DOI:
10.1039/D3FO01356A
(Paper)
Food Funct., 2023,
14, 9591-9605
Associations of dietary factors and xenobiotic intake with faecal microbiota composition according to the presence of intestinal mucosa damage†
Received
5th April 2023
, Accepted 30th August 2023
First published on 31st August 2023
Abstract
Diet is a major modulator of gut microbiota, which plays a key role in the health status, including colorectal cancer (CRC) development. Several studies and meta-analyses have evidenced an association of certain dietary factors and xenobiotic intake with the incidence of CRC. Nevertheless, how these dietary factors impact the first stages of intestinal mucosa damage is still uncertain. This study aimed at exploring the associations of relevant dietary factors with the gut microbiota of control individuals and subjects diagnosed with intestinal polyps. A total of 60 volunteers were recruited, clinically classified according to colonoscopy criteria and interviewed using food frequency questionnaires (FFQs). The nutritional status of each volunteer was determined and the intake of dietary xenobiotics was quantified. The relative abundance of faecal microbiota taxonomic groups was obtained through 16S rRNA gene sequencing. The association of dietary factors and xenobiotics with faecal microbiota composition showed differences according to the clinical diagnosis group. Our results showed that the intake of red meat (≥50 g day−1) and total polycyclic aromatic hydrocarbons (PAHs) (≥0.75 μg day−1) was associated with a decreased abundance of the family Bacteroidaceae and an increased abundance of Coriobacteriaceae in control subjects. The intake of the heterocyclic amines 2-amino-1-methyl-6-phenylimidazo(4,5-b)pyridine (PhIP) (≥40 ng day−1) and 2-amino-3,8 dimethylimidazo(4,5,f) quinoxaline (MeIQx) (≥50 ng day−1) was associated with a decreased abundance of Akkermansiaceae in the control diagnosis group. Moreover, N-nitroso compounds (NOCs), nitrites (≥1.69 mg day−1) and N-nitrosodimethylamine (NDMA) (≥0.126 μg day−1) were associated with a decreased abundance of Bifidobacteriaceae. The intake of ethanol (≥12 g day−1) in the polyps group was associated with an increased abundance of Peptostreptococcaceae and a decreased abundance of Veillonellaceae. Moreover, linear regression analyses allowed us to identify ethanol, calcium, bioactive compounds such as flavonoids, stilbenes, cellulose, phenolic acids or total polyphenols, and dietary xenobiotics such as PhIP and MeIQx, the NOC N-nitrosopyrrolidine (NPYR) or the total PAHs as potential predictors of faecal microbiota group abundances. These results indicated that the consumption of milk, red meat, processed meat and ethanol and the intake of polyphenols, dietary PAHs, HAs and NOCs are associated with specific groups of the intestinal microbiota, depending on the clinical diagnosis group.
1. Introduction
Colorectal cancer (CRC) has been estimated to be responsible for more than 1.9 million new cancer cases and 935
000 deaths worldwide.1 Despite the efforts made through screening programs, the incidence of CRC is still rising.2 Besides genetic factors, environmental factors, with a special focus on diet, are proposed to account for at least 70% of CRC cases.3,4 Several studies, including the Global Burden of Diseases, Injuries, and Risk Factors Study (GBD), pointed to certain risk factors for CRC, such as the consumption of red meat, processed meat and ethanol, and also to protective ones such as the consumption of milk, fibre, polyphenols and calcium.5–8 These findings were in accordance with previous data from the International Agency for Research on Cancer (IARC), the World Cancer Research Fund (WCRF) and the American Institute for Cancer Research (AICR).9,10 Therefore, diet as a whole becomes a key factor for CRC development. Apart from the traditional components, diet can be a source of ethanol and xenobiotic compounds such as heterocyclic amines (HAs), polycyclic aromatic hydrocarbons (PAHs), acrylamide and N-nitroso compounds (NOCs) largely formed during the processing and cooking of fish, meat and other foods at high temperature.11 The final toxicity of these compounds results from their absorption and metabolic transformation, in which intestinal microbiota is involved. For example, the intestinal microbiota can modulate the absorption of xenobiotic compounds by altering the intestinal permeability or modifying the thickness of the intestinal mucus layer.12 Moreover, gut microbes are also capable of transforming compounds, resulting in others with increased or decreased toxicity depending on the intestinal microbiota profile of the host.13 Direct binding of microorganisms to toxic compounds and the excretion of the latter in faeces are also possible and may contribute to the decrease in the damage in the host.14 Finally, conjugated molecules, ready to be excreted after their transformation by host phase II enzymes, can be reactivated by the gut microbiota as occurs with hydrolytic reactions carried out by β-glucuronidases.15,16 Nevertheless, this relationship is not unidirectional, as the gut microbiota can also be altered by the intake of dietary xenobiotics. In humans, a shift from healthy diets to poor quality ones, such as the so-called “western” pattern diet, promotes a decrease in the consumption of bioactive compounds, tends to enrich the intake of potentially carcinogenic compounds and may also modify the composition of the gut microbiota.8,17–19 In this sense, faecal samples represent a useful material to study changes occurring in the gut microbiota along disease development. The enrichment of species such as Fusobacterium nucleatum, Bacteroides fragilis, Enterococcus faecalis, Escherichia coli or Streptococcus bovis has been reported in faecal samples from individuals diagnosed with CRC. These microorganisms are associated with the promotion of tumorigenesis.20Bacteroides, Prevotella, Porphyromonas, Enterococcus or Streptococcus genera have also been found to be elevated in faecal samples from individuals diagnosed with CRC.21–24 Precancerous states also exhibited alterations in the gut microbiota. Thus, when the samples of patients with adenoma polyps were compared with healthy individuals, a decrease in families Ruminococcaceae, Clostridiaceae, and Lachnospiraceae was found, while the classes Bacilli and Gammaproteobacteria (order Enterobacterales) were increased.25 The hypothesis proposed in this work is that dietary factors can modulate the intestinal microbiota composition in different ways depending on the stage of mucosal damage in CRC development. To test it, major dietary components previously reported to be associated with CRC development were evaluated to assess their impact on the composition and activity of the faecal microbiota according to the clinical presence of mucosal damage in the form of intestinal polyps.
2. Materials and methods
2.1 Study design and volunteers
Data used in this study have been obtained in the context of the project “Effect of diet and exposure to xenobiotics generated during food processing on the genotoxic/cytotoxic capacity of the intestinal microbiota” (MIXED).
Physicians of the Digestive Service from the Central University Hospital of Asturias (HUCA) and the Carmen and Severo Ochoa Hospital, both located in Asturias, the northern region of Spain, have performed the recruitment of the sample population. Volunteers were randomly selected from those subjects who participated in a screening program of CRC and who fulfilled the inclusion criteria: age between 40 and 75 years, absence of cancer history, digestive pathology, immune-related diseases or drug treatment. Volunteers were recruited at the time of the first interview and scheduled for colonoscopy. Prior to enrolling, all the participants were informed about the objectives and procedures of the study. Once they agreed to participate, an informed consent form was signed. Human samples of faeces and anamnesis, and analytical data were also collected at the time of recruitment, as described elsewhere.26 A total of 60 volunteers for which dietary information and faecal samples were available were included in the study. According to the screening colonoscopy results, volunteers were classified as controls not showing any alteration of the intestinal mucosa (n = 25), or polyps (n = 35) in the case of those volunteers showing the presence of one or more intestinal polyps in the colonic mucosa. This project was evaluated and approved by the Regional Ethics Committee of Clinical Research of Asturias (Ref. 163/19) and by the Committee on Bioethics of CSIC (Ref. 174/2020). The procedures stated in the Declaration of Helsinki, the Oviedo Bioethics Convention, the Council of Europe Convention on Human Rights and Biomedicine, as well as in the Spanish legislation on bioethics were strictly followed.
2.2. Nutritional assessment and anthropometry
Dietary information was obtained from a validated semi-quantitative food-frequency questionnaire (FFQ) conducted by trained interviewers.26,27 This survey was performed at the time of medical consultation for the results of colonoscopy. The FFQ was composed of 155 food items, and portion sizes were obtained using the Pilot Study for Assessment of Nutrient Intake and Food Consumption Among Kids in Europe (PANCAKE).28 Moreover, the FFQ included specific questions regarding culinary preparations, type of food (i.e. chicken breast or thigh), consumption habits (i.e. thigh cooked and consumed with or without skin), cooking methods (i.e. boiled, fried, etc.) and degree of doneness (rare, medium, well done, very well done). The last question was accompanied by standardized pictures to illustrate the degree of cooking for meats, fried potatoes and bread. The Higher Education in Nutrition and Dietetics (CESNID) criteria was followed for the classification of food into groups.29 The nutritional information was obtained from the food composition tables of CESNID and the United States Department of Agriculture (USDA).29,30 Energy and macronutrient intake data were completed with information about phenolic compounds and dietary fibre consumption, extracted from Phenol Explorer 3.6 and the studies by Marlett and Cheung.31,32 Oxygen Radical Activity Capacity (ORAC) was calculated from the article by Wu et al.33 After fulfilling the FFQ, height (m) and weight (kg) of each volunteer were determined and the BMI was calculated using the formula weight (kg)/height (m)2. The criteria of the Spanish Society for the Study of Obesity (SEEDO) were applied to classify the sample into normal weight (18.5–24.9 kg m−2), overweight (25.0–29.9 kg m−2), and obese (≥30.0 kg m−2). Dietary data generated in this study are available from authors under reasonable request.
