DOI:
10.1039/D3CC05006H
(Feature Article)
Chem. Commun., 2023,
59, 14745-14758
Biohybrid neural interfaces: improving the biological integration of neural implants
Received
11th October 2023
, Accepted 10th November 2023
First published on 20th November 2023
Abstract
Implantable neural interfaces (NIs) have emerged in the clinic as outstanding tools for the management of a variety of neurological conditions caused by trauma or disease. However, the foreign body reaction triggered upon implantation remains one of the major challenges hindering the safety and longevity of NIs. The integration of tools and principles from biomaterial design and tissue engineering has been investigated as a promising strategy to develop NIs with enhanced functionality and performance. In this Feature Article, we highlight the main bioengineering approaches for the development of biohybrid NIs with an emphasis on relevant device design criteria. Technical and scientific challenges associated with the fabrication and functional assessment of technologies composed of both artificial and biological components are discussed. Lastly, we provide future perspectives related to engineering, regulatory, and neuroethical challenges to be addressed towards the realisation of the promise of biohybrid neurotechnology.
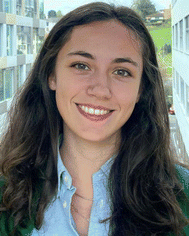
Marjolaine Boulingre
| Marjolaine Boulingre is a PhD student in the Department of Bioengineering at Imperial College London. She received her Master’s degree in Life Sciences Engineering from Ecole Polytechnique Fédérale de Lausanne (EPFL) in 2022. Before joining the Green lab, she conducted her Master Thesis at Harvard School of Engineering and Applied Sciences (SEAS) within the Mooney lab, where she was involved in the development of soft conductive hydrogel scaffold for neural tissue engineering. Her current research interest focuses on developing next-generation bioelectronic devices that integrate cellular components to improve tissue integration. |
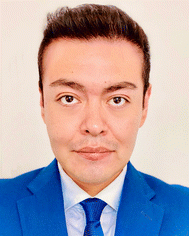
Roberto Portillo Lara
| Dr Roberto Portillo Lara is a biomedical scientist and bioengineer whose research focuses on the development of tissue engineering and regenerative medicine technologies for theragnostic applications. Throughout his career, he has been involved in variety of projects with a strong translational component, ranging from engineering smart wound dressings, surgical bioadhesives and systems for controlled drug release, to cardiovascular, orthopaedic, and musculoskeletal tissue analogues. At Imperial College, he leads the “Living Electrode” project, a multi-disciplinary effort aimed at developing tissue-engineered neural interfaces that could be biologically integrated into the host in order to drive the next generation of implantable bioelectronics. |
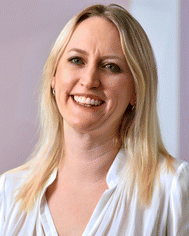
Rylie A Green
| Prof Rylie Green, FIET, is the Head of Bioengineering at Imperial College London. Prof. Green has developed a range of innovative materials to address the limitations that hinder the development of next-generation bioelectronic devices. Her focus has been in developing electrode technologies that are stretchable and mediate improved electrical charge transfer with the body, including pioneering living bioelectronics. She has also developed wearable diagnostic devices and drug delivery systems for localised chemotherapy. This research has initiated collaborations with Galvani Bioelectronics, Cochlear Ltd and the US Department of Defense. She is an Associate Editor for APL Bioengineering, Advanced Bionanomedicine and Biomaterials. |
Introduction
Neural interfaces (NIs) are devices that enable direct communication with the nervous system by leveraging the electrical excitability of nervous tissues to stimulate and/or record neural activity. NIs have been widely implemented in the management of neurological disorders affecting either the central (CNS) or the peripheral nervous systems (PNS).1–6 They have also been extensively used to develop brain–machine interfaces (BMIs) to control neuroprostheses and other assistive devices.7,8 In general, NIs rely on the use of arrays of electrodes that can be implantable or non-implantable.9 Non-implantable electrodes can be used to monitor brain signals from the scalp via electroencephalography (EEG),10 which circumvents the need for surgical implantation but limits spatial and temporal resolution.11 In contrast, implantable devices such as the Utah electrode array (UEA) or electrode leads for deep brain stimulation (DBS) are implanted into the cortex or deep brain structures, providing higher resolution for electrical stimulation (ES) and recording due to the intimate contact with the host tissues.12 Despite these advantages, conventional implantable NIs often undergo poor biological integration into the surrounding tissues, which in turn hinders long-term applications and clinical translation.
The implantation of an electrode array into the neural parenchyma triggers an inflammatory process mediated by the immune system, leading to a biological response known as the foreign body reaction (FBR) (Fig. 1). The initial trauma and the disruption of the blood brain barrier (BBB) result in the release of circulating blood cells and proteins that trigger an acute inflammatory response at the implant site13,14 (Fig. 1A). Early after implantation (0–24 h), migratory neutrophils increase vascular permeability and mediate monocyte recruitment and differentiation into macrophages via soluble pro-inflammatory cytokines. Activated macrophages attempt to phagocytose and degrade the implant, which results in electrode damage,15 increased impedance,16 and overall reduced device performance (Fig. 1C). Resident cells in the CNS such as microglia and astrocytes also become activated and contribute to this acute immune response.17–20 For chronically implanted devices (>4 weeks), the sustained inflammation gives way to a fibrotic process that ultimately leads to the formation of a fibrous scar that encapsulates and isolates the implant21–23 (Fig. 1B). In the context of ES, this physical barrier increases the level of charge injection required to elicit tissue activation, which induces further tissue damage and electrode degradation.24 Local tissue trauma may also be exacerbated due to mechanical mismatches and implant micromotion caused by normal respiratory and cardiovascular functions.25,26 This is mainly because conventional NIs rely on electrode arrays made from stiff and inert materials such as platinum (Pt), platinum–iridium (Pt–Ir) or stainless steel,27,28 which are mechanically distinct from the soft and viscoelastic neural tissues. The perpetuation of local tissue damage further aggravates neuronal depletion leading to a reduction of approximately 40% in the number of viable neurons at the device–tissue interface.29
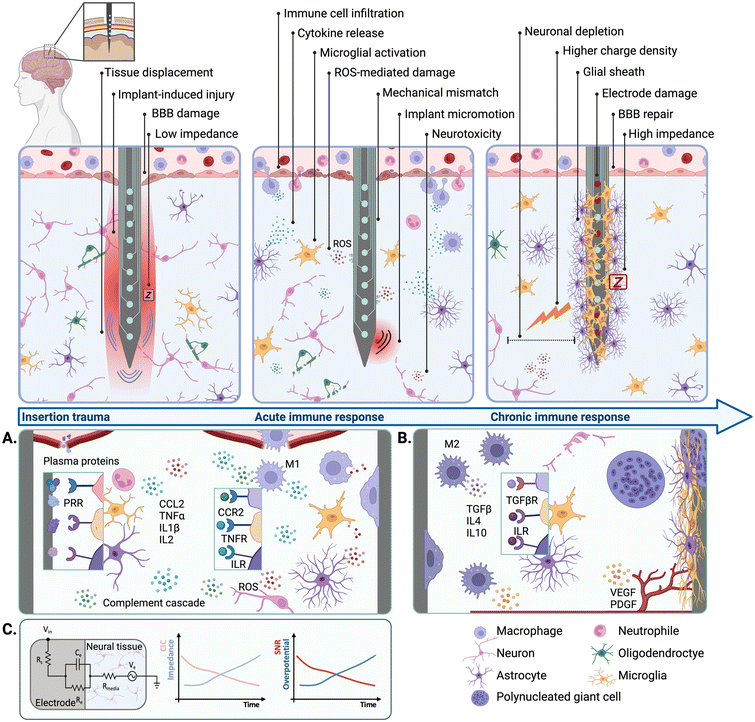 |
| Fig. 1 Tissue responses to neural implants and their impact on device performance. (A) The acute immune response. Upon implantation, plasma proteins adhere to the electrode surface triggering PRR-mediated signalling pathways in microglial cells, astrocytes, and infiltrating neutrophils. Activated cells secrete pro-inflammatory cytokines (TNF, IL1β, IL2) and chemokines (CCL2) increasing BBB permeability, as well as macrophage infiltration, activation, and polarization into an M1 pro-inflammatory phenotype. ROS and the activation of the complement cascade further contribute to neurotoxicity. (B) The chronic immune response. Macrophages polarize into an M2 phenotype and release anti-inflammatory cytokines (TGFβ, IL4, IL10). Astrocytes, microglia, and macrophages fused into multi-nucleated giant cells form a scar that encapsulates the implant. Pro-angiogenic factors such as VEGF and PDGF are involved in the vascularisation of the capsule surrounding the electrode. (C) Electrical model of the electrode–tissue interface. The FBR increases electrode impedance and decreases CSC over time, resulting in reduced SNR and increased risk of overpotential. BBB (blood brain barrier), CCL (chemokine ligand), CCR (chemokine receptor), CSC (charge storage capacity), IL (interleukin), ILR (interleukin receptor), PDGF (platelet-derived growth factor), PRR (patter recognition receptor), ROS (reactive oxygen species), SNR (signal-to-noise ratio), TGFβ (tumour-growth factor β), TGFβR (tumour-growth factor β receptor), TNFα (tumour necrosis factor α), TNFR (tumour necrosis factor receptor), VEGF (vascular endothelial growth factor). | |
Chronic inflammatory responses have been shown to hinder implant biointegration, longevity, and performance, and constitute one of the main limitations precluding the long-term applications of implantable NIs. The large majority of studies describing the negative effects of the FBR on implant performance have relied on research performed on animal models. However, clinical evidence has shown that the long-term implantation of NIs is also associated with the onset of inflammatory and fibrotic responses in human patients. For instance, post-mortem analysis has revealed that a UEA retrieved 7 months after implantation exhibited signs of fibrotic encapsulation and peri-implant tissue damage.30 Electrode tip degradation has also been observed following post-mortem explantation of UEAs after 987 and 182 days, with higher degrees of electrode damage corresponding to longer implantation timelines.15 Therefore, strategies aimed at mitigating the negative effects of the FBR and improving the long-term safety and performance of NIs have gathered significant research interest.
The biomechanical mismatch between soft living tissues and stiff implants is a major factor influencing the degree of the FBR. Therefore, implant design strategies are moving away from conventional stiff materials towards flexible and conformable alternatives based on biomimetic design.31–39 A variety of novel electrode substrates made from elastomers or organic materials combined with conductive polymers (CPs),40 carbon nanotubes (CNTs), or graphene (GF) have been developed for this application.41 Bio-integrative strategies based on surface modification or the incorporation of biomaterial coatings that provide a more biomimetic interface have also been widely explored.37,42,43 Lastly, as the field of NIs continues to evolve, there has been a shift towards alternate modalities for neural interfacing. For instance, neural recording through intracranial blood vessels via endovascular stentrode arrays has emerged as a promising alternative to conventional implantation techniques.44,45
Strategies that improve implant resolution and selectivity are also needed to enhance the discrimination of neuronal activity and to enable the stimulation of specific cells or cell populations. To this end, it has been hypothesised that biohybrid design approaches may be used to engineer high-resolution and chronically-stable bioelectronic interfaces by promoting the integration of implantable devices into the host tissues at the cellular level.46 This feature article will focus on the main experimental strategies in bioelectronics research aimed at engineering the device–tissue interface through the integration of biomaterial- and cell-based strategies. First, the use of biochemical and biophysical cues to guide the fate of endogenous cells at the implant site is discussed. Second, strategies based on biomaterial carriers for the delivery of exogenous cells to mediate implant biointegration are reviewed. Overall, biohybrid approaches may provide safer, longer-lasting, and more efficacious alternatives to conventional NIs by incorporating tools and principles from regenerative medicine and tissue engineering into bioelectronic development.
