Traffic, marine ships and nucleation as the main sources of ultrafine particles in suburban Shanghai, China†
Received
25th June 2023
, Accepted 7th October 2023
First published on 26th October 2023
Abstract
The health effects associated with ultrafine particles (UFPs) have received significant attention. Precisely identifying the sources of UFPs and quantifying their contributions pose considerable challenges. In this study, simultaneous observations were conducted at two suburban sites in Shanghai, a megacity in China, to measure the particle number size distributions (PNSD) and investigate the characteristics of UFPs. The application of the non-negative matrix factorization (NMF) algorithm on PNSD determines 5 factors at each site. The sources of UFPs are very different between the inland (Dianshan Lake, DSL) site and the seacoast (Dongtan, DT) site. By analyzing size distributions and daily variations of resolved factors, and examining their relationships with criteria pollutants and PM2.5 (particulate matter with diameters ≤2.5 μm) chemical compositions, we identify the main sources of UFPs. At the DSL site, fresh and aged traffic emissions and traffic nucleation are the main contributors to UFPs. At the DT site, photochemical nucleation and growth processes and marine ship emissions are the most important UFP sources. Industrial emissions and the regional background are characterized by a larger GMD (geometric mean diameter) and contribute more to particle volume concentrations (PVC) rather than particle number concentrations (PNC), indicating their association with PM2.5 rather than UFPs. These findings emphasize the underlying reasons why UFPs and PM2.5 belong to two different metrics. Concerning the respiratory deposition of particles, the main UFPs sources also pose the greatest health risk. Either during clean days (PM2.5 < 35 μg m−3) or during polluted days (PM2.5 > 35 μg m−3), PNCDeposits only varies slightly. And photochemical nucleation (i.e., new particle formation events) can significantly increase PNC deposited in the respiratory system.
Environmental significance
Shanghai is a Chinese megacity with bustling ports and well-developed industry. Efforts have been made to greatly reduce PM2.5 in Shanghai over the past decade, but it is crucial to recognize the health effects associated with ultrafine particles with diameters smaller than 100 nm. Traffic nucleation and emissions, marine ship emissions, and photochemical nucleation and growth processes are the main contributors to the particle number concentrations of ultrafine particles in suburban areas in Shanghai. The extent of their impact on respiratory deposits depends on their respective source profile of particle number size distribution. This understanding helps residents to take proactive measures and enables policymakers to develop targeted strategies for mitigating the health risks posed by ultrafine particles. Quantifying the sources of ultrafine particles is essential for a comprehensive health risk assessment by incorporating detailed toxicological analyses of the chemical compositions of ultrafine particles from different sources.
|
1. Introduction
Particulate matter pollution is a major public health risk to human beings.1 Exposure to PM2.5 (particulate matter with the diameter (Dp) ≤ 2.5 μm) led to an additional 1.8 million deaths globally in 2019.2 The adverse health effects of the particles are strongly linked to their sizes3 and there is accumulating evidence that ultrafine particles (UFPs, Dp ≤ 100 nm) dominate the health risks posed by PM2.5.4,5
Ambient UFPs can penetrate the pulmonary alveoli and traverse walls and translocate into the circulatory system,6–8 resulting in adverse health impacts, ranging from normal transient respiratory problems to cardiovascular and respiratory mortality and morbidity, lung cancer, brain disease, and mutagenic and carcinogenic impacts.9 UFPs can even enter the brain through the olfactory nerves10,11 and cause brain damage, which is associated with Alzheimer's and Parkinson's diseases.12,13 Exposure to UFPs might cause more severe health risks to the sensitive groups including older adults14 and children.15,16
Particle number concentration (PNC) is the most commonly used measure to characterize UFPs and to evaluate their exposure risks. The measurement technologies for PNC by particle number size distribution (PNSD) measurement have been well established. PNSD observations showed that, in most cities around the world, count median diameters (CMD) were lower than 100 nm,17 which indicated that UFPs are the main contributor to PNC in urban areas. Primary UFPs mainly originate from internal engine combustions such as gasoline and diesel vehicles,18–20 ships,21,22 and airplanes.23–25 A recent review revealed that traffic-related emissions are the most dominant source of PNC in more than 94.4% of cities by summarizing 245 studies on PNC observations over the world.26 Energy production and industrial combustion sources also make notable contributions to PNC.27 Secondary UFPs related to new particle formation (NPF) events can often result in increases in the PNC of UFPs by one to two orders of magnitude.28–32
The potential health risks associated with UFPs are related to their different sources.33 This is not only because the chemical compositions of UFPs are different by source and may have varying toxicity, but also because the different PNSD of these sources will determine their deposition in the respiratory system.3,5 Therefore, it is necessary to accurately assess the contribution of UFPs from different sources. The magnitude of mass concentrations of UFPs is usually insignificant in urban areas17,34 and precise measurement of UFPs' chemical compositions is difficult. Measuring UFPs' chemical compositions with fine time resolution and size separation is even more challenging. UFPs' chemical composition is mainly analyzed offline through filter samples collected by cascade impactors such as multiple-stage inertial impactors (MOUDI).35 These cascade impactors can separate UFPs from larger particles and collect them on filters. Limited by the sampling flow and by the instrumentation sensitivities, the sampling time usually lasts for several hours to a few days. A source apportionment study using MOUDI samples and a chemical mass balance (CMB) model showed that meat cooking, gasoline and diesel vehicles, motor oil and wood burning are the main sources of carbonaceous UFPs in California, US.35 However, the source apportionment method based on bulk chemical composition with low time resolution is not fully suitable for UFPs because UFPs are highly influenced by local emission sources and distinct anthropogenic activity patterns. In addition, the suggested sources of UFPs based on mass concentrations do not directly link to their contributions to PNC.
Source apportionment relying on PNSD observations combined with receptor models has been widely applied since the 2000s. The time resolution of PNSD observations can reach 5–15 min (ref. 36 and 37) and hourly average values were usually used.38 Zhou et al.36 pioneeringly introduced PNSD with 15 min time resolution into the receptor model and identified nucleation and growth, local and remote traffic, stationary combustion, and secondary particles as the main sources. PNSD datasets can be harmonized with the time scales of other pollution metrics such as criteria pollutants, meteorological variables, traffic flows and particulate chemical composition.38 PNSD observations can provide dozens of size bins in the UFPs' regime (up to several hundred bins in the sub-micrometer particle regime). The resolved source profiles (i.e., PNSD) can assist the source identification and straightforwardly quantify their corresponding contribution to PNC.36
The algorithms of receptor models for PNC source apportionment include principal component analysis (PCA),39 K-means clustering,40 positive matrix factorization (PMF)36 and non-negative matrix factorization (NMF).41 K-Means clustering is a semi-quantitative method based on occurrence frequency, which produces mixed sources.23,41 PMF is the most popular method for source apportionment of atmospheric pollutants. However, intensive computation of matrix inversion, singular value decomposition and error weighting during the solving process of PMF limit its calculation capacities for long-term and fine-size-resolution PNSD datasets.41 These studies, which deployed PMF with reduced particle size bins,32,36,42 sacrificed the particle size resolution of PNSD. The latest research indicates that NMF is a promising source apportionment method for PNSD.41,43 NMF algorithm implementation is flexible, allowing for a certain degree of non-negative bias and exhibiting good robustness and stability. The NMF algorithm employs the Kullback–Leibler divergence and utilizes a straightforward multiplicative updates format,44 which has been refined to avoid numerical underflow.45 Hence, NMF offers a fast computational speed, particularly when dealing with large datasets. In the study of Liang et al.,41 coal heating, as an important source of PNSD in Beijing, can be identified by using PMF and NMF algorithms. NMF apportions more distinctly (with less mixed source profiles) and runs 11–28 times faster than PMF.41
In this study, we performed three-month measurements on PNSD simultaneously at two suburban background sites in Shanghai, China and emphatically investigate the characteristics and sources of UFPs. The source apportionments of PNSD and PNC were analyzed by using the NMF algorithm. Through delving into the relationships between the resolved factors and other pollutants (including criteria pollutants and PM2.5 chemical compositions), the main sources of these factors were precisely identified, and the possible uncertainties were also discussed. The exposure risks of UFPs from different sources on the respiratory system were further evaluated by calculating their corresponding depositions.
2. Experimental
2.1. Sampling and data
Continuous measurement was performed from 1 January to 31 March 2021 at two suburban sites in Shanghai. As shown in Fig. 1a, Dianshan Lake (DSL, 31.10° N, 120.98° E) and Dongtan (DT, 31.52° N, 121.97° E) sites are located 49 km west and 55 km northeast to Shanghai city center, respectively. The DSL site is located in the gravity center of a triangle formed by three cities, i.e., Shanghai, Suzhou and Jiaxing. The DT site is inside the Chongming Dongtan Birds National Nature Reserve, which is on the east corner of Chongming island, as the entrance of the Yangtze River to the East China Sea. The reserve area is not open to the public. Chongming island has formulated a world-class ecological island construction plan, and the development of highly polluting industries is constrained.
