Source apportionment of oxidative potential depends on the choice of the assay: insights into 5 protocols comparison and implications for mitigation measures†
Received
11th January 2023
, Accepted 16th August 2023
First published on 21st August 2023
Abstract
The oxidative potential (OP) of particulate matter (PM) has recently been considered as a viable health-based metric of PM exposure. Several acellular assays have been developed to assess OP, but there is no clear consensus in the methodology or protocols used that allows inter-comparison between studies. This research investigates five different acellular OP assays and their sensitivities with the chemical composition of atmospheric particulate matter (PM10) and its emission sources. We revisited and evaluated PM10 samples collected over one year in the urban Alpine city of Grenoble, France. The OP of PM10 was assessed by integrating assays commonly found in the literature, such as ascorbic acid (AA), dithiothreitol (DTT) and 2,7-dichlorofluorescein (DCFH) but also adding novel and less explored assays such as Ferric-Xylenol Orange (FOX), and a direct ROS-quantification through ˙OH. Detailed source apportionment of PM using positive matrix factorisation (PMF) previously performed was coupled with multiple linear regression (MLR) models to determine the OP contribution of PM10 sources. The results highlight the importance of seasonality in the mass contributions of each source and its corresponding influence on OP. These seasonal differences helped to identify the specific reactivity for each studied OP assay. In winter, a good agreement was found between all the OP assays with anthropogenic sources. However, during warmer months, with a reduction in the share of anthropogenic emissions, a higher impact from biogenic and secondary organic-related aerosols has been found. Our results also show a dissimilar sensitivity of each OP to the PM10 sources, likely associated with the chemical composition and chemical processes involved. Thus, our findings show the importance of combining various OP assays to capture different sensitivities to redox-active species to get a clearer picture of the intrinsic capacity of PM sources to cause damaging oxidative reactions in the lung. Providing the heterogeneity of sources obtained with the different OP assays for a given ambient PM exposure, the choice of a single or a combination of OP method(s) must be rationally evaluated as part of the assessment strategy. Such a choice would offer valuable source-related information as a powerful tool to better understand the nature and the intensity of air pollution and envisage the targeted sources for future mitigation policies.
Environmental significance
In recent years, studies have been increasing to link oxidative potential (OP) acellular assays of particulate matter (PM) and adverse health outcomes. However, there is still no consensus on which OP test(s) should be applied to fully assess the intrinsic capacity of PM sources to cause damaging oxidative reactions in the lung. In this work, we integrate a comprehensive evaluation of the chemical composition of PM, their emission sources and their OP, obtained by five different assays: AA, DTT, DCFH, FOX and OH. Our results provide a better understanding of the underlying sensitivities of each OP assay and how the seasonality, concentration and chemical profiles, together with the OP assay selected, can reveal a different exposure ranking for PM sources. We also discuss the selection of the OP assay to be performed and the implications of this choice for future control mitigation strategies.
|
1. Introduction
In recent years, many studies have demonstrated associations between exposure to ambient air pollution and adverse human health outcomes.1–5 Epidemiological studies have suggested that the largest chronic effects of air pollution can be linked to particulate matter (PM), which can induce oxidative stress at the cellular level.6 PM comprises complex chemical mixtures resulting from natural and anthropogenic sources and atmospheric processes. By definition, oxidative stress is the excess production of reactive oxygen species (ROS) relative to antioxidant defence.7 ROS include radical and non-radical oxygen species such as superoxide anion (O2˙−), hydrogen peroxide (H2O2), and hydroxyl radical (OH˙), which represent the different steps of the ROS-formation-induced cascade. The capacity of PM to induce oxidative reactions or to catalytically generate ROS into a biological media is quantified by its oxidative potential (OP).8–11 As a consequence, some recent studies have confirmed that increased exposure to OP in PM (short or long-term) in urban and suburban environments can lead to an increased occurrence of cardiopulmonary or neurological diseases as well as mortality in cases of cancer, asthma, or stroke.8,12–24 In most studies using the ascorbic acid probe (OPAA), no association with adverse health outcomes was observed, such as respiratory and cardiovascular mortality, cardiorespiratory emergencies and lung cancer mortality.16,23,25,26 Nevertheless, some positive associations were found between OPAA and systemic inflammatory biomarkers in short-term exposure studies.18,27 Additionally, a recent study revealed strong associations between outdoor fine particles, oxidizing gases and sulfur and OP (mainly for OPGSH and also for specific cases of OPAA).19,28 These results so far suggest that OPAA provides limited information on the link between OP and adverse health effects, but the number of studies is still too limited to rule out its predictive capacity in terms of health. On the opposite, thiol-based OP probes showed more positive associations with health. OPDTT has been associated with various acute cardiac and respiratory endpoints in some studies.8,12,15,16,18,23,24,29 These findings agree with previous cohort studies showing that respiratory health was more strongly associated with OPDTT than with PM mass.24 Other effects, such as restrictions in foetal growth20,30 and reduced lung function in children,26 have been found in cohort studies where consistent associations with OPDTT have been observed. OP is a relatively simple measure of PM redox activity but also reflects a complex and dynamic interplay of particle size, composition, and chemistry. Consequently, OP of PM is increasingly being studied as a relevant metric associated with health impacts. Indeed, much of the ambient particle mass does not contribute to the toxicity of PM,31 and a current hypothesis is that OP would be more relevant than PM mass to assess exposure effects.
Over the last 10 years, very large developments in source apportionment methods were introduced to determine and quantify the main sources and processes influencing the PM measured at a given location. Particularly, the Positive Matrix Factorization (PMF) model has had several developments and benefited from a series of standardised procedures and quality assurance controls,32 together with the introduction of many new source tracers in the input data, mainly organic species. Specifically, many studies were recently developed on samples from several sites in the Grenoble area,33–36 which now allows to routinely quantify about 9 to 12 sources, both primary and secondary, from biogenic and anthropogenic sources. These developments enable us to perform source apportionment for the PM10 sources in this area, where the total mass of the founded sources is comparable to the total measured PM mass.
Despite those improvements in the state of the knowledge of PM chemistry and OP, there is still no consensus about the type of assay to use and their protocol standardisation, making results across studies difficult to compare. Consequently, there has been an increased interest in measuring and developing OP-related studies involving different in vivo or in vitro assays and extensive aerosol characterisation to estimate the main sources contributing to PM OP. Acellular assays have been considered more favourable for assessing the OP of atmospheric particles as they rely on faster measurement and can be run with less technical constraints than cellular assays (i.e. biological assays).37 Such acellular assays include the dithiothreitol assay (DTT), ascorbic acid assay (AA), 2,7-dichlorofluorescein assay (DCFH), AA-GSH in respiratory tract lining fluid (RTLF) assay, glutathione assay (GSH), and Ferric-Xylenol Orange assay (FOX). These acellular assays target different ROS and display different sensitivities to specific particle components. For example, organic compounds and some soluble transition metals contribute to increasing OP of PM when using the DTT assay,38–40 whereas the AA assay mainly responds to transition metals.29,41,42 In addition, applying these commonly used assays simultaneously allows different mechanisms of ROS generation to be assessed, contributing to the chemical/biological pathways leading to PM-induced oxidative stress. In recent years, there has been an increasing number of studies assessing the OP of PM; however, the analysis of more than two OP tests for a single dataset incorporating full PM chemistry remains scarce.33,38,43
In this context, this work aims first to evaluate the seasonal contribution of PM10 source exposure in an urban hyper-centre site in Grenoble, France. The main goals of this study are to discuss the measured OP assessed by 5 different acellular assays, integrating those commonly found in the literature (AA, DTT, and DCFH) but also the addition of less explored assays such as Ferric-Xylenol Orange (FOX), and a direct ROS-quantification through ˙OH assay. We also investigated the seasonal tendencies and the chemical dependencies associated with each OP assay. Finally, the results of the previous PM10 source apportionment enhanced model were used to deconvolute the inherent oxidising activity contribution of each PM source in terms of OP. This overall work allows, for the first time, a detailed comparison of specific sensitivities of a series of different OP assays, their association to the main drivers of PM10 OP according to the season and such respective implications for sources control.
2. Methods
The study area is located in an alpine valley city in Grenoble, France, with an altitude of 212 m a.s.l. The city is surrounded by several mountain ranges, which typically favour the entrapment of pollution in the valley, particularly during the winter.44 A more detailed description of the study area is available in Borlaza et al.36
The sampling site (Caserne de Bonne in Grenoble's downtown) is located in a 300 × 300 m square pedestrian area bordered by heavy-traffic streets on each side. PM10 measurements were performed by filters sampled for 24 hours in a 3 day sampling interval for a full year. The sampling was performed using a high-volume sampler (Digitel DA80, 30 m3 h−1) onto 150 mm-diameter pure quartz fibre filters (quartz PALL QAT-UP 2500 diameter 150 mm). A total of 127 samples and 20 field blanks were collected from February 28, 2017, to March 10, 2018 (starting at 00:00 LT). After collection, filters were wrapped in aluminium foils and preserved under refrigerated conditions (<4 °C) until analysis.
2.1 PM chemical composition
Comprehensive chemical analyses were performed at IGE for each sample and blank, including ions, sugars, polyols, organic acids, metals and organic and elemental carbon.
Soluble anions and cations (NO3−, SO42−, Cl− and NH4+, Mg2+, Na+, Ca2+, K+) were quantified by ionic chromatography using an ICS3000 dual-channel chromatograph (Thermo-Fisher) following the protocol detailed in Waked et al.45 Briefly, sample punches were soaked in 10 mL of ultra-pure water under orbital shaking and then filtered using 0.22 μm-porosity Acrodisc filters before analysis. The same extract was used for the measurements of sugar alcohols (arabitol, sorbitol, and mannitol, also called polyols) and anhydrous monosaccharides (levoglucosan, mannosan, and galactosan) that were quantified using high-performance liquid chromatography with pulsed amperometric detection (HPLC-PAD).46
Additionally, the identification of a large number of organic acids was performed using the same water extracts. Quantification was performed by HPLC-MS (GP40 Dionex with an LCQ-FLEET Thermos-Fisher ion trap) with negative electrospray ionisation. The separation column is a Synergi 4 μm Fusion – RP 80 Å (250 × 3 mm ID, 4 μm particle size, from Phenomenex). More details about this method can be found in Borlaza et al.38
Concentrations of a large range of inorganic elements, including 18 trace metals, were obtained by ICP-MS analysis (ELAN 6100 DRC II PerkinElmer or NEXION PerkinElmer) following a protocol similar to the one described in Alleman et al.47 Before ICP-MS analyses, each sub-sample was acid digested (with 5 mL of HNO3 and 1.25 mL of H2O2) for 30 min with a microwave oven at 180 °C (MARS 6, CEM).
