DOI:
10.1039/D2LC00611A
(Critical Review)
Lab Chip, 2023,
23, 25-43
Capturing the spatial and temporal dynamics of tumor stroma for on-chip optimization of microenvironmental targeting nanomedicine
Received
6th July 2022
, Accepted 9th October 2022
First published on 11th October 2022
Abstract
Malignant cells grow in a complex microenvironment that plays a key role in cancer progression. The “dynamic reciprocity” existing between cancer cells and their microenvironment is involved in cancer differentiation, proliferation, invasion, metastasis, and drug response. Therefore, understanding the molecular mechanisms underlying the crosstalk between cancer cells and their surrounding tissue (i.e., tumor stroma) and how this interplay affects the disease progression is fundamental to design and validate novel nanotherapeutic approaches. As an important regulator of tumor progression, metastasis and therapy resistance, the extracellular matrix of tumors, the acellular component of the tumor microenvironment, has been identified as very promising target of anticancer treatment, revolutionizing the traditional therapeutic paradigm that sees the neoplastic cells as the preferential objective to fight cancer. To design and to validate such a target therapy, advanced 3D preclinical models are necessary to correctly mimic the complex, dynamic and heterogeneous tumor microenvironment. In addition, the recent advancement in microfluidic technology allows fine-tuning and controlling microenvironmental parameters in tissue-on-chip devices in order to emulate the in vivo conditions. In this review, after a brief description of the origin of tumor microenvironment heterogeneity, some examples of nanomedicine approaches targeting the tumor microenvironment have been reported. Further, how advanced 3D bioengineered tumor models coupled with a microfluidic device can improve the design and testing of anti-cancer nanomedicine targeting the tumor microenvironment has been discussed. We highlight that the presence of a dynamic extracellular matrix, able to capture the spatiotemporal heterogeneity of tumor stroma, is an indispensable requisite for tumor-on-chip model and nanomedicine testing.
1. Introduction
It is widely recognized that a tumor is not a merely a mass of transformed epithelial cells but must be considered as a complete organ in which neoplastic cells coexist and evolve within the extracellular matrix (ECM) together with stromal cells. Despite this awareness, cancer research and therapy has been mainly focused on tumor epithelial cells, even if, more and more frequently, the effect of the tumor microenvironment (TME) has been shown to play a key role in tumor progression and drug resistance.1 The complex and heterogeneous milieu forming the TME is composed of both cellular and non-cellular components which can be further categorized into two main interacting domains: a “stromal part” and a “non-stromal part”. ECM components (collagen, elastin, hyaluronan, glycosaminoglycans, fibronectin, laminin and other molecules), remodeling enzyme metalloproteinases (MMPs), tissue inhibitors of metalloproteinases (TIMPs), lysyl oxidase (LOX) and cells, such as fibroblasts, cancer-associated fibroblasts (CAFs), vascular and immune system-derived cells, compose the stromal part, while tumor cells compose the non-stromal one. Both the parts of the TME can be targets of therapies. As a fundamental component of tumor stroma, ECM is receiving growing interest as a target for anticancer therapy, paving the way for new nanomedicine design criteria.2–4 Increasing data have revealed that nanomedicine is one of the most promising tools in oncology as a therapeutic modality for delivery of chemotherapeutics and/or biologics to solid tumors.5–7 To design nanomedicine to target the composition and biophysical properties of the tumoral ECM may indirectly improve tumor targeting because the unique features of the ECM surrounding the tumor mass can be exploited to deliver drugs, increasing therapeutic efficacy.8–10 In addition, molecules and processes involved in TME modifications occurring over time can be used as design criteria for the development of nano-targeting strategies. These are based on the most relevant TME hallmarks comprising ECM remodeling,7,11 tumor-associated macrophage activation,12,13 immune system escape,14 presence of CAFs,15 hypoxia and acidosis,16 and vascular network remodeling.17 The researchers' community is performing a big effort to develop new therapeutic strategies towards a more efficient targeting of the TME by combining conventional therapies and nanomedicines.7,18 However, at present, despite research advancements in the field of nanomedicine and the understanding of the alterations occurring in the TME, the translation of these new therapies into clinical practice is still a long way off the mark.19 This is partly due to the excessive simplicity of the most common method used to validate nanomedicine in vitro, such as 2D cell culture models. These in vitro models do not present the ECM at all and cannot replicate the spatiotemporal TME heterogeneity associated with cancer and the main cause of drug resistance, resulting in oversimplified models for both designing and testing nanomedicine approaches. Therefore, the need arises for reliable 3D in vitro models able to replicate the complexity and heterogeneity of cancer and to control and manipulate microenvironment conditions. In this perspective, tumor-on-chip hosting advanced 3D functional tumor models are emerging as a promising technology for nanomedicine evaluations due to the possibility of assessing with precision the transport and translocation of nanomedicines across the ECM and tissue–tissue interfaces under in vivo-relevant shear flow.
In this review, the spatiotemporal TME heterogeneity is discussed by highlighting the crucial but often underestimated role of the ECM in tumor dynamics and drug resistance. As a consequence, the need to replicate such complexity in 3D bioengineered tumor models to validate nanomedicine targeting the TME is critically examined. Finally, how the coupling of advanced 3D bioengineered tumor models with microfluidic tools can improve the design and testing of nanomedicine is summarized to give insight into future developments.
2. The origin of the TME's heterogeneity
The TME is the complex and dynamic context in which tumor formation and growth occur; it presents specific physical and biochemical features involved in regulating neoplastic cell differentiation, proliferation, invasion, and metastasis.20 The TME consists of tumor cells, tumor stromal cells including stromal fibroblasts, endothelial cells and immune cells and the non-cellular components of the ECM composed of several macromolecules including collagen, proteoglycans (i.e. heparan sulfate, versican and hyaluronan) and glycoproteins (i.e. laminin, elastin, fibronectin and tenascin)21 (a graphical representation of the TME is shown in Fig. 1). While in normal tissues, the stroma hinders the tumorigenesis process, in cancer the presence of tumor cells induces critical changes and pathological ECM remodeling to sustain disease development.22,23 These changes include fibroblast recruitment, immunocyte migration, interstitial matrix remodeling, development of tumor-specific vasculature, and aberrant epigenetic landscape. All these processes are guided by the dynamic and bidirectional interaction between cancer cells and the surrounding environment and are mediated by soluble molecules, factors and vesicles responsible for the transfer of signaling information between cellular and non-cellular communicating entities.21 The complex ECM remodeling process operated by cancer cells during tumor formation and progression induces change in concentration, structure and organization of individual ECM components, making the 3D spatial topology of the matrix around cells and its biochemical and biophysical properties strongly heterogeneous. Such TME heterogeneity is one of the main causes of multidrug resistance and therefore represents a key challenge in developing effective cancer therapy.5 Indeed, intratumoral heterogeneity, such as the spatiotemporal heterogeneity existing among the cancer cells of a single patient, drives the evolution of cancer and fosters drug resistance.24 The level of intratumoral heterogeneity strongly affect the patient's clinical outcomes.25 Recently, it is becoming clearer that the tumor heterogeneity is not linked only to genetic changes but involves transcriptomic, epigenetic, and/or phenotypic modifications.25,26 At the same time, the TME's regional heterogeneity in the hypoxia, acidity, vasculature organization and cytokines actively drive the tumorigenesis process and tumor heterogeneity. Arguably, distinct environmental scenarios within a given tumor, can select mutations that cause survival and expansion, thereby creating tumor cell heterogeneity.23 Additionally, the administration of therapy can result in selective pressure towards subpopulations with acquired resistance mechanisms. As a consequence, non-transformed cell types (i.e. fibroblasts, endothelial cells, immune cells etc.) within the TME co-evolve with the tumor cells continuously participating in the process of tumorigenesis and in TME heterogeneity.
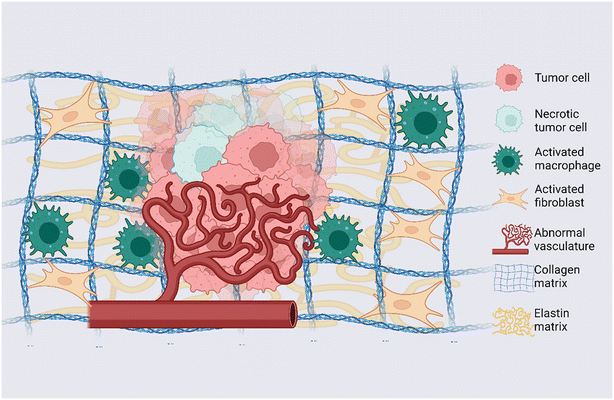 |
| Fig. 1 Dynamic TME: graphical representation of main elements and alterations observed in the TME. Tumor cells, necrotic tumor cells, activated macrophages, activated fibroblasts, and endothelial cells arranged into an abnormal vascular network represent the cellular counterpart of the TME; collagen and elastin are representative of the ECM of the TME. Created with https://BioRender.com. | |
2.1. The role of CAF, endothelial and immune cells in TME heterogeneity
CAFs, endothelial cells and immune cells are among the main cellular components of the TME that influence microenvironment heterogeneity and contribute to tumor stroma-dependent resistance to therapies.23 CAFs are the most abundant stromal cell type in the TME, and compared with normal fibroblasts, present enhanced proliferation, increased ECM synthesis and specific cytokine secretion including, for example, vascular endothelial growth factor (VEGF), platelet-derived growth factor (PDGF), and stromal cell-derived factor-1 (SDF-1). The role of CAFs in tumor progression is widely recognized, and recently, there is a growing interest also in investigating heterogeneity in CAFs as a source of TME heterogeneity. Phenotypic and functional heterogeneity involves both fibroblasts and CAFs and could be ascribable not only to damaged signals, e.g. tumor growth factor-β (TGF-b), fibroblast growth factor (FGF) and PDGF, to which fibroblasts are exposed, but also to the heterogeneous CAF's origin.23 Indeed, the main source of CAFs in the TME is represented by the resident normal fibroblasts which, as a result of tumor cell growth factor synthesis, are converted to CAFs. However, CAFs are found to originate also from other sources including mesenchymal stem cells recruited at the tumor site that differentiate toward CAFs, epithelial cells that undergo the process of epithelial-to-mesenchymal transition, pericytes and endothelial cells that under the effect of tumor secreted factors can transdifferentiate and contribute to the CAF population. All these sources of CAFs are not mutually exclusive and contribute to their heterogeneity within individual cancer types.27 CAFs secrete and remodel the ECM to support tumor growth and, due to their phenotypic heterogeneity, can activate several mechanisms, spatially and temporally regulated, independent of ECM remodeling, to support tumor growth. The result is a highly heterogeneous TME in terms of ECM composition and organization. However, these ECM alterations are pathology-specific and represent a very promising therapeutic target by using nanobody technology.22 For example, desmoplasia, a pathological alteration of ECM featured by increased deposition of fibronectin, hyaluronic acid and tenascin-C, is a key characteristic of specific cancers such as breast cancer and pancreatic ductal adenocarcinoma (PDAC).22 Analyzing the complex modification occurring in the ECM should be beneficial for early cancer detection. Unique ECM signatures that are characteristic of some tumors undergo alterations during cancer progression and are predictive of clinical outcomes.22 The modifications occurring in the vascular network of the TME are a further source of intratumor heterogeneity. The vascular network is a dynamic structure that presents aberrant features in the TME such as uneven vascularization, differences in vascular maturity, lack of drainage due to poor lymphatic vessel coverage and variable interstitial pressure. As a result, tumor vasculature presents inadequate function and is responsible for hypoxia and limited nutrient supply. All these aberrations in the vascular network can generate distinct micro-environments within the tumor and contribute to inter-/intratumor heterogeneity influencing in turn the clinical outcome.23 In general, hypoxia is a key driver for angiogenesis. The latter, however, can be promoted and/or inhibited by different ECM components. For example, periostin has proangiogenic functions, and tenascin-C and fibronectin have both pro- and antigenic functions depending on the context.22 In addition, the architecture of dense collagen in tumors triggers the formation of interconnected networks of cancer cells. These tumor cells activate a process called vasculogenic mimicry, the formation of ECM-rich vasculature-like structures able to sustain the tumor with blood without inducing angiogenesis.22 Vasculogenic mimicry is associated with an aggressive tumor phenotype and promotes metastasis.28 The underlying mechanisms of how the biophysical and biochemical properties of the ECM induce phenotypical changes in tumor cells leading to the formation of vascular-like structures are still not clear and highlight the need of further research focused on the spatiotemporal evolution of ECM in the TME. The immune system is also an active component of cancer disease and could affect the clinical outcome and drug resistance. The heterogeneity of the tumor immune system is influenced by various factors including those secreted by CAFs and the extent and the permeability of the vasculature and the tumor cells themselves. In general, the immune system recognizes and protects tissue from damage; nevertheless, cancer cells can activate the immune suppression mechanism to invade undisturbed tissues and organs.23 The immune infiltrate can include multiple cell types having both pro- and antitumor functions. Even within the same lesion the distribution of the immune cells is not uniform, and they can vary in their activation, contributing to the increase of the heterogeneous character of the TME. To summarize, it appears evident that the co-evolution of tumor cells with the ECM, endothelial, stromal and immune cells is at the base of the strong tumor heterogeneity.23
3. Impact of spatiotemporal TME heterogeneity on nanomedicine therapeutic treatment
The growing interest in investigating nanomedicine-based therapeutic approaches, alternatively to systemic administration, resides in the unique advantages that nanomedicine presents in terms of improved protection of the biological activities of the therapeutic agents, prolonged circulation periods in the bloodstream, enhanced permeability and retention effects, and improved tumor-targeting efficiency, among others.6 These advantages have fed the continuous research and development of “nano” approaches, even if at the same time important challenges persist. Specifically, the relationship between the delivery and the efficacy of nanomedicines has proved inconsistent. Indeed, physicochemical considerations in nanomedicine design arise from investigations into the pathophysiology of tumors. However, the identification and characterization of tumor-associated features is not univocally determinate because of tumor heterogeneity.5 Moreover, tumor heterogeneity affects the drug distribution in the tissue and affects tumor response.29,30 The physical and pathophysiological barriers existing in cancer are the result of alterations occurring in the ECM structure and affect the transport of molecules, inducing resistance mechanisms to therapy including nanomedicine-based therapeutic approaches.