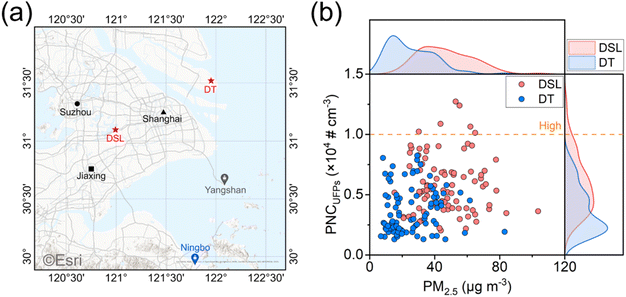 |
| Fig. 1 (a): Location of the sites on the map. DSL and DT sites are noted in red pentagrams. The city centers of Shanghai, Suzhou and Jiaxing are noted in black triangles, circles and squares, respectively. Yangshan Deep-Water Port and Ningbo port are marked with a grey pin and a blue pin, respectively. (b) Scatterplot between the daily averages of PNCUFPs and PM2.5 at the two sites, the shaded area indicates the probability distribution and the orange dashed line represents the daily high values (10 000 # cm−3) suggested by WHO AQG 2021. | |
The measurement of PNC (in units of # cm−3) at the two sites was simultaneously performed by using scanning mobility particle sizers (SMPS; TSI model 3938) over the size range of 13.6–710.5 nm with 111 channels. The datasets of 5 min resolution have been hourly averaged for further analysis. PNCUFPs and PNCTotal refer to the particles below 100 nm and 710.5 nm, respectively. The concentrations of criteria pollutants and PM2.5 chemical compositions and meteorological parameters have been measured simultaneously. More details can be found in ESI Text S1.†
2.2. Analysis algorithm
2.2.1. Non-negative matrix factorization (NMF).
Using NMF, for any given non-negative i-observation and j-variable matrix V, it is possible to find a non-negative i × a base matrix W and the a × j weight matrix H, satisfying the condition Vi,j ≈ Wi,a × Ha,j.46 The dimensionality reduction matrix of the original matrix can be realized and the data features can be obtained. In our study, the NMF analysis was performed using the “NMF” package47 in R. NMF offers good performance in dealing with the large matrix, composed of 111 PNSD channels (variables) and 2000 time slots (samples). The settings and operational parameters of NMF were obtained from the study by Liang et al.41 Implementation details of the NMF model are shown in ESI Text S2.†
2.2.2. Respiratory deposition model.
The International Commission on Radiological Protection (ICRP) model is used to assess the flux of particles with different sizes into the respiratory system.48 This model is utilized for estimating the sedimentation of the three main regions of the respiratory tract: Head Airway (HA, including nasal, pharyngeal and laryngeal passages), Tracheobronchial (TB), Alveolar (Alve, including pulmonary). The ICRP model predicts the total sedimentation rate and regional sedimentation rates based on the particle size as a function of the channels (Fig. S25†), ranging from 6 to 1000 nm.49 A detailed description of the respiratory deposition of particles based on the ICRP model and source profiles of the resolved factors is shown in ESI Text S4.†
3. Results
3.1. General characteristics of UFPs
As illustrated in Fig. S1,† PNCUFPs and PM2.5 concentrations at the DSL site are generally higher than those at the DT site. PNCUFPs accounts for more than 70% of PNCTotal on average at both sites (Fig. S2†). The mean concentrations of PNCUFPs and PM2.5 are 5831 # cm−3 and 46.7 μg m−3 at the DSL site, and 3724 # cm−3 and 25.6 μg m−3 at the DT site, respectively (Fig. S3†). During the three-month campaign (Fig. 1b), there are 6 days (7%) when PNCUFPs at the DSL site exceeds the daily high value (10
000 # cm−3) suggested by the World Health Organization's air quality guidelines released in 2021 (WHO AQG2021).50 Higher PNC, PM2.5, CO, SO2, and NOx at the DSL site (Fig. S1, S3 and S4†) apparently show that the DSL site is more polluted than the DT site.
The DSL site is surrounded by the massive and developed industries and road networks. Busy traffic around the DSL site is depicted by the clear morning and evening rush-hour peaks in NOx (Fig. S4f†). The DSL site is susceptible to pollution from adjacent land areas (Jiangsu and Zhejiang Provinces).51 In comparison, around the DT site, there is little industry and traffic. CO, SO2 and NO concentrations are low at the DT site and peak at noon, which might be attributable to remote air transport from the polluted area (Fig. S4b–d†). Clean ocean air masses often easily reach the site and remove the pollution.52 Comparing the two sites, it can be speculated that denser industrial and traffic pollution sources may lead to higher PNC (Fig. 1b).
PM2.5 and PNCUFPs show weak correlations (Table S2†) with each other at both sites. This is consistent with the global survey study conducted by de Jesus et al.53 who summarize that Pearson's R is in the range of 0.09–0.64 between PM2.5 and PNC, even when log-transformation is applied. But the underlying reasons have not been further investigated. At both DSL and DT sites, PNCUFPs shows positive correlations with NOx (RDSL = 0.67; RDT = 0.57) and SO2 (RDSL = 0.47; RDT = 0.37) (Fig. S5 and Table S2†). The correlations are not strong enough to indicate any source apportionments for PNCUFPs. This is in line with the statement that “no other pollutant is a good proxy for UFPs” suggested in WHO AQG2021.50
3.2. Source apportionment
The contour graph of Pearson's R between each particle size bin of the SMPS measurement data generally shows the strongest correlation between the neighboring bins (Fig. S8†). At the DSL site, a wider coverage of positive-correlated areas indicates some PNC sources characterized by multimode distribution. The NMF algorithm resolves 5 factors both at the DSL (N1–N5) site and the DT (N′1–N′5) site, respectively. The preferred resolution is based on the “NMF rank survey”47,54 and uncentered correlation coefficient (UCC),55 which is further explained in ESI Text S2.†
As illustrated in Fig. 2 and S14,† all factors are distinct in the PNSD and time series. N1–N3 and N′1–N′3 are the main contributors to PNCUFPs at DSL and DT sites, respectively. And they slightly contribute to PVC (Fig. 2e and f). Combined with their diurnal variations (Fig. 2g and h), correlation with criteria pollutants, meteorological parameters and chemical components of PM2.5 (Fig. 3 and S15†), and the upwind direction (Fig. S16 and S17†), these factors are further identified as traffic nucleation and emissions, marine ship emissions, photochemical nucleation and growth processes, industrial emissions, and the regional background.
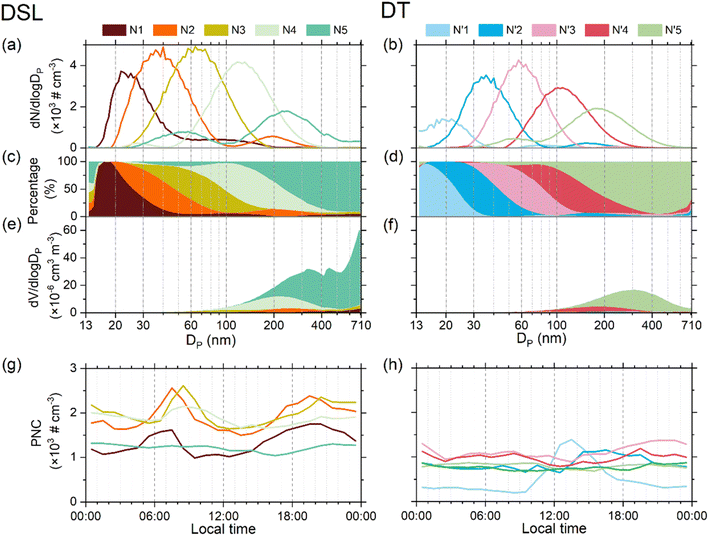 |
| Fig. 2 The 5-factor solution of PNSD at DSL and DT sites using the NMF model. PNSD of resolved factors (a and b), and corresponding contribution portions to PNC at different size bins (c and d), PVC distributions (e and f) and diurnal variations (g and h) of the averages resolved factors at the DSL site (left panel) and DT site (right panel), respectively. | |
3.2.1. Traffic nucleation and emissions at the DSL site.
At the DSL site, the PNC of N1–N3 exhibits distinct morning and evening rush hour peaks (Fig. 2g), which suggests that N1, N2 and N3 are associated with vehicular traffic. The mode (the peak in the PNSD curve) and GMD are 22 and 33 nm for N1, 40 and 45 nm for N2, and 64 nm and 65 nm for N3, respectively (Fig. 2a and Table 1).
Table 1 Summary of the resolved factors and the corresponding sources and size characteristics at DSL and DT sites
Sites |
Factors |
Sources |
Modea,d |
GMDb,d |
GSDc,d |
Mode: in particle size distribution, mode refers to the peak in the distribution curve that represents the most common particle size.
GMD: the geometric mean diameter of particulate matter.
GSD: the geometric standard deviation of the GMD.
Mode, GMD and GSD are all in units of nm.