Modulation of endogenous host cells
Implant surface modification strategies have been explored to instruct the growth and development of endogenous cells that could in turn mediate device biointegration. Moreover, the controlled release of biochemical cues and the delivery of biophysical stimuli has also been explored to elicit specific biological responses towards ameliorating the FBR and enhancing implant longevity and performance.
Biochemical cues: surface functionalisation, bioactive coatings, and controlled release
Inert materials such as Pt or graphite remain the most commonly used electrode substrates for NIs, while polymers such as polyimide (PI) or poly(dimethylsiloxane) (PDMS) are often used for insulation and encapsulation.27 Due to the lack of biological cues, these devices are intrinsically unable to support cell development to establish intimate contact with the host tissues. Because of this, the incorporation of biological cues to guide the fate of endogenous cells upon implantation has been widely investigated in the literature. One approach to present biological cues to endogenous cells relies on the functionalisation of the implant surface with bioactive molecules. For instance, the immobilization of cell adhesion proteins such as laminin47 or neural adhesion protein L148 on the electrode surface has been shown to promote the growth and development of endogenous cells at the interface (Fig. 2A). Neuronal survival and neurogenesis can be promoted via specific growth factors involved in neuroplasticity, such as brain-derived neurotrophic factor (BDNF).49,50 Molecules aimed at reducing microglial activation and gliosis can also be integrated at the interface to ameliorate neurotoxic responses.51,52 Moreover, bioactive patterns can be formed on the electrode surface by alternating cell adhesion inhibitors and cell-adhesive motifs53 to mimic tissue architectures found in vivo. Different strategies to functionalise the electrode surface with bioactive molecules have been reported in the literature. Although physical absorption is a versatile and facile approach, this often results in the formation of weak, non-specific, and reversible non-covalent bonds.54 In contrast, techniques based on chemical absorption such as silane chemistry produce covalent bonds that are comparatively stronger and can be used to graft proteins to different substrates.55 Surface pre-treatment with oxygen plasma has been explored to enhance biomolecule functionalisation by modifying implant hydrophilicity. However, this type of approach could result in unwanted effects such as etching of the underlying materials.53 Alternatively, surface hydrophilicity can be modulated to augment the adhesion of bioactive molecules without compromising implant integrity.56 Surface functionalisation via grafting of bioactive molecules directly to the implant surface enables the preservation of device footprint. However, this strategy is limited by the concentration of molecules that can be grafted, as well as the type of chemistry needed and the surface area available for functionalisation.
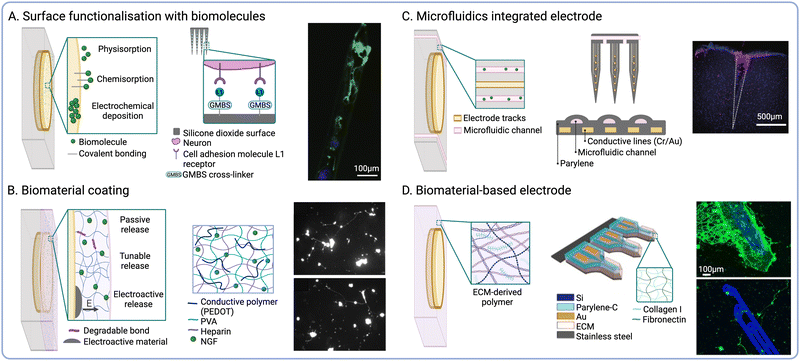 |
| Fig. 2 Biochemical cues to modulate endogenous cell fate. (A) Biomolecule surface functionalisation strategies. Schematic depicting the functionalisation of silicone electrode arrays with neural adhesion molecule L1 (middle) and fluorescence micrograph showing neuronal attachment on L1-coated probes (β-tubulin III is shown in green and cell nuclei in blue) (right) (reproduced with permission from ref. 48). (B) Biomaterial coating strategies for controlled release. Schematic showing PVA-PEDOT CHs biofunctionalized with NGF (middle) and fluorescence micrographs showing neurite outgrowth on CHs with soluble NGF (top) NGF-functionalised CHs (bottom) (reproduced with permission from ref. 57). (C) Microfluidic delivery. Schematic of a flexible penetrating microelectrode arrays with integrated microfluidic structures (middle) and fluorescence micrograph showing local delivery of Dextran Texas Red (red) at the interface (cell nuclei shown in blue) (right) (reproduced with permission from ref. 68). (D) Biomaterial-based electrode constructs. Schematic of ECM-based microelectrodes composed of collagen I and fibronectin (middle) and fluorescence micrograph showing cortical neurons growing on ECM-based electrodes (top), compared to parylene controls (bottom) (β-tubulin III is shown in green and cell nuclei in blue) (reproduced with permission from ref. 69). | |
Another approach to deliver bioactive stimuli to the implant site relies on the encapsulation of soluble cues into biodegradable material-based coatings (Fig. 2B). In this context, hydrogels provide several advantages owing to their high-water uptake capacity and their similarity to the native extracellular matrix (ECM). Hydrogels have been widely employed as reservoirs for the controlled release of neurogenesis-promoting drugs and growth factors, such as BDNF or nerve growth factor (NGF),57 as well as anti-inflammatory drugs such as dexamethasone.58,59 Moreover, biological cues could also be integrated into coatings that enhance the electrochemical performance of electrodes. CPs such as polypyrrole (Ppy) or poly(3,4-ethylenedioxythiophene) (PEDOT) have been functionalised with biological cues such as laminin-derived peptides60 or RGD motifs61 to produce coatings that could tune both charge transduction and cell development at the interface. For instance, Ppy films doped with hyaluronic acid (HA) with high molecular weight were used as electrode coating and exhibited reduced cell attachment.62 Such coating could be used to diminish scar tissue formation. Ppy has also been used in conjunction of polydopamine (PDA) to form conductive biomaterial coating supporting cell adhesion.63 Furthermore, research from our group has shown that the co-incorporation of laminin peptide dopants and neurotrophic growth factor (NGF) into PEDOT-based coatings could effectively promote neurite outgrowth in vitro.64,65 However, this biofunctionalization strategy was also shown to disrupt the PEDOT matrix, rendering it more brittle and subject to delamination.64 To overcome this, our group and others have reported the engineering of interpenetrating conductive hydrogels (CHs) via electrochemical polymerisation of the CP chains using an immobilized dopant.66 CHs hold great potential to develop controlled release systems for the delivery of drugs and other charged molecules via electroactive release.57,58,67 However, non-electroactive hydrogels could also be implemented for this application by fine-tunning the polymer composition, the degree of crosslinking, or the degradation rate of the scaffold. Despite these advantages, hydrogel coatings are susceptible to delamination during implantation, and they can only be loaded with a finite concentration of molecules. Furthermore, hydrogel swelling could significantly increase device footprint, resulting in greater tissue displacement upon implantation.
Despite the advantages of ECM-based coatings, these nanoscale structures are often rapidly biodegraded in vivo, which largely precludes their ability to modulate tissue responses across chronic timeframes. One approach to address this limitation is the integration of microfluidic structures within NIs to enable sustained and prolonged delivery of neurogenic factors or anti-inflammatory drugs68 (Fig. 2C). However, the integration of a microfluidic component could greatly increase the footprint of the device, which increases tissue displacement and compression during implantation. To address this limitation, previous groups have explored the engineering of electrode constructs that are predominantly composed of native ECM components. For instance, a study by Shen et al. reported the fabrication of ECM-encapsulated microelectrodes, which possessed similar dimensions and exhibited comparable performance to conventional silicon-based arrays in vivo. These ECM-based microelectrodes were shown to support the formation of a dense layer of viable neurons on the implant surface, while also undergoing reduced neuroinflammation and glial scarring following chronic implantation in rats69 (Fig. 2D). Furthermore, the ability of biological materials to be effectively biodegraded in vivo could be leveraged to engineer transient bioelectronics that could be cleared from the body after the therapeutic effect has been achieved. This approach could address issues related to chronic tissue damage associated with persistent NIs, while also circumventing the need for surgical retrieval.70,71
Physical cues: implant architecture, surface topography, and device form factor
The physical structure of the ECM provides specific architectures that influence cell migration and differentiation in vivo. Therefore, the recapitulation of biophysical cues found in the native ECM has been explored to influence the fate of endogenous cells at the interface56,72 (Fig. 3A). Based on this biomimetic approach, the modulation of implant surface nano-roughness has been shown to increase neural cell attachment in vitro.73,74 As neurons and glial cells exhibit unique responses to different surface topographies, this strategy has been employed to modulate the neuron-to-glia coverage along the implant surface.75,76 Surface topography could be used to modulate the fate of immune cells such as macrophages by promoting their polarization towards pro- or anti-inflammatory phenotypes.77,78 Furthermore, increasing the electrode surface roughness also increases the active surface area, thus reducing impedance and enhancing the charge storage capacity (CSC).79
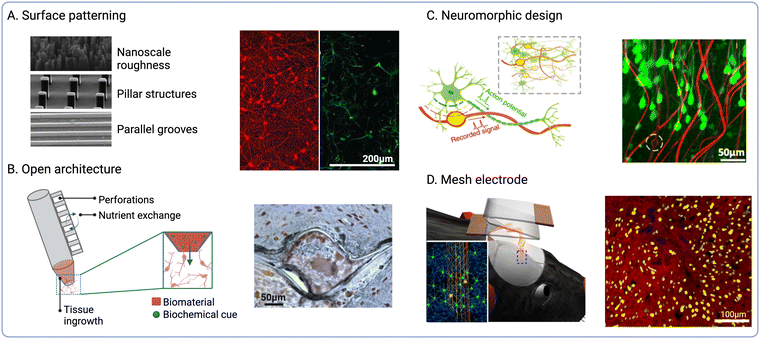 |
| Fig. 3 Biophysical cues to modulate endogenous cell fate. (A) Topographical cues to direct cell adhesion and differentiation (reproduced with permission from ref. 56) (right). Fluorescence micrographs of β-tubulin positive (red) and GFAP positive cells (green) on nanoporous-Au surfaces (left) (adapted with permission from ref. 75 Copyright 2023 American Chemical Society). (B) Open and perforated electrode arrays and immunohistochemical staining showing tissue ingrowth into perforated parylene sheath electrodes (NeuN is shown in brown and GFAP in blue) (reproduced with permission from ref. 84). (C) Schematic of NeuE arrays mimicking subcellular neuronal structures and fluorescence micrograph showing the interaction between NeuE electrodes (red) and neurons (green) (reproduced with permission from ref. 90). (D) Schematic showing the delivery of injectable mesh electronics and fluorescence micrograph of cortical brain slices at 12 weeks post-injection (neurofilaments shown in red and NeuN in yellow, mesh electronics are shown in blue) (reproduced with permission from ref. 98). | |
NIs with novel form factors have been designed to promote tissue integration by incorporating open or perforated device architectures80 (Fig. 3B). For instance, a pioneering study by Kennedy et al. reported the engineering of cone electrodes comprised of an insulated gold wire fixed inside a hollow glass cone.81 These glass structures were loaded with sciatic nerve segments, which promoted tissue ingrowth into the electrodes following intracortical implantation in rats and monkeys.81,82 Although long-term electrophysiological recordings could be obtained for up to 15 months after the implantation of single- and multi-electrode units, the low scalability of this approach greatly limits clinical implementation. More recently, polymeric materials such as parylene C (PaC) have been explored to fabricate electrode arrays using manufacturing techniques such as surface micromachining. For instance, PaC arrays with perforated sheath structures have been shown to improve tissue integration and the exchange of oxygen and nutrients upon implantation.83 These arrays were also shown to produce stable neural recordings for up to 12 months following implantation into the rat motor cortex, while also enabling tissue ingrowth within the perforated structures.84 Open architectures and compliant polymeric materials thus offer the possibility to establish more intimate contact with target cells by guiding tissue ingrowth within the device or reducing tissue damage at the interface. Despite these advantages, the preservation of structural integrity during and after implantation is critical to ensure optimal device performance. In addition, bespoke insertion methods such as shuttle devices35 or transient coatings for probe stiffening are often required to aid the implantation of these types of NIs.85,86
A variety of biomorphic designs that recapitulate tissue architecture and mechanics have been explored to improve the long-term stability of NIs. For instance, the reduction of implant footprint has been shown to diminish macrophage activation and the FBR by minimising tissue damage upon implantation87 (Fig. 3C). Millimetre-sized wireless NIs termed neural dust have been explored for the recording of electrical signals from nerves and muscles.88,89 In another study by Yang et al., nano-scaled bioinspired electrodes were fabricated with recording sites that matched the size of the soma of pyramidal neurons.90 These neuron-like electronics (NeuE) were implanted into mouse brains and produced stable recordings for up to 90 days post-implantation. However, one challenge associated with device miniaturization is the preservation of electrode properties, as reducing the surface area also increases electrode impedance. To overcome this limitation, surface roughening91,92 and electrode coatings93,94 could be employed to increase the surface area and reduce impedance, while also enhancing electrode performance and recording selectivity.
