DOI:
10.1039/D3FO02879H
(Paper)
Food Funct., 2023,
14, 10418-10429
Unveiling the influence of daily dietary patterns on brain cortical structure: insights from bidirectional Mendelian randomization†
Received
14th July 2023
, Accepted 4th November 2023
First published on 9th November 2023
Abstract
Cognitive impairment is a significant concern in aging populations. This study utilized Mendelian randomization analysis to explore the impact of dietary habits and macro-nutrients on cortical structure. A bidirectional Mendelian randomization approach was employed, incorporating large-scale genetic data on dietary habits and brain cortical structure. The results did not reveal significant causal relationships between dietary factors and overall cortical structure and thickness. However, specific dietary factors showed associations with cortical structure in certain regions. For instance, fat intake affected six cortical regions, while milk, protein, fruits, and water were associated with changes in specific regions. Reverse analysis suggested that cortical thickness influenced the consumption of alcohol, carbohydrates, coffee, and fish. These findings contribute to understanding the potential mechanisms underlying the role of dietary factors in cognitive function changes and provide evidence supporting the existence of the gut–brain axis.
Introduction
Cognitive impairment is observed during the aging process, spanning from mild cognitive impairment to Alzheimer's disease (AD). This condition is prevalent in all individuals. According to the 2021 mortality statistics from the National Center for Health Statistics in the United States, AD is the leading cause of death.1 Current treatment approaches primarily focus on alleviating symptoms,2 The amalgamation of stem cell therapy and nanoparticles in current research holds promising potential for advancing our understanding and enhancing approaches to the diagnosis, treatment, and study of neurodegenerative diseases.3 However, despite these promising developments, there remains a deficit in effective interventions for cognitive impairment disorders. This indicates the need for preventive measures to mitigate the burden of cognitive impairment in the post-aging period.
Certain dietary patterns have been found to reduce the risk of cognitive impairment. High adherence to the Mediterranean diet (MeDi)4 and the Mediterranean-DASH intervention for Neurodegenerative Delay diet5 (MIND) has been associated with a decreased risk of cognitive impairment, as evidenced by clinical trials and meta-analyses.6,7 In addition to dietary patterns, variations in the intake ratios of specific nutrients, including protein, fat, and carbohydrates, can exert an influence on cognitive function.8 A deficiency of dietary fiber, a type of carbohydrate, is associated with cognitive impairment and has been linked to disruptions in gut microbiota in mouse models, ultimately contributing to cognitive decline.9
To investigate the potential correlation between dietary patterns, nutrients, and changes in brain structure, recent studies examining the relationship between diet and imaging biomarkers have shown that greater adherence to MeDi is associated with greater brain volume10 and greater frontal, parietal and occipital volumes and mean cortical thickness (TH), higher intake of legumes and fish was related to increased cortical TH, while higher intake of carbohydrates and sugars was associated with lower olfactory cortex TH.11 While there is a correlation suggested by these studies between dietary patterns, nutrients, and brain structure, it remains unclear whether any causal relationship exists.
Mendelian randomization (MR) is a novel approach to assess the causal relationship between risk factors associated with outcomes using genetic instrumental variables (IVs). MR analysis has been utilized to investigate causal relationships between alcohol consumption,12 coffee intake,13 cereal consumption,14 and cognitive function, as well as associations between systemic inflammatory markers,15 blood biochemistry traits,16 and cortical structure. However, the causal relationship between dietary habits, macro-nutrients, and cortical structure has not been firmly established.
In conclusion, we know that diet has a certain impact on cognitive function, and there is an association between dietary measures and changes in brain structure as assessed by MRI. However, the directionality of this association remains uncertain. In this study, we employed a two-sample MR analysis to elucidate the potential causal relationship between 19 dietary habits and four macro-nutrients on brain cortical structure (Fig. 1), providing feasible strategies for early prevention and improvement of cognitive impairment.
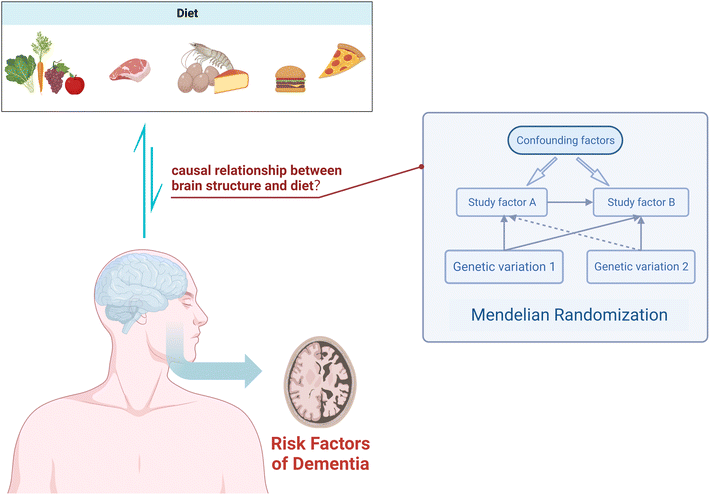 |
| Fig. 1 Using Mendelian randomization to study the causal relationship between dietary factors and cortical brain structure. | |
Materials and methods
Data sources for dietary factors
The data on dietary factors used in this study were derived from recent large-scale genome-wide association study (GWAS) on dietary habits.17 Here, the genetic heritability and GWAS analysis were reported for both single food item quantitative traits and principal component-derived dietary patterns analyzed using food frequency questionnaire data from 449
210 individuals of European ancestry in the UK. For all variables, linear mixed model association tests were performed using the BOLT-LMM software (V2.3.2) to account for relatedness. LDstore v1.157 was employed to calculate linkage disequilibrium (LD) and identify Single nucleotide polymorphisms (SNPs) in high LD (r2 ≥ 0.80) with any of the 77
229 SNPs in the 95% credible set. Strict Bonferroni correction thresholds (P < 0.05/460
317 = 1.09 × 10−7) were applied to all pairwise tests between 143 dietary habits and 3219 highly correlated or overlapping Neale Lab GWAS traits. A total of 814 LD-independent loci (defined as >500 kb apart) were identified, surpassing genome-wide significance (P < 5.0 × 10−8). For more detailed information about this study, please refer to the published research.
Data sources for macronutrients
The summary statistics of GWAS related to dietary composition were derived from the SSGAC19 consortium.18 GWAS analyses were conducted on four dietary composition phenotypes—relative intake of fat, carbohydrates, protein, and sugar—in individuals of European ancestry. The initial discovery analysis was performed within the UK Biobank, and replication analyses were conducted in several other GWAS cohorts, including ALSPAC, DietGen, EPIC InterAct, Fenland, FHS, HRS, Lifelines, RSI/II/III, and WHI (GARNET, HIPFX, and WHIMS+). Notably, DietGen specifically analyzed the intake of fat, protein, and carbohydrates as a percentage of total energy intake. Hence, the final sample sizes were Nsugar = 235
391 and Nfat = Nprotein = Ncarbohydrate = 268
922. The relative intake of carbohydrates, fat, and protein was assessed through comprehensive food questionnaires, measuring either the intake from the previous day (UKB) or habitual intake (other cohorts). The relative intake was defined as constant nutrient intake adjusted for total energy intake. Standard quality control measures were applied to each GWAS dataset to ensure data integrity and accuracy.
Data sources for the surface area (SA) and TH of the cerebral cortex
The GWAS data related to cortical brain structure were obtained from the ENIGMA consortium.19 MRI was used to measure the SA and TH of the cortical regions in a total of 51
665 individuals from 60 cohorts worldwide. The cortical regions were roughly parcellated based on the Desikan–Killiany atlas, defining 34 regions, and the boundaries between sulcal landmarks were determined to demarcate the regions.20 Genetic associations were computed using an additive model within each cohort, adjusting for covariates such as age, age squared, sex, age-by-sex interaction, the first four multidimensional scaling components, diagnostic status (when cohorts followed a case-control design), and scanner-specific virtual variables if applicable. It is important to note that our study utilized results from 49 cohorts of European ancestry participants from the ENIGMA consortium, excluding the UK Biobank (n = 23
909).