N′2 at the DT site is influenced by marine ship emissions.
|
DSL |
N1 |
Traffic nucleation |
22 |
33 |
1.8 |
N2 |
Fresh traffic emissions |
40 |
45 |
1.7 |
N3 |
Aged traffic emissions |
64 |
65 |
1.5 |
N4 |
Industrial emissions |
130 |
122 |
1.6 |
N5 |
Regional background |
55 & 240 |
166 |
2.2 |
DT |
N′1 |
Photochemical nucleation |
20 |
23 |
1.7 |
N′2 |
Nucleation growthe |
35 |
39 |
1.6 |
N′3 |
Marine ship emissions |
60 |
61 |
1.4 |
N′4 |
Industrial emissions |
100 |
110 |
1.5 |
N′5 |
Regional background |
50 & 180 |
160 |
1.8 |
Since particles originating from diesel vehicles are usually considered larger than those from gasoline vehicles,56 earlier studies identified the factors with a size of ∼40 nm as gasoline vehicles, and those with a size of ∼60 nm as diesel vehicles.38,57 To distinguish the contributions of gasoline and diesel vehicles, the flows of different vehicle types have been involved in their receptor models.38 And such information is missing in our study. Compared to PNCN2, PNCN3 is less positively correlated to NO and NOx (Fig. 3a). This does not support the speculation that N2 and N3 mainly originate from gasoline and diesel vehicles, respectively, since diesel vehicles are typically considered the main traffic source of NOx in cities.58
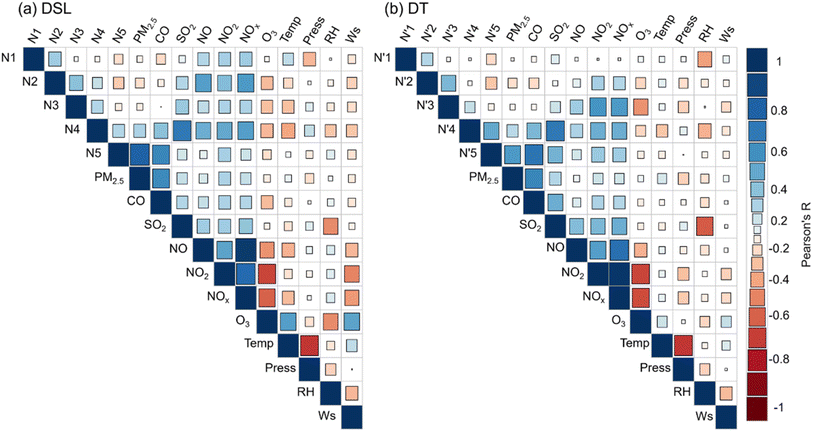 |
| Fig. 3 Pearson correlation heatmaps between the resolved factors, criteria pollutants, and meteorological parameters at DSL (a) and DT (b) sites. Temp, press, RH and Ws represents ambient temperature, surface pressure, relative humidity, and wind speed, respectively. N1–N5 and N′1 N′5 represent the PNC of the resolved factors at DSL and DT sites, respectively. | |
It is noteworthy that the NO concentrations could reach high levels in the morning at the DSL site, with an average of 10.9 ppbv and a maximum of 236.4 ppbv (Fig. S4d†). This is attributed to intense vehicular emissions of high NO (Fig. S6a†), which cannot be immediately fully titrated by O3 and converted to NO2 during morning rush hours. Thus, high NO concentrations (>100 ppbv) and high NO portions in NOx (NO/NOx > 60%) are used in combination as an indicator of fresh traffic emissions, which mostly occurs with high PNCN2 (the upper right section in Fig. 4a). In contrast, high PNCN3 seldomly appears with high NO concentrations and NO portions (the upper right section in Fig. 4b). Hence, N2 and N3 are identified as fresh and aged traffic emissions, respectively.
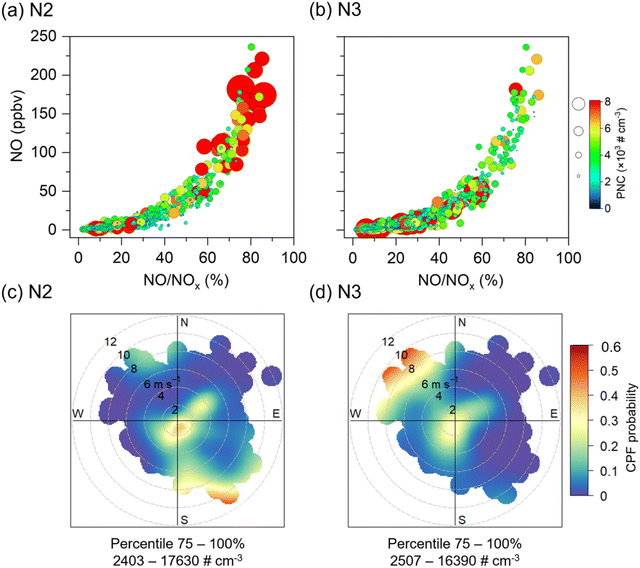 |
| Fig. 4 Scatterplots of NO against NO/NOx at the DSL site. The color scales and size of the dots represent PNCN2 (a) and PNCN3 (b), respectively. The conditional probability function (CPF) polar plot for the 75th–100th-percentiles of PNCN2 (c) and PNCN3. (d) Note that the scale of CPF ranges from 0 to 0.6. | |
The polar plots of conditional probability functions (CPF)59 indicate that the south section under slow wind and the southeast section under strong wind are the main sources of N2 (Fig. 4c), which is in line with the location of busy highways (G50 and G318) south to the DSL site. The northwest section under strong wind is the main source of N3 (Fig. 4d). The road network in the north direction to the DSL site is separated by a lake (Fig. 1a). The traffic emissions from northwest must experience a certain period of transport and aging before reaching the DSL site. The near-site hot area of PNCN3 under slow wind indicates that N3 also originates from freshly emitted particles experiencing local aging processes (Fig. 4d), such as coagulations.60 The morning peak of PNCN3 appears later than that of PNCN2 (Fig. 2g), possibly due to the longer aging time of its air masses. In a PMF study for London, fresh and aged traffic has also been distinguished by their differences in the peak time of PNC and mode of PNSD.23 Inland river navigation close to the DSL site can be an additional possible source of UFPs,61,62 but there is currently not enough evidence to prove that inland river navigation possesses a morning rush peak like that of vehicular traffic.
The morning peak of PNCN1 occurs before sunrise, indicating that N1 within the mode of “nucleation” is not relevant to photochemical reactions (Fig. 2g). Previous studies have identified the factor with a mode of ∼20 nm as traffic emission, measured in close proximity to roads.36,38 This mode is attributed to the generation of nucleation particles during the dilution of diesel exhaust emissions, as observed by Ntziachristos et al.63 in engine exhaust sampling. PNCN1 is positively correlated with NOx and PNCN2 (Fig. 3a), further supporting the association of N1 with traffic emissions. As illustrated in Fig. S24a,† in the evening of March 22nd and 24th, high PNCN1 levels were observed in conjunction with elevated NO concentrations and slow wind, clearly confirming local fresh traffic emission as the direct source of N1. The other plausible explanation for nighttime nucleation includes biomass burning,64 and the formation of low-volatility organics and ammonium nitrate through nocturnal oxidation involving NO3 radicals.65 Biomass burning is not a significant source in Shanghai,66 and a more comprehensive explanation of nighttime nucleation at the DSL site requires further detailed observations of oxidants and low-volatility compounds. Rapid increases in PNCN1 related to daytime nucleation is observed for only March 21st (Fig. S24a†) during the three-month observation. High PM2.5 concentrations and large sink surfaces do not facilitate photochemical NPF events at the DSL site. Typically, this nucleation mode is strongest in the early morning when temperatures are lowest of the day and becomes less significant in the afternoon.38 PNCN1 shows a general increasing trend from January to March (Fig. S14a†), which is not observed for either PNCN2 or PNCN3 as an indicator of traffic intensities. This phenomenon does not relate to the change of vehicular flows and cannot be explained with all the available datasets in our study.
3.2.2. Marine ship emissions at the DT site.
The PNSD of N′3 at the DT site is similar to that of N3 at the DSL site (Fig. 2a and b) and PNCN′3 is strongly correlated to NOx (Fig. 3b). However, the lack of a perceptible daily pattern in PNCN′3 (Fig. 2h) and the fact that there is no public road nearby suggest that N′3 is not a source related to vehicular traffic emissions. This is different from N3 at the DSL site.
The strong correlation between PNCN′3 and PM2.5-bound vanadium (V, R = 0.62) and nickle (Ni, R = 0.60) at the DT site (Fig. S15b†) suggests that N′3 is likely related to marine ship emissions from crude oil combustion. V and Ni are considered distinct tracers of marine ship emissions67,68 and have been widely used in source apportionment studies in coastal cities.69 The slope of Ni against V derived from the observations (excluding dust events) at the DT site is 1.05 ± 0.02 (R2 = 0.65) (Fig. 5a). This slope is higher than the value of 0.45 during the implementation of Domestic Emission Control Area (DECA) 2.0 in 2019 but lower than that of 2.14 during implementation of International Maritime Organization (IMO) 2020 regulation reported by Yu et al.70 in Shanghai (Fig. 5a). High PNCN′3 (75th–100th values) is often accompanied by significantly high V concentrations (Fig. 5b, ESI Text 3 and Table S3†). Thus, N′3 is considered related to marine ship emissions. This is consistent with the CPF polar plots of PNCN′3, V and Ni (Fig. S17 and S19†), whose hot zones point to south to the DT site with two busy ports and intensive navigation routes (Fig. 1 and S18†). High emission factors of marine ships have been reported for particles of 4.8 × 1015 # kg−1 fuel.71 The PNSD of marine ship emissions is reported in the range of 25–60 nm,61,62 which is in line with the PNSD of N′3. Liang et al.72 conducted cruise observations in the South China Sea and found that marine ship emissions accounted for about 44% of PNC500. Previous investigations have shown that coastal port cities are highly susceptible to the impact of UFPs emissions from ships, and the highest contribution to PNC can reach 65–70%.21
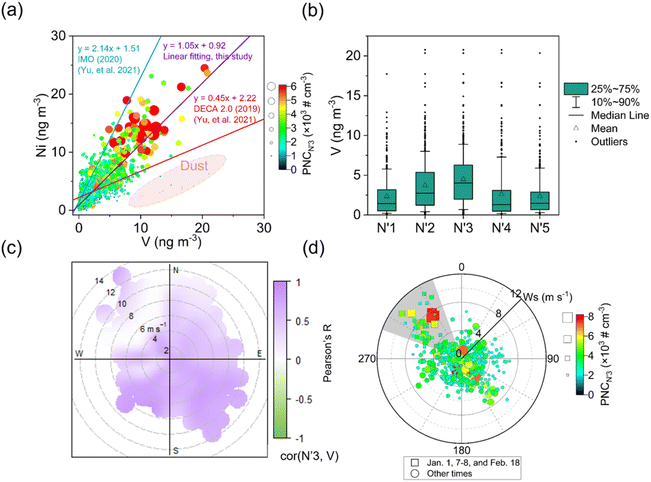 |
| Fig. 5 (a) Scatterplot between V and Ni at the DT site, and the color scales and size of the dots represent PNCN′3. The black line represents the linear fitting in this study, while the blue and red lines represent the linear fittings from Yu et al., 2021. (b) Boxplot of V for resolved factors (N′1–N′5) when their PNC exceeded their corresponding 75th-percentile values at the DT site. (c) The pairwise statistics polar plot between PNCN′3 and V at the DT site. (d) The polar plot for the 75th–100th-percentile of PNCN′3. Jan. 1, 7–8, and Feb. 18 are represented by squares. The color scales and size of the dots represent PNCN′3. | |
The polar plot of pairwise Pearson's R between PNCN′3 and V (Fig. 5c) shows that northwest (land) direction N′3 is related to other sources rather than marine ship emissions. These high PNCN′3 (75th–100th) accompanied by low V concentrations occurred only for four days (1 and 7–8 Jan, and 18 Feb) of the three-month observations (Fig. S14f†). As illustrated in Fig. 5d, they only appear in the angle range of 290–370° and are not mixed with other circle spots. It can be summarized that under northwest wind, N′3 is dominantly controlled by land transport pollution, whose sizes are similar to marine ship emissions. The time-weighted contribution of land transport pollution from northwest to N′3 is 7.5%. Hence, N′3 predominantly originates from marine ship emissions and is slightly influenced by land transport pollution.