Another strategy to enhance electrode–tissue interaction is the design of mesh or cloud electronics that can spread within and establish intimate contacts with nervous tissues.95 For instance, Liu et al. developed syringe-injectable mesh electronics, which were shown to support cell migration along the mesh when injected into rodent brains96,97 (Fig. 3D). These mesh electrodes were shown to enable long-term stimulation and recording,98 while also resulting in reduced FBR when compared to flexible thin-film probes.99 Another iteration of cloud electrodes was developed based on in situ electro-polymerisation of PEDOT.100,101 PEDOT monomers could be polymerized upon injection to form a polymer cloud that could spread beyond areas affected by neuronal depletion and interact with healthy neurons distal to the implant site.102 The long-term effects of in situ PEDOT deposition were investigated following injection into the hippocampus of rodents,103 which showed no impairment in neural function. Similarly, deployable intracortical electrode arrays have been developed based on liquid crystal elastomers (LCEs), which also enabled recording from viable neurons away from the insertion site and the FBR-induced glial sheath.104
Delivery of exogenous cell effectors
The functionalisation of NIs with live cells has emerged as a promising strategy to engineer biohybrid technologies that better approximate the properties and functionality of biological tissues.105 It has been hypothesised that transplanted cells could establish physiological connections with the host nervous system and mediate implant biointegration at the device–tissue interface. Physiological communication between neurons mainly relies on chemical and electrical synapses.106 While chemical communication is mediated by neurotransmitter release at the synaptic cleft, electrical communication is transmitted by cytoplasmic connections such as gap junctions. It can be hypothesised that mature endogenous cells would secrete factors guiding and directing the neurite extension of transplanted cells. This would mediate the establishment of chemical synapses between endogenous neurons and exogenous neurons.107 Furthermore, because of physical vicinity, cell-to-cell connections could be initiated between the two cell populations, thus mediating electrical communication and promoting neuronal integration.108 This approach may enable more natural mechanisms of neuromodulation,109 which in turn could reduce the risk of faradaic charge injection and the associated tissue damage.110 Different approaches to engineer biohybrid NIs have been reported in the literature,111 including grafting cells directly to the implant surface via immobilised ECM adhesion proteins (Fig. 4A). For instance, Azemi et al., investigated the functionalisation of silicon probes with laminin and murine neural progenitor cells (NPCs) to improve the biointegration of neural implants.55 Their results showed that NPCs on the probes remained viable after implantation into the murine cortex, and that the implants underwent reduced fibrotic encapsulation compared to the controls. A different strategy currently under development by our group relies on the selective engraftment of biotinylated cells to streptavidin-functionalised implant surfaces. This molecular anchoring approach has already been used in the clinic to enhance the endothelialisation of arterial stents following angioplasty112 and to increase neural cell attachment to biomaterial scaffolds.113 Although directly attaching cells to the electrode surface does not increase the footprint of the device, cell viability could be compromised due to shear stress experienced upon implantation.25
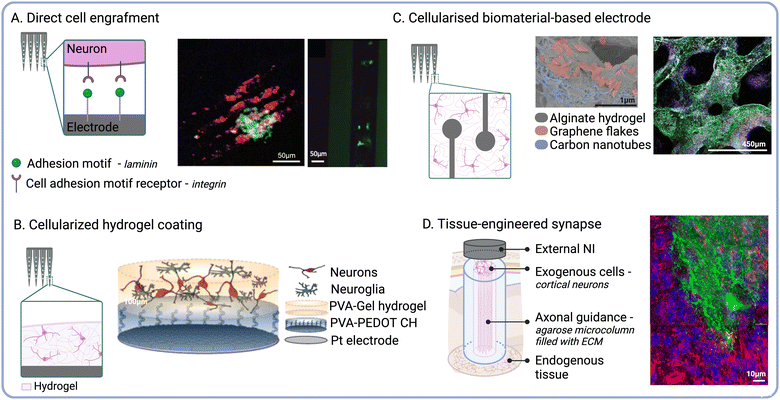 |
| Fig. 4 Delivery of exogenous cells to engineer the device–tissue interface. (A) Schematic showing direct cell engraftment via ECM adhesion proteins. Fluorescence micrographs of GFP-positive NPCs (green) cultured for 14 days on laminin-functionalised probes (autofluorescence in red) (middle) and 1 day after implant retrieval (right) (reproduced with permission from ref. 55). (B) Cell-laden biomaterial-based coatings. Schematic of layered living electrodes developed by Green et al. (reproduced with permission from ref. 116) (right). (C) Cellularised polymeric electrode constructs. SEM micrograph of a porous alginate scaffold embedded with carbon nanomaterials (middle) and fluorescence micrographs of encapsulated NPCs after 6 weeks in culture (Tuj1 shown in green, MBP in white, NPCs in red, and cell nuclei in blue) (right) (reproduced with permission from ref. 185). (D) Schematic of micro tissue-engineered neural networks (μTENN). Fluorescence micrograph of μTENN retrieved 1 month after implantation (GFP shown in green, NF200 in red, synapsin in purple, and cell nuclei in blue) (right) (adapted with permission from ref. 186). | |
Implant micro-displacement due to cardiorespiratory function induces constant mechanical stress and increases the severity of scaring around the implant.25,38 Owing to their tuneable mechanical properties, hydrogel scaffolds can be used to attenuate noxious responses induced by tissue micromotion and mechanical mismatches. Furthermore, hydrogels constitute ideal carriers to enhance the viability of exogenous cells delivered to the device–tissue interface due to their biomimetic properties114 (Fig. 4B). For instance, Faveri et al., reported the development of wire microelectrodes coated with a fibrin hydrogel containing encapsulated neurons or glial cells.115 Their results showed that both cell types were able to develop within the hydrogel, and that the biohybrid coating did not negatively impact the electrochemical performance of the electrodes. Another iteration of biohybrid NIs developed by our group consists of a tissue-engineered layered electrode coating. These living electrodes (LEs) are composed of a CH layer and a cell-laden biosynthetic hydrogel layer formed on top of a Pt electrode substrate.109 The CH enhances the electrochemical properties of the underlying electrode, while the biosynthetic hydrogel enables the combined delivery of relevant bioactive cues to control the fate of the encapsulated cells.116 This approach highlights the versatility and modularity of biomaterial systems for the development of tissue-engineered components that could be incorporated into biohybrid NIs.
Tissue-engineering design criteria for biohybrid NIs
Although neurons constitute the main functional cell type in the nervous system, glial cells such as astrocytes and oligodendrocytes are key modulators of neural function.117–119 Because of this, the engineering of functional neural constructs in vitro has been shown to rely heavily on the contribution of a functional glial component.120,121 For instance, work from our group showed that hydrogel carriers composed of poly(vinyl alcohol) (PVA) co-polymerised with sericin and gelatin (PVA-SG) could effectively support the differentiation of PC-12 cells.122,123 However, the restrictive polymer network failed to support the development of primary astrocytes, which in turn compromised the survival of co-encapsulated murine NPCs.124 These observations highlight the relevance of the cell phenotypes employed and the conditions in which they are maintained in vitro to fabricate complex heterocellular neural constructs. In recent years, patient-derived induced pluripotent stem cells (iPSCs)125 have emerged as a promising resource for personalized tissue-engineered technologies.126–129 As iPSC-derived NPCs have been shown to differentiate into various neural phenotypes,130 they represent an ideal platform to engineer immunocompatible biohybrid NIs. Issues related to the sourcing of cells with limited or aberrant functionality may hinder this strategy in patients affected by trauma or disease.131,132 However, genome editing and cell reprograming technologies may be employed to engineer designer cell phenotypes tailored to specific applications.133,134 Furthermore, bio-instructive materials could be rationally designed to deliver synergistic combinations of physicochemical cues for biofabrication. In this regard, four main design aspects may be considered for the development of tissue-engineered constructs for biohybrid NIs.135
Biological stimuli.
The recapitulation of native cues found in biological tissues through the use of material-based scaffolds is fundamental for neural tissue engineering.136 Although synthetic materials such as PVA or poly(ethylene glycol) (PEG)137 enable precise control over the physical properties of scaffolds, the lack of physiological cues often hinders cell development. To address this, synthetic polymers could be functionalised with ECM adhesion cues, such as the widely used arginine-glycine-aspartic (RGD) motif.138–141 They could also be used in combination with natural polymers such as gelatin and collagen to produce biosynthetic composites with enhanced bioactivity.142,143 The use of biosynthetic materials also enables scaffold remodelling and biodegradation via cell-secreted proteases such as matrix metalloproteinases (MMPs).144 In the context of biohybrid NIs, the precise modulation of scaffold biodegradability is fundamental to support the maturation of tissue-engineered constructs in vitro. This property is also critical to allow endogenous tissue ingrowth to form a chronically-stable interface upon implantation. For instance, a biosynthetic hydrogel system developed by our group has been shown to support the development of primary NPCs into synaptically active neural constructs.145 Moreover, these scaffolds could also support neural tissue ingrowth ex vivo, which highlights the potential of this biomaterial system as a platform to engineer bio-integrative NIs.
Biophysical features.
Scaffold micro-architecture greatly influences the determination of cell fate through the modulation of cell morphology and the promotion of specific gene expression pathways.146 The porosity of the polymer network also determines the movement of nutrients, bioactive factors, and metabolic by-products, as well as the ability of cells to migrate and spread across the scaffold.147 Biomaterial scaffolds with specific fibre alignments and diameters have been shown to guide neurite extension and the differentiation of neural stem cells (NSCs) into neurons.148 In turn, this property may be leveraged to engineer biohybrid NIs with bio-instructive architectures. For instance, Tang-Shomer et al., reported the engineering of a biohybrid NI based on thin and micro-patterned silk films.149 Their results showed that micro-grooves on the films could promote the alignment of astrocytes and nascent neuronal processes, but had no effect on the alignment of mature axons. Therefore, scaffolds with defined biophysical features hold great potential to elicit cell-specific responses without the need for soluble factors. This approach could also reduce manufacturing costs and complexity and overall help facilitate clinical translation.
Mechanical properties.
