Carbon monoxide fluxes measured using the eddy covariance method from an intensively managed grassland in Ireland†
Received
17th July 2023
, Accepted 6th November 2023
First published on 10th November 2023
Abstract
Carbon monoxide (CO) is classed as a secondary greenhouse gas (GHG) as it can extend the lifetime of GHGs such as methane and ozone by reacting with hydroxyl (OH) radicals and thus controlling the oxidizing capacity of the atmosphere. CO fluxes were measured from a fertilized and grazed temperate grassland in south-east Ireland using a high frequency quantum cascade laser (QCL) and the eddy covariance method. Measurements were carried out in 2019 (12 months) and 2020 (10 months). In both datasets, a diurnal pattern was observed where CO fluxes peaked between 8 am and 6 pm, and between 8 pm and 2 am during the colder months of the year. Quality controlled CO flux data was gap-filled using a general additive model (GAM) that incorporated photosynthetic photon flux density (PPFD), air temperature, water-filled pore space (WFPS) and month of the year. Modelled CO emissions showed less divergence with measured CO fluxes in 2020 compared to 2019, suggesting that the environmental variables in the GAM were the predominant driver of CO emissions in 2020 but not in 2019. Cumulative CO emissions in 2019 and 2020 were 39.7 ± 147.1 mgCO–C per m2 per month and 31.5 ± 75.2 mgCO–C per m2 per month, respectively. While soils are typically considered a sink of CO, our results show that managed pastures can act as a source of CO and thus warrant further investigation into the implications CO may have on GHG dynamics from agricultural landscapes.
Environmental significance
The manuscript entitled “Carbon monoxide fluxes measured using the eddy covariance method from an intensively managed grassland in Ireland” provides significant findings both at a national and international scale. Nationally, the dataset presented in this manuscript is novel as to date there are no published high resolution CO flux measurements, both in time and space, from agricultural soils. Internationally, there are very few datasets of measured CO fluxes from agricultural systems, and of those published the majority represent single point measurements in space and time and thus may not always encapsulate the dynamics of CO emissions at the field scale. The findings from this research show that a temperate grassland in South-East Ireland was a net source of CO, despite soils being globally considered a sink of CO. This finding warrants additional investigations into the drivers of CO fluxes and the implications this gas may have for carbon balances from agricultural systems.
|
1. Introduction
Carbon monoxide (CO) influences the oxidizing capacity of the atmosphere by reacting with hydroxyl (OH) radicals, mainly in the troposphere.1,2 The oxidation of CO can directly lead to the formation of carbon dioxide (CO2),1 and indirectly contribute to lengthening the lifetime (and increasing global warming potential) of other greenhouse gases (GHGs) such as methane and ozone.3 While CO has a relatively short lifetime (on average one to four months)4 and a weak radiative forcing of 0.234 W m−2,5 the total indirect radiative forcing at a global scale has been estimated to be greater than that of nitrous oxide (N2O), thus making CO an important gas species in forcing climate change.5
Global CO emissions are currently estimated at between 1550 and 2900 TgCO per year from both anthropogenic and natural sources6 but in general, soils are considered a global sink of CO due to microbial oxidation of atmospheric CO.7–9 The consumption of CO from the atmosphere is predominately governed by the anabolic activity of soil microorganisms (nitrifiers, carboxydotrophs and methantrophs) that consume CO during growth and release CO2.10 Previous studies have shown that the consumption of CO correlates well with soil carbon content as organic matter incorporated into the soil can serve as a source of energy and carbon for microorganisms.11,12 Moreover, CO exchange between the soil and the atmosphere dominantly occurs in the top 5 cm of the soil.13 Therefore, management practices which both incorporate additional C inputs into the soil (such as slurry application or animal excreta during grazing) and disturb the topsoil may affect the rate of CO emission and uptake.
Microbial activity in soils will also be influenced by changes in environmental conditions, such as humidly, soil moisture and temperature. Current understanding is that at the global scale, CO uptake is typically higher in carbon rich areas in the tropics near the equator where temperatures are generally >30 °C and soil moisture is sufficient enough to sustain microbial communities.6 Conversely, where soil moisture is high, such as in wetland systems, CO uptake is low as a high water-filled pore space (WFPS) will limit oxygen diffusion and gas transport.14
Additionally, CO emissions are also derived from abiotic processes including chemical and biochemical reactions which result in the degradation of organic matter and microorganisms.10 Previous studies have shown a linear relationship between CO emissions and solar radiation,10,15,16 where the exposure of solar radiation at <400 nm wavelength to soils, plant tissues and litter stimulates the production of CO.17 This photochemical production of CO involves the degradation of organic matter by radiation during daylight hours, where carboxyl bond are broken down producing CO2 and CO as a by-product.17,18 In the absence of radiation and possibly oxygen, temperature induced degradation of carbon in a process known as thermal degradation can produce CO emissions from decomposing organic materials and animal excreta,15,19 however the precise mechanisms governing this process are still unclear.20
Due to the many abiotic and biotic factors which influence CO emission dynamics, it is still a very challenging task to quantify field scale emissions of CO with low uncertainty. Historically, the most commonly used analytical techniques for measuring CO fluxes include gas chromatography in combination with a mercuric oxide (HgO) reduction detection or a flame ionization detector (FID), vacuum ultra-violet resonance fluorescence (VURF) and non-dispersive infrared absorption (NDIR).14 The majority of previously published studies on CO fluxes from agricultural landscapes are conducted over a relatively short time period (<1 year) and with few data points derived from static chamber measurements.21–23 In more recent years though, non-invasive, micro-meteorological methods such as eddy covariance (EC) have become available to continuously measure CO flux dynamics at the field scale when equipped with fast response and high frequency gas analysers, such as quantum cascade lasers (QCL).20,24,25 Nonetheless, there is still a lack of available long term data on CO flux dynamics from agricultural soils.26–30 Previously studies have reported contrasting findings in the role of agricultural soils as a source or sink of CO. For example, Cowan, Helfter20 showed that a grazed grassland in Scotland was a net source of CO ranging between 0.35 and 0.8 gC per m2 per year, Sanhueza, Donoso31 reported that a grassland in Venezuela transitioned from a net source of CO to a net sink following ploughing, and Liu, Zhuang6 found that globally, short and tall grasslands were a net sink of CO at a rate of 0.27 and 0.65 TgCO per year.
Quantifying CO emissions from agricultural landscapes is important in order to understand the potentially far-reaching environmental damage that may be caused from intensive management practices. However, the national GHG inventory in Ireland does not account for CO emissions from agricultural soils, thus leaving a considerable knowledge gap in terms of how agricultural landscapes and land-use change can influence the uptake and release of CO. Previous assessments of CO emissions from grassland soils in the UK, estimated that national annual emissions could range between 54.3 and 69.5 Gg CO or 3.4–4.3% of the 2018 GHG inventory total.20 In this study we aim to quantify CO emissions from an intensively managed grassland in Ireland, and identify whether variability in emissions is abiotically driven (i.e. environmental drivers) and if not, identify what are the other potential factors that could explain CO flux dynamics.
