Brown carbon absorptivity in fresh wildfire smoke: associations with volatility and chemical compound groups†
Received
15th May 2023
, Accepted 16th July 2023
First published on 17th July 2023
Abstract
Organic aerosol (OA) emissions from wildfires across the western United States have significant impacts on the climate and air quality. Brown carbon (BrC)—the light-absorbing component of OA—has been at the forefront of atmospheric aerosol research. In particular, how the different classes of organic compounds comprising OA relate to BrC light absorption remains an outstanding research question. In this study, we investigated the impact of OA chemical composition and volatility on the optical properties of BrC from fresh smoke emitted from three wildfires in the western United States. Our findings indicate that low volatility organics are well-correlated with both the methanol-soluble and water-insoluble components of BrC. We determined the volatility of the aerosols using two distinct methods and obtained consistent results using both approaches. Higher elemental carbon (EC) concentrations were associated with greater light absorption by BrC, suggesting the possible co-emission of strongly light-absorbing chromophores with EC. Using chemical speciation data, we performed a multivariate regression with the different compound groups and identified that a combination of polycyclic aromatic hydrocarbons, oxygenated aromatics, and nitrogen-containing organics is the best predictor of light-absorption by the methanol- and water-soluble components of BrC. Water-insoluble BrC accounted for 43 ± 11% of the methanol-soluble BrC light absorption at 405 nm, which is consistent with previous studies. This study validates previous laboratory observations of increasing light absorptivity of biomass burning BrC with decreasing volatility of OA molecules and solvent-solubility. We provide additional insight into specific compound groups that may act as BrC chromophores in wildfire emissions.
Environmental significance
Light-absorbing organic aerosol emissions from wildfires, also termed brown carbon (BrC), can have large impacts on climate and air quality. To accurately predict changes in BrC optical properties with atmospheric processing, understanding the relationship between BrC light absorptivity and its chemical composition is crucial. Our study revealed strong associations between BrC light absorptivity and low and extremely low volatility organic compounds in fresh wildfire plumes. These low volatility compounds were likely to be water-insoluble and co-emitted with elemental carbon during high-temperature combustion. Our findings suggest that for modeling of radiative forcing from BrC to be accurate, absorption by extremely low volatility and water-insoluble light absorbing organics needs to be explicitly included.
|
1 Introduction
Organic aerosol (OA) dominates the carbonaceous particulate emissions from wildfires, with the organic mass being up to 10 times larger than the corresponding black carbon (BC) emissions.1,2 Brown carbon (BrC) – a subclass of OA – primarily absorbs light at shorter-visible and ultraviolet (UV) wavelengths,3 while BC strongly absorbs visible wavelength light.4 The imaginary component of the BrC refractive index (indicative of particle light absorption) spans a wide range of values from 10−3 to 0.3 (ref. 3 and 5–8) at ultraviolet and lower visible wavelengths. The wide range of refractive index values indicates that different mechanisms and fuels lead to the formation of BrC with distinct optical properties. Understanding the relationship between BrC optical properties and their chemical composition is crucial for predicting the radiative effect of BrC during atmospheric chemical processing.
Pöschl9 initially proposed a classification system for BrC, relating optical and thermochemical classes of carbonaceous particles to various molecular groups. A comprehensive review by Laskin et al.10 provides current knowledge on the chemistry and of BrC, including both optical and chemical characterization methods. A powerful technique for chemical characterization of biomass burning BrC involves combining high-performance liquid chromatography with photodiode array detection and high-resolution mass spectrometry.11,12 This approach has successfully identified nitroaromatics and oxygenated aromatics as important BrC chromophores. However, it may overlook high molecular weight compounds that are not extractable in the solvents used for analysis. The importance of molecular size in determining BrC light absorptivity can be highlighted size-exclusion chromatography measurements.13–16 Online measurements of BrC using aerosol mass spectrometers have identified oxygenated organics,8,17,18 nitroaromatics,19–21 and low-volatility oxygenated organics22 as dominant light-absorbing groups in different regions. The wide range of compounds observed in BrC across various studies underscores the complexity of BrC composition and emphasizes the need for a more holistic metric to relate BrC optical properties to chemical composition.
The optical properties of BrC can be quantified by measuring the light absorption by molecules extracted in a solvent such as methanol or water. This technique isolates organic molecules from insoluble aerosol components like BC and mineral dust. This method reduces interference in light absorption measurements due to non-BrC components and is cost-effective, making it practical for locations where deploying in situ instrumentation is expensive. However, particle-phase light-absorption properties inferred from solvent-extracted BrC will have artifacts due to size-dependent absorption by aerosol particles and inefficient organic extraction by different solvents.23,24
Two-dimensional gas chromatography (GC × GC) is a powerful technique that provides a comprehensive chemical characterization of compounds by separating them according to both polarity and volatility. This approach has proven effective in characterizing biomass burning (BB) emissions from laboratory combustion experiments.25–27 While progress has been made in characterizing the chemical composition of wildfire emissions,28–31 a better understanding of how different chemical groups such as polycyclic aromatic hydrocarbons (PAHs), nitro- and oxygenated-aromatics, and low volatility compounds, relate to OA optical properties is still needed.11,32 Although nitro-aromatics and PAHs are important chromophores in BrC, these compounds account for less than half of the total BrC light-absorption, suggesting that other compound groups could serve as better predictors for BrC.12,33–35 Saleh et al.36,37 have proposed that the volatility of organics—characterized in aerosol plumes with BC fractions as a proxy—can better predict BrC optical properties. Through laboratory experiments, they demonstrated that organics darken as their volatility decreases, and they demonstrated that controlling combustion conditions, such as temperature and the fuel-to-air ratios, can result in BrC with predictable optical properties.38 Increased molecular collisions during high temperature combustion can lead to the formation of large, highly-conjugated molecules with lower volatilities.39,40 These low-volatility molecules are likely to strongly absorb ultraviolet and visible light.6,41 However, studies supporting this hypothesis from real-world biomass burning emissions are scarce.42 Furthermore, a comprehensive understanding of the relationship between the chemical composition of these low volatility compounds and BrC optical properties is still lacking.
Donahue et al.43 established a volatility basis set to classify organic molecules based on their saturation mass concentrations at ambient temperatures. A thermal-optical analysis (TOA)44 can be used to quantify and partition organics with different volatilities.37,45 Additionally, the parametrizations from Li et al.46 can be used to estimate the volatility of the molecules constituting an aerosol based on their molecular composition. Specific compound groups can then be correlated with BrC spectrophotometric measurements to ascertain their importance in determining the optical properties of BrC. In this study, we measured the spectral light-absorption and chemical composition of organic emissions from wildfires sampled during the August 2019 Fire Influence on Regional to Global Environments and Air Quality (FIREX-AQ) campaign. Ground-level sampling was performed at the Nethker fire in Idaho, the Castle and Ikes fires in Arizona, and the 204 Cow fire in eastern Oregon. We examined the dependence of the methanol-soluble (MeS) and water-insoluble (WI) BrC light-absorption coefficients on concentrations of low volatility organic carbon and observed a positive correlation with both components. Additionally, through multivariate regression analysis, we identified nitrogen-containing compounds (NOC), aromatics, and PAHs as the best predictors for the optical properties of the sampled BrC.