As multiple cellular pathways are influenced by mechanoresponsive mechanisms, the modulation of scaffold stiffness and viscoelasticity has been explored to guide neural proliferation and differentiation.150,151 Previous studies have shown that soft biomaterial substrates could enhance neuronal maturation and branching.152–156 They have also been shown to support optimal morphological development of encapsulated brain organoids.157 Soft and viscoelastic biomaterials have thus been widely explored to mimic the properties of nervous tissues and enable targeted cell mechanoregulation.158 Hydrogels are remarkably advantageous in this regard, as their mechanical properties can be readily modified by varying the polymer type and concentration, as well as the crosslinking strategy.137 Although soft hydrogels (0.1–10 kPa) have been shown to be more permissive for neuronal culture, glial cells generally require stiffer substrates (0.5–10 kPa) for adequate development in vitro.159 Furthermore, NSCs have been shown to preferentially differentiate into neuronal lineages when cultured on softer substrates (0.1–0.5 kPa), whereas stiffer scaffolds (1–10 kPa) are often conducive to glial differentiation.160 The mechanical properties of biomaterials are also of particular importance when designing biohybrid NIs in order to prevent noxious tissue responses.161 Therefore, there is a need to develop tailorable and multi-functional biomaterials to meet the mechanical requirements associated with different aspects of biohybrid design.
Electrical cues.
Neural cell populations, including neurons, glia, and NSCs have been shown to respond to a wide range of electroconductive substrates and stimulation regimes in vitro.162 Similarly, the intrinsic electrical activity of neurons has been shown to play a role in the maturation of cortical networks in vivo.163–166 Because of this, there has been a growing interest in the development of electroconductive materials for a variety of applications in neural engineering.167,168 Conductive scaffolds could be used to enhance intrinsic neuronal activity and strengthen newly formed synapses without the need for exogenous electrical stimuli.169 Different conductive materials have been explored for this application, including gold nanostructures170,171 and carbon allotropes such as GF and CNTs.167,172 For instance, CNT grids for neuronal culture have been shown to promote the formation of neural networks with enhanced neurite outgrowth and synaptic activity in vitro.173,174 Conductive scaffolds are also ideal substrates to study the effect of exogenous ES on neural growth and differentiation. A study by Koppes et al. demonstrated that ES resulted in enhanced neurite outgrowth in scaffolds loaded with single-walled CNTs, compared to controls without ES.175 Similarly, ES of human NSCs cultured on graphene oxide foams has been shown to promote cell proliferation and neuronal differentiation.176 CPs have also been used to engineer composite scaffolds for neuronal culture177 and have been widely used as coatings to improve electrode performance in biomedical implants.178 Although this approach has been widely explored for the fabrication of CHs, the incorporation of nanomaterials could significantly alter the mechanical properties of hydrogels. Moreover, concerns related to nanotoxicity and the fate of nanomaterials upon scaffold biodegradation remain. Therefore, there is a need to increase our understanding on the pharmacokinetics of nanomaterials and their interactions with proteins and cells in vivo in order to prevent potential neurotoxic effects.
Considerations for biohybrid NI fabrication
The integration of cellular components into the design of implantable NIs could enable the possibility of directly interfacing devices with the nervous system by harnessing intrinsic physiological mechanisms. However, the technological and biological complexity of biohybrid implants raises multiple challenges related to their fabrication, functional characterisation, and clinical translation. One challenging aspect of biohybrid fabrication are the potential compromises between device functionality and the viability of the tissue-engineered components. For instance, while soft biomaterial coatings may promote neuronal survival at the interface and help alleviate the FBR, they are susceptible to swelling and delamination and may be lost upon tissue insertion.14,179 Several studies reported swelling-induced delamination of hydrogels bound to rigid substrates.180,181 CH coating loss was also observed following implantation of CH-coated cochlear implants182 and DBS electrode arrays.183 As the use of stiffer materials that could better withstand implantation could compromise cell development at the interface, alternative strategies may be explored. Multiple techniques are available to enhance the adhesion of biohybrid coatings to electrode substrates, which include surface roughening to increase hydrogel grip115 or chemical pre-treatment to form functional handles.184 For instance, biohybrid LEs developed by our group rely on an electrochemically deposited pre-layer of PEDOT/p-toluenesulfonate (pTS) to enhance the mechanical grip of the CH to the underlying electrode substrate.66
Biohybrid NI design should aim to maximise the synergy between different experimental approaches according to the intended application in order to streamline technology development. For instance, the use of growth factors or cytokines to instruct cell fate for biofabrication may be circumvented by tailoring scaffold mechanics and architecture, or via targeted ES. The neurotrophic support provided by accessory feeder cells could be leveraged to avoid the need for growth factor or cytokine supplementation. Moreover, the delivery of exogenous cell effectors may be bypassed all together in some cases by exploiting instead the capabilities of resident host cells or migratory cells recruited to the implant site. Therefore, biohybrid NIs could be designed to leverage the complexity of the in vivo environment in order to simplify technology development and streamline clinical translation.
As the field of bioelectronics continues to shift towards the use of flexible organic materials for device fabrication, the direct integration of cellular components into the NI structure has been explored (Fig. 4C). For instance, neuron–electrode interfaces composed of silk films co-patterned with cells and electrodes have been used for neural recording and ES in vivo.149 Viscoelastic CHs composed of alginate and carbon allotropes have been shown to support the growth and differentiation of NPCs into both neurons and glia.185 As these CHs exhibited conductivities ranging between 4–10 S m−1, they hold great promise to fabricate implantable NIs for targeted ES. Micro-tissue engineered neural networks (μTENNS) have been engineered based on agarose hydrogel microcolumns filled with collagen and laminin to guide the ingrowth of neural 3D aggregates across the lumen105,186 (Fig. 4D). These tubular constructs may be used to modulate target tissues via synaptic connections established de novo between NIs and the host nervous system.187,188
Pre-clinical evaluation
Technical challenges may be encountered when evaluating the performance of active devices composed of both artificial and biological components. Although in vitro models have been developed to mimic some aspects of chronic implantation,189in vivo models remain the gold standard as they provide the highest physiological relevance. Multiple transgenic models of neurological disorders have also been established,190 which provide more accurate platforms for the assessment of theragnostic NIs. Although post-mortem analysis of explants has been traditionally used for functional evaluation, this usually requires extensive tissue processing and is only representative of discrete endpoints. Non-invasive imaging techniques are therefore required for real-time evaluation of device performance and to monitor tissue responses in vivo. Intravital imaging techniques such as two-photon microscopy191,192 and clinical imaging techniques such as magnetic resonance imaging (MRI) and computed tomography (CT) may be used for this application. These techniques could not only be used to monitor the development of biohybrid implants in situ, but also to assess the degree of biointegration and FBR without the need for implant retrieval.193,194 To achieve this, our group and others have focused on the development of advanced biomaterials for soft bioelectronics that are compatible with clinical imaging techniques such as MRI. Alternatively, emerging neuroimaging techniques based on functional ultrasound have been shown to enable large-scale and high-resolution neural imaging in human patients.195,196 In addition, near-infrared fluorescent (NIR) imaging197 or MRI198 may be used in combination with labelling techniques to monitor the fate of exogenous cells across chronic timeframes.
Electrochemical characterisation via electrochemical impedance spectroscopy (EIS) or cyclic voltammetry (CV) may be carried out to assess electrode performance and the degree of fibrotic encapsulation.199,200 When performed in vivo, faster CV scan rates may be used in order to reduce anaesthesia time, as well as the risk of charge build-up and faradaic damage. It is also important to consider the impact of the stimulation delivered for electrochemical characterisation on the biology of the tissue-engineered components and the host tissues. Moreover, as cellularised constructs are closely integrated with active electrode systems for biohybrid fabrication, it is crucial to better understand the influence of electrical stimuli on the fate of complex neural constructs. To this end, multi-electrode array (MEA) systems have been developed to enable real-time, label-free and high-throughput electrophysiological monitoring in vitro.201 In addition, custom-built devices could be developed to better approximate specific physiological scenarios or to accommodate the requirements of NIs with bespoke formats.202 However, experimental findings from in vitro ES studies remain poorly translatable, which is due in part to the lack of standardised set-ups and protocols. To address this limitation, computational in silico models have been explored to enhance the reproducibility of ES protocols and streamline clinical translation.203
Future perspectives
The realisation of biohybrid NIs that could one day be delivered to human subjects presents multiple engineering hurdles that need to be addressed to ensure implant and patient safety. For instance, the integration of wireless and battery-free capabilities for transcutaneous operation could help ameliorate surgical risks, while also extending device longevity and improving user experience.204,205 Wireless brain–computer interface (BCI) systems such as the one developed by the BrainGate research consortium are already being implemented in the clinic to allow tetraplegic patients to control point-and-select interfaces.206 However, despite the advantages of wireless interfacing, this approach may hinder information transfer rates and raise concerns related to data safety and protection.207 In addition, several neuroscientific questions remain unanswered, such as the long-term effects of neuromodulatory devices on brain function. Adverse effects such as depression, cognitive decline, and personality changes have been reported in Parkinson's Disease patients undergoing DBS.208 Although these effects could be partly attributed to medication or disease progression, the impact of chronic implantation and ES regimes remains to be elucidated. Moreover, if transplanted cells are able to establish synaptic connections with the host, they could potentially disturb endogenous neural activity due to ectopic activation or inhibition. Exogenous stem or progenitor phenotypes could also migrate out of the device and proliferate or differentiate in an uncontrolled way at off-target sites.209 Although conventional neuromodulatory devices may be switched off or surgically removed if needed, the modulation and removal of tissue-engineered components bring forward several challenges in this regard. Strategies derived from regenerative medicine and immunotherapy may be explored to address these issues, such as engineering cells sensitive to custom external stimuli or with a built-in “kill switch”.210 Therefore, there is a need to increase our understanding on the long-term adaptations that biohybrid implants and the host nervous system undergo across chronic timeframes to pre-emptively mitigate any potential risks.
Although biohybrid technologies are still in their infancy, the translation of implantable neurotechnologies is rapidly becoming a reality. This is exemplified by the increasing number or clinical trials approved by the Food and Drug Administration (FDA) that are being carried out by companies such as Synchron and Neuralink. As of 2023, more than 400 clinical trials related to neural interfaces were registered in the Cochrane database. Governments have been involved in the surge for neurotechnology research by launching multiple funding opportunities, such as the BRAIN initiative launched under Obama mandate, or the Stimulating Peripheral Activity to Relieve Conditions (SPARC) common fund. International collaborations have also been established such as the Human Brain Project supported by the European Union. The steady increase in private investment coupled with the rapid growth in artificial intelligence (AI) and machine-learning have greatly accelerated progress in the field of NIs. A growing number of studies have explored the implementation of deep-learning algorithms to enhance the speed and accuracy of speech neuroprostheses driven by implantable BCIs.211,212 However, despite this rapid technological advancement, current regulatory, ethical, and legal frameworks are lacking and should be revisited to adapt to these emerging developments. From the regulatory perspective, new categories of medical devices may need to be defined to capture biohybrid technologies with combined traits of active implants, drugs, and cell transplants. Regulatory agencies have already begun to establish specific guidelines for combination products containing two or more regulated components from different categories.193 Moreover, the standardisation of criteria from different international agencies is crucial to ensure that clear regulatory pathways are in place.
As biohybrid technologies continue to mature, the development of biointegrative NIs that could remain implanted across the entire lifetime of the user raises several ethical concerns related to privacy, responsibility, agency, and identity.213 Neural data recorded by NIs is highly sensitive since it can be used to decode the thoughts, feelings, or emotions of the user. It is therefore essential to ensure secure storage and controlled access to this information to prevent misuse by third parties, such as advertisers, employers or governments.214 Whether it is perceived as dystopic or utopic, the digitalisation of some aspects of the human mind is becoming a reality, and regulatory and legal frameworks must be pre-emptively defined. The increasing use of AI and closed-loop systems that could operate without input from the user raises multiple issues related to agency and autonomy.215 Clinical evidence has shown that ES could influence behaviour and self-determination, which could potentially compromise patient consent.216 DBS implantees have reported alterations in perception and embodiment,217,218 with some users describing NIs becoming a part of themselves.219 Biohybrid NIs also pose several ontological questions that challenge the neuroessentialist view of human identity and consciousness. For instance, neuroethicists have postulated that some form of morally relevant consciousness might develop in complex neural constructs grown in vitro, such as brain organoids or assembloids.220 Moreover, it has been previously postulated that technology could become so utterly intertwined with our cognitive machinery that it could be considered part of the machinery of thought itself.221 Moving forward, these and other metaphysical notions are particularly relevant in the context of biohybrid NIs, as technological progress continues to outpace our fundamental understanding of what it is to be human.