2. Materials and methods
2.1 Site description and management
Measurements were made between January 2019 and October 2020 over an intensively managed grassland at Teagasc Johnstown Castle (52.30°N, 6.40°W, 67 m above sea level)32 (Fig. S1†). A main road is located less than 150 meters north of the field site where traffic is heightened during rush hour times, typically in the morning, between 7 am and 9 am and in the late afternoon between 5 pm and 8 pm. The dominant grass species in the field site is perennial ryegrass (Lolium perenne L.) and the soil is a moderately drained sandy loam with a sand/silt/clay content of 53/33/44 in the top 30 cm of the soil profile with a pH of 5.5. The field site compromises of two paddocks, 10 and 11, with an approximate area of 2.65 ha−1 (Fig. S1†). In the north-east of paddock 10, the eddy covariance (EC) tower is located in order to maximize the fetch from the prevailing wind direction in the south-west. Over the last decade, the field site was mainly intensively grazed by Holstein-Friesian cattle with a mean stocking density of 3.2 LSU ha−1. In addition to this, the field site has also been managed for silage production on occasion, where typically the pasture receives 250 kgN ha−1 in the form of calcium ammonium nitrate (CAN) over three to four splits and is also treated with phosphate and phosphorus applications to maintain soil fertility. Details of the management for 2019 and 2020 are described in Murphy, Richards33 and Murphy, Saunders32 but a summary of the management activities is shown in Table S1.† In brief, the field site was under a silage production management in 2019, and received 40, 70, 80 and 40 kgCAN ha−1 on the 5th of March, 1st of April, 5th of June and 11th of September, respectively, and silage was cut on the 14th of May, 4th of July and 5th of September. In 2020, the field site was under a grazing management regime where the herd size varied between 22 and 30 cows and grazing events occurred on nine occasions, with three events in the spring (February, March and April), four events in the summer (May, June and July) and three events in the autumn (August, September and October). Fertilizer applications of CAN were typically made following grazing to promote grass growth for the subsequent grazing event.
2.2 Eddy covariance CO flux measurements
An eddy covariance (EC) mast was installed at 2.2 m and equipped with a 3D sonic anemometer (CSAT-3, Campbell Scientific Ancillary, Logan, UT, USA) to measure changes in the vertical, lateral and horizontal wind components as well as wind direction at a frequency of 10 Hz. CO fluxes were measured at 10 Hz using a quantum cascade laser (QCL) absorption spectrometer (LGR 23R N2O/CO/H2O, Los Gatos Research, California, USA) with a detection limit of 0.03 ppb at a 30 minute interval. Flux data was stored and collected weekly from the CR3000 Micrologger (Campbell Scientific, Logan, UT, USA). The QCL was enclosed within a temperature regulated trailer (161 cm × 98 cm × 127 cm). As the QCL is a closed path gas analyser, a 10 m long, 10 mm inner diameter perfluoroalkoxy (PFA) tube was positioned approximately 30 cm apart from the sonic anemometer in the same horizontal axis and connected to the inlet of the QCL. In order to draw air towards the cell of the QCL, a dry scroll vacuum pump (XDS35i, Edwards, West Sussex, UK) was connected to the QCL using a PDTE clear suction hose with spiral wired rings (2.4 m × 2.5 cm) (Tec Industry, Dublin, Ireland) with an approximate flow rate of 30–35 standard L min−1. To avoid obstructions from debris and contamination of the QCL cell from pollutants, a 2 mm fabric mesh was fitted roughly 2 cm from the tip of the inlet tubing, and the inlet tube was fitted with two inline 2 μm filters where the filter threads were wrapped in polytetrafluoroethylene (PFTE) tape to reduce air leaks. Furthermore, 2 (SS-4FW4-2, Swagelok™), and 10 μm filters (Los Gatos Research, California, USA) were fitted within the QCL at the entrance of the inlet tubing and downstream of the internal pump. The cell temperature was maintained at 34 °C ± 0.5 °C via the internal temperature regulator, and the pressure of the QCL was set at 85 Torr (11.3 kPa) and the replacement rate of air within the cell was 0.097 s−1.
EC fluxes of CO were calculated over 30 minute interval using the Eddypro software version 7.0.6 (http://www.licor.com/eddypro) based on the covariance between the CO concentration (ppb) (C) and wind speed (w) eqn (1)
|  | (1) |
In the flux calculation process, CO fluxes were initially screened for amplitude resolution, drop-outs, absolute limits, skewness and kurtosis, as described by Vickers and Mahrt.34 Double rotation (vertical and cross wind) was performed to nullify the mean cross-stream and vertical wind component.35 Corrections for the both the low and high frequency losses were made using analytical methods outlined in Fratini, Ibrom36 and Moncrieff, Clement,37 respectively. Due to the majority of flux measurements being relatively low in magnitude, the time lag between the sonic anemometer and the QCL was calculated outside of the Eddypro software, using the covariance maximization method as described by Murphy, Richards.33 Here, to reduce uncertainty in the maximization of covariance, data over a six hour block was used to determine time-lag over a window of 10 seconds and once a steady state time lag was determined over the measurement period a second covariance maximization of the same six hour block of data was conducted for a shorter window of 0.3 seconds, using a median running time lag over a 7 day period as the mid-point. The wind data and CO mixing ratio were then re-paired with a fixed time lag of −0.5 seconds based on the last maximization of covariance over a 30 minute period and Eddypro was run using “fixed time lag” settings.
Measured CO fluxes were filtered into two treatments – (a) broad CO fluxes (COBroad), where flux values were filtered but included measurements from potential sources of anthropogenic CO fluxes i.e. the main road and roads within the farm ground adjacent to the field site as well as during periods of rush-hour traffic and (b) filtered CO fluxes (COFilter), where CO fluxes believed to be influenced from anthropogenic sources were omitted.
For COBroad fluxes, flux values were retained within the dataset if at least 70% of the flux contribution came from inside of the boundaries of the field site, as determined by the analytical footprint model described by Kormann and Meixner.38 Additional quality control procedures included removing poor quality fluxes as defined by the quality control method of Foken39 for category 5 or higher; removing fluxes during low turbulent conditions defined as a friction velocity (u*) of <0.1 m−2 s−1 and fluxes that were deemed unrealistic for the field site. Uncertainty in measured CO fluxes was calculated using the method by Finkelstein and Sims40 integrated over a fixed 10 second correlation period. After removing flux values according to the above criteria, 30% of total measured flux values were retained in the dataset.
For COFilter fluxes the above filtering protocol was applied and additionally, CO flux values measured from potential anthropogenic sources were also removed. This included removing CO fluxes measured from the North-West and North-East as the main road and lanes within the farm were located in this wind direction (Fig. S1†). Furthermore, CO fluxes deemed to be related to vehicle exhaust emissions from rush hour traffic on the main road and from farm machinery were removed. This involved removing half-hourly CO fluxes where the measured CO concentration for that period was greater than the daily mean CO concentration. This method was chosen as opposed to removing CO fluxes over rush-hour traffic times, in order to retain flux values that were representative of the natural production of CO through photodegradation which otherwise would have been omitted for example, midday CO fluxes. Following this additional filtering step, 13% of total measured CO fluxes were retained.
