 A chemoenzymatic method has been developed for gel electrophoresis analysis of O-GlcNAcylated proteins through biotin-streptavidin recognition,8 and some fluorescence imaging strategies have been proposed for the visualization of O-GlcNAcylation in living cells.4,9 For example, Wittmann et al. employed green fluorescent protein and metabolic engineering to respectively label the target protein and O-GlcNAc for the visualization of protein-specific O-GlcNAcylation,4 and our previous work designed succinylated wheat germ agglutinin and GlcNAc functionalized gold nanoparticles (AuNPs) as a dual-color fluorescent probe to simultaneously visualize O-GlcNAcylated proteins and dissociated GlcNAc residues within living cells.9 Obviously, these methods cannot give quantitative results. The in situ quantification of O-GlcNAcylation inside single living cells is still a bottleneck.
Surface-enhanced Raman spectroscopy (SERS) is a highly sensitive analytical technique and can provide stable and inherent signals of report molecules without interference from complex biological systems.10,11 It has been used to detect the glycan12,13 and even protein-specific glycan14 on the living cell surface. To break through the bottleneck in in situ quantification of intracellular O-GlcNAcylation, here we develop a quantitative SERS imaging strategy to display for the first time the regional distribution of O-GlcNAcylation. This strategy can map the O-GlcNAcylation of single living cells through metabolic labeling of O-GlcNAcylated compounds (OGCs) with azide15–18 and then the competitive click reaction19 of dibenzocyclooctyne (DBCO) labeled 40 nm-AuNPs (Au40-PEG-DBCO) with azide labeled OGC and Au15-DTNB/PEG-N3, azide 15 nm-AuNPs modified with Raman reporter 5,5-dithiobis (2-nitrobenzoic acid) (DTNB) (Scheme 1). To avoid the effects of probe endocytosis and cellular vesicles on the click reaction, the cells were treated with a pore-forming protein, streptolysin O (SLO), to form pores of over 100 nm on the cell membrane,20 which leads to direct probe delivery and can maintain the cell activity.21 Using a calibration curve obtained in vitro, the concentrations of OGC in different areas of single living cells can be detected for O-GlcNAcylation mapping. The successful mapping of GlcNAcylation distributions in different target cells demonstrated its practicability and expansibility.
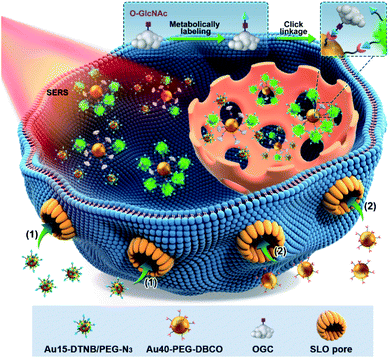 |
| Scheme 1 Schematic illustration of the quantitative SERS imaging strategy for O-GlcNAcylation mapping of signal living cells. After metabolic labeling and perforation by a pore-forming protein (SLO), Au15-DTNB/PEG-N3 and Au40-PEG-DBCO enter the cells in sequence and then a competitive click reaction with azide labeled O-GlcNAcylated compounds (OGCs) occurs, which form different aggregates due to different distributions of OGCs in single living cells. | |
Results and discussion
Copper-free click linkage between Au15-DTNB/PEG-N3 and Au40-PEG-DBCO
15 nm-AuNPs and 40 nm-AuNPs were synthesized22 for the preparation of Au15-DTNB/PEG-N3 and Au40-PEG-DBCO and characterized with transmission electron microscopy (TEM) images and UV-Vis spectra. Au15-DTNB/PEG-N3 and Au40-PEG-DBCO retained the original morphology of AuNPs (Fig. S1, S2a and b†), but exhibited a slight red shift of the absorption peak compared to Au15 and Au40 (Fig. 1a), respectively, indicating a slight size increase upon the modification. The individual Au15-DTNB/PEG-N3 did not show the Raman characteristic peaks of DTNB (Fig. 1b) due to the negligible SERS effect of single Au15.14,23 The MALDI-TOF mass spectra of both Au15-DTNB/PEG-N3 and Au40-PEG-DBCO (Fig. 1c and d) exhibited equally spaced m/z values of 44 corresponding to the ethylene glycol units in PEG, which indicated the successful PEG modification of Au15 and Au40.