3.2.3. Photochemical nucleation and growth processes at the DT site.
N′1 at the DT site is characterized by the smallest PNSD among all the factors with a size mode of 20 nm and GMD of 23 nm (Fig. 2b and Table 1). The variation pattern of PNCN′1 is consistent with that of PNC20 (Fig. S20†). PNCN′1 exhibits a boost during the midday hours (from 10:00 a.m. to 1:00 p.m.), accompanied by a banana-shaped growth pattern in PNSD during NPF days. This observation can be attributed to NPF events73 initiated by photochemical reactions, as described in earlier studies in Shanghai.74,75 The observation duration has been categorized into three groups as NPF (9 d), undefined (28 d) and non-NPF (53 d). Undefined NPF days are characterized by increases of PNCN′1 and PNC20 (i.e., nucleation mode particles) but without a banana-shaped growth pattern. Undefined NPF days can be attributed to interrupted or transported NPF events.
The variation of PNCN′1 across different months suggests that solar radiation alone does not determine the intensity and occurrence frequency of nucleation. H2SO4 and amines are proved to dominate the initial growth of new particles in urban environments.76 Estimation of the production rate of H2SO4 based on SO2 and J(NO2) does not conclusively elucidate the nucleation mechanism at the DT site. SO2 appears to be sufficient in most situations and is weakly positively correlated with PNCN′1. Conversely, the presence of pre-existing background aerosols can constrain the occurrence of NPF through condensation and coagulation scavenging processes.77 The condensation sink rate of H2SO4 is proportional to the particle surface area,78 whose largest contributor is N′5. The negative correlation between PNCN′1 and PNCN′5 (R = −0.19, Fig. 3b) indicates the scavenging effect of existing particles for low-volatility compounds. The lack of other key species such as amines and organic acids can also constrain NPF events.
During NPF days (9 d), the growth processes of NPF events lead to an increase in PNCN′2, which is several hours later after the boost of PNCN′1 (Fig. S14b, d and S22a†). This indicates nucleation growth as a source of N′2. PNCN′2 stays at high values during undefined NPF and non-NPF days (Fig. S14d†), inferring that there exist other sources for N′2. During non-NPF days, Pearson's R between PNCN′2 and V is 0.44, which is close to that between PNCN′3 and V of 0.62 (Fig. S22†), indicating the influence of crude oil combustion. Hence, N′2 is identified as nucleation growth but is influenced by marine ship emissions. Since average PNCN′1 is 179 # cm−3 during non-NPF days and 7 times low than that during NPF days (Table S4†), and it is reasonable to presume that photochemical nucleation does not contribute to N′2 during non-NPF days. Using day-weighted average PNCN′2, the contribution of marine ship emissions to N′2 can be up to 42% (Table S4†).
3.2.4. Industrial emissions and regional background.
N4 and N5 at the DSL site and N′4 and N′5 at the DT site all possess size modes and a GMD greater than 100 nm. They are the main contributor to PVC rather than PNC (Fig. 6a and b). PNCN4, PNCN5, PNCN′4 and PNCN′5 are all positively correlated to carbonaceous components (OC and EC), and some trace elements (such as Cr, Mn, Fe, Ni, Zn and As) (Fig. S15†), indicating that they are associated with industrial emissions.79,80 The largest difference between PNCN4 and PNCN5 (between PNCN′4 and PNCN′5) is their correlation with secondary inorganic aerosols in PM2.5. PNCN5 and PNCN′5 are significantly positively correlated with sulphate (SO42−), nitrate (NO3−) and ammonium (NH4+) (SNA) with Pearson's R > 0.8 (Fig. S15†), indicating that N5 and N′5 have undergone sufficient atmospheric aging and mixing. PNCN5 and PNCN′5 are strongly correlated to PM2.5 (and CO) with Pearson's R equal to 0.85 (0.64) and 0.60 (0.82) at DSL and DT sites, respectively (Fig. 3). And PNCN5 and PNCN′5 values are typically higher during periods of low SO2 concentrations and a high SOR (Fig. S23c and d†). These phenomena indicate that N5 and N′5 have experienced aging time long enough to thoroughly mix all the primary emission particles and secondary formation particles such as SNA. The smaller mode at 50 nm of N5 and N′5 is related to the formation of secondary aerosols (Fig. 2a and b). This is consistent with earlier studies that also found these bimodal size modes and identified them as secondary aerosols.57,81,82 Thus, N5 and N′5 are both identified as the regional background aerosol with sufficient mixing and aging. As shown in CPF polar plots (Fig. S17†), the main source direction of N5 is not apparent at the DSL site, and west is the main direction for N′5 at the DT site. The DT site is clearly influenced by the pollution plumes attributed to land-sea breeze transport from the metropolis (Fig. S17b†).83,84 Long-range transport of pollution from the North China Plain during the heating season is one important cause of haze events in Shanghai,85 which is consistent with the hot area in the north wind section with a high windspeed in the CPF polar plots of PNCN5 and PNCN′5 (Fig. S17†).
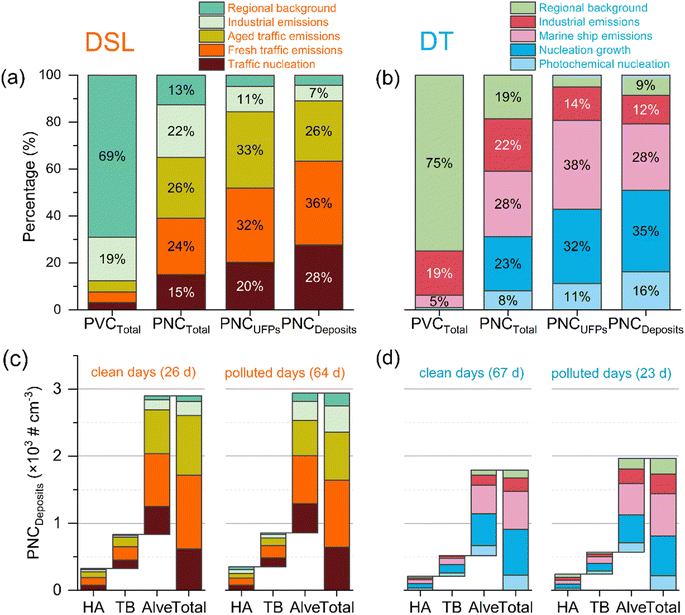 |
| Fig. 6 Contributions to PVCTotal, PNCTotal, PNCUFPs and PNCDeposits from 5 sources at DSL (a) and DT (b) sites, respectively, where PNCDeposits represents the total deposition of each source in the respiratory system. Stacked bridge plots of PNCDeposits in the respiratory system from each source for clean (PM2.5 < 35 μg m−3) and polluted (PM2.5 > 35 μg m−3) days at DSL (c) and DT (d) sites. | |
In contrast, PNCN4 and PNCN′4 show the strongest correlation with SO2 among all the factors (Fig. 3). SO2 is typically emitted from coal combustion in the Yangtze River Delta region, and is more related to industrial emissions using coals, such as power plants, rather than residential heating.86 The PNCN4 and PNCN′4 values are typically higher during periods of high SO2 concentrations and a low sulfur oxidation ratio (SOR) (Fig. S23a and b†), which indicates short aging time. Analysis of the CPF polar plot reveals that N4 primarily originates from the west direction (Fig. S17a†), which aligns with the location of the developed industrial region. And the DT site is mainly influenced by the industrial inland area in the west (Fig. S17b†). Hence, N4 and N′4 are identified as industrial emission sources.