2 Materials and methods
2.1 Particle sampling
During the 2019 FIREX-AQ campaign, we collected aerosol emissions on quartz-fiber filters from three fire locations across the western US. The ground-based Aerodyne Mobile Laboratory (AML) was used for all sampling activities, and the identification of biomass burning plumes and filter sampling procedures aboard the AML are described in detail in Sumlin et al.47 and Liang et al.28 We collected a total of 33 time-resolved (∼1 hour) emissions on 47 mm quartz-fiber filters (Pallflex Tissuquartz) during August 2019 from four wildfires across 3 locations: Nethker fire (Idaho), 204 Cow fire (Oregon), and Castle and Ikes fires (Arizona). The filters included 6 background samples, 2 field blanks and 1 sample from a stationary site in McCall, Idaho. Prior to sampling, we passed the aerosol emissions through a 2.5 μm cyclone at a flow rate of 10 L min−1 to eliminate larger particles. Subsequently, we stored the collected filters at −20 °C until analysis. Further information regarding the sample collection dates and times is available in Table S1 of the ESI.†
2.2 Sample preparation for optical analysis
To estimate BrC light absorption coefficients, a couple of ¼” diameter punches were taken from each of the 33 filters for solvent extraction. The particle-laden filter punches were placed in amber vials along with 800 μL of either water or methanol and sonicated for 1 hour. After extraction, the solvent extracts were passed through Teflon membrane syringe filters (PTFE, 0.22 μm, Fisherbrand) to remove any suspended insoluble particles introduced during the extraction process. Disregarding the blank and background samples, a total of 24 filters were analyzed to estimate BrC light absorption coefficients, with 22 data points available for water-soluble BrC due to the loss of two samples during water extraction.
2.3 Calculation of optical properties
The spectral light absorbance at a given wavelength λ (Aλ) of the solvent-extracted BrC was measured using a UV-Vis spectrophotometer (LAMBDA 35, PerkinElmer) at wavelengths ranging from 350 nm to 800 nm with a resolution of 1 nm. We used the Aλ value to calculate the BrC light absorption coefficient (BrC Abs) using the equation:24 |  | (1) |
where Vl is the volume of solvent used for extraction, Va is the volume of air sampled through the filter punch area, and l is the optical path length traveled by the beam (1 cm). The logarithm term is used to convert base 10 logarithm from the absorbance measurements to natural logarithm. The Aλ values were normalized per the absorbance measurements at 700 nm to account for signal drift within the instrument. The equation assumes that solvent-extracted BrC does not absorb light at 700 nm.
The MeS BrC Abs405 and WS BrC Abs405 values were estimated by measuring the absorbance of organics extracted in methanol and water, respectively. The WI BrC Abs405 values were estimated by subtracting WS BrC Abs405 from MeS BrC Abs405. These calculations assume that the WS BrC are a component of the MeS BrC.48,49 However, recent studies have indicated that a significant fraction of highly light-absorbing BrC may be insoluble in both methanol and water.50,51 Therefore, it should be noted that the WI BrC Abs405 values reported here represent the MeS–WI fraction on BrC. For clarity and readability, we will refer to the MeS–WI fraction of BrC as simply WI BrC throughout the manuscript.
2.4 Compound identification and quantification
The analysis technique and quantification of compounds are described in detail in Liang et al.28 Briefly, small punches were taken from each 47 mm filter and isotopically labeled internal standards were added. Each punch was then placed in a thermal desorption autosampler to desorb compounds in helium at 320 °C. Online derivatization of the compounds was done using MSTFA (N-methyl-N-(trimethylsilyl)trifluoroacetamide). The analytes were subsequently sent to a GC × GC system, where the first column separated the compounds based on their volatility, and the second column separated them based on polarity. A Tofwerk high-resolution (m/Δm ≈ 4000) time-of-flight mass spectrometer (HR ToF-MS) was used to detect compounds which were ionized using electron impact ionization (70 eV).
A total of 198 compounds were separated in the combined GC columns and were classified into 11 groups based on their functionality. These groups include mono-carboxylic acids, sugars, aromatics (monocyclic only), alcohols, alkanes, terpenoids, sulfur-containing compounds, PAHs, NOCs, other oxygenated compounds, and a group of compounds with unknown functional groups. All identified compounds and their corresponding groups are listed in Table S2 of the ESI.† The quantification procedure for individual compounds is detailed in Jen et al.27 and Liang et al.28 The individual compounds were quantified by generating a response curve for each compound using a 142-compound standard mixture and internal standards run on a blank filter. The compounds present in the filter sample were quantified using their corresponding response curves. If a compound was not present in the standard mixture, the response curve of a compound with a similar group nearest to it in the GC × GC space was used.
2.5 Volatility of aerosol constituents
The OC and EC concentrations along with the thermograms determining the OC–EC split were measured using a thermal-optical OC/EC analyzer (Model 5L, Sunset Lab), operated using the NIOSH870 protocol. Conventionally, volatility distributions of aerosol constituents are measured using a thermodenuder in tandem with an instrument to characterize OC concentrations.52 However, the peaks corresponding to different temperature ramp-ups during the thermal-optical analysis (TOA) can also be used to estimate the aerosol volatility distributions.53 Calculations detailing how the thermogram data were used to estimate the volatility distributions are provided in the ESI.† Volatility classifications estimated using this technique were termed as TOA-based classifications.
Li et al.46 analyzed over 30
000 compounds using a “molecular corridor” approach and developed parametrizations to predict the saturation mass concentration of organic compounds from their elemental composition. The parametrizations consist of a semi-empirical equation with different parameters as inputs based on the elements present in a particular molecule. We used these parametrizations with the data obtained from the HR ToF-MS as a secondary technique to predict the volatility distributions of the aerosols. Further details on how the parametrizations were used are provided in the ESI.†
3 Results and discussion
3.1 Volatility distribution of wildfire carbonaceous aerosols
Fig. 1 presents the volatilities of carbonaceous aerosols estimated from the thermogram and HR ToF-MS data for the wildfire emissions collected in this study. Samples were collected for the Nethker fire (n = 16) over 9 days, and the samples for 204 Cow (n = 3) and Castle/Ikes fires (n = 5) were collected over one day each. Fig. 1 shows data from filters with the highest mass loading for the 204 Cow and Castle/Ikes fires, as well as from the highest mass loaded filters collected at the start and end of sampling at the Nethker fire, labeled as early- and late-stage, respectively. To ensure a consistent comparison between the volatility bins estimated using two different methods, the OA mass was converted to OC mass for the ToF-MS data. This conversion allows for an equitable comparison of volatility bins between the two methods. While estimates of volatility from the ToF-MS data are likely to be more accurate than those from the TOA, the detailed molecular composition analysis missed more than 35% of OA, resulting in incomplete volatility estimates for those results.