Selection of genetic IVs
This study employed the following criteria to select IVs. (1) SNPs that reached the genome-wide significance level (P < 5.0 × 10−8) for association with each dietary habit category were considered as potential IVs. (2) LD of SNPs was calculated using data from European samples of the 1000 Genomes Project as a reference, with a truncation threshold of R2 < 0.001 and a clustering window of 10
000 kb. When SNPs were in LD, the SNP with the lowest P-value was retained. (3) To account for horizontal pleiotropy, methods such as MR-Egger and MR-PRESSO were employed in this study. MR-PRESSO global test was used to identify horizontal pleiotropy. MR-PRESSO outlier test was used to assess heterogeneity and obtain significant P-values for each SNP, thereby excluding horizontal pleiotropy. (4) The effect allele was inferred using allele frequency information, which is referred to as “action 2”.
MR analysis
In this study, we employed several different MR methods, including inverse-variance weighted (IVW), MR-Egger, weighted median, weighted mode, and MR-PRESSO, to assess the causal relationship between dietary habits and cortical brain structure. The primary MR analysis was conducted using the IVW method. This method combines Wald ratio estimates for each SNP into a single causal estimate for each risk factor, where each estimate is obtained by dividing the SNP-outcome association by the SNP-exposure association. If there is no horizontal pleiotropy, the IVW results are unbiased.21 The flowchart of the study is shown in Fig. 2. To assess the potential impact of horizontal pleiotropy, we conducted MR-Egger regression. MR-Egger regression is based on the InSIDE (Instrument Strength Independent of Direct Effect) assumption, which states that the intercept term is equal to zero in the absence of horizontal pleiotropy. If the intercept term is zero, it suggests the absence of horizontal pleiotropy, and the results of MR-Egger regression are consistent with IVW.22 In the presence of horizontal pleiotropy, the slope coefficient of MR-Egger regression provides a consistent estimate of the causal effect. Additionally, MR-PRESSO was employed to test for pleiotropy. Its function includes identifying horizontal pleiotropy and analyzing it by removing significant outliers to detect and attempt to reduce horizontal pleiotropy.23
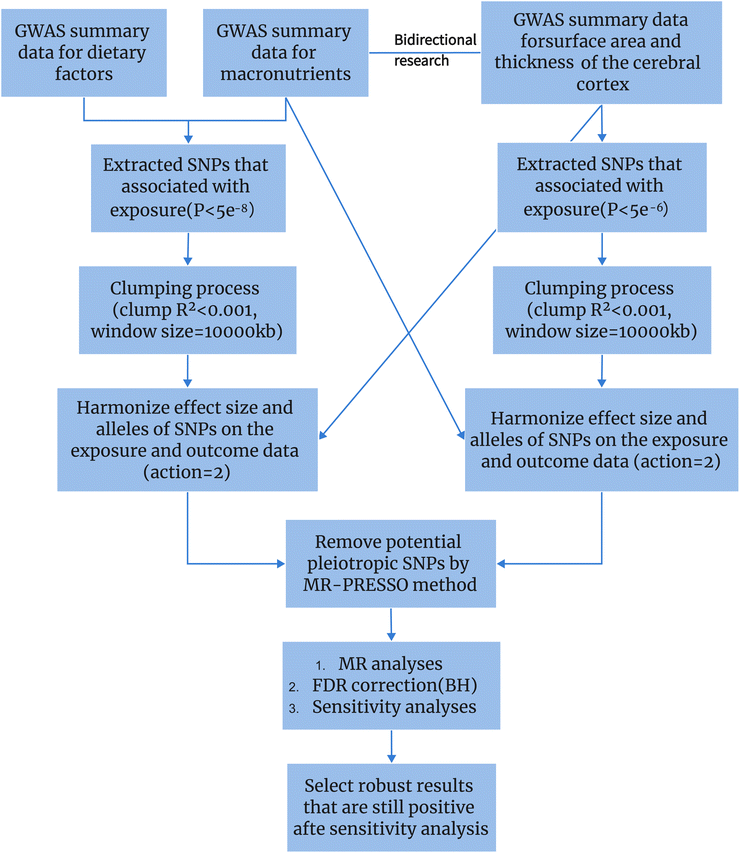 |
| Fig. 2 The whole workflow of MR analysis. | |
We calculated the F-statistic for each valid SNP using the following algorithm:24
If the corresponding F-statistic is greater than 10, it is considered to have no significant weak instrument bias.
A false discovery rate (FDR) was used to correct all P value. The significance threshold was set at q.adjusted < 0.05. When P < 0.05 and q ≥ 0.05, it is considered that there is a potential association between dietary habits and cortical brain structure. Therefore, our results fall into two scenarios: (1) if the results of action 2 are significant and remain significant after FDR correction, it indicates a highly significant association; (2) if the results of action 2 are significant but become non-significant after FDR correction, it suggests a potential association.
We also performed a reverse MR analysis on dietary habits to further evaluate their potential causal impact on cortical structure. In this step, due to the limited number of SNPs available for some cortical regions, we used a P-value threshold of P < 5.0 × 10−6, consistent with the methodology employed in the forward MR analysis.
Results
Selection of IVs
In the initial stage, under the genome-wide significance threshold (P < 5.0 × 10−8), a total of 97
275 SNPs were identified across 23 dietary habits. Additional details regarding the selected instrumental instruments can be found in ESI 2.† Following clumping and harmonization, a total of 2749 IVs were identified. For detailed information on IVs related to each dietary habit and cortical brain regions, please refer to ESI 3.† Subsequently, by removing SNPs identified to have multicollinearity through MR-PRESSO outlier test and MR-Egger regression (both MR-PRESSO global test P > 0.05 and MR-Egger regression P > 0.05), we ensured that the remaining IVs were free from any horizontal pleiotropy.
Diet and global analysis
At the global level, we conducted MR analyses on SA and TH of the global cortical brain using 23 different dietary habits. We employed five MR(IVW, MR-Egger, weighted median, weighted mode, and MR-PRESSO) analysis methods and found that increased frequency of total beef intake (β = 5763.16; 95% CI: 1202.48, 10
323.83; P = 0.013) and daily cup consumption of tea (β = 3423.52; 95% CI: 703.01, 6144.02; P = 0.014) were associated with increased SA, but showed no causal relationship with TH. Drinking hot water temperature (β = −0.029; 95% CI: −0.05, −0.01; P = 0.017) and frequency of non-oily fish intake (β = −0.029; 95% CI: −0.05, −0.01; P = 0.005) were associated with decreased TH, but showed no causal relationship with SA. However, after FDR correction, none of the above results remained statistically significant (q = 0.12, 0.12, 0.20, 0.11). Additional detailed information can be found in ESI 1, Sections 1.2 and 1.4, as well as Table 3.2 in ESI 3,† providing further insights into the data.
Association between dietary habits and cortical TH and SA in 34 brain regions
At the level of functional parcellation analysis, we conducted MR analysis on 34 globally weighted brain regions, examining their SA and cortical TH in relation to 23 dietary habits. All the results from this stage can be obtained from Table 1.1 and 1.3 in ESI 1, as well as Table 3.1 in ESI 3.† Our findings revealed significant influences of 6 dietary habits on the cortical structure of 18 functional brain regions (Table 1). As shown in Fig. 3, specifically, an increase in the daily consumption of fresh fruits was associated with higher TH in the insula (β = 0.041; 95% CI: 0.02, 0.06; q = 0.0026). The use of whole milk significantly impacted the cortical structure of 5 brain regions, with increased SA in the supramarginal (β = 297.63; 95% CI: 152.16, 443.11; q = 0.0013) and increased TH in the entorhinal (β = 0.14; 95% CI: 0.06, 0.22; q = 0.0076), and decreased SA in the precentral (β = −426.94; 95% CI: −713.99, −139.89; q = 0.041), TH in the insula (β = −0.079; 95% CI: −0.11, −0.05; q = 0.000082), and precuneus (β = −0.043; 95% CI: −0.06, −0.03; q = 0.00000000027). The consumption of skim milk had significant effects on the cortical structure of 4 brain regions, increasing SA in the precentral (β = 267.30; 95% CI: 93.89, 440.72; q = 0.043) and superior frontal (β = 209.36; 95% CI: 100.25, 318.47; q = 0.012), while decreasing SA in the lingual (β = −116.96; 95% CI: −182.41, −51.51; q = 0.016) and TH in the entorhinal (β = −0.14; 95% CI: −0.22, −0.06; q = 0.025). A higher daily water intake was associated with increased SA in the lateral occipital (β = 120.34; 95% CI: 53.81, 186.86; q = 0.027). In the presence of fat intake, the cortical structure of 6 brain regions showed changes, including increased SA in the inferior temporal (β = 114.37; 95% CI: 55.76, 172.99; q = 0.0029), middle temporal (β = 76.43; 95% CI: 47.51, 105.34; q = 0.0000075), and postcentral (β = 85.75; 95% CI: 32.60, 138.90; q = 0.022), and decreased SA in the pars opercularis (β = −78.18; 95% CI: −119.97, −36.4; q = 0.0041), and TH in the caudal middle frontal (β = −0.024; 95% CI: −0.03, −0.02; q = 0.00000010) and superior frontal (β = −0.030; 95% CI: −0.05, −0.01; q = 0.045), as well as decreased TH in the frontal pole (β = −0.10; 95% CI: −0.16, −0.04; q = 0.043) in response to protein consumption.