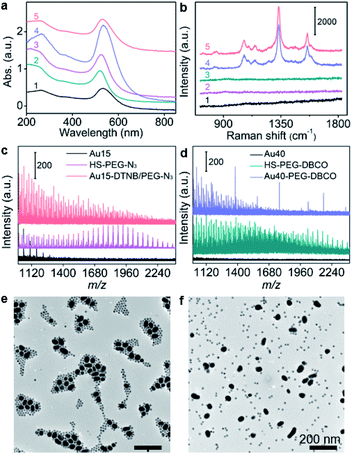 |
| Fig. 1 (a) UV-vis absorption spectra of Au40 (1), Au15 (2), Au15-DTNB/PEG-N3 (3), and Au40-PEG-DBCO (4) and click aggregates of Au15-DTNB/PEG-N3 and Au40-PEG-DBCO (5). (b) Raman spectra of Au15-DTNB/PEG-N3 (1), Au40-PEG-DBCO (2), and the mixture of Au15-DTNB/PEG-N3 and Au40-PEG-N3 as the control (3), and click aggregates of Au15-DTNB/PEG-N3 and Au40-PEG-DBCO in 1640 medium (4) or water (5). (c and d) MALDI-TOF mass spectra of Au15-DTNB/PEG-N3 (c) and Au40-PEG-DBCO (d). (e and f) TEM images of click aggregates of Au15-DTNB/PEG-N3 and Au40-PEG-DBCO (e) and the mixture of Au15-DTNB/PEG-N3 and Au40-PEG-N3 (f) in 1640 medium. Scale bars: 200 nm. | |
The copper-free click reaction between Au15-DTNB/PEG-N3 and Au40-PEG-DBCO was firstly validated with TEM images, which showed obvious aggregates (Fig. S2c†) and indicated the successful click linkage of Au15 and Au40 in water. The linkage led to a wider absorption peak than individual Au15-DTNB/PEG-N3 or Au40-PEG-DBCO (Fig. 1a) and the characteristic peaks of DTNB at 1332 cm−1 and 1580 cm−1 (Fig. 1b), proving the SERS effect of Au40 to DTNB. Moreover, the click aggregates obtained in 1640 medium showed the same Raman spectrum as those obtained in water (Fig. 1b, curves 4 and 5), indicating the negligible effect of cell medium on the click linkage and Raman signal. Besides, the click linkage also occurred in RPMI-1640 medium (Fig. 1b and e). In contrast, the mixture of Au15-DTNB/PEG-N3 and Au40-PEG-N3 did not show the characteristic.
The Raman peaks of DTNB (Fig. 1b) or obvious aggregates (Fig. 1f) demonstrate the good stability of these probes and exclude their aggregation in the absence of click linkage.
Calibration curve for OGC quantification
The quantification ability of the designed competitive strategy was verified in vitro using human p53 (ref. 24–27) as the model glycoprotein, which is O-GlcNAcylated at serine 149.24,28 The p53 and azide-labeled p53 (p53-N3) were immunocaptured from MCF-7 cells and GlcNAz treated MCF-7 cells, respectively, which exhibited a slight m/z difference in mass spectra (Fig. S3a†) and tiny band movement in SDS-PAGE analysis (Fig. S3b†), indicating the single N3 group on p53-N3. The concentration of the obtained p53-N3 could be detected to be 10.9 μM through its reaction with excessive DBCO-Cy5 and then recording the fluorescent signal of Cy5 after removing the extra DBCO-Cy5 by ultrafiltration (Fig. S4†). After the mixtures of different concentrations of p53-N3 and 1 nM Au15-DTNB/PEG-N3 were incubated with 1 nM Au40-PEG-DBCO in RPMI-1640 medium for 1 h, they were subjected to SERS imaging under the same conditions as cell imaging. The SERS signal responding to the aggregates of Au15-DTNB/PEG-N3 and Au40-PEG-DBCO increased with decreasing p53-N3 concentration (Fig. 2a), demonstrating the presence of competitive binding of Au15-DTNB/PEG-N3 and p53-N3 with Au40-PEG-DBCO.
