DOI:
10.1039/D3AN00380A
(Paper)
Analyst, 2023,
148, 3204-3216
Steady-state monitoring of oxygen in a high-throughput organ-on-chip platform enables rapid and non-invasive assessment of drug-induced nephrotoxicity†
Received
10th March 2023
, Accepted 5th June 2023
First published on 12th June 2023
Abstract
High-throughput, rapid and non-invasive readouts of tissue health in microfluidic kidney co-culture models would expand their capabilities for pre-clinical assessment of drug-induced nephrotoxicity. Here, we demonstrate a technique for monitoring steady state oxygen levels in PREDICT96-O2, a high-throughput organ-on-chip platform with integrated optical-based oxygen sensors, for evaluation of drug-induced nephrotoxicity in a human microfluidic co-culture model of the kidney proximal tubule (PT). Oxygen consumption measurements in PREDICT96-O2 detected dose and time-dependent injury responses of human PT cells to cisplatin, a drug with known toxic effects in the PT. The injury concentration threshold of cisplatin decreased exponentially from 19.8 μM after 1 day to 2.3 μM following a clinically relevant exposure duration of 5 days. Additionally, oxygen consumption measurements resulted in a more robust and expected dose-dependent injury response over multiple days of cisplatin exposure compared to colorimetric-based cytotoxicity readouts. The results of this study demonstrate the utility of steady state oxygen measurements as a rapid, non-invasive, and kinetic readout of drug-induced injury in high-throughput microfluidic kidney co-culture models.
Introduction
Pre-clinical assessment of drug-induced nephrotoxicity, injury to the kidney due to drug exposure, remains a challenge due to the failure of animal models and simple 2D cell culture to recapitulate the function of the human kidney.1 Standard pre-clinical models for drug-induced nephrotoxicity include animals, which are expensive, raise ethical concerns, and often fail to consistently predict human relevant responses to drug treatments. Standard in vitro cell-based assays that culture cells in static well plates are not predictive due to the lack of physiologically relevant cues, such as flow, present in the human kidney. Additionally, standard in vitro toxicological readouts rely on fluorescent imaging or colorimetric-based assays that are often limited to endpoint measurements and are invasive due to the addition of labels and/or extraction of samples for analysis in a separate instrument, such as a microplate reader.2 Furthermore, because drug-induced nephrotoxicity is thought to be dependent on exposure time in the kidney,3,4 there is a need for data collection techniques that enable non-invasive monitoring of renal tissue health in in vitro systems throughout the time-course of drug exposure. It is also important that new data collection techniques fit existing life science infrastructure to enable broad adoption in the drug development industry. Microfluidic organ-on-chip technology enables improved toxicological assessments due to both the capability to support human cell culture in a semi-complex microenvironment and accommodate sensors for rapid, label-free, and on-chip readouts of dynamic tissue responses during drug exposures.2 Additionally, recently developed high-throughput organ-on-chip platforms with industry standard formats and compatibility with existing life science workflows has increased the potential for translation of organ-on-chip technology in the pharmaceutical industry.5,6
Microfluidic organ-on-chip technology is advantageous for nephrotoxicity assessment due to its ability to support evaluation of the epithelial-microvascular endothelial structure of the proximal tubule (PT),7–9 a primary site for transport and accumulation of nephrotoxic agents in the kidney,10 in a precisely designed microenvironment. Studies have shown that PT cells exposed to flow have improved structure,11–13 function,7,14–16 and responses to nephrotoxic drug exposures9,17 compared to cells cultured in static conditions. Also, both shear stress and increased oxygen supply via flow have shown to increase oxygen consumption rates and oxygen-dependent metabolism,14,18 a key function in the human PT due to its high metabolic workload.19 The co-culture of human primary proximal tubule epithelial cells (hRPTECs) and human primary microvascular endothelial cells (hMVECs) results in cross-talk between the two cell types that alters PT epithelial cell metabolic activity and regulation of drug transport genes.20,21 Also, our group recently found that transepithelial electrical resistance, a functional readout of PT tissue, detected cisplatin-induced toxicity with greater sensitivity for hRPTECs in co-culture compared to in monoculture.22 Thus, inclusion of flow and multiple cell types in PT-on-chips is important to recapitulate physiologically relevant PT injury responses during nephrotoxic drug exposures. Additionally, high-throughput systems that support an array of independent organ-on-chips are needed to assess multiple variables, such as drug concentrations, in parallel with a minimum of 3 replicates for performing statistical analyses. Recently, we have published on a high-throughput platform that has been developed for culturing up to 96 epithelial-endothelial barrier models on a single microtiter plate format.5,22–24
Sensor integration in high-throughput organ-on-chip systems enables non-invasive and near real-time monitoring of tissue health during drug exposures.2 For example, electrical-based transepithelial-endothelial electrical resistance allows rapid and non-invasive measurement of tissue barrier function for toxicological assessment.22,25 Oxygen sensing is particularly advantageous for toxicological screening because it enables measurement of oxygen consumption as a readout of tissue metabolic function and viability.26 Optical-based and electrochemical-based sensors have been utilized in cell culture systems for monitoring oxygen consumption. Electrochemical-based sensing systems27–29 are generally low throughput due to the large footprint of electrical hardware and complex fabrication processes.30 Optical-based oxygen sensing is advantageous for integration in high-throughput microfluidic systems due to its low footprint, straightforward integration, and fast sensor response times.31,32 The Agilent Seahorse XFeAnalyzer, a commercial optical-based oxygen sensing system, measures cell oxygen consumption rates in static 96 well plates, however, does not support perfusion or operate in organ-on-chip systems.33,34 Oxygen sensor-integrated organ-on-chip systems reported to date remain low-throughput with at most 24 devices contained in an array35 and with the majority containing 9 or fewer devices.29,36–40 Previously, we reported a technique to measure oxygen consumption rates in an array of organ-on-chip devices,41 but it required cycling flow on and off for 1–2 minutes in each device sequentially, resulting in un-stable flow conditions and a long time-duration of 1.6–3.2 h for measurement in all 96 devices contained in a standard microtiter plate format. Oxygen consumption readouts based on steady state oxygen levels may address the above limitations by enabling continuous perfusion and a reduction in the time required to sequentially collect data across a large array of devices using a single fiber optic. A recent study demonstrated oxygen consumption measurements in an array of devices containing hepatocytes during continuous perfusion,35 however, the culture chambers were limited to single microchannels, which do not accommodate co-culture models of human tissue barriers, such as the epithelial-endothelial barrier in the PT. Therefore, there is a need for investigation of new high-throughput oxygen sensing techniques that enable non-invasive monitoring of metabolic activity and viability during continuous flow in systems that support tissue barrier models, whether comprised of single or multiple cell types.
In this study, we investigated a technique for the measurement of steady state oxygen levels in PREDICT96-O2 (P96-O2), a high-throughput microfluidic organ-on-chip platform with integrated optical-based oxygen sensors, for monitoring oxygen consumption and assessment of drug-induced injury in a microfluidic human primary co-culture model of the kidney PT (mcPT). Oxygen consumption measurements enabled detection of a dose and time-dependent injury response to cisplatin, a nephrotoxic compound, during a clinically relevant 5-day exposure duration. Measurement of a dose and time-dependent injury response to cisplatin allowed rapid prediction of an injury concentration threshold as a function of exposure time in the mcPT. Finally, we compared the performance of oxygen consumption and gold standard colorimetric-based cytotoxicity readouts for monitoring drug-induced injury in the mcPT. Our results demonstrated a new utility of oxygen sensing and associated methodology in a high-throughput organ-on-chip platform for pre-clinical evaluation of drug-induced nephrotoxicity and investigation of renoprotective strategies.