As discussed above, the TME is featured by a spatial heterogeneity that can be envisioned as the diversity in the distribution of a given parameter (e.g. CAFs, immune cells, tumor endothelial cells, ECM components: collagens, proteoglycans, fibronectin, elastins, laminins, hyaluronans) into a certain space of the tissue (i.e. around/in the proximity of/far from the tumor mass). In a similar manner, temporal heterogeneity can be estimated as the change in the manifestation of a parameter (e.g. expression, distribution) over time.5 The measurement of tumor spatial diversity should be more prognostic than its quantification alone.31 Delgado San Martin et al. have demonstrated that stromal morphology influences the nanomedicine drug effect using a patient-derived tumor xenograft (PDTX) model.5,32 They quantified the spatial distribution of drug-induced effect in tumors with two stromal morphologies – estuary and tumor island – and found that the response to treatment was different. Their imaging analysis demonstrated that estuary-like tumors, featured by a highly convoluted tumor–stroma interface, with most tumor cells in close proximity to vessels, were 8.8-fold more responsive to liposomal doxorubicin than tumor island-like tumors, which were almost unresponsive to liposomal doxorubicin. The two tumor morphologies were derived from a single donor (mouse) and presented the same microvascular density and percent of stroma; therefore the difference in nanomedicine responsiveness could only be ascribable to the variability in the tumor–stroma interface between the two models. Indeed, whereas in estuary tumors a larger proportion of tumor cells was in closer proximity to the vasculature, thus reducing the distance of drug penetration required to deliver therapeutically effective concentrations to tumor cells, the tumor island morphology presented a high degree of necrosis, suggesting limited oxygenation and perfusion within the tumor. These results highlighted the potential influence of tumor pathophysiology on therapeutic outcomes, suggesting that stromal morphology should be considered to improve the clinical translation and relevance of nanomedicine research.32 Clinical evidence demonstrates that tumor heterogeneity can impact therapeutic efficacy also in a time-dependent manner. Studies on tumor hypoxia heterogeneity, for example, reveal the need of recovering patient biopsies from multiple regions as well as at different time points to assess not only spatial but also temporal heterogeneity to correctly stratify patients.33 Moreover, the impact of time on single and repeated doses of nanomedicine is almost unknown and needs more investigations. Song et al. have evaluated the role of time heterogeneity of the TME in the variability of nanoparticle delivery and efficacy by investigating the interaction of pegylated liposomal doxorubicin (i.e. Doxil) with the TME up to 96 hours after administration and, in comparison, with free doxorubicin.34 They demonstrated that the tumor delivery of Doxil was significantly greater in the basal-like C3-TAg model compared to the claudin-low T11 model; in contrast, no difference between the two murine breast tumor models was found when free-doxorubicin was delivered. To evaluate which tumor-associated factors may contribute to the variable tumor delivery and efficacy of Doxil in breast tumor subtypes, they assessed tumor-associated macrophages (TAMs), collagen, blood and tumor vasculature, and chemokines after treatment with each agent in these mouse models.
They found that TAMs change differently over time in the two tumor models, suggesting a distinct behavior of nanomedicine depending on the model. Despite the higher anti-angiogenic effect observed in the T11 model after Doxil administration, a greater accumulation of nanomedicine was found in the C3-Tag model. These results highlight the importance of characterizing drug–TME interactions over time in evaluating the response to nanotherapy and suggest the need to design ECM-targeting strategies to modify the TME and improve the penetration of anti-cancer molecules.5,34
4. Nanomedicine targeting tumor ECM
The composition, remodeling and specific pathways featuring the tumor ECM are used as a target to localize nanoparticle-containing drugs close to the cancer cells. Once blocked, such nanoparticles can release their cargo locally. The most overexpressed ECM biomolecules in the TME are collagen, hyaluronan, fibronectin, tenascin-C, heparan sulfate, aggrecan, and chondroitin sulfate. Different nanocarriers have been developed to recognize such biomolecules via peptides or antibody recognition.4,5,38 For example, collagen has been targeted by using serum albumin nanoparticles functionalized with collagen binding peptides and used as a drug carrier for delivery of doxorubicin in a subcutaneous MC38 colon cancer mouse model.54 However, when nanoparticles are used to target specific tumor hallmarks, their penetration and accumulation capability in the tumor site remain an issue. The disassembly of the stiff and dense ECM to promote the entering of nanoparticles containing the drugs2,35 has been the first route to promote penetration. To this aim, collagenase and hyaluronidase have been used to induce the degradation of collagen and hyaluronic acid networks, respectively. Collagenase has been injected intravenously prior to the treatment of nanoparticles (liposome/plasmid DNA complex) or conjugated to gold nanocarriers containing the anticancer actives. In all cases, enhanced penetration of nanoparticles has been observed with increased anticancer efficacy.36,37 Provenzano et al. showed that the enzymatic targeting of hyaluronic acid in the tumor stroma increased tumor response to the chemotherapeutic gemcitabine.38 Hyaluronidase conjugated with PEG nanoparticles has also been used in different clinical trials which demonstrated both increased efficacy and penetration in solid tumors.14,39 Although these strategies have been proved to increase the penetration of nanoparticles containing anti-cancer drugs, different side effects have been reported. Firstly, uncontrolled disassembly of the ECM network can favor cancer cell invasion and metastasis; secondly, the formation of HA oligomers has been proved to have tumorigenic features as they interact with cell surface receptors promoting glycolysis, the principal source of energy of cancer cells. Low molecular weight HA signaling through CD44 is responsible for the resistance to cellular stress, promoting tumor development. Recent advances in the understanding of nanocarrier penetration and accumulation have led to more effective design of their physical–chemical properties depending upon their mechanism of action and ECM-related targets. As the first design criterion, both size and shape have been taken into account since they play a crucial role in nanoparticle penetration, as the hindrance offered by dense tumoral ECM, high interstitial pressure and heterogeneity along the TME can seriously compromise nanomedicine efficacy. Wang et al.40 formulated a series of silica nanoparticle (SN-38) micelles with a diameter ranging from 20 to 300 nm. They found that the smallest nanoparticles were able to better penetrate the tumor mass, whilst by using the larger ones, nanoparticle accumulation was enhanced. Based on this observation, the current practice is to design nanoparticles presenting a “large-to-small” transition41 in the presence of specific stimuli encountered in the tumor ECM. In this direction, gelatin-based nanocarriers have been developed having an initial diameter of 100 nm. The cleavage due to the action of MMP2 allowed the degradation of 100 nm nanocarriers and the release of 10 nm gelatin nanocarriers contained in their surface, increasing the penetration capability. Large-to-small transition has also been obtained by exploiting the pH change found in the TME. For instance, polymeric clustered nanoparticles, in which small platinum–poly(amidoamine) (PAMAM) conjugates (PAMAM/Pt) (5 nm) were linked to poly(ethylene glycol)-b-poly(ε-caprolactone) (PEG–PCL), having a diameter of 100 nm, were able to release 5 nm PAMAM/Pt nanoparticles after the rupture of an acid-sensitive linker.41 Particle shape is another important factor controlling the nanoparticle penetration and distribution. Different attempts have been made in this direction42–44 by fabricating nanoparticles in the shape of nanospheres, nanodisks, nanorods, and nanocages. For these studies, Au-based nanosystems with different shapes were used by Black et al.42 in EMT6 tumors; Smith et al. compared single-walled carbon nanotubes versus spherical nanosystems in U87MG brain tumor and LS174T colon cancer;45 peptide-modified pH-sensitive wormlike micelles with a diameter of 20 nm and length of 60 nm were used in a U87MG model.46 In general, linear and semiflexible configuration seems to diffuse better in the interstitial matrix compared with the spherical counterparts, but the penetration and the accumulation strongly depend on the tumor type and stage. Surface modification is another optimization strategy used to improve nanocarrier penetration and accumulation. A general approach relies on decorating the surface of nanosystems with tumor-penetrating peptides such as cyclic Lyp-1, iNGR, and iRGD.47–50 Due to their cationic nature, such peptides have been shown to lead to nonspecific interactions that can be avoided by shielding the tumor-penetrating peptides with ligands that are specifically cleaved by MMPs or in the presence of pH levels found in the TME. Such sensitive peptides have been added to both polymeric micelles and bioinspired lipoproteins.51–53 Other than to increase the penetration and accumulation of drugs, nanoparticles have been designed to target specific tumor ECM components, its related cells (i.e. CAFs) and the local microenvironment as well. Depending upon the nanocarrier cargo, targeting TGF-b led to inhibition of CAF proliferation, local ECM disassembly reduction of collagen, hyaluronic acid synthesis, and versican and tenascin C synthesis. TGF-b has been targeted by peptide self-assembly nanofilament containing losartan (in breast carcinoma), glycolipid-based polymeric micelles containing telmisartan (in breast carcinoma), liposomes containing hydralazine in melanoma and breast cancer. ECM remodeling has also been targeted via modulation of Wnt signaling by using quercetin phosphate-loaded lipid calcium phosphate nanoparticles decorated with a targeting ligand of aminoethylanisamide in bladder cancer xenografts.54 A wider treatment on the materials used for nanocarrier fabrication is out of the scope of this review. As we want to provide a critical focus on the relevance in replicating functional ECMs in vitro that are able to catch those modifications featuring tumor tissues, we invite the readers who want to learn more about nanocarrier science and nanomedicine development to refer to other reviews.18,41,55–57
5. 3D bioengineered approaches to mimic tumoral ECM for nanomedicine testing