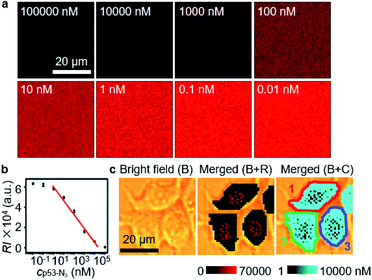 |
| Fig. 2 (a) SERS imaging of click aggregates of Au15-DTNB/PEG-N3 and Au40-PEG-DBCO in 1640 medium containing different concentrations of p53-N3. (b) Calibration curve for OGC quantification. (c) SERS imaging (R) and O-GlcNAcylation mapping (C) of MCF-7 cells. | |
The competitive binding aggregates at different p53-N3 concentrations (1 and 10
000 nM) could be obviously distinguished in TEM images (Fig. S5†). Besides, the SERS signal of the aggregates in 1 nM (Fig. S6a and c†) and 10
000 nM (Fig. S6b and d†) p53-N3 solution remained unchanged from 0.5 h to 8 h, which indicated the stability of the aggregates. The average signal intensity (RI) was inversely proportional to the logarithm value of p53-N3 concentration (lgcp53-N3) ranging from 1 to 10
000 nM (Fig. 2b) with an equation of RI = −13946
lgcp53-N3 + 61
308 (R2 = 0.98616). Thus, a quantitative method could be provided for p53-N3 analysis and detecting the concentration of p53 or OGCs in single living cells after metabolic labeling with GlcNAz due to the negligible effect of cell medium on the click linkage and Raman signal (Fig. 1b, curves 4 and 5).
In situ O-GlcNAcylation mapping of living cells
To achieve the quantification of metabolically labeled OGCs in living cells, the GlcNAz-treated cells were firstly perforated with SLO for avoiding the effects of probe endocytosis and cellular vesicles on the click reaction. Propidium iodide (PI) staining confirmed the formation of pores on SLO-treated cells (Fig. S7a and b†).21 In addition, the pores could remain on the cell membrane for 12 h, which was sufficient for the entry of probes (Fig. S7c†). The conditions for competitive click linkage in metabolically labeled and perforated living cells were optimized in the presence of iodoacetamide (IAM), which could block the undesired thiol to inhibit its reaction with DBCO and improve the cycloaddition efficiency between –N3 and DBCO.29 The optimal concentrations and incubation times of Au15-DTNB/PEG-N3 and Au40-PEG-DBCO were 1 nM for 2 h and 0.8 nM for 4 h (Figs. S8–S11†), respectively. After all these treatments, the cells could retain approximate 90% viability (Fig. S12†), suggesting their acceptable compatibility for living cell analysis.
Under the optimized incubation conditions, obvious SERS signals were distributed in different regions of MCF-7 cells (Fig. 2c, B+R), which could be converted to the concentration of OGCs with the average RI and the calibration curve, and thus led to a concentration mapping of OGCs (Fig. 2c, B+C). By merging the bright field image, the average concentration of OGCs in living single cells could be quantified (Fig. 2c). Thus, the in situ O-GlcNAcylation mapping of living MCF-7 cells was for the first time achieved.
The developed strategy for O-GlcNAcylation mapping was firstly applied to quantitatively evaluate the distribution of O-GlcNAcylation in MCF-10A, HeLa and A549 cells (Fig. 3a). Similarly, O-GlcNAcylation was unevenly distributed in these cells. The average concentrations of OGCs in single cells were calculated to be 11.0 μM for A549 cells, 10.1 μM for MCF-7 cells, 9.5 μM for HeLa cell, and 0.21 μM for MCF-10A (Fig. 3b), which revealed significantly different O-GlcNAcylation levels between tumor and healthy cells. Moreover, confocal fluorescence imaging and flow cytometric analysis qualitatively validated the results. After metabolically labeled and perforated cells were stained with DBCO-Cy5, the cells were subjected to CLSM imaging (Fig. S13†) and flow cytometric assay (Fig. S14†), which showed the same difference of O-GlcNAcylation levels among these cells. However, these fluorescence methods provided only the qualitative results and rely on the complete cleanup of the unconjugated dyes inside cells, which brings about false positive results. Therefore, the designed competitive SERS strategy exhibits great reliability and convenience in in situ quantitative analysis of intracellular O-GlcNAcylated compounds.
