DOI:
10.1039/D2CB00214K
(Perspective)
RSC Chem. Biol., 2023,
4, 110-120
Finding a vocation for validation: taking proteomics beyond association and location
Received
6th October 2022
, Accepted 1st December 2022
First published on 2nd December 2022
Abstract
First established in the seventies, proteomics, chemoproteomics, and most recently, spatial/proximity-proteomics technologies have empowered researchers with new capabilities to illuminate cellular communication networks that govern sophisticated decision-making processes. With an ever-growing inventory of these advanced proteomics tools, the onus is upon the researchers to understand their individual advantages and limitations, such that we can ensure rigorous implementation and conclusions derived from critical data interpretations backed up by orthogonal series of functional validations. This perspective—based on the authors’ experience in applying varied proteomics workflows in complex living models—underlines key book-keeping considerations, comparing and contrasting most-commonly-deployed modern proteomics profiling technologies. We hope this article stimulates thoughts among expert users and equips new-comers with practical knowhow of what has become an indispensable tool in chemical biology, drug discovery, and broader life-science investigations.
At the point of writing this piece, the human genome, as well as many others, has been sequenced.1 This gamut of information alone must be sufficient to create and regulate all the proteins within a cell, and indeed an organism. Nevertheless, the genomic blueprint has emerged to be an inadequate basis for us to predict complex cellular functions/interactions/pathways and the like. Unsurprisingly, efforts have moved to unravel these problems using approaches that investigate cellular apparatuses functioning downstream of DNA. A large amount of work has been levied on investigating transcriptional programs, either through RNA-seq, or ChIP-seq. Such methods continue to provide keen insights into cellular diversity and responsivity. These investigations can derive meaningful information on the workhorses of the cell, and its proteins, particularly in terms of expression regulation and upstream activating factors, among others. Such insights may give some hints as to protein function or associations. Nonetheless, these experiments can only provide broad strokes, and afford little information and insight at the molecular level. However, such molecular-level understanding is particularly important for pathway analyses, mechanistic investigations, and therapeutic interventions.
To investigate life at the protein level in molecular detail, a special branch of biology has developed. This has been given the moniker “proteomics”. This branch of biological investigation is distinct from nucleic acid–based sequencing methods which rely upon amplification of nucleic acid polymers catalytically, often introducing new information in the process, such as bar coding, followed by a sequencing experiment. Proteomics experiments use purely extant (i.e., there is no amplification) proteins in a cell/organism and use the high sensitivity of mass spectrometry (MS) to identify specific proteins enriched from the bulk proteome post-cell lysis or tissue homogenization.
We will break down proteomics experiments to serve as a guide for choice of specific MS-based target-ID approaches, and critically, follow-up steps after target-ID. Thus, we will start with different variations of proteomics experiments, their typical uses, and potential issues. We will move on to methods to perform the MS experiment (some of which impinge on the initial planning phase of the experiment) and analysis of data. Then, as proteomics experiments seek to unveil new functional properties of proteins, we will focus heavily on functional validations, discussing different model systems, different levels of validation, and correct analysis of data. Although we cede that such a piece is unlikely ever to cover all eventualities, and that there are likely many exceptions to recommendations we propose and trends we discuss, we hope that the logic and conceptual outline will provide some aid in planning, and especially validation of proteomics and other “global” analysis data. Indeed, given the varied number of relatively overlapping proteomics experiments that exist, it is likely that there will always be several options open to address almost any question. Critical planning, understanding of the positive points and limitations will allow for a high possibility of success. As we have previously written,2,3 blending methods, i.e., performing a similar experiment using different approaches to account for artifacts, and broaden search criteria, may also be helpful. But, as stated above, regardless of the proteomics method(s) used, the principal goal of a proteomics experiment is to provide hits for downstream validation. Thus, the experiment is not over till adequate downstream experimentation has been undertaken. Finally, our primer will discuss aspects of experimental design mostly in the context of cultured cells. However, we, and many other labs, also perform similar experiments, using similar considerations, on model organisms and we will discuss some of these aspects in relevant sections.
General methods of mass spectrometry for peptide identification
Mass spectrometry (MS) is used to compare protein compositions within different samples. As noted above, what is used in these experiments are only the specific proteins present in each sample, that are not amplified, or at least in terms of the backbone chemically changed. It is possible to examine intact proteins by MS,4 although in complex mixtures, the most common procedure, often referred to as bottom-up proteomics, is to perform a protease digest to create smaller peptides (∼10–25 amino acid fragments) followed by chromatographic separation and identification of the resulting fragments by tandem MS. Tandem MS is a particularly sensitive technique that can give both total composition and primary structural information on peptides by measuring the total mass of the peptide, and its fragmentation pattern. A similar argument applies to identification of chemical modification of peptides (note: in proteomics by default, we use the “native” peptide sequence, which aids identification of modifications). The identified peptides can be compared against the proteomic database for the species of interest to identify to which protein(s) they belong. Note, therefore all valid fragments must be attributable to cleavage by the specific protease used (e.g., for tryptic peptides, since the trypsin cleavage site must be proceeded by a K or an R, they must end in K or R, and must also be immediately proceeded by a K or an R is the protein sequence). Particularly for diagnostic purposes, those that are unique peptides, i.e., attributable to a single protein, are more relevant than those that are not.
One of the key issues in this field is therefore being able to quantitatively compare sample compositions over multiple different preparations/conditions. Unfortunately, despite its awesome sensitivity, MS is not in itself particularly quantitative: different peptides ionize with different efficiencies, meaning that even quite similar peptides may be intrinsically detected differently by spectrometers. Moreover, buffer compositions, for instance, that may vary from sample to sample, may also affect ionization. Thus, very careful normalization is needed in order to perform quantitative proteomics experiments that are state-of-the-art in the field. With modern instrumentation and careful experimental technique, it is possible to perform multiple independent experiments, comparing composition between different samples, and looking for outliers between different preparations. This is called label-free quantification (LFQ) MS, and it broadly relies on using spectral counting, or ion-intensity changes between different samples as a metric for enrichment (Fig. 1A). These different methods of quantifying proteins may be better suited to answering subtly-different questions.5 Nonetheless, the label-free method is in principle unlimited in the number of samples that can be analyzed, allowing a large number of replicates to be performed, along with a wide range of timepoints or experimental conditions. It is thus particularly useful for multivariable procedures, and when several conditions need to be tried.