Regardless of the recognition principle characterizing the nanocarriers, currently the unique preclinical model able to replicate the complex and dynamic tumoral ECM is the animal model. However, the discrepancy between animals and humans may result in serious misunderstanding of nanomedicine efficacy and toxicity.66 In addition, both ethical and economical concerns strongly discourage the use of animal models, making the development of alternative in vitro models an urgent need. Therefore, bioengineering approaches aiming at replicating the TME including a functional ECM part are expected to be the most promising in vitro models to replace the animal ones. It is well known that the native tumoral ECM is a complex network composed of approximatively 300 bio-macromolecules opportunely arranged depending on body location or tumor grade. Besides its biochemical composition, native ECM undergoes tremendous modifications in concert with tumor progression. This spatiotemporal heterogeneity strongly affects both trafficking and efficacy of nanocarriers due to variations of mesh size and fiber diameters, ECM composition, local alignments, pH, interstitial pressure and local electrical charge.67 To date, different attempts have been made to build up more complex 3D bioengineered models in which the connective part of the TME is integrated. Gupta et al.68 developed a compartmentalized tri-culture system, resembling PDAC, consisting of an inner core containing cancer cells embedded in fibronectin and an outer core containing stellate and endothelial cells embedded in a mix composed of collagen and polyurethane. The authors showed the activation of stellate cells and a change in morphology of collagen fibers resembling desmoplasia. Y. Shinsato et al. conducted studies on different 3D matrices mimicking the TME.69 In particular, HT1080 fibrosarcoma and MDA-MB-231 breast carcinoma cell lines were seeded onto (i) 3D rat tail collagen possessing short, randomly oriented collagen fibrils, (ii) bovine collagen possessing larger fibril bundles and (iii) cell-derived matrices with strongly oriented architecture. In that work it was demonstrated that the context in which cancer cells were inserted strongly affected the migration and persistence of cancer cells. C. Liu et al. used a nanostructured collagen hydrogel with tunable mechanical and architectural properties to create a tumor-like ECM in which human mammary fibroblasts remodeled the surrounding matrix in a biomimetic fashion.70 C. L. Hedegaard et al. have developed a 3D matrix formed by assembling peptide amphiphiles (PAs). Specific epitopes, PA nanofibers, and ECM macromolecules were assembled to culture human ovarian cancer and endothelial and mesenchymal stem cells. The system was validated by using clinically used chemotherapeutics.71 Although such a plethora of 3D in vitro tumor models aimed at mimicking the TME, they present different drawbacks. Firstly, all of them presented an ECM having an exogenous nature (i.e. not synthesized by cells). This poses some doubts about the correct spatial ECM organization, composition and signal presentation to the cells. Secondly, it is not clear if exogenous ECMs are able to modify their compositional, architectural and biophysical properties during cancer progression as the in vivo counterpart does. Finally, to the best of our knowledge, few studies are reported about the use of such models as a validation tool for nanomedicines targeting tumoral ECM hallmarks. As a surrogate of 3D cancer, the spheroid still represents the most used model for nanomedicine testing in vitro. In this direction, Iaccarino et al.72 developed a cross-linked polyelectrolyte nanocapsule based on an oil core and a matrix metalloproteinase-2 (MMP-2)-cleavable shell tested in vitro in two different 3D spheroid models: (i) a healthy model (made of human fibroblasts) and (ii) a tumor model (made of U87 cells). The authors showed that the efficacy of the drug contained in the nanocapsules was higher in the 3D system presenting the higher level of MMPs (i.e., the U87 model). However, the absence of a functional ECM network implied that key phenomena affecting the “nanomedicine journey” such as nanocarrier–ECM interactions and, most importantly, spatiotemporal ECM modifications cannot be replicated and investigated. Further limitations of spheroids have been well highlighted by Netti's group that showed how either fibroblasts or CAFs behave differently depending upon their 3D arrangement. Firstly, Brancato et al.73 established a bioengineering method to produce connective microtissues in which either fibroblasts or CAFs synthesized and assembled their own ECM.26,94,124 Then fibroblasts or CAFs were arranged in the form of both spheroids and microtissues and the biophysical properties (i.e. ECM composition, mechanical stiffness, oxygen consumption rate) in each 3D configuration were evaluated. Spheroids did not show the presence of a functional ECM which was present only in the pericellular regions, therefore presenting a spatial and compositional arrangement different from the native counterparts. On the other hand, microtissues were featured by the presence of the endogenous ECM, allowing a more realistic replication of the in vivo situation in terms of transport properties (i.e., diffusivity) of drugs and tight junction density. By co-culturing MCF-7 cells on such connective microtissues, a complex tumor breast model was produced to investigate the transport and the effectiveness of MMP-sensitive nanocarriers containing anticancer drugs.80 By following the microtissue-based approach, Netti's group replicated several kinds of cancer models such as PDAC, vascularized breast cancer and cervical neoplasia. In the 3D PDAC model, the connective part of bioengineered microtissues was able to display a desmoplastic reaction at molecular, cellular and extracellular levels.58 In the vascularized breast cancer model (Fig. 2A and B), intratumor heterogeneity in terms of ECM rearrangement and vascular network remodeling was correctly replicated.79 In this native environment, a collagen network with heterogeneous architecture surrounded tumor cell clusters with different morphologies (Fig. 2C/F). In fact, in the same microtissue, both randomly distributed (Fig. 2C and D) and highly oriented collagen fibers (Fig. 2E and F) could be identified. The latter configuration was specifically observed around tumor cells arranged in solid strands with tip cells on a side, a shape that in vivo is usually associated with active cell migration and invasion into the stroma (Fig. 2E and F). At the same time, the tip of these clusters pointed the vessels into the 3D microtissue (Fig. 2G).26 Finally, in organotypic cervical neoplasia, the epithelial mesenchymal transition with the associated ECM activation and remodeling was correctly replicated.59,60 These findings suggest that the presence of cell-synthesized ECM in 3D tumor models allows replicating the spatiotemporal variations of the TME, which represent the missing link of the current in vitro tumor model. This may result in great relevance for nanomedicine development, since such a spatiotemporal variation could be used as a design criterion for engineering nanocarriers having dynamic and adaptive features.83,86 Such connective microtissues can also be inserted in a microfluidic platform to build TME-on-chip devices presenting a functional ECM as discussed in the next sections.
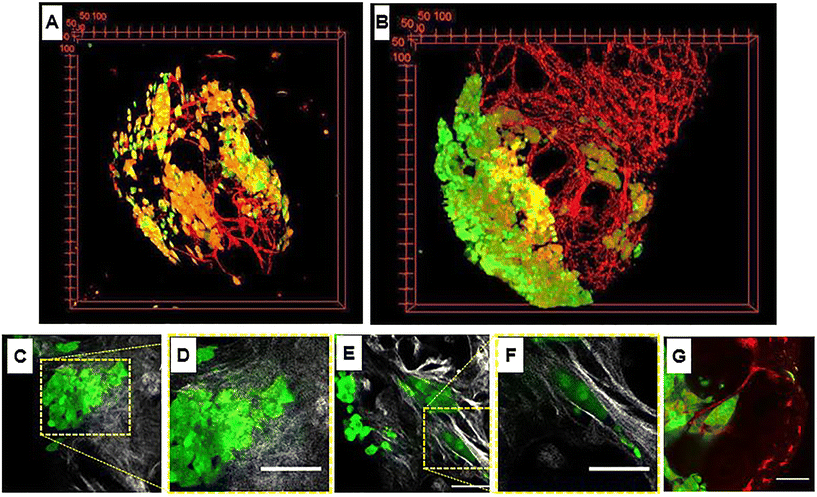 |
| Fig. 2 Heterogeneity in tumor microtissue. (A and B) 3D view of tumor microtissue with breast tumor cells MCF7 in green and endothelial cells (HUVECs) at day 3 and day 10. (C) Image showing random collagen orientation marked by second harmonic generation signal (gray) and tumor cells in green. (D) Magnification of (C). Image showing collagen oriented around tumor cells arranged in solid strands (collagen in gray and tumor cells in green). (F) Magnification of (E). Image of a tumor cell cluster with a tip pointing to the endothelial cell network (tumor cells in green and endothelial cells in red). G) Image showing a cluster of tumor cells arranged as a solid strand with leader cells on a tip (green) and the vascular network (red). Scale bars: 75 μm. Reproduced from ref. 26 with permission from Elsevier, copyright 2018. | |
6. Tumor-on-chip models for nanomedicine testing
6.1. General aims of tumor-on-chip technology
As discussed so far, the bioengineered 3D models used to mimic cancer in its complexity play a pivotal role if one wishes to reproduce the spatial and temporal heterogeneities featuring the TME. In addition, the recreation of specific barriers, spatial organization and fluid dynamic conditions must also be considered to better imitate the intricacy of the in vivo situation.28 Continuous advances in microfluidic, cell culture strategies and tissue engineering lay the foundation to tumor-on-chip technology aiming at (i) mimicking complex biological/chemical/physical interactions occurring in the TME under controlled conditions and (ii) giving reproducible and quantitative information about tumor response to therapeutics.57,61–65 The presence of porous membranes and fluidic channels enables the spatial organization and continuous paracrine communication between tissues in different chip compartments, as does the in vivo situation. At the same time, the dynamic medium supplementation in-flow allows cell feeding, waste disposal, and the delivery of chemical (e.g., small molecules and growth factors) and physical stimuli (e.g., shear stresses and gradients) in a time–space-controlled manner.91,93 Indeed, drug administration on-chip better recapitulates the complexity of in vivo pharmacokinetics thanks to the fluidic time-controlled release of the substances and the presence of multiple cells/tissues involved in drug metabolism.25 Cell culture monitoring on-chip can be obtained thanks to the presence of electric, chemo-, or biosensors, as shown by Weltin et al.,92 who measured pH and oxygen in the cell culture area and placed sensors for lactate and glucose downstream in the microfluidic channel, with the aim to analyze the change in tumor metabolism in culture. Lately, advanced high-throughput platforms have been developed with integrated micropumps for flow control and sensors for real-time monitoring.95 These platforms allow the culture of heterotypic tissues and are compatible with high content screening and RNA sequencing (RNA-seq).96 Generally, microfluidic chips are transparent to guarantee optical access and real-time sample observations. Therefore, microfluidic devices are commonly fabricated by using polydimethylsiloxane (PDMS), which is a biocompatible, gas-permeable, flexible and transparent polymer. Recently, also the use of the poly(methyl methacrylate) (PMMA) is increasing because it overcomes the problem of PDMS hydrophobicity which may hinder the transport of hydrophobic molecules, causing the phenomenon of binding or adsorption and consequently altering drug concentration in solution.25 Nevertheless, PMMA is not as flexible as PDMS and worst, reproduces the mechanical properties of the in vivo microenvironment. At the same time, drug-encapsulating strategies or surface treatments can help to face the problem of PDMS hydrophobicity.97,98 Besides PDMS and PMMA, other biomaterials have also been used for the development of tissue-on-chip, thanks to advancements in 3D printing technologies. As an example, Bertassoni et al.99 developed a network of perfusable microchannels inside methacrylate gelatin (GelMA), star poly(ethylene glycol-co-lactide)acrylate (SPELA), poly(ethylene glycol)di-methacrylate (PEGDMA) and poly(ethylene glycol)diacrylate (PEGDA) hydrogels at different concentrations. Instead, Cheng et al. explored the possibility of using bacterial cellulose for the construction of tissue-on-chip devices. They demonstrated the possibility to obtain microchannel structures in cellulose paper that remain hollow after drying and can be populated by cells.100 Thanks to good mechanical properties, high chemical flexibility, and ability to assemble in nanostructures, the bacterial cellulose may also improve the delivery of drug-encapsulated nanocarriers on-chip, as shown by Di Natale et al.,101 who studied the process of loading and releasing of drug-charged nanocarriers embedded into the cellulose nanonetwork. Nowadays, tumor-on-chip systems represent a tempting tool for the study of cancer progression and therapy keeping into account the peculiar role of the TME which is influenced by and in turn influences tumor cell behavior and drug efficacy.66 Indeed, microfluidic chips allow the study of cell–cell and cell–matrix interaction in a dynamic environment resembling in vivo conditions. Consequently, tumor/microenvironment crosstalk in pathogenesis and drug screening can be replicated on-chip by reproducing the interaction of neoplastic cells with endothelial, fibroblast and immunity cells, all populating the ECM.20 Furthermore, these miniaturized systems offer the chance to high-throughput screen a plethora of molecules and substances, reducing costs and ethical issues and enhancing reproducibility. Tumor-on-chip platforms can be used for evaluating novel nanomedical approaches based on target therapy with the aim to minimize drug toxicity, increase specificity and speed up clinical translation.6 Indeed, tumor-on-chip models should be designed in order to represent all the pathophysiological barriers imposed by the TME to nanoparticle delivery such as vascular and stromal abnormalities and tumor heterogeneity.67 The latter part of the review will show the latest attempts in the literature to replicate TME interactions on-chip and the possibility to use these platforms to evaluate nanomedicine applications targeting the TME. Each paragraph will emphasize a specific element of the tumor stroma, such as vasculature, CAFs and ECM. However, all the presented microfluidic systems present several TME elements, since tumor behavior and drug resistance are the result of the combined action of different entities (multiple cell types, growth factors, ECM components).