2.3. Xenobiotic intake estimation
The content of HAs, PAHs, NOCs and acrylamide xenobiotics were obtained and compiled from the European Prospective Investigation into Cancer and Nutrition (EPIC) carcinogen database.34 This information was completed with data from the Computerized Heterocyclic Amines Resource for Research in Epidemiology of Disease (CHARRED), the European Food Safety Authority (EFSA), the U.S. Food Drug Administration (FDA) along with other references.35–46 The content of these compounds was estimated by considering the preservation method, type of cooking, degree of browning and temperature.
2.4. Dietary factors
These xenobiotics together with certain dietary variables showing protective or hazardous roles in previous studies and meta-analyses with defined intake cut-off values were included in the analyses. From the GBD,5 the selected variables and their cut-off values were as follows: milk ≥120 g day−1, red meat ≥50 g day−1, processed meat ≥25 g day−1, ethanol ≥12 g day−1, fibre ≥20 g day−1 and calcium ≥900 mg day−1. From the Southern Community Cohort Study:47 total polyphenols ≥650 mg day−1. From the EPIC:48N-nitrosodimethylamine (NDMA) ≥0.125 μg day−1. From the study conducted by Martínez Góngora et al.:49 amino-1-methyl-6-phenylimidazo(4,5-b)pyridine (PhIP) ≥40 ng day−1 and 2-amino-3,8 dimethylimidazo (4,5,f) quinoxaline (MeIQx) ≥50 ng day−1. Moreover, those dietary variables showing significant associations (p < 0.05) or closeness to significance (p < 0.10) with shifts in the risk of being in the group of volunteers diagnosed with polyps in a previous study of our research team conducted with the same sample of volunteers were also included:26 soluble pectin ≥0.57 g day−1, flavonoids ≥82.18 mg day−1, other polyphenols ≥32.15 mg day−1, nitrites ≥1.69 mg day−1, dibenzo (a) anthracene (DiB(a)A) ≥0.07 μg day−1 and total PAH ≥0.75 μg day−1.
2.5. Faecal samples’ processing
A total of 4 g of frozen faecal samples were weighed, diluted 1/10 and homogenized with sterile PBS in a LabBlender 400 Stomacher (Seward Medical, London, UK) for 3 min at the maximum speed. After 15 min centrifugation at 4° C and 14
000 rpm, supernatants and pellets were separated and kept frozen at −20 °C until use.
2.6. Faecal short chain fatty acids
The concentration of the major short chain fatty acids (SCFAs) such as acetic, propionic, butyric as well as isobutyric, isovaleric, valeric and caproic acids in the faeces was determined through the procedure previously described, with some minor modifications.50 Faecal supernatants were diluted with methanol and 20% v/v formic acid; an internal standard reaching a dilution of 10/65 was added to the sample. Then, preparations were centrifuged for 10 min at room temperature and 14
000 rpm and the obtained supernatants were transferred to suitable chromatography vials. A chromatograph 6890N (Agilent Technologies Inc., Palo Alto, CA, USA) connected to a mass spectrometry detector (MS) 5973N (Agilent Technologies) and a flame ionization detector (FID) was used for the identification and quantification of SCFAs. Theoretical detection limit values were calculated for less abundant SCFAs and applied to those samples under the limit of detection.
2.7. Faecal amino acids and biogenic amines
Amino acid and biogenic amine levels in faeces were determined in faecal supernatants using the method described by Redruello et al. and adapted to the faecal supernatants analyzed by Salazar et al.51,52 Briefly, the faecal supernatants were filtered through 3 kDa centrifugal filters (Amicon Ultra-0.5, Merck KGaA, Germany) and derivatized using diethyl ethoxymethylenemalonate (DEEMM, Sigma-Aldrich, USA). Before injection, the samples were also filtered using 0.22 μm-pore diameter polytetrafluoroethylene membranes (VWR International, USA). An H-Class Acquity UPLC™ system (Waters, Milford, MA, USA) coupled to a photodiode array detector at 280 nm was used to quantify amino acids and biogenic amines. The detection limit values were applied when necessary.
2.8. DNA extraction and metataxonomic analyses
The pellets obtained after the dilution and homogenization of faeces were used to extract the DNA. Q protocol for DNA extraction defined by the International Human Microbiome Standards Consortium was applied using a QIAamp Fast DNA Stool Mini Kit (Qiagen, Sussex, UK).53 After extraction, the 260/280 ratio was quantified using a Take3 Micro-Volume plate and a Gen5 microplate reader (BioTek Instruments, Inc., Winooski, VT, USA). DNA was kept frozen at −20 °C until analysis. The whole procedure of bacterial 16S rRNA gene sequencing and annotation was conducted at Novogene Bioinformatics Technology Co., Ltd. First, the variable region V3–V4 of bacterial 16S rRNA genes was amplified by PCR using specific primers (341F and 806R) connected with barcodes and the DNA library was prepared. Libraries were sequenced on an Illumina NovaSeq 6000 platform instrument. The obtained individual reads were assigned to samples using barcodes and merged using FLASH (V 1.2.7).54 High quality clean tags were obtained by filtration using QIIME (V 1.7.0), allowing for the removal of low-quality sequences.55,56 The tags were then compared with the reference SILVA 138 database and chimera sequences were removed using the UCHIME algorithm.57,58 Effective tags were utilized by Uparse software (Uparse V 7.0.1090) to perform the sequencing analysis. Sequences sharing ≥97% homology were assigned to the same OTUs, and the OTU abundance was normalized. To annotate species at each taxonomic rank, the representative sequence for each OTU was determined against the SSUrRNA database of the SILVA138 database using QIIME (V 1.7.0) in the Mothur method.59 Moreover, to obtain the phylogenetic relationship of all OTU representative sequences, MUSCLE (V 3.8.3) was used.60 The ACE index of alpha diversity was obtained from QIIME (V 1.7.0). The datasets generated during metataxonomic sequencing of faecal DNA samples in the MIXED project were deposited in the NCBI Sequencing Read Archive PRJNA994445 (https://www.ncbi.nlm.nih.gov/bioproject/994445).
2.9. Statistical analyses
The data obtained were analysed using SPSS software version 25.0 (IBM SPSS, Inc., Chicago, IL, USA), RStudio software version 1.4.3 and Galaxy web tool.61 GraphPad Prism 9 and RStudio software were used for graphical representations. Goodness of fit to the normal distribution was checked by means of the Kolmogorov–Smirnov test. As normality of the metabolite variables (SCFAs, amino acids and biogenic amines) was not achieved, Mann–Whitney U tests were performed to detect group differences. Linear discriminant analysis effect size (LEfSe) analyses were conducted to differentiate at the family taxonomic level the microorganisms contributing to discriminate between study groups by using the Galaxy web tool.61 These analyses consist of a Kruskal–Wallis sum-rank test and a Wilcoxon test for pairwise comparison, followed by a logarithmic linear discriminant analysis (LDA) to estimate the effect-size at a threshold of 2.0. To determine whether the different diagnosis groups could lead to a statistically significant influence on the relative abundance of microbiological families, a MANOVA analysis was performed. In this multivariate test, clinical diagnosis group levels were selected as the explanatory variables, while the most abundant bacterial families in the sample (relative abundance >1%) were introduced as the dependent variables. Abundance-based coverage estimator (ACE) index differences of alpha diversity were evaluated according to dietary consumption and diagnosis groups by the Mann–Whitney tests. To explore the associations between microbial families and metabolic and food group variables, Spearman correlation analyses were performed. Significant correlations were adjusted for multiple tests using the Benjamini–Hochberg procedure. Heatmaps were generated using the “pheatmap” R package. Redundancy analyses (RDA) of food groups and dietary compounds were performed using the “vegan” R package. To discard collinearity, bioactive and potentially carcinogenic compounds were examined as predictors of gut microbial families, faecal SCFAs and amino acids by regression analyses, adjusting by gender and BMI to reduce their potential confounding role on CRC as previously described.62,63
3. Results
General characteristics and anthropometric parameters of the sample are presented in Table 1 according to the clinical diagnosis groups. No significant differences were found for the variables considered between groups.
Table 1 General characteristics of the study sample according to clinical diagnosis
|
Control (n = 25) |
Polyps (n = 35) |
Values are shown as mean ± standard deviation for continuous variables or number and percentage (%) for the categorical ones. BMI, body mass index. |
Male gender |
12 (48.00%) |
18 (51.40%) |
Age (years) |
58.08 ± 9.61 |
61.03 ± 6.31 |
Energy intake (kcal day−1) |
2036.90 ± 752.13 |
2117.44 ± 850.13 |
BMI (kg m−2) |
26.22 ± 3.88 |
27.70 ± 3.90 |
3.1. Differential microbiota profiles according to dietary intake
The relative abundance of the faecal microbiota in the samples at the family taxonomic level and metabolites is presented in Table S1† according to the clinical diagnosis groups. The faecal microbial composition of volunteers was then analysed according to dietary factors for each diagnosis group (Fig. 1). Given the high taxonomic complexity of the microbiota, we focused the present study to the family level, in order to not split results and maintain the main aim of the current work. When milk consumption was evaluated, control volunteers with an intake under 120 g day−1 showed lower abundances of Peptostreptococcaceae, Coriobacteriaceae and Erysipelotrichaceae and higher abundance of Bacteroidaceae (Fig. 1A) (Table S2†). A higher abundance of Akkermansiaceae was detected in the polyps group with milk intake above 120 g day−1. A differentially increased abundance of Coriobacteriaceae and decreased abundance of Bacteroidaceae and Akkermansiaceae was observed in control volunteers consuming more than 50 g of red meat per day compared to those whose consumption of this food was lower (Fig. 1B) (Table S3†). On the other hand, the consumption of ≥25 g of processed meat per day was accompanied by a reduced abundance of Bifidobacteriaceae and by an increased abundance of Prevotellaceae and Erysipelatoclostridiaceae in the control group, while these families were also increased in the group diagnosed with polyps consuming high levels of processed meat (Fig. 1C) (Table S4†). When fibre consumption was analysed, increased concentrations of isobutyric and isovaleric acids and Coriobacteriaceae relative abundance were found in the control volunteers consuming ≥20 g fibre per day (Fig. 1D) (Table S5†). Moreover, no differences were found in faecal microbiota abundances when soluble pectin intake was examined (Table S6†). Regarding total polyphenols, increased abundances of Coriobacteriaceae, Veillonellaceae and Erysipelotrichaceae and decreased abundances of Peptostreptococcaceae, Prevotellaceae and Bacteroidaceae were detected in the control group with intake of total polyphenols ≥650 mg day−1 (Fig. 1E) (Table S7†). Among them, the intake of flavonoids (≥82.18 mg day−1) was not associated with any shift in microbial families (Table S8†). Furthermore, the control group with an intake of other polyphenols ≥32.15 mg day−1 showed a decreased abundance of Bifidobacteriaceae and higher concentrations of SCFAs such as acetic, butyric and propionic acids (Fig. 1F) (Table S9†).