Finally, organic and elemental carbon (OC and EC) were analysed using a Sunset Lab. Instrument (Model 150C) following the EUSAAR2 thermo-optical protocol.48
The 20 field blank filters were analysed using the same analytical techniques to determine limits of detection (LoD) and to check for the absence of contamination during sample transport, setup, and recovery. These blank values were considered for the calculation of atmospheric concentrations.
2.2 Source apportionment model
The Positive Matrix Factorization (PMF) model was applied to identify and quantify the emission sources making up the PM10 concentrations. Its application to this specific sampling series is described in detail in Borlaza et al.36 In brief, it was conducted using the United States Environmental Protection Agency (US-EPA) software PMF 5.0.49 PMF is based on the factor analysis technique50 applying a weighted least-squares fit algorithm allowing the resolution of eq.1X = (G × F) + E, where: X is an (n × m) matrix representing the species concentration (m) for each sample (n), G is the (n × p) matrix representing the source contributions (p) of each sample, F is the (p × m) matrix representing the factor composition, and E is the residuals matrix (i.e., the difference between measurements and model output). In their study,38 selected 35 chemical species as input variables, namely OC, EC, large series of ions and trace metals, and specific organic tracers: methanesulfonic acid (MSA), levoglucosan, mannosan, polyols (sum of arabitol and mannitol), pinic acid, 3-methyl-1,2,3-butanetricarboxylic acid (3-MBTCA), and phthalic acid. The search for the best solution follows a classical iteration of trials and errors, including the introduction of a series of chemical constraints applied to chemical profiles or PMF factors based on expert geochemical knowledge once a base case solution is obtained,50 as tested in many studies over France and Europe.33 The result obtained for this urban hyper-centre site is a solution with 11 factors, as described below, rather similar to results obtained at other sites within the Grenoble area.36 The PMF-resolved sources were then used as the input variables in the OP deconvolution method. The repartition of chemical species into each PMF source is presented in Fig. S1†.36
2.3 OP assays
2.3.1 The DTT, AA and DCFH assays.
The OP of PM10 samples obtained during the year-long campaign was assessed by extracting the PM in a simulated lung fluid (SLF) solution comprising a mixture of Gamble + DPPC (dipalmitoylphosphatidylcholine) at 37 °C, as described in Calas et al.43 The filter punches extracted were calculated to obtain an extract in SLF at iso-mass at 25 μg mL−1 to keep a constant amount of PM extracted, including both water-soluble and insoluble particles (without filtration). Blank filters were also analysed to blank-correct all measurements. These PM extracts were subjected to three different acellular assays. The dithiothreitol (DTT) assay (OPDTT) is based on the ability of PM to transfer electrons from the DTT molecule to oxygen, generating a superoxide anion and thereby producing ROS. The filter extracts reacted with the DTT solution, resulting in the consumption of DTT, which was then titrated with dithionitrobenzoic acid (DTNB) in direct proportion to the amount of reduced DTT in the solution. The measurements were conducted according to the protocol detailed in Calas et al.,43 adapted from Cho et al.9
The Ascorbic Acid (AA) assay (OPAA) measures the depletion of this lung antioxidant when in contact with PM. Our protocol is fully described in Calas et al.43 This assay measures AA consumption (nmol min−1) inferred by the electrons transfer from AA to oxygen (O2). AA test is usually applied to quantify the transition-metal-based redox activities but is also sensitive to organic tracers.43,51
For both AA and DTT assays, the SLF extracts were injected into a 96-well plate using a microplate reader spectrophotometer (TECAN Infinite M200 Pro). The DTT consumption (nmol min−1) was determined by following the TNB absorbance at 412 nm wavelength at 10 min intervals for 30 min of analysis. For the AA assays, the absorbance was measured at 265 nm at 4 min intervals for 30 min of analysis.
The acellular dichlorofluorescein (DCFH) (OPDCFH) assay relies on a non-fluorescent probe, which is oxidised to a fluorescent product in the presence of ROS and horseradish peroxidase (HRP). The method follows the protocol developed by Foucaud et al.52 The ROS concentration in the sample is calculated in terms of H2O2 equivalent. Then, the DCF is measured by fluorescence at the excitation and emission wavelengths of 485 and 530 nm, respectively, every 2 min for 30 min. This acellular probe is known to be sensitive to organic compounds and some metals51,53 but also to reactive nitrogen species.54
The OP activity is frequently represented by two different normalisation units, including the mass-normalised OP (OPm), where OP values are normalised by the mass of particles (μg), and the volume-normalised OP (OPv), where OP values are normalised by the volume of air sampled (m3). The OPm is the intrinsic OP property associated with 1 μg of PM. Conversely, OPv represents the exposure per volume of sampled air. All samples were subjected to triplicate analysis, resulting in the mean of such a triplicate.
In the next sections, we describe the new OP assays applied in this study: OPOH and OPFOX.
2.3.2 The ˙OH assay.
Hydroxyl radicals (˙OH) induced by PM were measured after the reaction with TerePhtalic Acid (TPA) by measuring the adduct that formed, namely hydroxyterephtalic acid (hTPA). One or two filter punches (13 mm diameter) were extracted in volumes of pure H2O determined from the PM mass accumulated on the filter to reach the targeted concentration of 20 μg PM mL−1. Fluorescence emission of hTPA at λem = 424 nm (λexc = 310 nm) was recorded by using a Tecan® M200 microplate reader over 75 min (with a time step of 2 min) at 30 °C, after the addition of solutions of TPA in phosphate buffer (pH 7.4) and ascorbate (Asc) (final concentrations in wells of 1 and 0.1 mM, respectively). The plate was shaken for 30 seconds before the first measurement and 5 seconds before each subsequent measurement. In the absence of an initial supply of H2O2, ˙OH production catalysed by PM is only significant in the presence of a reducing agent that helps to recycle PM components (chiefly transition metals in the case of Asc) to their reduced form, allowing a small amount of PM to generate a measurable amount of ROS.
The rate of ˙OH production (POH in nM min−1) was derived from the slope of the kinetic curve, obtained from triplicate measurements, which was subtracted from the slope of the blank solution (obtained from a non-exposed filter), using daily calibration curves with hTPA standard solutions (0 to 5.3 nM) and considering the yield of the ˙OH + TPA reaction (γ = 0.35 at pH 7.4) (Page et al.,55 2010). All the samples in the series were processed over 20 days of analysis. The LoD was established daily by analysing blank triplicates (0.4 ± 0.2 nM min−1) and was lower than the lowest POH value measured, i.e. 1.0 nM min−1. Uncertainties were estimated through the interday analysis (at least n = 3 analyses) of randomly chosen filters belonging to the samples database. One particular filter serving as a control and belonging to the series was reserved and systematically extracted and analysed for POH each day of analysis. The POH values obtained for the samples analysed on a given day were then corrected for variations observed for the control filter analysed on the same day. No significant trend in the POH of the control was observed over the 20 days of analysis, confirming that the filter used had maintained its chemical integrity throughout the analysis period.
A coefficient of variation at the 90% confidence level of 20 to 25% (independent of the POH value) can reasonably be proposed for the P˙OH values from the analysis of the samples. Mass-normalized OPOHm (in nM min−1 μg−1) were obtained by dividing POH by the PM10 mass in the wells (i.e., 2.14 μg). Volume-normalized OPOHv (in nM min−1 m−3) were derived from OPOHm values by multiplying them by the atmospheric PM concentration (in μg m−3).
2.3.3 The ferrous-orange xylenol (FOX) assay.
This analytical technique and the associated instrument were previously described in Laulagnet et al.56 In short, the colorimetric Ferrous-Orange Xylenol (FOX) assay is based on the measurement of the oxidation rate of Fe2+ to Fe3+ in the presence of a specific ligand (orange xylenol) and sorbitol. When oxidants are introduced into this reactive mixture, the produced Fe3+ combines with orange xylenol, resulting in an increase of the absorbance at 580 nm. This change in absorbance is followed by a home-made instrument that relies on the multi scattering-enhanced absorbance principle (MEA), a strategy that enables dramatic improvement of the LoD and sensitivity of the analytical device. As a result, LoD as low as 5 pmol H2O2 is reached.56 In addition, the presence of sorbitol in the FOX mixture acts as a chemical amplifier through the iterative conversions of HO˙ into H2O2via radical reactions.57 The FOX assay is a recognised method allowing the determination of oxidants (hydroperoxides) in fats and oil material.58
Briefly, a punch of filter was extracted in water (iso-concentration of 25 μg mL−1), and 100 μl of this extract was injected into a vial containing 1 mL of FOX solution. The solution is vortexed and introduced into the photonic instrument. Once operated, the instrument records the absorbance for 6 min with a time -step of 5 seconds. The average slope obtained from the absorbance plot as a function of time is calculated for triplicate measurements. This slope is converted to H2O2 equivalents using daily calibration curves obtained with H2O2 standard solutions (0–2.5 μM). The coefficient of variation, corresponding to the duplicate analysis of the same loaded filter on six different days, reaches 24%.
2.4 Linking PM sources and OP
Spearman correlations were performed between the PM concentrations and the five OP assays using the RStudio program, which sorts the observations into a rank and computes the levels of similarity between them.
Additionally, multiple linear regression (MLR) analysis has been applied to model observations from the time series of the different OP assays. In the regression models, OP assays are treated as the dependent variables, and the contribution of the 11 sources from the PMF model are the explanatory variables. For the MLR model, a weighted least-square regression (WLS) was used to consider the uncertainties of the OP measurements,33 an improvement to ordinary regression approaches used in the method first developed by Weber et al.59 The MLR model provides the relation between OP and PM sources through the β coefficients for each source. These coefficients represent the intrinsic oxidative capacity of each emission source (intrinsic OP in nmol min−1 μg−1). The MLR model is applied 500 times in the randomly 70% of the dataset to evaluate the uncertainty and the variation of the intrinsic oxidative capacity. Finally, the contribution of PM sources to OP (nmol min−1 m−3) was accessed by multiplying the contribution of each source by the total PM10 mass (μg m−3) by its intrinsic oxidative capacity.