Results and discussion
Design and workflow for oxygen-sensing in P96-O2 and assessment of drug-induced PT injury
We developed a workflow to assess oxygen consumption and drug-induced injury to hRPTECs in a mcPT during continuous perfusion. P96-O2 contained an array of 96 organ-on-chip devices with integrated optical-based oxygen sensors in a microtiter plate format similar to an industry standard well plate commonly used in drug development.5,41Fig. 1a shows an image of the bottom of a P96-O2 culture plate. Each device of the P96-O2, shown schematically in Fig. 1b, was constructed in a membrane bilayer format, which consisted of top and bottom microchannels separated by a porous membrane. The plate was constructed primarily from cyclo-olefin polymer, a thermoplastic with low oxygen permeability. Each device contained a single oxygen sensor bonded to the floor of the bottom microchannel in the center of the overlap region between the top and bottom microchannels, as shown in Fig. 1b and c. The mcPT consisted of hRPTECs and human primary microvascular endothelial cells (hMVECs) on the bottom and top of the membrane, respectively, as shown schematically in Fig. 1c. For mimicking the flow conditions in the PT,42 unidirectional and recirculating perfusion in the top and bottom microchannels provided convection-based delivery of a nephrotoxic compound (illustrated as purple dots in Fig. 1c) to hRPTECs cultured on the membrane. hMVECs cultured on the top side of the membrane, shown in Fig. 1d and hRPTECs on the bottom of the membrane, shown in Fig. 1e after 11 days of co-culture in P96-O2 demonstrated monolayer formation of both cell types. hRPTECs had high barrier integrity, visualized via expression of tight junction protein ZO-1, and displayed a cobblestone-like morphology, as shown in Fig. 1e. An optical-based sensor in each device measured steady state oxygen levels during continuous perfusion and delivery of a nephrotoxic compound in the bottom microchannel. The schematics in Fig. 1f illustrate the working principle of our sensing technique which relies on a change in steady state oxygen levels that occurs in the bottom microchannel as hRPTECs transition from healthy (Fig. 1fi) to injured (Fig. 1fii) during a nephrotoxic drug exposure. Fig. 1g illustrates an expected qualitative increase in oxygen levels as the hRPTECs undergo injury and consume less oxygen over time following a nephrotoxic exposure. P96-O2 and our experimental workflow, shown in Fig. 1h, allowed assessment of drug-induced injury during a 5-day exposure to cisplatin, a nephrotoxic drug, via oxygen sensing, a conventional colorimetric cytotoxicity assay, and endpoint fluorescent imaging. The P96-O2 platform was well-suited for studying drug-induced nephrotoxicity in the human PT barrier due to its high-throughput capability to co-culture PT epithelial and microvascular endothelial cells in a fluidic environment and simultaneously monitor oxygen in the microchannel containing hRPTECs. P96-O2 provided an improvement in throughput over existing systems that contain at most 24 devices.29,35,36,43 Additionally, the membrane bilayer format of each device in P96-O2 provided benefit over single microchannels utilized in existing high-throughput systems35 for modelling multi-cell type tissue barriers with perfusion on both sides, such as the PT barrier.
 |
| Fig. 1 Experimental design and workflow for oxygen sensing and assessment of drug-induced nephrotoxicity in a human microfluidic co-culture PT model. (a) Bottom view of a P96-O2 plate with 96 organ-on-chip devices. (b) Schematic of a single device with a top (blue) and bottom microchannel (green) and an oxygen sensor located centrally on the floor of the bottom microchannel. (c) A schematic that highlights the key components for assessment of drug-induced nephrotoxicity in P96-O2. hMVECs and hRPTECs cultured on the top and bottom surfaces of the porous membrane, respectively, were exposed to a nephrotoxic compound (purple dots) via controlled and unidirectional flow in the top and bottom microchannels. An optical-based oxygen sensor located in the bottom microchannel measured oxygen via a fiber optic and commercial oxygen meter. Confocal images of (d) hMVECs and (e) hRPTECs stained for nuclei (blue), actin (red), and ZO-1 (green) formed confluent monolayers on the membrane. (f) Schematic illustrating an increase in steady state oxygen levels that occurs in the bottom microchannel following injury to the hRPTECs. (g) Illustration depicting an increase in steady state oxygen levels over time as hRPTECs undergo injury during exposure to a nephrotoxic compound. (h) Experimental timeline for co-culture and assessment of drug-induced nephrotoxicity in P96-O2. | |
Detection of oxygen consumption in the mcPT during continuous perfusion
We developed an approach to monitor steady state oxygen levels in the bottom microchannel of all 96 devices in P96-O2 for rapid, label-free, and on-chip detection of oxygen consumption of hRPTECs. A fiber optic positioned beneath each sensor spot sequentially, shown in Fig. 2a, measured oxygen via a commercial oxygen meter (Pyroscience, Germany) during continuous perfusion and recirculation of media in the top and bottom microchannels. Oxygen was measured, shown schematically in Fig. 2b, via automated and sequential positioning of the fiber optic beneath each sensor using a standard programmable microscope stage. 10–12 data samples were acquired in each device, shown in Fig. S1a,† resulting in a total duration of 32 minutes for automated measurement of oxygen in all 96 devices in P96-O2. The 10–12 oxygen samples acquired in each device had negligible variability with an average standard deviation of 0.25 ± 0.1 hPa (n = 96 devices), shown in Fig. S1b,† which indicated that oxygen measurements were steady with respect to time during each device measurement period. During oxygen measurements, P96-O2 devices contained hRPTECs + hMVECs, hRPTECs only, or no tissue conditions, as shown in the plate format in Fig. S2.† A representative example of the average steady state oxygen levels measured in each device of a single P96-O2 plate containing devices with tissue and without tissue was shown in Fig. 2c. Oxygen levels in devices containing tissue were slightly lower near the center of the plate, compared to devices located at the edge, shown in Fig. S3b,† which was likely due to greater oxygen supply at the periphery of the plate. Despite variability in oxygen levels, the relative change in oxygen over 24 hours in each device did not depend significantly (p = 0.28) on each device's position within the plate, as shown in Fig. S3d.† Further work is still needed to investigate potential variability due to device position within a plate for durations longer than 24 hours, in which proliferation or changes in cellular respiration can occur. Oxygen was significantly lower (p < 0.001) in devices containing hRPTECs + hMVECs and hRPTECs only compared to control devices containing water and culture medium only, shown in Fig. 2d. There was no significant difference (p > 0.05) between oxygen levels in devices containing hRPTECs and hMVECs compared to those with hRPTECs only, which indicated that oxygen measurements in the bottom microchannel were sensitive to hRPTEC oxygen consumption with negligible contribution from hMVECs in the top microchannel. Fig. 2e shows oxygen measurements in devices containing hRPTECs and hMVECs compared to control devices containing water and culture medium over the course of 8 days. Oxygen levels in control devices containing water and culture medium remained steady near 200 hPa, the partial pressure of oxygen in water equilibrated in air. Oxygen levels in devices containing hRPTECs and hMVECs was significantly lower than oxygen in the water and culture medium controls, as shown in Fig. 2e. Oxygen consumption was estimated as the difference between steady state oxygen levels in devices containing tissue and devices containing only culture medium. Oxygen consumption increased over time during 7 days of culture in P96-O2, as shown in Fig. 2f. Oxygen consumption was significantly greater in devices containing hRPTECs and hMVECs compared to devices containing only culture medium over 8 days of culture. The increase in oxygen consumption over 8 days, shown in Fig. 2f, is likely attributed to continued proliferation of hRPTECs, which was supported by hRPTEC expression of Ki67, a protein associated with proliferation, following 10 days of culture in the mcPT, shown in Fig. S4.†