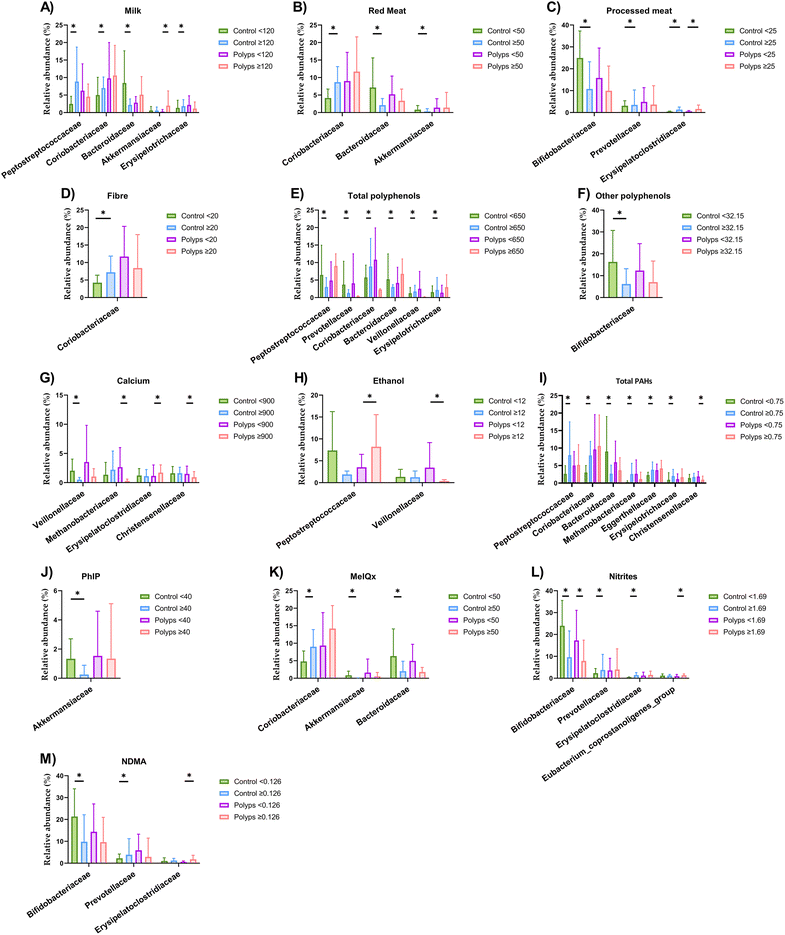 |
| Fig. 1 Differential abundance according to linear effect size discriminant analysis (LEfSe) at the taxonomical family level in the faecal microbiota of volunteers according to the clinical diagnosis group and cut-off intake values for (A) milk (g day−1), (B) red meat (g day−1), (C) processed meat (g day−1), (D) fibre (g day−1), (E) total polyphenols (mg day−1), (F) other polyphenols (mg day−1), (G) calcium (mg day−1), (H) ethanol (g day−1), (I) total PAH (μg day−1), (J) PhIP (ng day−1), (K) MeIQx (ng day−1), (L) nitrites (mg day−1) and (M) NDMA (μg day−1). Only families showing relative abundances higher than 1% in the sample are represented. Total PAHs, total polycyclic aromatic hydrocarbons; PhIP, 2-amino-1-methyl-6-phenylimidazo(4,5-b)pyridine; MeIQx, 2-amino-3,8 dimethylimidazo (4,5,f) quinoxaline; NDMA, N-nitrosodimethylamine. | |
A lower abundance of the family Veillonellaceae was detected in the control group with intake of calcium ≥900 mg day−1 (Fig. 1G) (Table S10†). Furthermore, the polyps group showing higher intake of calcium revealed an increase in the abundance of Erysipelatoclostridiaceae and in the concentrations of acetic and propionic acids, together with a decrease in the abundance of Methanobacteriaceae and Christensenellaceae. Regarding xenobiotics, in those subjects diagnosed with intestinal polyps, higher ethanol intake was concomitantly associated with a reduced abundance of Veillonellaceae and increased abundance of Peptostreptococcaceae (Fig. 1H) (Table S11†). No statistically significant differences were found according to DiB(a)A intake (Table S12†). When the dietary consumption of total PAHs was evaluated, many differences were revealed in microbial faecal abundances and SCFA concentrations (Fig. 1I) (Table S13†). The control volunteers with a total PAH intake of ≥0.75 μg day−1 displayed increased abundances of Peptostreptococcaceae, Coriobacteriaceae, Eggerthellaceae and Erysipelotrichaceae in contrast to the decreased abundance of Bacteroidaceae. The control group also showed higher concentrations of isobutyric and isovaleric acids together with total amino acids and protein amino acids in the faeces. In the polyps group, the total PAH intake of ≥0.75 μg day−1 was associated with a decreased abundance of Christensenellaceae and higher concentrations of acetic acid, propionic acid and biogenic amines. Control volunteers with PhIP intake higher than 40 ng day−1 revealed a decreased abundance of Akkermansiaceae (Fig. 1J) (Table S14†). Also, in the control diagnosis group, a decreased abundance of Bacteroidaceae and increased abundance of Erysipelatoclostridiaceae was detected when the intake of MeIQx was higher than 50 ng day−1 (Fig. 1K) (Table S15†). In both the control and polyps groups, the intake of ≥1.69 mg day−1 of nitrites was associated with a decreased abundance of Bifidobacteriaceae (Fig. 1L) (Table S16†). Moreover, in the control group, higher intake of nitrites was associated with an increased abundance of Prevotellaceae and Erysipelatoclostridiaceae, while in the polyps groups, this increase occurred in the Eubacterium coprostanoligenes group. When the intake of NDMA was evaluated, a reduction in Bifidobacteriaceae abundance accompanied by an increase in Prevotellaceae was observed for the control volunteers with consumption ≥0.126 μg day−1, while in the polyps group, this intake was associated with an increased abundance of Erysipelatoclostridiaceae (Fig. 1M) (Table S17†).
A MANOVA analysis was conducted to determine whether the clinical diagnosis groups and microbial profiles were associated. The value observed for the multivariate significance test was not statistically significant (overall F statistic: 0.932, p-value: 0.551).
3.2. Microbiological richness according to dietary intake
To determine the differences in the alpha diversity according to the dietary intake in each diagnosis group, ACE index values were evaluated (Fig. 2). In the control group, richness decreased significantly with elevated consumption of red meat (≥50 g day−1) and total PAH intake (≥0.75 μg day−1). Moreover, in the subjects diagnosed with polyps, DiB(a)A intake ≥0.07 μg day−1 was associated with a lower ACE index.
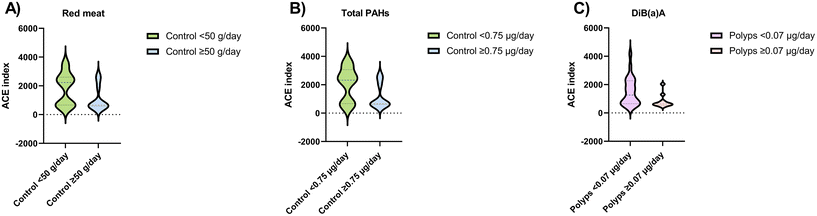 |
| Fig. 2 Statistically significant differences in alpha diversity (ACE index) obtained from the faecal microbiota of volunteers according to clinical diagnosis group and cut-off intake values for (A) red meat (g day−1), (B) total PAH (μg day−1) and (C) DiB(a)A (μg day−1). ACE, abundance-based coverage estimator; total PAHs, total polycyclic aromatic hydrocarbons; DiB(a)A, dibenzo (a) anthracene. | |
3.3. Food groups, faecal metabolites and microbiota correlations
Exploration into the association of food groups with faecal microbiota at the taxonomic family level was conducted through Spearman correlation analyses and was represented by heatmaps (Fig. 3A).