3. Results and discussion
3.1 Temporal and seasonal evolution of PM and OP assays
The previous PMF results showed that biomass burning, sulfate-rich, nitrate-rich and road traffic are the main sources that drive the average PM10 mass yearly.36 However, when those results are analysed on a seasonal basis, the contribution of PM10 emission sources shows a different picture. Fig. 1 shows the average daily contribution of PM10 sources categorised into warm (May, June, July, August, and September) and cold months (November, December, January, February, and March) in Grenoble. During colder months, a similar contribution was observed to that obtained for the whole year. This result indicates that the main sources controlling average PM10 concentrations are mainly related to the ones present in the winter when the maximum PM10 levels were observed. The median contributions were also obtained and presented in Fig. S2 of the ESI† to minimise the effect of extreme measurements. There were slight changes observed in the ranking of sources, with road traffic being the third highest contributor during colder months (Fig. S2†).
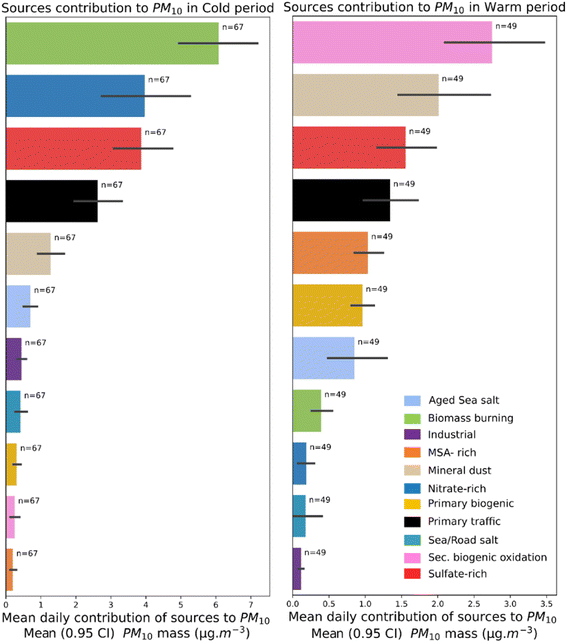 |
| Fig. 1 Average daily contribution of PM10 sources separated on a seasonal basis. Left: average contribution considering cold months (November, December, January, February, and March). Right: average contribution considering warm months (May, June, July, August, and September). | |
The average contribution of sources during the warmer months showed a different ranking of contributions. Secondary biogenic oxidation contributed the most to PM10 concentration, followed by mineral dust, sulfate-rich, and road traffic sources. The higher contribution of secondary organic aerosols in the summer months is likely related to the seasonality of biogenic sources, whose emissions are temperature- and light-dependent. Regarding mineral dust, previous results indicate that this source presents a strong local component coupled with atmospheric dynamics at the urban scale.36 As a result, the median contribution of PM10 sources in warmer months relocates mineral dust as the fourth highest contributor, preceded by secondary biogenic oxidation, sulfate-rich, and road traffic (Fig. S2†).
Fig. S3† shows the daily temporal evolution of PM10 concentrations and the volume-normalised OP (OPv) obtained for each OP assay. Higher average PM10 concentrations were observed in winter (20.69 ± 10.52 μg m−3) than in summer (16.08 ± 6.40 μg m−3). Similarly, higher concentrations for all the OP assays were observed in the winter compared to the summer in Grenoble. This seasonality was already observed in various sites in mountainous areas in France, related to the development of thermal inversion layers in such valleys, inducing the accumulation of pollutants and endorsing the secondary formation processes of aerosol with a significant impact on the OP.33,36,60
The average OPAAv was 2.38 ± 1.66 nmol min−1 m−3 in the cold months, whereas a mean value of 0.72 ± 0.39 nmol min−1 m−3 was observed in the warm months. The average values of OPAAv obtained in our study are in the same range as those observed in urban sites in Switzerland (4.1–1.7 nmol min−1 m−3)61 and urban areas in France (2.3–1.0 nmol min−1 m−3),33 or in Spain (1.9 nmol min−1 m−3).62
Average values for OPDTTv obtained in the cold and warm months were 1.92 ± 1.31 and 1.46 ± 0.71 nmol min−1 m−3, respectively. The mean values of OPDTTv observed in this study are within the range of those measured in similar studies in urban valleys in France (Chamonix = 2.3 and Marnaz = 1.8 nmol min−1 m−3,33) or in a Swiss urban site (Bern = 2.9 nmol min−1 m−3).61
Mean OPDCFHv values were 1.53 ± 1.10 and 0.50 ± 0.28 nmol min−1 m−3 in the winter and summer, which are higher than those observed in previous studies in Switzerland (0.4–0.7 nmol min−1 m−3,61).
A similar seasonal trend is observed in the additional OP assays presented in this study. For example, the average of OPFOXv in the winter was 430.7 ± 304.0 pmol H2O2 eq. m−3 compared to 210.6 ± 127.5 in the summer. This is also observed for the OPOHv assay with mean values of 72.61 ± 67.74 and 45.25 ± 42.96 nmol min−1 m−3 in the winter and summer, respectively. However, since these two OPs are less explored assays for ambient PM, an inter-study comparison was not possible.
3.2 Relationships between OP assays and PM10
Fig. 2 shows the correlation and distribution plots of daily OPv activity related to PM10 mass on a seasonal basis, filtered into winter (blue, cold months) and summer (orange, warm months) seasons. When correlated with PM10 mass concentration, all five OPv showed a stronger correlation in the winter compared to the summer. This behaviour is consistent with results observed in several locations in France (Grenoble, Passy, Marnaz, and Chamonix)33,60 and Beijing, China.63 For instance, OPAAv presents better correlation coefficients with PM10 mass concentration (μg m−3) in winter (Spearman coefficient r = 0.84) than in summer (r = 0.36) (Fig. 2), similarly for OPDCFHv, which correlates well in winter and poorly in summer, with r = 0.83 vs. 0.35, respectively. However, seasonal correlations for OPDTTv with PM10 are less pronounced, with slightly higher coefficients in winter (r = 0.83) than in summer (r = 0.56). The weak correlations between PM10 mass concentration and OP during warmer months highlight the importance of considering the impacts of sources with low mass contribution but relevant contribution in OP.
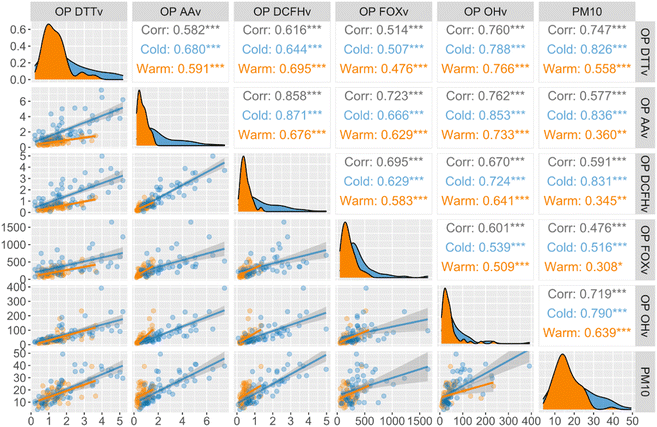 |
| Fig. 2 Correlation and distribution plots of daily OPv activity related to PM10 mass (μg m−3), coloured by seasonality, in blue for cold months and orange for warm months. The Spearman correlation coefficients are calculated for the total data (black), cold (blue), and warm (orange) periods. The stars represent the p-values (*) resulting from the test of association between paired samples: for p-value <0.001 (“***”); p-value <0.01 (“**”); p-value r < 0.05 (“*”). | |
Similar to OPDTTv, the differences between the seasons observed for OPOHv were also less pronounced between OPvvs. PM10 correlations (r = 0.79 vs. 0.64 for cold and warm months, respectively). The poorest correlation coefficients were found for the OPFOXv assay (r = 0.52 and 0.31, respectively), even if seasonality was observed (Fig. 2).
The seasonal variability of OP activity observed to a different extent by our tests is consistent with the previous studies.33,38,43,64,65 These results suggest that the five assays are sensitive to different PM components and that different PM sources, components, or atmospheric processes are predominantly reactive for the assay responses in winter and summer. The higher correlation coefficients presented in Fig. 2 demonstrate that PM mass could reasonably predict total OPv in winter. Still, in summer, a more detailed understanding is necessary to elucidate the OP of PM. In contrast, the similarity observed in the correlations of
and PM10 between the two seasons suggests that species influencing ˙OH variability (i.e., compounds related to or mediated by ˙OH) in the aerosols are relatively stable.
Regarding the correlation between the OP assays, our results show higher Spearman coefficients between OPOHv and OPAAv correlation (r = 0.76), which present higher values during winter observations. This strong relationship between both assays could be related to the fact that OPOH uses the ascorbic acid as a reductant, looking at the ˙OH produced by the AA depletion.
A good correlation is also observed with OPDTTv (r = 0.76) but with less seasonality than the one displayed by OPAAv. Interestingly, higher linear correlation slopes are observed in summer than in winter for OPOHv compared with DCFH, AA, and FOX, suggesting its higher sensitivity to the aerosol chemistry observed in summer. These results could be related to the higher production of atmospheric oxidants, such as OH radical (the most active oxidant in the troposphere66) and the increase of the atmospheric oxidation capacity (AOC) in summer.67 The AOC refers to the total rate at which the atmosphere removes pollutants through oxidation and determines the formation of secondary pollution67 and the generation of secondary particles in the troposphere.68
Our results showing a good agreement between OPOH and OPAA (and to a lesser extent with OPDTT) confirms the feasibility of using assays that evaluates ROS, albeit indirect (such as AA and DTT), as a good proxy to evaluate the ROS production due to PM exposure.