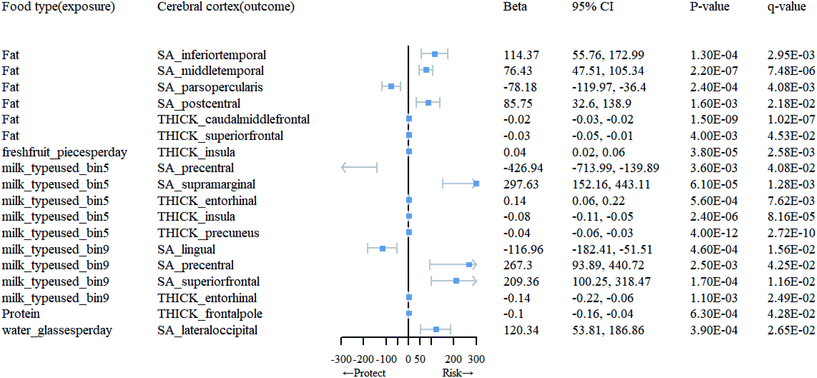 |
| Fig. 3 Mendelian randomization results of causal effects between food type and cortical regions. | |
Table 1 Full significant association of MR estimates for the association between food type and cerebral cortex. SA: surface area; THICK: thickness; freshfruit_piecesperday: pieces of fresh fruit per day; milk_typeused_bin5: milk type: full cream; milk_typeused_bin9: milk type: skimmed; water_glassesperday: glasses of water per day
Food type (exposure) |
Cerebral cortex (outcome) |
MR method |
nsnp |
F
|
95% CI |
p-Value |
q-Value |
Fat |
SA_inferiortemporal |
IVW(MRE) |
5 |
31.6 |
(55.76, 172.99) |
1.30 × 10−4 |
2.95 × 10−3 |
SA_middletemporal |
IVW(MRE) |
5 |
31.6 |
(47.51, 105.34) |
2.20 × 10−7 |
7.48 × 10−6 |
SA_parsopercularis |
IVW(MRE) |
5 |
31.6 |
(−119.97, −36.4) |
2.40 × 10−4 |
4.08 × 10−3 |
SA_postcentral |
IVW(MRE) |
5 |
31.6 |
(32.6, 138.9) |
1.60 × 10−3 |
2.18 × 10−2 |
THICK_caudalmiddlefrontal |
IVW(MRE) |
5 |
31.6 |
(−0.03, −0.02) |
1.50 × 10−9 |
1.02 × 10−7 |
THICK_superiorfrontal |
IVW(MRE) |
5 |
31.6 |
(−0.05, −0.01) |
4.00 × 10−3 |
4.53 × 10−2 |
Freshfruit_piecesperday |
THICK_insula |
IVW(MRE) |
74 |
29.36 |
(0.02, 0.06) |
3.80 × 10−5 |
2.58 × 10−3 |
Milk_typeused_bin5 |
SA_precentral |
IVW(MRE) |
5 |
31.64 |
(−713.99, −139.89) |
3.60 × 10−3 |
4.08 × 10−2 |
SA_supramarginal |
IVW(MRE) |
5 |
31.64 |
(152.16, 443.11) |
6.10 × 10−5 |
1.28 × 10−3 |
THICK_entorhinal |
IVW(MRE) |
5 |
31.64 |
(0.06, 0.22) |
5.60 × 10−4 |
7.62 × 10−3 |
THICK_insula |
IVW(MRE) |
5 |
31.64 |
(−0.11, −0.05) |
2.40 × 10−6 |
8.16 × 10−5 |
THICK_precuneus |
IVW(MRE) |
5 |
31.64 |
(−0.06, −0.03) |
4.00 × 10−12 |
2.72 × 10−10 |
Milk_typeused_bin9 |
SA_lingual |
IVW(MRE) |
5 |
32.23 |
(−182.41, −51.51) |
4.60 × 10−4 |
1.56 × 10−2 |
SA_precentral |
IVW(MRE) |
5 |
32.23 |
(93.89, 440.72) |
2.50 × 10−3 |
4.25 × 10−2 |
SA_superiorfrontal |
IVW(MRE) |
5 |
32.23 |
(100.25, 318.47) |
1.70 × 10−4 |
1.16 × 10−2 |
THICK_entorhinal |
IVW(MRE) |
5 |
32.23 |
(−0.22, −0.06) |
1.10 × 10−3 |
2.49 × 10−2 |
Protein |
THICK_frontalpole |
IVW(MRE) |
7 |
32.04 |
(−0.16, −0.04) |
6.30 × 10−4 |
4.28 × 10−2 |
Water_glassesperday |
SA_lateraloccipital |
IVW(MRE) |
59 |
28.58 |
(53.81, 186.86) |
3.90 × 10−4 |
2.65 × 10−2 |
Sensitivity analyses
In this study, all MR methods produced consistent estimates of the direction of causal association in the results. The forest plot, funnel plot, leave-one-out plot, and scatter plots are provided in the ESI 9 (Fig. 3–10†). The F-statistics for all IVs were greater than 10, indicating the mitigation of bias caused by weak IVs. Additionally, as shown in ESI 4, based on the analysis of MR-Egger regression intercept, there was no significant evidence of directional horizontal pleiotropy.
Reverse MR analysis
Detailed information on the selected IVs for reverse MR analysis can be found in ESI 6.† Subsequently, in ESI 7,† we present the SNPs after clumping and harmonization. Based on the results of the reverse MR analysis, as shown in ESI 5 (Tables 5.3 and 5.4†), we found significant evidence of association between brain cortical structure and dietary factors (Table 2). As depicted in Fig. 4, we observed that an increase in cortical TH in the caudal anterior cingulate (cACC) promotes the use of carbohydrates (β = 0.29; 95% CI: 0.12, 0.45; q = 0.031), while an increase in TH in the lateral orbitofrontal and lateral occipital inhibits the use of coffee (β = −0.29, −0.48; 95% CI: −0.45, −0.13; −0.71, −0.24; q = 0.01, 0.0037). Moreover, an increase in TH in the paracentral region suppresses the consumption of oily fish (β = −0.47; 95% CI: −0.72, −0.23; q = 0.012), and an increase in TH in the cACC inhibits alcohol intake (β = −0.18; 95% CI: 0.28, −0.09; q = 0.0095). Additionally, an increase in SA in the medial orbitofrontal cortex (OFC) suppresses the consumption of non-oily fish (β = −0.00027; 95% CI: 0, 0, q = 0.000018). We found that, following sensitivity analysis (see ESI 9), the causal relationships between them are unlikely to be biased by horizontal pleiotropy.