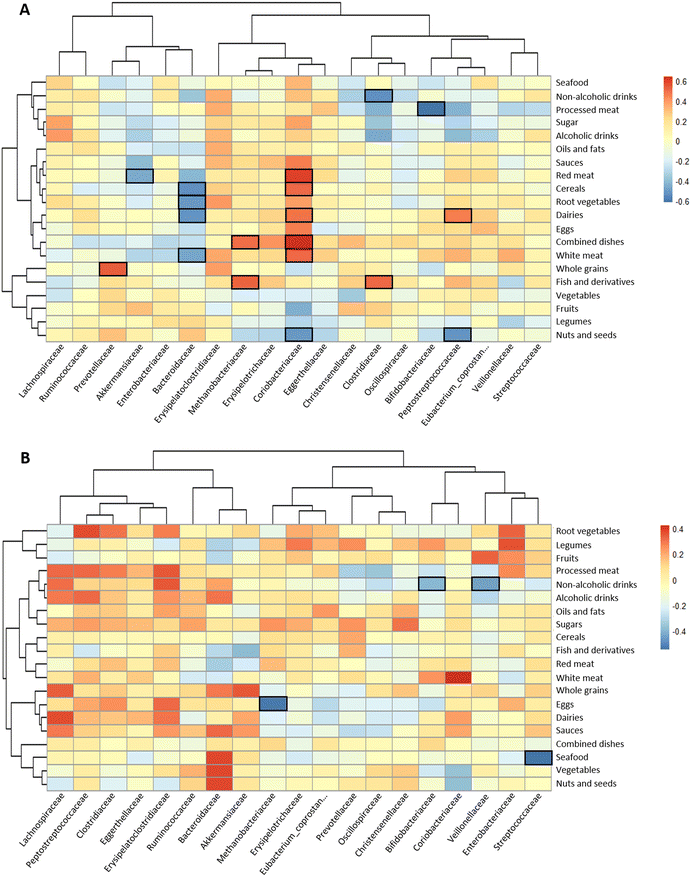 |
| Fig. 3 Heatmaps defined by Spearman correlations between food groups and faecal microbiota at the taxonomic family level for the most abundant bacterial families (relative abundance ≥1%) for clinical diagnosis groups: (A) control or (B) polyps. Blue and red colours denote negative and positive associations, respectively. The intensity of the colour is proportional to the degree of association between variables. Bordered cells indicate a statistically significant association adjusted for multiple testing by the Benjamini–Hochberg procedure. | |
In the controls, the consumption of processed meat was negatively correlated with the relative abundance of Bifidobacteriaceae in the faeces (Fig. 3A), whereas red meat showed an inverse association with Akkermansiaceae and a direct association with Coriobacteriaceae. In contrast, the abundance of this last family was inversely correlated with nuts and seeds. Bacteroidaceae was inversely linked to the consumption of cereals, root vegetables and dairy products. Clostridiaceae family was negatively correlated with non-alcoholic drinks and positively correlated with fish and derivatives. In polyps, only statistically significant inverse relationships were found: sea food with Streptococcaceae, eggs with Methanobacteriaceae, and non-alcoholic drinks with Veillonellaceae and Bifidobacteriacae (Fig. 3B). In contrast, abundant correlations were found for some food groups and faecal metabolites (Fig. 4). In the control group, branched chain amino acids isobutyric and isovaleric directly correlated with white meat and fish and derivatives (Fig. 4A). The former food group also correlated with total amino acids and ammonium. Non-alcoholic drinks were positively associated with most of the variables except for biogenic amines and caproic acid. On the other hand, in the group of volunteers diagnosed with polyps, red meat, dairy products and processed meat were positively correlated with biogenic amines (Fig. 4B). Moreover, red meat and dairy products correlated with acetic acid and propionic or butyric acid, respectively. Three major SCFAs (acetic, propionic and butyric) were associated directly with non-alcoholic drinks and fish and derivatives. The direction of the associations of drinks with ammonium and total amino acids shifted from positive in the controls to negative in the polyps group.
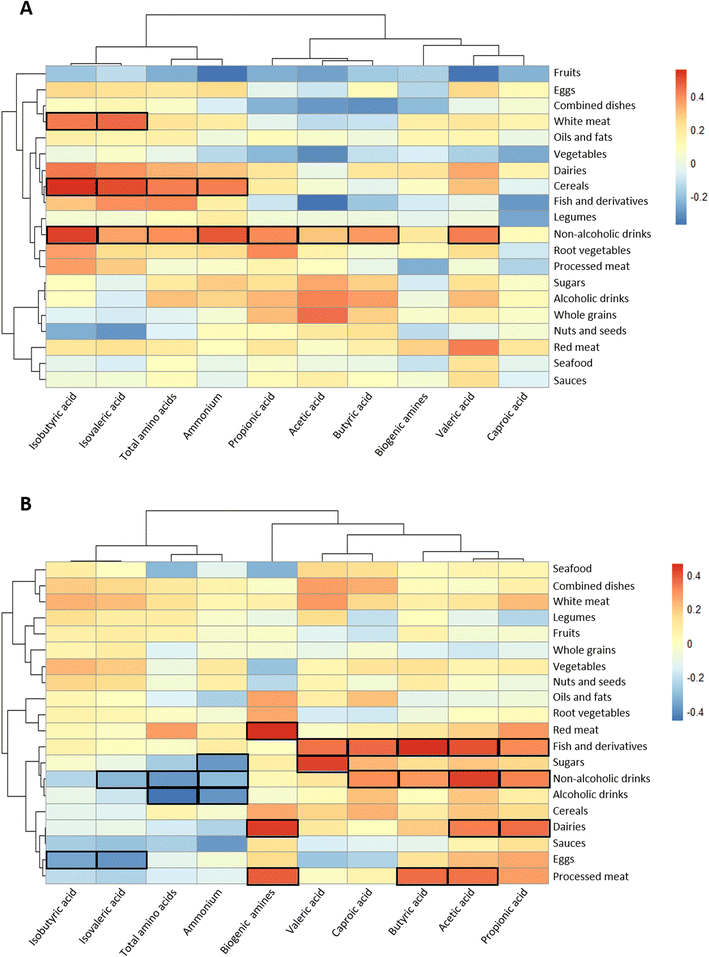 |
| Fig. 4 Heatmaps defined by Spearman correlations between food groups and faecal metabolites (SCFAs) and amino acids for the clinical diagnosis groups: (A) control or (B) polyps. Blue and red colours denote negative and positive associations, respectively. The intensity of the colour is proportional to the degree of association between variables. Bordered cells indicate a statistically significant association adjusted for multiple testing by the Benjamini–Hochberg procedure. | |
3.4. Linear regression analyses between dietary compounds and microbiological families
Food groups and their components were further examined to obtain the underlying basis of the previous findings about the associations between diet and microbiological families. However, RDA models searching for the best explanatory variables did not reveal statistically significant results (data not shown). Therefore, to identify potential dietary predictors of faecal microbiological families, linear regression analyses adjusted by gender and BMI were performed (Table 2). In the control group, NPYR, total PAHs, and total ORAC variables were identified as negative predictors of Bacteroidaceae, Bifidobacteriaceae and Christensenellaceae levels, respectively. Flavonoids were positive predictors of Lachnospiraceae and ethanol was found to be inversely associated with Clostridiaceae. While MeIQ was found as a direct predictor of Clostridiaceae, acrylamide and MeIQx were associated with the relative abundance of Coriobacteriaceae. Acrylamide was the main variable appearing in amino acid regression analysis. Other polyphenols were found to be the best predictors of the major SCFAs (acetic, propionic and butyric). In the polyps group, gender and BMI appeared as significant adjusting parameters when Prevotellaceae and Bacteroidaceae were analysed, respectively. Nitrites were positive predictors of Peptostreptococcaceae, and calcium – of Lachnospiraceae. The xenobiotics NDMA and PhIP directly predicted Erysipelatoclostridiaceae. A bioactive compound, insoluble cellulose, was positively associated with the abundance of Erysipelotrichaceae. Total ORAC was a positive predictor of Ruminococcaceae. Ethanol was inversely related to the total amino acid concentration in the faeces. Regarding SCFAs, acetic acid was significantly adjusted by gender, and nitrites and phenolic acid were found as positive predictors of butyric and caproic acids, respectively. To explore the correlation between the bacterial family Bifidobacteriaceae and SCFAs, Spearman correlations were conducted, and no statistically significant associations were obtained, not even according to the clinical diagnosis groups (Table S18†).