 |
| Fig. 2 Steady state oxygen measurements in the bottom microchannel enabled detection of hRPTEC consumption in each mcPT. (a) Schematic of a single device with online oxygen measurements in the bottom microchannel. (b) Illustration of the path of the fiber optic during sequential oxygen measurements in an entire P96-O2 plate. (c) Representative heatmap displaying steady state oxygen readouts across an entire P96-O2 plate with devices containing tissue and no tissue controls (d) Oxygen measurements in tissue devices containing hRPTECs + hMVECs (n = 46) and only hRPTECs (n = 12) compared to control devices containing culture medium (n = 8) and water (n = 8) on day 6 of culture (N.S. p = 0.8, *p < 0.001, Kruskal–Wallis and Dunn's post-hoc test). (e) Oxygen and (f) oxygen consumption measurements on days 4–11 in devices containing hRPTECs + hMVECs (n = 5) compared to devices containing water (n = 16) and culture medium (n = 4) (*p < 0.05, **p < 0.01, ***p < 0.001 compared to culture medium controls, Kruskal–Wallis and Dunn's post-hoc test). | |
The presented technique for monitoring oxygen consumption provided novelty and improvement over existing methods due to the combination of (1) high-throughput acquisition (96 devices per 32 minutes), (2) compatibility with tissue models consisting of multiple cell types in a membrane bi-layer format, (3) capability to detect oxygen consumption during continuous perfusion and (4) minimal hardware requirements and simple setup for measurements. The commercial Agilent Seahorse XFeAnalyzer can measure oxygen consumption in 96 wells in 2 minutes,44 however, does not operate in organ-on-chip systems. Existing techniques for measurement of oxygen consumption in high-throughput organ-on-chip systems have several limitations including the necessity to modulate flow on and off,41 the large footprint and complex integration of sensing hardware,35 and limitation of measurements to a single monolayer of cells.35,36 While steady state oxygen levels provided an indicator of oxygen consumption in the hRPTEC channel, further work is needed to investigate the relationship between steady state oxygen levels and hRPTEC oxygen consumption rates in P96-O2. In future work, finite element analysis-based models could be employed to estimate hRPTEC oxygen consumption rates from steady state oxygen measurements, similar to an approach reported in Moya et al.29 Additionally, this technique for monitoring oxygen consumption is immediately applicable to a broad range of studies that utilize a microfluidic membrane bi-layer format for culturing complex tissue models including the gut intestines,45 lung,24 blood brain barrier,46 and liver.47 Finally, only a single fiber optic was required to achieve measurements in 96 devices, which resulted in a significantly lower footprint and simpler fabrication and set up compared to current systems.35
Oxygen sensor-enabled monitoring of cisplatin-induced nephrotoxicity
Measurement of oxygen consumption in P96-O2 during continuous perfusion enabled non-invasive monitoring of drug-induced nephrotoxicity during a clinically relevant 5-day exposure duration to cisplatin,48 a compound with nephrotoxic effects in the human PT.49 Representative images of hRPTECs stained with nuclei, actin, and ZO-1, shown in Fig. 3a, indicated a dose-dependent injury response following 5 days of exposure to cisplatin. Dose-dependent cytotoxicity was evident due to changes in the hRPTEC nuclei density on day 5, as shown in Fig. 3b. Additionally, there was a dose-dependent reduction in the expression of key structural proteins actin and ZO-1, shown in Fig. 3a and Fig. S5.† hRPTEC nuclei density was significantly lower than the untreated group at 25, 100, and 200 μM (p < 0.05). Oxygen consumption on day 5 of the cisplatin exposure correlated with hRPTEC nuclei density with an R2 value of 0.735 (p < 0.001), as shown in Fig. 3c. This correlation indicated oxygen consumption could be utilized as a label-free, non-invasive, and rapid surrogate indicator of hRPTEC density decrease and injury in P96-O2.
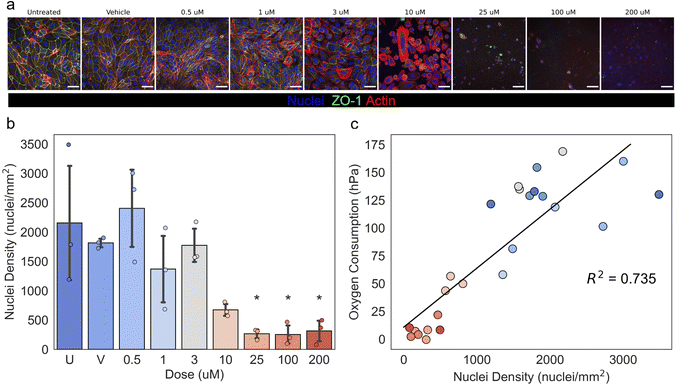 |
| Fig. 3 Oxygen consumption correlated with hRPTEC nuclei density in the mcPT following 5 days of cisplatin exposure. (a) Representative confocal images of hRPTECs on the membrane stained with nuclei, actin, and ZO-1 (scalebar = 50 μm) and (b) density of hRPTEC nuclei on the membrane for each dose of cisplatin following 5 days of treatment (*p < 0.05 relative to untreated group, Kruskal–Wallis and Dunn's post hoc test). (c) Correlation between oxygen consumption measurements and density of hRPTEC nuclei on the membrane (R2 = 0.735, p < 0.001, n = 25). | |
Post/predrug oxygen consumption in the mcPT was defined as oxygen consumption over the course of cisplatin exposure relative to oxygen consumption measured prior to the initial exposure at t = 0 h. Doses of cisplatin were delivered in P96-O2 according to the plate format shown in Fig. S6.† We did not expect significant biases in the post/predrug oxygen consumption readouts due to the plate format design. This was because the post/predrug oxygen consumption readouts were based on relative changes in oxygen over time in each device, which did not depend significantly on each device's position within the plate, as shown in Fig. S3d.† Post/predrug oxygen consumption, shown in Fig. 4a, was measured at 24 h timepoints for 5 days during exposure of hRPTECs to cisplatin and a time- and dose-dependent response was observed. Within 24 h, post/predrug oxygen consumption in devices treated with 100 and 200 μM decreased significantly relative to the untreated devices (p < 0.01). At 48 h, post/predrug oxygen consumption in devices treated with 25 μM decreased significantly relative to the untreated devices (p < 0.001). At 72 h, post/predrug oxygen consumption in devices treated with 10 μM decreased significantly relative to the untreated devices (p < 0.05). There was no significant decrease in post/predrug oxygen consumption in devices treated in the range of 0–3 μM. Post/predrug oxygen consumption in devices treated with 0–3 μM increased above 100% over time, which was most likely due to proliferation of hRPTECs in the bottom microchannel, as discussed previously.
 |
| Fig. 4 Oxygen sensor-enabled monitoring of cisplatin-induced injury to hRPTECs in the mcPT. (a) Post/predrug oxygen consumption measurements over time for a range of cisplatin doses. Maximal injury response occurred within 48 h (black arrow). (b) Dose-dependent reduction in post/predrug oxygen consumption (markers) with fitted hill equations (solid lines) for estimation of the IC20 at different timepoints. The IC20s at 48 and 120 h are shown as orange and blue dotted lines, respectively. (c) The IC20 (red circles) for cisplatin decreased exponentially over time in the mcPT. Data are mean ± standard deviation of n = 4–6 devices per dose. *p < 0.05, **p < 0.01, ***p < 0.001 relative to untreated (Kruskal–Wallis and Dunn's post-hoc test). | |
Dose response curves based on the conventional 4-parameter Hill equation50,51 were estimated for data collected at 48, 72, 96, and 120 h for prediction of an IC20, the concentration at which 20% reduction in post/predrug oxygen consumption was observed. Maximal injury in the mcPT was observed at 48 h, indicated by a black arrow in Fig. 4a. Therefore, data collected prior to 48 h was not included in the hill function curve fitting. Fig. 4b shows dose response curves (solid lines) and experimental data (markers) for each timepoint from 48–120 h of cisplatin exposure. The IC20 decreased as exposure to cisplatin increased between the 48 and 120 h timepoints, shown as orange and blue dotted lines, respectively, in Fig. 4b and followed an exponential decay, shown in Fig. 4c. The fitted exponential, shown in eqn (1), fit the experimental data closely with an R2 of 1.00 and predicted a long-term IC20 of 2.2 μM. The predicted IC20s at 24 h timepoints over 5 days were provided in Table 1 below.