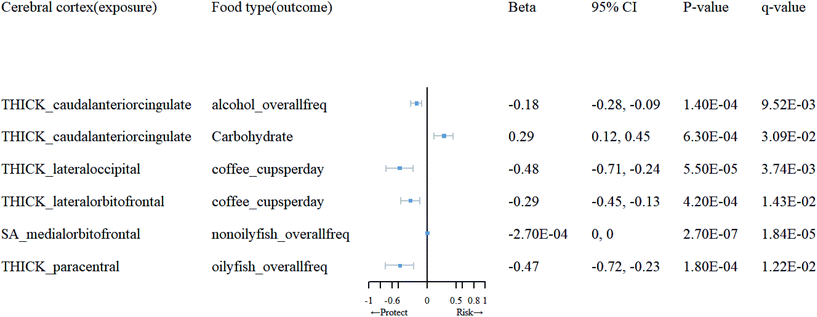 |
| Fig. 4 Mendelian randomization results of causal effects between cortical regions and food type. | |
Table 2 Full significant association of MR estimates for the association between cerebral cortex and food type. SA: Surface area; THICK: Thickness; alcohol_overallfreq: Alcohol consumption; coffee_cupsperday: Cups of coffee per day; nonoilyfish_overallfreq: Non-oily fish consumption; oilyfish_overallfreq: Oily fish consumption
Cerebral cortex (exposure) |
Food type(outcome) |
MR method |
nsnp |
F
|
95% CI |
p-Value |
q-Value |
THICK_caudalanteriorcingulate |
Alcohol_overallfreq |
IVW(MRE) |
10 |
20.72 |
(−0.28, −0.09) |
1.40 × 10−4 |
9.52 × 10−3 |
Carbohydrate |
IVW(MRE) |
9 |
20.72 |
(0.12, 0.45) |
6.30 × 10−4 |
3.09 × 10−2 |
THICK_lateraloccipital |
Coffee_cupsperday |
IVW(MRE) |
13 |
20.75 |
(−0.71, −0.24) |
5.50 × 10−5 |
3.74 × 10−3 |
THICK_lateralorbitofrontal |
Coffee_cupsperday |
IVW(MRE) |
7 |
20.6 |
(−0.45, −0.13) |
4.20 × 10−4 |
1.43 × 10−2 |
SA_medialorbitofrontal |
Nonoilyfish_overallfreq |
IVW(MRE) |
10 |
22.02 |
(0, 0) |
2.70 × 10−7 |
1.84 × 10−5 |
THICK_paracentral |
Oilyfish_overallfreq |
IVW(MRE) |
14 |
20.25 |
(−0.72, −0.23) |
1.80 × 10−4 |
1.22 × 10−2 |
Discussion
In this study, we systematically assessed the causal relationship between genetically predicted dietary preferences and brain cortical structure. Multiple dietary habits were found to influence the structure of specific brain regions, and alterations in the cortical structure of certain regions also affect dietary habits, suggesting potential interactions between dietary habits and cortical structure of the brain. Our results indicate that while certain dietary habits may have an impact on the overall cortical TH or SA of the brain, the effects are not statistically significant.
Our study revealed that the consumption of whole milk is associated with a decrease in the SA of the precentral gyrus, as well as a decrease in the cortical TH of the insula and precuneus. Whole milk contains milk fat.25 which is high in saturated fatty acids (SFAs) and has been associated with atherosclerosis, cardiovascular diseases,26,27 cognitive decline, and dementi.28,29 The precuneus, a densely connected central region in the brain,30 is involved in the default mode network (DMN).31 and plays a crucial role in AD development.32 The precentral gyrus, known as the primary motor cortex, is actively involved in cognitive activities.33 Previous studies have found reduced gray matter volume in the precentral gyrus of carriers of the apolipoprotein E (APOE)-ε4 allele, which is a recognized risk gene for AD.34 The anterior insula has been implicated in cognitive neurodegenerative disorders, including AD-related hallucinations.35 Therefore, it can be inferred that certain non-beneficial components present in whole milk may contribute to cognitive impairments related to AD by altering the cortical TH of the brain. The underlying mechanisms warrant further investigation.
Skimmed milk, characterized by its reduced fat content compared to whole milk, consequently exhibits a lower concentration of SFAs. Our research findings indicate that the consumption of skim milk leads to an increase in SA within the precentral gyrus, as opposed to the impact of whole milk consumption. This observation substantiates our earlier hypothesis that SFAs in whole milk may exert an influence on cortical structures associated with cognition. Furthermore, the intake of skim milk results in an augmented SA within the superior frontal cortex, while fat consumption elicits an opposing effect. Aggressive behavior in patients with AD has been linked to cortical TH in the left superior frontal gyrus (SFG),36,37 and an appropriate dietary fat intake may mitigate this symptom by reducing the SA of the superior frontal cortex. Notably, it is intriguing to observe that the consumption of skim milk concurrently diminishes TH in the entorhinal cortex and SA in the lingual cortex, whereas an opposing protective effect is noted for TH in the entorhinal cortex when whole milk is consumed. The entorhinal and lingual cortices are recognized brain structures associated with AD,38,39 and it is worth noting that high blood glucose levels are a recognized risk factor for AD. The influence of whole milk on the timing of gastric emptying may favor the regulation of blood glucose.40 This could potentially elucidate the protective effect of whole milk on the presence of TH in the entorhinal cortex.
The relationship between dietary fat intake and the heightened risk of AD has been extensively investigated. Prior studies have established a connection between elevated fat and saturated SFA intake with hyperinsulinemia, which, in turn, has been associated with an increased susceptibility to AD.41,42 Consistent with these findings, our results showed that fat consumption resulted in a reduction in SA of the pars opercularis cortex, as well as the TH of the caudal middle frontal and superior frontal cortices. Intriguingly, we also observed an increase in the SA of the inferior temporal, middle temporal, and postcentral cortices. It is noteworthy that ketone bodies, metabolic byproducts of fat metabolism, have been suggested to protect against neuronal loss by preventing apoptosis and necrosis. Consequently, the ketogenic diet has gained interest as a potential therapy for neurodegenerative conditions such as AD.43 This proposition may offer an explanation for the observed increase in SA within specific brain regions as identified in our study.
Fresh fruits are key components of the MeDi, known for their rich antioxidants and vitamins. These antioxidants and anti-inflammatory polyphenols in fruits and vegetables are believed to have anti-aging effects.44 Recent research has indicated that the consumption of fruits rich in vitamin C, geraniol, anthocyanins, and total flavonoids, such as strawberries, may lower the risk of AD.45 Correspondingly, our findings support this hypothesis by showing an increase in cortical TH of the insula associated with fresh fruit consumption.
Limited research exists regarding the role of protein in the diet of individuals with AD. Some researchers propose that a sufficient lifelong protein supply may have preventive effects against AD.46,47 Additionally, the right frontal pole has emerged as a significant predictor of prodromal symptoms in AD patients.48 Contrary to expectations, our findings reveal a reduction in the cortical TH of the frontal pole associated with protein consumption. Notably, an animal study demonstrated that AD mice fed a high-protein/low-carbohydrate diet exhibited lower brain weight compared to those on a standard diet,49 aligning with our results. Nonetheless, further investigation is required to determine the implications of these findings on the aging human brain.
Our study revealed that a higher daily water consumption is associated with an increase in the cortical SA of the lateral occipital region. However, despite numerous studies on temperature,50 lithium,51 and aluminum52 content in drinking water in relation to AD, there is currently a lack of research exploring the correlation between water intake volume and AD.
In our reverse MR analysis, we observed that an increase in the cortical TH of the cACC was associated with higher carbohydrate intake and reduced alcohol consumption. The ACC plays a pivotal role in the brain's reward circuitry. Research has shown that foods rich in sugar and fat can enhance the brain's response to food and associative learning independent of food cues or rewards.53 This suggests that frequent consumption of sugary foods may lead to changes in brain activity, reinforcing a preference for such foods. Additionally, alcohol addiction progresses through various stages, with positive reinforcement being characteristic of early-stage addiction and later stages involving negative reinforcement.54,55 Alcohol and certain opioid drugs can reduce mesolimbic dopamine signaling in the midbrain after dependence and cessation, resulting in a loss of reward and pleasure.56–58 The midbrain-limbic reward pathway has been targeted in addiction treatment with some success.59 This may explain the observed increase in ACC cortical TH in the reward circuitry and the subsequent reduction in alcohol consumption. Nevertheless, further research is required to fully comprehend the complex relationship between ACC cortical TH, carbohydrate intake, and alcohol consumption.
An increase in cortical TH in the lateral occipital and lateral OFC is linked to a decrease in coffee consumption. The OFC receives information related to the taste, smell, visual, and textural properties of food.60 Additionally, the OFC handles various forms of reward-related data, including social cues,61 while the lateral OFC plays a role in processing aversive and subjectively unpleasant stimuli.62 A study has demonstrated that a larger SA of the lateral OFC reliably predicts reduced indulgence in calorie-dense foods.63 Similarly, considering the involvement of the lateral OFC in modulating behavior during punishment or non-rewarding stimuli,61,62 we speculate that the decrease in caffeine intake may be due to the greater sensitivity of the larger lateral OFC cortical TH to the non-rewarding attributes of caffeine. The lateral occipital cortex is responsible for the processing and analysis of visual information. Research has indicated that brain regions involved in prioritizing visual processing, reward, and sensory experience, including the lateral occipital cortex, exhibit differential blood oxygen level dependent activity based on the energy density of food, with higher reactivity to high-energy density foods compared to low-energy density foods.64 Coffee, being a low-energy density food, may not elicit significant sensitivity in the lateral occipital cortex, which appears to be more responsive to high-energy density foods.