Author contributions
The paper was written by MB with editing by RPL and RG. Funding was obtained by RG.
Conflicts of interest
There are no conflicts to declare.
Acknowledgements
The authors acknowledge funding support from the ERC Consolidator Grant in Living Bionics (771985-1). The figures were created with https://BioRender.com.
Notes and references
- W. H. Bosking, M. S. Beauchamp and D. Yoshor, Annu. Rev. Vision Sci., 2017, 3, 141–166 CrossRef PubMed.
- M. S. Beauchamp, D. Oswalt, P. Sun, B. L. Foster, J. F. Magnotti, S. Niketeghad, N. Pouratian, W. H. Bosking and D. Yoshor, Cell, 2020, 181, 774–783.e5 CrossRef CAS PubMed.
- J. K. Krauss, N. Lipsman, T. Aziz, A. Boutet, P. Brown, J. W. Chang, B. Davidson, W. M. Grill, M. I. Hariz, A. Horn, M. Schulder, A. Mammis, P. A. Tass, J. Volkmann and A. M. Lozano, Nat. Rev. Neurol., 2021, 17, 75–87 CrossRef PubMed.
- P. Ryvlin, S. Rheims, L. J. Hirsch, A. Sokolov and L. Jehi, Lancet Neurol., 2021, 20, 1038–1047 CrossRef PubMed.
- M. Roet, J. Boonstra, E. Sahin, A. E. P. Mulders, A. F. G. Leentjens and A. Jahanshahi, J. Clin. Med., 2020, 9, 2729 CrossRef PubMed.
- B. Kundu, A. A. Brock, D. J. Englot, C. R. Butson and J. D. Rolston, Neurosurg. Focus, 2018, 45, E14 Search PubMed.
- D. A. Moses, S. L. Metzger, J. R. Liu, G. K. Anumanchipalli, J. G. Makin, P. F. Sun, J. Chartier, M. E. Dougherty, P. M. Liu, G. M. Abrams, A. Tu-Chan, K. Ganguly and E. F. Chang, N. Engl. J. Med., 2021, 385, 217–227 CrossRef PubMed.
- F. R. Willett, D. T. Avansino, L. R. Hochberg, J. M. Henderson and K. V. Shenoy, Nature, 2021, 593, 249–254 CrossRef CAS PubMed.
- S. Waldert, Front. Neurosci, 2016, 10, 295 Search PubMed.
-
A. Kilicarslan, S. Prasad, R. G. Grossman and J. L. Contreras-Vidal, in 2013 35th Annual International Conference of the IEEE Engineering in Medicine and Biology Society (EMBC), 2013, pp. 5606–5609.
- B. Gunasekera, T. Saxena, R. Bellamkonda and L. Karumbaiah, ACS Chem. Neurosci., 2015, 6, 68–83 CrossRef CAS PubMed.
- E. M. Maynard, C. T. Nordhausen and R. A. Normann, Electroencephalogr. Clin. Neurophysiol., 1997, 102, 228–239 CrossRef CAS PubMed.
- H. W. Bedell, N. J. Schaub, J. R. Capadona and E. S. Ereifej, Acta Biomater., 2020, 102, 205–219 CrossRef CAS PubMed.
- A. Lecomte, E. Descamps and C. Bergaud, J. Neural Eng., 2018, 15, 031001 CrossRef PubMed.
- K. Woeppel, C. Hughes, A. J. Herrera, J. R. Eles, E. C. Tyler-Kabara, R. A. Gaunt, J. L. Collinger and X. T. Cui, Front. Bioeng. Biotechnol, 2021, 9, 759711 CrossRef PubMed.
- J. C. Williams, J. A. Hippensteel, J. Dilgen, W. Shain and D. R. Kipke, J. Neural Eng., 2007, 4, 410 CrossRef PubMed.
- J. K. Hermann and J. R. Capadona, Crit. Rev. Bioeng., 2018, 46, 341–367 Search PubMed.
- R. D. Dorand, B. L. Benson, L. F. Huang, A. Petrosiute and A. Y. Huang, Front. Neurosci, 2019, 13, 737 CrossRef PubMed.
- C. Bennett, F. Mohammed, A. Álvarez-Ciara, M. A. Nguyen, W. D. Dietrich, S. M. Rajguru, W.
J. Streit and A. Prasad, Biomaterials, 2019, 188, 144–159 CrossRef CAS PubMed.
- J. W. Salatino, K. A. Ludwig, T. D. Y. Kozai and E. K. Purcell, Nat. Biomed. Eng., 2017, 1, 862–877 CrossRef CAS PubMed.
- F. Lotti, F. Ranieri, G. Vadalà, L. Zollo and G. D. Pino, Front. Neurosci, 2017, 11, 497 CrossRef PubMed.
- Y. Chandorkar, K. Ravikumar and B. Basu, ACS Biomater. Sci. Eng., 2019, 5, 19–44 CrossRef CAS PubMed.
- A. Carnicer-Lombarte, S.-T. Chen, G. G. Malliaras and D. G. Barone, Front. Bioeng. Biotechnol., 2021, 9, 622524 CrossRef PubMed.
- L. Poole-Warren, N. Lovell, S. Baek and R. Green, Expert Rev. Med. Devices, 2010, 7, 35–49 CrossRef CAS PubMed.
- D. Prodanov and J. Delbeke, Front. Neurosci, 2016, 10, 11 Search PubMed.
- A. Gilletti and J. Muthuswamy, J. Neural Eng., 2006, 3, 189 CrossRef PubMed.
- C. M. Tringides and D. J. Mooney, Adv. Mater., 2022, 34, 2107207 CrossRef CAS PubMed.
- Y. Wang, X. Yang, X. Zhang, Y. Wang and W. Pei, Microsyst. Nanoeng., 2023, 9, 7 CrossRef PubMed.
- C. H. Thompson, M. J. Zoratti, N. B. Langhals and E. K. Purcell, Tissue Eng., Part B, 2016, 22, 125–135 CrossRef PubMed.
- L. J. Szymanski, S. Kellis, C. Y. Liu, K. T. Jones, R. A. Andersen, D. Commins, B. Lee, D. B. McCreery and C. A. Miller, J. Neural Eng., 2021, 18, 0460b9 CrossRef PubMed.
- S. P. Lacour, G. Courtine and J. Guck, Nat. Rev. Mater., 2016, 1, 1–14 Search PubMed.
- S. M. Won, E. Song, J. Zhao, J. Li, J. Rivnay and J. A. Rogers, Adv. Mater., 2018, 30, 1800534 CrossRef PubMed.
- N. Vachicouras, O. Tarabichi, V. V. Kanumuri, C. M. Tringides, J. Macron, F. Fallegger, Y. Thenaisie, L. Epprecht, S. McInturff, A. A. Qureshi, V. Paggi, M. W. Kuklinski, M. C. Brown, D. J. Lee and S. P. Lacour, Sci. Transl. Med., 2019, 11, eaax9487 CrossRef PubMed.
- C. M. Tringides, N. Vachicouras, I. de Lázaro, H. Wang, A. Trouillet, B. R. Seo, A. Elosegui-Artola, F. Fallegger, Y. Shin, C. Casiraghi, K. Kostarelos, S. P. Lacour and D. J. Mooney, Nat. Nanotechnol., 2021, 16, 1019–1029 CrossRef CAS PubMed.
- L. Luan, X. Wei, Z. Zhao, J. J. Siegel, O. Potnis, C. A. Tuppen, S. Lin, S. Kazmi, R. A. Fowler, S. Holloway, A. K. Dunn, R. A. Chitwood and C. Xie, Sci. Adv., 2017, 3, e1601966 CrossRef PubMed.
- Z. J. Du, C. L. Kolarcik, T. D. Y. Kozai, S. D. Luebben, S. A. Sapp, X. S. Zheng, J. A. Nabity and X. T. Cui, Acta Biomater., 2017, 53, 46–58 CrossRef CAS PubMed.
- D. Shi, V. Dhawan and X. T. Cui, Curr. Opin. Biotechnol, 2021, 72, 54–61 CrossRef CAS PubMed.
- A. Sridharan, J. K. Nguyen, J. R. Capadona and J. Muthuswamy, J. Neural Eng., 2015, 12, 036002 CrossRef PubMed.
- Y. Liu, J. Li, S. Song, J. Kang, Y. Tsao, S. Chen, V. Mottini, K. McConnell, W. Xu, Y.-Q. Zheng, J. B.-H. Tok, P. M. George and Z. Bao, Nat. Biotechnol., 2020, 38, 1031–1036 CrossRef CAS PubMed.
- E. Cuttaz, J. Goding, C. Vallejo-Giraldo, U. Aregueta-Robles, N. Lovell, D. Ghezzi and R. A. Green, Biomater. Sci., 2019, 7, 1372–1385 RSC.
- M. Hejazi, W. Tong, M. R. Ibbotson, S. Prawer and D. J. Garrett, Front. Neurosci, 2021, 15, 658703 CrossRef PubMed.
- A. Golabchi, K. M. Woeppel, X. Li, C. F. Lagenaur and X. T. Cui, Biosens. Bioelectron., 2020, 155, 112096 CrossRef CAS PubMed.
- R. Portillo-Lara, J. A. Goding and R. A. Green, Curr. Opin. Biotechnol, 2021, 72, 62–68 CrossRef CAS PubMed.
- T. J. Oxley, N. L. Opie, S. E. John, G. S. Rind, S. M. Ronayne, T. L. Wheeler, J. W. Judy, A. J. McDonald, A. Dornom, T. J. H. Lovell, C. Steward, D. J. Garrett, B. A. Moffat, E. H. Lui, N. Yassi, B. C. V. Campbell, Y. T. Wong, K. E. Fox, E. S. Nurse, I. E. Bennett, S. H. Bauquier, K. A. Liyanage, N. R. van der Nagel, P. Perucca, A. Ahnood, K. P. Gill, B. Yan, L. Churilov, C. R. French, P. M. Desmond, M. K. Horne, L. Kiers, S. Prawer, S. M. Davis, A. N. Burkitt, P. J. Mitchell, D. B. Grayden, C. N. May and T. J. O’Brien, Nat. Biotechnol., 2016, 34, 320–327 CrossRef CAS PubMed.
- T. J. Oxley, P. E. Yoo, G. S. Rind, S. M. Ronayne, C. M. S. Lee, C. Bird, V. Hampshire, R. P. Sharma, A. Morokoff, D. L. Williams, C. MacIsaac, M. E. Howard, L. Irving, I. Vrljic, C. Williams, S. E. John, F. Weissenborn, M. Dazenko, A. H. Balabanski, D. Friedenberg, A. N. Burkitt, Y. T. Wong, K. J. Drummond, P. Desmond, D. Weber, T. Denison, L. R. Hochberg, S. Mathers, T. J. O’Brien, C. N. May, J. Mocco, D. B. Grayden, B. C. V. Campbell, P. Mitchell and N. L. Opie, J. Neurointerv. Surg., 2021, 13, 102–108 CrossRef PubMed.
- R. A. Green, APL Bioeng., 2021, 5, 040401 CrossRef CAS PubMed.
- G. E. Slaughter, E. Bieberich, G. E. Wnek, K. J. Wynne and A. Guiseppi-Elie, Langmuir, 2004, 20, 7189–7200 CrossRef CAS PubMed.
- E. Azemi, C. F. Lagenaur and X. T. Cui, Biomaterials, 2011, 32, 681–692 CrossRef CAS PubMed.
- T. Numakawa, S. Suzuki, E. Kumamaru, N. Adachi, M. Richards and H. Kunugi, Histol. Histopathol., 2010, 25, 237–258 CAS.