| IC20(t) = 58.46e−0.045t + 2.16 | (1) |
Table 1 Summary of predicted IC20s during a 5-day exposure to cisplatin
Timepoint (h) |
24 |
48 |
72 |
96 |
120 |
Infinity |
IC20 (μM) |
19.8 |
7.5 |
3.8 |
2.7 |
2.3 |
2.2 |
These results demonstrated a new capability of a high-throughput oxygen sensor integrated organ-on-chip platform to rapidly predict a time-dependent concentration threshold at which drug-induced injury occurs in a human PT model. The 5-day IC20 for cisplatin of 2.3 μM was below expected blood concentrations of cisplatin reported in 5-day infusions,48 which may explain the prevalence of cisplatin-induced kidney injury in the clinic. Additionally, the time-dependent decrease in the IC20 of cisplatin in the mcPT supports data in animal models suggesting that cisplatin-induced kidney injury is dose and time-dependent.3,4 Our predicted 5-day IC20 in the mcPT was in close agreement with a 5-day cisplatin toxicity threshold of 2.8 μM predicted using perfused vascularized human kidney spheroids.52 Interestingly, the toxicity thresholds for cisplatin were similar for hRPTECs cultured in spheroids and a membrane bilayer format, despite significant differences in the tissue architecture between the two systems. While spheroids have shown promise for toxicological studies,52 there are key advantages of performing toxicological assessments in membrane bilayer formats including access to both apical and basal surfaces of the tissue layer for transport studies, independent control of flow rates5,22 in each microchannel, and compatibility with barrier-specific assays.53,54 We believe that this system and the oxygen measurement technique could aid clinicians and drug developers in optimizing treatment regimens of nephrotoxic agents and investigation of protective strategies for mitigation of drug-induced nephrotoxicity. Additionally, this technique is broadly applicable for toxicological assessment in other complex tissue models that utilize a membrane bilayer format.24,45,47,55
Oxygen vs. colorimetric-based measurements for monitoring hRPTEC injury
We compared our oxygen sensing technique with the CyQUANT™ LDH Cytotoxicity assay, a commercial colorimetric-based assay, for assessment of hRPTEC injury during the 5-day exposure to cisplatin. The CyQUANT™ assay quantifies cytotoxicity via extraction of cell culture medium from each device and measurement of the amount of lactate dehydrogenase (LDH), an enzyme in healthy cells that is released upon damage to the cell membrane. Fig. 5a and b shows cumulative LDH, the total LDH released in culture medium relative to t = 0 h, and post/predrug oxygen consumption for doses in the range of 0–200 μM and at 24 h timepoints. Both cumulative LDH and post/predrug oxygen consumption readouts displayed an expected dose-dependent response at the early timepoints of 24 and 48 h of exposure. Interestingly, between 72–120 h, post/predrug oxygen consumption continued to display an expected dose-dependent trend, whereas cumulative LDH did not. Specifically, cumulative LDH in the middle dose groups of 10 and 25 μM was higher than in the high dose groups of 100 and 200 μM, as shown in Fig. 5a, which was not consistent with the dose response in the post/predrug oxygen consumption readouts, data from other studies,22 and the general expectation that injury increases with dose of a toxic drug. This inconsistency was likely due to differences in the time at which maximum cytotoxicity, quantified via LDH release, occurred between the middle and high dose groups. Specifically, maximum cytotoxicity occurred within 24 h in the high dose group compared to 48–96 h in the middle dose group, as shown in Fig. S7.† We found that the combination of different timepoints of maximum cytotoxicity and expected hRPTEC proliferation over time to likely be significant factors leading to an unexpected dose-dependent response in the cumulative LDH readouts between 72–120 h. On the other hand, post/predrug oxygen consumption readouts resulted in an expected dose–response at all timepoints similar to dose–response data for cisplatin reported in other studies.22,52 Therefore, we found post/predrug oxygen consumption to be a more robust and consistent metric for evaluating dose-dependent injury over multiple days of exposure compared to cumulative LDH. It should be noted that post/predrug oxygen consumption in the vehicle group was slightly lower than the untreated and low dose groups. This was likely due to a higher concentration of saline present in the vehicle group compared to the untreated and low dose groups, to maintain equivalent concentrations of saline between the vehicle and highest dose groups.
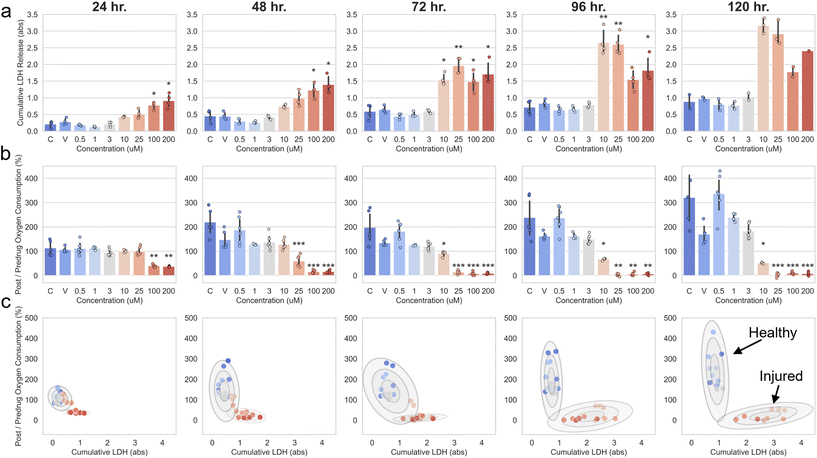 |
| Fig. 5 Comparison between oxygen consumption and colorimetric-based cytotoxicity readouts for monitoring hRPTEC injury in the mcPT. (a) Cumulative LDH in the hRPTEC microchannel and (b) post/predrug oxygen consumption for each timepoint during exposure of hRPTECs to cisplatin for 5 days (*p < 0.05, **p < 0.01, ***p < 0.0001 relative to untreated within each timepoint between 24–96 h using Kruskal–Wallis and Dunn's post-hoc test). There were insufficient replicates in cumulative LDH data at 120 h for statistical testing. (c) Unsupervised clustering of individual mcPTs into separate groups, representing healthy and injured tissue, at each timepoint based on post/predrug oxygen consumption and cumulative LDH data. | |
Combination of post/predrug oxygen consumption and cumulative LDH readouts for each mcPT allowed visualization of the time-dependent formation of two distinct clusters of mcPTs representing healthy and injured tissue following 5 days of cisplatin exposure. Fig. 5c shows the relationship between post/predrug oxygen consumption and cumulative LDH readouts at each timepoint during cisplatin exposure, in which each datapoint represents an individual mcPT. Unsupervised classification, based on probabilistic Gaussian mixture models,56 of the datapoints in Fig. 5c into two distinct clusters resulted in prediction of mcPTs that were healthy or injured. At 120 h, the predicted healthy and injured clusters contained mcPTs in the 0–3 μM and 10–200 μM range, respectively, which supported image-based observations of cell death occurring in the 10–200 μM range at 120 h, shown previously in Fig. 3a and b. Changes in the shapes of the two clusters and in the occupancy of mcPTs in each cluster over time, shown in Fig. 5c, were dynamic indicators of injury occurring in the mcPT based on two different metrics of cell health. Specifically, between 24–96 h, mcPTs exposed to cisplatin in the range of 10–25 μM switched occupancy from the healthy cluster to the injured cluster.