Finally, we observed that an increase in the cortical SA of the medial OFC is associated with a decrease in the consumption of non-oily fish, while an increase in the cortical TH of the paracentral region is associated with a decrease in the consumption of oily fish. Omega-3 fatty acids, an important component of oily fish, have been found to improve brain function and slow the progression of dementia.65 However, there is currently no research available on the association between the cortical structure of the medial orbitofrontal and paracentral regions and omega-3 fatty acids.
Our study provides evidence suggesting that dietary factors can influence the TH or SA of specific brain regions. However, the underlying mechanisms remain unclear. Increasing evidence suggests a close association between neurological and psychiatric disorders and disturbances in gut microbial composition, leading to the formulation of the microbe-gut–brain axis concept. This concept posits that the gut microbiota may participate in neuroendocrine-immune regulation, influencing neuropsychiatric disorders.66 Importantly, dietary patterns have been shown to alter gut microbiota composition,67 and the interplay between environmental risk factors associated with cognitive impairment can shape the gut microbiota's species composition. Furthermore, certain dietary and hypoxic stress-responsive bacteria derived from the gut microbiota may disrupt hippocampal function and cognition.68 it is plausible that the microbial-gut–brain axis serves as one of the mechanisms through which diet impacts cerebral cortex structure. However, the precise biological mechanisms involved in this process warrant further investigation.
This study has several limitations. Firstly, the participants we selected were all of European descent, so the causal relationship between dietary factors and cortical structure in other populations remains unclear. Secondly, in the reverse MR analysis, we used a significance threshold of P < 5 × 10−6 to select IVs, which may include false positive variants and introduce bias. However, when we used a more stringent significance threshold of P < 5 × 10−8, several food items did not have available IVs, and these unselected SNPs may also be associated with the outcomes of interest. Moreover, previous studies have successfully identified bidirectional causal relationships between adult physical activity and depression using more lenient P-values (P < 1 × 10−6).69 Therefore, in our reverse MR analysis, we adopted a more lenient threshold to identify potential associations between cortical structure and dietary factors. Finally, although our study provides some evidence for the causal relationship between dietary factors and cortical structure, the detailed biological mechanisms underlying these relationships still require further investigation.
Conclusions
In conclusion, this two-sample MR study revealed causal relationships between certain dietary habits and cortical TH or SA in the brain. Further research is needed to elucidate the impact of dietary habits on cortical structure, including the specific mechanisms involved. This study contributes to further elucidating the potential mechanisms by which dietary factors play a role in cognitive function changes, and provides evidence for the existence of the gut-brain axis.
Author contributions
Cong Li: investigation, methodology, validation, writing–original-draft, writing–review & editing. Zhe Chen: investigation, validation, methodology. Shaqi He: investigation, methodology, conceptualization. Yanjing Chen: investigation, conceptualization. Jun Liu: project administration, funding acquisition, supervision. All authors have read and approved the final version of the manuscript.
Conflicts of interest
All authors have no conflicts of interest to declare.
Acknowledgements
This research was funded by grants from the Innovative Province Special Construction Foundation of Hunan Province (2020SK4001). This study is based on data provided by the DietGen consortium, SSGAC19 consortium, ENIGMA consortium. We thank the researchers of the previous study who provided valuable genetic summary statistics for this study. The Fig. 1 is made with BioRender.
References
- . CDC. Data Brief - No. 456 - Month <> Year. 2022. https://www.cdc.gov/nchs/products/databriefs/db456.htm, (accessed April 25, 2023).
- J. Hugo and M. Ganguli, Dementia and Cognitive Impairment, Clin. Geriatr. Med., 2014, 30, 421–442 CrossRef.
- S. Srivastava, R. Ahmad and S. K. Khare, Alzheimer's disease and its treatment by different approaches: A review, Eur. J. Med. Chem., 2021, 216, 113320 CrossRef CAS PubMed.
- M. Guasch-Ferré and W. C. Willett, The Mediterranean diet and health: a comprehensive overview, J. Intern. Med., 2021, 290, 549–566 CrossRef PubMed.
- L. L. Barnes, K. Dhana, X. Liu, V. J. Carey, J. Ventrelle, K. Johnson, C. S. Hollings, L. Bishop, N. Laranjo, B. J. Stubbs, X. Reilly, P. Agarwal, S. Zhang, F. Grodstein, C. C. Tangney, T. M. Holland, N. T. Aggarwal, K. Arfanakis, M. C. Morris and F. M. Sacks, Trial of the MIND Diet for Prevention of Cognitive Decline in Older Persons, N. Engl. J. Med., 2023, 389, 602–611 CrossRef CAS PubMed.
- H. J. Coelho-Júnior, A. Trichopoulou and F. Panza, Cross-sectional and longitudinal associations between adherence to Mediterranean diet with physical performance and cognitive function in older adults: A systematic review and meta-analysis, Ageing Res. Rev., 2021, 70, 101395 CrossRef PubMed.
- D. E. Hosking, R. Eramudugolla, N. Cherbuin and K. J. Anstey, MIND not Mediterranean diet related to 12−year incidence of cognitive impairment in an Australian longitudinal cohort study, Alzheimer's Dementia, 2019, 15, 581–589 CrossRef PubMed.
- X. Shang, E. Hill, Y. Li and M. He, Energy and macronutrient intakes at breakfast and cognitive declines in community-dwelling older adults: a 9-year follow-up cohort study, Am. J. Clin. Nutr., 2021, 113, 1093–1103 CrossRef PubMed.
- H. Shi, X. Ge, X. Ma, M. Zheng, X. Cui, W. Pan, P. Zheng, X. Yang, P. Zhang, M. Hu, T. Hu, R. Tang, K. Zheng, X.-F. Huang and Y. Yu, A fiber-deprived diet causes cognitive impairment and hippocampal microglia-mediated synaptic loss through the gut microbiota and metabolites, Microbiome, 2021, 9, 223 CrossRef CAS PubMed.
- Y. Gu, A. M. Brickman, Y. Stern, C. G. Habeck, Q. R. Razlighi, J. A. Luchsinger, J. J. Manly, N. Schupf, R. Mayeux and N. Scarmeas, Mediterranean diet and brain structure in a multiethnic elderly cohort, Neurology, 2015, 85, 1744–1751 CrossRef CAS PubMed.
- S. C. Staubo, J. A. Aakre, P. Vemuri, J. A. Syrjanen, M. M. Mielke, Y. E. Geda, W. K. Kremers, M. M. Machulda, D. S. Knopman, R. C. Petersen, C. R. Jack, Jr. and R. O. Roberts, Mediterranean diet, micronutrients and macronutrients, and MRI measures of cortical thickness, Alzheimer's Dementia, 2017, 13, 168–177 CrossRef PubMed.
- O. P. Almeida, G. J. Hankey, B. B. Yeap, J. Golledge and L. Flicker, Alcohol consumption and cognitive impairment in older men: A mendelian randomization study, Neurology, 2014, 82, 1038–1044 CrossRef CAS PubMed.
- A. Zhou, A. E. Taylor, V. Karhunen, Y. Zhan, S. P. Rovio, J. Lahti, P. Sjögren, L. Byberg, D. M. Lyall, J. Auvinen, T. Lehtimäki, M. Kähönen, N. Hutri-Kähönen, M. M. Perälä, K. Michaëlsson, A. Mahajan, L. Lind, C. Power, J. G. Eriksson, O. T. Raitakari, S. Hägg, N. L. Pedersen, J. Veijola, M.-R. Järvelin, M. R. Munafò, E. Ingelsson, D. J. Llewellyn and E. Hyppönen, Habitual coffee consumption and cognitive function: a Mendelian randomization meta-analysis in up to 415,530 participants, Sci. Rep., 2018, 8, 7526 CrossRef PubMed.
- J. Kang, T. Jia, Z. Jiao, C. Shen, C. Xie, W. Cheng, B. J. Sahakian, D. Waxman and J. Feng, Increased brain volume from higher cereal and lower coffee intake: shared genetic determinants and impacts on cognition and metabolism, Cereb. Cortex, 2022, 32, 5163–5174 CrossRef PubMed.
- J. Luo, S. le Cessie, G. J. Blauw, C. Franceschi, R. Noordam and D. van Heemst, Systemic inflammatory markers in relation to cognitive function and measures of brain atrophy: a Mendelian randomization study, GeroScience, 2022, 44, 2259–2270 CrossRef CAS PubMed.