3.3 Toxicity of PM10 sources: intrinsic OP
The intrinsic OP (OPm) denotes the ability of each PM source to induce oxidative stress, as estimated by the β coefficients obtained from the MLR model. Briefly, higher intrinsic OP values imply a possibly higher capability of the source to induce oxidative stress. Different OP assays capture different redox reactions involved in the production of different ROS species. Table 1 shows the average and standard deviation of intrinsic OP obtained from the bootstrapped WLS model. OP intrinsic values for all PM10 sources ranged from −0.18 ± 0.14 to 0.44 ± 0.17 nmol min−1 μg−1 for OPDTT, −0.04 ± 0.06 to 0.44 ± 0.33 nmol min−1 μg−1 for OPAA, −0.05 ± 0.25 to 1.15 ± 0.68 nmol min−1 μg−1 for OPDCFH, 0.14 ± 1.11 to 22.12 ± 12.9 nmol min−1 μg−1 for OPOH and −7.15 ± 12.3 to 71.9 ± 73.5 pmol H2O2 eq μg−1 for OPFOX (Table 1 and Fig. S4†). The differences in the average values are related to measurement units; while AA, DTT, and DCFH assays measure the oxidation of biological antioxidants or surrogates/probes by different ROS species, ˙OH is related to the generation of hydroxyl radicals (˙OH) and FOX to the equivalent amount of hydroperoxides in general (including H2O2).
Table 1 Mean and standard deviation of the regression coefficient (i.e., intrinsic OP of the 500 bootstraps) obtained from the MLR (WLS) for the PM10 sources in nmol min−1 μg−1 (and pmol H2O2 eq. μg−1 for OPFOX) and constant in nmol min−1 m−3 (pmol min−1 m−3 for OPFOX) from the MLR. Bold values represent the sources with the highest intrinsic activity for each OP assay. Boxplots for each source and OP assay are shown in Fig. S4
source |
OPDTT |
OPAA |
OPDCFH |
OPOH |
OPFOX |
Aged sea salt |
0.038 ± 0.070 |
−0.003 ± 0.02 |
−0.016 ± 0.079 |
0.729 ± 0.637 |
−9.93 ± 12.6 |
Biomass burning |
0.19 ± 0.066
|
0.14 ± 0.028
|
0.090 ± 0.059
|
2.37 ± 0.743
|
21.9 ± 8.35
|
Industrial |
−0.010 ± 0.51 |
0.44 ± 0.33
|
1.1 ± 0.68
|
22.1 ± 12.9
|
71.9 ± 73.5
|
MSA-rich |
0.43 ± 0.17
|
−0.021 ± 0.038 |
0.096±0.17
|
0.138 ± 1.11 |
−5.96 ± 16.0 |
Mineral dust |
0.15 ± 0.074
|
−0.033 ± 0.027 |
0.025 ± 0.12 |
1.06 ± 0.738 |
14.4 ± 12.3 |
Nitrate-rich |
0.009 ± 0.05 |
0.025 ± 0.015 |
0.036 ± 0.068 |
1.22 ± 0.367 |
−7.14 ± 12.3 |
Primary biogenic |
0.32 ± 0.11 |
−0.041 ± 0.065 |
0.11±0.21 |
0.884 ± 1.12 |
7.51 ± 24.8 |
Primary traffic |
0.17 ± 0.071 |
0.22±0.034 |
−0.001 ± 0.07 |
2.88 ± 0.844 |
23.7 ± 14.2 |
Sea/road salt |
−0.18 ± 0.14 |
0.11 ± 0.10 |
−0.046 ± 0.25 |
2.00 ± 1.59 |
21.5 ± 23.2 |
Secondary biogenic oxidation |
0.022 ± 0.057 |
0.042 ± 0.018 |
−0.024 ± 0.11 |
3.48 ± 0.69 |
7.66 ± 8.07 |
Sulfate-rich |
−0.053 ± 0.054 |
0.057 ± 0.015 |
0.061 ± 0.074 |
0.627 ± 0.572 |
−0.195 ± 7.97 |
Const |
−0.084 |
0.13 |
0.019 |
4.38 |
49.3 |
Positive intrinsic OP values were observed for most PM sources resolved by the PMF model (Table 1). However, it can be noted that intrinsic OP values varied from one assay to another. For example, slightly negative mean values were observed for the industrial source for the DTT assay. However, this source presents the highest values for the four other OP assays. Furthermore, biomass burning (BB) and primary traffic also presented high intrinsic OP values for three of five assays (OPAA, OPDCFH, and OPFOX for BB and OPAA, OPOH, and OPFOX for traffic). These results show that anthropogenic sources such as industrial, BB, and traffic dominate OP activity at this urban site. Furthermore, these results are linked with the chemical composition of sources, all associated with high loadings of metals (As, Cu, Fe, Mn, Mo, Ni, Sb, and Sn) and organic compounds (EC, OC, sugars, and organic acids).38
Conversely, some differences are observed. MSA-rich and mineral dust were among the sources with the highest intrinsic OPDTT, but they did not show any substantial contribution to other assays. In addition, some OP tests show a contribution to natural sources such as primary biogenic (OPDTT and OPDCFH) and secondary biogenic oxidation (OPOH). Even if the PMF sources do not show a proper biogenic profile and some impact from resuspension dust can be noted (Fig. S1†), our results suggest that those OP tests could be reactive in the presence of biogenic sources, as previously shown in the contribution of PM concentration during warm months.69
Previous studies have shown that DTT, AA, and DCFH tests are sensitive to the presence of metals,43 DTT being also responsive to organic compounds, such as quinones, HULIS, and polycyclic aromatic hydrocarbons (PAH).9,70,71 Thus, the DTT assay is generally considered a wide-spectrum multipollutant assay, making it the most common OP test discussed in the literature.39
Furthermore, FOX presents a similar sensitivity to AA; both assays share the same dominant sources regarding intrinsic OP. On the contrary, OPOH presents a dissimilar behaviour, showing its reactivity towards the presence of metals and secondary oxidation products, such as 3-MBTCA and phthalic acid. These differences observed between the intrinsic coefficients exhibit the sensitivities associated with each OP assay, which depends on the chemical composition of each PM10 source (Fig. S1†).
3.4 Seasonal exposure to PM10
Identifying emissions sources that contribute to OP could be substantial for developing targeted air quality control strategies aiming to protect human health. In this section, we evaluate the relative contributions of the PM sources to the total OPv, weighted by their different intrinsic OP values. In addition, we assessed the seasonal contributions of the sources in terms of PM mass and OP. Fig. 3 presents the average daily contribution of PM10 sources based on each OP test separated into cold and warm periods. Generally, the seasonality of OP exposure follows the one associated with the concentrations of the sources (Fig. 1), with some differences between the OP tests.
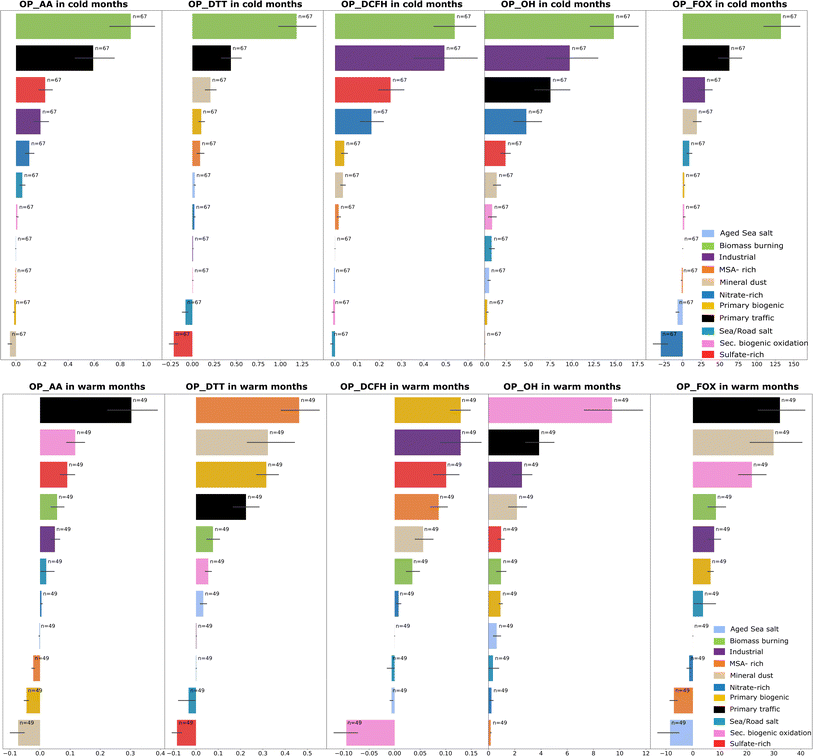 |
| Fig. 3 Average daily contribution of PM10 sources based on five OPv assays in Grenoble, France. Top: source-specific OP exposition during cold months, Bottom: source-specific OP exposition during warm months in nmol of each oxidant min−1 m−3 (and in pmol min−1 m−3 for OPFOX). | |
During cold months, a reasonable consensus is observed between the different OP assays. Biomass burning is the source that contributes the most to the OP measured by the five assays. This is followed by primary traffic in the case of OPAA, OPDTT, and OPFOX and by the industrial source in OPDCFH and OPOH. The sulfate-rich and nitrate-rich sources, although high contributors to PM mass concentrations, showed much lower contributions in terms of OP, except for OPDCFH, and even for some tests, they present a negative contribution (OPDTT and OPFOX). This suggests that even if they largely contribute to the PM mass in winter, the sources of secondary inorganic aerosols do not have a high impact on the OP observed.
The results obtained for the OP exposure during warm months presented more heterogeneous profiles. There is no clear picture of a common source as observed in winter; however, primary traffic is highly ranked for the OPAA, OPFOX, and OPOH assays. For these three assays, the secondary biogenic oxidation source also depicts a high impact, mainly for the OPOH and the OPAA tests.
These results show the significance of considering the seasonal variability of PM sources that drive the OP of PM. Although overall, the analysis of the combined annual data is similar to the winter season. This can be explained by the fact that extreme PM concentrations occur in winter and drive the annual average values of OP, as well as by the high intrinsic OP values for winter sources (such as biomass burning). Furthermore, our findings underline that the most dominant OP sources in summer are not only those related to anthropogenic activities (biomass burning, primary traffic, industrial) but also the ones related to natural emissions (dominated by primary biogenic and secondary biogenic oxidation and followed by mineral dust and MSA-rich sources). Finally, these results highlight the sensitivities of each OP assay to specific sources and the underlying mechanisms associated with each assay, implying that some OP tests could be more appropriate in sites where their sensitivities are suitable.
3.5 Highlights on OPOH and OPFOX
Our work integrates the results from two less commonly explored OP assays. The integration of these tests allows for a comprehensive view of the different oxidative mechanisms and to assess the differences and commonalities in terms of OP sensitivities to different PM sources.