2.3 Ancillary data
A range of ancillary sensors were equipped to the EC mast and data was recorded using the CR3000 Micrologger (Campbell Scientific, Logan, UT, USA). These sensors included an air temperature and relative humidity probe (HMP155C, Campbell Scientific, Logan, UT, USA), tipping bucket rain gauge (Young, Michigan, USA), photosynthetic active radiation (PAR) (PQS1, Kipp and Zonen, Delft, The Netherlands), two net radiation sensors (NR-Lite, Kipp and Zonen, Delft, The Netherlands), two self-calibrating soil heat flux plates installed at 5 cm soil depth (HFP01SC, Hukseflux, Delft, The Netherlands), averaging soil temperature probes (TCAV-L, Campbell Scientific, Logan, UT, USA) that were installed at 2 cm and 6 cm depth above the soil heat flux plates and time domain reflectometers (CS616, Campbell Scientific, Logan, UT, USA) that measured soil volumetric water content (VWC) in the upper 15 cm of soil. The bulk density of the field site was determined by taking soils samples from the surface topsoil across the field site (n = 30) using sharpened cylindrical rings (10 cm depth; 3.7 cm diameter) prior to commencing the experiment. Measurements of soil bulk density were used to calculate water-filled pore space (WFPS) by dividing VWC by the total porosity of a given bulk density sample.41
2.4 Black carbon concentrations
In order to further assess the influence that advection contamination to the field site may have on measured CO fluxes, black carbon concentration data was used as an indicator for regional anthropogenic activity. Black carbon is produced from the burning of fossil fuels, namely through gas and diesel exhausts, as well as coal-fired power plants,42 and thus is a strong indicator of anthropogenic activity. Black carbon concentrations (ng m−3) were measured at a long term weather and GHG monitoring station at a height of 6 m at Carnsore Point (52° 10′N, 6° 21′W). Carnsore Point is approximately 24 km from the experimental field site and therefore provides an indication of the regional air pollution. This meteorological station is part of the national Atmospheric Composition and Climate Change (AC3) Network which monitors black carbon concentrations.43 Black carbon concentrations were measured using an aethalometer (Model AE33 Magee Scientific, Berkeley, USA) at 1 Hz and blocked averaged over an hourly timescale. The AE33 aethalometer operates over seven wavelengths of light ranging from 370 to 9550 nm, including 880 nm, the wavelength at which black carbon absorbs most strongly relative to other substances. Black carbon concentrations are calculated as the rate of change from gas samples collected at different wavelengths.44 The limit of detection from the AE33 was <0.005 μg m−3 and the flow rate was 5 L min−1. The AE33 was equipped with an inlet tube (100 mm) to draw air into the cell of the instrument at a rate of 39 m3 h−1. The intakes are positioned 12 m above the ground surface and equipped with a PM10 sizer which filters particles sizes below 10 μm diameter.44 A detailed description of routine instrument maintenance procedures including leak checks, gas cylinder pressure control, filter replacement, valco valve cleaning and replacement are outlined in Martin and O'Dowd.45
2.5 Data analysis
Measured half-hourly CO fluxes were normalized using the Anderson–Darling normality test46 from the package nortest in rstudio. The Anderson–Darling normality test was chosen as it allows normality screening on large datasets in rstudio (n > 5000). Normalized CO fluxes were incorporated into a multivariate linear model which consisted of half-hourly measurements of PPFD, air temperature and WFPS to identify significant differences in measured CO fluxes and environmental variables.
COBroad and COFilter fluxes were gap-filled using a general additive model (GAM). The GAM method is essentially a linear model that accounts for the non-linear responses of environmental variables to temporal changes in CO fluxes by fitting a smooth response with cubic splines.47,48 The GAM was similar to that described by Cowan, Helfter,20 where gaps in measured half-hourly CO fluxes were modelled based on environmental variables which included PPFD, air temperature and WFPS as well as month of the year to account for temporal variability in measured CO fluxes, using the mgcv package in the R software. The uncertainty in modelled CO fluxes from the GAM method was calculated as the 95% confidence interval from the standard error associated with the posterior distribution of the model coefficients.47 A linear regression between gap-filled COBroad and COFilter fluxes was performed to identify the influence of advection contamination from traffic on measured CO fluxes. Additionally, modelled CO fluxes from both treatments were used to calculate cumulative CO emissions in both experimental years. Throughout this paper positive CO fluxes represent CO emissions from the soil to the atmosphere, and negative CO fluxes represent CO uptake from the atmosphere to the soil.
3. Results
3.1 Temporal patterns in measured CO fluxes
Mean monthly fluxes of CO showed greater variability in 2019 compared to 2020 in both COBroad and COFilter flux datasets, with an overall range of −0.32 to 0.88 nmolCO m−2 s−1 in 2019 and −0.05 to 0.73 nmolCO m−2 s−`1 in 2020 (Table 1). Higher monthly emissions typically coincided with high rainfall (>70 mm) and subsequently a high soil moisture content (WFPS ≥ 60%), while a lower soil moisture content (WFPS < 60%) and high temperatures (15 °C) coincided with CO uptake (Table 1). Trends in CO fluxes and binned values of PPFD, air temperature, WFPS and rainfall showed that CO fluxes increased with increasing PPFD, but the response of measured CO fluxes to air temperature, WFPS and rainfall was more varied (Fig. 1). Half-hourly CO fluxes were significantly different (as defined as a p-value < 0.05) with PPFD, air temperature and WFPS. A diurnal pattern was observed in the measured CO flux data, where baseline half-hourly emissions of CO (<1 nmolCO m−2 s−1) were elevated (approximately 1 to 5 nmolCO m−2 s−1) between 8 am and 6 pm, and occasionally between 8 pm and 2 am in the spring and winter months (Fig. 2 and 3). At night, mean flux values typically reached near zero or negative (generally less than −2 nmolCO m−2 s−1).