- D. J. Kiltschewskij, W. R. Reay and M. J. Cairns, Evidence of genetic overlap and causal relationships between blood-based biochemical traits and human cortical anatomy, Transl. Psychiatry, 2022, 12, 373 CrossRef CAS PubMed.
- J. B. Cole, J. C. Florez and J. N. Hirschhorn, Comprehensive genomic analysis of dietary habits in UK Biobank identifies hundreds of genetic associations, Nat. Commun., 2020, 11, 1467 CrossRef CAS PubMed.
- S. F. W. Meddens, R. de Vlaming, P. Bowers, C. A. P. Burik, R. K. Linnér, C. Lee, A. Okbay, P. Turley, C. A. Rietveld, M. A. Fontana, M. Ghanbari, F. Imamura, G. McMahon, P. J. van der Most, T. Voortman, K. H. Wade, E. L. Anderson, K. V. E. Braun, P. M. Emmett, T. Esko, J. R. Gonzalez, J. C. Kiefte-de Jong, C. Langenberg, J. a. Luan, T. Muka, S. Ring, F. Rivadeneira, H. Snieder, F. J. A. van Rooij, B. H. R. Wolffenbuttel, G. D. Smith, O. H. Franco, N. G. Forouhi, M. A. Ikram, A. G. Uitterlinden, J. V. van Vliet-Ostaptchouk, N. J. Wareham, D. Cesarini, K. P. Harden, J. J. Lee, D. J. Benjamin, C. C. Chow and P. D. Koellinger, Genomic analysis of diet composition finds novel loci and associations with health and lifestyle, Mol. Psychiatry, 2020, 26, 2056–2069 CrossRef PubMed.
- K. L. Grasby, N. Jahanshad, J. N. Painter, L. Colodro-Conde, J. Bralten, D. P. Hibar, P. A. Lind, F. Pizzagalli, C. R. K. Ching, M. A. B. McMahon, N. Shatokhina, L. C. P. Zsembik, S. I. Thomopoulos, A. H. Zhu, L. T. Strike, I. Agartz, S. Alhusaini, M. A. A. Almeida, D. Alnæs, I. K. Amlien, M. Andersson, T. Ard, N. J. Armstrong, A. Ashley-Koch, J. R. Atkins, M. Bernard, R. M. Brouwer, E. E. L. Buimer, R. Bülow, C. Bürger, D. M. Cannon, M. Chakravarty, Q. Chen, J. W. Cheung, B. Couvy-Duchesne, A. M. Dale, S. Dalvie, T. K. de Araujo, G. I. de Zubicaray, S. M. C. de Zwarte, A. den Braber, N. T. Doan, K. Dohm, S. Ehrlich, H.-R. Engelbrecht, S. Erk, C. C. Fan, I. O. Fedko, S. F. Foley, J. M. Ford, M. Fukunaga, M. E. Garrett, T. Ge, S. Giddaluru, A. L. Goldman, M. J. Green, N. A. Groenewold, D. Grotegerd, T. P. Gurholt, B. A. Gutman, N. K. Hansell, M. A. Harris, M. B. Harrison, C. C. Haswell, M. Hauser, S. Herms, D. J. Heslenfeld, N. F. Ho, D. Hoehn, P. Hoffmann, L. Holleran, M. Hoogman, J.-J. Hottenga, M. Ikeda, D. Janowitz, I. E. Jansen, T. Jia, C. Jockwitz, R. Kanai, S. Karama, D. Kasperaviciute, T. Kaufmann, S. Kelly, M. Kikuchi, M. Klein, M. Knapp, A. R. Knodt, B. Krämer, M. Lam, T. M. Lancaster, P. H. Lee, T. A. Lett, L. B. Lewis, I. Lopes-Cendes, M. Luciano, F. Macciardi, A. F. Marquand, S. R. Mathias, T. R. Melzer, Y. Milaneschi, N. Mirza-Schreiber, J. C. V. Moreira, T. W. Mühleisen, B. Müller-Myhsok, P. Najt, S. Nakahara, K. Nho, L. M. Olde Loohuis, D. P. Orfanos, J. F. Pearson, T. L. Pitcher, B. Pütz, Y. Quidé, A. Ragothaman, F. M. Rashid, W. R. Reay, R. Redlich, C. S. Reinbold, J. Repple, G. Richard, B. C. Riedel, S. L. Risacher, C. S. Rocha, N. R. Mota, L. Salminen, A. Saremi, A. J. Saykin, F. Schlag, L. Schmaal, P. R. Schofield, R. Secolin, C. Y. Shapland, L. Shen, J. Shin, E. Shumskaya, I. E. Sønderby, E. Sprooten, K. E. Tansey, A. Teumer, A. Thalamuthu, D. Tordesillas-Gutiérrez, J. A. Turner, A. Uhlmann, C. L. Vallerga, D. van der Meer, M. M. J. van Donkelaar, L. van Eijk, T. G. M. van Erp, N. E. M. van Haren, D. van Rooij, M.-J. van Tol, J. H. Veldink, E. Verhoef, E. Walton, M. Wang, Y. Wang, J. M. Wardlaw, W. Wen, L. T. Westlye, C. D. Whelan, S. H. Witt, K. Wittfeld, C. Wolf, T. Wolfers, J. Q. Wu, C. L. Yasuda, D. Zaremba, Z. Zhang, M. P. Zwiers, E. Artiges, A. A. Assareh, R. Ayesa-Arriola, A. Belger, C. L. Brandt, G. G. Brown, S. Cichon, J. E. Curran, G. E. Davies, F. Degenhardt, M. F. Dennis, B. Dietsche, S. Djurovic, C. P. Doherty, R. Espiritu, D. Garijo, Y. Gil, P. A. Gowland, R. C. Green, A. N. Häusler, W. Heindel, B.-C. Ho, W. U. Hoffmann, F. Holsboer, G. Homuth, N. Hosten, C. R. Jack, M. Jang, A. Jansen, N. A. Kimbrel, K. Kolskår, S. Koops, A. Krug, K. O. Lim, J. J. Luykx, D. H. Mathalon, K. A. Mather, V. S. Mattay, S. Matthews, J. Mayoral Van Son, S. C. McEwen, I. Melle, D. W. Morris, B. A. Mueller, M. Nauck, J. E. Nordvik, M. M. Nöthen, D. S. O’Leary, N. Opel, M.-L. P. Martinot, G. B. Pike, A. Preda, E. B. Quinlan, P. E. Rasser, V. Ratnakar, S. Reppermund, V. M. Steen, P. A. Tooney, F. R. Torres, D. J. Veltman, J. T. Voyvodic, R. Whelan, T. White, H. Yamamori, H. H. H. Adams, J. C. Bis, S. Debette, C. Decarli, M. Fornage, V. Gudnason, E. Hofer, M. A. Ikram, L. Launer, W. T. Longstreth, O. L. Lopez, B. Mazoyer, T. H. Mosley, G. V. Roshchupkin, C. L. Satizabal, R. Schmidt, S. Seshadri, Q. Yang, M. K. M. Alvim, D. Ames, T. J. Anderson, O. A. Andreassen, A. Arias-Vasquez, M. E. Bastin, B. T. Baune, J. C. Beckham, J. Blangero, D. I. Boomsma, H. Brodaty, H. G. Brunner, R. L. Buckner, J. K. Buitelaar, J. R. Bustillo, W. Cahn, M. J. Cairns, V. Calhoun, V. J. Carr, X. Caseras, S. Caspers, G. L. Cavalleri, F. Cendes, A. Corvin, B. Crespo-Facorro, J. C. Dalrymple-Alford, U. Dannlowski, E. J. C. de Geus, I. J. Deary, N. Delanty, C. Depondt, S. Desrivières, G. Donohoe, T. Espeseth, G. Fernández, S. E. Fisher, H. Flor, A. J. Forstner, C. Francks, B. Franke, D. C. Glahn, R. L. Gollub, H. J. Grabe, O. Gruber, A. K. Håberg, A. R. Hariri, C. A. Hartman, R. Hashimoto, A. Heinz, F. A. Henskens, M. H. J. Hillegers, P. J. Hoekstra, A. J. Holmes, L. E. Hong, W. D. Hopkins, H. E. Hulshoff Pol, T. L. Jernigan, E. G. Jönsson, R. S. Kahn, M. A. Kennedy, T. T. J. Kircher, P. Kochunov, J. B. J. Kwok, S. Le Hellard, C. M. Loughland, N. G. Martin, J.-L. Martinot, C. McDonald, K. L. McMahon, A. Meyer-Lindenberg, P. T. Michie, R. A. Morey, B. Mowry, L. Nyberg, J. Oosterlaan, R. A. Ophoff, C. Pantelis, T. Paus, Z. Pausova, B. W. J. H. Penninx, T. J. C. Polderman, D. Posthuma, M. Rietschel, J. L. Roffman, L. M. Rowland, P. S. Sachdev, P. G. Sämann, U. Schall, G. Schumann, R. J. Scott, K. Sim, S. M. Sisodiya, J. W. Smoller, I. E. Sommer, B. St Pourcain, D. J. Stein, A. W. Toga, J. N. Trollor, N. J. A. Van der Wee, D. van ‘t Ent, H. Völzke, H. Walter, B. Weber, D. R. Weinberger, M. J. Wright, J. Zhou, J. L. Stein, P. M. Thompson and S. E. Medland, The genetic architecture of the human cerebral cortex, Science, 2020, 367, 1340 CrossRef PubMed.