Our reported method for measurement of steady state oxygen levels has key advantages over conventional colorimetric-based assays, as summarized in Table 2 below. Additionally, we found that monitoring oxygen levels in P96-O2 allowed measurement of an expected dose-dependent injury response for cisplatin across all timepoints during a 5-day drug exposure. This demonstrated a key advantage over cumulative LDH readouts, which resulted in a dose–response that was inconsistent with expectations between 72–120 h of exposure. Additionally, we showed that the combination of oxygen consumption measurements and colorimetric-based cytotoxicity readouts in P96-O2 allowed visualization of distinct clusters, representing healthy and injured mcPTs, which provided a useful new readout for monitoring the progression of injury in mcPTs during multiple-day drug exposures.
Table 2 Key advantages of oxygen sensing compared to colorimetric-based assays for toxicological assessment in high-throughput microfluidic organ-on-chip systems
Key performance metrics |
Oxygen sensing |
Colorimetric-based assay |
An additional 30–45 minutes was required for a P96-O2 plate to reach temperature equilibrium prior to oxygen measurements.
|
Data collection speed |
32 min per platea |
∼1–2 h per plate |
On-chip measurements |
Yes; label-free & non-invasive |
No; requires extraction of culture medium and multiple liquid handling steps |
Enables perfusion during data collection |
Yes |
No |
Chemical reagents required |
No |
Yes |
Conclusions
For the first time, we demonstrated a technique to monitor oxygen consumption during continuous perfusion in 96 mcPTs within 32 minutes, which enabled rapid and non-invasive assessment of drug-induced nephrotoxicity. Oxygen consumption measurements detected time and dose-dependent injury responses to cisplatin, a nephrotoxic drug, during a clinically relevant 5-day exposure duration. The IC20 of cisplatin decreased as a function of exposure time in the mcPT, which agreed with findings in animal studies and in other in vitro models that cisplatin toxicity is dose and time dependent. Furthermore, the 5-day IC20 in the mcPT was below clinically reported blood concentrations of cisplatin during 5-day infusions,48 supporting incidences of cisplatin-induced kidney injury in the clinic. Also, oxygen consumption readouts in the mcPT provided more robust and expected dose–response data than conventional colorimetric-based cytotoxicity readouts at later timepoints of cisplatin exposure. Our results demonstrated the utility of monitoring steady state oxygen levels in a high-throughput organ-on-chip platform for non-invasive and rapid assessment of oxygen consumption and nephrotoxicity in a human co-culture PT model. The presented technique will provide a valuable tool in drug development for evaluating injury in microfluidic kidney models for toxicological screening and investigation of potential therapies.
Experimental
Cell culture
Primary human renal proximal tubule epithelial cells (hRPTECs) were purchased from ScienCell Research Laboratories (Lot: 5340) and expanded through passage 5 prior to seeding in P96-O2 plates. hRPTECs were cultured in culture medium consisting of 50
:
50 DMEM/F12 (Gibco, USA) supplemented with 0.5% fetal bovine serum (Life Technologies, USA), 10 ng mL−1 recombinant human epidermal growth factor (Thermo Fisher, USA), 5 μg mL−1 insulin (Sigma-Aldrich, USA), 6.5 ng mL−1 3,3′,5-triiodo-L-thyronine sodium salt (Sigma-Aldrich, USA), 10 μg mL−1 human transferrin (Sigma-Aldrich, USA), and 1% penicillin–streptomycin (Thermo Fisher, USA). Primary human dermal microvascular endothelial cells (hMVECS) were purchased from Lonza (CC-2543) and expanded through passage 6. hMVECs were cultured in a commercial medium kit (CC-3156, Lonza, USA) consisting of EBM™-2 Basal Medium (CC-3156) and EGM™-2 MV microvascular endothelial cell growth medium supplements (CC-4146). hRPTECs were cultured in a T-150 flask (Thermo Fisher, USA) with 30 mL of hRPTEC culture medium for 2 days prior to seeding in P96-O2. hMVECs were cultured in a T-150 flask coated with 1 μg cm−2 human plasma fibronectin with 30 mL of hMVEC culture medium for 2 days prior to seeding in P96-O2.
Preparation of the P96-O2 and micropump array
The fabrication of the P96-O2 culture plates and micropump array was described previously in detail.5,41 Briefly, P96-O2 plates contained 96 optical-based oxygen-sensitive sensor spots (0.75 mm in diameter and 50 μm thick) that were cut from a sheet of photosensitive oxygen sensor foil (item No. OXFOIL-TN, Pyroscience, Germany). Each P96-O2 plate contained an 11 μm thick polycarbonate track-etched porous membrane with a pore diameter of 1 μm (Sterlitech, WA, USA). P96-O2 plates and the micropump array were sterilized with ethylene oxide exposure for 24 hours, followed by a minimum of 48 hours of degassing. P96-O2 plates were treated with oxygen plasma for 60 seconds to increase the hydrophilicity of the microchannel surfaces. Microchannels of the P96-O2 were filled with 70% ethanol and subsequently rinsed 3 times with phosphate-buffered saline (PBS).
Cell seeding in P96-O2
Prior to seeding cells in P96-O2, each microchannel was filled with a solution of 60 μg mL−1 of collagen IV from human placenta (Cat #: CC076, Sigma Aldrich, USA) in PBS and the plate was placed on a rocker for 1 hour at room temperature. Co-cultures of hMVECs and hRPTECs and monocultures of hRPTECs were cultured in specified regions of the P96-O2 plate, as shown in the plate map in Fig. S2.† hMVECs were seeded in the top microchannel two days prior to seeding of hRPTECs in the bottom microchannel. 30 μL of a 1 million per mL cell suspension was delivered to the inlet of each top microchannel via a gravity seeding method previously described.5 For seeding of hRPTECs, 30 μL of a 1 million per mL cell suspension was delivered to the inlet of each bottom microchannel and the plate was subsequently flipped face-down and incubated at 37 °C for 1 hour to allow cells to adhere to the porous membrane. Devices with suboptimal cell densities below approximately 500 cells per mm2 (25% of the expected density) were identified as outliers following cell seeding via phase contrast imaging. Suboptimal densities likely occurred due to error sources in the cell seeding process including low seeding densities and introduction of bubbles that interfere with adhesion. 14 devices were identified as outliers and excluded from all data presented in this manuscript. Following 1 hour, the plate was flipped face-up, culture medium in each microchannel was refreshed, and flow was initiated at 1 μL min−1 (0.01 dyne per cm2) for 1 day. Flow was increased to 70 μL min−1 (0.73 dyne per cm2) on day 2 for the remainder of the experiment and the culture medium in each microchannel was refreshed daily.