Table 1 Monthly summary statistics on weather (air temperature, water-filled pore space [WFPS], photosynthetic photon flux density [PPFD] and rainfall) and broad filtered CO fluxes (COBroad) and filtered CO fluxes (COFilter) accounting for anthropogenic sources, February, March and April represent spring months, May, June and July represent summer months, August, September and October represent autumn months and November, December and January represent winter months
Date |
Weather |
CO flux (nmol m−2 s−1) |
Year |
Month |
Air temperature (°C) |
WFPS (%) |
PPFD (μmol m−2 s−1) |
Rainfall (mm) |
COBroad fluxes |
COFilter fluxes |
Mean |
Percentiles (2.5–97.5%) |
Mean |
Percentiles (2.5–97.5%) |
Lower |
Upper |
Lower |
Upper |
2019 |
January |
6.4 |
53.1 |
65.7 |
38.4 |
0.02 |
−2.76 |
3.07 |
0.05 |
−1.21 |
2.85 |
February |
7.8 |
58.2 |
134 |
48.3 |
0.15 |
−1.87 |
3.61 |
0.22 |
−1.23 |
3.67 |
March |
7.6 |
61.3 |
218.7 |
97.8 |
0.52 |
−1.56 |
4.23 |
0.58 |
−1.21 |
4.06 |
April |
8.6 |
64.3 |
283.9 |
85.9 |
0.31 |
−4.01 |
4.01 |
0.35 |
−3.56 |
3.42 |
May |
10.9 |
59.1 |
449 |
24.2 |
0.23 |
−1.47 |
3.36 |
0.21 |
−1.14 |
3.73 |
June |
12.7 |
58 |
484.4 |
77.1 |
0.40 |
−1.28 |
3.56 |
0.46 |
−1.10 |
3.39 |
July |
15.8 |
46.5 |
461.3 |
32.7 |
−0.06 |
−1.16 |
2.71 |
−0.32 |
−1.15 |
1.18 |
August |
15.5 |
56 |
380.4 |
122.2 |
−0.24 |
−1.57 |
1.98 |
−0.25 |
−1.08 |
1.25 |
September |
13.8 |
51 |
282 |
74.8 |
−0.12 |
−1.76 |
3.20 |
−0.28 |
−1.58 |
2.42 |
October |
10.4 |
63.6 |
155.2 |
136.2 |
0.26 |
−1.22 |
2.69 |
0.15 |
−1.16 |
2.66 |
November |
7.3 |
62.1 |
78.4 |
150.8 |
0.88 |
−1.86 |
6.13 |
0.88 |
−1.39 |
4.66 |
December |
7 |
60.5 |
50.9 |
71 |
0.87 |
−2.33 |
5.79 |
0.66 |
−2.39 |
3.85 |
2020 |
January |
6.8 |
61.8 |
63.3 |
101.8 |
0.73 |
−1.73 |
4.08 |
0.73 |
−1.43 |
4.44 |
February |
6.6 |
64.9 |
121.6 |
152.8 |
0.72 |
−1.34 |
3.93 |
0.61 |
−0.82 |
2.86 |
March |
6.6 |
63.5 |
236.3 |
47.9 |
0.59 |
−0.81 |
3.58 |
0.50 |
−0.78 |
2.39 |
April |
9.4 |
60.3 |
361.9 |
50.5 |
0.26 |
−1.72 |
2.47 |
0.20 |
−1.28 |
1.26 |
May |
11.3 |
52.8 |
510 |
17.7 |
0.27 |
−0.72 |
2.36 |
0.24 |
−0.58 |
2.46 |
June |
13.5 |
42.8 |
411.5 |
91.1 |
0.56 |
−0.37 |
1.76 |
0.44 |
−0.24 |
1.52 |
July |
14.5 |
53.9 |
379.7 |
88.5 |
0.52 |
−0.25 |
1.81 |
0.40 |
−0.22 |
1.39 |
August |
15.4 |
58 |
303.4 |
129.5 |
0.36 |
−0.32 |
1.38 |
0.36 |
−0.13 |
1.15 |
September |
13.5 |
58.4 |
270.8 |
47.6 |
0.32 |
−0.61 |
1.84 |
0.24 |
−0.53 |
1.47 |
October |
10.5 |
69.1 |
164.2 |
135.7 |
0.13 |
−0.97 |
1.69 |
−0.05 |
−0.51 |
0.53 |
 |
| Fig. 1 Mean measured COBroad flux data binned by environmental data including photosynthetic photon flux density (PPFD), air temperature, water-filled pore space (WFPS) and rainfall where the shaded areas represent the standard deviation. | |
 |
| Fig. 2 Temporal trends in measured (grey) broad filtered CO fluxes (COBroad), and modelled (orange) CO fluxes derived from the GAM model for (a) 2019 and (b) 2020. The centre line in the box plot represents the median and the whiskers either side represent the 95% confidence interval. Spring months include February, March and April, summer months include May, June and July, autumn months include August, September and October, and winter months include November, December and January. | |
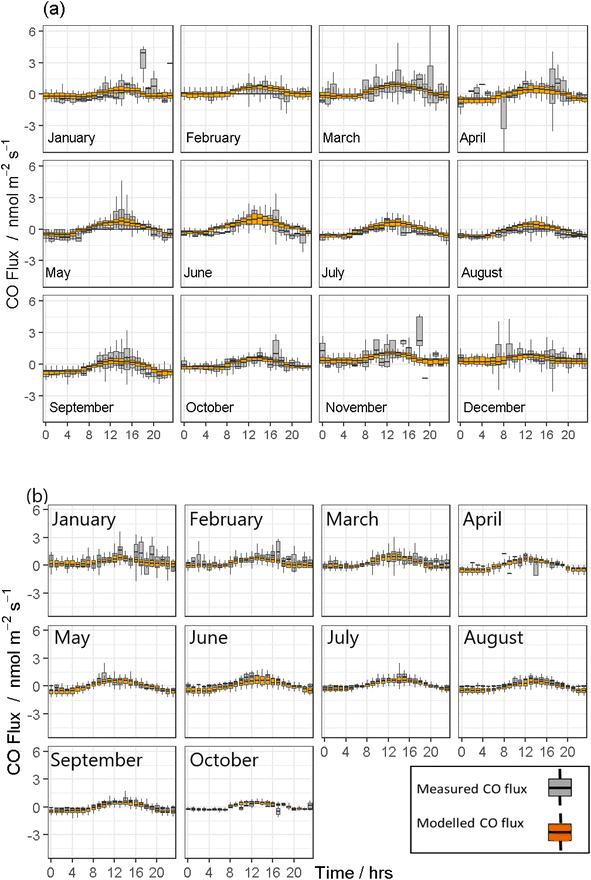 |
| Fig. 3 Temporal trends in measured (grey) filtered CO fluxes (COFilter) and modelled (orange) CO fluxes derived from the GAM model for (a) 2019 and (b) 2020. The centre line in the box plot represents the median and the whiskers either side represent the 95% confidence interval. Spring months include February, March and April, summer months include May, June and July, autumn months include August, September and October, and winter months include November, December and January. | |
Both modelled CO fluxes from the COBroad (Fig. 2) and COFilter (Fig. 3) datasets followed a similar temporal pattern to measured CO fluxes as described above. In general, the COBroad fluxes showed greater disparities with the modelled fluxes compared to the COFilter dataset. This pattern is most noticeable in 2019, for example, in the COBroad dataset, the model showed over-estimations between 4 am and 8 am in January, March, June and September, and between 7 am and 4 pm in August. This suggests that natural drivers are not of primary importance in driving observed CO flux dynamics for this period. These times correlate with periods where higher fossil fuel consumption would be expected (i.e. from farm machinery, vehicles and heating systems in the adjacent surroundings) and in turn, suggests some form of contamination to measured field fluxes of CO. Similar temporal trends were also observed in black carbon concentrations measured in 2019 (Fig. 4a). Baseline concentrations of <100 ngC m−3 were frequently elevated to >300 ngC m−3 between the hours of 4 am and 8 am as well as 4 pm to 8 pm, most noticeably in the colder months of the year i.e. January, February, October and November. Maximum black carbon concentrations were approximately 1500 ngC m−3 and were typically measured between 6 pm and 8 pm during the spring and winter months. In contrast, the COFilter fluxes in 2019 showed less variation, with typically lower flux values compared to the COBroad fluxes, for example in January, November and December. The COFilter fluxes also showed greater alignment with the modelled CO fluxes suggesting that there was less CO contamination from anthropogenic sources in this dataset, and that natural abiotic drivers of CO were more dominant in influencing CO dynamics at the field site.