Table 2 Results obtained from linear regression analyses identifying ORAC, bioactive and potential carcinogenic compounds as predictors of faecal microbiota relative abundances, faecal amino acids and SCFAs in each clinical diagnosis group of volunteers
Diagnosis group |
Dependent variable |
Independent variable |
R
2
|
β
|
p
|
Linear regression analyses were adjusted by gender and BMI. Only the variables with p < 0.05 in each model are shown. R2, coefficient of multiple determination; β, standardized regression coefficient; BMI, body mass index; I, insoluble; B(a)P, benzo (a) pyrene; total PAHs, total polycyclic aromatic hydrocarbons; PhIP, 2-amino-1-methyl-6-phenylimidazo(4,5-b)pyridine; MeIQx, 2-amino-3,8 dimethylimidazo (4,5,f) quinoxaline; MeIQ, 2-amino-3,4 dimethylimidazo (4,5,f) quinoline; NDMA, N-nitrosodimethylamine; NPYR, N-nitrosopyrrolidine; ORAC, oxygen radical absorbance capacity. |
Control |
Bifidobacteriaceae |
NPYR |
0.168 |
−0.450 |
0.024 |
Lachnospiraceae |
Flavonoids |
0.244 |
0.525 |
0.007 |
Coriobacteriaceae |
Acrylamide |
0.672 |
0.533 |
0.001 |
MeIQx |
0.672 |
0.462 |
0.002 |
Bacteroidaceae |
Total PAHs |
0.140 |
−0.419 |
0.037 |
Clostridiaceae |
MeIQ |
0.544 |
0.620 |
0.001 |
Ethanol |
0.544 |
−0.317 |
0.037 |
Oscillospiraceae |
MeIQ |
0.291 |
0.566 |
0.003 |
Christensenellaceae |
Total ORAC |
0.142 |
−0.422 |
0.036 |
Eubacterium coprostanoligenes group |
MeIQ |
0.282 |
0.559 |
0.004 |
Total amino acids |
Acrylamide |
0.263 |
0.542 |
0.005 |
Protein amino acids |
Acrylamide |
0.254 |
0.534 |
0.006 |
Ammonium |
Acrylamide |
0.345 |
0.573 |
0.002 |
Total polyphenols |
0.345 |
0.350 |
0.047 |
Acetic acid |
Other polyphenols |
0.371 |
0.511 |
0.005 |
MeIQ |
0.371 |
−0.407 |
0.020 |
Propionic acid |
Other polyphenols |
0.192 |
0.475 |
0.016 |
Butyric acid |
Stilbenes |
0.424 |
0.487 |
0.005 |
Other polyphenols |
0.424 |
0.421 |
0.013 |
Isobutyric acid |
Acrylamide |
0.322 |
0.592 |
0.002 |
Isovaleric acid |
Calcium |
0.212 |
0.495 |
0.012 |
Valeric acid |
Acrylamide |
0.532 |
0.743 |
0.001 |
|
Polyps |
Peptostreptococcaceae |
Nitrites |
0.109 |
0.368 |
0.030 |
Prevotellaceae |
Gender |
0.098 |
0.353 |
0.038 |
Lachnospiraceae |
Calcium |
0.102 |
0.358 |
0.035 |
Bacteroidaceae |
BMI |
0.288 |
0.445 |
0.005 |
Lipophilic ORAC |
0.288 |
−0.313 |
0.039 |
Ruminococcaceae |
Total ORAC |
0.311 |
0.575 |
0.001 |
Erysipelatoclostridiaceae |
NDMA |
0.363 |
0.466 |
0.002 |
PhIP |
0.363 |
0.358 |
0.015 |
Erysipelotrichaceae |
Cellulose (I) |
0.114 |
0.374 |
0.027 |
Oscillospiraceae |
Total PAHs |
0.122 |
−0.384 |
0.023 |
Total amino acids |
Ethanol |
0.097 |
−0.351 |
0.038 |
Protein amino acids |
Ethanol |
0.097 |
−0.351 |
0.039 |
Ammonium |
Ethanol |
0.098 |
−0.353 |
0.037 |
Acetic acid |
Gender |
0.135 |
−0.400 |
0.017 |
Butyric acid |
Nitrites |
0.178 |
0.450 |
0.007 |
Caproic acid |
Phenolic acids |
0.163 |
0.433 |
0.009 |
4. Discussion
Assessing the relationship between diet and intestinal mucosal health as related with faecal microbiota composition is a complex issue. This goal could be addressed by individual analysis of key dietary factors, as those analysed in this work. The evaluation of each dietary factor revealed significant shifts in gut microbial families with differences according to the clinical diagnosis group.
The findings of a lower abundance of Bifidobacteriaceae in the group of individuals with a higher intake of processed meat, nitrites, NDMA and other polyphenols are in line with the literature describing the association of plant-based diets with higher faecal excretion of SCFAs and increased Bifidobacterium abundance, in contrast to meat-based diets.64 However, any statistically significant correlation between Bifidobacteriaceae and the SCFA concentration in faeces was found in the sample. The differences in the diet–microbiota binomial between the different study groups are probably one of the most interesting findings of the present work. In this sense, while in the polyps group, the abundance of Peptostreptococcaceae increased with an intake of ethanol ≥12 g day−1 and was positively predicted by nitrites, in the control group, Clostridiaceae abundance was reduced, when the intake of ethanol was above 12 g day−1. In previous studies, Peptostreptococcaceae has been found to be increased in alcohol consumers in comparison with non-drinkers, and in the faecal samples of CRC patients.65,66 It is important to remark that in our sample the mean ethanol intake was higher in the polyps group than in the control group (15.8 g day−1vs. 6.8 g day−1), although this difference was not significant. These findings could help to support the possible relationship between alcohol intake and the increased risk of intestinal polyps development.26 In the control subjects, volunteers with intake of total polyphenols ≥650 mg day−1 and or calcium intake <900 mg day−1 revealed an increased abundance of Veillonellaceae. A decreased abundance of the genus Veillonella, a member of the family Veillonellaceae, has been previously associated with gut mucosal damage in humans.67 We also detected lower levels of Christensenellaceae in the faecal microbiota of individuals belonging to the polyps group, which showed a total PAH intake ≥0.75 μg day−1. The main route of exposure to PAHs for the human body is food and smoking.68 In this regard, the presence of PAHs in food and their consequent direct accession by gut microbiota members could cause reduction of the abundance of certain bacteria such as Christensenellaceae, whose presence has been related with a positive impact on human health.69 We found that a higher intake of calcium in the polyps group was associated with a reduced abundance of Methanobacteriaceae and Christensenellaceae families and also higher concentrations of acetic acid and propionic acid. The metabolism of calcium has been previously bidirectionally associated with shifts in the gut microbiota, with SCFAs playing key roles in these processes.70,71 In this sense, SCFAs are capable of lowering pH in the intestinal environment, reducing calcium–phosphorus complexes and improving dietary calcium release and absorption.72,73 An increased abundance of Coriobacteriaceae was detected in the control individuals with an intake of ≥120 g day−1 milk, ≥50 g day−1 red meat, ≥20 g day−1 fibre, ≥650 mg day−1 total polyphenols, ≥0.75 μg day−1 total PAH or ≥50 ng day−1 MeIQx. Indeed, in our sample, Coriobacteriaceae was negatively associated with nuts and seeds and positively associated with dairy products, cereals, and red and white meat in the controls. The HA MeIQx, which can be generated during meat cooking, was revealed as a direct predictor of Coriobacteriaceae abundance in the faeces from control volunteers. Members of the Coriobacteriaceae family have been found to be increased in individuals with some pro-inflammatory diseases and obesity, and in individuals with low fibre consumption.74 Interestingly, some species belonging to the family Coriobacteriaceae could be involved in polyphenol metabolism, which could partly contribute to explain our results. However, the potential role of Coriobacteriaceae in human health is still poorly understood.75 This study evidenced that the abundance of Bacteroidaceae in faeces was negatively correlated with the intake of white meat, dairy products, root vegetables and cereals in the control group. In our sample, the main root vegetables consumed were potatoes, which can be prepared using various cooking methods, such as frying, with different impacts on the data obtained. Previous studies have detected a higher proportion of Bacteroidaceae in individuals consuming enriched plant diets.76 Moreover, Bacteroidaceae was negatively predicted by total PAHs in the controls and lipophilic ORAC in the polyps group. When Akkermansiaceae was evaluated, the control group volunteers with an intake ≥50 g day−1 red meat, ≥40 ng day−1 PhIP and ≥50 ng day−1 MeIQx showed a decreased abundance of this family. There is reportedly evidence of the regulatory and beneficial effects in studies about Akkermansia muciniphila, a mucin-degrading microorganism.77,78 Furthermore, in the polyps group, an increase in the faecal abundance of Erysipelatoclostridiaceae was detected when the consumption of processed meat, calcium, nitrites and NDMA was over the cut-off points. In this diagnosis group, this family was also positively predicted by NDMA and PhIP. There is scarce bibliography about the possible interaction between the family Erysipelatoclostridiaceae and diet, as this group was usually combined at the taxonomic level with the Erysipelotrichaceae family, in the order Erysipelotrichales, which makes it difficult to extract confident hypotheses and conclusions. The Erysipelatoclostridiaceae family has been linked with class I obesity (BMI between 30 and 34.9), but no previous associations with intestinal damage have been described.79 In our study, we detected an increased abundance of members from the Eubacterium coprostanoligenes group in individuals from the polyps group when the intake of nitrites was ≥1.60 mg day−1. This family has been identified in the mucosal samples of adenomatous polyps patients, hypothesizing a harmful role.80 In our sample, the mean consumption of processed meat, a dietary source of nitrites, was higher in the polyps group compared to the control group (78 g day−1vs. 61 g day−1). These values were high as compared with the reported global mean intake of 17 g of processed meat per day reported in a survey across 185 countries around the world.81 Also, the GBD study established that the intake of processed meat over 25 g day−1 was associated with an increased relative risk of being diagnosed with CRC in 1.06 (1.02–1.10), a threshold that is clearly surpassed in our sample.5 The consumption of vegetables was lower in the polyps group than in the control group (256 g day−1vs. 279 g day−1), and again, higher in our sample than the estimated global mean intake (208.8 g day−1).82 This could suggest that although the consumption of food groups with harmful potential remains elevated in our sample, their impact on health may be reduced through higher intake of bioactive compounds naturally present in vegetables. These distinct dietary features may also contribute to explain the differences found in the microbiota profiles. Whether all these shifts on the microbiota are causes or consequences or are concomitant to the gut mucosal damage is still uncertain and remains to be determined.
In the present work, we assessed the relationships of bioactive and xenobiotic compounds together with microbiological families, by performing linear regressions. Proceeding in this way, we observed that in the control group, the variable “other polyphenols”, which includes compounds such as curcuminoids, hydroxybenzaldehydes, phenolic terpenes or tyrosols, predicted faecal concentrations of the major SCFAs (acetic, propionic and butyric), whereas total polyphenols predicted the concentration of ammonium in the faeces. In this direction, recent studies have suggested the role of some polyphenols as prebiotic substrates, contributing to explain the positive relationship between some types of polyphenols and faecal levels of SCFAs.83 On the other hand, we hypothesize that the higher faecal levels of ammonium predicted by the intake of total polyphenols in the controls could be mediated by red meat consumption through the metabolism of members from the family Coriobacteriaceae, among others. Other possible hypotheses for the relationship between the intake of phenolic compounds and ammonium is that some phenolic compounds (i.e. tannins) are capable of trapping amino acid metabolites such as ammonia.84 Therefore, increased ammonium concentrations in faeces could be linked to phenolic molecules. Although the directionality of the results could not be established due to the observational nature of our study, this work links the consumption of some groups of foods and xenobiotics with specific microorganisms of the intestinal microbiota. Moreover, along the study, opposite tendencies have been observed in the abundances of certain microorganisms according to the diagnosis group. Although not reaching statistical significance in some cases, these results support the hypothesis that the impact of the diet could be different depending on the diagnosis group. In this sense, the observation of a higher impact on the microbiota of the control group than that of the polyps groups for certain dietary components could be related to the intestinal anatomopathological homogeneity in the control group with regard to higher heterogeneity in the polyps group. Despite the absence of a significant association of clinical diagnosis groups and the relative abundance of microbial families obtained by MANOVA tests, our results suggest that once mucosal damage has been established, the microbiota of volunteers showing intestinal polyps may be less susceptible to variations as compared to that of the controls.