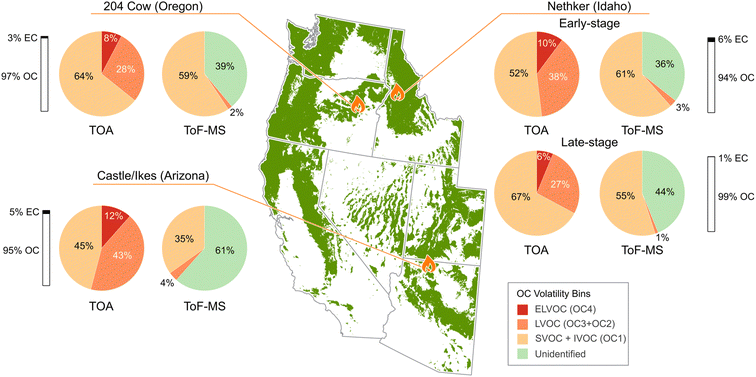 |
| Fig. 1 Volatility distributions of carbonaceous aerosols sampled across three wildfire locations in Idaho, Oregon, and Arizona. Filter samples were collected during the flaming-dominant and smoldering-dominant stages of the Nethker fire, and the results from these are labeled as early- and late-stage, respectively. The volatility bins were based on the volatility basis set defined by Donahue et al.43 and estimated using the organic carbon peaks obtained from Thermal-Optical Analysis (TOA) and the parametrizations by Li et al.46 used on the chemical speciation data obtained from the High-Resolution Time of Flight-Mass Spectrometry (HR ToF-MS) data. | |
The emissions were sampled at ground level aboard the Aerodyne Mobile Laboratory (AML). Aerosols were sampled close to the fire source with some of the samples collected when the AML was stationary and others collected when the AML was in motion.28,47 Comparisons in volatility were only made for samples collected when the AML was stationary, as it was difficult to estimate variations due to atmospheric processing with changing distance from the fire.
During the Nethker fire, the fraction of elemental carbon (EC) decreased from the early to late stage of the emissions, which is expected as soot emissions decrease as a fire decays.54,55 The OC1 peak from TOA can be considered to be a combination of semi-volatile organic compounds (SVOC) and intermediate volatility compounds (IVOC).28,53 Based on our calculations, the sum of OC2 and OC3 peaks represent low volatility organic compounds (LVOCs), and the OC4 peaks correspond to extremely low volatility organic compounds (ELVOCs).42,53 We were unable to differentiate between the IVOC and SVOC concentrations from the temperature protocol used during the TOA measurements. Results depicting the IVOC data from HR Tof-MS are presented in Fig. S2 in the ESI.† The low concentration of LVOCs and no ELVOCs measured using ToF-MS were expected due to these compounds not volatilizing at 320 °C.56,57 The unidentified compounds from the ToF-MS data are likely to be EL/LVOC molecules.
The SVOC + IVOC fraction from TOA was greater for the late-stage sample of the Nethker fire than the early stage. If the emissions were dominated by primary emissions, the difference may be due to the lower volatility organics being greater from flaming combustion than smoldering.58 Conversely, if the sampled emissions were dominated by secondary organics, greater reactivity of volatile organics in the optically denser aerosol plumes of the early-stage emissions could have led to formation of more lower volatility organics.59 However, as the plumes only travelled less than an hour before reaching the sampling locations,47 it is likely that the aerosols had undergone minimal atmospheric processing. ELVOC concentrations had the best correlation (R2 = 0.5) with EC. Previous studies have seen associations between EC and low volatility organics37,42 indicating that these components may be emitted concomitantly from wildfires. Large concentrations of EL/LVOC compounds were also associated with larger amount of unidentified compound concentrations, further indicating that the unidentified molecules likely have lower volatilities.
3.2 Dependence of BrC light absorptivity on low volatility OC and EC concentrations
In Fig. 2A and C, BrC light absorption coefficient at 405 nm (BrC Abs405) for the MeS and WI component of OA are plotted against the ToF-MS-based LVOC concentration. In Fig. 2B and D, BrC Abs405 for both the MeS and WI BrC components are plotted against the TOA-based ELVOC concentration. The color bar represents the EC concentrations obtained from TOA. The black solid lines in each panel show linear fits to the data, and the gray shaded regions display the 95% confidence interval of the fit. Among the different volatility groups, we observed the strongest correlations for the MeS and WI BrC Abs405 with the ToF-MS-based LVOC concentration and the TOA-based ELVOC concentration. Samples with higher EC concentrations had greater light absorption coefficients at similar EL/LVOC concentrations. Phillips and Smith60 posit that methanol extraction may also extract BC, resulting in greater light absorption measurements for MeS BrC. However, studies that do not use solvent-extraction techniques still find a relationship between light absorptivity of BrC from BB and the BC content of the aerosol.37,61,62 It has been proposed that the high-temperatures during flaming combustion produce conditions that are optimal for the production of BrC with greater light-absorptivity.38,63 Our findings corroborate this hypothesis for fresh wildfire emissions using volatility measurements obtained from two separate techniques.