6.2. Tumor vasculature-on-chip
Tumor growth and response to treatment are profoundly influenced by vascular phenomena and vice versa. Indeed, the vasculature sustains tumor progression, but it is also the route for the delivery of drugs administered through the systemic blood flow and specifically addressed to the malignant mass. As normal tissues, tumors greater than 1–2 mm in diameter require to be vascularized to guarantee the right intake of oxygen and nutrients and the release of waste products.74 Tumor angiogenesis leads to the formation of a peculiar network of vessels, with a tortuous shape, different diameters, atypical branching pattern, and heterogeneous distribution, with highly vascularized areas and zones of ischemia and necrosis. Furthermore, tumor blood vessels have a thin wall that presents gaps or fenestrae and can be a mosaic of both tumor and endothelial cells. Several pieces of evidence ascribe the formation of such an abnormal vascular network to the loss of balance between positive and negative controls in the TME in favor of pro-angiogenesis,75,76 the so-called angiogenic switch described by Judah Folkman in 1991.77 Tumor vasculature-on-chip can be used not only to study these alterations but also to (i) evaluate the effect of anti-angiogenic substances, pointing to vascular network normalization; (ii) investigate the ability of a drug targeting the tumor to pass across the endothelial barrier; and (iii) analyze the metastatic potential of single/cluster of neoplastic cells through intravasation and extravasation. Tumor metastasis is a process involving multiple phases such as tumor invasion into the ECM, intravasation and migration to secondary sites, extravasation, colonization, and formation of metastatic foci.78 The direction of cell movement during metastasis is not random, as recognized since 1889 by the “seed and soil” hypothesis, but it is driven by factors released and stored in the specific microenvironment of host organs. According to this well-known theory, tumor cells represent the “seed”, whereas the “soil” is the favorable microenvironment able to guarantee the survival and growth of the “seed”.79–81 The presence of multiple compartmentalized chambers and connecting channels on-chip makes possible the reproduction of the complex metastatic cascade in vitro. Chen et al. developed a protocol for replicating tumor cell extravasation through human microcirculation on-chip (Fig. 3). The device presented 7 channels. In the central one a perfusable vascular network was formed after the culture of HUVECs in fibrin gel, while fibroblasts were cultured in two adjacent channels separated from the vascularized one by triangular PDMS microposts spaced 100 μm apart (Fig. 3A). Tumor cells with different metastatic potentials (A375, MCF-10A, SUM-159, MDA-MB-231_LM2, PC-3) were flowed into the vascular network and tracked during extravasation by high-resolution confocal microscopy, enabling the detailed observation of tumor–endothelium interaction (Fig. 3B).82 In another work, Cui et al. fabricated a multilayer microfluidic extravasation device with a 24 μm pore membrane. An endothelial layer was developed on one side of the membrane and the ability of MDA-MB-213 breast cancer cells to migrate across the endothelium was studied. Migrating cells were isolated and characterized and showed a high body aspect ratio, fast planar migration, less consistent stress fiber alignment, and a high nuclear palladin expression (a phosphoprotein that colocalizes with actin-rich structures acting as a molecular scaffold to bundle actin fibers).83 These kinds of microfluidic chips open the understanding of the biological basis of cancer–endothelial cell interaction in cancer progression and may be useful to identify novel strategies for target therapy. Nevertheless, we point out that the study of cancer metastasis and drug screening cannot neglect the role of the ECM which provides structural support to the tissues, acts as a reservoir of growth factors and cytokines, and is an unavoidable path to go for both drugs and tumor cells.84
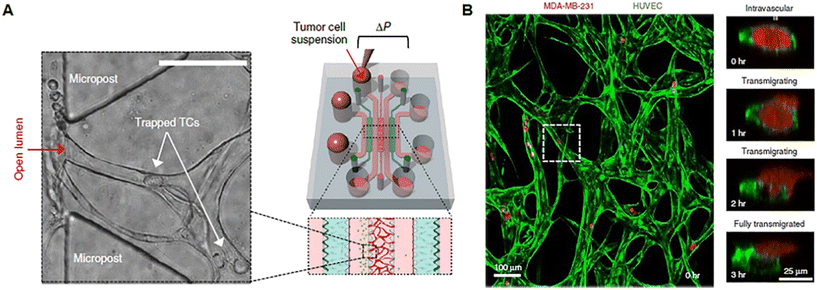 |
| Fig. 3 Tumor vasculature-on-chip. (A) Illustration of a microfluidic chip with a perfusable capillary network for the flow of tumor cells and analysis of tumor extravasation by confocal imaging. (B) The capillary network is shown in green (formed by HUVEC) and tumor cells in red (MDA-MB-231). Reproduced from ref. 82 with permission from Springer Nature, copyright 2017. | |
6.3. CAF-on-chip
In their review, Kuzet and Gaggioli defined CAFs and tumor cells as “partners in crime” in the evolution of cancer pathology. Indeed, CAFs are responsible for the production of the “soil” where tumor cells grow, move and toward which they are directed after metastasis. This is possible because CAFs can secrete growth factors, ECM elements and enzymes influencing cell behavior and matrix composition, structure and stiffness.85 CAFs can derive not only from normal fibroblasts but also from epithelial or endothelial cells undergoing mesenchymal transition as well as progenitor cells and adipocytes.86,87 Tumor cells endorse fibroblast activation and, in turn, factors produced by fibroblasts influence the behavior not only of cancer cells but also of endothelial and immune cells, strongly contributing to microenvironmental alterations observed in the TME.88,89 Several studies show that CAFs promote tumor growth, migration, invasion and metastasis. Moreover, it seems that CAFs can migrate with cancer cells during metastasis and have a role in the formation of the pre-metastatic niche, the favorable environment for tumor invasion and growth.85,90 Advances in microfluidics give the opportunity to study this harmful interaction and nanomedicine strategies, with the aim to reduce tumor growth and invasiveness. A 7-channel microfluidic chip demonstrated the mutual interaction between colorectal fibroblasts (CCD-18Co) and human colorectal cancer cells (HT-29) by the biochemical analysis of secreted soluble factor, molecular investigation of gene expression and morphological assessment. Fibroblasts and cancer cells were embedded into a 3D collagen matrix in different channels of the chip interspaced with channels for the culture medium, hence not in direct contact (Fig. 4A). Results showed that this co-culture on-chip induced a reduction of Ki67 and an increase in fibronectin expression in tumor spheroids and high α-SMA detection and migratory activity in fibroblasts.91 A microfluidic chip with single MDA-MB-231 breast cancer cells entrapped into the holes of a PDMS membrane, and fibroblasts on the bottom, revealed a mechanism of fibroblast activation by TGFβ1-induced autophagy (Fig. 4B). Furthermore, this chip allowed the retrieval of individual cancer cells, useful for single cell genetic analysis.93 In a paper published by Netti's group, stromal and tumoral microtissues were inserted in 2 separated, but communicating, chambers of a tumor-on-chip (Fig. 4C) to investigate the neoplastic cell migration from the tumoral chamber toward the stromal one, the activation of normal fibroblasts toward CAFs and the remodeling of the ECM during cancer cell invasion. After 48 hours, fibroblasts showed an increased positivity for α-SMA and PDGFβ-r, whereas higher quantities of MMP2, MMP9, hyaluronic acid and fibronectin were detected in the stroma.94 In a different chip configuration, Liu et al. showed that CAFs activate tumor invasiveness. The 3D device presented 6 co-culture units, all connected to a central channel in which the medium flows (3 on one side and 3 on the other side). These units were composed of 2 chambers, one for salivary gland adenoid cystic carcinoma (ACC) cell culture, and the other for CAF culture (Fig. 4D). Both ACC and CAF were embedded into a basement membrane extract (BME) as ECM. Tumor cells in the BME spontaneously formed 3D spheroids with variable size and the presence of CAFs increased cell invasiveness toward the adjacent chamber. The delivery of MMP inhibitor GM6001 reduced the neoplastic cell invasion (Fig. 4E, a/f).95,96 Zhao et al. implemented a high-content microwell array microfluidic device to study tumor–stroma interaction in metastasis. Fibroblast and tumor spheroids in contact in the single well of the array allowed the monitoring of cell migration by confocal imaging and quantitative analysis of the ability of tumor cells to leave the spheroids to envelop the close one formed by the fibroblasts.97 The presence of CAFs in the TME has also been associated with an altered response to therapy and mechanisms of drug resistance. Chi et al. developed an L-TumorChip microfluidic array with multiple units comprising microvascular endothelial cells on the top of a porous PDMS membrane and cancer and stromal cells in Matrigel in the bottom part of the device. The chip was geometrically adjusted to obtain physiological flow parameters by computational fluid dynamics simulation in the upper part of the membrane. The inlet velocity was set to 100 μm s−1, as in the range of velocity in human capillary, and the hydraulic permeability of 2 × 10–14 m2 of a 4 mg mL−1 Matrigel was used for the bottom chamber. The analysis of the intensity of red fluorescent protein, expressed by MDA-MB-231 breast cancer cells, demonstrated that cell number was very similar in each unit of the chip, thus highlighting the possibility to use the arrayed units as replicates (Fig. 4F). This high-throughput screening device was inspired by the in vivo TME under perfusion flow. The leaky endothelial barrier mimicked a typical aspect of tumor angiogenesis. The authors demonstrated that CAFs enhanced tumor cell proliferation and reduced doxorubicin-induced apoptosis, thus showing a delay in drug pharmacokinetics.98 Similarly, Nguyen et al. observed that CAFs are able to antagonize the effect of trastuzumab, a targeted antibody therapy directed against the human epidermal growth factor receptor 2 (HER2) in breast cancer.102 Instead, by using a tumor-on-chip model, Haque et el. demonstrated that targeting stromal cells in the TME significantly enhances the chemotherapy effect on cancer cells.103 Given not only the mutual interaction between CAFs and cancer cells but also the role of the fibroblasts in shaping and remodeling the entire microenvironment, the CAFs have appeared as a promising target of therapy.104,105 These cells specifically express the fibroblast activation protein (FAP), which is a membrane-bound serine protease, considered as a possible drug target. Lang et al. adsorbed anti-FAP monoclonal antibodies on the surface of nanoparticles carrying CXCL12 silencing siRNA to selectively target CAF.106 CXCL12 (also known as stromal-derived factor-1 (SDF-1)) is a pro-inflammatory chemokine released by CAFs and responsible for many of the changes observed in the desmoplastic TME through bonding with CXCR4. The latter is a receptor expressed by multiple cell types, such as immune cells, endothelial cells, cancer cells, fibroblasts, and stem cells.107 This targeting strategy induced the downregulation of CXCL12 by stromal cells and, consequently, the inhibition of tumor cell invasion, migration, and angiogenesis both in vitro and in vivo, as demonstrated by the repression of tumor metastasis in an orthotopic prostate murine model.106 The mentioned study described a way to reduce CAF function instead of cell presence because previous investigations showed that CAF depletion can be associated with an increased risk of metastasis and poor survival.108–110 At the same time, clinical trials with sibrotuzumab, a monoclonal antibody targeting FAP, or with small-molecule inhibitors, did not show beneficial outcomes for patients.105 Probably on account of that, none of the recent nanotechnologies tested on-chip seems to target CAFs. Instead, other emerging therapeutic strategies focus on targeting ECM proteins, enzymes, and molecular signals produced by CAFs rather than the presence of the cells themselves.111