To evaluate the interactions between PM10 sources and to capture the non-linear atmospheric dynamics causing potential synergistic or antagonistic effects on the OP activity, the multilayer perceptron (MLP) neural network was applied besides the MLR model. Detailed information about this method was discussed in a previous paper.36 Briefly, MLP produces a predictive output model for one or more variables (OPv) based on the interconnection layers of artificial neurons obtained from the values of the input variables (source contributions of PM10). In this study, the MLP model was applied to the new OP assays, ˙OH and FOX, limited to one hidden layer as implemented in the study of Borlaza et al.36 for DTT, AA and DCFH.
Fig. S6† shows the results obtained from the two models, MLR and MLP, relative to the OP observations. The OPFOX presents a higher Pearson correlation for the MLP model; however, OPOH shows better coefficients between observations and modelled OP using the MLR method. The OPFOX results align with those previously observed for OPAA, OPDTT, and OPDCFH,38 with the MLP model generally performing slightly better than the MLR one.
To visualise such possible non-linear behaviour, the MLP models obtained were applied to the mass contributions of each source (in μg m−3, from the PMF results). After that, the original modelled OP (MLP) (containing all source contributions) was compared to the sum of source-specific modelled OP activity. Our comparison shows a high Pearson correlation between MLP and MLP_sum modelled OP for both tests, FOX and ˙OH (Fig. S7†). These results could indicate weaker synergistic/antagonistic effects between sources both for ˙OH and FOX measured OP, contrarily to what was observed for other OP assays (DTT, DCFH and AA).38 Our findings show a discrepancy from those obtained in a previous laboratory model, where OPOH displayed additional synergistic or antagonistic effects in the metal–organics interactions applied.72 Thus, even if our results present low synergistic/antagonistic effects for FOX and ˙OH assays, their application to other PM samples with dissimilar chemical content and concentrations could provide a different insight, so the translation of our results to other studies needs caution.
3.6 Evaluation of OP tests variability
To assess the collinearity between OP assays, a dimension reduction technique such as principal components analysis (PCA) was also applied to see the correlations between the OPm of each assay. The results from the PCA showed complementary information about the joint variability of the OP assays. Therefore, those tests presenting an analogous sensitivity to PM chemical composition will depict a similar covariance, and they will be then associated with a specific PC or dimension. Fig. 4 shows the square cosine (cos2, depicting the quality of the representation by a component for a given variable) of all OPv assays with PC1 (i.e., Dim.1) and PC2 (i.e., Dim.2), which explains the highest variability of the data set (Fig. S8†). All the OP assays were positively and highly represented by the principal components (arrows near the circumference of the correlation circle equal to 1). A similar variability is observed between OPDTT and OPOH, between OPDCFH and OPAA, and to a lesser extent with OPFOX. These results suggest similar sensitivities of OPDTT and OPOH, which could be related to the sensitivity towards organic species, especially highly oxidised organics, as shown in the literature.9,73,74 Moreover, the similar variability observed between OPAA, OPDCFH and OPFOX could represent their higher sensitivity towards metal-rich species. However, OPFOX also presents a higher sensitivity towards peroxides species,57 which could explain the lower representation in PC1 and PC2 and their presence in PC3 (counting for about 14% of the variability, Fig. S8†). Even if these results could seem to oppose the ones discussed in Section 3.2, the correlation obtained between OP assays and PCA dimensions agrees quite well with those observed from spearman correlations (Fig. S9†).
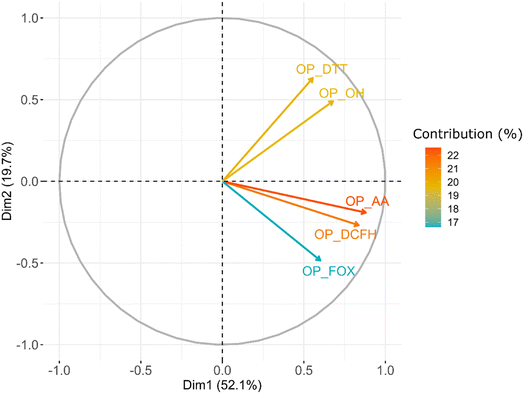 |
| Fig. 4 Principal component analysis (PCA) using OPm observations. Principal component 1 (Dim1) and principal component 2 (Dim2) are plotted on the x and y axes, respectively. The distance of the arrow between the variables and the origin measures the quality and representativeness of the variables on the PC. Colours represent the contribution of each variable to the total variability of the PC1 and PC2, and the circumference represents the cos2 correlation circle equal to 1. | |
The reduced contribution of PC1 and PC2 (71%) explaining the total variability of OP measurements suggests that other atmospheric, chemical or biological processes could be responsible for modulating the OP observed. Finally, our PCA result suggests that for those sampling sites with PM chemistry showing high metal compositions, AA and DCFH tests seem to be the more appropriate to use, while for peroxide-rich PM, FOX is the more suitable test, and finally, for organics and highly oxidised organics, DTT and ˙OH can better address the ROS-formation mechanisms.
3.7 Normalised sensitivity of OP assays to PM sources
To closer evaluate the sensitivities of each OP assay towards specific PM10 sources, we assessed the normalised sensitivity of each OP. For that, and in order to avoid the differences in the units, the mass contribution of PM10 sources to each OP was normalised to the total relative PM10 mass associated with each source provided by the PMF solution ((Average OPvi sourcex/ΣOPv sourcex × 100)/sourcex mass).
Fig. 5 shows the PM normalised relative mass contribution of each PM source to each OP assay. It can be noted that OP activity presents a different sensitivity to the PM sources. For example, OPAA shows a high response to biomass burning and sulfate-rich, followed by primary traffic and nitrate-rich sources. In addition, it shows a negative contribution to mineral dust, aged sea salt, MSA-rich, and primary biogenic sources. Even if there are differences in the response of the AA test by the sources obtained, it indicates an impact from those sources with a high loading of trace metals as well as secondary inorganic processing aerosols.
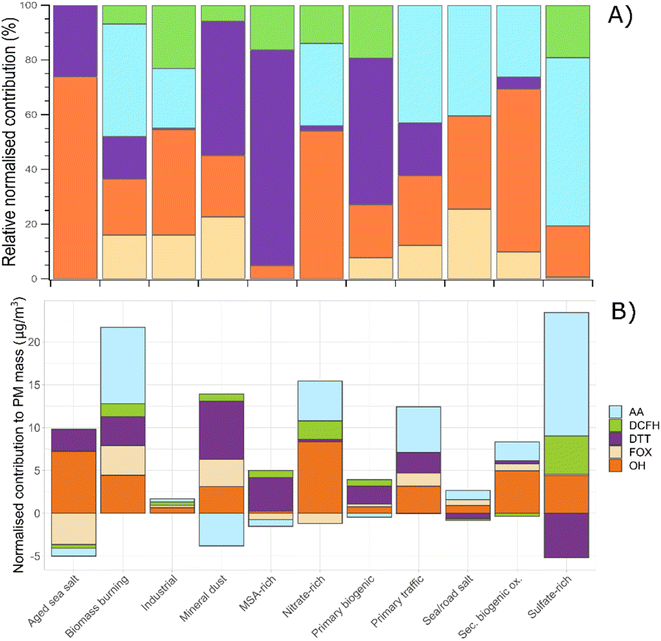 |
| Fig. 5 OP sensitivity contribution of each PM source. (A) Relative normalised contribution of OP assays to the PM10 sources and (B) normalised OP to the PM10 mass of the source. Colours represent each OP test evaluated. | |
In the case of OPDTT, it shows a high positive sensitivity mainly towards mineral dust and MSA-rich, followed by biomass burning, primary biogenic, and aged sea salt sources. On the other hand, opposite to AA, the DTT test presents a low or negative response to secondary inorganic aerosols. Nonetheless, as previous studies already discussed, our results confirm the DTT sensitive response toward sources with the presence of trace metals and organic compounds.9,43,51,71
For OPDCFH, the sensitivity shows a higher positive impact on the contributions from the nitrate-rich and sulfate-rich sources, commonly associated with secondary anthropogenic emissions. On the contrary, OPFOX displays a higher response towards biomass burning, mineral dust, and primary traffic sources. However, this test presents an insignificant or negative sensitivity towards aged sea salt, nitrate-rich, and sulfate-rich, showing a lower response to processed (aged) PM sources.
OPOH activity shows sensitivity to all the sources of PM observed. The higher impacts are related to aged sea salt, nitrate-rich, secondary biogenic oxidation, and sulfate-rich, followed by primary sources such as biomass burning, traffic, and mineral dust.
Our results show that even if the sensitivity of the different OP tests depends on the chemical composition of the PM sources, the OP exposure can also be controlled by the seasonality and, in some cases, by the mass concentration of aerosol sources, as shown in Fig. 2.
Furthermore, our results strengthened the idea that the OP observations and the assignment of its source-specific contributions depend on the assay used, which will consequently have implications in the decision of future mitigation actions. Therefore, a panel of assays are recommended to evaluate the diversity of site typologies, and at least two complementary assays are necessary to provide useful insight from a wide range of redox-active species and to weigh the harmful sources. Our results, obtained in this urban alpine site in France, may vary in other regions under the presence of contrasting emission sources and different size fractions. Hence, the selection of the complementary OP tests to be applied in a given place will strongly depend on knowing beforehand the geochemistry of sources, concentrations and chemical composition of particles evaluated.
3.8 Limitations of the study
Our study assesses 5 different acellular OP assays, together with the seasonal tendencies of emission sources of PM10 and their chemical dependencies associated with each OP assay. Part of our results revisited some of the OP values obtained in the previous work of Borlaza et al.36 and were gathered with new OP analysis for the same time series. This has some limitations since part of the analysis were performed at different time. Even though the samples were kept refrigerated under controlled conditions before analysis, an inherent ageing process may have affected OP absolute values over time. However, it should not have affected the variability (considering homogenous ageing) of the time series, which is of importance when looking at associations. Nevertheless, the stability of filters (and OP) over time need to be evaluated in the future to estimate and quantify the ageing losses.