Oxygen consumption measurements
Oxygen measurements were acquired with a commercial optical oxygen meter, optical fiber, and software (FireStingO2, Pyroscience, Germany). P96-O2 was placed in a standard confocal microscope with an incubation chamber (LSM, 780) and the fiber optic was secured parfocally onto the objective turret using a custom fixture, described in a previous work.41 The microscope's 10× objective and programmable stage was used to visually locate each sensor spot to ensure that the distance between the fiber optic and each sensor spot was consistent across all 96 devices. The fiber optic positions for all 96 devices were manually assigned and saved using the microscope's software. A 2-point calibration of the oxygen sensors was performed using the FiresStingO2 software. Microchannels were filled with water and 30 g L−1 of sodium sulfite in water to obtain the high and low setpoint values corresponding to 100% air saturated and 0% air saturated solutions, respectively. Measurement of steady state oxygen in a P96-O2 plate was accomplished via automated and sequential positioning of the fiber optic beneath each device with a dwell time of approximately 12 seconds per device as the FireStingO2 acquired oxygen measurements at a sampling rate of 1 Hz. A custom-built algorithm in MATLAB was used to convert the raw data containing oxygen measurements acquired at 1 Hz for an entire P96-O2 plate into average readouts for each device in P96-O2. Oxygen measurements in each device were the average of 10–12 samples acquired at 1 Hz. Oxygen consumption was estimated as the difference between steady state oxygen measurements in devices filled with air saturated culture medium and devices containing culture medium and tissue.
Cisplatin treatment
cis-Diammineplatinum(II) dichloride (cisplatin) was purchased from Sigma, Aldrich (P4394-25MG) and dissolved in 0.9% sodium chloride in water to form a 2380 μM stock solution. The stock solution was diluted in culture medium to produce working concentrations of 0.5, 1, 3, 5, 10, 25, 100, and 200 μM. The vehicle group contained 8.4% sodium chloride solution, which was equivalent to the concentration of sodium chloride solution used in the highest cisplatin dose. Cisplatin doses were delivered via the inlets of the top and bottom microchannels of specified devices, shown in the plate map in Fig. S6.† Oxygen was measured in the bottom microchannel of each device at 24 h timepoints for the 9 doses of cisplatin ranging from 0–200 μM. Culture medium with the proper cisplatin dose was refreshed in each device every 24 hours following oxygen measurements.
Lactate dehydrogenase (LDH) assay
The CyQUANT™ LDH Cytotoxicity Assay (Thermo Fisher, USA), a standard colorimetric-based assay for assessing cellular cytotoxicity, was used for quantifying extracellular lactate dehydrogenase (LDH) in the culture medium. 30 μL of culture medium was extracted from the inlet and outlet of each bottom microchannel following oxygen measurements on day 7, 8, 9, 10, 11 (t = 24, 48, 72, 96, 120 h following initial cisplatin exposure) and transferred to a 96 well plate. Samples were subsequently transferred in duplicates to two 96 well plates. 15 μL of the CyQUANT™ LDH Cytotoxicity Assay reaction mixture was added to each medium sample for 30 minutes at room temperature. Following 30 minutes, 15 μL of the CyQUANT™ LDH Cytotoxicity Assay stop solution was added and plates were centrifuged to remove any bubbles present in the wells. Absorbance was measured at 490 nm and 680 nm in each well with a standard microplate reader. LDH content for each device was quantified from the absorbance readings, as shown in eqn (2) below, in which i represents the technical replicate number. |  | (2) |
Culture medium was refreshed following LDH measurements at 24 h timepoints. Cumulative LDH, the amount of LDH released in the culture medium over multiple timepoints, was computed by summing LDH at each timepoint with LDH from all previous timepoints.
Immunofluorescent staining and imaging
On day 11, hRPTECs and hMVECs were fixed with 4% paraformaldehyde (Thermo Fisher) in PBS for 10 minutes followed by three rinses with PBS. Microchannels were filled with 0.1% Triton-X (Thermo Fisher, USA) with PBS for 5 minutes to permeabilize the cells. Microchannels were blocked with 3% normal goat serum (NGS) in PBS for 1 h on a rocker at room temperature. Mouse primary ZO-1 antibodies (Abcam, USA) were diluted 1
:
200 in NGG and incubated in each microchannel for 24 h at 4 °C. Microchannels were rinsed 3 times with PBS. Goat anti-mouse secondary antibodies (IgG H&L Alexa Fluor 488, Thermo Fisher, USA) were diluted 1
:
250 in NGS. For labelling nuclei and filamentous actin, Hoechst 33342 and Phalloidin-iFluor 633 were diluted 1
:
500 and 1
:
1000, respectively, in NGS. Microchannels were filled with NGS containing secondary antibodies, nuclei, and filamentous actin labels for 3 h at room temperature on a rocker. Microchannels were rinsed 3 times with PBS prior to imaging. Images were acquired with a scanning confocal microscope (Zeiss, LSM710) using a 40× water immersion objective and lasers with excitations wavelengths of 405, 488, and 637 nm.
hRPTEC nuclei count quantification
Two 40× images of nuclei for 317 × 317 μm regions of interest were captured on the membrane on the left and right side of the light absorbing sensor spot. Stardist,57 an open-source image processing pipeline in python, was used for segmentation and counting of nuclei in each image. Nuclei counts were divided by the surface area of the region of interest to compute nuclei density on the left and right side of the sensor. The nuclei densities on the left and right side of the sensor were averaged to yield an averaged nuclei density for a single device.
Mathematical modelling of dose response curves
The dose response curves of cisplatin were computed using the standard Hill equation,58 shown in eqn (3) below, where x is the concentration, y0 is the minimum response, y∞ is the maximum response, H is the Hill coefficient and x50 is the concentration resulting in 50% of a maximal response. |  | (3) |
Eqn (3) was fitted to the experimental data via minimization of the least residual squares to compute predicted dose response curves at each timepoint. The IC20, concentration resulting in 20% of maximal response, was computed based on eqn (4), where F is the percent response.
| 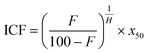 | (4) |
All computational analysis related to fitting dose–response curves based on the Hill equation was performed using custom-made pipelines in python with the SciPy module.59
Gaussian mixture model-based clustering
Unsupervised clustering was performed based on probabilistic Gaussian mixture models and implemented using the Sklearn.mixture module in sci-kit learn.60 Clusters were assumed to contain datapoints following a Gaussian distribution. The expectation-maximization algorithm61 was implemented to return parameters for Gaussian distributions that resulted in the highest probability of containing the datapoints. For visualization purposes, the optimized Gaussian distributions were projected to two-dimensional space and represented as ellipses.
Statistical analysis
Data are presented as mean ± standard deviation of measurements. All n values represent the number of individual devices per condition. Kruskal–Wallis & Dunn's post-hoc tests were used to calculate significant differences between different groups. All statistical tests were performed in python using Scipy59 and Sci-kit learn.60
Author contributions
Kann, Samuel H.: designed/conceived measurement methodology, led and executed engineering and biological experiments, analyzed & interpreted data, wrote manuscript. Shaughnessey, Erin M.: executed biological experiments, supported development of measurement methodology, analyzed & interpreted data, wrote manuscript. Zhang, Xin: led experimental strategy, supported development of measurement methodology, analyzed & interpreted data, wrote manuscript, funding acquisition. Charest, Joseph L.: designed/conceived measurement methodology, designed/conceived platform technology, led experimental strategy, analyzed & interpreted data, wrote manuscript, funding acquisition. Vedula, Else M.: designed/conceived measurement methodology, designed/conceived platform technology, led experimental strategy, analyzed & interpreted data, wrote manuscript, funding acquisition.
Conflicts of interest
There are no conflicts to declare.
Acknowledgements
The authors would like to acknowledge significant contributors to this work. Brian Cain and Yazmin Obi for fabrication of PREDICT96-O2 and micropump array. Hesham Azizgolshani, Jonathan Coppeta, and Brett Isenberg for their contributions to the oxygen sensing hardware and methodology. This material is based upon work supported by the National Science Foundation under Grant No. 1804845 and 1804787. Any opinions, findings and conclusions or recommendations expressed in this material are those of the author(s) and do not necessarily reflect the views of the National Science Foundation. This work was also supported by the Draper Scholar Program.