- R. S. Desikan, F. Ségonne, B. Fischl, B. T. Quinn, B. C. Dickerson, D. Blacker, R. L. Buckner, A. M. Dale, R. P. Maguire, B. T. Hyman, M. S. Albert and R. J. Killiany, An automated labeling system for subdividing the human cerebral cortex on MRI scans into gyral based regions of interest, NeuroImage, 2006, 31, 968–980 CrossRef PubMed.
- S. Burgess, F. Dudbridge and S. G. Thompson, Combining information on multiple instrumental variables in Mendelian randomization: comparison of allele score and summarized data methods, Stat Med., 2015, 35, 1880–1906 CrossRef.
- J. Bowden, G. Davey Smith and S. Burgess, Mendelian randomization with invalid instruments: effect estimation and bias detection through Egger regression, Int. J. Epidemiol., 2015, 44, 512–525 CrossRef PubMed.
- M. Verbanck, C.-Y. Chen, B. Neale and R. Do, Detection of widespread horizontal pleiotropy in causal relationships inferred from Mendelian randomization between complex traits and diseases, Nat. Genet., 2018, 50, 693–698 CrossRef CAS PubMed.
-
S. Burgess and S. G. Thompson, Mendelian Randomization: Methods for Causal Inference Using Genetic Variants (2nd ed.), Chapman and Hall/CRC, 2021 Search PubMed.
- M. S. Mohan, T. F. O'Callaghan, P. Kelly and S. A. Hogan, Milk fat: opportunities, challenges and innovation, Crit. Rev. Food Sci. Nutr., 2020, 61, 2411–2443 CrossRef PubMed.
- M. Dehghan, A. Mente, S. Rangarajan, P. Sheridan, V. Mohan, R. Iqbal, R. Gupta, S. Lear, E. Wentzel-Viljoen, A. Avezum, P. Lopez-Jaramillo, P. Mony, R. P. Varma, R. Kumar, J. Chifamba, K. F. Alhabib, N. Mohammadifard, A. Oguz, F. Lanas, D. Rozanska, K. B. Bostrom, K. Yusoff, L. P. Tsolkile, A. Dans, A. Yusufali, A. Orlandini, P. Poirier, R. Khatib, B. Hu, L. Wei, L. Yin, A. Deeraili, K. Yeates, R. Yusuf, N. Ismail, D. Mozaffarian, K. Teo, S. S. Anand and S. Yusuf, Association of dairy intake with cardiovascular disease and mortality in 21 countries from five continents (PURE): a prospective cohort study, Lancet, 2018, 392, 2288–2297 CrossRef.
- M. Chen, Y. Li, Q. Sun, A. Pan, J. E. Manson, K. M. Rexrode, W. C. Willett, E. B. Rimm and F. B. Hu, Dairy fat and risk of cardiovascular disease in 3 cohorts of US adults, Am. J. Clin. Nutr., 2016, 104, 1209–1217 CrossRef CAS PubMed.
- V. Pala, S. Sieri, P. Chiodini, G. Masala, D. Palli, A. Mattiello, S. Panico, R. Tumino, G. Frasca, F. Fasanelli, F. Ricceri, C. Agnoli, S. Grioni and V. Krogh, Associations of dairy product consumption with mortality in the European Prospective Investigation into Cancer and Nutrition (EPIC)–Italy cohort, Am. J. Clin. Nutr., 2019, 110, 1220–1230 CrossRef PubMed.
- Y. Gu, J. W. Nieves, Y. Stern, J. A. Luchsinger and N. Scarmeas, Food combination and Alzheimer disease risk: a protective diet, Arch. Neurol., 2010, 67, 699–706 CrossRef PubMed.
- D. A. Gusnard and M. E. Raichle, Searching for a baseline: Functional imaging and the resting human brain, Nat. Rev. Neurosci., 2001, 2, 685–694 CrossRef CAS PubMed.
- R. L. Buckner, J. Sepulcre, T. Talukdar, F. M. Krienen, H. Liu, T. Hedden, J. R. Andrews-Hanna, R. A. Sperling and K. A. Johnson, Cortical Hubs Revealed by Intrinsic Functional Connectivity: Mapping, Assessment of Stability, and Relation to Alzheimer's Disease, J. Neurosci., 2009, 29, 1860–1873 CrossRef CAS PubMed.
- W. E. Klunk, H. Engler, A. Nordberg, Y. Wang, G. Blomqvist, D. P. Holt, M. Bergström, I. Savitcheva, G.-F. Huang, S. Estrada, B. Ausén, M. L. Debnath, J. Barletta, J. C. Price, J. Sandell, B. J. Lopresti, A. Wall, P. Koivisto, G. Antoni, C. A. Mathis and B. Långström, Imaging brain amyloid in Alzheimer's disease with Pittsburgh Compound-B, Ann. Neurol., 2004, 55, 306–319 CrossRef CAS PubMed.
- F. Peters, F. Collette, C. Degueldre, V. Sterpenich, S. Majerus and E. Salmon, The neural correlates of verbal short-term memory in Alzheimer's disease: an fMRI study, Brain, 2009, 132, 1833–1846 CrossRef PubMed.
- R. Cacciaglia, J. L. Molinuevo, C. Falcón, A. Brugulat-Serrat, G. Sánchez-Benavides, N. Gramunt, M. Esteller, S. Morán, C. Minguillón, K. Fauria and J. D. Gispert, Effects of APOE–ε4 allele load on brain morphology in a cohort of middle–aged healthy individuals with enriched genetic risk for Alzheimer's disease, Alzheimer's Dementia, 2018, 14, 902–912 CrossRef PubMed.
- L. Chao, F. Blanc, V. Noblet, N. Philippi, B. Cretin, J. Foucher, J.-P. Armspach and F. Rousseau, Right Anterior Insula: Core Region of Hallucinations in Cognitive Neurodegenerative Diseases, PLoS One, 2014, 9, e114774 CrossRef PubMed.
- D. Aarsland, J. L. Cummings, G. Yenner and B. Miller, Relationship of aggressive behavior to other neuropsychiatric symptoms in patients with Alzheimer’s disease, Am. J. Psychiatry, 1996, 153, 243–247 CrossRef CAS PubMed.
- X. Zhu, K. Wang, A. Cao, Y. Zhang and J. Qiu, Personality traits and negative affect mediate the relationship between cortical thickness of superior frontal cortex and aggressive behavior, Neurosci. Lett., 2020, 718, 134728 CrossRef.
- S.-D. Chen, W. Zhang, Y.-Z. Li, L. Yang, Y.-Y. Huang, Y.-T. Deng, B.-S. Wu, J. Suckling, E. T. Rolls, J.-F. Feng, W. Cheng, Q. Dong and J.-T. Yu, A Phenome-wide Association and Mendelian Randomization Study for Alzheimer's Disease: A Prospective Cohort Study of 502,493 Participants From the UK Biobank, Biol. Psychiatry, 2023, 93, 790–801 CrossRef.
- X. Liu, W. Chen, H. Hou, X. Chen, J. Zhang, J. Liu, Z. Guo and G. Bai, Decreased functional connectivity between the dorsal anterior cingulate cortex and lingual gyrus in Alzheimer's disease patients with depression, Behav. Brain Res., 2017, 326, 132–138 CrossRef PubMed.