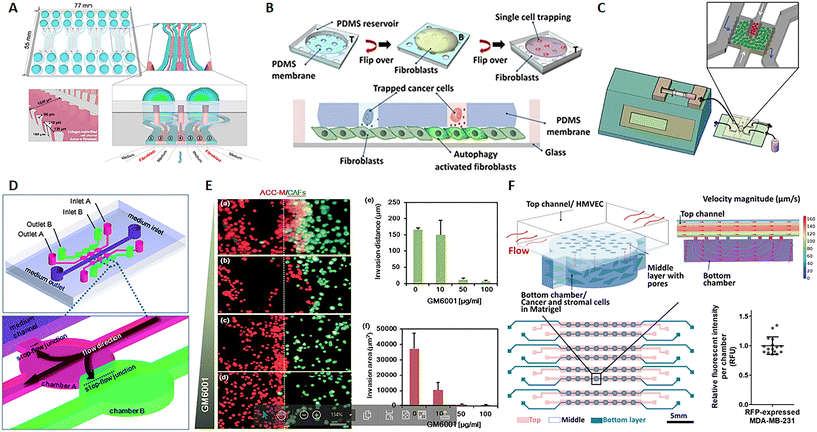 |
| Fig. 4 CAF-on-chip. (A) Design of a 7-channel chip for the co-culture of tumor spheroids and fibroblasts. Tumor spheroids were placed in the center of the device (channel 4) and fibroblasts in channels 2, interspaced by channels for medium flow (channels 1). Reproduced from ref. 91 and available under Creative Commons Attribution License, copyright 2016. (B) Design of a microfluidic chip for single cancer cell entrapment and communication with fibroblasts through a PDMS porous membrane. Reproduced from ref. 93 and available under Creative Commons CC BY license, copyright 2017. (C) Design of a microfluidic chip operated in dynamic mode by using a syringe pump and allowing the crosstalk between tumor and stromal microtissues. Reproduced from ref. 94 with permission from John Wiley and Sons, copyright 2016. (D) Design of a microfluidic chip and magnification of the chambers for tumor cell (pink) and fibroblast (green) culture. (E) Fluorescence analysis of the ability of tumor cells (red) to invade the stromal compartment with fibroblasts (green) at different doses of the MMP inhibitor GM6001 and quantification of the invasion distance and area. (a–d) Salivary gland adenoid cystic carcinoma cells (ACC-M) co-cultured with CAFs in 3D matrix for 6 days with different concentrations of GM6001. (a), 0 mg ml−1; (b), 10 mg ml−1; (c), 50 mg ml−1; (d), 100 mg ml−1. (e) The invading distance of ACC-M in 3D matrix induced by CAFs was inhibited by GM6001 in a dose-dependent manner. (f) The invading area of ACC-M in 3D matrix induced by CAFs was inhibited by GM6001 in a dose-dependent manner. Scale bar 100 mm. Reproduced from ref. 95, with permission conveyed through Copyright Clearance Center, Inc., on behalf of the Royal Society of Chemistry, copyright 2010. (F) Design of the L-TumorChip with a schematic representation of the array and magnification on a chamber for the communication between cancer and stromal cells; the fluid dynamic simulation with COMSOL shows the range of fluid velocity on-chip and the graphic of the relative fluorescence intensity per chamber demonstrating the presence of similar cell numbers per unit and consequently the possibility to use the device for high-throughput studies by using multiple replicates. Reproduced from ref. 98 with permission from John Wiley and Sons, copyright 2020. | |
6.4. Tumor ECM-on-chip
As described above, the ECM is the non-cellular component of the TME, capable of storing and releasing signals, with a profound effect on tumor and non-tumor cells. The ECM is secreted by stromal cells, and it is composed of the basement membrane (rich in type IV collagen, laminin, and fibronectin) and the interstitial matrix (fibrillar collagens, proteoglycans, and glycoproteins). The ECM provides structural tissue support and acts as a reservoir of growth factors and cytokines. Generally, after stromal cell recruitment and activation in cancer, the ECM undergoes a progressive remodeling, leading to desmoplastic reaction and enhanced deposition of dense fibrous connective tissue rich in collagen and other ECM molecules. This heterogeneous reshaping of the ECM alters the diffusion pattern of molecules, creates multiple barriers to drug transport, and increases tumor cell metastatic potential.112 At the same time, heterogeneous factors produced by cellular components of the TME can modify fibroblast capability to produce ECM elements and enhance the production of enzymes able to remodel the ECM structure (e.g., MMPs and LOX). ECM reshaping around the tumor mass, with collagen and fibronectin alignment, has been recognized as a marker of tumor invasiveness, drug resistance, and poor clinical outcomes.113,114 In the tentative to reproduce matrix changes influencing cell migration, Han et al. developed an interesting tumor-on-chip model (Fig. 5A) and highlighted the impact of different collagen orientations on cancer cell intravasation. To reproduce the interface between the interstitial matrix and the vessels, they built a mixed ECM with collagen populated by tumor cells in the center and Matrigel on the perimeter (Fig. 5B). Different collagen orientations were obtained after ECM solidification, collagen shrinking, and Matrigel swelling. Collagen fibers resulted horizontally distributed in the center and vertically aligned toward the interface with Matrigel (Fig. 5C, C1 and C2). Tumor cell organization followed the same heterogeneous orientation of collagen fibers pointing to a directional effect (Fig. 5D, a/f). The strand of cells vertically oriented to the matrix interface invaded the Matrigel with a speed of 3 μm h−1, demonstrating that collagen alignment enhanced tumor cell migration in comparison with randomly oriented fibers. Cell migration was accompanied by changes in matrix organization, suggesting a remodeling induced by MMPs. Tumor cell intravasation was evident with high metastatic breast cancer MDA-MB-231 cells but not with the weakly aggressive MCF-7 cells.115 Next, Lugo-Cintrón et al. proposed a microfluidic system (LumeNEXT) to analyze how different ECM compositions of the stroma (collagen or fibronectin-rich collagen, populated by normal or activated fibroblasts) influence the migration of breast cancer cell MDA-MB-231 (Fig. 5E and F). The device included a stromal environment with variable composition and a central lumen filled with MDA-MB-231 in collagen (Fig. 5G and H). The aim of the authors was to mimic tumor movement into the matrix. Results showed that the presence of fibronectin enhanced cell migratory ability. Furthermore, the CAFs were able to secrete fibronectin in the collagen matrix and, at the same time, the fibronectin-rich matrix could activate normal fibroblasts, thus increasing tumor cell migration. The authors analyzed the expression level of MMPs under the different culture conditions as mediators of ECM remodeling and cell migration. They highlighted a higher MMP-2, MMP-3, and MMP-9 expression in the co-culture of tumor cells and normal fibroblasts into the fibronectin-rich collagen matrix. Instead, the co-culture with CAFs produced an increase in MMP-3 expression in the collagen matrix and MMP-9 in the fibronectin-rich collagen matrix. These changes in MMP expression were reflected by the presence of gaps in the ECM. The treatment with the MMP inhibitor marimastat decreased cancer cell migration and gap presence in all the conditions but not in the co-culture of tumor cells and normal fibroblasts into the fibronectin-rich collagen matrix. However, most of the ECMs used on-chip are matrix surrogates, mainly collagen or Matrigel, with a specific composition that cannot vary over time and cannot reproduce the dynamicity of the TME. Instead, it is well known that the composition of tumor ECM is complex and dynamic, constantly changing in both composition and architecture. In this respect, as discussed in section 5, a bioengineered model based on fibroblast-synthesized ECM better replicates the features of this dynamic environment, thanks to a continuous remodeling operated by the same fibroblasts in culture. Gioiella et al. showed that during breast cancer cell invasion (Fig. 6A/D), other than the activation of the fibroblasts toward the CAF phenotype, the fibroblast-synthesized ECM also passed from a normal state to an activated one (Fig. 6E/G). The resulting ECM changed its composition in terms of collagen, hyaluronic acid and fibronectin content. Also, changes in architecture, collagen bundle diameter and assembly of collagen were observed. These modifications led to the variation of diffusivity of large macromolecules during the cancer invasion (Fig. 6H and I), demonstrating the possibility to replicate the dynamic and adaptive scenario of the tumoral ECM from a cellular, extracellular and biophysical point of view. The realization of a physiologically relevant human ECM-on-chip may constitute the keystone for the development of suitable and reliable in vitro models. Furthermore, the correct replication of the tumor ECM and its dynamicity on the chip may also have an impact on the diagnostic field.116
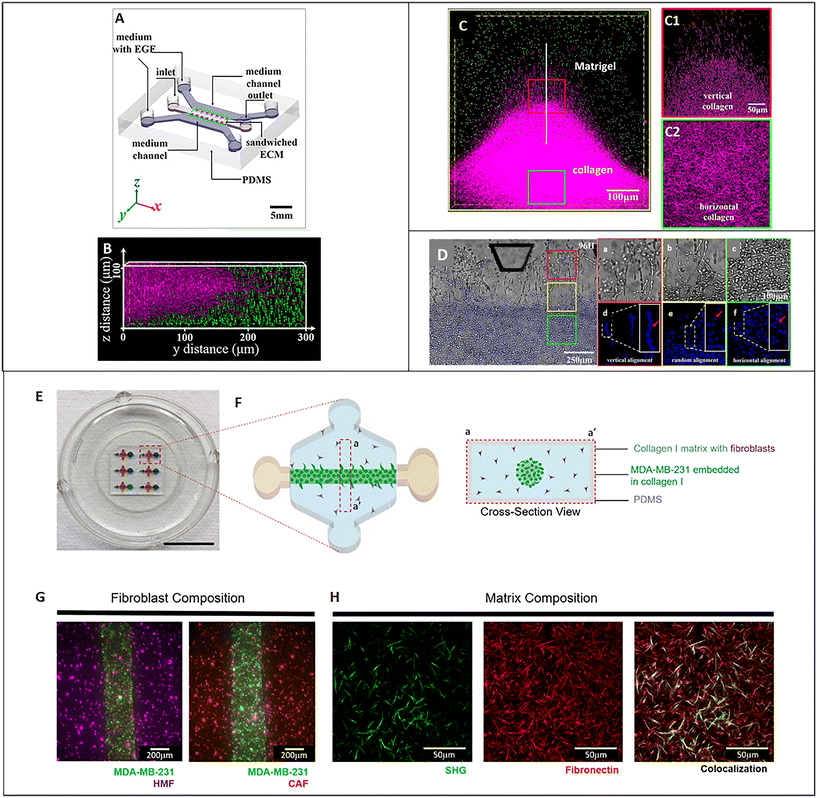 |
| Fig. 5 Tumor ECM-on-chip. (A) Illustration of the microfluidic chip. (B) Fluorescence 3D reconstruction of the composite ECM developed in the central channel of the device with collagen in the center and Matrigel on the perimeter. (C) Fluorescence images showing the different collagen fiber orientations obtained on-chip (vertical in proximity of the Matrigel in C1 and horizontal in the center in C2). (D) Bright-field and fluorescence images showing tumor cell organization following collagen matrix orientation. Enlarged insets respectively show the bright field (a–c) and fluorescent (e–g) images of the spatial distribution and morphology of cells invading the Matrigel region, (a and d), vertical alignment (b and e) random aligment and, horizontal alignment (c and f). Reproduced from ref. 115 with permission from PNAS; a copyright note was not needed because the paper was published after 2008 (2016). (E) Picture of the microfluidic device. (F) Design of a device unit for the culture of tumor cells embedded in collagen into a matrix populated by fibroblasts and cross-sectional view. (G) Fluorescence images of variable fibroblast compositions in the matrix (normal human mammary fibroblasts or activated cancer-associated fibroblasts). (H) Images of the matrix showing collagen (green) by second-harmonic generation signal (SHG) and fluorescently labeled fibronectin (red). Reproduced from ref. 126 and available under an open access Creative Commons CC BY license, copyright 2020. | |
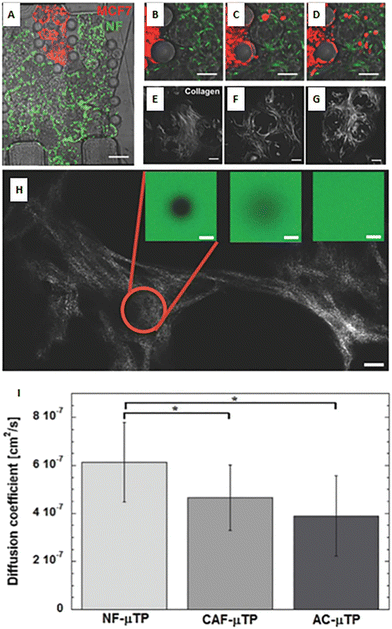 |
| Fig. 6 A bioengineered model of a dynamic connective TME. (A) Micro device composed of an inner region (in red) containing malignant MCF7 breast cells invading a connective breast tissue formed by normal breast fibroblast microtissues (in green). (B–D) Online monitoring of MCF7 invasion in the connective compartment. (E–G) Online monitoring of the evolution of endogenous collagen matrix architecture in the microtissues during cancer cell invasion via real-time acquisition of second-harmonic generation signal from collagen. (H) Measurement of diffusivity in the collagen matrix during cancer cell invasion by using fluorescence recovery after photobleaching method. (I) Values of the diffusion coefficient in the collagen matrix produced by normal fibroblasts (NF), cancer associated fibroblasts (CAF), and normal fibroblasts activated in the presence of MCF7 (AC). Reproduced from ref. 94 with permission from John Wiley and Sons, copyright 2016. | |
6.5. Tumor-on-chip for evaluating nanomedicine approaches targeting TME hallmarks