References
- M. J. Wilmer, C. P. Ng, H. L. Lanz, P. Vulto, L. Suter-Dick and R. Masereeuw, Trends Biotechnol., 2016, 34, 156–170 CrossRef CAS PubMed.
- S. R. A. Kratz, G. Höll, P. Schuller, P. Ertl and M. Rothbauer, Biosensors, 2019, 9, 110 CrossRef CAS PubMed.
- Z. Pezeshki, A. Khosravi, M. Nekuei, S. Khoshnood, E. Zandi, M. Eslamian, A. Talebi, S. N. Emami and M. Nematbakhsh, J. Nephropathol., 2017, 6, 163–167 CrossRef PubMed.
- P. Zhang, J. Chen, Y. Wang, Y. Huang, Y. Tian, Z. Zhang and F. Xu, Chem. Res. Toxicol., 2016, 29, 776–783 Search PubMed.
- H. Azizgolshani, J. R. Coppeta, E. M. Vedula, E. E. Marr, B. P. Cain, R. J. Luu, M. P. Lech, S. H. Kann, T. J. Mulhern, V. Tandon, K. Tan, N. J. Haroutunian, P. Keegan, M. Rogers, A. L. Gard, K. B. Baldwin, J. C. de Souza, B. C. Hoefler, S. S. Bale, L. B. Kratchman, A. Zorn, A. Patterson, E. S. Kim, T. A. Petrie, E. L. Wiellette, C. Williams, B. C. Isenberg and J. L. Charest, Lab Chip, 2021, 21, 1454–1474 RSC.
- K. M. Bircsak, R. DeBiasio, M. Miedel, A. Alsebahi, R. Reddinger, A. Saleh, T. Shun, L. A. Vernetti and A. Gough, Toxicology, 2021, 450, 152667 CrossRef CAS PubMed.
- E. M. Vedula, J. L. Alonso, M. A. Arnaout and J. L. Charest, PLoS One, 2017, 12, e0184330 CrossRef PubMed.
- T. T. G. Nieskens, M. Persson, E. J. Kelly and A.-K. Sjögren, Drug Metab. Dispos., 2020, 48, 1303–1311 CrossRef CAS PubMed.
- K.-J. Jang, A. P. Mehr, G. A. Hamilton, L. A. McPartlin, S. Chung, K.-Y. Suh and D. E. Ingber, Integr. Biol., 2013, 5, 1119–1129 CrossRef CAS PubMed.
- S. K. Nigam, W. Wu, K. T. Bush, M. P. Hoenig, R. C. Blantz and V. Bhatnagar, Clin. J. Am. Soc. Nephrol., 2015, 10, 2039–2049 CrossRef CAS PubMed.
- Y. Duan, N. Gotoh, Q. Yan, Z. Du, A. M. Weinstein, T. Wang and S. Weinbaum, Proc. Natl. Acad. Sci. U. S. A., 2008, 105, 11418–11423 CrossRef CAS PubMed.
- E. M. Frohlich, X. Zhang and J. L. Charest, Integr. Biol., 2012, 4, 75–83 CrossRef CAS PubMed.
- M. Essig, F. Terzi, M. Burtin and G. Friedlander, Am. J. Physiol.: Renal, Fluid Electrolyte Physiol., 2001, 281, F751–F762 CrossRef CAS PubMed.
- Q. Ren, M. L. Gliozzi, N. L. Rittenhouse, L. R. Edmunds, Y. Rbaibi, J. D. Locker, A. C. Poholek, M. J. Jurczak, C. J. Baty and O. A. Weisz, Traffic, 2019, 20, 448–459 CrossRef CAS PubMed.
- S. J. Kunnen, T. B. Malas, C. M. Semeins, A. D. Bakker and D. J. M. Peters, J. Cell. Physiol., 2018, 233, 3615–3628 CrossRef CAS PubMed.
- J. Vriend, J. G. P. Peters, T. T. G. Nieskens, R. Škovroňová, N. Blaimschein, M. Schmidts, R. Roepman, T. J. J. Schirris, F. G. M. Russel, R. Masereeuw and M. J. Wilmer, Biochim. Biophys. Acta, Gen. Subj., 2020, 1864, 129433 CrossRef CAS PubMed.
- L. Yin, G. Du, B. Zhang, H. Zhang, R. Yin, W. Zhang and S.-M. Yang, Sci. Rep., 2020, 10, 6568 CrossRef CAS PubMed.
- G. Nowak and R. G. Schnellmann, Am. J. Physiol.: Cell Physiol., 1995, 268, C1053–C1061 CrossRef CAS PubMed.
- P. Bhargava and R. G. Schnellmann, Nat. Rev. Nephrol., 2017, 13, 629–646 CrossRef CAS PubMed.
- F. Tasnim and D. Zink, Am. J. Physiol.: Renal, Fluid Electrolyte Physiol., 2012, 302, F1055–F1062 CrossRef CAS PubMed.
- S. Aydin, S. Signorelli, T. Lechleitner, M. Joannidis, C. Pleban, P. Perco, W. Pfaller and P. Jennings, Am. J. Physiol.: Cell Physiol., 2008, 294, C543–C554 CrossRef CAS PubMed.
- E. M. Shaughnessey, S. H. Kann, H. Azizgolshani, L. D. Black, J. L. Charest and E. M. Vedula, Sci. Rep., 2022, 12, 13182 CrossRef CAS PubMed.
- M. T. Rogers, A. L. Gard, R. Gaibler, T. J. Mulhern, R. Strelnikov, H. Azizgolshani, B. P. Cain, B. C. Isenberg, N. J. Haroutunian, N. E. Raustad, P. M. Keegan, M. P. Lech, L. Tomlinson, J. T. Borenstein, J. L. Charest and C. Williams, Sci. Rep., 2021, 11, 12225 CrossRef CAS PubMed.
- A. L. Gard, R. J. Luu, C. R. Miller, R. Maloney, B. P. Cain, E. E. Marr, D. M. Burns, R. Gaibler, T. J. Mulhern, C. A. Wong, J. Alladina, J. R. Coppeta, P. Liu, J. P. Wang, H. Azizgolshani, R. F. Fezzie, J. L. Balestrini, B. C. Isenberg, B. D. Medoff, R. W. Finberg and J. T. Borenstein, Sci. Rep., 2021, 11, 14961 CrossRef CAS PubMed.
- A. Nicolas, F. Schavemaker, K. Kosim, D. Kurek, M. Haarmans, M. Bulst, K. Lee, S. Wegner, T. Hankemeier, J. Joore, K. Domansky, H. L. Lanz, P. Vulto and S. J. Trietsch, Lab Chip, 2021, 21, 1676–1685 RSC.
- M. Azimzadeh, P. Khashayar, M. Amereh, N. Tasnim, M. Hoorfar and M. Akbari, Biosensors, 2021, 12, 6 CrossRef PubMed.
- E. Tanumihardja, R. H. Slaats, A. D. van der Meer, R. Passier, W. Olthuis and A. van den Berg, ACS Sens., 2021, 6, 267–274 CrossRef CAS PubMed.
- A. Weltin, K. Slotwinski, J. Kieninger, I. Moser, G. Jobst, M. Wego, R. Ehret and G. A. Urban, Lab Chip, 2014, 14, 138–146 RSC.
- A. Moya, M. Ortega-Ribera, X. Guimerà, E. Sowade, M. Zea, X. Illa, E. Ramon, R. Villa, J. Gracia-Sancho and G. Gabriel, Lab Chip, 2018, 18, 2023–2035 RSC.
- O. S. Wolfbeis, BioEssays, 2015, 37, 921–928 CrossRef CAS PubMed.