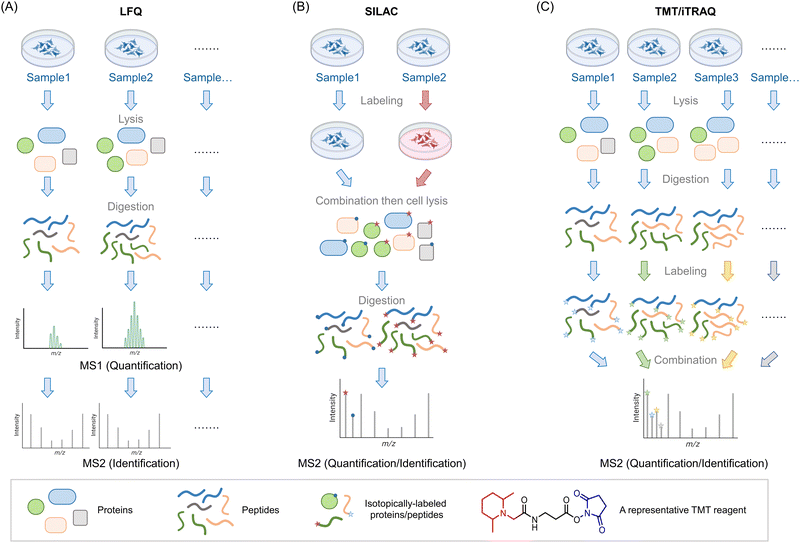 |
| Fig. 1 Introduction of three MS-based proteomics methods for peptide/protein identification. (A) Workflow of label-free quantification (LFQ) MS for biological samples. The proteins are extracted from lysed samples (cultured cells, C. elegans, animal tissues, etc.). After digestion by specific proteases (typically, trypsin), the peptides are analyzed by MS without tagging any labels. The quantitative protein signals are usually calculated by spectral counting or ion-intensity changes between different groups based on the MS1 spectra. The tandem MS2 spectra are used for peptide identification. (B) Workflow of stable isotope labeling in cell culture (SILAC). In traditional SILAC, cells are grown in media with isotope-labeled amino acids. For comparing two groups, a ‘heavy’ (red) and ‘light’ (blue) pair of media containing isotopic labeled arginine and lysine. A ‘medium’ preparation is also available, which allows comparison of 3 different groups in one single experiment. Samples with different isotopic labels are mixed 1 : 1 (: 1) typically prior to cell lysis/tissue homogenization (note: (approximately) equal protein amount across all groups is ensured by equivalent cell number or by normalization of lysate content). Because isotope distribution in the different samples is not the same, peptides with identical sequences from different groups arise in the same chromatographic peak but have different mass distributions, allowing quantification of relative changes within specific protein targets from specific groups. SILAC has been extended to several model organisms (e.g., C. elegans,8 zebrafish,10 mice9). (C) Workflow of tandem mass tag (TMT)- and isobaric tags for relative and absolute quantitation (iTRAQ)-based MS analysis. In these strategies, the cells/organs/tissues from different groups will be lysed/homogenized and separately digested by proteases such as trypsin. By introducing a set of small molecules with special reactivity towards amino groups (blue regions), peptides in each group are labeled by unique small molecule tags with special mass-reporter groups (red regions). After combining labeled peptides from different groups, peaks from reporter groups will provide information about the peptide abundance at the MS2 level. Peptides with identical sequences but labeled with different tags have the same MS1 peaks due to the balance group (black region) in these tags. The balance group contains a complementary isotope labeling to the reporter groups, enabling an identical mass of linked parts to the peptides. Up to 16-plex TMT tags are commercially available, enabling parallel analysis of large-scale experiments. | |
Of course, such sample-by-sample analyses can give rise to errors and may mask subtle changes. An early and still commonly-used solution to this problem is stable isotopes labeling in cell culture (SILAC6,7), a method now extended to multiple model organisms8–10 (including mice,9 although the application of SILAC to mammals is far from routine) (Fig. 1B). In this system, one set of cells/organisms is grown or fed in media derived from isotope-labeled amino acids (usually arginine and lysine), and another is grown or fed in normal media. Critically, the ionization properties and chromatographic retention times of isotopomers are identical, meaning that relative quantitation of each peptide in the two samples can be calculated by the isotope ratio. Thus, protein extracts from the two samples can be pooled together and analyzed as an ensemble to quantify differences in each protocol, using one set as an internal control for another. A “medium” preparation of amino acids is also available, allowing comparison between 3 different conditions simultaneously. For cell biology, this number of samples performed multiple times, may be sufficient. However, for chemical- or synthetic-biology techniques, which tend to have multiple sets of perturbation (e.g., ectopic gene expression, treatment with small-molecule probes, optical triggers, etc., where each aspect needs to be controlled for),2 3 different conditions may not be sufficient. Note also, it is important to validate that isotope incorporation into the proteome of interest is complete (>99% typically), and the use of correct media components is crucial to achieving this.
A solution to the poor multiplexing capability of SILAC, and potentially low % isotope incorporation, for instance, in some organisms, is labeling with isobaric tags, post digestion. Although not perhaps as accurate as using pre-labeled proteins, this worry needs to be offset against the fact that the same (or similarly treated) cells, i.e., non-isotopomeric cells, are compared in the beginning. Moreover, as labeling occurs post harvesting, isobaric tagging can be performed on samples derived from any living systems/tissues/models. Finally, as there are many possible isobaric tags available, tens of samples can be analyzed at the same time, in the same experiment. The two most common methods are iTRAQ11 and TMT12 (Fig. 1C).
There have been several comparisons of these methods in terms of detecting post-translational modifications and specific proteins. Some studies have found that label-free relative to tagging, gives better total coverage and identification rates. Unsurprisingly, some studies claim that label-free is more variable than labeling methods. SILAC labeling is overall higher coverage and is less variable than TMT labeling approaches.13 This variation may be dependent on amount of sample processing,14,15 at least for some labeling approaches. Isobaric tagging also tends to compress the spread of data points observed.16,17 Thus it is important to be mindful of how many samples you will need to compare when planning your experiments. If you can compare 2 or 3 groups, SILAC is probably the best option. If you want to compare more groups or compare across independent replicates as well as across different groups, other methods are overall better. Note: these methods have other benefits that are method-specific which we will outline below.