3.3. Respiratory deposition of UFPs
Respiratory PNC deposition is employed to evaluate the potential hazards of particle sources according to the ICRP model (ESI Text S4†).48 The contribution of each source to PVC, PNCTotal, PNCUFPs and PNCDeposits is illustrated in Fig. 6. N1–N3 at the DSL site and N′1 N′3 at the DT site contributed 84% (90%) and 81% (79%) to PNCUFPs (PNCDeposits), respectively. At the DSL site, the predominant sources contributing to respiratory deposition are traffic nucleation (N1, 28%), fresh traffic (N2, 36%) and aged traffic (N3, 26%) emissions. Ma et al.87 evaluated the deposited PNC dose by combining the Multiple-Path Particle Dosimetry Model (MPPD) and hygroscopicity estimates in respiratory tracts and found that traffic emissions are the main source influencing pulmonary-related impairment in Beijing regardless of the season. The other studies using the lung deposited surface area (LDSA) as the measure of health risk assessment concluded that traffic emissions are the main contributor in urban environments.88,89 At the DT site, the predominant sources contributing to respiratory deposition are photochemical nucleation (N′1, 16%), nucleation growth (N′2, 35%) and marine ship emissions (N′3, 28%). The alveolar region is the main deposition region for all factors at both sites (Fig. 6c and d). PNCDeposits reaches the apexes in the morning and evening at the DSL site and at noon at the DT site, respectively (Fig. S27†).
The total PNCDeposits varies slightly between clean (PM2.5 < 35 μg m−3) and polluted (PM2.5 > 35 μg m−3) days at each site (Fig. 6c and d). Meanwhile, the relative contributions from each source do not significantly change, neither. This is because PNCDeposits is mainly related to UFPs rather than those main contributors to PVC, i.e., industrial emissions and regional background, with larger sizes. As illustrated in Fig. 7, at the DT site, PNCDeposits during NPF days is on average two times higher than that during non-NPF days. The difference is attributed to the contribution from sources of photochemical nucleation (N′1) and nucleation growth (N′2). In Beijing, the deposited PNC is attributed to NPF events during clean days and comparable to that during polluted days.87 Hence, the occurrence of NPF events in urban environments30,31,90 is an unexpected potential health risk.
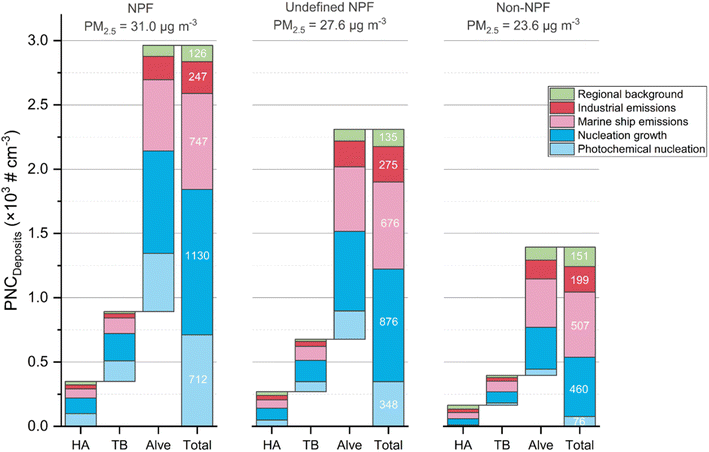 |
| Fig. 7 Stacked bridge plots of PNCDeposits in the respiratory system from each source for three categories, which are NPF, undefined NPF, and non-NPF days at the DT site. | |
4. Implications
Our study presents measurements of PNSD and PNC using SMPS at two suburban sites in Shanghai, China from January to March 2021. The source apportionment of PNC is resolved by matrix decomposition using NMF on PNSD data, which provides a comprehensive understanding of the sources influencing PNC in this region. The identified sources include photochemical nucleation and growth processes, traffic nucleation and emissions, marine ship and industrial emissions, and regional background. The NMF algorithm separates the matrix of three-month PNSD into 5 factors at each site. All the source profiles are continuous and smooth with an appropriate width of PNSD. The number of resolved factors are indicated by the NMF survey rank and reordered consensus matrices based on an objective consideration of the quantitative cophenetic coefficient.
In addition to the characteristics of the resolved factors such as size distributions, diurnal variations and CPF polar plots, the utilization of other indicators is also necessary to further identify the sources categories. In this study, the NO and NO/NOx ratio are utilized in combination to distinct fresh and aged traffic-related sources (N2 and N3); the correlation with V and Ni is utilized to identify marine ship emissions (N′3). The industrial emissions (N4 and N′4) and regional background (N5 and N′5) can be distinguished based on their relationship to SO2 and the SOR.
PNCUFPs (Dp = 13–100 nm) accounts for more than 70% of PNCTotal (Dp = 13–710 nm) on average at both sites. The specific sources contributing to PNCUFPs vary dependent on the surrounding environment and emission sources at each site. At the DSL site, the influence of vehicular traffic is prominent and PNCUFPs is mainly attributed to traffic-related emissions, i.e., N2 and N3. N1 is characterized by morning and evening peaks and with the smallest mode at 22 nm (nucleation mode) is very likely related to traffic. In contrast, PNCUFPs at the DT site originates from photochemical nucleation (N′1) and growth (N′2) processes, and marine ship emissions (N′3). At the DT site, NPF events are associated with the photochemical aging of land air masses and marine ship emissions are indicated with high V and Ni concentrations from the port direction. The sources of industrial emissions and the regional background are characterized by a larger GMD and contribute more to PVC rather than PNC, indicating their association with PM2.5 rather than UFPs.
By recognizing the different sources of UFPs and larger particles, these findings emphasize the underlying reasons why UFPs and PM2.5 belong to two different metrics.53 Targeted mitigation strategies to reduce UFPs pollution should focus on local emission sources, which is different from the PM2.5 reduction strategies relying more on national and regional measures. The latest European emission standard for vehicular exhausts (EURO 7) involves PNC as a standard, which reflects the growing recognition of the health risks associated with UFPs. Shipping activities, while contributing to economic prosperity in port cities, also pose a threat of particulate pollution.21 A model study showed that ship emissions can contribute 10.6% (6.7 μg m−3) to PM2.5 in Shanghai.91 Its contribution to UFPs shall receive as much attention as its contribution to PM2.5. The significant contribution of secondary nucleation particles to UFPs deserves more attention. Since the lower limit of our measurements starts from 13 nm, smaller particles, especially for NPF events, are underestimated.
Traffic nucleation and emissions, marine ship emissions, photochemical nucleation and growth are the main contributors to both of PNCUFPs and PNCDeposits. A large portion of the deposition in the deepest region of the alveoli indicated a considerable health risk from UFPs. The boost in PNCUFPs initiated by NPF events can cause extra exposure risk with respect to respiratory deposition. The nucleation processes exhibit distinct diurnal variation patterns at the two sites, indicating the need for further investigation into the underlying mechanisms. From the perspective of daily variations in deposition, individuals need to take appropriate measures to avoid UFPs exposure during peak traffic hours in the morning and evening and during the occurrence of NPF events at noon.
Investigations on the UFPs' sources at DSL and DT sites provide a comprehensive result over the region. But these findings may not directly apply to urban areas with denser populations and more pollution sources. Intensive residential and commercial cooking, for example, can be an important source of UFPs in urban areas in China.82 There are two busy international airports in Shanghai which can be important sources of UFPs. The health risk assessment of UFPs in our study is based on their particle size and corresponding deposition in the respiratory system. To conduct a more thorough health risk assessment, future studies can incorporate detailed toxicological analysis of the chemical compositions of UFPs emitted from different sources. Our study reveals that the identified sources can sometimes mix with other collinear sources, a common phenomenon in source apportionment studies using receptor models. The marine ship-related source (N′3) at the DT site is occasionally influenced by remote pollution transport. Meanwhile, marine ship-related PNC can be underestimated because N′2 can be influenced by marine ship emissions during non-NPF days. The diesel and gasoline vehicular emission is not distinguished at the DSL site. To address the challenges and improve the precision of source apportionment of UFPs, there is indeed an urgent need for technology that can provide chemical composition analysis of UFPs with size separation and with high time resolution.
Data availability
Data will be available on request.
Author contributions
H. C., Y. D. and L. L. conceptualized and designed the study, and coordinated and supervised the data analysis. Q. W. and J. H. conducted the statistical analysis, interpreted the results and wrote the first and successive drafts of the manuscript. J. H., Y. D., Q. F. and Y. S. organized and performed the measurement. K. Z., L. H., Y. W., J. T., L. W. and D. L. participated in the interpretation and finalized the manuscript. C. G., A. M. and J. C. provided comments and critically reviewed manuscripts.
Conflicts of interest
The authors declare that they have no conflict of interest.
Acknowledgements
This study was financially sponsored by the National Key Research and Development Program of China (2022YFC3703501), the Key Research and Development Projects of Shanghai Science and Technology Commission, China (20dz1204000), the National Natural Science Foundation of China (22122601 and 22376033), the special fund of State Environmental Protection Key Laboratory of Formation and Prevention of Urban Air Pollution Complex (SEPAir-2022080597), and LAC/CMA (2022B10).
References
- GBD, Global, regional, and national comparative risk assessment of 84 behavioural, environmental and occupational, and metabolic risks or clusters of risks for 195 countries and territories, 1990–2017: a systematic analysis for the Global Burden of Disease Study 2017, Lancet, 2018, 392, 1923–1994 CrossRef PubMed.
- V. A. Southerland, M. Brauer, A. Mohegh, M. S. Hammer, A. van Donkelaar, R. V. Martin, J. S. Apte and S. C. Anenberg, Global urban temporal trends in fine particulate matter (PM2.5) and attributable health burdens: estimates from global datasets, Lancet Planet. Health, 2022, 6, e139–e146 CrossRef PubMed.
- D. E. Schraufnagel, The health effects of ultrafine particles, Exp. Mol. Med., 2020, 52, 311–317 CrossRef CAS PubMed.