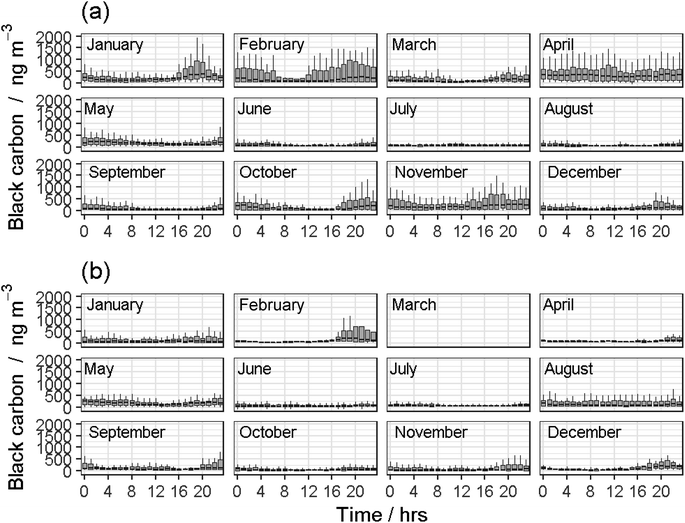 |
| Fig. 4 Temporal trends in hourly black carbon concentrations measured in (a) 2019 and (b) 2020. Note that measurements were not available in March in 2020. The centre line in the box plot represents the median and the whiskers either side represent the 95% confidence interval. Spring months include February, March and April, summer months include May, June and July, autumn months include August, September and October, and winter months include November, December and January. | |
In contrast, measured CO emissions from both the COBroad and COFilter datasets in 2020 showed less divergence from the modelled emissions and in particular, between April and October which coincided with the national lockdown in response to the COVID-19 global pandemic. The reduction in anthropogenic emissions in response to the national lockdown was also evident in measurements of black carbon concentrations in 2020. The maximum upper (97.5% quantile) concentration value was measured at 1584 ngC m−3 in February prior to the lockdown, and was 655 ngC m−3 in August during the lockdown (Fig. 4b). In contrast, the maximum upper concentration value measured in 2019 between April and October (for comparison with the lockdown period in 2020) was an order of magnitude greater at 1443 ngC m−3 in April.
3.2 Cumulative CO fluxes
Gapfilled CO fluxes were used to calculate the annual cumulative CO flux for 2019 and the total CO flux from January to October in 2020 for both COBroad (Fig. 5a) and COFilter datasets (Fig. 5b) (Table 2). Overall, the grassland was a net source of CO in both years, showing higher total losses in 2019 compared to 2020, and higher CO emissions from the COBroad dataset (2019: 67.1 ± 108.7 mgCO–C per m2 per month; 2020: 62.5 ± 73.9 mgCO–C per m2 per month) compared to the COFilter data set (2019: 39.7 ± 147.1 mgCO–C per m2 per month; 2020: 31.5 ± 75.2 mgCO–C per m2 per month). Annual cumulative emissions calculated from COBroad fluxes were on average, 55% higher compared to annual cumulative emissions calculated from the COFilter fluxes. In both years the system was a net source of CO between January and March and transitioned into a net sink of CO in April.
 |
| Fig. 5 Monthly cumulative emissions (positive values) and uptake (negative values) of CO calculated for 2019 (orange) and 2020 (blue) for (a) COBroad and (b) COFilter CO fluxes. Error bars represent the 95% confidence interval. The purple and green trend line represents the net monthly flux in 2019 and 2020, respectively. | |
Table 2 Summary statistics on monthly cumulative CO fluxes calculated in 2019 and 2020 for broad filtered CO fluxes (COBroad), and CO fluxes filtered for anthropogenic sources of CO (COFilter)
|
Cumulative CO flux/mgC per m2 per month |
COBroad |
COFilter |
Year |
Month |
Uptake |
95% C.I. |
Emission |
95% C.I. |
Net flux |
Uptake |
95% C.I. |
Emission |
95% C.I. |
Net flux |
2019 |
January |
−3.3 |
2.4 |
9.0 |
7.3 |
5.7 |
−5.2 |
4.8 |
6.0 |
4.4 |
0.7 |
February |
−2.3 |
2.6 |
10.1 |
5.9 |
7.8 |
−3.0 |
2.7 |
9.3 |
5.3 |
6.4 |
March |
−3.3 |
3.2 |
15.2 |
8.2 |
11.8 |
−3.4 |
3.0 |
13.5 |
6.6 |
10.1 |
April |
−11.2 |
5.8 |
7.5 |
4.5 |
−3.7 |
−9.1 |
5.5 |
6.8 |
4.2 |
−2.3 |
May |
−6.5 |
3.7 |
8.8 |
3.7 |
2.3 |
−6.3 |
4.0 |
8.7 |
4.3 |
2.4 |
June |
−4.8 |
3.0 |
12.4 |
5.6 |
7.6 |
−3.6 |
3.0 |
11.9 |
5.5 |
8.3 |
July |
−8.2 |
3.6 |
7.1 |
3.4 |
−1.1 |
−8.2 |
4.2 |
6.5 |
3.7 |
−1.6 |
August |
−12.0 |
5.3 |
5.2 |
3.1 |
−6.8 |
−10.4 |
4.7 |
4.3 |
2.8 |
−6.1 |
September |
−13.0 |
5.1 |
6.3 |
3.3 |
−6.7 |
−13.9 |
5.7 |
3.8 |
2.4 |
−10.1 |
October |
−4.5 |
3.6 |
8.2 |
4.8 |
3.7 |
−6.1 |
4.8 |
6.4 |
4.2 |
0.3 |
November |
−0.8 |
0.9 |
22.3 |
7.6 |
21.4 |
−0.9 |
1.4 |
18.1 |
8.6 |
17.2 |
December |
−1.6 |
1.5 |
26.6 |
10.6 |
25.1 |
−1.5 |
1.9 |
15.9 |
8.5 |
14.4 |
Total
|
−71.6
|
12.7
|
138.6
|
21.1
|
67.1
|
−71.6
|
14.0
|
111.1
|
18.7
|
39.6
|
|
Total January to October
|
−69.1
|
12.6
|
89.8
|
16.6
|
20.6
|
−69.2
|
13.8
|
77.2
|
14.2
|
8.1
|
2020 |
January |
−2.0 |
1.9 |
19.9 |
9.9 |
17.9 |
−1.4 |
2.1 |
14.5 |
7.5 |
13.1 |
February |
−1.9 |
1.5 |
18.3 |
9.7 |
16.4 |
−2.0 |
2.4 |
12.0 |
6.5 |
9.9 |
March |
−2.0 |
2.0 |
14.9 |
7.2 |
12.9 |
−3.4 |
3.2 |
11.4 |
4.9 |
8.0 |
April |
−9.8 |
4.1 |
5.4 |
2.8 |
−4.4 |
−7.2 |
4.1 |
6.4 |
3.8 |
−0.9 |
May |
−7.0 |
3.2 |
7.9 |
3.3 |
0.9 |
−7.5 |
3.9 |
7.4 |
4.0 |
−0.1 |
June |
−3.1 |
2.5 |
11.0 |
4.8 |
7.8 |
−6.0 |
4.6 |
7.2 |
4.3 |
1.2 |
July |
−2.7 |
1.8 |
10.9 |
4.0 |
8.2 |
−3.7 |
2.9 |
8.5 |
3.7 |
4.8 |
August |
−5.8 |
3.4 |
6.1 |
2.6 |
0.3 |
−7.1 |
4.2 |
4.8 |
2.8 |
−2.3 |
September |
−5.8 |
3.1 |
6.3 |
3.0 |
0.5 |
−7.7 |
4.2 |
4.7 |
2.6 |
−2.9 |
October |
−1.5 |
1.3 |
3.5 |
1.8 |
2.0 |
−1.9 |
1.8 |
2.6 |
1.7 |
0.7 |
Total
|
−41.7
|
8.3
|
104.2
|
17.9
|
62.5
|
−47.9
|
11.0
|
79.5
|
14.2
|
31.5
|
In 2019, the grassland returned back into a net source of CO in May and June followed by a shift to a net sink in July and September. Between October and December, the grassland was a large net source of CO, showing a higher net monthly cumulative flux in the COBroad dataset (maximum in December at a rate of 25.1 1 mgCO–C per m2 per month) compared to the COFilter dataset (maximum in November at a rate of 17.2 1 mgCO–C per m2 per month). For comparability with the same timeframe as 2020 CO flux measurements, total cumulative CO fluxes were recalculated excluding CO measurements from November and December. Re-calculated net cumulative CO fluxes were lower relative to 2020 but the system remained a source of CO at a rate of 8.1 ± 14.2 mgCO–C per m2 per month.