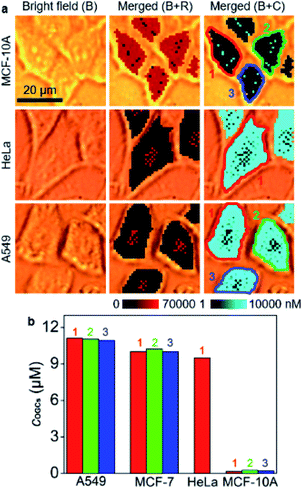 |
| Fig. 3 (a) SERS imaging and O-GlcNAcylation mapping of MCF-10A, HeLa and A549 cells. (b) Statistical O-GlcNAcylation mapping intensities of every single cell in (a) and Fig. 2c. | |
The proposed strategy was then used for monitoring of O-GlcNAcylation variation upon alloxan treatment, which can down-regulate the O-GlcNAcylation level.9,30 With increasing alloxan concentration, a stronger SERS signal was observed, indicating a down-regulated O-GlcNAcylation level (Fig. S15a†). From the concentration maps, the average concentrations of OGCs in every single cell with different inhibitions could be obtained (Fig. S15b†). The inhibition effect quantitatively agreed with the fluorescence imaging analysis (Fig. S16†).
O-GlcNAcylation plays a crucial role in regulating DNA replication, cell-cycle progression and mitosis, and is related to cancerization.31–33 It also protects genome integrity by modifying histones, kinases and scaffold proteins in the face of an altered cell cycle.34 Therefore, the quantification of O-GlcNAcylation during cell cycling is of great importance. To use the developed strategy for the quantification of the O-GlcNAcylation level in different cell phases, MCF-7 cells were synchronized at G1/S using the thymidine double-block method35,36 and then incubated in fresh medium for 6 h, 13 h, 23 h, and 24 h to reach S-, G2-, M- and G1-phases, respectively (Fig. S17†). Afterward, the cells were metabolically labeled with GlcNAz for 34 h, which was shorter than a complete cycle of MCF-7 cells35,36 for guaranteeing them in the original cell phases (Fig. S18†). Thus, the O-GlcNAcylation levels as well as their distributions in different cell phases could be detected with the proposed quantitative SERS imaging method (Fig. 4a). The average concentrations of OGCs in single cells were calculated to be 11.6 μM in the G1 phase, 10.3 μM in the S phase, 11.7 μM in the G2 phase, and 10.0 μM in the M phase of MCF-7 cells (Fig. 4b). The difference of O-GlcNAcylation levels in different cell phases could be attributed to the corresponding activity of O-GlcNAc transferase37,38 and O-GlcNAcase.39 The O-GlcNAcylation variation during the cell cycle was coincident with the intensity change of fluorescence images (Fig. S19†), demonstrating the reliability.
 |
| Fig. 4 (a) SERS imaging and O-GlcNAcylation mapping in MCF-7 cells in G1, S, G2, and M phases. (b) Statistical O-GlcNAcylation mapping intensities of every single cell in (a). | |
Conclusions
In conclusion, we develop a quantitative SERS imaging strategy to display for the first time the regional distribution of O-GlcNAcylation inside single living cells through metabolic labeling of OGCs with azide for linking to Au40-PEG-DBCO competitively with azide labeled Au15-DTNB/PEG-N3, which lead to a signal switch negatively correlated OGC concentration. Combining with the in vitro obtained calibration curve with a linear range from 1 to 10
000 nM, the concentrations of OGCs in different areas of a single cell can be directly quantified. The developed strategy has successfully been used for the detection of O-GlcNAcylation levels in MCF-7, MCF-10A, A549, and HeLa cells, and the monitoring of O-GlcNAcylation variation responding to its inhibitor and cell cycle. The designed cell imaging procedure, including metabolic labelling, SLO perforation, probe delivery and intracellular competitive click linkage, can maintain the viability of target cells. This strategy can be conveniently expanded to quantitatively map other –N3 labelled biomolecules in living cells, and provides a practical tool for the exploration of O-GlcNAcylation related biological mechanisms.