Despite our findings, the present study also showed some limitations. The relative abundances of Methanobacteriaceae obtained in this study should be considered with caution as the primers used for amplification were more optimized for bacteria than for archaea. Moreover, the metataxonomic analyses by 16S RNA gene sequencing did not provide precise information about the microbial species present in the samples. Furthermore, as foods are consumed together as a part of a diet, it is possible that we are losing the effect of interactions among dietary components.
5. Conclusions
The gut microbiota composition in different intestinal mucosa damage statuses has been associated with the consumption of some foods and dietary components. Further studies elucidating the mechanisms by which these compounds are involved in the alteration of the intestinal microbiota could be helpful to develop dietary recommendations in order to reduce the intake of dietary xenobiotics for preventing intestinal mucosal damage and further development of CRC.
Author contributions
Conceptualization: SG and CGRG. Medical resources: AS, YD, and CGR. Investigation: SRS, NS, SA, AZ, MG, CGRG and SG. Methodology: SRS and AZ. Formal analysis: SRS and SG. Funding acquisition: CGRG and SG. Writing – original draft: SRS. Writing, review and editing: SRS, SG and CGRG. All authors contributed to manuscript revision, and read and approved the submitted version.
Conflicts of interest
There are no conflicts of interest to declare.
Acknowledgements
This work received support from the Project RTI2018-098288-B-I00 (acronym MIXED) financed by MCIN/AEI/10.13039/501100011033/FEDER, “Una manera de hacer Europa”, and by the Project AYUD/2021/50981 financed with regional funds from the Plan Regional de Investigación, Principality of Asturias. SRS is a recipient of a predoctoral contract Severo Ochoa (2021-BP20-012) granted by Plan Regional de Investigación, Principality of Asturias. AZ is a recipient of a predoctoral contract from the Health Research Institute of Asturias (ISPA). SA is a recipient of an ISPA Junior Postdoctoral Researcher Contract (ITM-Junior-22). NS received the Grant RYC2021-033521-I funded by MCIN/AEI/10.13039/501100011033 and by European Union NextGenerationEU/PRTR. We deeply acknowledge all volunteers who have participated in this study. We also thank the excellent technical assistance and support from Scientific and Technical Services at IPLA-CSIC.
References
- H. Sung, J. Ferlay, R. L. Siegel, M. Laversanne, I. Soerjomataram, A. Jemal and F. Bray, Global cancer statistics 2020: GLOBOCAN Estimates of incidence and mortality worldwide for 36 cancers in 185 countries, CA Cancer J. Clin., 2021, 71, 209–249 CrossRef PubMed.
- E. Morgan, M. Arnold, A. Gini, V. Lorenzoni, C. J. Cabasag, M. Laversanne, J. Vignat, J. Ferlay, N. Murphy and F. Bray, Global burden of colorectal cancer in 2020 and 2040: Incidence and mortality estimates from GLOBOCAN, Gut, 2023, 72, 338–344 CrossRef PubMed.
- M. Pericleous, D. Mandair and M. E. Caplin, Diet and supplements and their impact on colorectal cancer, J. Gastrointest. Oncol., 2013, 4, 409–423 CAS.
- J. Yang and J. Yu, The association of diet, gut microbiota and colorectal cancer: what we eat may imply what we get, Protein Cell, 2018, 9, 474–487 CrossRef PubMed.
- GBD 2019 Colorectal Cancer Collaborators, Global, regional, and national burden of colorectal cancer and its risk factors, 1990–2019: A systematic analysis for the Global Burden of Disease Study 2019, Lancet Gastroenterol. Hepatol., 2022, 7, 627–647 CrossRef PubMed.
- A. Reynolds, J. Mann, J. Cummings, N. Winter, E. Mete and L. Te Morenga, Carbohydrate quality and human health: A series of systematic reviews and meta-analyses, Lancet, 2019, 393, 434–445 CrossRef CAS PubMed.
- A. G. Hullings, R. Sinha, L. M. Liao, N. D. Freedman, B. I. Graubard and E. Loftfield, Whole grain and dietary fiber intake and risk of colorectal cancer in the NIH-AARP Diet and Health Study Cohort, Am. J. Clin. Nutr., 2020, 112, 603–612 CrossRef PubMed.
- S. J. D. O'Keefe, J. V. Li, L. Lahti, J. Ou, F. Carbonero, K. Mohammed, J. M. Posma, J. Kinross, E. Wahl, E. Ruder, K. Vipperla, V. Naidoo, L. Mtshali, S. Tims, P. G. B. Puylaert, J. DeLany, A. Krasinskas, A. C. Benefiel, H. O. Kaseb, K. Newton, J. K. Nicholson, W. M. de Vos, H. R. Gaskins and E. G. Zoetendal, Fat, Fibre and cancer risk in african americans and rural africans, Nat. Commun., 2015, 6, 1–14 Search PubMed.
-
International Agency for Research on Cancer (IARC) working group on the Evaluation of Carcinogenic Risks to Humans, Red meat and processed meat, International Agency for Research on Cancer, Lyon, 2018. Available online: https://monographs.iarc.who.int/wp-content/uploads/2018/06/mono114.pdf (accessed February 2023) Search PubMed.
-
World Cancer Research Fund (WCRF) and American Institute for Cancer Research (AICR), Diet, nutrition, physical activity and cancer: A global perspective, Imperial College London WCRF/AICR Systematic Literature Review, 2018. Available online: https://www.wcrf.org/wp-content/uploads/2021/02/Colorectal-cancer-report.pdf (accessed February 2023) Search PubMed.
- A. J. Cross and R. Sinha, Meat-related mutagens/carcinogens in the etiology of colorectal cancer, Environ. Mol. Mutagen., 2004, 44, 44–55, DOI:10.1002/em.20030.
- I. J. Dikeocha, A. M. Al-Kabsi, M. Miftahussurur and M. A. Alshawsh, Pharmacomicrobiomics: Influence of gut microbiota on drug and xenobiotic metabolism, FASEB J., 2022, 36, e22350 CrossRef CAS PubMed.
- N. Koppel, V. M. Rekdal and E. P. Balskus, Chemical transformation of xenobiotics by the human gut microbiota, Science, 2017, 356, 1246–1257 CrossRef CAS PubMed.
- A. M. Nogacka, M. Gómez-Martín, A. Suárez, O. González-Bernardo, C. G. de los Reyes-Gavilán and S. González, Xenobiotics formed during food processing: Their relation with the intestinal microbiota and colorectal cancer, Int. J. Mol. Sci., 2019, 20, 2051 CrossRef CAS PubMed.
- V. L. Sutherland, C. A. McQueen, D. Mendrick, D. Gulezian, C. Cerniglia, S. Foley, S. Forry, S. Khare, X. Liang, J.
E. Manautou, D. Tweedie, H. Young, A. V. Alekseyenko, F. Burns, R. Dietert, A. Wilson and C. Chen, The gut microbiome and xenobiotics: Identifying knowledge gaps, Toxicol. Sci., 2020, 176, 1–10 CrossRef CAS PubMed.
- T. Sousa, R. Paterson, V. Moore, A. Carlsson, B. Abrahamsson and A. W. Basit, The gastrointestinal microbiota as a site for the biotransformation of drugs, Int. J. Pharm., 2008, 363, 1–25 CrossRef CAS PubMed.
- S. J. D. O'Keefe, Diet, microorganisms and their metabolites, and colon cancer, Nat. Rev. Gastroenterol. Hepatol., 2016, 13, 691–706 CrossRef PubMed.
- L. A. David, C. F. Maurice, R. N. Carmody, D. B. Gootenberg, J. E. Button, B. E. Wolfe, A. V. Ling, A. S. Devlin, Y. Varma, M. A. Fischbach, S. B. Biddinger, R. J. Dutton and P. J. Turnbaugh, Diet rapidly and reproducibly alters the human gut microbiome, Nature, 2013, 505, 559–563 CrossRef PubMed.
- F. Vernia, S. Longo, G. Stefanelli, A. Viscido and G. Latella, Dietary factors modulating colorectal carcinogenesis, Nutrients, 2021, 13, 143 CrossRef CAS PubMed.
- Y. Zhang, X. Yu, E. Yu, N. Wang, Q. Cai, Q. Shuai, F. Yan, L. Jiang, H. Wang, J. Liu, Y. Chen, Z. Li and Q. Jiang, Changes in gut microbiota and plasma inflammatory factors across the stages of colorectal tumorigenesis: A case-control study, BMC Microbiol., 2018, 18, 1–10 CrossRef CAS PubMed.
- B. Flemer, D. B. Lynch, J. M. R. Brown, I. B. Jeffery, F. J. Ryan, M. J. Claesson, M. O'Riordain, F. Shanahan and P. W. O'Toole, Tumour-associated and non-tumour-associated microbiota in colorectal cancer, Gut, 2017, 66, 633–643 CrossRef CAS PubMed.