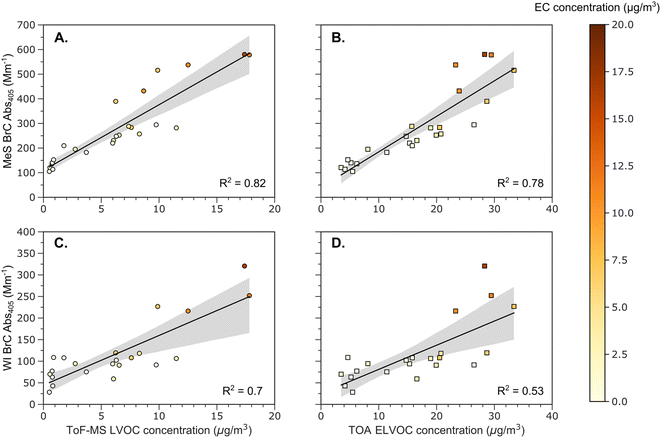 |
| Fig. 2 Dependence of the light-absorption coefficient at 405 nm by the methanol-soluble and water-insoluble components of BrC on (A and C) the low volatility OA concentrations estimated from the HR ToF-MS data and (B and D) the extremely low volatility OC concentrations estimated using TOA. The solid black lines represent linear fits to the data with the shaded region representing 95% confidence intervals. The color bar depicts the EC concentration for each sample obtained from TOA. | |
The WI BrC Abs405 accounted for 43 ± 11% of the MeS BrC Abs405, which is consistent with findings from previous studies.64,65 The observed correlations between the BrC light absorption coefficient and low volatility organic concentration were weaker for the WI component of BrC. This weaker correlation could be due to the incomplete measurement of WI BrC Abs405 or the dependence of light absorptivity on factors in addition to the volatility of molecules comprising the aerosol. Comprehensive measurements involving multiple instruments and techniques such as photoacoustic spectrometers, single particle soot photometers, particle in liquid samplers, and electron energy loss spectroscopy6,64,66 in addition to solvent-extraction based methods are needed to provide a more complete understanding of the different solvent-soluble fractions of BrC. Knowledge of the WS fraction of BrC is important to understand the hygroscopic growth of these particles and their interaction with clouds and the hydrologic cycle.67,68
Previous studies have observed an increase in the water-insoluble BrC with the EC concentration of the aerosol for urban samples49,69 indicating that the two have similar sources. To corroborate if this relationship holds true for wildfire emissions, we investigated the dependence of WI BrC Abs405 on EC concentration in Fig. 3. Similar to the urban samples, we observed an increase in the water-insoluble BrC Abs405 values with the EC concentration of the aerosol, suggesting that both components have similar sources from wildfires as well. Additionally, we performed a Johnson–Neyman analysis on the data to observe the moderating effect of EL/LVOC concentrations on WI BrC Abs405 with EC. The Johnson–Neyman analysis provides the range for a moderating variable over which the effect of a predictor variable on the output is significant. Details on how the Johnson–Neyman analysis was performed are in the ESI.† We observed that the dependence of WI BrC Abs405 on EC concentration was significant (p value = 0.01) at ToF-MS based LVOC concentrations above 8 μg m−3 and TOA-based ELVOC concentrations above 20.2 μg m−3. This suggests that the increase in WI BrC Abs405 with EC is likely due to an increase in EL/LVOC concentrations, which are also likely to be water-insoluble. The Johnson–Neyman analysis provides further support for the hypothesis that the combustion conditions which lead to the formation of WI BrC are also conducive to the formation of EL/LVOC and EC.38,70 However, it is important to note that combustion of different biomass fuels might form organics with different properties, so the EL/LVOC concentrations obtained from the Johnson–Neyman analysis might not be applicable to other combustion systems. As our values for the WI BrC Abs405 are likely biased low, it is reasonable to assume that a majority of BrC chromophores for the investigated fires could be water-insoluble. Instruments such as a particle into liquid sampler primarily captures water-soluble BrC and would miss out strongly light-absorbing chromophores that are insoluble in water.66 Studies which only use WS BrC to estimate the radiative effects of biomass burning BrC would severely underpredict these values.48,71
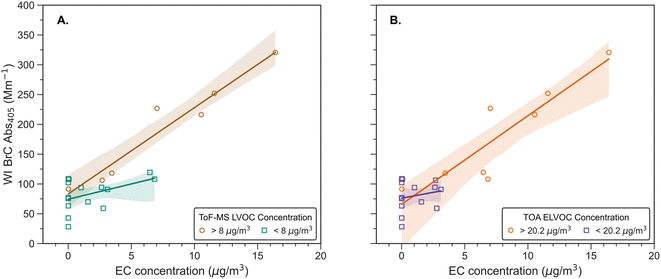 |
| Fig. 3 Dependence of the water-insoluble (WI) BrC light absorption coefficient at 405 nm (Abs405) on EC concentration. The different panels represent regression fits estimated based on the Johnson–Neyman analysis using (A) the ToF-MS based LVOC concentrations and (B) the TOA based ELVOC concentrations as the moderating variables. The circles represent data points for which a change in EC had a significant impact on WI BrC Abs405 and squares represent data where change in EC had minimal effect on WI BrC Abs405. The solid lines are linear fit lines, and the shaded regions represent 95% confidence intervals. | |
3.3 Association between BrC light absorptivity and chemical compound groups
Establishing a relationship between various chemical compound classes and BrC light absorptivity is crucial for predicting how alterations in the chemical composition of aerosols may impact their optical properties. Table 1 presents the correlation coefficient (R2) values obtained from a linear correlation between MeS and WS BrC Abs405 and various chemical compound classes that had strong correlations with biomass burning BrC or were identified as significant chromophores in previous studies. The previously recognized chromophores consisted of NOCs which were primarily nitroaromatics, aromatic compounds mainly comprised of oxygenated aromatics, and PAHs which included substituted and oxygenated compounds. Our analysis revealed that the aromatics had the best correlation with MeS and WS BrC Abs405. Previous studies have noted that oxygenated aromatics are important contributors to BB secondary OA32,72 and BrC in urban aerosols.33,73 Our findings suggest that oxygenated aromatic compounds are also present in wildfire emissions and could act as BrC chromophores. In contrast to previous studies, PAHs and NOCs demonstrated weaker correlations with BrC light absorption coefficients.32,34 Nitroaromatics are primarily generated in dark plumes or during nighttime oxidation chemistry.59,74 Since the wildfire emissions in this study were sampled within an hour of being emitted, it is unlikely that they had undergone substantial atmospheric processing to produce large amounts of nitroaromatics.47 Based on their structure, the observed PAHs have likely formed due to the decomposition of terpenoids.28 Consequently, the PAHs may not be significantly present in the OA emissions, leading to a weak linear correlation with BrC light absorptivity. Our study primarily examines BrC from fresh wildfire emissions, and it is probable that the correlation coefficients may change as the aerosols undergo further atmospheric processing. The linear fits using the WS BrC Abs405 exhibited correlation coefficients that were equal to or greater than those obtained with the MeS BrC Abs405. The MeS fraction of BrC is likely to be composed of compounds that remained unidentified using our chemical characterization method. Consequently, the identified compound groups would be better predictors for the WS component of BrC than the MeS component. Moreover, the correlations were even weaker with WI BrC Abs405 than those with the MeS BrC Abs405. The subset of MeS and WI organics are probably better represented by compounds that remained unquantified with our chemical characterization method.
Table 1 Correlation coefficient (R2) values for linear fits of the methanol- and water-soluble components of BrC to the different identified compound classes
Compound class |
MeS BrC Abs405 (Mm−1) |
WS BrC Abs405 (Mm−1) |
PAH |
0.32 |
0.46 |
Aromatic |
0.52 |
0.58 |
NOC |
0.33 |
0.32 |
Terpenoid |
0.55 |
0.65 |
Sugar |
0.67 |
0.68 |
In addition to the previously known chromophores, we also observed correlations between BrC light absorptivity and sugars and terpenoids. Levoglucosan was dominant in the characterized sugar concentrations, and it is a well-known tracer for fresh biomass burning OA emissions.75 Numerous previous studies have observed correlations between levoglucosan and BrC from biomass combustion.76–78 A growing number of studies show that levoglucosan may not be stable in the atmosphere for long periods of time79–81 which further suggests that we sampled BB plumes that had undergone minimal atmospheric processing. The quantified terpene concentrations were comparable to the sugar concentrations and were likely formed from heat-induced volatilization of unburnt biomass in the wildfires.28 Terpenes are reactive toward ozone and should deplete quickly from BB plumes,82 so the high concentrations in our samples indicate that we sampled primary emissions. The correlation with BrC Abs405 is likely due to the terpenes and terpenoids acting as biomass burning indicators for primary emissions. Oxidation of terpenes has been shown to produce secondary BrC;83,84 however, as the samples underwent minimal atmospheric processing, it is unlikely that the correlation with BrC Abs405 is due to secondary BrC.