Data availability
All relevant data is presented in the paper and ESI.† Raw data is available upon request by email to the corresponding author.
Author contributions
Y. Y., Y. C., and H.J. designed the projects, planned the experiments and wrote the manuscript; Y. Y. performed Raman and cell related experiments. Y. C. carried out fluorescence experiments. S. Z. prepared and characterized the AuNP probe. H. L. and J. G. cultured cells and analyzed data. H. J. supervised and coordinated all investigators for this project.
Conflicts of interest
There are no conflicts to declare.
Acknowledgements
This work was supported by the National Natural Science Foundation of China (21827812, 21890741, and 21974063), National Key Research and Development Program of China (2018YFC1004704), and State Key Laboratory of Analytical Chemistry for Life Science (SKLACLS2104).
Notes and references
- C.-R. Torres and G. W. Hart, J. Biol. Chem., 1984, 259, 3308–3317 CrossRef CAS PubMed.
- G. D. Holt and G. W. Hart, J. Biol. Chem., 1986, 261, 8049–8057 CrossRef CAS PubMed.
- L. Wells, K. Vosseller and G. W. Hart, Science, 2001, 291, 2376–2378 CrossRef CAS PubMed.
- F. Doll, A. Buntz, A.-K. Spate, V. F. Schart, A. Timper, W. Schrimpf, C. R. Hauck, A. Zumbusch and V. Wittmann, Angew. Chem., Int. Ed., 2016, 55, 2262–2266 CrossRef CAS PubMed.
- G. W. Hart, C. Slawson, G. Ramirez-Correa and O. Lagerlof, Annu. Rev. Biochem., 2011, 80, 825–858 CrossRef CAS PubMed.
- G. W. Hart, M. P. Housley and C. Slawson, Nature, 2007, 446, 1017–1022 CrossRef CAS PubMed.
- K. D. Greis and G. W. Hart, Methods Mol. Biol., 1998, 76, 19–33 CAS.
- N. Khidekel, S. Arndt, N. Lamarre-Vincent, A. Lippert, K. G. Poulin-Kerstien, B. Ramakrishnan, P. K. Qasba and L. C. Hsieh-Wilson, J. Am. Chem. Soc., 2003, 125, 16162–16163 CrossRef CAS PubMed.
- Y. L. Chen, H. P. Liu, L. Ding and H. X. Ju, Anal. Chem., 2018, 90, 3073–3078 CrossRef CAS PubMed.
- H. Abramczyk and B. Brozek-Pluska, Chem. Rev., 2013, 113, 5766–5781 CrossRef CAS PubMed.
- C. Zong, M. X. Xu, L. J. Xu, T. Wei, X. Ma, X. S. Zheng, R. Hu and B. Ren, Chem. Rev., 2018, 118, 4946–4980 CrossRef CAS PubMed.
- L. Lin, X. D. Tian, S. L. Hong, P. Dai, Q. C. You, R. Y. Wang, L. S. Feng, C. Xie, Z.-Q. Tian and X. Chen, Angew. Chem., Int. Ed., 2013, 52, 7266–7271 CrossRef CAS PubMed.
- Y. L. Chen, L. Ding, J. Q. Xu, W. Y. Song, M. Yang, J. J. Hu and H. X. Ju, Chem. Sci., 2015, 6, 3769–3774 RSC.
- Y. L. Chen, L. Ding, W. Y. Song, M. Yang and H. X. Ju, Chem. Sci., 2016, 7, 569–574 RSC.
- S. T. Laughlin and C. R. Bertozzi, Proc. Natl. Acad. Sci. U. S. A., 2009, 106, 12–17 CrossRef CAS PubMed.
- R. Xie, S. L. Hong and X. Chen, Curr. Opin. Chem. Biol., 2013, 17, 747–752 CrossRef CAS PubMed.
- R. Xie, S. L. Hong, L. S. Feng, J. Rong and X. Chen, J. Am. Chem. Soc., 2012, 134, 9914–9917 CrossRef CAS PubMed.