Our results investigate the link between OP and sources for the PM10 fraction but would result in certain differences (in terms of the absolute value of OP and the contribution of each source) for the PM2.5 fraction since the size range affects the source contributions. When assessing the link between OP and PM, which is the subject of this study, PM10, for including all the particles <10 μm (i.e. it integrates the PM2.5), has the main advantage of identifying more PM sources than is commonly resolved by PM2.5 source apportionment (break-wear, road wear, biogenic emissions…). PM10vs. PM2.5 are more influenced by mechanically generated particles that affect OP, such as brake wear and mineral dust.75 Moreover, sources of PM are a mixture of particles with varying sizes, forming a size distribution spread across different orders. Consequently, the “coarse fraction” of PM10 can be misleading as it might suggest that these particles are relatively harmless to health when it contains particles small enough to have health implications.76 In the last years, epidemiological studies evidenced that PM2.5 accounted for a stronger association with the adverse effects of PM. However, the association of short-term mortality upon exposure to both PM10 and PM2.5 does not demonstrate a threshold effect.77 Moreover, recent epidemiological studies revisited the link between PM10 and cardiovascular diseases, with consistent associations for both PM2.5 and PM10.78 Nevertheless, it must be acknowledged that PM10 has historically been the most widely reported measure of particulate matter in aerosol science and continues to be a relevant indicator for many epidemiological studies. Thus, using PM10 and OP of PM10 in epidemiological studies may lower the strength of the association compared to PM2.5 but may also allow a better source identification, which can further be used to investigate the sources that most contribute to the adverse effects of PM10.
4. Conclusions
In this study, we evaluated five different OP assays together with a comprehensive chemistry associated with PM10 sources in an urban alpine valley in France. A large change in the profile of PM10 sources was observed when considering the seasonality, with major contributors in winter related to biomass burning, primary traffic, and secondary inorganic aerosols formed during long-range transport. On the other hand, in warm months, the main PM mass contributors are mostly secondary organic and inorganic oxidation products, as well as traffic and mineral road dust, with traffic presenting a relatively homogeneous contribution throughout the year. These differences also implied an effect on the OP activity of PM associated with each OP assay. A good agreement was found with anthropogenic sources leading the OP exposure (mainly biomass burning and primary traffic) for all the OP assays assessed during winter. However, this trend changes during warm months, with a reduction of the share of anthropogenic emissions and a higher impact from biogenic and secondary organic-related aerosols.
The OP encompasses the relevant redox reactions induced by main PM sources that may lead to oxidative stress, acting as a good indicator for understanding the link between PM composition and health exposure, and this is beyond what the aerosol mass can provide. Our findings showed good correlation coefficients between the direct OP˙OH assay and the widely used indirect OPAA and OPDTT assays. This supports the feasibility of using indirect OP assays to assess ROS production by PM in biological media.
In addition, our results imply that the choice of a given OP methodology affects the resulting PM source exposure ranking. We recommend combining at least two acellular assays like DTT or OH with AA, DCFH, or FOX in order to capture a wider sensitivity to redox active species presented in PM sources. However, the selection of different OP assays must be rationally evaluated and will rely on previous knowledge about the chemical composition and concentration of PM. Such a strategy is essential since this affects the message to be delivered about the targeted sources for future mitigation policies.
In the last years, they are some studies supporting the growing body of evidence about the significance of OP of PM as a valuable health-based exposure metric. However, it is important to note that the epidemiological evidence in this field of research remains limited due to its relatively recent emergence. The application of OP in health studies has only become feasible in the past decade, thanks to the development of less time-consuming OP methodologies. Therefore, further epidemiological studies assessing the relevance of OP for health outcomes are still needed. Future studies must be conducted using standardized OP protocols to determine the capacity of OP to serve as an additional health predictor beyond PM mass. Therefore, the ongoing development and evaluation of diverse OP assays with broader sensitivities to redox-active substances should persist. This research will allow exploring the relationship between OP and health outcomes, identifying the strongest predictor of health effects, and providing a comprehensive understanding of the toxicity associated with different sources of PM.
Author contributions
Conceptualization: P. A. D, G. U., L. J. B., J. L. J., resources: J. J. S., S. H., G. S., S. T., C. T., S. H., E. M., G. M., formal analysis: P. A. D., L. J. B., V. D. N. T., visualization: P. A. D. and V. D. N. T., funding acquisition: G. U. and J. L.J., writing – original draft: P. A. D., writing – review and editing: all authors.
Conflicts of interest
The authors declare no conflicts of interest.
Acknowledgements
This work was supported by the French National Research Agency in the framework of the Investissements d’Avenir Program (ANR-15-IDEX-02), the MobilAir program, the European Union Horizon 2020 research and innovation programme under grant agreement 101036245 (RI-URBANS), and ANR Get-OP Stand-OP (ANR-19-CE34-0002-01). The FOX OP analyses presented here were funded by the TOXinTRANSPORT Program (ANSES). The postdoc of Pamela Dominutti was funded by the ANR Get-OP Stand-OP project (Project-ANR-19-CE34-0002-01). The postdoc of Lucille Joanna Borlaza is funded by the Predict'air project (grant Fondation UGA-UGA 2022-16 and grant PR-PRE-2021 FUGA-Fondation Air Liquide).
References
- J. Lelieveld, A. Pozzer, U. Pöschl, M. Fnais, A. Haines and T. Münzel, Loss of life expectancy from air pollution compared to other risk factors: a worldwide perspective, Cardiovasc. Res., 2020, 116(11), 1910–1917 CrossRef CAS PubMed . Available from: https://academic.oup.com/cardiovascres/article/116/11/1910/5770885.
- J. E. Hart, X. Liao, B. Hong, R. C. Puett, J. D. Yanosky and H. Suh,
et al., The association of long-term exposure to PM2.5 on all-cause mortality in the Nurses' Health Study and the impact of measurement-error correction, Environ. Health, 2015, 14(1), 38 CrossRef PubMed . Available from: https://ehjournal.biomedcentral.com/articles/10.1186/s12940-015-0027-6.
- R. Burnett, H. Chen, M. Szyszkowicz, N. Fann, B. Hubbell and C. A. Pope,
et al., Global estimates of mortality associated with longterm exposure to outdoor fine particulate matter, Proc. Natl. Acad. Sci. U. S. A., 2018, 115(38), 9592–9597 CrossRef CAS PubMed.
- J. Lepeule, F. Laden, D. Dockery and J. Schwartz, Chronic Exposure to Fine Particles and Mortality: An Extended Follow-up of the Harvard Six Cities Study from 1974 to 2009, Environ. Health Perspect., 2012, 120(7), 965–970 CrossRef PubMed . Available from: https://ehp.niehs.nih.gov/doi/10.1289/ehp.1104660.
- F. Laden, J. Schwartz, F. E. Speizer and D. W. Dockery, Reduction in Fine Particulate Air Pollution and Mortality, Am. J. Respir. Crit. Care Med., 2006, 173(6), 667–672 CrossRef CAS PubMed . Available from: https://www.atsjournals.org/doi/10.1164/rccm.200503-443OC.
- N. Li, T. Xia and A. E. Nel, The role of oxidative stress in ambient particulate matter-induced lung diseases and its implications in the toxicity of engineered nanoparticles, Free Radical Biol. Med., 2008, 44(9), 1689–1699 CrossRef CAS PubMed . Available from: https://linkinghub.elsevier.com/retrieve/pii/S0891584908000713.
-
K. Shankar and H. M. Mehendale, Oxidative Stress, Encyclopedia of Toxicology, Elsevier, Third Edit., 3rd edn, 2014, Vol. 3, pp. 735–737 Search PubMed.
- J. T. Bates, R. J. Weber, J. Abrams, V. Verma, T. Fang and M. Klein,
et al., Reactive Oxygen Species Generation Linked to Sources of Atmospheric Particulate Matter and Cardiorespiratory Effects, Environ. Sci. Technol., 2015, 49(22), 13605–13612 CrossRef CAS PubMed.
- A. K. Cho, C. Sioutas, A. H. Miguel, Y. Kumagai, D. A. Schmitz and M. Singh,
et al., Redox activity of airborne particulate matter at different sites in the Los Angeles Basin, Environ. Res., 2005, 99(1), 40–47 CrossRef CAS PubMed . Available from: https://linkinghub.elsevier.com/retrieve/pii/S0013935105000058.
- G. Uzu, J. J. Sauvain, A. Baeza-Squiban, M. Riediker, M. Sánchez Sandoval Hohl and S. Val,
et al., In vitro assessment of the pulmonary toxicity and gastric availability of lead-rich particles from a lead recycling plant, Environ. Sci. Technol., 2011, 45(18), 7888–7895 CrossRef CAS PubMed.
- J. J. Sauvain, S. Deslarzes and M. Riediker, Nanoparticle reactivity toward dithiothreitol, Nanotoxicology, 2008, 2(3), 121–129 CrossRef CAS.
- J. Y. Abrams, R. J. Weber, M. Klein, S. E. Samat, H. H. Chang and M. J. Strickland,
et al., Associations between Ambient Fine Particulate Oxidative Potential and Cardiorespiratory Emergency Department Visits, Environ. Health Perspect., 2017, 125(10), 107008, DOI:10.1289/EHP1545.
- C. Cristina, M. Cosetta, D. Christina, K. Frank, P. Shah and C. Cielito,
et al., Geographic disparities in US mortality, Epidemiology, 2014, 25(3), 467–468 CrossRef PubMed.
- A. Dabass, E. O. Talbott, J. R. Rager, G. M. Marsh, A. Venkat and F. Holguin,
et al., Systemic inflammatory markers associated with cardiovascular disease and acute and chronic exposure to fine particulate matter air pollution (PM2.5) among US NHANES adults
with metabolic syndrome, Environ. Res., 2018, 161, 485–491, DOI:10.1016/j.envres.2017.11.042.
- R. J. Delfino, C. Sioutas and S. Malik, Potential role of ultrafine particles in associations between airborne particle mass and cardiovascular health, Environ. Health Perspect., 2005, 113(8), 934–946 CrossRef PubMed.
- T. Fang, H. Guo, L. Zeng, V. Verma, A. Nenes and R. J. Weber, Highly Acidic Ambient Particles, Soluble Metals, and Oxidative Potential: A Link between Sulfate and Aerosol Toxicity, Environ. Sci. Technol., 2017, 51(5), 2611–2620 CrossRef CAS PubMed.
- D. Gao, S. Ripley, S. Weichenthal and K. J. Godri Pollitt, Ambient particulate matter oxidative potential: Chemical determinants, associated health effects, and strategies for risk management, Free Radical Biol. Med., 2020, 151, 7–25 CrossRef CAS PubMed.