Getting a feel for it: proteomics for interactome/protein–ligand mapping
Perhaps the most common use of proteomics is to identify protein associations, either with other proteins, or with other ligands (Fig. 2). In these experiments, a protein, referred to as the bait, is enriched, either under non-denaturing or sometimes denaturing conditions, and proteins that bind to the bait (often referred to as prey) are determined. It is important to note that under true denaturing conditions, only covalent associators can be enriched, but under non-denaturing conditions, non-covalent and covalent associators can be identified as interacting with the bait. As enrichment is a critical component of such experiments, separation of the protein of interest (POI) from the bulk of the mixture used, is required. This typically necessitates the use of a resin to catch the POI (functionalized, for instance, with an antibody to the target protein) followed by a series of washing steps prior to analysis. During this procedure, reversible associators with fast off-rates, typically those with high Kd, may be lost from the pool of associators. Reversible associators that bind with slow off-rates, typically those with low Kd (and those that bind covalently, i.e., have zero off-rate), will be retained. Thus, such procedures favor associations that release slowly, effectively those of high affinity. Indeed, many protein–protein interactions are constitutive in unperturbed cells, such as the MALT1–Bcl10 interaction18 and the Keap1–Nrf2 interaction.19 Others are covalent, such as protein ubiquitination,20 phosphorylation,21 and Neddylation,22 or interaction of a protein with an electrophilic drug.23–25 However, in order to respond to stimuli, the cell must be able to rapidly change associations between proteins and ligands. One example is enzyme-substrate interactions; a substrate or product that dissociates slowly from the enzyme is effectively an inhibitor. Others include allosteric activity regulators, chaperones, receptors, etc. These interactions can be weak, often Kd can be on the order of 10's to 100's micromolar. Moreover, it is also appreciated that optimal responsivity occurs when a ligand is present around the Kd, thus proteins regulated allosterically by ligands present at high concentrations in cells (e.g., ubiquitin, actin), likely have high Kds.26
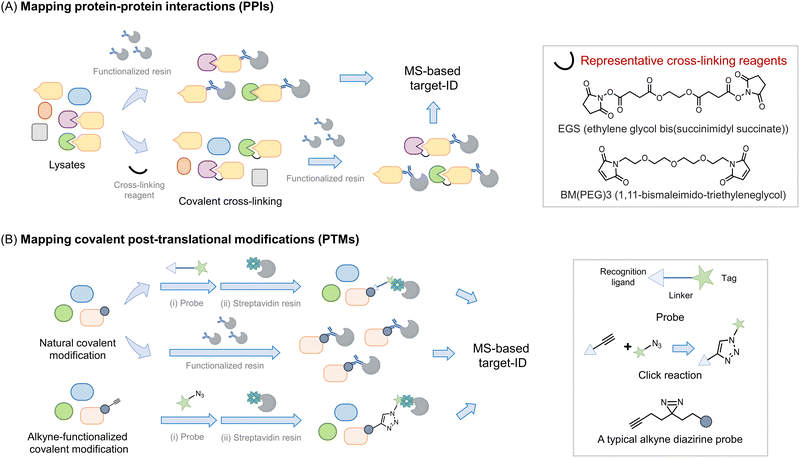 |
| Fig. 2 Introduction of proteomics strategy for interactome and protein–ligand mapping. (A) Proteomics applied to study interactomes. To map the interactome of a target protein of interest (POI, or bait) (in yellow), a functionalized resin, e.g., displaying an antibody specific to the bait protein, is required. The POI-interactome can be enriched by the resin, and analyzed by MS. Given that most transient interactions (mostly those with a high Kd) will likely be lost during washing steps/processing, cross-linking reagents (see inset) may be applied before the enrichment. Cross-linkers usually have groups like N-hydroxysuccinimide or maleimide that target solvent-exposed nucleophilic residues (lysine and cysteine) forming covalent bonds between proximal interacting proteins, typically within the intermolecular distance spanned by the given cross-linker. (B) Proteomics is widely used in mapping covalent post-translational modifications. For numerous PTMs, if resins functionalized with either a PTM-specific antibody or PTM-specific reactive chemical entity are available, allowing the PTM to be enriched and proteins so modified to be analyzed by MS. Alternatively, a chemically functional probe can be introduced to identify such PTMs. Usually, such probes are bifunctional housing a chemical entity reactive to the PTM on one end, and a handle, at the other hand for detection or enrichment, with the linker in between.34,35 [Note: A two-step procedure involving azide and alkyne coupling (Click reaction) is also often deployed as opposed to a bifunctional probe with the linker.36,37 Probes that incorporate photo-crosslinking groups (e.g., diazirine) provide added value by allowing interactome mapping beyond the enrichment of specific PTM-bearing proteins.38,39] | |
To favor identification of transient associations, chemical procedures that trap bound states have been deployed. The first of these was protein cross-linking27 (Fig. 2A). This procedure can identify self-associations, such as aggregation states,28,29 but can also aid identification of protein–protein, and potentially, protein–ligand interactions in proteomics workflows.27 In this method, a bifunctional ligand is added, ideally to cells, but often to lysates and proteins that are within the distance of the length of two ends of the linker are trapped out. Several different chemical functions can be used (acyl halide, N-hydroxysuccinimide, enone), targeting specific residues (cysteine, lysine, and cysteine, respectively). Methods such as this lack temporal control, as the cross-linker is constantly reactive. To surmount this issue, photocaged crosslinking has been applied. This is most commonly applied to situations where a ligand is allowed to bind to its target, then a photocaged group is sprung into action to label proteins with which the molecule associates. Binding the target could be engendered, for instance, through a specific protein–ligand interaction, or through the use of a target protein fused to a reactive domain, such as SNAP30 or Halo.31 However, single photocaged non-specific crosslinkers32 and bifunctional crosslinkers also exist. One critical parameter to consider in photocaging is the wavelength of light used: generally, the further blue (or better red)-shifted the light, the less invasive the uncaging procedure. Although light of low-powered light at 360 nm (5 mW cm−2), over a brief period (minutes) has a relatively limited impact on numerous cellular processes. Linker length can also be changed, modifying the distance covered by the cross-linker. As elution from affinity resins, particularly streptavidin can require harsh conditions, can be difficult to perform completely, cleavable linkers are also available.
All the above experiments are compatible with the different MS methods outlined above. Typically for all the above treatments, it is critical to have control samples, i.e. samples treated without a crosslinking molecule, or an analog of the ligand that does not undergo crosslinking. These can be run as different isotopomers, with different isobaric tags, or as a separate run in LFQ. Of course such samples cannot account for non-specific or artifactual results borne from the systems used, and again it is for that purpose that phenotypic experiments are vital. Some effort has been input to identify common generic targets/preferred labeling sites specific cross-linkers.33 However, how relevant these data are to specific systems is, in our opinion, unclear. Moreover, many associations that are real may not be linked to the mechanism of action of a molecule or function of a protein.