- N. Li, S. Georas, N. Alexis, P. Fritz, T. Xia, M. A. Williams, E. Horner and A. Nel, A work group report on ultrafine particles (American Academy of Allergy, Asthma & Immunology): why ambient ultrafine and engineered nanoparticles should receive special attention for possible adverse health outcomes in human subjects, J. Allergy Clin. Immunol., 2016, 138, 386–396 CrossRef CAS PubMed.
- H.-S. Kwon, M. H. Ryu and C. Carlsten, Ultrafine particles: unique physicochemical properties relevant to health and disease, Exp. Mol. Med., 2020, 52, 318–328 CrossRef CAS PubMed.
- G. Oberdörster, E. Oberdörster and J. Oberdörster, Nanotoxicology: An Emerging Discipline Evolving from Studies of Ultrafine Particles, Environ. Health Perspect., 2005, 113, 823–839 CrossRef PubMed.
- H. Nakane, Translocation of particles deposited in the respiratory system: a systematic review and statistical analysis, Environ. Health Prev. Med., 2012, 17, 263–274 CrossRef PubMed.
- D. Lu, Q. Luo, R. Chen, Y. Zhuansun, J. Jiang, W. Wang, X. Yang, L. Zhang, X. Liu, F. Li, Q. Liu and G. Jiang, Chemical multi-fingerprinting of exogenous ultrafine particles in human serum and pleural effusion, Nat. Commun., 2020, 11, 2567 CrossRef CAS PubMed.
- M. U. Ali, S. Lin, B. Yousaf, Q. Abbas, M. A. M. Munir, A. Rashid, C. Zheng, X. Kuang and M. H. Wong, Pollution characteristics, mechanism of toxicity and health effects of the ultrafine particles in the indoor environment: current status and future perspectives, Crit. Rev. Environ. Sci. Technol., 2022, 52, 436–473 CrossRef CAS.
- B. A. Maher, I. A. M. Ahmed, V. Karloukovski, D. A. MacLaren, P. G. Foulds, D. Allsop, D. M. A. Mann, R. Torres-Jardón and L. Calderon-Garciduenas, Magnetite pollution nanoparticles in the human brain, Proc. Natl. Acad. Sci. U. S. A., 2016, 113, 10797–10801 CrossRef CAS PubMed.
- E. Underwood, The polluted brain, Science, 2017, 355, 342–345 CrossRef CAS PubMed.
- L. Calderón-Garcidueñas and A. Ayala, Air Pollution, Ultrafine Particles, and Your Brain: Are Combustion Nanoparticle Emissions and Engineered Nanoparticles Causing Preventable Fatal Neurodegenerative Diseases and Common Neuropsychiatric Outcomes?, Environ. Sci. Technol., 2022, 56, 6847–6856 CrossRef PubMed.
- J. A. Flood-Garibay, A. Angulo-Molina and M. Á. Méndez-Rojas, Particulate matter and ultrafine particles in urban air pollution and their effect on the nervous system, Environ. Sci.: Processes Impacts, 2023, 25, 704–726 RSC.
- V. Viher Hrženjak, A. Kukec, I. Eržen and D. Stanimirović, Effects of Ultrafine Particles in Ambient Air on Primary Health Care Consultations for Diabetes in Children and Elderly Population in Ljubljana, Slovenia: A 5-Year Time-Trend Study, Int. J. Environ. Res. Public Health, 2020, 17, 4970 CrossRef.
- N. M. Johnson, A. R. Hoffmann, J. C. Behlen, C. Lau, D. Pendleton, N. Harvey, R. Shore, Y. Li, J. Chen, Y. Tian and R. Zhang, Air pollution and children's health—a review of adverse effects associated with prenatal exposure from fine to ultrafine particulate matter, Environ. Health Prev. Med., 2021, 26, 72 CrossRef PubMed.
- J. Fang, X. Song, H. Xu, R. Wu, J. Song, Y. Xie, X. Xu, Y. Zeng, T. Wang, Y. Zhu, N. Yuan, J. Jia, B. Xu and W. Huang, Associations of ultrafine and fine particles with childhood emergency room visits for respiratory diseases in a megacity, Thorax, 2022, 77, 391–397 CrossRef PubMed.
- T. Wu and B. E. Boor, Urban aerosol size distributions: a global perspective, Atmos. Chem. Phys., 2021, 21, 8883–8914 CrossRef CAS.
- L. Morawska, Z. Ristovski, E. R. Jayaratne, D. U. Keogh and X. Ling, Ambient nano and ultrafine particles from motor vehicle emissions: characteristics, ambient processing and implications on human exposure, Atmos. Environ., 2008, 42, 8113–8138 CrossRef CAS.
- P. Pant and R. M. Harrison, Estimation of the contribution of road traffic emissions to particulate matter concentrations from field measurements: a review, Atmos. Environ., 2013, 77, 78–97 CrossRef CAS.
- P. Kumar, L. Morawska, W. Birmili, P. Paasonen, M. Hu, M. Kulmala, R. M. Harrison, L. Norford and R. Britter, Ultrafine particles in cities, Environ. Int., 2014, 66, 1–10 CrossRef CAS PubMed.
- Y. González, S. Rodríguez, J. C. Guerra García, J. L. Trujillo and R. García, Ultrafine particles pollution in urban coastal air due to ship emissions, Atmos. Environ., 2011, 45, 4907–4914 CrossRef.
- P. Eger, T. Mathes, A. Zavarsky and L. Duester, Measurement report: inland ship emissions and their contribution to NOx and ultrafine particle concentrations at the Rhine, EGUsphere, 2023, 2023, 1–30 Search PubMed.
- M. Masiol, R. M. Harrison, T. V. Vu and D. C. S. Beddows, Sources of sub-micrometre particles near a major international airport, Atmos. Chem. Phys., 2017, 17, 12379–12403 CrossRef CAS.
- B. Stacey, Measurement of ultrafine particles at airports: a review, Atmos. Environ., 2019, 198, 463–477 CrossRef CAS.
- F. Ungeheuer, L. Caudillo, F. Ditas, M. Simon, D. van Pinxteren, D. Kılıç, D. Rose, S. Jacobi, A. Kürten, J. Curtius and A. L. Vogel, Nucleation of jet engine oil vapours is a large source of aviation-related ultrafine particles, Commun. Earth Environ., 2022, 3, 319 CrossRef.
- Y. Zhu, I. D. Sulaymon, X. Xie, J. Mao, S. Guo, M. Hu and J. Hu, Airborne particle number concentrations in China: a critical review, Environ. Pollut., 2022, 307, 119470 CrossRef CAS PubMed.
- M. P. Keuken, M. Moerman, P. Zandveld, J. S. Henzing and G. Hoek, Total and size-resolved particle number and black carbon concentrations in urban areas near Schiphol airport (the Netherlands), Atmos. Environ., 2015, 104, 132–142 CrossRef CAS.
- M. Brines, M. Dall'Osto, D. C. S. Beddows, R. M. Harrison, F. Gómez-Moreno, L. Núñez, B. Artíñano, F. Costabile, G. P. Gobbi, F. Salimi, L. Morawska, C. Sioutas and X. Querol, Traffic and nucleation events as main sources of ultrafine particles in high-insolation developed world cities, Atmos. Chem. Phys., 2015, 15, 5929–5945 CrossRef CAS.
- H. Wu, Z. Li, M. Jiang, C. Liang, D. Zhang, T. Wu, Y. Wang and M. Cribb, Contributions of traffic emissions and new particle formation to the ultrafine particle size distribution in the megacity of Beijing, Atmos. Environ., 2021, 262, 118652 CrossRef CAS.
- J. Shen, A. Bigi, A. Marinoni, J. Lampilahti, J. Kontkanen, G. Ciarelli, J. P. Putaud, T. Nieminen, M. Kulmala, K. Lehtipalo and F. Bianchi, Emerging Investigator Series: COVID-19 lockdown effects on aerosol particle size distributions in northern Italy, Environ. Sci.: Atmos., 2021, 1, 214–227 CAS.
- S. Gani, S. E. Chambliss, K. P. Messier, M. M. Lunden and J. S. Apte, Spatiotemporal profiles of ultrafine particles differ from other traffic-related air pollutants: lessons from long-term measurements at fixed sites and mobile monitoring, Environ. Sci.: Atmos., 2021, 1, 558–568 CAS.
- L. Tao, Z. Zhou, J. Tao, L. Zhang, C. Wu, J. Li, D. Yue, Z. Wu, Z. Zhang, Z. Yuan, J. Huang and B. Wang, High contribution of new particle formation to ultrafine particles in four seasons in an urban atmosphere in south China, Sci. Total Environ., 2023, 889, 164202 CrossRef CAS PubMed.
- N. V. S. Vallabani, O. Gruzieva, K. Elihn, A. T. Juárez-Facio, S. S. Steimer, J. Kuhn, S. Silvergren, J. Portugal, B. Piña, U. Olofsson, C. Johansson and H. L. Karlsson, Toxicity and health effects of ultrafine particles: towards an understanding of the relative impacts of different transport modes, Environ. Res., 2023, 116186, DOI:10.1016/j.envres.2023.116186.
- T. Hussein, A. Puustinen, P. P. Aalto, J. M. Mäkelä, K. Hämeri and M. Kulmala, Urban aerosol number size distributions, Atmos. Chem. Phys., 2004, 4, 391–411 CrossRef CAS.