- N. A. H. Janssen, M. Strak, A. Yang, B. Hellack, F. J. Kelly and T. A. J. Kuhlbusch,
et al., Associations between three specific a-cellular measures of the oxidative potential of particulate matter and markers of acute airway and nasal inflammation in healthy volunteers, Occup. Environ. Med., 2015, 72(1), 49–56 CrossRef PubMed . Available from: https://oem.bmj.com/lookup/doi/10.1136/oemed-2014-102303.
- J. Korsiak, E. Lavigne, H. You, K. Pollitt, R. Kulka and M. Hatzopoulou,
et al., Air Pollution and Pediatric Respiratory Hospitalizations: Effect Modification by Particle Constituents and Oxidative Potential, Am. J. Respir. Crit. Care Med., 2022, 206(11), 1370–1378, DOI:10.1164/rccm.202205-0896OC.
- E. Lavigne, R. T. Burnett, D. M. Stieb, G. J. Evans, K. J. Godri Pollitt and H. Chen,
et al., Fine particulate air pollution and adverse birth outcomes: Effect modification by regional nonvolatile oxidative potential, Environ. Health Perspect., 2018, 126(7), 077012 CrossRef PubMed.
- M. Strak, N. Janssen, R. Beelen, O. Schmitz, D. Karssenberg and D. Houthuijs,
et al., Associations between lifestyle and air pollution exposure: Potential for confounding in large administrative data cohorts, Environ. Res., 2017, 156, 364–373 CrossRef CAS PubMed . Available from: https://linkinghub.elsevier.com/retrieve/pii/S0013935116306612.
- S. Weichenthal, E. Lavigne, A. Traub, D. Umbrio, H. You and K. Pollitt,
et al., Association of Sulfur, Transition Metals, and the Oxidative Potential of Outdoor PM2.5 with Acute Cardiovascular Events: A Case-Crossover Study of Canadian Adults, Environ Health Perspect., 2021, 129(10), 107005, DOI:10.1289/EHP9449.
- S. Weichenthal, D. L. Crouse, L. Pinault, K. Godri-Pollitt, E. Lavigne and G. Evans,
et al., Oxidative burden of fine particulate air pollution and risk of cause-specific mortality in the Canadian Census Health and Environment Cohort (CanCHEC), Environ. Res., 2016, 146, 92–99 CrossRef CAS PubMed . Available from: https://linkinghub.elsevier.com/retrieve/pii/S0013935115301742.
- A. Yang, N. A. H. Janssen, B. Brunekreef, F. R. Cassee, G. Hoek and U. Gehring, Children's respiratory health and oxidative potential of PM2.5: The PIAMA birth cohort study, Occup. Environ. Med., 2016, 73(3), 154–160 CrossRef PubMed.
- C. L. Maikawa, S. Weichenthal, A. J. Wheeler, N. A. Dobbin, A. Smargiassi and G. Evans,
et al., Particulate Oxidative Burden as a Predictor of Exhaled Nitric Oxide in Children with Asthma, Environ. Health Perspect., 2016, 124(10), 1616–1622, DOI:10.1289/EHP175.
- A. Marsal, R. Slama, S. Lyon-Caen, L. J. S. Borlaza, J. L. Jaffrezo and A. Boudier,
et al., Prenatal Exposure to PM2.5 Oxidative Potential and Lung Function in Infants and Preschool- Age Children: A Prospective Study, Environ. Health Perspect., 2023, 131(1), 017004, DOI:10.1289/EHP11155.
- L. Liu, B. Urch, M. Szyszkowicz, G. Evans, M. Speck and A. Van Huang,
et al., Metals and oxidative potential in urban particulate matter influence systemic inflammatory and neural biomarkers: A controlled exposure study, Environ.
Int., 2018, 121, 1331–1340 CrossRef CAS PubMed . Available from: https://linkinghub.elsevier.com/retrieve/pii/S0160412018320014.
- O. Toyib, E. Lavigne, A. Traub, D. Umbrio, H. You and S. Ripley,
et al., Long-term Exposure to Oxidant Gases and Mortality: Effect Modification by PM2.5 Transition Metals and Oxidative Potential, Epidemiology, 2022, 33(6), 767–776, DOI:10.1097/EDE.0000000000001538.
- T. Fang, V. Verma, J. T Bates, J. Abrams, M. Klein and J. M. Strickland,
et al., Oxidative potential of ambient water-soluble PM2.5 in the southeastern United States: Contrasts in sources and health associations between ascorbic acid (AA) and dithiothreitol (DTT) assays, Atmos. Chem. Phys., 2016, 16(6), 3865–3879 CrossRef CAS.
- L. J. S. Borlaza, G. Uzu, M. Ouidir, S. Lyon-Caen, A. Marsal and S. Weber,
et al., Personal exposure to PM2.5 oxidative potential and its association to birth outcomes, J. Exposure Sci. Environ. Epidemiol., 2022, 33, 416–426, DOI:10.1038/s41370-022-00487-w.
- M. Park, H. S. Joo, K. Lee, M. Jang, S. D. Kim and I. Kim,
et al., Differential toxicities of fine particulate matters from various sources, Sci. Rep., 2018, 8(1), 17007 CrossRef PubMed.
-
C. A. Belis, O. Favez, M. Mircea, E. Diapouli, M.-I. Manousakas, S. Vratolis, et al.European Guide on Air Pollution Source Apportionment with Receptor Models: Revised Version 2019, [Internet], Joint Research Center, Publications Office, 2019, Available from: DOI:10.2760/439106.
- S. Weber, G. Uzu, O. Favez, L. J. S. Borlaza, A. Calas and D. Salameh,
et al., Source apportionment of atmospheric PM10 oxidative potential: synthesis of 15 year-round urban datasets in France, Atmos. Chem. Phys., 2021, 21(14), 11353–11378 CrossRef CAS . Available from: https://acp.copernicus.org/articles/21/11353/2021/.
- D. Srivastava, S. Tomaz, O. Favez, G. M. Lanzafame, B. Golly and J. L. Besombes,
et al., Speciation of organic fraction does matter for source apportionment. Part 1: A one-year campaign in Grenoble (France), Sci. Total Environ., 2018, 624, 1598–1611, DOI:10.1016/j.scitotenv.2017.12.135.
- S. Weber, D. Salameh, A. Albinet, L. Y. Alleman, A. Waked and J. L. Besombes,
et al., Comparison of PM10 sources profiles at 15 french sites using a harmonized constrained positive matrix factorization approach, Atmosphere, 2019, 10(6), 1–22 CrossRef.
- L. J. S. Borlaza, S. Weber, G. Uzu, V. Jacob, T. Cañete and S. Micallef,
et al., Disparities in particulate matter (PM10) origins and oxidative potential at a city scale (Grenoble, France) – Part 1: Source apportionment at three neighbouring sites, Atmos. Chem. Phys., 2021, 21(7), 5415–5437 CrossRef CAS.
- J. T. Bates, T. Fang, V. Verma, L. Zeng, R. J. Weber and P. E. Tolbert,
et al., Review of Acellular Assays of Ambient Particulate Matter Oxidative Potential: Methods and Relationships with Composition, Sources, and Health Effects, Environ. Sci. Technol., 2019, 53(8), 4003–4019 CrossRef CAS PubMed.
- L. J. S. Borlaza, S. Weber, J. L. Jaffrezo, S. Houdier, R. Slama and C. Rieux,
et al., Disparities in particulate matter (PM10) origins and oxidative potential at a city scale (Grenoble, France) – Part 2: Sources of PM10 oxidative potential using multiple linear regression analysis and the predictive applicability of multilayer perceptron n, Atmos. Chem. Phys., 2021, 21(12), 9719–9739 CrossRef CAS . Available from: https://acp.copernicus.org/articles/21/9719/2021/.
- J. T. Bates, T. Fang, V. Verma, L. Zeng, R. J. Weber and P. E. Tolbert,
et al., Review of Acellular Assays of Ambient Particulate Matter Oxidative Potential: Methods and Relationships with Composition, Sources, and Health Effects, Environ. Sci. Technol., 2019, 53(8), 4003–4019 CrossRef CAS PubMed.
- J. G. Charrier and C. Anastasio, On dithiothreitol (DTT) as a measure of oxidative potential for ambient particles: evidence
for the importance of soluble transition metals, Atmos. Chem. Phys., 2012, 12(19), 9321–9333 CrossRef CAS.
- M. Visentin, A. Pagnoni, E. Sarti and M. C. Pietrogrande, Urban PM2.5 oxidative potential: Importance of chemical species and comparison of two spectrophotometric cell-free assays, Environ. Pollut., 2016, 219, 72–79 CrossRef CAS PubMed.
- K. J. Godri, S. T. Duggan, G. W. Fuller, T. Baker, D. Green and F. J. Kelly,
et al., Particulate matter oxidative potential from waste transfer station activity, Environ. Health Perspect., 2010, 118(4), 493–498 CrossRef CAS PubMed.
- A. Calas, G. Uzu, F. J. Kelly, S. Houdier, J. M. F. Martins and F. Thomas,
et al., Comparison between five acellular oxidative potential measurement assays performed with detailed chemistry on PM10 samples from the city of Chamonix (France), Atmos. Chem. Phys., 2018, 18(11), 7863–7875 CrossRef CAS . Available from: https://acp.copernicus.org/articles/18/7863/2018/.
- B. Bessagnet, L. Menut, R. Lapere, F. Couvidat, J. L. Jaffrezo and S. Mailler,
et al., High Resolution Chemistry Transport Modeling with the On-Line CHIMERE-WRF Model over the French Alps—Analysis of a Feedback of Surface Particulate Matter Concentrations on Mountain Meteorology, Atmosphere, 2020, 11(6), 565 CrossRef CAS.
- A. Waked, O. Favez, L. Y. Alleman, C. Piot, J. E. Petit and T. Delaunay,
et al., Source apportionment of PM10 in a north-western Europe regional urban background site (Lens, France) using positive matrix factorization and including primary biogenic emissions, Atmos. Chem. Phys., 2014, 14(7), 3325–3346 CrossRef.
- A. Samaké, J. L. Jaffrezo, O. Favez, S. Weber, V. Jacob and A. Albinet,
et al., Polyols and glucose particulate species as tracers of primary biogenic organic aerosols at 28 French sites, Atmos. Chem. Phys., 2019, 19(5), 3357–3374 CrossRef.