From April onwards in 2020, the grassland was a net source of CO from the COBroad dataset showing highest net fluxes of CO at a rate of 7.8 and 8.2 mgCO–C per m2 per month in June and July, respectively. In contrast, the COFilter flux dataset showed that the grassland was a net sink of CO in May, August and September, varying between −0.1 and −2.9 mgCO–C per m2 per month.
4. Discussion
4.1 CO fluxes
Globally, there are very few studies which have quantified CO fluxes from terrestrial ecosystems, of which the majority are based on single point measurements in space and time by the chamber technique.31 At the national scale, the data in this study has not been previously measured at the same temporal or spatial scales, where we present a long term (22 months) CO flux dataset by eddy covariance. Moreover, the data shown was measured during the COVID-19 pandemic and thus represents rare experimental conditions that are relevant to CO flux dynamics i.e. contamination from surrounding sources of fossil fuel combustion.
The fluxes reported in this study are within the same order of magnitude to previously reported fluxes of CO from grasslands using either static chambers or eddy covariance, ranging from −3 to 14 nmol m−2 s−1.20,24,30 Uptake of CO generally occurred at night and is likely related to the soil microbial consumption of CO whereby microorganisms oxidize CO, utilizing the C as an energy source.10 Emissions of CO typically peaked during 8 am and 6 pm with coincides with both periods of frequent traffic as well as the highest recorded PPFD fluxes (Fig. S2†) which suggests that CO emissions during this period could be in part produced by abiotic processes (i.e. photo-degradation). It is also possible that CO emissions in 2019 were in part derived from silage production events in May, July and September (Table S1†). For example, Zhao49 showed elevated CO concentrations between 3 and 5 ppm, measured using a QCL from silage sources in San Joaquin Valley and California. In general, these findings are consistent with previous reports of diurnal trends in CO emissions from other managed grasslands,20 as well as croplands,25 wetlands24 and forests.50
The correlation between modelled COBroad and COFilter fluxes was relatively low (r2 = 0.44; Fig. S3†), indicating that the method for stripping out CO fluxes from traffic and surrounding anthropogenic sources (Section 2.2) has had a considerable impact on the data. In general, the divergence between measured and modelled CO fluxes is greater in the spring and winter months, which represents colder times of the year. During these periods, it is typical to expect an increase in emissions of CO from insulation systems from nearby buildings as well as from car exhausts, while microbial activity will slow. This assumption is further reinforced in temporal trends in the black carbon concentration data which showed elevated concentrations during the same colder periods of the year. The seasonal variation in black carbon concentrations has previously been reported at Carnsore Point.45 It is important to note however, that black carbon measurements are only an indication of the regional air pollution and not local air pollution at the field site as measurements of CO and black carbon were made over different spatial domains. At local scales, similar observations in the temporal variability in CO fluxes and other anthropogenic gas indicators have been reported. For example, Cowan, Helfter20 showed increases in nitric oxide (NO) and nitrogen dioxide (NO2) concentrations which coincided with increases in CO emissions during the winter months from a Scottish managed grassland. Interestingly, measured CO fluxes in 2020 from May to October show nearly no divergence from the modelled flux which suggests that emissions were primarily driven by environmental variables. It is important to note that this period of the experimental campaign represents a nationwide lockdown, whereby citizens were not permitted to travel freely within the country in an effort to curtail the spread of the coronavirus disease. Temporal trends and lower concentrations in measured black carbon data in 2020 further indicate a reduction in anthropogenic activity during the lockdown period which suggests that advection contamination from adjacent anthropogenic sources was less influential in driving CO emissions in 2020 compared to 2019.
4.2 Cumulative CO emissions
In both experimental years and treatments, the grassland was a net source of CO, where higher cumulative CO emissions were consistently measured in the COBroad datasets compared to the COFilter datasets, and a higher net cumulative flux was measured in 2019 compared to 2020. However, it is important to note that the 2020 dataset was shorter (January to October) compared to the 2019 dataset (January to December) which ultimately contributes to why cumulative CO fluxes reported in this study are lower in 2020 compared to 2019. When comparing net cumulative CO fluxes over the same time period (January to October), 2020 had higher total CO fluxes relative to 2019 in both the COBroad and COFilter dataset. This was due to an overall higher net emission relative to net uptake in 2020, namely during January, February and March. This period was wetter in 2020 (61.8–63.5% WFPS) compared to 2019 (53.1–61.3% WFPS), thus favouring greater anaerobic conditions which reduce the potential for CO consumption by soil microbes. Similar findings have been reported for soil derived methane (CH4) emissions from agricultural soils, where the presence of a high soil moisture content (>80% VWC) hinders the microbial consumption of CH4 by creating anaerobic soil conditions.51 The highest rates of monthly net uptake of CO were measured in 2019, namely in August (−6.1 ± 2.8 mgCO–C per m2 per month) and September (−10.1 ± 2.4 mgCO–C per m2 per month). Higher net uptake during these months is likely due to the presence of favourable conditions for CO consumption by microbial communities such as high temperatures (13.8–15.8 °C) and adequate soil moisture (46.5–56%) and rainfall (32.7–122.2 mm), in combination with possible CO deposition from adjacent sources for example, car exhausts from the main road located <150 meters from the field site as well as from farm machinery exhausts.
What is interesting however, is when anthropogenic sources of CO surrounding the field site are removed from the dataset, the grassland is still a source of CO although far lower than when contaminated CO fluxes are retained in the data (59% lower in 2019 and 50% lower in 2020). Globally grassland soils are considered a sink of CO ranging from −0.27 to −0.65 TgCO per year,6 however our study shows that these ecosystems can serve as a source of CO. While measurements of CO flux at the annual scale are scarce, previous studies have reported net CO fluxes of 350 to 380 mgCO–C per m2 per year from grassland soils,20 −111 mgCO per m2 per year from bio-energy crops25 and −86 to 16 TgCO per year from tropical evergreen forests globally.6 As there is still much inconsistency between previously reported CO fluxes, there is a necessity for longer term datasets of CO fluxes from different managements, climates and soil types to improve our understanding of CO flux dynamics from agricultural landscapes.