To determine the combined contribution of the different compound groups to BrC Abs405, we conducted a multivariate regression analysis using all 11 quantified compound classes. A synergistic combination of PAHs, aromatics, and NOCs was found to be a significant (p < 0.05) predictor of BrC light absorption after we removed groups which were linearly correlated. Fig. 4 depicts a comparison of the measured MeS and WS BrC Abs405 values with values predicted from the multivariate regression analysis. The different compound classes were unable to predict the WI BrC Abs405 well, and as a result that regression is not presented here. There was a significant improvement in the adjusted R2 values for the multivariate regressions as compared to the individual linear fits described in Table 1. The improvement is expected as BrC would constitute multiple molecules from different compounds groups. It is worth noting that the homoscedasticity (a measure of the residuals along with the value of the dependent variable) and R2 are improved when the NOC values are transformed to their square roots, likely due to their uneven distribution of concentrations. The regression coefficients with the transformed NOC values are presented in Table S4.† The regression coefficients for the NOCs were higher than those for the other compound groups, likely due to their low concentration among the quantified compounds. While the PAHs and NOCs were individually poor predictors of BrC Abs405, a combination of PAHs, aromatics, and NOCs was good at predicting BrC light absorption coefficients. The aforementioned three compound groups have been shown to be efficient light absorbers,85–87 so the poorer correlations with individual groups are likely a result of different concentrations of the compound classes in the sampled plumes. It is informative to discover that the correlation between BrC and these compound classes can be observed using even a subset of the chemical compounds as well. Similar to the direct linear correlations, the multivariate regression analysis worked better for predicting WS BrC Abs405 as compared to the MeS component. Oxygenated aromatics and NOCs have been shown to constitute a significant fraction of water-soluble chromophores,88 so it is expected that they contribute to WS BrC Abs405. However, PAHs are expected to be water-insoluble and should have better correlations with the MeS BrC Abs405.89 The structures of the PAHs quantified in this study suggest that they might be decomposition products from the measured terpenoids,28 which might be the reason for the weaker correlations with the MeS BrC Abs405. While our correlations are informative for predicting BrC absorption coefficients in this study, they are likely not applicable to BrC from other combustion emissions.
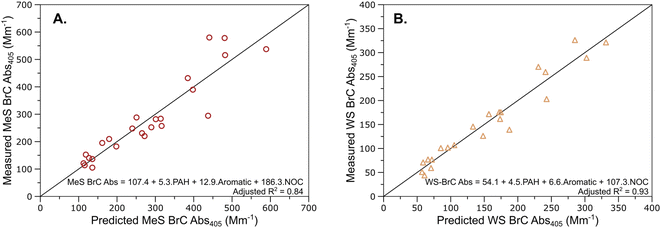 |
| Fig. 4 Measured values of (A) MeS BrC Abs405 and (B) WS BrC Abs405 compared to the values as predicted by a multivariate regression analysis using PAHs, aromatics, and NOCs as the independent variables. There was significant improvement in the fit correlation coefficient as compared to simple linear regressions with the individual groups as shown in Table 1. The black diagonal corresponds to the 1 : 1 equivalence line. | |
4 Conclusions
This study aimed to investigate the optical properties of BrC from western US wildfires, with a focus on low volatility aerosols and different chemical compound classes. Consistent with prior laboratory-based biomass burning studies, we found a strong association between the low and extremely low volatility organics and solvent-extracted BrC light absorption coefficients for fresh real-world wildfires emissions. WI BrC was strongly correlated with EC concentrations, which suggests that organics emitted during high-temperature or flaming-dominated combustion might be water-insoluble. Our findings imply that the amount of WI BrC emitted from wildfires may decrease as the combustion-stage of a fire progresses from flaming to smoldering. Future studies could focus on the change in PAH, NOC, and aromatic concentrations in a wildfire plume as these were identified as important BrC chromophores for the sampled wildfires. Our observations were limited to measurements close to the fire source and provided information for aerosols that had undergone minimal atmospheric processing. Future studies could track wildfire plumes to determine whether the dependence of BrC light absorptivity on volatility remains applicable as aerosols undergo additional reactions in the atmosphere. Our measurements were based on solvent-extraction-based methods, which may underestimate the BrC emissions due to incomplete extraction of organics. Future studies could employ a combination of online and offline measurements to better quantify BrC emissions.
Author contributions
N. S. and R. K. C. designed research; N. S., Y. L., B. S., C. D., and S. H. performed the field sampling experiments; N. S., P. L., Y. L., A. H. G., and R. K. C. analyzed data; N. S. and R. K. C. wrote the original draft incorporating edits from all co-authors.
Conflicts of interest
The authors declare no conflicts of interest.
Acknowledgements
The research has been supported by National Oceanic and Atmospheric Administration (grant no. NA16OAR4310104 and NA16OAR4310107) and National Science Foundation (grant no. AGS-1455215 and AGS-1926817). The authors would like to acknowledge Christos Stamatis from UC Riverside for collecting the filters during FIREX-AQ. The authors would also like to thank Rob Roscioli, Edward Fortner, and Jordan Krechmer of Aerodyne Research Inc. for processing AML data during the FIREX-AQ campaign.
References
- J. C. Chow, J. G. Watson, D. H. Lowenthal, L.-W. A. Chen and N. Motallebi, J. Air Waste Manage. Assoc., 2010, 60, 497–507 CrossRef CAS PubMed.
- M. Evans, N. Kholod, T. Kuklinski, A. Denysenko, S. J. Smith, A. Staniszewski, W. M. Hao, L. Liu and T. C. Bond, Atmos. Environ., 2017, 163, 9–21 CrossRef CAS.
- R. Chakrabarty, H. Moosmüller, L.-W. Chen, K. Lewis, W. Arnott, C. Mazzoleni, M. Dubey, C. Wold, W. Hao and S. Kreidenweis, Atmos. Chem. Phys., 2010, 10, 6363–6370 CrossRef CAS.
- T. C. Bond, S. J. Doherty, D. Fahey, P. Forster, T. Berntsen, B. DeAngelo, M. Flanner, S. Ghan, B. Kärcher and D. Koch, J. Geophys. Res. Atmos., 2013, 118, 5380–5552 CrossRef CAS.