- Y. Jiao, B. Palmgren, E. Novozhilova, U. Englund Johansson, A. L. Spieles-Engemann, A. Kale, S. I. Stupp and P. Olivius, BioMed Res. Int., 2014, 2014, 356415 Search PubMed.
- M. L. Block, L. Zecca and J.-S. Hong, Nat. Rev. Neurosci., 2007, 8, 57–69 CrossRef CAS PubMed.
- J. R. Eles, A. L. Vazquez, N. R. Snyder, C. Lagenaur, M. C. Murphy, T. D. Y. Kozai and X. T. Cui, Biomaterials, 2017, 113, 279–292 CrossRef CAS PubMed.
- J. Slavík, J. Skopalík, I. Provazník and J. Hubálek, Sensors, 2019, 19, 5379 CrossRef PubMed.
- C. Chen, X. Kong and I.-S. Lee, Biomed. Mater., 2015, 11, 014108 CrossRef PubMed.
- E. Azemi, G. T. Gobbel and X. T. Cui, J. Neurosurg., 2010, 113, 673–681 Search PubMed.
- S. Khan and G. Newaz, J. Biomed. Mater. Res., Part A, 2010, 93A, 1209–1224 CrossRef CAS PubMed.
- G. L. Mario Cheong, K. S. Lim, A. Jakubowicz, P. J. Martens, L. A. Poole-Warren and R. A. Green, Acta Biomater., 2014, 10, 1216–1226 CrossRef CAS PubMed.
- R. Wadhwa, C. F. Lagenaur and X. T. Cui, J. Controlled Release, 2006, 110, 531–541 CrossRef CAS PubMed.
- C. Boehler, C. Kleber, N. Martini, Y. Xie, I. Dryg, T. Stieglitz, U. G. Hofmann and M. Asplund, Biomaterials, 2017, 129, 176–187 CrossRef CAS PubMed.
- N. Bhagwat, R. E. Murray, S. I. Shah, K. L. Kiick and D. C. Martin, Acta Biomater., 2016, 41, 235–246 CrossRef CAS PubMed.
- J. A. Chikar, J. L. Hendricks, S. M. Richardson-Burns, Y. Raphael, B. E. Pfingst and D. C. Martin, Biomaterials, 2012, 33, 1982–1990 CrossRef CAS PubMed.
- S. Kim, Y. Jang, M. Jang, A. Lim, J. G. Hardy, H. S. Park and J. Y. Lee, Acta Biomater., 2018, 80, 258–268 CrossRef CAS PubMed.
- S. Kim, L. K. Jang, M. Jang, S. Lee, J. G. Hardy and J. Y. Lee, ACS Appl. Mater. Interfaces, 2018, 10, 33032–33042 CrossRef CAS PubMed.
- R. A. Green, N. H. Lovell and L. A. Poole-Warren, Acta Biomater., 2010, 6, 63–71 CrossRef CAS PubMed.
- R. A. Green, N. H. Lovell and L. A. Poole-Warren, Biomaterials, 2009, 30, 3637–3644 CrossRef CAS PubMed.
- J. Goding, A. Gilmour, P. Martens, L. Poole-Warren and R. Green, Adv. Healthcare Mater., 2017, 6, 1601177 CrossRef PubMed.
- C. A. R. Chapman, E. A. Cuttaz, J. A. Goding and R. A. Green, Appl. Phys. Lett., 2020, 116, 010501 CrossRef CAS.
- Y. N. Kang, N. Chou, J.-W. Jang, H. K. Choe and S. Kim, Microsyst. Nanoeng., 2021, 7, 1–11 CrossRef PubMed.
- W. Shen, S. Das, F. Vitale, A. Richardson, A. Ananthakrishnan, L. A. Struzyna, D. P. Brown, N. Song, M. Ramkumar, T. Lucas, D. K. Cullen, B. Litt and M. G. Allen, Microsyst. Nanoeng., 2018, 4, 1–15 CrossRef PubMed.
- A. Fanelli and D. Ghezzi, Curr. Opin. Biotechnol, 2021, 72, 22–28 CrossRef CAS PubMed.
- L. Ferlauto, P. Vagni, A. Fanelli, E. G. Zollinger, K. Monsorno, R. C. Paolicelli and D. Ghezzi, Biomaterials, 2021, 274, 120889 CrossRef CAS PubMed.
- W. Cho, S. Yoon and T. D. Chung, Chem. Sci., 2023, 14, 4463–4479 RSC.
- V. Brunetti, G. Maiorano, L. Rizzello, B. Sorce, S. Sabella, R. Cingolani and P. P. Pompa, Proc. Natl. Acad. Sci. U. S. A., 2010, 107, 6264–6269 CrossRef CAS PubMed.
- S. P. Khan, G. G. Auner and G. M. Newaz, Nanomedicine, 2005, 1, 125–129 CrossRef CAS PubMed.
- C. A. R. Chapman, H. Chen, M. Stamou, J. Biener, M. M. Biener, P. J. Lein and E. Seker, ACS Appl. Mater. Interfaces, 2015, 7, 7093–7100 CrossRef CAS PubMed.
-
R. Lahoud, MPhil thesis, American University of Beirut, 2021 Search PubMed.
- M. J. Vassey, G. P. Figueredo, D. J. Scurr, A. S. Vasilevich, S. Vermeulen, A. Carlier, J. Luckett, N. R. M. Beijer, P. Williams, D. A. Winkler, J. de Boer, A. M. Ghaemmaghami and M. R. Alexander, Adv. Sci., 2020, 7, 1903392 CrossRef CAS PubMed.
- N. E. Paul, C. Skazik, M. Harwardt, M. Bartneck, B. Denecke, D. Klee, J. Salber and G. Zwadlo-Klarwasser, Biomaterials, 2008, 29, 4056–4064 CrossRef CAS PubMed.
- R. Ahmed and K. Reifsnider, Int. J. Electrochem. Sci, 2011, 1159–1174 CrossRef CAS.
-
J. P. Seymour and D. R. Kipke, in 2006 International Conference of the IEEE Engineering in Medicine and Biology Society, 2006, pp. 4606–4609.
- P. R. Kennedy, J. Neurosci. Methods, 1989, 29, 181–193 CrossRef CAS PubMed.
- P. R. Kennedy, S. S. Mirra and R. A. E. Bakay, Neurosci. Lett., 1992, 142, 89–94 CrossRef CAS PubMed.
- J. T. W. Kuo, B. J. Kim, S. A. Hara, C. D. Lee, C. A. Gutierrez, T. Q. Hoang and E. Meng, Lab Chip, 2013, 13, 554–561 RSC.
- S. A. Hara, B. J. Kim, J. T. W. Kuo, C. D. Lee, E. Meng and V. Pikov, J. Neural Eng., 2016, 13, 066020 CrossRef PubMed.
- P. R. Patel, K. Na, H. Zhang, T. D. Y. Kozai, N. A. Kotov, E. Yoon and C. A. Chestek, J. Neural Eng., 2015, 12, 046009 CrossRef PubMed.
- F. He, R. Lycke, M. Ganji, C. Xie and L. Luan, iScience, 2020, 23, 101387 CrossRef CAS PubMed.
-
J. L. Skousen, S. M. E. Merriam, O. Srivannavit, G. Perlin, K. D. Wise and P. A. Tresco, in Progress in Brain Research, ed. J. Schouenborg, M. Garwicz and N. Danielsen, Elsevier, 2011, vol. 194, pp. 167–180 Search PubMed.
- Implantable “Neural Dust” Enables Precise Wireless Recording of Nerve Activity, https://www.darpa.mil/news-events/2016-08-03, (accessed January 24, 2023).
- K. Patch, Nat. Biotechnol., 2021, 39, 255–256 CrossRef CAS PubMed.
- X. Yang, T. Zhou, T. J. Zwang, G. Hong, Y. Zhao, R. D. Viveros, T.-M. Fu, T. Gao and C. M. Lieber, Nat. Mater., 2019, 18, 510–517 CrossRef CAS PubMed.
- T. Chung, J. Q. Wang, J. Wang, B. Cao, Y. Li and S. W. Pang, J. Neural Eng., 2015, 12, 056018 CrossRef CAS PubMed.
-
M. A. Elms, mscresearch, University of Leeds, 2017.
- R. T. Hassarati, W. F. Dueck, C. Tasche, P. M. Carter, L. A. Poole-Warren and R. A. Green, Trans. Neural Syst. Rehabil. Eng., 2014, 22, 411–418 Search PubMed.
- R. A. Green, R. T. Hassarati, J. A. Goding, S. Baek, N. H. Lovell, P. J. Martens and L. A. Poole-Warren, Macromol. Biosci., 2012, 12, 494–501 CrossRef CAS PubMed.
- G. Hong, X. Yang, T. Zhou and C. M. Lieber, Curr. Opin. Neurobiol., 2018, 50, 33–41 CrossRef CAS PubMed.
- J. Liu, T.-M. Fu, Z. Cheng, G. Hong, T. Zhou, L. Jin, M. Duvvuri, Z. Jiang, P. Kruskal, C. Xie, Z. Suo, Y. Fang and C. M. Lieber, Nat. Nanotechnol., 2015, 10, 629–636 CrossRef CAS PubMed.
- C. Xie, J. Liu, T.-M. Fu, X. Dai, W. Zhou and C. M. Lieber, Nat. Mater., 2015, 14, 1286–1292 CrossRef CAS PubMed.
- T.-M. Fu, G. Hong, T. Zhou, T. G. Schuhmann, R. D. Viveros and C. M. Lieber, Nat. Methods, 2016, 13, 875–882 CrossRef CAS PubMed.
- T. Zhou, G. Hong, T.-M. Fu, X. Yang, T. G. Schuhmann, R. D. Viveros and C. M. Lieber, Proc. Natl. Acad. Sci. U. S. A., 2017, 114, 5894–5899 CrossRef CAS PubMed.
- S. M. Richardson-Burns, J. L. Hendricks and D. C. Martin, J. Neural Eng., 2007, 4, L6 CrossRef PubMed.
- S. M. Richardson-Burns, J. L. Hendricks, B. Foster, L. K. Povlich, D.-H. Kim and D. C. Martin, Biomaterials, 2007, 28, 1539–1552 CrossRef CAS PubMed.
-
S. J. Wilks, A. J. Woolley, L. Ouyang, D. C. Martin and K. J. Otto, in 2011 Annual International Conference of the IEEE Engineering in Medicine and Biology Society, 2011, pp. 5412–5415.
- L. Ouyang, C. L. Shaw, C. Kuo, A. L. Griffin and D. C. Martin, J. Neural Eng., 2014, 11, 026005 CrossRef PubMed.
- R. T. Rihani, A. M. Stiller, J. O. Usoro, J. Lawson, H. Kim, B. J. Black, V. R. Danda, J. Maeng, V. D. Varner, T. H. Ware and J. J. Pancrazio, Acta Biomater., 2020, 111, 54–64 CrossRef CAS PubMed.
- D. O. Adewole, M. D. Serruya, J. A. Wolf and D. K. Cullen, Front. Neurosci, 2019, 13, 269 CrossRef PubMed.
- A. E. Pereda, Nat. Rev. Neurosci., 2014, 15, 250–263 CrossRef CAS PubMed.
- D. W. Munno and N. I. Syed, J. Physiol., 2003, 552, 1–11 CrossRef CAS PubMed.
- M. J. Vaughn and J. S. Haas, Front. Cell. Neurosci., 2022, 16, 910015 CrossRef PubMed.
-
R. A. Green, K. S. Lim, W. C. Henderson, R. T. Hassarati, P. J. Martens, N. H. Lovell and L. A. Poole-Warren, in 2013 35th Annual International Conference of the IEEE Engineering in Medicine and Biology Society (EMBC), 2013, pp. 6957–6960.