5. Conclusions
In this study we reported that an intensively managed Irish grassland was a source of CO in both 2019 and 2020, despite grassland soils being globally recognized as a sink of CO. After local pollution sources were filtered from the data, total CO emissions in 2019 and 2020 were 39.7 ± 147.1 mgCO–C per m2 per month and 31.5 ± 75.2 mgCO–C per m2 per month, respectively. Our study shows that without filtering out identified anthropogenic sources of CO, soil derived annual CO emissions from this grassland system would have been overestimated by 50–59%. In general, the GAM model with natural variables showed less divergence with measured fluxes in 2020 relative to 2019, which suggests that measured CO fluxes in 2020 were mainly environmentally driven with less contamination from fossil fuel combustion sources compared to 2019. Furthermore, where anthropogenic sources of CO were excluded from the dataset in both years, the GAM fitted better compared to when anthropogenic sources were included. The influence of environmental variables (WFPS, PPFD, rainfall and air temperature) on CO flux dynamics was observed in the data in both years through evidence of a diurnal trend in CO fluxes. CO fluxes generally peaked between 8 am and 6 pm, and during the colder months of the year, between 8 pm and 2 am. CO fluxes measured during the night-time typically reached near zero or negative. In order to fully understand atmospheric CO flux dynamics from agricultural landscapes, additional research is warranted under different agricultural systems, weather conditions and soils, with the overarching goal of identifying and mitigating against any harmful environmental impacts that could arise from increases CO emissions.
Conflicts of interest
The authors declare that they have no known competing financial interests or personal relationships that could have appeared to influence the work reported in this paper.
Acknowledgements
The authors gratefully acknowledge A. Lawless for facilitating the research on the Johnstown Castle Dairy Farm and J. Rambaud for technical support with the QCL. This research was financially supported under the National Development Plan, through the Research Stimulus Fund, administered by the Department of Agriculture, Food and the Marine, Manipulation and Integration of Nitrogen Emissions (MINE) grant number 15S655. We acknowledge contribution from UKSCAPE Programme, funded by the Natural Environment Research Council as National Capability (award number NE/R016429/1). We acknowledge contribution form the Environmental Protection Agency (EPA) for instrumentation for black carbon concentrations and infrastructure at Carnsore Point.
References
- J. A. Logan, M. J. Prather, S. C. Wofsy and M. B. Mcelroy, Tropospheric chemistry–A global perspective, J. Geophys. Res., 1981, 86, 7210–7254 CrossRef CAS.
-
M. Prather,D. Ehhalt, F. Dentener, R. Derwent, E. Dlugokencky, E. Holland, et al., Atmospheric Chemistry and Greenhouse Gases, 2001, pp. 239–287 Search PubMed.
- Z. Tan and Q. Zhuang, An analysis of atmospheric CH4 concentrations from 1984 to 2008 with a single box atmospheric chemistry model, Atmos. Chem. Phys. Discuss., 2012, 12(11), 30259–30282 Search PubMed.
-
V. Masson-Delmotte, P. Zhai, A. Pirani, S. L. Connors, C. Péan, S. Berger, et al., Climate change 2021: the physical science basis. Contribution of working group I to the sixth assessment report of the intergovernmental panel on climate change, 2021 Jun, 2 Search PubMed.
- G. Myhre, D. Shindell, F. Bréon, W. Collins, J. Fuglestvedt, J. Huang, A. Robock, G. Stephens, T. Takemura and H. Zhang, Anthropogenic and Natural Radiative Forcing, Clim. Change, 2013, 659–740 Search PubMed.
- L. Liu, Q. Zhuang, Q. Zhu, S. Liu, H. van Asperen and M. Pihlatie, Global soil consumption of atmospheric carbon monoxide: an analysis using a process-based biogeochemistry model, Atmos. Chem. Phys., 2018, 18(11), 7913–7931 CrossRef CAS.
- G. M. King and C. F. Weber, Distribution, diversity and ecology of aerobic CO-oxidizing bacteria, Nat. Rev. Microbiol., 2007, 5(2), 107–118 CrossRef CAS PubMed.
-
W. Seiler, The influence of the biosphere on the atmospheric CO and H2 cycles, Environmental Biogeochemistry and Geomicrobiology, 3 WE Krumbein, 773–810, Ann Arbor Science Publishers, Ann Arbor, Michigan. 1978 Search PubMed.
- S. Whalen and W. Reeburgh, Carbon monoxide consumption in upland boreal forest soils, Soil Biol. Biochem., 2001, 33(10), 1329–1338 CrossRef CAS.
- R. Conrad and W. Seiler, Role of microorganisms in the consumption and production of atmospheric carbon monoxide by soil, Appl. Environ. Microbiol., 1980, 40(3), 437–445 CrossRef CAS PubMed.
- R. Ingersoll, R. Inman and W. Fisher, Soil's potential as a sink for atmospheric carbon monoxide, Tellus, 1974, 26(1–2), 151–159 CAS.
- R. E. Inman, R. B. Ingersoll and E. A. Levy, Soil: a natural sink for carbon monoxide, Science, 1971, 172(3989), 1229–1231 CrossRef CAS PubMed.
- G. King, Attributes of atmospheric carbon monoxide oxidation by Maine forest soils, Appl. Environ. Microbiol., 1999, 65(12), 5257–5264 CrossRef CAS PubMed.
- K. Sobieraj, S. Stegenta-Dąbrowska, G. Luo, J. A. Koziel and A. Białowiec, Carbon Monoxide Fate in the Environment as an Inspiration For Biorefinery Industry: A Review, Front. Environ. Sci., 2022, 10 DOI:10.3389/fenvs.2022.822463.
- H. van Asperen, T. Warneke, S. Sabbatini, G. Nicolini, D. Papale and J. Notholt, The role of photo- and thermal degradation for CO2 and CO fluxes in an arid ecosystem, Biogeosciences, 2015, 12(13), 4161–4174 CrossRef.
- S. Yonemura, S. Kawashima and H. Tsuruta, Carbon monoxide, hydrogen, and methane uptake by soils in a temperate arable field and a forest, J. Geophys. Res.: Atmos., 2000, 105(D11), 14347–14362 CrossRef CAS.
- R. L. Valentine and R. G. Zepp, Formation of carbon monoxide from the photodegradation of terrestrial dissolved organic carbon in natural waters, Environ. Sci. Technol., 1993, 27(2), 409–412 CrossRef CAS.
- W. L. Miller and R. G. Zepp, Photochemical production of dissolved inorganic carbon from terrestrial organic matter: Significance to the oceanic organic carbon cycle, Geophys. Res. Lett., 1995, 22(4), 417–420 CrossRef CAS.
- H. J. Hellebrand and W.-D. Kalk, Emission of carbon monoxide during composting of dung and green waste, Nutr. Cycling Agroecosyst., 2001, 60(1), 79–82 CrossRef CAS.
- N. Cowan, C. Helfter, B. Langford, M. Coyle, P. Levy and J. Moxley,
et al., Seasonal fluxes of carbon monoxide from an intensively grazed grassland in Scotland, Atmos. Environ., 2018, 194, 170–178 CrossRef CAS PubMed.