- Y. Guo and H.-Y. Li, Association between Helicobacter pylori infection and colorectal neoplasm risk: A meta-analysis based on east asian population, J. Cancer Res. Ther., 2014, 10, C263–C266, DOI:10.4103/0973-1482.151482.
- C. M. Pillar and M. S. Gilmore, Enterococcal virulence - pathogenicity island of E. faecalis, Front. Biosci., 2004, 9, 2335–2346 CrossRef CAS PubMed.
- J. Yang, A. McDowell, E. K. Kim, H. Seo, W. H. Lee, C.-M. Moon, S.-M. Kym, D. H. Lee, Y. S. Park, Y.-K. Jee and Y.-K. Kim, Development of a colorectal cancer diagnostic model and dietary risk assessment through gut microbiome analysis, Exp. Mol. Med., 2019, 51, 1–15 Search PubMed.
- B. A. Peters, C. Dominianni, J. A. Shapiro, T. R. Church, J. Wu, G. Miller, E. Yuen, H. Freiman, I. Lustbader, J. Salik, C. Friedlander, R. B. Hayes and J. Ahn, The gut microbiota in conventional and serrated precursors of colorectal cancer, Microbiome, 2016, 4, 1–14 CrossRef PubMed.
- S. Ruiz-Saavedra, A. Zapico, C. G. del Rey, C. Gonzalez, A. Suárez, Y. Díaz, C. G. de los Reyes-Gavilán and S. González, Dietary xenobiotics derived from food processing: Association with fecal mutagenicity and gut mucosal damage, Nutrients, 2022, 14, 3482 CrossRef CAS PubMed.
- A. Zapico, S. Ruiz-Saavedra, M. Gómez-Martín, C. G. de los Reyes-Gavilán and S. González, Pilot study for the dietary assessment of xenobiotics derived from food processing in an adult Spanish sample, Foods, 2022, 11, 470 CrossRef CAS PubMed.
- M. Ocké, E. de Boer, H. Brants, J. van der Laan, M. Niekerk, C. van Rossum, L. Temme, H. Freisling, G. Nicolas, C. Casagrande, N. Slimani, E. Trolle, M. Ege, T. Christensen, S. Vandevijvere, M. Bellemans, M. De Maeyer, S. Defourny, J. Ruprich, M. Dofkova, I. Rehurkova, M. Jakubikova, J. Blahova, Z. Piskackova and M. Maly, PANCAKE – Pilot study for the assessment of nutrient intake and food consumption among kids in Europe, EFSA Supporting Publ., 2012, 9, 339E Search PubMed.
-
A. Farran, R. Zamora and P. Cervera, Tablas de composición de alimentos del Centro de Enseñanza Superior en Nutrición y Dietética (CESNID), McGraw-Hill, New York, USA, 2004. ISBN: 84-486-0590-X Search PubMed.
-
United States Department of Agriculture (USDA), Food composition databases. Available online: https://fdc.nal.usda.gov/(accessed February 2023).
- V. Neveu, J. Perez-Jiménez, F. Vos, V. Crespy, L. du Chaffaut, L. Mennen, C. Knox, R. Eisner, J. Cruz, D. Wishart and A. Scalbert, Phenol-Explorer: An online comprehensive database on polyphenol contents in foods, Database, 2010, 2010, bap024 CrossRef CAS PubMed.
- J. A. Marlett and T.-F. Cheung, Database and quick methods of assessing typical dietary fiber intakes using data for 228 commonly consumed foods, J. Am. Diet. Assoc., 1997, 97, 1139–1151 CrossRef CAS PubMed.
- X. Wu, G. R. Beecher, J. M. Holden, D. B. Haytowitz, S. E. Gebhardt and R. L. Prior, Lipophilic and hydrophilic antioxidant capacities of common foods in the United States, J. Agric. Food Chem., 2004, 52, 4026–4037 CrossRef CAS PubMed.
- P. Jakszyn;, A. Agudo, R. Ibáñez, R. García-Closas, G. Pera, P. Amiano and C. A. González, Development of a food database of nitrosamines, heterocyclic amines, and polycyclic aromatic hydrocarbons, J. Nutr., 2004, 134, 2011–2014 CrossRef PubMed.
-
National Cancer Institute: Division of Cancer Epidemiology & Genetics, Computerized Heterocyclic Amines Resource for Research in Epidemiology of Disease (CHARRED). Available online: https://dceg.cancer.gov/tools/design/charred (accessed February 2023).
- European Food Safety Authority (EFSA), Nitrate in vegetables–Scientific opinion of the Panel on contaminants in the food chain, EFSA J., 2008, 6, 689 CrossRef.
-
Food and Drug Administration (FDA), Survey data on acrylamide in food. Available online: https://www.fda.gov/food/chemical-contaminants-food/survey-data-acrylamide-food-total-diet-study-results (accessed February 2023).
- K. Svensson, L. Abramsson, W. Becker, A. Glynn, K.-E. Hellenäs, Y. Lind and J. Rosén, Dietary intake of acrylamide in Sweden, Food Chem. Toxicol., 2003, 41, 1581–1586 CrossRef CAS PubMed.
-
K.-E. Hellenäs, P. Fohgelberg, U. Fäger, L. Busk, A. Zetterberg, C. Ionescu and J. S. Färnstrand, Acrylamide in Swedish Food—Targeted Sampling 2011 and 2012. Available online: https://www.livsmedelsverket.se/globalassets/publikationsdatabas/rapporter/2013/<?pdb_no 2013?>2013<?pdb END?>_national_food_agency_25_acrylamide_in_swedish_food_targeted_sampling_2011_and_2012.pdf (accessed February 2023).
- E. J. M. Konings, A. J. Baars, J. D. van Klaveren, M. C. Spanjer, P. M. Rensen, M. Hiemstra, J. A. van Kooij and P. W. J. Peters, Acrylamide exposure from foods of the Dutch population and an assessment of the consequent risks, Food Chem. Toxicol., 2003, 41, 1569–1579 CrossRef CAS PubMed.
- G. Falcó, J. L. Domingo, J. M. Llobet, A. Teixidó, C. Casas and L. Müller, Polycyclic aromatic hydrocarbons in foods: Human exposure through the diet in Catalonia, Spain, J. Food Prot., 2003, 66, 2325–2331 CrossRef PubMed.
- L. Palacios Colón, A. J. Rascón and E. Ballesteros, Trace-Level Determination of polycyclic aromatic hydrocarbons in dairy products available
in Spanish supermarkets by semi-automated solid-phase extraction and gas chromatography-mass spectrometry detection, Foods, 2022, 11, 713 CrossRef PubMed.
- E. De Mey, K. De Klerck, H. De Maere, L. Dewulf, G. Derdelinckx, M.-C. Peeters, I. Fraeye, Y. Vander Heyden and H. Paelinck, The occurrence of N-nitrosamines, residual nitrite and biogenic amines in commercial dry fermented sausages and evaluation of their occasional relation, Meat Sci., 2014, 96, 821–828 CrossRef CAS PubMed.
- N. Campillo, P. Viñas, N. Martínez-Castillo and M. Hernández-Córdoba, Determination of volatile nitrosamines in meat products by microwave-assisted extraction and dispersive liquid–liquid microextraction coupled to gas chromatography–mass spectrometry, J. Chromatogr. A, 2011, 1218, 1815–1821 CrossRef CAS PubMed.
- H. S. Lee, Literature compilation of volatile N-nitrosamines in processed meat and poultry products - an update, Food Addit. Contam.: Part A, 2019, 36, 1491–1500 CrossRef CAS PubMed.
- J.-E. Park, J.-E. Seo, J.-Y. Lee and H. Kwon, Distribution of seven N-nitrosamines in food, Toxicol. Res., 2015, 31, 279–288 CrossRef CAS PubMed.
- L. T. Fike, H. Munro, D. Yu, Q. Dai and M. J. Shrubsole, Dietary polyphenols and the risk of colorectal cancer in the Prospective Southern Community Cohort Study, Am. J. Clin. Nutr., 2022, 115, 1155–1165 CrossRef PubMed.
- Y. H. Loh, P. Jakszyn, R. N. Luben, A. A. Mulligan, P. N. Mitrou and K.-T. Khaw, N-Nitroso compounds and cancer incidence: The European Prospective Investigation into Cancer and Nutrition (EPIC)-Norfolk Study, Am. J. Clin. Nutr., 2011, 93, 1053–1061 CrossRef CAS PubMed.
- V. Martínez Góngora, K. L. Matthes, P. R. Castaño, J. Linseisen and S. Rohrmann, Dietary heterocyclic amine intake and colorectal adenoma risk: A systematic review and meta-analysis, Cancer Epidemiol. Biomarkers Prev., 2019, 28, 99–109 CrossRef PubMed.
- N. Salazar, M. Gueimonde, A. M. Hernández-Barranco, P. Ruas-Madiedo and C. G. de los Reyes-Gavilán, Exopolysaccharides produced by intestinal Bifidobacterium strains act as fermentable substrates for human intestinal bacteria, Appl. Environ. Microbiol., 2008, 74, 4737–4745 CrossRef CAS PubMed.
- B. Redruello, V. Ladero, I. Cuesta, J. R. Álvarez-Buylla, M. C. Martín, M. Fernández and M. A. Alvarez, A fast, reliable, ultra high performance liquid chromatography method for the simultaneous determination of amino acids, biogenic amines and ammonium ions in cheese, using diethyl ethoxymethylenemalonate as a derivatising agent, Food Chem., 2013, 139, 1029–1035 CrossRef CAS PubMed.