-
R. K. Shepherd, Neurobionics: The Biomedical Engineering of Neural Prostheses, John Wiley & Sons, 2016 Search PubMed.
- A. E. Rochford, A. Carnicer-Lombarte, V. F. Curto, G. G. Malliaras and D. G. Barone, Adv. Mater., 2020, 32, 1903182 CrossRef CAS PubMed.
- I. S. Alferiev, B. Hooshdaran, B. B. Pressly, P. W. Zoltick, S. J. Stachelek, M. Chorny, R. J. Levy and I. Fishbein, Sci. Rep., 2022, 12, 19212 CrossRef CAS PubMed.
- S. Feng, Z. Yan, C. Guo, Z. Chen, K. Zhang, X. Mo and Y. Gu, J. Biomed. Mater. Res., Part A, 2011, 97, 321–329 CrossRef PubMed.
-
N. A. Peppas, B. V. Slaughter and M. A. Kanzelberger, in Polymer Science: A Comprehensive Reference, ed. K. Matyjaszewski and M. Möller, Elsevier, Amsterdam, 2012, pp. 385–395 Search PubMed.
- S. De Faveri, E. Maggiolini, E. Miele, F. De Angelis, F. Cesca, F. Benfenati and L. Fadiga, Front. Neuroeng, 2014, 7(7) Search PubMed.
- J. A. Goding, A. D. Gilmour, U. A. Aregueta-Robles, E. A. Hasan and R. A. Green, Adv. Funct. Mater., 2018, 28, 1702969 CrossRef.
- K. R. Jessen, Int. J. Biochem. Cell Biol., 2004, 36, 1861–1867 CrossRef CAS PubMed.
- C. S. von Bartheld, J. Bahney and S. Herculano-Houzel, J. Comp. Neurol., 2016, 524, 3865–3895 CrossRef PubMed.
-
Glial Cells in Health and Disease of the CNS, ed. R. von Bernhardi, Springer International Publishing, Cham, 2016, vol. 949 Search PubMed.
- Y. Bamba, T. Shofuda, D. Kanematsu, M. Nonaka, M. Yamasaki, H. Okano and Y. Kanemura, Biochem. Biophys. Res. Commun., 2014, 447, 683–688 CrossRef CAS PubMed.
- M. P. Lichtenstein, N. M. Carretero, E. Pérez, M. Pulido-Salgado, J. Moral-Vico, C. Solà, N. Casañ-Pastor and C. Suñol, Neurotoxicology, 2018, 68, 115–125 CrossRef CAS PubMed.
- U. A. Aregueta-Robles, P. J. Martens, L. A. Poole-Warren and R. A. Green, J. Polym. Sci., Part B: Polym. Phys., 2018, 56, 273–287 CrossRef CAS.
-
U. A. Aregueta-Robles, K. S. Lim, P. J. Martens, N. H. Lovell, L. A. Poole-Warren and R. Green, in 2015 37th Annual International Conference of the IEEE Engineering in Medicine and Biology Society (EMBC), 2015, pp. 2600–2603.
- C. Vallejo-Giraldo, M. Genta, O. Cauvi, J. Goding and R. Green, Front. Bioeng. Biotechnol, 2020, 8, 601704 CrossRef PubMed.
- K. Takahashi and S. Yamanaka, Cell, 2006, 126, 663–676 CrossRef CAS PubMed.
- H. Okano, Trends Mol. Med., 2022, 28, 897–899 CrossRef PubMed.
- K.-C. Sonntag, B. Song, N. Lee, J. H. Jung, Y. Cha, P. Leblanc, C. Neff, S. W. Kong, B. S. Carter, J. Schweitzer and K.-S. Kim, Prog. Neurobiol., 2018, 168, 1–20 CrossRef PubMed.
- N. Nagoshi and H. Okano, Cell. Mol. Life Sci., 2018, 75, 989–1000 CrossRef CAS PubMed.
- F. Sivandzade and L. Cucullo, Int. J. Mol. Sci., 2021, 22, 2153 CrossRef CAS PubMed.
- M. Lam, T. Sanosaka, A. Lundin, K. Imaizumi, D. Etal, F. H. Karlsson, M. Clausen, J. Cairns, R. Hicks, J. Kohyama, M. Kele, H. Okano and A. Falk, Genes Cells, 2019, 24, 836–847 CrossRef CAS PubMed.
- B. S. Casas, G. Vitória, M. N. do Costa, R. Madeiro da Costa, P. Trindade, R. Maciel, N. Navarrete, S. K. Rehen and V. Palma, Transl. Psychiatry, 2018, 8, 1–15 CrossRef CAS PubMed.
- J. Triebelhorn, I. Cardon, K. Kuffner, S. Bader, T. Jahner, K. Meindl, T. Rothhammer-Hampl, M. J. Riemenschneider, K. Drexler, M. Berneburg, C. Nothdurfter, A. Manook, C. Brochhausen, T. C. Baghai, S. Hilbert, R. Rupprecht, V. M. Milenkovic and C. H. Wetzel, Mol. Psychiatry, 2022, 1–11 Search PubMed.
- M. Ohnuki and K. Takahashi, Philos. Trans. R. Soc., B, 2015, 370, 20140367 CrossRef PubMed.
- D. Hockemeyer and R. Jaenisch, Cell Stem Cell, 2016, 18, 573–586 CrossRef CAS PubMed.
- S. Peressotti, G. E. Koehl, J. A. Goding and R. A. Green, ACS Biomater. Sci. Eng., 2021, 7, 4136–4163 CrossRef CAS PubMed.
- A. D. Theocharis, S. S. Skandalis, C. Gialeli and N. K. Karamanos, Adv. Drug Delivery Rev., 2016, 97, 4–27 CrossRef CAS PubMed.
- J. L. Drury and D. J. Mooney, Biomaterials, 2003, 24, 4337–4351 CrossRef CAS PubMed.
- S. J. Bidarra, C. C. Barrias and P. L. Granja, Acta Biomater., 2014, 10, 1646–1662 CrossRef CAS PubMed.
- S. A. DeLong, A. S. Gobin and J. L. West, J. Controlled Release, 2005, 109, 139–148 CrossRef CAS PubMed.
- K. Y. Lee and D. J. Mooney, Prog. Polym. Sci., 2012, 37, 106–126 CrossRef CAS PubMed.
- I. Sandvig, K. Karstensen, A. M. Rokstad, F. L. Aachmann, K. Formo, A. Sandvig, G. Skjåk-Bræk and B. L. Strand, J. Biomed. Mater. Res., Part A, 2015, 103, 896–906 CrossRef PubMed.
- R. Boni, A. Ali, A. Shavandi and A. N. Clarkson, J. Biomed. Sci., 2018, 25, 90 CrossRef CAS PubMed.
- M. C. Catoira, L. Fusaro, D. Di Francesco, M. Ramella and F. Boccafoschi, J. Mater. Sci.: Mater. Med., 2019, 30, 115 CrossRef PubMed.
- S. Chattopadhyay and R. T. Raines, Biopolymers, 2014, 101, 821–833 CrossRef CAS PubMed.
-
M. Genta, PhD thesis, Imperial CollegeLondon, 2023.
- D. Hoffman-Kim, J. A. Mitchel and R. V. Bellamkonda, Annu. Rev. Biomed. Eng., 2010, 12, 203–231 CrossRef CAS PubMed.
- R. D. Bierman-Duquette, G. Safarians, J. Huang, B. Rajput, J. Y. Chen, Z. Z. Wang and S. K. Seidlits, Adv. Healthcare Mater., 2022, 11, 2101577 CrossRef CAS PubMed.
- F. Yang, R. Murugan, S. Wang and S. Ramakrishna, Biomaterials, 2005, 26, 2603–2610 CrossRef CAS PubMed.
- M. D. Tang-Schomer, X. Hu, M. Hronik-Tupaj, L. W. Tien, M. J. Whalen, F. G. Omenetto and D. L. Kaplan, Adv. Funct. Mater., 2014, 24, 1938–1948 CrossRef CAS PubMed.
- A. W. Orr, B. P. Helmke, B. R. Blackman and M. A. Schwartz, Dev. Cell, 2006, 10, 11–20 CrossRef CAS PubMed.
- J. M. Barnes, L. Przybyla and V. M. Weaver, J. Cell Sci., 2017, 130, 71–82 CrossRef CAS PubMed.
- A. Banerjee, M. Arha, S. Choudhary, R. S. Ashton, S. R. Bhatia, D. V. Schaffer and R. S. Kane, Biomaterials, 2009, 30, 4695–4699 CrossRef CAS PubMed.
- A. I. Teixeira, S. Ilkhanizadeh, J. A. Wigenius, J. K. Duckworth, O. Inganäs and O. Hermanson, Biomaterials, 2009, 30, 4567–4572 CrossRef CAS PubMed.
- O. Chaudhuri, L. Gu, M. Darnell, D. Klumpers, S. A. Bencherif, J. C. Weaver, N. Huebsch and D. J. Mooney, Nat. Commun., 2015, 6, 6365 CrossRef CAS PubMed.
- O. Chaudhuri, L. Gu, D. Klumpers, M. Darnell, S. A. Bencherif, J. C. Weaver, N. Huebsch, H. Lee, E. Lippens, G. N. Duda and D. J. Mooney, Nat. Mater., 2016, 15, 326–334 CrossRef CAS PubMed.
- O. Chaudhuri, J. Cooper-White, P. A. Janmey, D. J. Mooney and V. B. Shenoy, Nature, 2020, 584, 535–546 CrossRef CAS PubMed.
- C. Cassel de Camps, S. Aslani, N. Stylianesis, H. Nami, N.-V. Mohamed, T. M. Durcan and C. Moraes, ACS Appl. Bio Mater., 2022, 5, 214–224 CrossRef CAS PubMed.
- S. P. Lacour, S. Benmerah, E. Tarte, J. FitzGerald, J. Serra, S. McMahon, J. Fawcett, O. Graudejus, Z. Yu and B. Morrison, Med. Biol. Eng. Comput., 2010, 48, 945–954 CrossRef PubMed.
- E. R. Aurand, K. J. Lampe and K. B. Bjugstad, Neurosci. Res. Lett., 2012, 72, 199–213 CrossRef CAS PubMed.
- K. Saha, A. J. Keung, E. F. Irwin, Y. Li, L. Little, D. V. Schaffer and K. E. Healy, Biophys. J., 2008, 95, 4426–4438 CrossRef CAS PubMed.
-
A. Carnicer-Lombarte, D. G. Barone, I. B. Dimov, R. S. Hamilton, M. Prater, X. Zhao, A. L. Rutz, G. G. Malliaras, S. P. Lacour, C. E. Bryant, J. W. Fawcett and K. Franze, bioRxiv, 2022, preprint DOI:10.1101/829648.
- C. Bertucci, R. Koppes, C. Dumont and A. Koppes, Brain Res. Bull., 2019, 152, 265–284 CrossRef PubMed.
- N. Yamamoto and G. López-Bendito, Eur. J. Neurosci., 2012, 35, 1595–1604 CrossRef PubMed.
- X.-L. Yin, H.-Q. Jie, M. Liang, L.-N. Gong, H.-W. Liu, H.-L. Pan, Y.-Z. Xing, H.-B. Shi, C.-Y. Li, L.-Y. Wang and S.-K. Yin, Front. Mol. Neurosci., 2018, 11, 183 CrossRef PubMed.
- F. J. Martini, T. Guillamón-Vivancos, V. Moreno-Juan, M. Valdeolmillos and G. López-Bendito, Neuron, 2021, 109, 2519–2534 CrossRef CAS PubMed.
- H. J. Luhmann, A. Sinning, J.-W. Yang, V. Reyes-Puerta, M. C. Stüttgen, S. Kirischuk and W. Kilb, Front. Neural Circuits, 2016, 10, 40 Search PubMed.