From birth to location, beyond association
Other areas where proteomics has proven particularly useful is identifying components of proteomes, particularly nascent or subcellular proteomes. Methods investigating nascent proteomes have leveraged pulse-chase SILAC labeling to compare induced proteomes relative to extant proteins, for instance, post-infection, or stimulus40 (Fig. 3A). Other similar protocols, such as BONCAT41,42 leverage a modifiable amino acid analog that allows nascent proteins to be enriched, increasing signal to noise, and allowing multiplexing abilities to be increased. This method has also been applied to studying cell-specific proteomes in the whole organisms.43
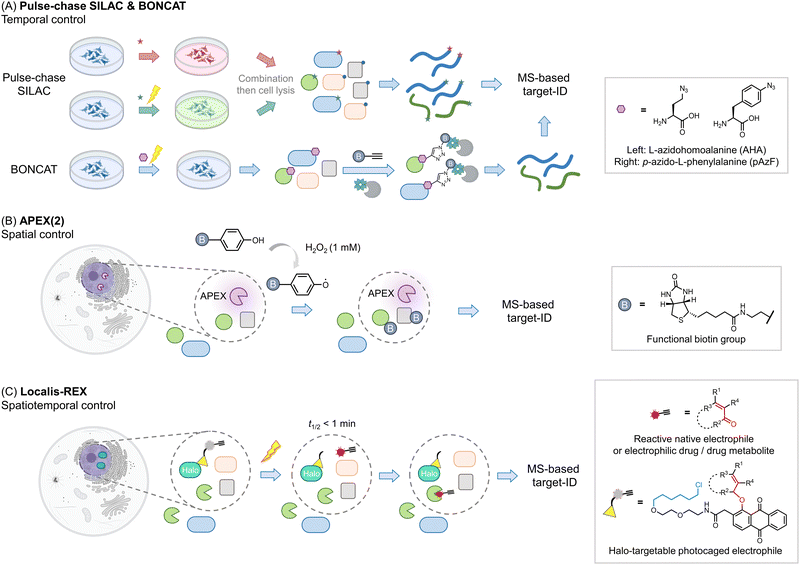 |
| Fig. 3 Beyond the association – get the spatiotemporal resolution. (A) Pulse-chase SILAC enables time-resolved proteomics studies. For example, both groups are cultured in ‘light’ media (blue). At a given point a stimulus is produced (such as antigen priming, stimulation, or drug treatment), then in those samples, the media is changed to ‘heavy’ (red); ‘green’ (medium), and proteins newly synthesized due to the stimulus can be identified.40,63 The media components are not restricted to such order, which can be performed from ‘light’ to ‘heavy’63 or other kinds as needed. In many instances, specific unnatural amino acids are added together with the media change, for enriching the newly-synthesized proteins (combination with BONCAT,41i.e., QuaNCAT64). Based on this strategy, new proteins in a certain period in different conditions can be identified. (B) Proximity-mapping proteomics allows probing protein localization (spatial control), in methods such as APEX (2), Bio-ID, Turbo-ID, μMap, etc. These methods are based on an enzyme and/or small molecule that creates reactive species in a subcellular or protein-specific level under control.45,52 By using a small molecule with a reporter tag that can be identified by mass-spec, a local proteome can be mapped. For example, in APEX (2) method, the engineered ascorbate peroxidase (APEX) generates biotin-phenoxy radical following bulk administration of biotin phenol and 1 mM H2O2.46 The radical species covalently reacts with nucleophilic residues, such as tyrosine, on the surface of proximal proteins within 1 minute.47 If the phenol was replaced by biotin-phenol, the surrounding proteins will be labeled by biotin. (C) G-REX54 and Localis-REX44 constitute spatial and functional proteomics methods to map the electrophile/electrophilic-drug-fragment-responsive local protein targets with spatiotemporal resolution. The REX technology relies on Halotag and a bioinert and cell/animal-permeable photocaged precursor to a reactive electrophile (gray sphere with yellow triangle). Similar to proximity-proteomics strategies, the Halotag can be expressed in specific subcellular locales or tissues in cells/whole animals. At a specific time, exposure to a hand-held lamp [5 mW cm−2; 366 nm] rapidly (t1/2 ∼ 1 min) liberates reactive electrophile. Different from the other spatial-proteomics tools, REX technologies capture only functional protein sensors (in green), namely first responders to reactive electrophiles available locally at close-to-endogenous concentrations. | |
Proteins also undergo important subcellular changes in localization that can have severe implications for their interactomes, functions28,44 and drug mechanisms.45 Several methods have arisen to probe protein localization, including APEX(2),46,47 Bio-ID,48 Turbo-ID,49 μMap,50 and so on (Fig. 3B). These methods use a protein or small molecule that can create reactive species on demand, either in a subcellular or even protein specific level. The reactive species generated covalently tag proximal proteins that can be identified using MS, to create a map of the specific local proteome. Indeed, dependent on the diffusion distance of the generated electrophile, and the timing of the experiment, subtly different aspects of local proteomes can be uncovered.50 As these methods can tag a relatively spatially-defined region,51,52 (which is defined by the half-life of the reactive species liberated), they are also increasingly used to profile protein associations or complex associations. Moreover, these methods also have the ability to catch transient interactions, subcellular-specific, or tissue-specific interactions, among others. Labeling also occurs in cells (or in vivo), meaning that associations captured are more native than those derived from traditional co-IP experiments, which [due to rupture of cell membranes/leaching from different organ(elles)] may allow interactions that typically do not occur to happen. Clearly for proximity labeling methods, such as APEX, to be deployed, a domain, or a small molecule, needs to be brought into proximity of a specific locale or protein. This can be achieved in several ways, all of which are effectively a perturbation to the ground state. We have previously discussed specific experimental nuances of these techniques that lend themselves to particular research problems and discussed using these methods in tandem.2
Beyond protein associations: finding your vocation
One issue with many of the above experiments is that they do not deal with function in any direct way. It is usually assumed that upregulation of a protein upon a stimulus means such a protein is important for responsivity, or it is assumed that a specific association gives insights into proteins that work together. However, even if this is true, these experiments inform little on how function can be intervened chemically, or even how such a proposed function may come about. Several proteomics methods have been created to identify functions of proteins. Activity-based protein profiling (ABPP) uses the ability of specific protein cysteines (or more recently other reactive residues) to be labeled by a modified iodoacetamide ligand as a metric for activity.53 Should the cysteine (or other residues) in question be changed by the presence of an exogenous ligand or stimulus, the labeling of that cysteine by iodoacetamide will be changed. As this approach leverages covalent engagement of specific cysteines, it is perhaps best applied to covalent labeling events, either by native, or unnatural ligands. However, it can also be applied to non-covalent interactions. Of course, loss of signal approaches engender potential false positive outcomes, and these labeling events are not directly linked to protein function. Thus, other methods, that can also be carried out in sub-proteome-specific manners, such as G-REX and Localis-REX have also been developed44,54 (Fig. 3C). These methods release a very small amount of a reactive native or praeternatural electrophile in a specific region of a cell to identify what proteins are reactive in that vicinity under native conditions. The electrophiles are modifiable by biotin using click chemistry, allowing targeted proteins to be enriched. Hits from REX methods can be used as the basis for native reactive metabolite signaling, drug design,55 or as molecular probes. Several studies for instance have shown that endogenous proteins identified by G-REX/Localis-REX as protein sensors of specific electrophiles are functionally changed upon ligand engagement, even when that ligand is present at endogenous concentrations.24,44,56–60 Many of these processes occur through gain of function.
Validation
Your proteomics experiment(s) are likely to generate a relatively large amount of potential candidate proteins (Fig. 4A). Ideally, some of the hits will overlap with previously-published literature data. Such results are indicative of a successful experiment, and can be used as positive controls for validation experiments. Of course, it is also likely that there will be new proteins identified. Dependent on the nature of the experiment used, and the stringency of the thresholds used to cut off “hits” from background, the number of these proteins could range from a handful to dozens, or more. Triaging these hits can be made based on several parameters, such as confidence levels, putative players in (a) pathway(s) of interest, and homology to known interactors. For typical proteomic screens that use a fold change in enrichment, the link between absolute values and likelihood of success is not so clear cut: fold change is a function of binding and non-specific binding, folding, etc. For screens that give absolute occupancy, such as ABPP, hit data are on the surface more clearly interpretable: a certain proportion of the protein was blocked by the addition of a specific small molecule or other procedure. Nevertheless particularly for methods that are absolute, but use a loss of signal, confounding outputs are possible. Such observations could be due to degradation, unfolding, oxidation, and so on all of which would provide a loss of signal, but may not be directly linked to engagement as predicted. Moreover, it is becoming clear that many processes, such as electrophile signaling, are regulated at the subcellular level, meaning that total occupancy obtainable may not be 100%,61,62 or fractional modification is sufficient to trigger signaling.