- J. Xue, W. Xue, M. H. Sowlat, C. Sioutas, A. Lolinco, A. Hasson and M. J. Kleeman, Seasonal and Annual Source Appointment of Carbonaceous Ultrafine Particulate Matter (PM0.1) in Polluted California Cities, Environ. Sci. Technol., 2019, 53, 39–49 CrossRef CAS PubMed.
- L. Zhou, E. Kim, P. K. Hopke, C. O. Stanier and S. Pandis, Advanced Factor Analysis on Pittsburgh Particle Size-Distribution Data Special Issue of Aerosol Science and Technology on Findings from the Fine Particulate Matter Supersites Program, Aerosol Sci. Technol., 2004, 38, 118–132 CrossRef CAS.
- J. Kasumba, P. K. Hopke, D. C. Chalupa and M. J. Utell, Comparison of sources of submicron particle number concentrations measured at two sites in Rochester, NY, Sci. Total Environ., 2009, 407, 5071–5084 CrossRef CAS PubMed.
- R. M. Harrison, D. C. S. Beddows and M. Dall'Osto, PMF Analysis of Wide-Range Particle Size Spectra Collected on a Major Highway, Environ. Sci. Technol., 2011, 45, 5522–5528 CrossRef CAS PubMed.
- M. Cusack, N. Pérez, J. Pey, A. Alastuey and X. Querol, Source apportionment of fine PM and sub-micron particle number concentrations at a regional background site in the western Mediterranean: a 2.5 year study, Atmos. Chem. Phys., 2013, 13, 5173–5187 CrossRef.
- A. Charron, W. Birmili and R. M. Harrison, Fingerprinting particle origins according to their size distribution at a UK rural site, J. Geophys. Res.: Atmos., 2008, 113, D07202 CrossRef.
- C.-S. Liang, H. Wu, H.-Y. Li, Q. Zhang, Z. Li and K.-B. He, Efficient data preprocessing, episode classification, and source apportionment of particle number concentrations, Sci. Total Environ., 2020, 744, 140923 CrossRef CAS PubMed.
- J.-H. Tan, J.-C. Duan, F.-H. Chai, K.-B. He and J.-M. Hao, Source apportionment of size segregated fine/ultrafine particle by PMF in Beijing, Atmos. Res., 2014, 139, 90–100 CrossRef CAS.
- C.-S. Liang, D. Yue, H. Wu, J.-S. Shi and K.-B. He, Source apportionment of atmospheric particle number concentrations with wide size range by nonnegative matrix factorization (NMF), Environ. Pollut., 2021, 289, 117846 CrossRef CAS PubMed.
-
D. D. Lee and H. S. Seung, Presented in Part at the Proceedings of the 13th International Conference on Neural Information Processing Systems, Denver, CO, 2000 Search PubMed.
- J.-P. Brunet, P. Tamayo, T. R. Golub and J. P. Mesirov, Metagenes and molecular pattern discovery using matrix factorization, Proc. Natl. Acad. Sci. U. S. A., 2004, 101, 4164–4169 CrossRef CAS PubMed.
- D. D. Lee and H. S. Seung, Learning the parts of objects by non-negative matrix factorization, Nature, 1999, 401, 788–791 CrossRef CAS PubMed.
-
R. Gaujoux and C. Seoighe, Using the package NMF: Algorithms and Framework for Nonnegative Matrix Factorization (NMF), https://cran.r-project.org/package=NMF, accessed January, 2023.
- ICRP, The New ICRP Model for the Respiratory Tract, Radiat. Prot. Dosim., 1994, 53, 107–114 CrossRef.
-
W. C. Hinds and Y. Zhu, Aerosol Technology: Properties, Behavior, and Measurement of Airborne Particles, John Wiley & Sons, 2022 Search PubMed.
-
WHO, WHO Global Air Quality Guidelines: Particulate Matter (PM2.5 and PM10), Ozone, Nitrogen Dioxide, Sulfur Dioxide and Carbon Monoxide, Report 9789240034228, World Health Organization, Geneva, 2021 Search PubMed.
- G. Yang, J. Huo, L. Wang, Y. Wang, S. Wu, L. Yao, Q. Fu and L. Wang, Total OH Reactivity Measurements in a Suburban Site of Shanghai, J. Geophys. Res.: Atmos., 2022, 127, e2021JD035981 CrossRef CAS.
- Y. Tang, S. Wang, Q. Wu, K. Liu, L. Wang, S. Li, W. Gao, L. Zhang, H. Zheng, Z. Li and J. Hao, Recent decrease trend of atmospheric mercury concentrations in East China: the influence of anthropogenic emissions, Atmos. Chem. Phys., 2018, 18, 8279–8291 CrossRef CAS.
- A. L. de Jesus, M. M. Rahman, M. Mazaheri, H. Thompson, L. D. Knibbs, C. Jeong, G. Evans, W. Nei, A. Ding, L. Qiao, L. Li, H. Portin, J. V. Niemi, H. Timonen, K. Luoma, T. Petäjä, M. Kulmala, M. Kowalski, A. Peters, J. Cyrys, L. Ferrero, M. Manigrasso, P. Avino, G. Buonano, C. Reche, X. Querol, D. Beddows, R. M. Harrison, M. H. Sowlat, C. Sioutas and L. Morawska, Ultrafine particles and PM2.5 in the air of cities around the world: are they representative of each other?, Environ. Int., 2019, 129, 118–135 CrossRef CAS PubMed.
- R. Gaujoux and C. Seoighe, A flexible R package for nonnegative matrix factorization, BMC Bioinf., 2010, 11, 367 CrossRef PubMed.
- I. M. Ulbrich, M. R. Canagaratna, Q. Zhang, D. R. Worsnop and J. L. Jimenez, Interpretation of organic components from Positive Matrix Factorization of aerosol mass spectrometric data, Atmos. Chem. Phys., 2009, 9, 2891–2918 CrossRef CAS.
- T. Rönkkö and H. Timonen, Overview of sources and characteristics of nanoparticles in urban traffic-influenced areas, J. Alzheimer's Dis., 2019, 72, 15–28 Search PubMed.
- M. H. Sowlat, S. Hasheminassab and C. Sioutas, Source apportionment of ambient particle number concentrations in central Los Angeles using positive matrix factorization (PMF), Atmos. Chem. Phys., 2016, 16, 4849–4866 CrossRef CAS.
- C. McCaffery, H. Zhu, T. Tang, C. Li, G. Karavalakis, S. Cao, A. Oshinuga, A. Burnette, K. C. Johnson and T. D. Durbin, Real-world NOx emissions from heavy-duty diesel, natural gas, and diesel hybrid electric vehicles of different vocations on California roadways, Sci. Total Environ., 2021, 784, 147224 CrossRef CAS PubMed.
- L. L. Ashbaugh, W. C. Malm and W. Z. Sadeh, A residence time probability analysis of sulfur concentrations at grand Canyon National Park, Atmos. Environ., 1985, 19, 1263–1270 CrossRef CAS.
- Q. Zhang, Z. Ning, Z. Shen, G. Li, J. Zhang, Y. Lei, H. Xu, J. Sun, L. Zhang, D. Westerdahl, N. K. Gali and X. Gong, Variations of aerosol size distribution, chemical composition and optical properties from roadside to ambient environment: a case study in Hong Kong, China, Atmos. Environ., 2017, 166, 234–243 CrossRef CAS.
- H. Jiang, G. Wu, T. Li, P. He and R. Chen, Characteristics of Particulate Matter Emissions from a Low-Speed Marine Diesel Engine at Various Loads, Environ. Sci. Technol., 2019, 53, 11552–11559 CrossRef CAS PubMed.
- J. Alanen, M. Isotalo, N. Kuittinen, P. Simonen, S. Martikainen, H. Kuuluvainen, M. Honkanen, K. Lehtoranta, S. Nyyssönen, H. Vesala, H. Timonen, M. Aurela, J. Keskinen and T. Rönkkö, Physical Characteristics of Particle Emissions from a Medium Speed Ship Engine Fueled with Natural Gas and Low-Sulfur Liquid Fuels, Environ. Sci. Technol., 2020, 54, 5376–5384 CrossRef CAS PubMed.
- L. Ntziachristos, Z. Ning, M. D. Geller and C. Sioutas, Particle Concentration and Characteristics near a Major Freeway with Heavy-Duty Diesel Traffic, Environ. Sci. Technol., 2007, 41, 2223–2230 CrossRef CAS PubMed.
- S. Mishra, S. N. Tripathi, V. P. Kanawade, S. L. Haslett, L. Dada, G. Ciarelli, V. Kumar, A. Singh, D. Bhattu, N. Rastogi, K. R. Daellenbach, D. Ganguly, P. Gargava, J. G. Slowik, M. Kulmala, C. Mohr, I. El-Haddad and A. S. H. Prevot, Rapid night-time nanoparticle growth in Delhi driven by biomass-burning emissions, Nat. Geosci., 2023, 16, 224–230 CrossRef CAS.
- H. Man, Y. Zhu, F. Ji, X. Yao, N. T. Lau, Y. Li, B. P. Lee and C. K. Chan, Comparison of Daytime and Nighttime New Particle Growth at the HKUST Supersite in Hong Kong, Environ. Sci. Technol., 2015, 49, 7170–7178 CrossRef CAS PubMed.
- H. Chen, J. Huo, Q. Fu, Y. Duan, H. Xiao and J. Chen, Impact of quarantine measures on chemical compositions of PM2.5 during the COVID-19 epidemic in Shanghai, China, Sci. Total Environ., 2020, 743, 140758 CrossRef CAS PubMed.
- V. Celo, E. Dabek-Zlotorzynska and M. McCurdy, Chemical Characterization of Exhaust Emissions from Selected Canadian Marine Vessels: The Case of Trace Metals and Lanthanoids, Environ. Sci. Technol., 2015, 49, 5220–5226 CrossRef CAS PubMed.