- A. Burnstine-Townley, Y. Eshel and N. Amdursky, Adv. Funct. Mater., 2020, 30, 1901369 CrossRef CAS.
- J. H. Min, M. Patel and W.-G. Koh, Polymers, 2018, 10, 1078 CrossRef PubMed.
- M. Imaninezhad, K. Pemberton, F. Xu, K. Kalinowski, R. Bera and S. P. Zustiak, J. Neural Eng., 2018, 15, 056034 CrossRef PubMed.
- K. Baranes, M. Shevach, O. Shefi and T. Dvir, Nano Lett., 2016, 16, 2916–2920 CrossRef CAS PubMed.
- N. Saderi, M. Rajabi, B. Akbari, M. Firouzi and Z. Hassannejad, J. Mater. Sci.: Mater. Med., 2018, 29, 134 CrossRef PubMed.
- A. Vashist, A. Kaushik, A. Vashist, V. Sagar, A. Ghosal, Y. K. Gupta, S. Ahmad and M. Nair, Adv. Healthcare Mater., 2018, 7, 1701213 CrossRef PubMed.
- V. Lovat, D. Pantarotto, L. Lagostena, B. Cacciari, M. Grandolfo, M. Righi, G. Spalluto, M. Prato and L. Ballerini, Nano Lett., 2005, 5, 1107–1110 CrossRef CAS PubMed.
- A. Fabbro, A. Villari, J. Laishram, D. Scaini, F. M. Toma, A. Turco, M. Prato and L. Ballerini, ACS Nano, 2012, 6, 2041–2055 CrossRef CAS PubMed.
- A. N. Koppes, K. W. Keating, A. L. McGregor, R. A. Koppes, K. R. Kearns, A. M. Ziemba, C. A. McKay, J. M. Zuidema, C. J. Rivet, R. J. Gilbert and D. M. Thompson, Acta Biomater., 2016, 39, 34–43 CrossRef CAS PubMed.
- O. Akhavan, E. Ghaderi, S. A. Shirazian and R. Rahighi, Carbon, 2016, 97, 71–77 CrossRef CAS.
- L. Sordini, F. F. F. Garrudo, C. A. V. Rodrigues, R. J. Linhardt, J. M. S. Cabral, F. C. Ferreira and J. Morgado, Front. Bioeng. Biotechnol., 2021, 9, 591838 CrossRef PubMed.
- R. Green and M. R. Abidian, Adv. Mater., 2015, 27, 7620–7637 CrossRef CAS PubMed.
-
D.-H. Kim, S. Richardson-Burns, L. Povlich, M. R. Abidian, S. Spanninga, J. L. Hendricks and D. C. Martin, in Indwelling Neural Implants: Strategies for Contending with the In Vivo Environment, ed. W. M. Reichert, CRC Press/Taylor & Francis, Boca Raton (FL), 2008 Search PubMed.
- S. S. Velankar, V. Lai and R. A. Vaia, ACS Appl. Mater. Interfaces, 2012, 4, 24–29 CrossRef CAS PubMed.
- A. Augustine, M. Veillerot, N. Gauthier, B. Zhu, C.-Y. Hui, Y. Tran, E. Verneuil and A. Chateauminois, Soft Matter, 2023, 19, 5169–5178 RSC.
- A. N. Dalrymple, U. A. Robles, M. Huynh, B. A. Nayagam, R. A. Green, L. A. Poole-Warren, J. B. Fallon and R. K. Shepherd, J. Neural Eng., 2020, 17, 026018 CrossRef PubMed.
- T. Hyakumura, U. Aregueta-Robles, W. Duan, J. Villalobos, W. K. Adams, L. Poole-Warren and J. B. Fallon, Front. Neurosci, 2021, 15, 761525 CrossRef PubMed.
- K. C. Spencer, J. C. Sy, K. B. Ramadi, A. M. Graybiel, R. Langer and M. J. Cima, Sci. Rep., 2017, 7, 1952 CrossRef PubMed.
- C. M. Tringides, M. Boulingre, A. Khalil, T. Lungjangwa, R. Jaenisch and D. J. Mooney, Adv. Healthcare Mater., 2023, 12, 2202221 CrossRef CAS PubMed.
- D. O. Adewole, L. A. Struzyna, J. C. Burrell, J. P. Harris, A. D. Nemes, D. Petrov, R. H. Kraft, H. I. Chen, M. D. Serruya, J. A. Wolf and D. K. Cullen, Sci. Adv., 2021, 7, eaay5347 CrossRef CAS PubMed.
- L. A. Struzyna, J. P. Harris, K. S. Katiyar, H. I. Chen and D. K. Cullen, Neural Regener. Res., 2015, 10, 679–685 CrossRef PubMed.
- L. A. Struzyna, J. A. Wolf, C. J. Mietus, D. O. Adewole, H. I. Chen, D. H. Smith and D. K. Cullen, Tissue Eng., Part A, 2015, 21(21–22) CAS.
- V. S. Polikov, M. L. Block, J.-M. Fellous, J.-S. Hong and W. M. Reichert, Biomaterials, 2006, 27, 5368–5376 CrossRef CAS PubMed.
- T. M. Dawson, T. E. Golde and C. L. Tourenne, Nat. Neurosci., 2018, 21, 1370–1379 CrossRef CAS PubMed.
- T. D. Y. Kozai, A. L. Vazquez, C. L. Weaver, S.-G. Kim and X. T. Cui, J. Neural Eng., 2012, 9, 066001 CrossRef PubMed.
- R. Tognatta, M. Merlini, Z. Yan, R. Schuck, D. Davalos and K. Akassoglou, STAR Protoc., 2021, 2, 100638 CrossRef CAS PubMed.
- A. Fernández-Colino, F. Kiessling, I. Slabu, L. De Laporte, P. Akhyari, S. K. Nagel, J. Stingl, S. Reese and S. Jockenhoevel, Adv. Healthcare Mater., 2023, 12, 2300991 CrossRef PubMed.
- S. S. Dalal, E. Edwards, H. E. Kirsch, N. M. Barbaro, R. T. Knight and S. S. Nagarajan, J. Neurosci. Methods, 2008, 174, 106–115 CrossRef PubMed.
- C. Rabut, S. Yoo, R. C. Hurt, Z. Jin, H. Li, H. Guo, B. Ling and M. G. Shapiro, Neuron, 2020, 108, 93–110 CrossRef CAS PubMed.
- T. Deffieux, C. Demené and M. Tanter, Neuroscience, 2021, 474, 110–121 CrossRef CAS PubMed.
- S. H. Kim, J. S. Kwon, J. G. Cho, K. G. Park, T. H. Lim, M. S. Kim, H. S. Choi, C. H. Park and S. J. Lee, Bioeng. Transl. Med., 2021, 6, e10216 CrossRef CAS PubMed.
- R. Guzman, N. Uchida, T. M. Bliss, D. He, K. K. Christopherson, D. Stellwagen, A. Capela, J. Greve, R. C. Malenka, M. E. Moseley, T. D. Palmer and G. K. Steinberg, Proc. Natl. Acad. Sci. U. S. A., 2007, 104, 10211–10216 CrossRef CAS PubMed.
- S. R. Kane, S. F. Cogan, J. Ehrlich, T. D. Plante, D. B. McCreery and P. R. Troyk, IEEE Trans. Biomed. Eng., 2013, 60, 2153–2160 Search PubMed.
- N. A. Alba, Z. J. Du, K. A. Catt, T. D. Y. Kozai and X. T. Cui, Biosensors, 2015, 5, 618–646 CrossRef CAS PubMed.
- C.-F. V. Latchoumane, L. Jackson, M. S. E. Sendi, K. F. Tehrani, L. J. Mortensen, S. L. Stice, M. Ghovanloo and L. Karumbaiah, Sci. Rep., 2018, 8, 10957 CrossRef PubMed.
- M. O’Hara-Wright, S. Mobini and A. Gonzalez-Cordero, Front. Cell Dev. Biol., 2022, 10, 901652 CrossRef PubMed.
- J. Zimmermann, F. Sahm, N. Arbeiter, H. Bathel, Z. Song, R. Bader, A. Jonitz-Heincke and U. van Rienen, Bioelectrochemistry, 2023, 151, 108395 CrossRef CAS PubMed.
- N. Even-Chen, D. G. Muratore, S. D. Stavisky, L. R. Hochberg, J. M. Henderson, B. Murmann and K. V. Shenoy, Nat. Biomed. Eng., 2020, 4, 984–996 CrossRef PubMed.
- S. M. Won, L. Cai, P. Gutruf and J. A. Rogers, Nat. Biomed. Eng., 2023, 7, 405–423 CrossRef PubMed.
- J. D. Simeral, T. Hosman, J. Saab, S. N. Flesher, M. Vilela, B. Franco, J. N. Kelemen, D. M. Brandman, J. G. Ciancibello, P. G. Rezaii, E. N. Eskandar, D. M. Rosler, K. V. Shenoy, J. M. Henderson, A. V. Nurmikko and L. R. Hochberg, IEEE Trans. Biomed. Eng., 2021, 68, 2313–2325 Search PubMed.
- A. B. Rapeaux and T. G. Constandinou, Curr. Opin. Biotechnol, 2021, 72, 102–111 CrossRef CAS PubMed.
- P. Limousin and T. Foltynie, Nat. Rev. Neurol., 2019, 15, 234–242 CrossRef PubMed.
- A. S. Lee, C. Tang, M. S. Rao, I. L. Weissman and J. C. Wu, Nat. Med., 2013, 19, 998–1004 CrossRef CAS PubMed.
- Strategies to Control CAR-T Cell Therapy, https://insights.bio/cell-and-gene-therapy-insights/journal/article/336/Strategies-to-Control-CAR-T-Cell-Therapy-Perspective-on-Next-Generation-CARs, (accessed August 24, 2023).
- S. L. Metzger, K. T. Littlejohn, A. B. Silva, D. A. Moses, M. P. Seaton, R. Wang, M. E. Dougherty, J. R. Liu, P. Wu, M. A. Berger, I. Zhuravleva, A. Tu-Chan, K. Ganguly, G. K. Anumanchipalli and E. F. Chang, Nature, 2023, 620, 1037–1046 CrossRef CAS PubMed.
- F. R. Willett, E. M. Kunz, C. Fan, D. T. Avansino, G. H. Wilson, E. Y. Choi, F. Kamdar, M. F. Glasser, L. R. Hochberg, S. Druckmann, K. V. Shenoy and J. M. Henderson, Nature, 2023, 620, 1031–1036 CrossRef CAS PubMed.
- T. Stieglitz, Neuroethics, 2021, 14, 5–16 CrossRef.
- R. Portillo-Lara, B. Tahirbegi, C. A. R. Chapman, J. A. Goding and R. A. Green, APL Bioeng., 2021, 5, 031507 CrossRef PubMed.
- K. Jebari, Neuroethics, 2013, 6, 617–625 CrossRef.
- J. Clausen, Neuroethics, 2013, 6, 429–434 CrossRef.
- L. Drew, Nature, 2019, 571, S19–S21 CrossRef CAS PubMed.
- F. Gilbert, A. Brown, I. Dasgupta, H. Martens, E. Klein and S. Goering, Neuroethics, 2021, 14, 333–340 CrossRef.
- F. Gilbert, M. Ienca and M. Cook, Brain-Stimul., 2023, 16, 783–789 CrossRef PubMed.
- T. Sawai, Y. Hayashi, T. Niikawa, J. Shepherd, E. Thomas, T.-L. Lee, A. Erler, M. Watanabe and H. Sakaguchi, AJOB Neurosci., 2022, 13, 81–94 CrossRef PubMed.
- A. Clark and D. Chalmers, Analysis, 1998, 58, 7–19 CrossRef.
|
This journal is © The Royal Society of Chemistry 2023 |