As discussed above, tumor-on-chip technology represents a promising tool for nanomedicine testing. Here we report main examples from the literature demonstrating the efficacy of these miniaturized platforms to replicate fundamental hallmarks of the TME as a target of nanomedicine and evaluate their efficacy. One of the main hallmarks of cancer is the ability to induce angiogenesis. Currently, relevant anti-angiogenic agents target the vascular endothelial growth factor (VEGF), such as bevacizumab, ramucirumab, and sorafenib. The efficacy of anti-angiogenic drugs in adjuvant or neoadjuvant therapy has been estimated in clinical trials of advanced breast cancer.117 However, the outcomes of anti-angiogenic treatments are highly heterogeneous because of tumor vascular variability. As a consequence, the need for robust preclinical models and accurate screening methods continues to be a challenge. To deal with this issue, Lee et al. developed a 3D model of tumor angiogenesis-on-chip and network mapping, suitable to study RNAi-based antiangiogenic nanomedicine. Specifically, they tested the effect of mesoporous silica nanoparticles (MSNs) carrying siRNA on angiogenesis and demonstrated that the siVEGFR-loaded MSN significantly reduced the tumor angiogenesis without interfering with cell viability. The microfluidic platform was composed of two separated channels for fibroblast and endothelial cell culture, spaced by a central chamber loaded with an acellular fibrin hydrogel. Pro-angiogenic factors secreted by fibroblasts induced endothelial cell sprouts into the central chamber. Tumor cells were added into an additional chamber in the center of the device to evaluate the effect of siVEGFR-loaded MSNs on tumor angiogenesis (Fig. 7A). The results obtained in vitro agreed with in vivo data, demonstrating the normalization of the blood vessel network after treatment with siVEGFR/MSN, as shown by 3D imaging-based analysis after tissue clearing (Fig. 7B).118 On the other hand, Chen et al. developed a breast cancer model on-chip featuring the presence of an endothelial barrier, with the aim to study drug transport across the microvascular wall. More in detail, the system comprised a channel for the endothelial monolayer and a chamber for multicellular tumor spheroid housing, spaced by a channel for the ECM in the form of BME (Fig. 7C). The model was used to analyze the transport process and cytotoxicity of carbon dots (CDs) functionalized with polyethylene glycol (PEG) and folic acid (FA) and loaded with doxorubicin (DOX), named CD–PEG–FA/DOX. Drug transport across the endothelium and ECM as well as drug infiltration into the tumor and cell cytotoxicity could be evaluated in real-time with spatial–temporal resolution and allow high-throughput screening. The work showed that CD–PEG–FA/DOX permeation into spheroids and cytotoxicity were different depending on the cell type used to obtain the spheroid (BT549 or T47D9), thus underlining the importance of accurately reproducing TME-on-chip to study nanomedicine strategies.67 In another work, Chen et al. demonstrated that the interaction of nanoparticles with endothelial cells and adsorption are influenced by the flow rate and particle functionalization. Modulating these parameters can enhance nanomedicine targeting tumor or the TME by facilitating nanoparticle transit across the endothelium.119 As widely discussed above, ECM remodeling is another recognized hallmark of the TME. Indeed, ECM alterations can dramatically influence nanoparticle ability to reach the tumor mass. Albanese et al. demonstrated that the capability of nanoparticles to move into the tumor ECM toward the tumor depends on particle diameter. They showed that a nanoparticle having a diameter of 100 nm may reflect the upper limit of ECM diffusivity because particles with a bigger diameter could not enter and cross the ECM network. Furthermore, high flow rates induced nanoparticle accumulation and immobilization in the ECM around the tumor. Receptor binding enhanced retention. but unspecific binding to the ECM acted as a barrier for nanoparticle diffusion. For their study, the authors used a single-channel microfluidic chip with melanoma cell spheroids embedded into a laminin matrix under fluidic flow conditions controlled by a syringe pump.120 In another work, Wang et al. developed a device to mimic the biological barriers which hinder nanoparticle delivery such as the endothelium (represented by a layer of HUVECs) and the ECM (represented by Matrigel). They studied the movement of PEGylated and fluorescent nanoparticles (liposomes and poly(lactide-co-glycolic acid) nanoparticles) across the endothelial and stromal barrier and showed that the coexistence of these microenvironmental elements significantly decreased nanoparticle permeability.121 Therefore, on-chip experiments confirmed that the presence of the ECM represents an indispensable requirement for modeling the TME in vitro and for accurately evaluating the efficacy of target therapies.
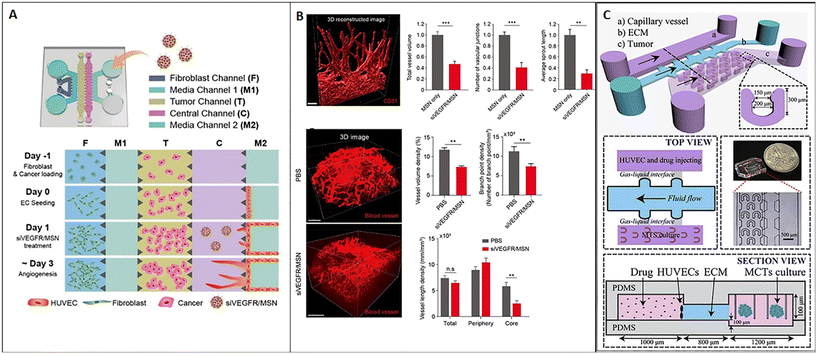 |
| Fig. 7 Tumor-on-chip for evaluating nanomedicine approaches targeting TME hallmarks. (A) Schematic representation of the chip and cell loading sequence; mesoporous silica nanoparticles (MSNs) carrying siVEGF significantly reduced tumor angiogenesis (the vascular network is represented in red in 3D reconstructions) on-chip, as shown by the decrease of the total vessel volume, the number of vascular junctions and the average sprout length (B, top); a similar situation has been observed in vivo, as demonstrated by the vessel volume and branch point evaluation as well as vessel length density (B, bottom). Reproduced from ref. 118 with permission from the American Chemical Society, copyright 2021. (C) Schematic representation of the microfluidic chip with 3 microchannels: one channel hosts the capillary vessel, another the ECM (basement membrane extract or BME), and the last hosts multiple U-shaped microchambers in which tumor spheroids are accommodated; the top and the lateral views of the device are shown in detail as well as a macroscopic picture. Reproduced from ref. 67 with permission from Elsevier, copyright 2018. | |
7. Conclusion and perspectives
The current understanding about cancers has established that targeting the neoplastic cells is not the unique route of intervention to fight the tumor. As the TME has been recognized to play a pivotal role in cancer progression, the urgency of designing nanotherapeutic approaches targeting the TME components is emerging. Besides the engineering of nanoparticles targeting specific TME signatures, the development of experimental models to validate their effectiveness and mechanisms of action is still challenging. Several biomimetic human cancer models with increasingly improved architectural and functional similarity to their in vivo counterparts have been produced thanks to the impressive technological progress made in the microfluidics and tissue engineering fields. However, most of the tumor-on-chip platforms developed integrate sophisticated miniaturized instruments for controlling and sensing directly on-chip, but suffer for the extreme simplicity of the bioengineered cancer models used. Indeed, the use of either tumoral spheroids or exogenous materials, even if it guarantees the tridimensionality for cells, suffers the absence of native-like ECM, strongly limiting the relevance of the 3D bioengineered models as an in vitro platform for testing novel nanomedicine approaches. Furthermore, only a few in vitro models have been used to study the efficiency of nanomedicines targeting the ECM. Table 1 summarizes some of the models reported in this review used to study target therapy. It is evident that most of the strategies targeting the ECM have been studied in the xenograft model or directly introduced in clinical trials, unfortunately with poor outcomes.122 Most probably, the cause of this failure may be related to the inadequacy of the preclinical models to replicate the heterogeneity of the native human ECM. Moreover, the enhanced delivery efficiency of several nanomedicines in mouse xenograft cancer models may be an “artifact” of the animal model itself that has limited clinical translation in human cancer patients. Many researchers suggest that a more reliable in vivo model to estimate the delivery efficiency of nanomedicines in comparison with free drugs may be the transgenic mouse spontaneous cancer model. However, very few spontaneous cancer models are available for different types of cancers; even so the difference in species between mice and humans can affect the response to drug treatments, resulting in a wrong preclinical result.122 The result of this situation is that despite the increasing number of nanoparticles developed for targeting the TME only a few of them have entered in clinical applications due to both the poor knowledge about the optimal ECM component to target and the lack of robust models able to provide precise information about the nanomedicine's effectiveness during the preclinical phases. The spatial and temporal dynamicity of the TME seems to be one of the most relevant features that needs to be replicated in 3D bioengineered models, as the variations in terms of organization and composition compromise the effectiveness of the nanomedicines. In this regard we have shown how tissue engineering approaches based on in vitro reproduction of an endogenous ECM seem to be very promising. Indeed, in vitro tissues composed of fibroblasts embedded in their own ECM (endogenous ECM) are able to reproduce the main feature of their in vivo counterpart.123 Moreover, such models, despite those based on the use of exogenous biopolymers, possess two key elements that enhance the relevance of tumor-on-chip models: (i) by using CAFs from a specific cancer tissue environment it is possible to reproduce the specific ECM of the cancer of interest; (ii) in the presence of cancer cells, the CAF-synthesized ECM displays spatiotemporal compositional, architectural and biophysical variations resembling the in vivo situations.26,94,123,124 Such dynamic models, once coupled with microfluidic devices, may offer the possibility to test nanomedicines in a robust and reliable context.94 Other than to test the effectiveness, it will be possible to understand the modifications that a nanomedicine will induce at the TME level and if the same nanomedicine will still be effective in the modified TME. In this way, the properties of nanomedicines (surface chemistry, charge, shape and size) used to optimize both trafficking and targeting can be engineered in order to adapt themselves to the evolving TME and be more effective.125
Table 1 Summary of the strategies and experimental models exploited to study therapies targeting the tumor microenvironment reported in this review
Target |
Therapeutic agent |
TME component |
Experimental model |
Reference |
TGF-β |
TGF-β signaling inhibitors that interfere with TGF-β bioavailability/receptor interaction/receptor kinase function |
ECM |
In vivo murine model |
127, 128 |
Clinical trials |
Collagen |
Collagenase injected intravenously |
ECM |
Tumor xenograft model |
36, 37 |
Collagenase conjugated to gold nanocarriers |
Hyaluronic acid |
Hyaluronidase conjugated to PEG nanoparticles |
ECM |
Phase III clinical trials |
14, 39 |
Cyclooxygenase-2 (COX-2) |
Celecoxib (COX-2 inhibitor) |
CAF |
Tumor xenograft model |
129
|
ECM |
Lysyl oxidase (LOX) |
Poly(D,L-lactide-co-glycolide) nanoparticles coated with a LOX |
ECM |
Tumor xenograft model |
130
|
Inhibitory antibody |
MMP |
MMP inhibitor GM6001 |
ECM |
Tumor on chip |
95
|
MMP-2 |
Cross-linked polyelectrolyte nanocapsule based on an oil core and a matrix metalloproteinase-2 (MMP-2)-cleavable shell |
ECM |
3D spheroid model |
78
|
VEGFR |
Mesoporous silica nanoparticles (MSNs) carrying siVEGFR |
Endothelial cells |
3D model of tumor angiogenesis-on-chip |
118
|
Tumor xenograft model |
FAP |
Anti-FAP monoclonal antibodies on the surface of nanoparticles carrying silencing siCXCL12 |
CAFs |
2D wound healing model and invasion assay in Transwell |
106
|
Orthotopic prostate murine model |
Author contributions
All authors contributed to the writing, discussion, and correction of the review.