- B. J. Sumlin, Y. W. Heinson, N. Shetty, A. Pandey, R. S. Pattison, S. Baker, W. M. Hao and R. K. Chakrabarty, J. Quant. Spectrosc. Radiat. Transfer, 2018, 206, 392–398 CrossRef CAS.
- D. T. Alexander, P. A. Crozier and J. R. Anderson, Science, 2008, 321, 833–836 CrossRef CAS PubMed.
- P. Shamjad, S. Tripathi, N. M. Thamban and H. Vreeland, Sci. Rep., 2016, 6, 1–7 CrossRef PubMed.
- R. Washenfelder, A. Attwood, C. Brock, H. Guo, L. Xu, R. Weber, N. Ng, H. Allen, B. Ayres and K. Baumann, Geophys. Res. Lett., 2015, 42, 653–664 CrossRef CAS.
- U. Pöschl, Angew. Chem., Int. Ed., 2005, 44, 7520–7540 CrossRef PubMed.
- A. Laskin, J. Laskin and S. A. Nizkorodov, Chem. Rev., 2015, 115, 4335–4382 CrossRef CAS PubMed.
- A. P. S. Hettiyadura, V. Garcia, C. Li, C. P. West, J. Tomlin, Q. He, Y. Rudich and A. Laskin, Environ. Sci. Technol., 2021, 55, 2511–2521 CrossRef CAS PubMed.
- P. Lin, P. K. Aiona, Y. Li, M. Shiraiwa, J. Laskin, S. A. Nizkorodov and A. Laskin, Environ. Sci. Technol., 2016, 50, 11815–11824 CrossRef CAS PubMed.
- R. A. Di Lorenzo, R. A. Washenfelder, A. R. Attwood, H. Guo, L. Xu, N. L. Ng, R. J. Weber, K. Baumann, E. Edgerton and C. J. Young, Environ. Sci. Technol., 2017, 51, 3128–3137 CrossRef CAS PubMed.
- J. P. Wong, A. Nenes and R. J. Weber, Environ. Sci. Technol., 2017, 51, 8414–8421 CrossRef CAS PubMed.
- R. A. Di Lorenzo and C. J. Young, Geophys. Res. Lett., 2016, 43, 458–465 CrossRef CAS.
- J. P. S. Wong, M. Tsagkaraki, I. Tsiodra, N. Mihalopoulos, K. Violaki, M. Kanakidou, J. Sciare, A. Nenes and R. J. Weber, Atmos. Chem. Phys., 2019, 19, 7319–7334 CrossRef CAS.
- W. Jiang, L. Ma, C. Niedek, C. Anastasio and Q. Zhang, ACS Earth Space Chem, 2023, 1107–1119 CrossRef CAS PubMed.
- N. Y. Kasthuriarachchi, L.-H. Rivellini, M. G. Adam and A. K. Lee, Environ. Sci. Technol., 2020, 54, 10808–10819 CrossRef CAS PubMed.
- Y. Desyaterik, Y. Sun, X. Shen, T. Lee, X. Wang, T. Wang and J. L. Collett Jr, J. Geophys. Res. Atmos., 2013, 118, 7389–7399 CrossRef CAS.
- Y. Chen, X. Ge, H. Chen, X. Xie, Y. Chen, J. Wang, Z. Ye, M. Bao, Y. Zhang and M. Chen, Atmos. Environ., 2018, 187, 230–240 CrossRef CAS.
- Y. Chen, X. Xie, Z. Shi, Y. Li, X. Gai, J. Wang, H. Li, Y. Wu, X. Zhao and M. Chen, Atmos. Res., 2020, 244, 105028 CrossRef CAS.
- Y. M. Qin, H. B. Tan, Y. J. Li, Z. J. Li, M. I. Schurman, L. Liu, C. Wu and C. K. Chan, Atmos. Chem. Phys., 2018, 18, 16409–16418 CrossRef CAS.
- N. J. Shetty, A. Pandey, S. Baker, W. M. Hao and R. K. Chakrabarty, Atmos. Chem. Phys., 2019, 19, 8817–8830 CrossRef CAS.
- J. Liu, M. Bergin, H. Guo, L. King, N. Kotra, E. Edgerton and R. Weber, Atmos. Chem. Phys., 2013, 13, 12389–12404 CrossRef.
- L. E. Hatch, W. Luo, J. F. Pankow, R. J. Yokelson, C. E. Stockwell and K. Barsanti, Atmos. Chem. Phys., 2015, 15, 1865–1899 CrossRef CAS.
- L. E. Hatch, A. Rivas-Ubach, C. N. Jen, M. Lipton, A. H. Goldstein and K. C. Barsanti, Atmos. Chem. Phys., 2018, 18, 17801–17817 CrossRef CAS.
- C. N. Jen, L. E. Hatch, V. Selimovic, R. J. Yokelson, R. Weber, A. E. Fernandez, N. M. Kreisberg, K. C. Barsanti and A. H. Goldstein, Atmos. Chem. Phys., 2019, 19, 1013–1026 CrossRef CAS.
- Y. Liang, C. Stamatis, E. C. Fortner, R. A. Wernis, P. Van Rooy, F. Majluf, T. I. Yacovitch, C. Daube, S. C. Herndon and N. M. Kreisberg, Atmos. Chem. Phys., 2022, 22, 9877–9893 CrossRef CAS.
- S. Tomaz, T. Cui, Y. Chen, K. G. Sexton, J. M. Roberts, C. Warneke, R. J. Yokelson, J. D. Surratt and B. J. Turpin, Environ. Sci. Technol., 2018, 52, 11027–11037 CrossRef CAS PubMed.
- E. Schneider, H. Czech, O. Popovicheva, H. Lütdke, J. Schnelle-Kreis, T. Khodzher, C. P. Rüger and R. Zimmermann, ACS Earth Space Chem, 2022, 6, 1095–1107 CrossRef CAS.
- M. A. Brege, S. China, S. Schum, A. Zelenyuk and L. R. Mazzoleni, ACS Earth Space Chem, 2021, 5, 2729–2739 CrossRef CAS.
- B. B. Palm, Q. Peng, C. D. Fredrickson, B. H. Lee, L. A. Garofalo, M. A. Pothier, S. M. Kreidenweis, D. K. Farmer, R. P. Pokhrel and Y. Shen, Proc. Nat. Acad. Sci., 2020, 117, 29469–29477 CrossRef CAS PubMed.
- R.-J. Huang, L. Yang, J. Shen, W. Yuan, Y. Gong, J. Guo, W. Cao, J. Duan, H. Ni and C. Zhu, Environ. Sci. Technol., 2020, 54, 7836–7847 CrossRef CAS PubMed.