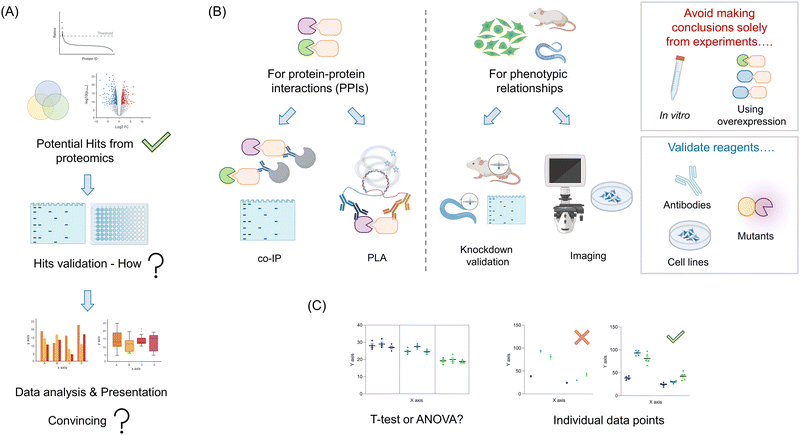 |
| Fig. 4 Validate your hits. (A) A relatively large number of potential candidate proteins is normally found in proteomics studies. Depending on the goal of the experiment, the identified proteins can be shown either in a volcano plot, ID-ratio plot, or Venn diagram. By applying a specific threshold, several to dozens of ‘significant’ hits will remain post filter. To answer specific biological questions, hits validation and corresponding data analysis are necessary. (B) Before doing any kind of functional validation, the reagents, including antibodies and cell lines, as well as the mutants used in the study require careful and correct validation. For validating PPIs, among several options, traditional co-IP or proximity ligation assay (PLA) (the latter has the higher sensitivity) can be used to detect interactions at the endogenous level. For most functional proteomics studies, the goal is to link these hits back to a phenotype. Targeted knockdown/knockout in cells or model organisms, is helpful for validating specific functional binding events. In vitro experiments and overexpression are not recommended for functional study. (C) Finally, when analyzing and presenting the quantified data, robust analysis requires choosing the correct type of statistical treatment. For example, ANOVA-based analysis is necessary when multiple variable experiments are performed. Showing all individual data points in graphs and describing the number of biological and technical replicates also represents robust data processing. | |
As we noted above, the principal goal of a proteomics experiment should be to understand a phenotypically-relevant process. The proteomics experiment itself could be leveraged in a bid to find a mechanism for an observed phenotype, for instance, observed in knockout versus a control line, or drug versus vehicle-treated cells. Otherwise, the goal could be simply to inform on what the function of a specific protein is, which can later inform phenotypic investigations. Whatever the ultimate goal(s) be, these should focus on understanding specific processes that occur in a living system (minimally cells, or ideally model organisms/humans). Thus, our recommendation is to tailor validation experiments to be executed under as biologically-relevant conditions as possible: avoid in vitro experiments, aside from calculating relevant parameters, such as Ki, Kd, kinact/Ki, etc. Moreover, experiments heavily leveraging overexpression should also be avoided (Fig. 4B). These can force interactions that do not happen typically, (and/or suppress those that do), be it with an inhibitor or with another protein. Thus, despite being widely leveraged in the literature, we will not discuss such methods here, as we deem them inadequate to answer relevant biological questions.
Validation of protein–protein interactions (PPIs)
There exist now several options in this vein. For hits derived from traditional immunoprecipitation (IP) or proximity-mapping experiments, co-IP is arguably the most commonly executed (Fig. 4B). Despite being a gold standard, these experiments are heavily dependent upon the specificity of antibodies, expression, and also the stability of the interactions post cell/tissue lysis. We briefly discuss methods to validate antibodies below, although application of several antibodies achieving a positive result, relative to other control antibodies achieving a negative output, provides strong evidence in itself. Conversely, loss of the interacting partner when performing “co-IP” in a knockout of the bait protein, is also good evidence that the interaction is specific. The most common problem that occurs when performing co-IP experiments, bar low specificity of antibodies, and appearance of heavy and light chains of the IP-ing antibody in western blots,28 is low expression making detection of the interaction difficult. The low expression may be solved using overexpression, but as discussed above, this is not ideal. One alternative is to knockout the endogenous protein, and express a tagged version of the protein at endogenous levels using lentiviral transduction, followed by sorting to find cells expressing close to endogenous levels of the tagged protein. This process can equally be applied to mutant versions of the protein proposed to be unable to associate with the target. We have deployed such a technique recently.44 Several work arounds have been published to allow weak interactions to be detected in endogenous proteins. Perhaps the most topical method is proximity ligation assay (PLA).65 PLA can work for a variety of endogenous PPIs, providing species-orthogonal antibodies that can be found for the two partners that are specific (see validation below). Other examples include cross-linking, chemical labeling, FRET, or potentially in vitro analysis, although that once again does not show that interaction is possible in a biologically-relevant system.
Validation that a specific protein candidate is involved in a phenotype
Assuming that the protein/drug that you chose to investigate gives a phenotype, for instance, malignant transformation or toxicity, regardless of interaction validation, it is critical to investigate whether your identified interaction/translocation contributes to a specific phenotype. Note: an association/translocation/modification change does not prove that a particular interaction is biologically relevant, and it certainly does not prove that it is relevant to your intended phenotype.
One simple method to validate the importance of one's identified interactor is to show a change in phenotypic outputs in knockdown/knockout lines.24 Expected outcomes in this manifold depend on how the interaction functions: for an inhibitory interaction, an augmented phenotype is expected; for stimulatory interactions, the diminution is expected. Of course, such a relationship does not prove that the two proteins interact, nor that they function in the same pathway. To investigate this further, knockout, or perhaps more informatively partial knockdown of the two proteins, compared against single knockdowns, is most informative. Essentially, synergy in the double knockout/knockdown system is indicative of genetic interaction, consistent with the MS data. More insight can be determined through the use of point mutants of the interactor that retain function but lose association. These can be used to validate that a “direct” interaction occurs, and that that interaction is necessary for the phenotype. The same principles should be applied to validate protein–ligand interactions.
We have investigated the effects of translocation using locale-specific tagging.28,66 These molecular zip codes can be incorporated into the primary sequence of a specific protein and can overwhelm endogenous localization tags. Upregulation of a phenotype in such lines is an indication that translocation is a trigger for the specific phenotype. Knockdown of the protein in combination with a stimulus that initiates translocation can further be used to investigate these mechanisms.