- S. M. Grist, L. Chrostowski and K. C. Cheung, Sensors, 2010, 10, 9286–9316 CrossRef CAS PubMed.
- J. Ehgartner, P. Sulzer, T. Burger, A. Kasjanow, D. Bouwes, U. Krühne, I. Klimant and T. Mayr, Sens. Actuators, B, 2016, 228, 748–757 CrossRef CAS.
- M. Koopman, H. Michels, B. M. Dancy, R. Kamble, L. Mouchiroud, J. Auwerx, E. A. A. Nollen and R. H. Houtkooper, Nat. Protoc., 2016, 11, 1798–1816 CrossRef CAS PubMed.
- P. Wolf, M. Brischwein, R. Kleinhans, F. Demmel, T. Schwarzenberger, C. Pfister and B. Wolf, Biosens. Bioelectron., 2013, 50, 111–117 CrossRef CAS PubMed.
- M. Busche, D. Rabl, J. Fischer, C. Schmees, T. Mayr, R. Gebhardt and M. Stelzle, EXCLI J., 2022, 21, 144–161 Search PubMed.
- H. Zirath, S. Spitz, D. Roth, T. Schellhorn, M. Rothbauer, B. Müller, M. Walch, J. Kaur, A. Wörle, Y. Kohl, T. Mayr and P. Ertl, Lab Chip, 2021, 21, 4237–4248 RSC.
- R. Krenger, M. Cornaglia, T. Lehnert and M. A. M. Gijs, Lab Chip, 2020, 20, 126–135 RSC.
- F. Bunge, S. van den Driesche, M. Waespy, A. Radtke, G. Belge, S. Kelm, A. M. Waite, U. Mirastschijski and M. J. Vellekoop, Sens. Actuators, B, 2019, 289, 24–31 CrossRef CAS.
- S. A. Mousavi Shaegh, F. De Ferrari, Y. S. Zhang, M. Nabavinia, N. Binth Mohammad, J. Ryan, A. Pourmand, E. Laukaitis, R. Banan Sadeghian, A. Nadhman, S. R. Shin, A. S. Nezhad, A. Khademhosseini and M. R. Dokmeci, Biomicrofluidics, 2016, 10, 044111 CrossRef PubMed.
- B. Müller, P. Sulzer, M. Walch, H. Zirath, T. Buryška, M. Rothbauer, P. Ertl and T. Mayr, Sens. Actuators, B, 2021, 334, 129664 CrossRef.
- S. H. Kann, E. M. Shaughnessey, J. R. Coppeta, H. Azizgolshani, B. C. Isenberg, E. M. Vedula, X. Zhang and J. L. Charest, Microsyst. Nanoeng., 2022, 8, 109 CrossRef CAS PubMed.
- E. H. J. Verschuren, C. Castenmiller, D. J. M. Peters, F. J. Arjona, R. J. M. Bindels and J. G. J. Hoenderop, Nat. Rev. Nephrol., 2020, 16, 337–351 CrossRef CAS PubMed.
- A. Weltin, S. Hammer, F. Noor, Y. Kaminski, J. Kieninger and G. A. Urban, Biosens. Bioelectron., 2017, 87, 941–948 CrossRef CAS PubMed.
- A. A. Gerencser, A. Neilson, S. W. Choi, U. Edman, N. Yadava, R. J. Oh, D. A. Ferrick, D. G. Nicholls and M. D. Brand, Anal. Chem., 2009, 81, 6868–6878 CrossRef CAS PubMed.
- H. J. Kim and D. E. Ingber, Integr. Biol., 2013, 5, 1130 CrossRef CAS PubMed.
- T.-E. Park, N. Mustafaoglu, A. Herland, R. Hasselkus, R. Mannix, E. A. FitzGerald, R. Prantil-Baun, A. Watters, O. Henry, M. Benz, H. Sanchez, H. J. McCrea, L. C. Goumnerova, H. W. Song, S. P. Palecek, E. Shusta and D. E. Ingber, Nat. Commun., 2019, 10, 2621 CrossRef PubMed.
- K. Tan, P. Keegan, M. Rogers, M. Lu, J. R. Gosset, J. Charest and S. S. Bale, Lab Chip, 2019, 19, 1556–1566 RSC.
- P. Salem, M. Khalyl, K. Jabboury and L. Hashimi, Cancer, 1984, 53, 837–840 CrossRef CAS PubMed.
- C. Tang, M. J. Livingston, R. Safirstein and Z. Dong, Nat. Rev. Nephrol., 2022, 1–20 Search PubMed.
- A. Hill, J. Physiol., 1910, 40iv–4vii Search PubMed.
- S. Goutelle, M. Maurin, F. Rougier, X. Barbaut, L. Bourguignon, M. Ducher and P. Maire, Fundam. Clin. Pharmacol., 2008, 22, 633–648 CrossRef CAS PubMed.
- A. Cohen, K. Ioannidis, A. Ehrlich, S. Regenbaum, M. Cohen, M. Ayyash, S. S. Tikva and Y. Nahmias, Sci. Transl. Med., 2021, 13, eabd6299 CrossRef CAS PubMed.
- M. W. van der Helm, O. Y. F. Henry, A. Bein, T. Hamkins-Indik, M. J. Cronce, W. D. Leineweber, M. Odijk, A. D. van der Meer, J. C. T. Eijkel, D. E. Ingber, A. van den Berg and L. I. Segerink, Lab Chip, 2019, 19, 452–463 RSC.
- M. K. Vormann, L. Gijzen, S. Hutter, L. Boot, A. Nicolas, A. van den Heuvel, J. Vriend, C. P. Ng, T. T. G. Nieskens, V. van Duinen, B. de Wagenaar, R. Masereeuw, L. Suter-Dick, S. J. Trietsch, M. Wilmer, J. Joore, P. Vulto and H. L. Lanz, AAPS J., 2018, 20, 90 CrossRef PubMed.
- A. Agarwal, J. A. Goss, A. Cho, M. L. McCain and K. K. Parker, Lab Chip, 2013, 13, 3599 RSC.
-
G. J. McLachlan and K. E. Basford, Mixture models: Inference and applications to clustering, 1988 Search PubMed.
-
U. Schmidt, M. Weigert, C. Broaddus and G. Myers, in Medical Image Computing and Computer Assisted Intervention – MICCAI 2018, ed. A. F. Frangi, J. A. Schnabel, C. Davatzikos, C. Alberola-López and G. Fichtinger, Springer International Publishing, Cham, 2018, pp. 265–273 Search PubMed.
- R. L. Prentice, Biometrics, 1976, 32, 761–768 CrossRef CAS PubMed.
- SciPy 1.0: fundamental algorithms for scientific computing in Python | Nature Methods, https://www.nature.com/articles/s41592-019-0686-2?report=reader, (accessed November 1, 2022).
- F. Pedregosa, G. Varoquaux, A. Gramfort, V. Michel, B. Thirion, O. Grisel, M. Blondel, P. Prettenhofer, R. Weiss, V. Dubourg, J. Vanderplas, A. Passos, D. Cournapeau, M. Brucher, M. Perrot and É. Duchesnay, J. Mach. Learn. Technol., 2011, 12, 2825–2830 Search PubMed.
- A. P. Dempster, N. M. Laird and D. B. Rubin, J. R. Stat. Soc., B: Stat. Methodol., 1977, 39, 1–22 Search PubMed.
Footnotes |
† Electronic supplementary information (ESI) available. See DOI: https://doi.org/10.1039/d3an00380a |
‡ Currently at Biogen, 225 Binney Street, Cambridge, MA 02142, USA. |
|
This journal is © The Royal Society of Chemistry 2023 |
Click here to see how this site uses Cookies. View our privacy policy here.