- L. T. Fleming, P. Lin, J. M. Roberts, V. Selimovic, R. Yokelson, J. Laskin, A. Laskin and S. A. Nizkorodov, Atmos. Chem. Phys., 2020, 20, 1105–1129 CrossRef CAS.
- P. Lin, L. T. Fleming, S. A. Nizkorodov, J. Laskin and A. Laskin, Anal. Chem., 2018, 90, 12493–12502 CrossRef CAS PubMed.
- R. Saleh, Curr. Pollut. Rep., 2020, 6, 90–104 CrossRef CAS.
- R. Saleh, E. S. Robinson, D. S. Tkacik, A. T. Ahern, S. Liu, A. C. Aiken, R. C. Sullivan, A. A. Presto, M. K. Dubey and R. J. Yokelson, Nature Geosci, 2014, 7, 647 CrossRef CAS.
- R. Saleh, Z. Cheng and K. Atwi, Environ. Sci. Technol. Lett., 2018, 5, 508–513 CrossRef CAS.
- R. J. Evans and T. A. Milne, Energy Fuels, 1987, 1, 123–137 CrossRef CAS.
- P. Desgroux, X. Mercier and K. A. Thomson, Proceedings of the Combustion Institute, 2013, 34, 1713–1738 CrossRef CAS.
- A. Hoffer, Á. Tóth, M. Pósfai, C. E. Chung and A. Gelencsér, Atmos. Meas. Tech., 2017, 10, 2353–2359 CrossRef CAS.
- M. M. Islam, S. E. Neyestani, R. Saleh and A. P. Grieshop, Aerosol Sci. Technol., 2022, 1–15 Search PubMed.
- N. M. Donahue, J. Kroll, S. N. Pandis and A. L. Robinson, Atmos. Chem. Phys., 2012, 12, 615–634 CrossRef CAS.
- J. C. Chow, J. G. Watson, L.-W. A. Chen, M. O. Chang, N. F. Robinson, D. Trimble and S. Kohl, J. Air Waste Manage. Assoc., 2007, 57, 1014–1023 CrossRef CAS PubMed.
- R. P. Pokhrel, N. L. Wagner, J. M. Langridge, D. A. Lack, T. Jayarathne, E. A. Stone, C. E. Stockwell, R. J. Yokelson and S. M. Murphy, Atmos. Chem. Phys., 2016, 16, 9549–9561 CrossRef CAS.
- Y. Li, U. Pöschl and M. Shiraiwa, Atmos. Chem. Phys., 2016, 16, 3327–3344 CrossRef CAS.
- B. Sumlin, E. Fortner, A. Lambe, N. J. Shetty, C. Daube, P. Liu, F. Majluf, S. Herndon and R. K. Chakrabarty, Atmos. Chem. Phys., 2021, 21, 11843–11856 CrossRef CAS.
- R. Satish, N. Rastogi, A. Singh and D. Singh, Environ. Sci. Pollut. Res., 2020, 27, 33339–33350 CrossRef CAS PubMed.
- X. Zhang, Y.-H. Lin, J. D. Surratt and R. J. Weber, Environ. Sci. Technol., 2013, 47, 3685–3693 CrossRef CAS PubMed.
- Z. Bai, L. Zhang, Y. Cheng, W. Zhang, J. Mao, H. Chen, L. Li, L. Wang and J. Chen, Environ. Sci. Technol., 2020, 54, 14889–14898 CrossRef CAS PubMed.
- K. Atwi, Z. Cheng, O. El Hajj, C. Perrie and R. Saleh, Environ. Sci.: Atmos., 2022, 182–191 CAS.
- J. J. Devi, M. H. Bergin, M. Mckenzie, J. J. Schauer and R. J. Weber, Atmos.
Environ., 2016, 136, 95–104 CrossRef CAS.
- J. Ma, X. Li, P. Gu, T. R. Dallmann, A. A. Presto and N. M. Donahue, Aerosol Sci. Technol., 2016, 50, 638–651 CrossRef CAS.
- L. Hong, G. Liu, L. Zhou, J. Li, H. Xu and D. Wu, Particuology, 2017, 31, 181–190 CrossRef CAS.
- L.-W. A. Chen, H. Moosmüller, W. P. Arnott, J. C. Chow, J. G. Watson, R. A. Susott, R. E. Babbitt, C. E. Wold, E. N. Lincoln and W. M. Hao, Environ. Sci. Technol., 2007, 41, 4317–4325 CrossRef CAS PubMed.
- E. E. Louvaris, K. Florou, E. Karnezi, D. K. Papanastasiou, G. I. Gkatzelis and S. N. Pandis, Atmos. Environ., 2017, 158, 138–147 CrossRef CAS.
- R. Saleh, E. S. Robinson, A. T. Ahern and N. M. Donahue, Aerosol Sci. Technol., 2017, 51, 501–508 CrossRef CAS.
- J. Huffman, K. Docherty, C. Mohr, M. Cubison, I. Ulbrich, P. Ziemann, T. Onasch and J. Jimenez, Environ. Sci. Technol., 2009, 43, 5351–5357 CrossRef CAS PubMed.
- Z. C. J. Decker, M. A. Robinson, K. C. Barsanti, I. Bourgeois, M. M. Coggon, J. P. DiGangi, G. S. Diskin, F. M. Flocke, A. Franchin, C. D. Fredrickson, G. I. Gkatzelis, S. R. Hall, H. Halliday, C. D. Holmes, L. G. Huey, Y. R. Lee, J. Lindaas, A. M. Middlebrook, D. D. Montzka, R. Moore, J. A. Neuman, J. B. Nowak, B. B. Palm, J. Peischl, F. Piel, P. S. Rickly, A. W. Rollins, T. B. Ryerson, R. H. Schwantes, K. Sekimoto, L. Thornhill, J. A. Thornton, G. S. Tyndall, K. Ullmann, P. Van Rooy, P. R. Veres, C. Warneke, R. A. Washenfelder, A. J. Weinheimer, E. Wiggins, E. Winstead, A. Wisthaler, C. Womack and S. S. Brown, Atmos. Chem. Phys., 2021, 21, 16293–16317 CrossRef CAS.
- S. M. Phillips and G. D. Smith, Aerosol Sci. Technol., 2017, 51, 1113–1121 CrossRef CAS.
- G. Adler, N. L. Wagner, K. D. Lamb, K. M. Manfred, J. P. Schwarz, A. Franchin, A. M. Middlebrook, R. A. Washenfelder, C. C. Womack and R. J. Yokelson, Aerosol Sci. Technol., 2019, 53, 976–989 CrossRef CAS.
- C. D. McClure, C. Y. Lim, D. H. Hagan, J. H. Kroll and C. D. Cappa, Atmos. Chem. Phys., 2020, 20, 1531–1547 CrossRef CAS.
- Y. Chen and T. Bond, Atmos. Chem. Phys., 2010, 10, 1773–1787 CrossRef CAS.