The considerations above have also been applied to reactive molecule signaling, particularly in the domain of REX technologies.54 Therein we have decoded a residue-specific, locale-specific electrophile signaling in CDK9. For instance, CDK9 bearing a nuclear-localization sequence (NLS) tag did not sense electrophiles: CDK9 bearing a nuclear-exclusion sequence (NES) tag was permissive to electrophile modification. However, only CDK9 that was present in the cytosol (electrophile-sensing competent), but could also translocate to the nucleus (pathway signaling competent) could affect transcription. This mechanism appears to function at the endogenous level.44
Validation of reagents
As we have reviewed over the recent years, in complex systems such as cells, and whole organisms, little should be taken for granted.2,3,62 Thus, it is critical that all reagents used are correctly validated. Perhaps the most overlooked issue is antibodies (Fig. 4B). Each antibody used for an intended protein should be validated in the particular assay you intend to use it dose specificity. If this is imaging, loss of the signal in fixed cells due to the primary antibody should be shown in several knockout or knockdown lines relative to controls. In western blots and IP, ditto. Note: the lines generated in this validation step are also useful for phenotype experiments and as negative controls in, for instance, IP experiments. Hence, this is by no means a wasted exercise.
As noted above, several methods, APEX(2), Bio-ID, and REX technologies require introduction of an ectopic domain or fusion of an ectopic domain to a protein of interest. It is critical that these, and all mutants created (such as point mutants) be examined for perturbation of normal cellular function [proliferation, signaling properties (not) related to the specific process investigated, canonical activity, structure, etc.] These experiments should be performed in the line(s) to be tested, and further investigated in KO lines reconstituted with the fusion protein of interest where needed. However, in vitro experiments for folding, and activity can also be useful.
Cell lines are commonly used in chemical biology experiments. It is important to note that such lines can contain mutations and duplications that render their biological functions distinct from those of normal proteins or cells. Thus, it is often advisable to sequence specific genes that are investigated to ensure that they are normal.57 This issue can be assuaged by working with multiple lines, or by using primary lines/model organisms, although these options may not be available to all laboratories and in all circumstances.
Data analysis and presentation
As seen above, methods of validation can vary, from directly validating MS data (for instance, showing PPIs) to addressing directly if a specific protein from the screen changes a phenotype. Although there are exceptions, such as in vitro binding or inhibition assays, all data analysis will need to be carried out on multiple independent data points. These are usually best analyzed as an ensemble. The typical method to distinguish differences between specific conditions used in many papers is a t-test. This test is formally used to determine if there is a difference between two different data points that exist independently of others. However, when analyzing lead data from proteomic screens, such as how knockdown of 6 different pathway players affects signaling, t-test analysis is not robust enough (Fig. 4C). In this instance, the use of ANOVA to assign whether there be differences across the whole data set, followed by a post hoc test that accounts for numerous comparisons is typical. There have been papers proposing that in several instances the ANOVA test can be ignored, as post hoc tests may have higher statistical power. However, two-step, decision tree-based approaches remain arguably the standard analysis regimens. Regardless, considerations of using an appropriate test are not only applicable to experiments where multiple different players are assayed, but also in follow-up experiments, where, in cultured cells especially, it is correct to deploy multiple siRNAs or CRISPR-gRNAs targeting the same gene. Although it is common in some fields to use monoclonal lines for such experiments, these are not suggested. This is because variations across clones can be significant. Indeed, typically strong knockdown or knockout is achievable in polyclonal populations if sufficient siRNAs or gRNA are tested. Moreover, testing multiple clones of the same shRNA or gRNA does little to assuage the worry of off-target effects impacted by the particular shRNA or gRNA. The ANOVA-based analysis is equally necessary when multiple variable experiments are performed.
Moreover, experiments that use a stimulus, or seek to measure other time-dependent effects also require careful consideration. For instance, it is not acceptable to measure association as a function of time post a stimulus, by performing t-tests between each point, as is often presented. In this case, fitting, or ANOVA/post hoc tests are more insightful. It is preferable to show in a table, or graphic form, how your statistical analyses were performed. All equations used for fitting should also be clearly stated, and residuals to fits should be presented. Finally, it is important to show all individual points in graphs and describe the number of biological and technical replicates.
Conclusions
The above considerations hopefully show that despite being commonly performed, there are many factors to bear in mind when planning proteomics experiments. Aside from choosing the correct system(s) to perform the experiments, practical considerations are particularly relevant and often not well considered before the outset. We hope this primer will be of some use to aid planning and move the focus more to validation of experimental data in relevant and informative systems.
Author contributions
Manuscript drafts (M. J. C. L., Y. A.). Figures and figure legends (J. L.). All authors proofread and agree to the final version of the manuscript.
Conflicts of interest
Small-molecule inhibitors derived from applications of REX technologies have been filed for patent.
Acknowledgements
We acknowledge Swiss National Science Funding (SNSF) (Project 310030_184729), and Swiss Federal Institute of Technology Lausanne (EPFL) (Y. A.).
References
- S. Nurk, S. Koren, A. Rhie, M. Rautiainen, A. V. Bzikadze, A. Mikheenko, M. R. Vollger, N. Altemose, L. Uralsky, A. Gershman, S. Aganezov, S. J. Hoyt, M. Diekhans, G. A. Logsdon, M. Alonge, S. E. Antonarakis, M. Borchers, G. G. Bouffard, S. Y. Brooks, G. V. Caldas, N. C. Chen, H. Cheng, C. S. Chin, W. Chow, L. G. de Lima, P. C. Dishuck, R. Durbin, T. Dvorkina, I. T. Fiddes, G. Formenti, R. S. Fulton, A. Fungtammasan, E. Garrison, P. G. S. Grady, T. A. Graves-Lindsay, I. M. Hall, N. F. Hansen, G. A. Hartley, M. Haukness, K. Howe, M. W. Hunkapiller, C. Jain, M. Jain, E. D. Jarvis, P. Kerpedjiev, M. Kirsche, M. Kolmogorov, J. Korlach, M. Kremitzki, H. Li, V. V. Maduro, T. Marschall, A. M. McCartney, J. McDaniel, D. E. Miller, J. C. Mullikin, E. W. Myers, N. D. Olson, B. Paten, P. Peluso, P. A. Pevzner, D. Porubsky, T. Potapova, E. I. Rogaev, J. A. Rosenfeld, S. L. Salzberg, V. A. Schneider, F. J. Sedlazeck, K. Shafin, C. J. Shew, A. Shumate, Y. Sims, A. F. A. Smit, D. C. Soto, I. Sović, J. M. Storer, A. Streets, B. A. Sullivan, F. Thibaud-Nissen, J. Torrance, J. Wagner, B. P. Walenz, A. Wenger, J. M. D. Wood, C. Xiao, S. M. Yan, A. C. Young, S. Zarate, U. Surti, R. C. McCoy, M. Y. Dennis, I. A. Alexandrov, J. L. Gerton, R. J. O'Neill, W. Timp, J. M. Zook, M. C. Schatz, E. E. Eichler, K. H. Miga and A. M. Phillippy, Science, 2022, 376, 44–53 CrossRef CAS PubMed.
- M. J. C. Long, M. Assari and Y. Aye, ACS Chem. Biol., 2022, 17, 1285–1292 CrossRef CAS PubMed.
- M. J. C. Long, X. Liu and Y. Aye, Front Chem., 2019, 7, 125 CrossRef CAS PubMed.