- L. Y. Alleman, L. Lamaison, E. Perdrix, A. Robache and J. C. Galloo, PM10 metal concentrations and source identification using positive matrix factorization and wind sectoring in a French industrial zone, Atmos. Res., 2010, 96(4), 612–625 CrossRef CAS.
- F. Cavalli, M. Viana, K. E. Yttri, J. Genberg and J. P. Putaud, Toward a standardised thermal-optical protocol for measuring atmospheric organic and elemental carbon: the EUSAAR protocol, Atmos. Meas. Tech., 2010, 3(1), 79–89 CrossRef CAS.
-
G. Norris, R. Duvall, S. Brown and S. Bai, Positive Matrix Factorization (PMF) 5.0 Fundamentals and User Guide, 2014, vol. 136 Search PubMed.
- P. Paatero and U. Tapper, Positive matrix factorization: A non-negative factor model with optimal utilization of error estimates of data values, Environmetrics, 1994, 5(2), 111–126 CrossRef.
- J. J. Sauvain, M. J. Rossi and M. Riediker, Comparison of three acellular tests for assessing the oxidation potential of nanomaterials, Aerosol Sci. Technol., 2013, 47(2), 218–227 CrossRef CAS.
- L. Foucaud, M. Wilson, D. Brown and V. Stone, Measurement of reactive species production by nanoparticles prepared in biologically relevant media, Toxicol. Lett., 2007, 174(1–3), 1–9 CrossRef CAS PubMed.
- M. G. Perrone, J. Zhou, M. Malandrino, G. Sangiorgi, C. Rizzi and L. Ferrero,
et al., PM chemical composition and oxidative potential of the soluble fraction of particles at two sites in the urban area of Milan, Northern Italy, Atmos. Environ., 2016, 128, 104–113, DOI:10.1016/j.atmosenv.2015.12.040.
- H. R. Cheng, H. Guo, S. M. Saunders, S. H. M. Lam, F. Jiang and X. M. Wang,
et al., Assessing photochemical ozone formation in the Pearl River Delta with a photochemical trajectory model, Atmos. Environ., 2010, 44(34), 4199–4208 CrossRef CAS.
- S. E. Page, W. A. Arnold and K. McNeill, Terephthalate as a probe for photochemically generated hydroxyl radical, J. Environ. Monit., 2010, 12, 1658–1665, 10.1039/c0em00160k.
- A. Laulagnet, J. J. Sauvain, N. Concha-Lozano, M. Riediker and G. Suárez, Sensitive Photonic System to Measure Oxidative Potential of Airborne Nanoparticles and ROS Levels in Exhaled Air, Procedia Eng., 2015, 120, 632–636, DOI:10.1016/j.proeng.2015.08.659.
- C. Gay and J. M. Gebicki, A critical evaluation of the effect of sorbitol on the ferric-xylenol orange hydroperoxide assay, Anal. Biochem., 2000, 284(2), 217–220 CrossRef CAS PubMed.
- R. Bou, R. Codony, A. Tres, E. A. Decker and F. Guardiola, Determination of hydroperoxides in foods and biological samples by the ferrous oxidation–xylenol orange method: A review of the factors that influence the method's performance, Anal. Biochem., 2008, 377(1), 1–15 CrossRef CAS PubMed . Available from: https://linkinghub.elsevier.com/retrieve/pii/S0003269708001103.
- S. Weber, G. Uzu, A. Calas, F. Chevrier, J. L. Besombes and A. Charron,
et al., An apportionment method for the oxidative potential of atmospheric particulate matter sources: application to a one-year study in Chamonix, Atmos. Chem. Phys., 2018, 18(13), 9617–9629 CrossRef CAS . Available from: https://acp.copernicus.org/articles/18/9617/2018/.
- A. Calas, G. Uzu, J. L. Besombes, J. M. F. Martins, M. Redaelli and S. Weber,
et al., Seasonal Variations and Chemical Predictors of Oxidative Potential (OP) of Particulate Matter (PM), for Seven Urban French Sites, Atmosphere, 2019, 10(11), 698 CrossRef CAS.
- S. K. Grange, G. Uzu, S. Weber, J. Jaffrezo and C. Hueglin, Linking Switzerland's PM;10 and PM2.5 oxidative potential (OP) with emission sources, Atmos. Chem. Phys., 2022, 22(10), 7029–7050 CrossRef CAS . Available from: https://acp.copernicus.org/articles/22/7029/2022/.
- M. in ’t Veld, M. Pandolfi, F. Amato, N. Pérez, C. Reche and P. Dominutti,
et al., Discovering oxidative potential (OP) drivers of atmospheric PM10, PM2.5, and PM1 simultaneously in North-Eastern Spain, Sci. Total Environ., 2022, 857, 159386 CrossRef PubMed . Available from: https://linkinghub.elsevier.com/retrieve/pii/S0048969722064853.
- S. J. Campbell, K. Wolfer, B. Utinger, J. Westwood, Z. H. Zhang and N. Bukowiecki,
et al., Atmospheric conditions and composition that influence PM2.5 oxidative potential in Beijing, China, Atmos. Chem. Phys., 2021, 21(7), 5549–5573 CrossRef CAS PubMed.
- V. Verma, T. Fang, H. Guo, L. King, J. T. Bates and R. E. Peltier,
et al., Reactive oxygen species associated with water-soluble PM2.5 in the southeastern United States: spatiotemporal trends and source apportionment, Atmos. Chem. Phys., 2014, 14(23), 12915–12930 CrossRef.
- S. J. Campbell, K. Wolfer, B. Utinger, J. Westwood, Z. H. Zhang and N. Bukowiecki,
et al., Atmospheric conditions and composition that influence PM& lt;sub& gt;2.5& lt;/sub& gt; oxidative potential in Beijing, China, Atmos. Chem. Phys., 2021, 21(7), 5549–5573 CrossRef CAS PubMed . Available from: https://acp.copernicus.org/articles/21/5549/2021/.
- N. Cheng, Z. Chen, F. Sun, R. Sun, X. Dong and X. Xie,
et al., Ground ozone concentrations over Beijing from 2004 to 2015: Variation patterns, indicative precursors and effects of emission-reduction, Environ. Pollut., 2018, 237, 262–274 CrossRef CAS PubMed.
- Y. Yang, Y. Wang, W. Huang, D. Yao, S. Zhao and Y. Wang,
et al., Parameterized atmospheric oxidation capacity and speciated OH reactivity over a suburban site in the North China Plain: A comparative study between summer and winter, Sci. Total Environ., 2021, 773, 145264 CrossRef CAS PubMed . Available from: https://linkinghub.elsevier.com/retrieve/pii/S0048969721003302.
- T. Feng, S. Zhao, N. Bei, J. Wu, S. Liu and X. Li,
et al., Secondary organic aerosol enhanced by increasing atmospheric oxidizing capacity in Beijing–Tianjin–Hebei (BTH), China, Atmos. Chem. Phys., 2019, 19(11), 7429–7443 CrossRef CAS . Available from: https://acp.copernicus.org/articles/19/7429/2019/.
- A. Samake, G. Uzu, J. M. F. Martins, A. Calas, E. Vince and S. Parat,
et al., The unexpected role of bioaerosols in the Oxidative Potential of PM, Sci. Rep., 2017, 7(1), 10978, DOI:10.1016/j.freeradbiomed.2017.06.019 , http://www.nature.com/articles/s41598-017-11178-0.
- A. I. Totlandsdal, M. Låg, E. Lilleaas, F. Cassee and P. Schwarze, Differential proinflammatory responses induced by diesel exhaust particles with contrasting PAH and metal content, Environ. Toxicol., 2015, 30(2), 188–196, DOI:10.1002/tox.21884.
- M. Y. Chung, R. A. Lazaro, D. Lim, J. Jackson, J. Lyon and D. Rendulic,
et al., Aerosol-Borne Quinones and Reactive Oxygen Species Generation by Particulate Matter Extracts, Environ. Sci. Technol., 2006, 40(16), 4880–4886 CrossRef CAS PubMed.
- P. Lin and J. Z. Yu, Generation of Reactive Oxygen Species Mediated by Humic-like Substances in Atmospheric Aerosols, Environ. Sci. Technol., 2011, 45(24), 10362–10368, DOI:10.1021/es2028229.
- R. D. McWhinney, K. Badali, J. Liggio, S. M. Li and J. P. D. Abbatt, Filterable Redox Cycling Activity: A Comparison between Diesel Exhaust Particles and Secondary Organic Aerosol Constituents, Environ. Sci. Technol., 2013, 47(7), 3362–3369, DOI:10.1021/es304676x.
- Q. Xiong, H. Yu, R. Wang, J. Wei and V. Verma, Rethinking Dithiothreitol-Based Particulate Matter Oxidative Potential: Measuring Dithiothreitol Consumption versus Reactive Oxygen Species Generation, Environ. Sci. Technol., 2017, 51(11), 6507–6514 CrossRef CAS PubMed.
- J. K. Gietl, R. Lawrence, A. J. Thorpe and R. M. Harrison, Identification of brake wear particles and derivation of a quantitative tracer for brake dust at a major road, Atmos. Environ., 2010, 44(2), 141–146 CrossRef CAS . Available from: https://linkinghub.elsevier.com/retrieve/pii/S1352231009008735.
- A. Izzotti, P. Spatera, Z. Khalid and A. Pulliero, Importance of Punctual Monitoring to Evaluate the Health Effects of Airborne Particulate Matter, Int. J. Environ. Res. Public Health, 2022, 19(17), 10587 CrossRef CAS PubMed . Available from: https://www.mdpi.com/1660-4601/19/17/10587.
- C. Liu, R. Chen, F. Sera, A. M. Vicedo-Cabrera, Y. Guo and S. Tong,
et al., Ambient Particulate Air Pollution and Daily Mortality in 652 Cities, N. Engl. J. Med., 2019, 381(8), 705–715, DOI:10.1056/NEJMoa1817364.
- J. Bont, S. Jaganathan, M. Dahlquist, Å. Persson, M. Stafoggia and P. Ljungman, Ambient air pollution and cardiovascular diseases: An umbrella review of systematic reviews and meta-analyses, J. Intern. Med., 2022, 291(6), 779–800 CrossRef PubMed . Available from: https://onlinelibrary.wiley.com/doi/10.1111/joim.13467.
|
This journal is © The Royal Society of Chemistry 2023 |
Click here to see how this site uses Cookies. View our privacy policy here.