- N. Salazar, M. Ponce-Alonso, M. Garriga, S. Sánchez-Carrillo, A. M. Hernández-Barranco, B. Redruello, M. Fernández, J. I. Botella-Carretero, B. Vega-Piñero, J. Galeano, J. Zamora, M. Ferrer, C. G. de Los Reyes-Gavilán and R. Del Campo, Fecal metabolome and bacterial composition in severe obesity: impact of diet and bariatric surgery, Gut Microbes, 2022, 14, 2106102 CrossRef PubMed.
-
IHMS Consortium, IHMS_SOP 06 V2: Standard operating procedure for fecal samples DNA extraction, Protocol Q, International Human Microbiome Standards (IHMS). Available online: https://brd.nci.nih.gov/brd/sop/download-pdf/2105 (accessed February 2023).
- T. Magoč and S. L. Salzberg, FLASH: Fast length adjustment of short reads to improve genome assemblies, Bioinformatics, 2011, 27, 2957–2963 CrossRef PubMed.
- J. G. Caporaso, J. Kuczynski, J. Stombaugh, K. Bittinger, F. D. Bushman, E. K. Costello, N. Fierer, A. G. Peña, J. K. Goodrich, J. I. Gordon, G. A. Huttley, S. T. Kelley, D. Knights, J. E. Koenig, R. E. Ley, C. A. Lozupone, D. McDonald, B. D. Muegge, M. Pirrung, J. Reeder, J. R. Sevinsky, P. J. Turnbaugh, W. A. Walters, J. Widmann, T. Yatsunenko, J. Zaneveld and R. Knight, QIIME allows analysis of high-throughput community sequencing data, Nat. Methods, 2010, 7, 335–336 CrossRef CAS PubMed.
- N. A. Bokulich, S. Subramanian, J. J. Faith, D. Gevers, J. I. Gordon, R. Knight, D. A. Mills and J. G. Caporaso, Quality-filtering vastly improves diversity estimates from Illumina amplicon sequencing, Nat. Methods, 2013, 10, 57–59 CrossRef CAS PubMed.
- R. C. Edgar, B. J. Haas, J. C. Clemente, C. Quince and R. Knight, UCHIME improves sensitivity and speed of chimera detection, Bioinformatics, 2011, 27, 2194–2200 CrossRef CAS PubMed.
- C. Quast, E. Pruesse, P. Yilmaz, J. Gerken, T. Schweer, P. Yarza, J. Peplies and F. O. Glöckner, The SILVA ribosomal RNA gene database project: Improved data processing and web-based tools, Nucleic Acids Res., 2013, 41, D590–D596 CrossRef CAS PubMed.
- S. F. Altschul, W. Gish, W. Miller, E. W. Myers and D. J. Lipman, Basic local alignment search tool, J. Mol. Biol., 1990, 215, 403–410 CrossRef CAS PubMed.
- R. C. Edgar, MUSCLE: Multiple sequence alignment with high accuracy and high throughput, Nucleic Acids Res., 2004, 32, 1792–1797 CrossRef CAS PubMed.
- N. Segata, J. Izard, L. Waldron, D. Gevers, L. Miropolsky, W. S. Garrett and C. Huttenhower, Metagenomic biomarker discovery and explanation, Genome Biol., 2011, 12, R60 CrossRef PubMed.
- D. J. Harriss, G. Atkinson, K. George, N. T. Cable, T. Reilly, N. Haboubi, M. Zwahlen, M. Egger and A. G, Renehan and C-CLEAR group, Lifestyle factors and colorectal cancer risk (1): Systematic review and meta-analysis of associations with body mass index, Colorectal Dis., 2009, 11, 547–563 CrossRef CAS PubMed.
- Y. Ning, L. Wang and E. L. Giovannucci, A quantitative analysis of body mass index and colorectal cancer: Findings from 56 observational studies, Obes. Rev., 2010, 11, 19–30 CrossRef CAS PubMed.
- A. Tomova, I. Bukovsky, E. Rembert, W. Yonas, J. Alwarith, N. D. Barnard and H. Kahleova, The effects of vegetarian and vegan diets on gut microbiota, Front. Nutr., 2019, 6, 47 CrossRef PubMed.
- Q. Zhu, R. Gao, W. Wu and H. Qin, The role of gut microbiota in the pathogenesis of colorectal cancer, Tumor Biol., 2013, 34, 1285–1300 CrossRef PubMed.
- P. Puri, S. Liangpunsakul, J. E. Christensen, V. H. Shah, P. S. Kamath, G. J. Gores, S. Walker, M. Comerford, B. Katz, A. Borst, Q. Yu, D. P. Kumar, F. Mirshahi, S. Radaeva, N. P. Chalasani, D. W. Crabb and A. J. Sanyal, (TREAT consortium), The circulating microbiome signature and inferred functional metagenomics in alcoholic hepatitis, Hepatology, 2018, 67, 1284–1302 CrossRef CAS PubMed.
- V. L. Hale, J. Chen, S. Johnson, S. C. Harrington, T. C. Yab, T. C. Smyrk, H. Nelson, L. A. Boardman, B. R. Druliner, T. R. Levin, D. K. Rex, D. J. Ahnen, P. Lance, D. A. Ahlquist and N. Chia, Shifts in the fecal microbiota associated with adenomatous polyps, Cancer Epidemiol. Biomarkers Prev., 2017, 26, 85–94 CrossRef CAS PubMed.
- A. T. Lawal, Polycyclic aromatic hydrocarbons. A review, Cogent Environ. Sci., 2017, 3, 1339841 CrossRef.
- J. L. Waters and R. E. Ley, The human gut bacteria Christensenellaceae are widespread, heritable and associated with health, BMC Biol., 2019, 17, 1–11 CrossRef PubMed.
- J. Wang, S. Wu, Y. Zhang, J. Yang and Z. Hu, Gut microbiota and calcium balance, Front. Microbiol., 2022, 13, 5067 Search PubMed.
- K. Skrypnik and J. Suliburska, Association between the gut microbiota and mineral metabolism, J. Sci. Food Agric., 2018, 98, 2449–2460 CrossRef CAS PubMed.
- C. M. Weaver, Diet, gut microbiome and bone health, Curr. Osteoporos. Rep., 2015, 13, 125–130 CrossRef PubMed.
- Z. Asemi and A. Esmaillzadeh, A effect of daily consumption of probiotic yoghurt on serum levels of calcium, iron and liver enzymes in pregnant women, Int. J. Prev. Med., 2013, 4, 949–955 Search PubMed.
- O. Illescas, M. Rodríguez-Sosa and M. Gariboldi, Mediterranean diet to prevent the development of colon diseases: A meta-analysis of gut microbiota studies, Nutrients, 2021, 13, 2234 CrossRef CAS PubMed.
- F. A. Tomás-Barberán, M. V. Selma and J. C. Espín, Interactions of gut microbiota with dietary polyphenols and consequences to human health, Curr. Opin. Clin. Nutr. Metab. Care, 2016, 19, 471 CrossRef PubMed.
- H. B. Jang, M.-K. Choi, J. H. Kang, S. I. Park and H.-J. Lee, Association of dietary patterns with the fecal microbiota in Korean adolescents, BMC Nutr., 2017, 3, 1–11 CrossRef.
- M. Derrien, C. Belzer and W. M. de Vos, Akkermasia muciniphila and its role in regulating host functions, Microb. Pathog., 2017, 106, 171–181 CrossRef PubMed.
- Q. Zhao, J. Yu, Y. Hao, H. Zhou, Y. Hu, C. Zhang, H. Zheng, X. Wang, F. Zeng, J. Hu, L. Gu, Z. Wang, F. Zhao, C. Yue, P. Zhou, H. Zhang, N. Huang, W. Wu, Y. Zhou and J. Li, Akkermansia muciniphila plays critical roles in host health, Crit. Rev. Microbiol., 2023, 49, 82–100 CrossRef CAS PubMed.
- J. Hu, P. Guo, R. Mao, Z. Ren, J. Wen, Q. Yang, T. Yan, J. Yu, T. Zhang and Y. Liu, Gut microbiota signature of obese adults across different classifications, DMSO, 2022, 15, 3933–3947 CrossRef CAS PubMed.
- T. Senthakumaran, A. E. F. Moen, T. M. Tannæs, A. Endres, S. A. Brackmann, T. B. Rounge, V. Bemanian and H. S. Tunsjø, Microbial dynamics with CRC progression: A study of the mucosal microbiota at multiple sites in cancers, adenomatous polyps and healthy controls, Eur. J. Clin. Microbiol. Infect. Dis., 2023, 42, 305–322 CrossRef CAS PubMed.
- Global Dietary Database Collaborators, Global, regional, and national consumption of animal-source foods between 1990 and 2018: Findings from the Global Dietary Database, Lancet Planet. Health, 2022, 6, e243–e256 CrossRef PubMed.
- Global Burden of Diseases Nutrition and Chronic Diseases Expert Group (NutriCoDE), Global, regional, and national consumption levels of dietary fats and oils in 1990 and 2010: A systematic analysis including 266 country-specific nutrition surveys, Br. Med. J., 2014, 348, g2272 CrossRef PubMed.
- A. M. Alves-Santos, C. S. A. Sugizaki, G. C. Lima and M. M. V. Naves, Prebiotic effect of dietary polyphenols: A systematic review, J. Funct. Foods, 2020, 74, 104169 CrossRef CAS.
- F. Braghiroli, V. Fierro, A. Pizzi, K. Rode, W. Radke, L. Delmotte, J. Parmentier and A. Celzard, Reaction of condensed tannins with ammonia, Ind. Crops Prod., 2013, 44, 330–335 CrossRef CAS.
|
This journal is © The Royal Society of Chemistry 2023 |