- L. Zeng, J. Dibb, E. Scheuer, J. M. Katich, J. P. Schwarz, I. Bourgeois, J. Peischl, T. Ryerson, C. Warneke and A. E. Perring, Atmos. Chem. Phys. Discuss., 2022, 1–45 Search PubMed.
- P. Lin, J. Liu, J. E. Shilling, S. M. Kathmann, J. Laskin and A. Laskin, Phys. Chem. Chem. Phys., 2015, 17, 23312–23325 RSC.
- L. Zeng, A. P. Sullivan, R. A. Washenfelder, J. Dibb, E. Scheuer, T. L. Campos, J. M. Katich, E. Levin, M. A. Robinson and R. J. Weber, Atmos. Meas. Tech., 2021, 14, 6357–6378 CrossRef CAS.
- Y. Feng, V. Ramanathan and V. Kotamarthi, Atmos. Chem. Phys., 2013, 13, 8607–8621 CrossRef.
- V. Ramanathan, C. Chung, D. Kim, T. Bettge, L. Buja, J. T. Kiehl, W. M. Washington, Q. Fu, D. R. Sikka and M. Wild, Proc. Nat. Acad. Sci., 2005, 102, 5326–5333 CrossRef CAS PubMed.
- S. Park, G.-H. Yu and S. Lee, Atmos. Res., 2018, 203, 16–27 CrossRef CAS.
- N. K. Kumar, J. C. Corbin, E. A. Bruns, D. Massabó, J. G. Slowik, L. Drinovec, G. Močnik, P. Prati, A. Vlachou, U. Baltensperger, M. Gysel, I. El-Haddad and A. S. H. Prévôt, Atmos. Chem. Phys., 2018, 18, 17843–17861 CrossRef CAS.
- P. Chen, S. Kang, L. Tripathee, K. Ram, M. Rupakheti, A. K. Panday, Q. Zhang, J. Guo, X. Wang and T. Pu, Environ. Pollut., 2020, 261, 114239 CrossRef CAS PubMed.
- J. P. Haynes, K. E. Miller and B. J. Majestic, Environ. Sci. Technol., 2018, 53, 682–691 CrossRef PubMed.
- J. Li, Q. Zhang, G. Wang, J. Li, C. Wu, L. Liu, J. Wang, W. Jiang, L. Li and K. F. Ho, Atmos. Chem. Phys., 2020, 20, 4889–4904 CrossRef CAS.
- H. Jiang, A. L. Frie, A. Lavi, J. Y. Chen, H. Zhang, R. Bahreini and Y.-H. Lin, Environ. Sci. Technol. Lett., 2019, 6, 184–190 CrossRef CAS.
- A. Sullivan, A. Holden, L. Patterson, G. McMeeking, S. Kreidenweis, W. Malm, W. Hao, C. Wold and J. Collett Jr, J. Geophys. Res. Atmos., 2008, 113, D22 Search PubMed.
- J. M. Rincón-Riveros, M. A. Rincón-Caro, A. P. Sullivan, J. F. Mendez-Espinosa, L. C. Belalcazar, M. Quirama Aguilar and R. Morales Betancourt, Atmos. Chem. Phys., 2020, 20, 7459–7472 CrossRef.
- A. P. Sullivan, R. P. Pokhrel, Y. Shen, S. M. Murphy, D. W. Toohey, T. Campos, J. Lindaas, E. V. Fischer and J. L. Collett Jr, Atmos. Chem. Phys., 2022, 22, 13389–13406 CrossRef CAS.
- Y. Cheng, Q.-q. Yu, X.-b. Cao, C.-q. Yan, Y.-j. Zhong, Z.-y. Du, L.-l. Liang, W.-l. Ma, H. Qi and M. Zheng, Environ. Sci. Technol. Lett., 2021, 8, 732–738 CrossRef CAS.
- J. P. Wong, M. Tsagkaraki, I. Tsiodra, N. Mihalopoulos, K. Violaki, M. Kanakidou, J. Sciare, A. Nenes and R. J. Weber, Atmos. Chem. Phys., 2019, 19, 7319–7334 CrossRef CAS.
- D. Hoffmann, A. Tilgner, Y. Iinuma and H. Herrmann, Environ. Sci. Technol., 2010, 44, 694–699 CrossRef CAS PubMed.
- V. Pratap, Q. Bian, S. A. Kiran, P. K. Hopke, J. R. Pierce and S. Nakao, Atmos. Environ., 2019, 199, 224–232 CrossRef CAS.
- Z. C. Decker, K. J. Zarzana, M. Coggon, K.-E. Min, I. Pollack, T. B. Ryerson, J. Peischl, P. Edwards, W. P. Dubé and M. Z. Markovic, Environ. Sci. Technol., 2019, 53, 2529–2538 CrossRef CAS PubMed.
- D. L. Bones, D. K. Henricksen, S. A. Mang, M. Gonsior, A. P. Bateman, T. B. Nguyen, W. J. Cooper and S. A. Nizkorodov, J. Geophys. Res. Atmos., 2010, 115, D5 CrossRef.
- C. J. Kampf, A. Filippi, C. Zuth, T. Hoffmann and T. Opatz, Phys. Chem. Chem. Phys., 2016, 18, 18353–18364 RSC.
- Y. Kuang, J. Shang and Q. Chen, J. Hazard. Mater., 2021, 413, 125406 CrossRef CAS PubMed.
- J. Xu, A. P. S. Hettiyadura, Y. Liu, X. Zhang, S. Kang and A. Laskin, Environ. Sci. Technol. Lett., 2022, 9, 219–225 CrossRef CAS.
- M. Xie, X. Chen, M. D. Hays and A. L. Holder, Atmos. Chem. Phys., 2019, 19, 2899–2915 CrossRef CAS PubMed.
- Y. Zhou, C. P. West, A. P. Hettiyadura, W. Pu, T. Shi, X. Niu, H. Wen, J. Cui, X. Wang and A. Laskin, Environ. Sci. Technol., 2022, 56, 4173–4186 CrossRef CAS PubMed.
- C. S. Zhu, L. J. Li, H. Huang, W. T. Dai, Y. L. Lei, Y. Qu, R. J. Huang, Q. Y. Wang, Z. X. Shen and J. J. Cao, J. Geophys. Res. Atmos., 2020, 125, e2020JD032666 CAS.
Footnotes |
† Electronic supplementary information (ESI) available. See DOI: https://doi.org/10.1039/d3ea00067b |
‡ Present address: Department of Civil and Environmental Engineering, Virginia Tech, Blacksburg, VA 24061, USA. |
§ Present address: Institute of Chemical Physics, School of Chemistry and Chemical Engineering, Beijing Institute of Technology, Beijing 100081, China. |
|
This journal is © The Royal Society of Chemistry 2023 |
Click here to see how this site uses Cookies. View our privacy policy here.