- D. P. Donnelly, C. M. Rawlins, C. J. DeHart, L. Fornelli, L. F. Schachner, Z. Lin, J. L. Lippens, K. C. Aluri, R. Sarin, B. Chen, C. Lantz, W. Jung, K. R. Johnson, A. Koller, J. J. Wolff, I. D. G. Campuzano, J. R. Auclair, A. R. Ivanov, J. P. Whitelegge, L. Paša-Tolić, J. Chamot-Rooke, P. O. Danis, L. M. Smith, Y. O. Tsybin, J. A. Loo, Y. Ge, N. L. Kelleher and J. N. Agar, Nat. Methods, 2019, 16, 587–594 CrossRef CAS PubMed.
- W. M. Old, K. Meyer-Arendt, L. Aveline-Wolf, K. G. Pierce, A. Mendoza, J. R. Sevinsky, K. A. Resing and N. G. Ahn, Mol. Cell. Proteomics, 2005, 4, 1487–1502 CrossRef CAS PubMed.
- S.-E. Ong, B. Blagoev, I. Kratchmarova, D. B. Kristensen, H. Steen, A. Pandey and M. Mann, Mol. Cell. Proteomics, 2002, 1, 376–386 CrossRef CAS PubMed.
- B. Blagoev, I. Kratchmarova, S.-E. Ong, M. Nielsen, L. J. Foster and M. Mann, Nat. Biotechnol., 2003, 21, 315–318 CrossRef CAS PubMed.
- M. Larance, A. P. Bailly, E. Pourkarimi, R. T. Hay, G. Buchanan, S. Coulthurst, D. P. Xirodimas, A. Gartner and A. I. Lamond, Nat. Methods, 2011, 8, 849–851 CrossRef CAS PubMed.
- M. Krüger, M. Moser, S. Ussar, I. Thievessen, C. A. Luber, F. Forner, S. Schmidt, S. Zanivan, R. Fässler and M. Mann, Cell, 2008, 134, 353–364 CrossRef PubMed.
- A. Westman-Brinkmalm, A. Abramsson, J. Pannee, C. Gang, M. K. Gustavsson, M. von Otter, K. Blennow, G. Brinkmalm, H. Heumann and H. Zetterberg, J. Proteomics, 2011, 75, 425–434 CrossRef CAS PubMed.
- P. L. Ross, Y. N. Huang, J. N. Marchese, B. Williamson, K. Parker, S. Hattan, N. Khainovski, S. Pillai, S. Dey, S. Daniels, S. Purkayastha, P. Juhasz, S. Martin, M. Bartlet-Jones, F. He, A. Jacobson and D. J. Pappin, Mol. Cell. Proteomics, 2004, 3, 1154–1169 CrossRef CAS PubMed.
- A. Thompson, J. Schäfer, K. Kuhn, S. Kienle, J. Schwarz, G. Schmidt, T. Neumann and C. Hamon, Anal. Chem., 2003, 75, 1895–1904 CrossRef CAS PubMed.
- M. Stepath, B. Zülch, A. Maghnouj, K. Schork, M. Turewicz, M. Eisenacher, S. Hahn, B. Sitek and T. Bracht, J. Proteome Res., 2020, 19, 926–937 CrossRef CAS PubMed.
- Z. Li, R. M. Adams, K. Chourey, G. B. Hurst, R. L. Hettich and C. Pan, J. Proteome Res., 2012, 11, 1582–1590 CrossRef CAS PubMed.
- H. T. Lau, H. W. Suh, M. Golkowski and S. E. Ong, J. Proteome Res., 2014, 13, 4164–4174 CrossRef CAS PubMed.
- S. Y. Ow, M. Salim, J. Noirel, C. Evans, I. Rehman and P. C. Wright, J. Proteome Res., 2009, 8, 5347–5355 CrossRef CAS PubMed.
- N. A. Karp, W. Huber, P. G. Sadowski, P. D. Charles, S. V. Hester and K. S. Lilley, Mol. Cell. Proteomics, 2010, 9, 1885–1897 CrossRef CAS PubMed.
- L. Israël and F. Bornancin, Cell. Mol. Immunol., 2018, 15, 8–11 CrossRef PubMed.
- J. D. Hayes and A. T. Dinkova-Kostova, Trends Biochem. Sci., 2014, 39, 199–218 CrossRef CAS PubMed.
- M. Rape, Nat. Rev. Mol. Cell Biol., 2018, 19, 59–70 CrossRef CAS PubMed.
- J. A. Ubersax and J. E. Ferrell, Jr., Nat. Rev. Mol. Cell Biol., 2007, 8, 530–541 CrossRef CAS PubMed.
- R. I. Enchev, B. A. Schulman and M. Peter, Nat. Rev. Mol. Cell Biol., 2015, 16, 30–44 CrossRef CAS PubMed.
- M. J. C. Long and Y. Aye, Cell Chem. Biol., 2017, 24, 787–800 CrossRef CAS PubMed.
- J. R. Poganik, K. T. Huang, S. Parvez, Y. Zhao, S. Raja, M. J. C. Long and Y. Aye, Nat. Commun., 2021, 12, 5736 CrossRef CAS PubMed.
- J. Singh, R. C. Petter, T. A. Baillie and A. Whitty, Nat. Rev. Drug Discovery, 2011, 10, 307–317 CrossRef CAS PubMed.
- M. J. C. Long, P. Ly and Y. Aye, Subcell. Biochem., 2022, 99, 155–197 Search PubMed.
- S. Lenz, L. R. Sinn, F. J. O'Reilly, L. Fischer, F. Wegner and J. Rappsilber, Nat. Commun., 2021, 12, 3564 CrossRef CAS PubMed.
- Y. Fu, M. J. C. Long, S. Wisitpitthaya, H. Inayat, T. M. Pierpont, I. M. Elsaid, J. C. Bloom, J. Ortega, R. S. Weiss and Y. Aye, Nat. Chem. Biol., 2018, 14, 943–954 CrossRef CAS PubMed.
- Y. Aye, E. J. Brignole, M. J. Long, J. Chittuluru, C. L. Drennan, F. J. Asturias and J. Stubbe, Chem. Biol., 2012, 19, 799–805 CrossRef CAS PubMed.
- D. C. McCutcheon, G. Lee, A. Carlos, J. E. Montgomery and R. E. Moellering, J. Am. Chem. Soc., 2020, 142, 146–153 CrossRef CAS PubMed.
- P. K. Mishra, M.-G. Kang, H. Lee, S. Kim, S. Choi, N. Sharma, C.-M. Park, J. Ko, C. Lee, J. K. Seo and H.-W. Rhee, Chem. Sci., 2022, 13, 955–966 RSC.
- J. Liu, L. Cai, W. Sun, R. Cheng, N. Wang, L. Jin, S. Rozovsky, I. B. Seiple and L. Wang, Angew. Chem., Int. Ed., 2019, 58, 18839–18843 CrossRef CAS PubMed.
- A. V. West, G. Muncipinto, H.-Y. Wu, A. C. Huang, M. T. Labenski, L. H. Jones and C. M. Woo, J. Am. Chem. Soc., 2021, 143, 6691–6700 CrossRef CAS PubMed.