- T. A. J. Kuhlbusch, R. G. Zepp, W. L. Miller and R. A. Burke, Carbon monoxide fluxes of different soil layers in upland Canadian boreal forests, Tellus B, 1998, 50(4), 353–365 CrossRef.
- K. W. Kisselle, R. G. Zepp, R. A. Burke, A. de Siqueira Pinto, M. M. C. Bustamante and S. Opsahl,
et al., Seasonal soil fluxes of carbon monoxide in burned and unburned Brazilian savannas, J. Geophys. Res.: Atmos., 2002, 107(D20), LBA 18 CrossRef.
- R. Varella, M. Bustamante, A. Pinto, K. Kisselle, R. Santos and R. Burke,
et al., Soil fluxes of CO2, CO, NO, and N2O from an old pasture and from native savanna in Brazil, Ecol. Appl., 2004, 14(sp4), 221–231 CrossRef.
-
A. Laasonen, Biogenic carbon monoxide fluxes in four terrestrial ecosystems, Master's thesis, University of Helsinki, 2021.
- M. Pihlatie, Ü. Rannik, S. Haapanala, O. Peltola, N. Shurpali and P. J. Martikainen,
et al., Seasonal and diurnal variation in CO fluxes from an agricultural bioenergy crop, Biogeosciences, 2016, 13(19), 5471–5485 CrossRef CAS.
- D. Bruhn, K. R. Albert, T. N. Mikkelsen and P. Ambus, UV-induced carbon monoxide emission from living vegetation, Biogeosciences, 2013, 10(12), 7877–7882 CrossRef.
- G. M. King and H. Crosby, Impacts of plant roots on soil CO cycling and soil–atmosphere CO exchange, Global Change Biol., 2002, 8(11), 1085–1093 CrossRef.
- H. Lee, T. Rahn and H. Throop, An accounting of C-based trace gas release during abiotic plant litter degradation, Global Change Biol., 2012, 18(3), 1185–1195 CrossRef.
- J. Moxley and K. Smith, Factors affecting utilisation of atmospheric CO by soils, Soil Biol. Biochem., 1998, 30(1), 65–79 CrossRef CAS.
- J. Moxley and J. Cape, Depletion of carbon monoxide from the nocturnal boundary layer, Atmos. Environ., 1997, 31(8), 1147–1155 CrossRef CAS.
- E. Sanhueza, L. Donoso, D. Scharffe and P. J. Crutzen, Carbon monoxide fluxes from natural, managed, or cultivated savannah grasslands, J. Geophys. Res.: Atmos., 1994, 99(D8), 16421–16427 CrossRef CAS.
- R. M. Murphy, M. Saunders, K. G. Richards, D. J. Krol, A. W. Gebremichael and J. Rambaud,
et al., Nitrous oxide emission factors from an intensively grazed temperate grassland: A comparison of cumulative emissions determined by eddy covariance and static chamber methods, Agric., Ecosyst. Environ., 2022, 324, 107725 CrossRef CAS.
- R. M. Murphy, K. G. Richards, D. J. Krol, A. W. Gebremichael, L. Lopez-Sangil and J. Rambaud,
et al., Assessing nitrous oxide emissions in time and space with minimal uncertainty using static chambers and eddy covariance from a temperate grassland, Agric. For. Meteorol., 2022, 313, 108743 CrossRef.
- D. Vickers and L. Mahrt, Quality control and flux sampling problems for tower and aircraft data, J. Atmos. Ocean. Technol., 1997, 14(3), 512–526 CrossRef.
-
J. C. Kaimal and J. J. Finnigan, Atmospheric boundary layer flows: their structure and measurement, Oxford University Press, 1994 Search PubMed.
- G. Fratini, A. Ibrom, N. Arriga, G. Burba and D. Papale, Relative humidity effects on water vapour fluxes measured with closed-path eddy-covariance systems with short sampling lines, Agric. For. Meteorol., 2012, 165, 53–63 CrossRef.
-
J. Moncrieff, R. Clement, J. Finnigan and T. Meyers, Averaging, detrending, and filtering of eddy covariance time series, Handbook of Micrometeorology, Springer, 2004, pp. 7–31 Search PubMed.
- R. Kormann and F.
X. Meixner, An analytical footprint model for non-neutral stratification, Boundary-Layer Meteorol., 2001, 99(2), 207–224 CrossRef.
-
T. Foken, Mikrometeorologische Methoden, 2003 Search PubMed.
- P. L. Finkelstein and P. F. Sims, Sampling error in eddy correlation flux measurements, J. Geophys. Res.: Atmos., 2001, 106(D4), 3503–3509 CrossRef CAS.
- D. M. Linn and J. W. Doran, Effect of water-filled pore space on carbon dioxide and nitrous oxide production in tilled and nontilled soils, Soil Sci. Soc. Am. J., 1984, 48(6), 1267–1272 CrossRef CAS.
-
EPA, Black carbon research and future strategies, Reducing emissions, improving human health and taking action on climate change, 2011 Search PubMed.
- T. K. Spohn, D. Martin, M. Geever and C. O'Dowd, Black carbon measurements from Ireland's Atmospheric Composition and Climate Change (AC3) network, Atmos. Environ., 2022, 271, 118932 CrossRef CAS.
- L. Drinovec, G. Močnik, P. Zotter, A. Prévôt, C. Ruckstuhl, E. Coz, J. Sciare, T. Müller, A. Wiedensohler and A. D. A. Hansen, Atmos. Meas. Tech. Discuss., 2014, 608(7), 10179–10220 Search PubMed.
-
D. Martin and C. O'Dowd, EPA RESEARCH PROGRAMME 2014–2020 Ireland's Atmospheric Composition and Climate Change Network (2013-CCRP-FS.15) EPA Research Report, An Ghníomhaireacht um Chaomhnú Comhshaoil, Environmental Protection Agency, PO Box 3000, Johnstown Castle, Co. Wexford, Ireland, 2020 Search PubMed.
- L. S. Nelson, The Anderson-Darling test for normality, J. Qual. Technol., 1998, 30(3), 298–299 CrossRef.
- C. D. Dorich, D. De Rosa, L. Barton, P. Grace, D. Rowlings and M. D. A. Migliorati,
et al., Global Research Alliance N2O chamber methodology guidelines: Guidelines for gap-filling missing measurements, J. Environ. Qual., 2020, 49(5), 1186–1202 CrossRef CAS PubMed.
- S. Wood and N. B. Pya, Smoothing parameter and model selection for general smooth models, J. Am. Stat. Assoc., 2016, 111, 1548–1575 CrossRef CAS.
-
Y. Zhao, Carbon Monoxide Emissions from Corn Silage, 2021 Search PubMed.
- G. M. King, Land use impacts on atmospheric carbon monoxide consumption by soils, Global Biogeochem. Cycles, 2000, 14(4), 1161–1172 CrossRef CAS.
- N. Cowan, J. Maire, D. Krol, J. M. Cloy, P. Hargreaves and R. Murphy,
et al., Agricultural soils: A sink or source of methane across the British Isles?, Eur. J. Soil Sci., 2021, 72(4), 1842–1862 CrossRef CAS.
|
This journal is © The Royal Society of Chemistry 2023 |
Click here to see how this site uses Cookies. View our privacy policy here.