- K. M. Sanggaard, J. J. Holst, J. F. Rehfeld, B. Sandström, A. Raben and T. Tholstrup, Different effects of whole milk and a fermented milk with the same fat and lactose content on gastric emptying and postprandial lipaemia, but not on glycaemic response and appetite, Br. J. Nutr., 2007, 92, 447–459 CrossRef PubMed.
- J. A. Luchsinger and R. Mayeux, Dietary factors and Alzheimer's disease, Lancet Neurol., 2004, 3, 579–587 CrossRef PubMed.
- J. A. Luchsinger, M. X. Tang, S. Shea and R. Mayeux, Hyperinsulinemia and risk of Alzheimer disease, Neurology, 2004, 63, 1187–1192 CrossRef PubMed.
- M. Rusek, R. Pluta, M. Ułamek-Kozioł and S. J. Czuczwar, Ketogenic Diet in Alzheimer’s Disease, Int. J. Mol. Sci., 2019, 20, 3892 CrossRef CAS PubMed.
- J. A. Joseph, B. Shukitt-Hale and F. C. Lau, Fruit Polyphenols and Their Effects on Neuronal Signaling and Behavior in Senescence, Ann. N. Y. Acad. Sci., 2007, 1100, 470–485 CrossRef CAS PubMed.
- P. Agarwal, T. M. Holland, Y. Wang, D. A. Bennett and M. C. Morris, Association of Strawberries and Anthocyanidin Intake with Alzheimer’s Dementia Risk, Nutrients, 2019, 11, 3060 CrossRef CAS PubMed.
- M. Studnicki, K. J. Dębski and D. Stępkowski, Proportions of macronutrients, including specific dietary fats, in prospective anti-Alzheimer’s diet, Sci. Rep., 2019, 9, 20143 CrossRef CAS PubMed.
- H. J. Leidy, P. M. Clifton, A. Astrup, T. P. Wycherley, M. S. Westerterp-Plantenga, N. D. Luscombe-Marsh, S. C. Woods and R. D. Mattes, The role of protein in weight loss and maintenance, Am. J. Clin. Nutr., 2015, 101, 1320S–1329S CrossRef CAS PubMed.
- E. Finger, J. Zhang, B. Dickerson, Y. Bureau and M. Masellis, Disinhibition in Alzheimer's Disease is Associated with Reduced Right Frontal Pole Cortical Thickness, J. Alzheimer's Dis., 2017, 60, 1161–1170 Search PubMed.
- S. Pedrini, C. Thomas, H. Brautigam, J. Schmeidler, L. Ho, P. Fraser, D. Westaway, P. S. Hyslop, R. N. Martins, J. D. Buxbaum, G. M. Pasinetti, D. L. Dickstein, P. R. Hof, M. E. Ehrlich and S. Gandy, Dietary composition modulates brain mass and solubilizable Aβ levels in a mouse model of aggressive Alzheimer’s amyloid pathology, Mol. Neurodegener., 2009, 4, 40 CrossRef PubMed.
- J.-p. Wei, W. Wen, Y. Dai, L.-x. Qin, Y.-q. Wen, D. D. Duan and S.-j. Xu, Drinking water temperature affects cognitive function and progression of Alzheimer's disease in a mouse model, Acta Pharmacol. Sin., 2020, 42, 45–54 CrossRef PubMed.
- M. Muronaga, T. Terao, K. Kohno, H. Hirakawa, T. Izumi and M. Etoh, Lithium in drinking water and Alzheimer's dementia: Epidemiological Findings from National Data Base of Japan, Bipolar Disord., 2022, 24, 788–794 CrossRef PubMed.
- C. N. Martyn, D. N. Coggon, H. Inskip, R. F. Lacey and W. F. Young, Aluminum concentrations in drinking water and risk of Alzheimer's disease, Epidemiology, 1997, 8, 281–286 CrossRef CAS PubMed.
- S. Edwin Thanarajah, A. G. DiFeliceantonio, K. Albus, B. Kuzmanovic, L. Rigoux, S. Iglesias, R. Hanßen, M. Schlamann, O. A. Cornely, J. C. Brüning, M. Tittgemeyer and D. M. Small, Habitual daily intake of a sweet and fatty snack modulates reward processing in humans, Cell Metab., 2023, 35, 571–584 CrossRef CAS PubMed.
- J. Kramer, D. M. Dick, A. King, L. A. Ray, K. J. Sher, A. Vena, L. F. Vendruscolo and L. Acion, Mechanisms of Alcohol Addiction: Bridging Human and Animal Studies, Alcohol Alcohol., 2020, 55, 603–607 CrossRef CAS PubMed.
- S. Bin Cho, J. N. Su, S. I. C. Kuo, K. K. Bucholz, G. Chan, H. J. Edenberg, V. V. McCutcheon, M. A. Schuckit, J. R. Kramer and D. M. Dick, Positive and negative reinforcement are differentially associated with alcohol consumption as a function of alcohol dependence, Psychol. Addict. Behav., 2019, 33, 58–68 Search PubMed.
- R. A. Wise, Dopamine and reward: The anhedonia hypothesis 30 years on, Neurotoxic. Res., 2008, 14, 169–183 CrossRef PubMed.
- R. A. Wise and G. F. Koob, The Development and Maintenance of Drug Addiction, Neuropsychopharmacology, 2013, 39, 254–262 CrossRef PubMed.
-
D. A. Wiss, in The Assessment and Treatment of Addiction, 2019, pp. 21–42, DOI:10.1016/b978-0-323-54856-4.00002-x.
- M. S. Gold, K. Blum, M. Febo, D. Baron, E. J. Modestino, I. Elman and R. D. Badgaiyan, Molecular role of dopamine in anhedonia linked to reward deficiency syndrome (RDS) and anti- reward systems, Front. Biosci., Scholar Ed., 2018, 10, 309–325 CrossRef PubMed.
- F. Grabenhorst and E. T. Rolls, Value, pleasure and choice in the ventral prefrontal cortex, Trends Cognit. Sci., 2011, 15, 56–67 CrossRef PubMed.
- E. T. Rolls, The functions of the orbitofrontal cortex, Brain Cogn., 2004, 55, 11–29 CrossRef PubMed.
- E. T. Rolls, W. Cheng and J. Feng, The orbitofrontal cortex: reward, emotion and depression, Brain Commun., 2020, 2, 196 CrossRef PubMed.
- P. A. Hall, J. R. Best, J. Danckert, E. A. Beaton and J. A. Lee, Morphometry of the lateral orbitofrontal cortex is associated with eating dispositions in early adolescence: findings from a large population-based study, Soc. Cogn. Affect Neurosci., 2023, 18 DOI:10.1093/scan/nsab084.
- P. Mengotti, F. Foroni and R. I. Rumiati, Neural correlates of the energetic value of food during visual processing and response inhibition, NeuroImage, 2019, 184, 130–139 CrossRef CAS PubMed.
- M. Burckhardt, M. Herke, T. Wustmann, S. Watzke, G. Langer and A. Fink, Omega-3 fatty acids for the treatment of dementia, Cochrane Database Syst. Rev., 2016,(4), CD009002 Search PubMed.
- T. Ge, X. Yao, H. Zhao, W. Yang, X. Zou, F. Peng, B. Li and R. Cui, Gut microbiota and neuropsychiatric disorders: Implications for neuroendocrine-immune regulation, Pharmacol. Res., 2021, 173, 105909 CrossRef CAS PubMed.
- C. A. Olson, H. E. Vuong, J. M. Yano, Q. Y. X. Liang, D. J. Nusbaum and E. Y. Hsiao, The Gut Microbiota Mediates the Anti-Seizure Effects of the Ketogenic Diet, Cell, 2018, 173, 1728–1741 CrossRef CAS PubMed.
- C. A. Olson, A. J. Iñiguez, G. E. Yang, P. Fang, G. N. Pronovost, K. G. Jameson, T. K. Rendon, J. Paramo, J. T. Barlow, R. F. Ismagilov and E. Y. Hsiao, Alterations in the gut microbiota contribute to cognitive impairment induced by the ketogenic diet and hypoxia, Cell Host Microbe, 2021, 29, 1378–1392 CrossRef CAS PubMed.
- K. W. Choi, C. Y. Chen, M. B. Stein, Y. C. Klimentidis, M. J. Wang, K. C. Koenen and J. W. Smoller, Assessment of Bidirectional Relationships Between Physical Activity and Depression Among Adults: A 2-Sample Mendelian Randomization Study, JAMA Psychiatry, 2019, 76, 399–408 CrossRef PubMed.
|
This journal is © The Royal Society of Chemistry 2023 |