- S. G. Codreanu, H. Y. Kim, N. A. Porter and D. C. Liebler, Methods Mol. Biol., 2012, 803, 77–95 CrossRef CAS PubMed.
- B. Emenike, O. Nwajiobi and M. Raj, Front. Chem., 2022, 10, 868773 CrossRef CAS PubMed.
- C. G. Parker and M. R. Pratt, Cell, 2020, 180, 605–632 CrossRef CAS PubMed.
- Y. Chen, Y. Liu, T. Lan, W. Qin, Y. Zhu, K. Qin, J. Gao, H. Wang, X. Hou, N. Chen, J. P. Friedmann Angeli, M. Conrad and C. Wang, J. Am. Chem. Soc., 2018, 140, 4712–4720 CrossRef CAS PubMed.
- X. Li, E. A. Foley, K. R. Molloy, Y. Li, B. T. Chait and T. M. Kapoor, J. Am. Chem. Soc., 2012, 134, 1982–1985 CrossRef CAS PubMed.
- J. Lin, X. Bao and X. D. Li, Mol. Cell, 2021, 81, 2669–2681.e2669 CrossRef CAS PubMed.
- I. Fierro-Monti, J. Racle, C. Hernandez, P. Waridel, V. Hatzimanikatis and M. Quadroni, PLoS One, 2013, 8, e80423 CrossRef PubMed.
- D. C. Dieterich, A. J. Link, J. Graumann, D. A. Tirrell and E. M. Schuman, Proc. Natl. Acad. Sci. U. S. A., 2006, 103, 9482–9487 CrossRef CAS PubMed.
- W. S. Glenn, S. E. Stone, S. H. Ho, M. J. Sweredoski, A. Moradian, S. Hess, J. Bailey-Serres and D. A. Tirrell, Plant Physiol., 2017, 173, 1543–1553 CrossRef CAS PubMed.
- K. P. Yuet, M. K. Doma, J. T. Ngo, M. J. Sweredoski, R. L. Graham, A. Moradian, S. Hess, E. M. Schuman, P. W. Sternberg and D. A. Tirrell, Proc. Natl. Acad. Sci. U. S. A., 2015, 112, 2705–2710 CrossRef CAS PubMed.
- Y. Zhao, P. A. Miranda Herrera, D. Chang, R. Hamelin, M. J. C. Long and Y. Aye, Proc. Natl. Acad. Sci. U. S. A., 2022, 119(5), e2120687119 CrossRef CAS PubMed.
- M. J. C. Long, Y. Zhao and Y. Aye, RSC Chem. Biol., 2020, 1, 42–55 RSC.
- H. W. Rhee, P. Zou, N. D. Udeshi, J. D. Martell, V. K. Mootha, S. A. Carr and A. Y. Ting, Science, 2013, 339, 1328–1331 CrossRef CAS PubMed.
- S. S. Lam, J. D. Martell, K. J. Kamer, T. J. Deerinck, M. H. Ellisman, V. K. Mootha and A. Y. Ting, Nat. Methods, 2015, 12, 51–54 CrossRef CAS PubMed.
- K. J. Roux, D. I. Kim, M. Raida and B. Burke, J. Cell Biol., 2012, 196, 801–810 CrossRef CAS PubMed.
- T. C. Branon, J. A. Bosch, A. D. Sanchez, N. D. Udeshi, T. Svinkina, S. A. Carr, J. L. Feldman, N. Perrimon and A. Y. Ting, Nat. Biotechnol., 2018, 36, 880–887 CrossRef CAS PubMed.
- J. V. Oakley, B. F. Buksh, D. F. Fernández, D. G. Oblinsky, C. P. Seath, J. B. Geri, G. D. Scholes and D. W. C. MacMillan, Proc. Natl. Acad. Sci. U. S. A., 2022, 119, e2203027119 CrossRef CAS PubMed.
- Y. Aye, Chimia, 2022, 76, 598 CrossRef CAS.
- C. P. Seath, A. D. Trowbridge, T. W. Muir and D. W. C. MacMillan, Chem. Soc. Rev., 2021, 50, 2911–2926 RSC.
- C. Wang, E. Weerapana, M. M. Blewett and B. F. Cravatt, Nat. Methods, 2014, 11, 79–85 CrossRef CAS PubMed.
- M. J. C. Long, C. Rogg and Y. Aye, Acc. Chem. Res., 2021, 54, 618–631 CrossRef CAS PubMed.
- X. Liu, M. J. C. Long, B. D. Hopkins, C. Luo, L. Wang and Y. Aye, ACS Cent. Sci., 2020, 6, 892–902 CrossRef CAS PubMed.
- S. Parvez, Y. Fu, J. Li, M. J. Long, H. Y. Lin, D. K. Lee, G. S. Hu and Y. Aye, J. Am. Chem. Soc., 2015, 137, 10–13 CrossRef CAS PubMed.
- M. J. Long, H. Y. Lin, S. Parvez, Y. Zhao, J. R. Poganik, P. Huang and Y. Aye, Cell Chem. Biol., 2017, 24, 944–957.e947 CrossRef CAS PubMed.
- M. J. Long, S. Parvez, Y. Zhao, S. L. Surya, Y. Wang, S. Zhang and Y. Aye, Nat. Chem. Biol., 2017, 13, 333–338 CrossRef CAS PubMed.
- Y. Zhao, M. J. C. Long, Y. Wang, S. Zhang and Y. Aye, ACS Cent. Sci., 2018, 4, 246–259 CrossRef CAS PubMed.
- J. R. Poganik, M. J. C. Long, M. T. Disare, X. Liu, S. H. Chang, T. Hla and Y. Aye, FASEB J., 2019, 33, 14636–14652 CrossRef CAS PubMed.
- S. Parvez, M. J. C. Long, J. R. Poganik and Y. Aye, Chem. Rev., 2018, 118, 8798–8888 CrossRef CAS PubMed.
- X. Liu, M. J. C. Long and Y. Aye, Trends Biochem. Sci., 2019, 44, 75–89 CrossRef CAS PubMed.
- D. F. Bogenhagen and J. D. Haley, J. Biol. Chem., 2020, 295, 2544–2554 CrossRef CAS PubMed.
- A. J. M. Howden, V. Geoghegan, K. Katsch, G. Efstathiou, B. Bhushan, O. Boutureira, B. Thomas, D. C. Trudgian, B. M. Kessler, D. C. Dieterich, B. G. Davis and O. Acuto, Nat. Methods, 2013, 10, 343–346 CrossRef CAS PubMed.
- S. Fredriksson, M. Gullberg, J. Jarvius, C. Olsson, K. Pietras, S. M. Gústafsdóttir, A. Ostman and U. Landegren, Nat. Biotechnol., 2002, 20, 473–477 CrossRef CAS PubMed.
- M. J. C. Long, Y. Zhao and Y. Aye, Cell Chem. Biol., 2020, 27, 122–133.e125 CrossRef CAS PubMed.
|
This journal is © The Royal Society of Chemistry 2023 |
Click here to see how this site uses Cookies. View our privacy policy here.