- J. C. Corbin, A. A. Mensah, S. M. Pieber, J. Orasche, B. Michalke, M. Zanatta, H. Czech, D. Massabò, F. Buatier de Mongeot, C. Mennucci, I. El Haddad, N. K. Kumar, B. Stengel, Y. Huang, R. Zimmermann, A. S. H. Prévôt and M. Gysel, Trace Metals in Soot and PM2.5 from Heavy-Fuel-Oil Combustion in a Marine Engine, Environ. Sci. Technol., 2018, 52, 6714–6722 CrossRef CAS PubMed.
- Y.-L. Tseng, C.-S. Yuan, K.-W. Wong and C. Lin, Chemical fingerprints and source resolution of atmospheric fine particles in an industrial harbor based on one-year intermittent field sampling data, Sci. Total Environ., 2023, 868, 161335 CrossRef CAS PubMed.
- G. Yu, Y. Zhang, F. Yang, B. He, C. Zhang, Z. Zou, X. Yang, N. Li and J. Chen, Dynamic Ni/V Ratio in the Ship-Emitted Particles Driven by Multiphase Fuel Oil Regulations in Coastal China, Environ. Sci. Technol., 2021, 55, 15031–15039 CrossRef CAS PubMed.
- B. Alföldy, J. B. Lööv, F. Lagler, J. Mellqvist, N. Berg, J. Beecken, H. Weststrate, J. Duyzer, L. Bencs, B. Horemans, F. Cavalli, J. P. Putaud, G. Janssens-Maenhout, A. P. Csordás, R. Van Grieken, A. Borowiak and J. Hjorth, Measurements of air pollution emission factors for marine transportation in SECA, Atmos. Meas. Tech., 2013, 6, 1777–1791 CrossRef.
- B. Liang, M. Cai, Q. Sun, S. Zhou and J. Zhao, Source apportionment of marine atmospheric aerosols in northern South China Sea during summertime 2018, Environ. Pollut., 2021, 289, 117948 CrossRef CAS PubMed.
- K. Lu, S. Guo, Z. Tan, H. Wang, D. Shang, Y. Liu, X. Li, Z. Wu, M. Hu and Y. Zhang, Exploring atmospheric free-radical chemistry in China: the self-cleansing capacity and the formation of secondary air pollution, Natl. Sci. Rev., 2018, 6, 579–594 CrossRef PubMed.
- Y. Ling, Y. Wang, J. Duan, X. Xie, Y. Liu, Y. Peng, L. Qiao, T. Cheng, S. Lou, H. Wang, X. Li and X. Xing, Long-term aerosol size distributions and the potential role of volatile organic compounds (VOCs) in new particle formation events in Shanghai, Atmos. Environ., 2019, 202, 345–356 CrossRef CAS.
- Y. Zhang, D. Li, Y. Ma, C. Dubois, X. Wang, S. Perrier, H. Chen, H. Wang, S. a. Jing, Y. Lu, S. Lou, C. Yan, W. Nie, J. Chen, C. Huang, C. George and M. Riva, Field Detection of Highly Oxygenated Organic Molecules in Shanghai by Chemical Ionization–Orbitrap, Environ. Sci. Technol., 2022, 56, 7608–7617 CrossRef CAS PubMed.
- L. Yao, O. Garmash, F. Bianchi, J. Zheng, C. Yan, J. Kontkanen, H. Junninen, S. B. Mazon, M. Ehn, P. Paasonen, M. Sipilä, M. Wang, X. Wang, S. Xiao, H. Chen, Y. Lu, B. Zhang, D. Wang, Q. Fu, F. Geng, L. Li, H. Wang, L. Qiao, X. Yang, J. Chen, V.-M. Kerminen, T. Petäjä, D. R. Worsnop, M. Kulmala and L. Wang, Atmospheric new particle formation from sulfuric acid and amines in a Chinese megacity, Science, 2018, 361, 278–281 CrossRef CAS PubMed.
- C. Deng, R. Cai, C. Yan, J. Zheng and J. Jiang, Formation and growth of sub-3 nm particles in megacities: impact of background aerosols, Faraday Discuss., 2021, 226, 348–363 RSC.
- A. Kukui, M. Chartier, J. Wang, H. Chen, S. Dusanter, S. Sauvage, V. Michoud, N. Locoge, V. Gros, T. Bourrianne, K. Sellegri and J. M. Pichon, Role of Criegee intermediates in the formation of sulfuric acid at a Mediterranean (Cape Corsica) site under influence of biogenic emissions, Atmos. Chem. Phys., 2021, 21, 13333–13351 CrossRef CAS.
- J. Xu, C. Jia, H. Yu, H. Xu, D. Ji, C. Wang, H. Xiao and J. He, Characteristics, sources, and health risks of PM2.5-bound trace elements in representative areas of Northern Zhejiang Province, China, Chemosphere, 2021, 272, 129632 CrossRef CAS PubMed.
- Y. Yi, Q. Li, K. Zhang, R. Li, L. Yang, Z. Liu, X. Zhang, S. Wang, Y. Wang, H. Chen, L. Huang, J. Z. Yu and L. Li, Highly time-resolved measurements of elements in PM2.5 in Changzhou, China: temporal variation, source identification and health risks, Sci. Total Environ., 2022, 853, 158450 CrossRef CAS PubMed.
- D. C. S. Beddows, R. M. Harrison, D. C. Green and G. W. Fuller, Receptor modelling of both particle composition and size distribution from a background site in London, UK, Atmos. Chem. Phys., 2015, 15, 10107–10125 CrossRef CAS.
- Z. Liu, B. Hu, J. Zhang, J. Xin, F. Wu, W. Gao, M. Wang and Y. Wang, Characterization of fine particles during the 2014 Asia-Pacific economic cooperation summit: number concentration, size distribution and sources, Tellus B, 2017, 69, 1303228 CrossRef.
- F. Wang, T. Lin, Y. Li, Z. Guo and N. L. Rose, Comparison of PM2.5 carbonaceous pollutants between an urban site in Shanghai and a background site in a coastal East China Sea island in summer: concentration, composition and sources, Environ. Sci.: Processes Impacts, 2017, 19, 833–842 RSC.
- Q. Zhang, W. Hu, H. Ren, J. Yang, J. Deng, D. Wang, Y. Sun, Z. Wang, K. Kawamura and P. Fu, Diurnal variations in primary and secondary organic aerosols in an eastern China coastal city: the impact of land-sea breezes, Environ. Pollut., 2023, 319, 121016 CrossRef CAS PubMed.
- W. Sun, D. Wang, L. Yao, H. Fu, Q. Fu, H. Wang, Q. Li, L. Wang, X. Yang, A. Xian, G. Wang, H. Xiao and J. Chen, Chemistry-triggered events of PM2.5 explosive growth during late autumn and winter in Shanghai, China, Environ. Pollut., 2019, 254, 112864 CrossRef CAS PubMed.
- J. Tan, J. S. Fu, K. Huang, C.-E. Yang, G. Zhuang and J. Sun, Effectiveness of SO2 emission control policy on power plants in the Yangtze River Delta, China—post-assessment of the 11th Five-Year Plan, Environ. Sci. Pollut. Res., 2017, 24, 8243–8255 CrossRef CAS PubMed.
- L. Ma, Y. Zhang, Z. Lin, Y. Zhou, C. Yan, Y. Zhang, W. Zhou, W. Ma, C. Hua, X. Li, C. Deng, Y. Qi, L. Dada, H. Li, F. Bianchi, T. Petäjä, J. Kangasluoma, J. Jiang, S. Liu, T. Hussein, M. Kulmala and Y. Liu, Deposition potential of 0.003–10 μm ambient particles in the humidified human respiratory tract: contribution of new particle formation events in Beijing, Ecotoxicol. Environ. Saf., 2022, 243, 114023 CrossRef CAS PubMed.
- L. Salo, A. Hyvärinen, P. Jalava, K. Teinilä, R. K. Hooda, A. Datta, S. Saarikoski, H. Lintusaari, T. Lepistö, S. Martikainen, A. Rostedt, V. P. Sharma, M. H. Rahman, S. Subudhi, E. Asmi, J. V. Niemi, H. Lihavainen, B. Lal, J. Keskinen, H. Kuuluvainen, H. Timonen and T. Rönkkö, The characteristics and size of lung-depositing particles vary significantly between high and low pollution traffic environments, Atmos. Environ., 2021, 255, 118421 CrossRef CAS.
- T.-L. Chen, C.-H. Lai, Y.-C. Chen, Y.-H. Ho, A. Y. Chen and T.-C. Hsiao, Source-oriented risk and lung-deposited surface area (LDSA) of ultrafine particles in a Southeast Asia urban area, Sci. Total Environ., 2023, 870, 161733 CrossRef CAS PubMed.
- M. Kulmala, V. M. Kerminen, T. Petäjä, A. J. Ding and L. Wang, Atmospheric gas-to-particle conversion: why NPF events are observed in megacities?, Faraday Discuss., 2017, 200, 271–288 RSC.
- J. Mao, Y. Zhang, F. Yu, J. Chen, J. Sun, S. Wang, Z. Zou, J. Zhou, Q. Yu, W. Ma and L. Chen, Simulating the impacts of ship emissions on coastal air quality: importance of a high-resolution emission inventory relative to cruise- and land-based observations, Sci. Total Environ., 2020, 728, 138454 CrossRef CAS PubMed.
|
This journal is © The Royal Society of Chemistry 2023 |