Conflicts of interest
All authors declare no conflict of interest.
Acknowledgements
The research leading to these results has received funding from AIRC under IG 2021 - ID. 26185 project – P.I. Netti Paolo Antonio.
References
- M.-J. Tsai, W.-A. Chang, M.-S. Huang and P.-L. Kuo, ISRN Biochem., 2014, 2014, 1–8 CrossRef PubMed.
- Y. Zhao, X. Zheng, Y. Zheng, Y. Chen, W. Fei, F. Wang and C. Zheng, Front. Oncol., 2021, 11, 1–14 Search PubMed.
- N. Subrahmanyam and H. Ghandehari, J. Pers. Med., 2021, 11, 1–19 CrossRef PubMed.
- D. Rodenhizer, T. Dean, E. D'Arcangelo and A. P. McGuigan, Adv. Healthcare Mater., 2018, 7, 1701174 CrossRef PubMed.
- S. N. Ekdawi, D. A. Jaffray and C. Allen, Nano Today, 2016, 11, 402–414 CrossRef CAS.
- Y. S. Zhang, Y. N. Zhang and W. Zhang, Drug Discovery Today, 2017, 22, 1392–1399 CrossRef CAS PubMed.
- E. L. Siegler, Y. J. Kim and P. Wang, J. Cell. Immunother., 2016, 2, 69–78 CrossRef.
- J. Rodrigues, M. A. Heinrich, L. M. Teixeira and J. Prakash, Trends Cancer, 2021, 7, 249–264 CrossRef CAS PubMed.
- K. C. Valkenburg, A. E. De Groot and K. J. Pienta, Nat. Rev. Clin. Oncol., 2018, 15, 366–381 CrossRef PubMed.
- D. L. Vitale, A. Icardi, P. Rosales, F. M. Spinelli, I. Sevic and L. D. Alaniz, Front. Oncol., 2021, 11, 1–17 Search PubMed.
- L. Miao, C. M. Lin and L. Huang, J. Controlled Release, 2015, 219, 192–204 CrossRef CAS.
- K. Binnemars-Postma, G. Storm and J. Prakash, Int. J. Mol. Sci., 2017, 18, 979 CrossRef PubMed.
- X. Li, X. Guo, J. Ling, Z. Tang, G. Huang, L. He and T. Chen, Nanoscale, 2021, 13, 4705–4727 RSC.
- J. Li and D. J. Burgess, Acta Pharm. Sin. B, 2020, 10, 2110–2124 CrossRef CAS PubMed.
- M. Truffi, S. Mazzucchelli, A. Bonizzi, L. Sorrentino, R. Allevi, R. Vanna, C. Morasso and F. Corsi, Int. J. Mol. Sci., 2019, 20, 1263 CrossRef CAS.
- J. Liu, X. Ai, H. Cabral, J. Liu, Y. Huang and P. Mi, Biomaterials, 2021, 273, 120847 CrossRef CAS PubMed.
- Q. Liang, L. Zhou, Y. Li, J. Liu and Y. Liu, J. Drug Targeting, 2022, 30, 119–130 CrossRef CAS.
- P. T. Kutoka, T. A. Seidu, V. Baye, A. M. Khamis, C. T. Qizi Omonova and B. Wang, OpenNano, 2022, 7, 100041 CrossRef.
- P. Boix-Montesinos, P. M. Soriano-Teruel, A. Armiñán, M. Orzáez and M. J. Vicent, Adv. Drug Delivery Rev., 2021, 173, 306–330 CrossRef CAS PubMed.
- G. Imparato, F. Urciuolo and P. A. Netti, Bioengineering, 2022, 9, 1–24 CrossRef.
- R. Baghban, L. Roshangar, R. Jahanban-Esfahlan, K. Seidi, A. Ebrahimi-Kalan, M. Jaymand, S. Kolahian, T. Javaheri and P. Zare, Cell Commun. Signaling, 2020, 18(1), 59 CrossRef PubMed.
- J. Winkler, A. Abisoye-Ogunniyan, K. J. Metcalf and Z. Werb, Nat. Commun., 2020, 11, 1–19 CrossRef.
- M. R. Junttila and F. J. De Sauvage, Nature, 2013, 501(7467), 346–354 CrossRef CAS PubMed.
- N. El-Sayes, A. Vito and K. Mossman, Cancers, 2021, 13, 1–14 CrossRef.
- I. Dagogo-Jack and A. T. Shaw, Nat. Rev. Clin. Oncol., 2017, 15(2), 81–94 CrossRef.
- C. Mazio, C. Casale, G. Imparato, F. Urciuolo and P. A. Netti, Acta Biomater., 2018, 73, 236–249 CrossRef PubMed.
- A. K. Patel and S. Singh, Front. Biosci.-Landmark, 2020, 25, 961–978 CrossRef CAS PubMed.
- E. Wagenblast, M. Soto, S. Gutiérrez-Ángel, C. A. Hartl, A. L. Gable, A. R. Maceli, N. Erard, A. M. Williams, S. Y. Kim, S. Dickopf, J. C. Harrell, A. D. Smith, C. M. Perou, J. E. Wilkinson, G. J. Hannon and S. R. V. Knott, Nature, 2015, 520, 358–362 CrossRef CAS.
- S. Garattini, I. Fuso Nerini and M. D'Incalci, Ann. Oncol., 2018, 29, 13–18 CrossRef CAS PubMed.
- P. Gilson, J. L. Merlin and A. Harlé, Cancers, 2022, 14, 1384 CrossRef PubMed.
- A. Dhawan, T. A. Graham and A. G. Fletcher, Cancer Prev. Res., 2016, 9(4), 283–295 CrossRef CAS PubMed.
- J. A. Delgado San Martin, J. I. Hare, J. W. T. Yates and S. T. Barry, Nanomedicine, 2015, 11, 1247–1252 CrossRef CAS PubMed.
- R. Ge, Z. Wang and L. Cheng, npj Precis. Oncol., 2022, 6(1), 31 CrossRef CAS PubMed.
- G. Song, D. B. Darr, C. M. Santos, M. Ross, A. Valdivia, J. L. Jordan, B. R. Midkiff, S. Cohen, N. Nikolaishvili-Feinberg, C. R. Miller, T. K. Tarrant, A. B. Rogers, A. C. Dudley, C. M. Perou and W. C. Zamboni, Clin. Cancer Res., 2014, 20, 6083–6095 CrossRef CAS PubMed.
- J. Liu, L. Tian, R. Zhang, Z. Dong, H. Wang and Z. Liu, ACS Appl. Mater. Interfaces, 2018, 10, 43493–43502 CrossRef CAS PubMed.
- S. Murty, T. Gilliland, P. Qiao, T. Tabtieng, E. Higbee, A. Al Zaki, E. Puré and A. Tsourkas, Part. Part. Syst. Charact., 2014, 31, 1307–1312 CrossRef CAS PubMed.
- M. Kato, Y. Hattori, M. Kubo and Y. Maitani, Int. J. Pharm., 2012, 423, 428–434 CrossRef CAS PubMed.
- P. P. Provenzano, C. Cuevas, A. E. Chang, V. K. Goel, D. D. Von Hoff and S. R. Hingorani, Cancer Cell, 2012, 21, 418–429 CrossRef CAS PubMed.
- G. J. Doherty, M. Tempero and P. G. Corrie, Future Oncol., 2018, 14, 13–22 CrossRef CAS.
- J. Wang, W. Mao, L. L. Lock, J. Tang, M. Sui, W. Sun, H. Cui, D. Xu and Y. Shen, ACS Nano, 2015, 9(7), 7195–7206 CrossRef CAS.
- X. Xu, Y. Wu, X. Qian, Y. Wang, J. Wang, J. Li, Y. Li and Z. Zhang, Adv. Healthcare Mater., 2022, 11, 2101428 CrossRef CAS.
- K. C. L. Black, Y. Wang, H. P. Luehmann, X. Cai, W. Xing, B. Pang, Y. Zhao, C. S. Cutler, V. Wang, Y. Liu and Y. Xia, ACS Nano, 2014, 8, 4385–4394 CrossRef CAS PubMed.
- V. P. Chauhan, Z. Popovi, O. Chen, J. Cui, D. Fukumura, M. G. Bawend and R. K. Jain, Angew. Chem., Int. Ed., 2011, 50, 11417–11420 CrossRef CAS PubMed.
- W. Zhang, M. Yu, Z. Xi, D. Nie, Z. Dai, J. Wang, K. Qian, H. Weng, Y. Gan and L. Xu, ACS Appl. Mater. Interfaces, 2019, 11, 46614–46625 CrossRef CAS PubMed.
- B. R. Smith, P. Kempen, D. Bouley, A. Xu, Z. Liu, N. Melosh, H. Dai, R. Sinclair and S. S. Gambhir, Nano Lett., 2012, 12, 3369 CrossRef CAS PubMed.
- L. Zeng, L. Zou, H. Yu, X. He, H. Cao, Z. Zhang, Q. Yin, P. Zhang, W. Gu, L. Chen and L. Yaping, Adv. Funct. Mater., 2016, 26, 4201–4212 CrossRef CAS.
- L. Agemy, D. Friedmann-Morvinski, V. Ramana Kotamraju, L. Roth, K. N. Sugahara, O. M. Girard, R. F. Mattrey, I. M. Verma and E. Ruoslahti, Proc. Natl. Acad. Sci. U. S. A., 2011, 108(42), 17450–17455 CrossRef CAS PubMed.
- D. Miao, M. Jiang, Z. Liu, G. Gu, Q. Hu, T. Kang, Q. Song, L. Yao, W. Li, X. Gao, M. Sun and J. Chen, Mol. Pharmaceutics, 2014, 11, 90–101 CrossRef CAS PubMed.
- Q. Hu, G. Gu, Z. Liu, M. Jiang, T. Kang, D. Miao, Y. Tu, Z. Pang, Q. Song, L. Yao, H. Xia, H. Chen, X. Jiang, X. Gao and J. Chen, Biomaterials, 2013, 34, 1135–1145 CrossRef CAS PubMed.
- L. Alberici, L. Roth, K. N. Sugahara, L. Agemy, V. R. Kotamraju, T. Teesalu, C. Bordignon, C. Traversari, G. P. Rizzardi and E. Ruoslahti, Cancer Res., 2013, 73, 804–812 CrossRef CAS.
- H. Wang, J. Li, Z. Wang, Y. Wang, X. Xu, X. Gong, J. Wang, Z. Zhang and Y. Li, Biomaterials, 2021, 269, 120609 CrossRef CAS PubMed.
- J. Li, H. Wang, Y. Wang, X. Gong, X. Xu, X. Sha, A. Zhang, Z. Zhang and Y. Li, Adv. Mater., 2020, 32, 2002380 CrossRef CAS PubMed.
- J. Wang, Y. Wang, H. Cao, H. Wang, J. Li, Y. Li, Y. Li and Z. Zhang, J. Controlled Release, 2020, 323, 59–70 CrossRef CAS PubMed.
- K. Hu, L. Miao, T. J. Goodwin, J. Li, Q. Liu and L. Huang, ACS Nano, 2017, 11, 4916–4925 CrossRef CAS PubMed.
- R. Raavé, T. H. van Kuppevelt and W. F. Daamen, J. Controlled Release, 2018, 274, 1–8 CrossRef PubMed.