- D. J. Vocadlo, H. C. Hang, E.-J. Kim, J. A. Hanover and C. R. Bertozzi, Proc. Natl. Acad. Sci. U. S. A., 2003, 100, 9116–9121 CrossRef CAS PubMed.
- E. M. Sletten and C. R. Bertozzi, Angew. Chem., Int. Ed., 2009, 48, 6974–6998 CrossRef CAS PubMed.
- Y. Y. Liu, T. Z. Zhang, Y. B. Zhou, J. P. Li, X. Y. Liang, N. N. Zhou, J. D. Lv, J. Xie, F. R. Cheng, Y. L. Fang, Y. F. Gao, N. Wang and B. Huang, Cell. Mol. Immunol., 2018, 16, 611–620 CrossRef PubMed.
- Y. J. Yang, Y. L. Chen, J. X. Guo, H. P. Liu and H. X. Ju, iScience, 2021, 24, 102980 CrossRef CAS PubMed.
- G. Frens, Nature Physical Science, 1973, 241, 20–22 CrossRef CAS.
- M. Moskovits, J. Raman Spectrosc., 2005, 36, 485–496 CrossRef CAS.
- W. H. Yang, J. E. Kim, H. W. Nam, J. W. Ju, H. S. Kim, Y. S. Kim and J. W. Cho, Nat. Cell Biol., 2006, 8, 1074–1083 CrossRef CAS PubMed.
- K. H. Vousden, Cell, 2000, 103, 691–694 CrossRef CAS PubMed.
- A. M. Bode and Z. G. Dong, Nat. Rev. Cancer, 2004, 4, 793–805 CrossRef CAS PubMed.
- K. T. Bieging, S. S. Mello and L. D. Attardi, Nat. Rev. Cancer, 2014, 14, 359–370 CrossRef CAS PubMed.
- Z. Gurel, B. W. Zaro, M. R. Pratt and N. Sheibani, PLoS One, 2014, 9, e95561 CrossRef PubMed.
- R. van Geel, G. J. M. Pruijn, F. L. van Delft and W. C. Boelens, Bioconjugate Chem., 2012, 23, 392–398 CrossRef CAS PubMed.
- S. Lenzen and U. Panten, Diabetologia, 1988, 31, 337–342 CrossRef CAS PubMed.
- E. P. Tan, F. E. Duncan and C. Slawson, Biochem. Soc. Trans., 2017, 45, 313–322 CrossRef CAS PubMed.
- Y. P. Tian, Q. Zhu, Z. Y. Sun, D. D. Geng, B. Y. Lin, X. L. Su, J. H. He, M. Guo, H. Xu, Y. Zhao, W. J. Qin, P. G. Wang, L. Q. Wen and W. Yi, Angew. Chem., Int. Ed., 2021, 60, 26128–26135 CrossRef CAS PubMed.
- J. L. Liu, Y. Hao, Y. W. He, X. Li, D. E. Sun, Y. Zhang, P. Y. Yang and X. Chen, ACS Chem. Biol., 2021, 16, 1917–1923 CrossRef CAS PubMed.
- C. F. Liu and J. Li, Front. Endocrinol., 2018, 9, 415 CrossRef PubMed.
- X. F. Dong, Y. Berthois, E. Colomb and P. M. Martin, Endocrinology, 1991, 129, 2719–2728 CrossRef CAS PubMed.
- H. Ouadid-Ahidouch, M. Roudbaraki, A. Ahidouch, P. Delcourt and N. Prevarskaya, Biochem. Biophys. Res. Commun., 2004, 316, 244–251 CrossRef CAS PubMed.
- R. S. Haltiwanger, G. D. Holt and G. W. Hart, J. Biol. Chem., 1990, 265, 2563–2568 CrossRef CAS PubMed.
- W. A. Lubas, D. W. Frank, M. Krause and J. A. Hanover, J. Biol. Chem., 1997, 272, 9316–9324 CrossRef CAS PubMed.
- Y. Gao, L. Wells, F. I. Comer, G. J. Parker and G. W. Hart, J. Biol. Chem., 2001, 276, 9838–9845 CrossRef CAS PubMed.
|
This journal is © The Royal Society of Chemistry 2022 |
Click here to see how this site uses Cookies. View our privacy policy here.