- C. E. Roată, Ş. Iacob, Ş. Morăraşu, C. Livadaru, I. Tudorancea, S. Luncă and M. G. Dimofte, Crystals, 2021, 11, 1–11 CrossRef.
- D. Van Den Brand, L. F. Massuger, R. Brock and W. P. R. Verdurmen, Bioconjugate Chem., 2017, 28, 846–856 CrossRef CAS PubMed.
- V. Brancato, V. Comunanza, G. Imparato, D. Corà, F. Urciuolo, A. Noghero, F. Bussolino and P. A. Netti, Acta Biomater., 2017, 49, 152–166 CrossRef CAS PubMed.
- V. De Gregorio, G. Imparato, F. Urciuolo, M. L. Tornesello, C. Annunziata, F. M. Buonaguro and P. A. Netti, Adv. Healthcare Mater., 2017, 6(11), 1601199 CrossRef PubMed.
- V. De Gregorio, A. La, F. Urciuolo, C. Annunziata, M. Lina, F. Maria, P. Antonio and G. Imparato, Acta Biomater., 2020, 116, 209–222 CrossRef CAS PubMed.
- J. Ahn, Y. J. Sei, N. L. Jeon and Y. Kim, Bioengineering, 2017, 4, 1–17 CrossRef PubMed.
- X. Liu, J. Fang, S. Huang, X. Wu, X. Xie, J. Wang, F. Liu, M. Zhang, Z. Peng and N. Hu, Microsyst. Nanoeng., 2021, 7, 50 CrossRef CAS PubMed.
- J. Hwang, M. O. Sullivan and K. L. Kiick, Front. Bioeng. Biotechnol., 2020, 8, 69 CrossRef PubMed.
- D. R. Mertz, T. Ahmed and S. Takayama, Lab Chip, 2018, 18, 2378 RSC.
- S. J. Hachey and C. C. W. Hughes, Lab Chip, 2018, 18, 2893–2912 RSC.
- B. R. Seo, P. DelNero and C. Fischbach, Adv. Drug Delivery Rev., 2014, 69–70, 205–216 CrossRef CAS PubMed.
- Y. Chen, D. Gao, Y. Wang, S. Lin and Y. Jiang, Anal. Chim. Acta, 2018, 1036, 97–106 CrossRef CAS PubMed.
- P. Gupta, P. A. Pérez-Mancera, H. Kocher, A. Nisbet, G. Schettino and E. G. Velliou, Front. Bioeng. Biotechnol., 2020, 8, 290 CrossRef PubMed.
- Y. Shinsato, A. D. Doyle, W. Li and K. M. Yamada, Cancer Rep., 2020, 3(5), 1257 Search PubMed.
- C. Liu, B. Chiang, D. Lewin Mejia, K. E. Luker, G. D. Luker and A. Lee, Acta Biomater., 2019, 83, 221–232 CrossRef CAS PubMed.
- C. L. Hedegaard, C. Redondo-Gómez, B. Y. Tan, K. W. Ng, D. Loessner and A. Mata, Sci. Adv., 2020, 6(40), eabb3298 CrossRef CAS PubMed.
- G. Iaccarino, M. Profeta, R. Vecchione and P. A. Netti, Acta Biomater., 2019, 89, 265–278 CrossRef CAS PubMed.
- V. Brancato, A. Garziano, F. Gioiella, F. Urciuolo, G. Imparato, V. Panzetta, S. Fusco and P. A. Netti, Acta Biomater., 2017, 47, 1–13 CrossRef PubMed.
- P. Carmeliet and R. K. Jain, Nature, 2000, 407(6801), 249–257 CrossRef CAS PubMed.
- L. Gelao, C. Criscitiello, L. Fumagalli, M. Locatelli, S. Manunta, A. Esposito, I. Minchella, A. Goldhirsch and G. Curigliano, Ecancermedicalscience, 2013, 7, 1–15 Search PubMed.
- G. Bergers and L. E. Benjamin, Nat. Rev. Cancer, 2003, 3, 401–410 CrossRef CAS PubMed.
- N. Weidner, J. P. Semple, W. R. Welch and J. Folkman, N. Engl. J. Med., 1991, 324, 1991 CrossRef PubMed.
- Y.-H. V. Ma, K. Middleton, L. You and Y. Sun, Microsyst. Nanoeng., 2018, 4, 1–13 CrossRef PubMed.
- S. Paget, Cancer Metastasis Rev., 1989, 8(2), 98–101 CAS.
- M. Akhtar, A. Haider, S. Rashid and A. D. M. H. Al-Nabet, Adv. Anat. Pathol., 2019, 26, 69–74 CrossRef CAS PubMed.
- L. Mathot and J. Stenninger, Cancer Sci., 2012, 103, 626–631 CrossRef CAS PubMed.
- M. B. Chen, J. A. Whisler, J. Fröse, C. Yu, Y. Shin and R. D. Kamm, Nat. Protoc., 2017, 12, 865–880 CrossRef CAS PubMed.
- X. Cui, W. Guo, Y. Sun, B. Sun, S. Hu, D. Sun and R. H. W. Lam, Biomicrofluidics, 2017, 11, 014105 CrossRef PubMed.
- A. Villasante and G. Vunjak-Novakovic, Expert Opin. Drug Discovery, 2015, 10, 257–268 CrossRef CAS PubMed.
- S. Kuzet and C. Gaggioli, Cell Tissue Res., 2016, 365, 607–619 CrossRef CAS PubMed.
- N. Eddine Sounni and N. Agnes, Clin. Chem., 2013, 59, 85–93 CrossRef PubMed.
- K. Kim, Y. J. Sohn, R. Lee, H. J. Yoo, J. Y. Kang, N. Choi, D. Na and J. H. Yeon, Int. J. Mol. Sci., 2020, 21, 1–18 Search PubMed.
- W. Wang, Q. Li, T. Yamada, K. Matsumoto, I. Matsumoto, M. Oda, G. Watanabe, Y. Kayano, Y. Nishioka, S. Sone and S. Yano, Clin. Cancer Res., 2009, 15(21), 6630–6638 CrossRef CAS PubMed.
- T. Liu, C. Han, S. Wang, P. Fang, Z. Ma, L. Xu and R. Yin, J. Hematol. Oncol., 2019, 1, 1–15 Search PubMed.
- D. G. Duda, A. M. M. J. Duyverman, M. Kohno, M. Snuderl, E. J. A. Steller, D. Fukumura and R. K. Jain, Proc. Natl. Acad. Sci. U. S. A., 2010, 107, 21677–21682 CrossRef CAS PubMed.
- S. Y. Jeong, J. H. Lee, Y. Shin, S. Chung and H. J. Kuh, PLoS One, 2016, 11, 1–17 Search PubMed.
- A. Weltin, K. Slotwinski, J. Kieninger, I. Moser, G. Jobst, M. Wego, R. Ehret and G. A. Urban, Lab Chip, 2014, 14, 138–146 RSC.
- H. Ezgi Karakas, J. Kim, P. Juhee, J. Min Oh, Y. Choi, D. Gozuacik and Y.-K. Cho, Sci. Rep., 2017, 7, 1–11 CrossRef PubMed.
- F. Gioiella, F. Urciuolo, G. Imparato, V. Brancato and P. A. Netti, Adv. Healthcare Mater., 2016, 5, 3074–3084 CrossRef CAS PubMed.
- T. Liu, B. Lin and J. Qin, Lab Chip, 2010, 10, 1671–1677 RSC.
- M. Rothbauer, H. Zirath and P. Ertl, Lab Chip, 2018, 18, 249–270 RSC.
- L. Zhao, Y. Liu, Y. Liu, M. Zhang and X. Zhang, Anal. Chem., 2020, 92, 7638–7645 CrossRef CAS PubMed.
- C. W. Chi, Y. H. Lao, A. H. R. Ahmed, E. C. Benoy, C. Li, Z. Dereli-Korkut, B. M. Fu, K. W. Leong and S. Wang, Adv. Healthcare Mater., 2020, 9, 1–8 Search PubMed.
- L. E. Bertassoni, M. Cecconi, V. Manoharan, M. Nikkhah, J. Hjortnaes, A. L. Cristino, G. Barabaschi, D. Demarchi, M. R. Dokmeci, Y. Yang and A. Khademhosseini, Lab Chip, 2014, 13, 2202–2211 RSC.
- F. Cheng, X. Cao, H. Li, T. Liu, X. Xie, D. Huang, S. Maharjan, H. P. Bei, A. Gómez, J. Li, H. Zhan, H. Shen, S. Liu, J. He and Y. S. Zhang, Nano Lett., 2019, 19, 3603–3611 CrossRef CAS PubMed.
- C. Di Natale, V. De Gregorio, E. Lagreca, F. Mauro, B. Corrado, R. Vecchione and P. A. Netti, Front. Bioeng. Biotechnol., 2022, 10, 1–10 Search PubMed.
- M. Nguyen, A. De Ninno, A. Mencattini, F. Mermet-Meillon, G. Fornabaio, S. S. Evans, M. Cossutta, Y. Khira, W. Han, P. Sirven, F. Pelon, D. Di Giuseppe, F. R. Bertani, A. Gerardino, A. Yamada, S. Descroix, V. Soumelis, F. Mechta-Grigoriou, G. Zalcman, J. Camonis, E. Martinelli, L. Businaro and M. C. Parrini, Cell Rep., 2018, 25, 3884–3893 CrossRef CAS PubMed , e3.
- M. R. Haque, C. R. Wessel, D. D. Leary, C. Wang, A. Bhushan and F. Bishehsari, Microsyst. Nanoeng., 2022, 8(1), 1–13 CrossRef PubMed.
- F. Xing, J. Saidou and K. Watabe, Front. Biosci.-Landmark, 2011, 15, 166–179 CrossRef PubMed.
- C. Roma-Rodrigues, R. Mendes, P. V. Baptista and A. R. Fernandes, Int. J. Mol. Sci., 2019, 20, 840 CrossRef CAS.
- J. Lang, X. Zhao, Y. Qi, Y. Zhang, X. Han, Y. Ding, J. Guan, T. Ji, Y. Zhao and G. Nie, ACS Nano, 2019, 13, 12357–12371 CrossRef CAS PubMed.
- F. Guo, Y. Wang, J. Liu, S. C. Mok, F. Xue and W. Zhang, Oncogene, 2016, 35, 816–826 CrossRef CAS PubMed.
- B. C. Özdemir, T. Pentcheva-Hoang, J. L. Carstens, X. Zheng, C. C. Wu, T. R. Simpson, H. Laklai, H. Sugimoto, C. Kahlert, S. V. Novitskiy, A. DeJesus-Acosta, P. Sharma, P. Heidari, U. Mahmood, L. Chin, H. L. Moses, V. M. Weaver, A. Maitra, J. P. Allison, V. S. LeBleu and R. Kalluri, Cancer Cell, 2014, 25, 719–734 CrossRef PubMed.
- J. Barbazán and D. Matic Vignjevic, Curr. Opin. Cell Biol., 2019, 56, 71–79 CrossRef PubMed.
- C. Yunna, H. Mengru, W. Fengling, W. Lei and C. Weidong, Eur. J. Pharm. Biopharm., 2021, 165, 75–83 CrossRef PubMed.
- T. Liu, L. Zhou, D. Li, T. Andl and Y. Zhang, Front. Cell Dev. Biol., 2019, 7, 1–14 CrossRef PubMed.
- A. A. Sainio and H. Järveläinen, J. Carcinog. Mutagen., 2013, S13, 2157–2518 Search PubMed.
- M. W. Conklin, J. C. Eickhoff, K. M. Riching, C. A. Pehlke, K. W. Eliceiri, P. P. Provenzano, A. Friedl and P. J. Keely, Am. J. Pathol., 2011, 178, 1221–1232 CrossRef PubMed.
- P. P. Provenzano, D. R. Inman, K. W. Eliceiri, J. G. Knittel, L. Yan, C. T. Rueden, J. G. White and P. J. Keely, BMC Med., 2008, 6, 11 CrossRef PubMed.
- W. Han, S. Chen, W. Yuan, Q. Fan, J. Tian, X. Wang, L. Chen, X. Zhang, W. Wei, R. Liu, J. Qu, Y. Jiao, R. H. Austin and L. Liu, Proc. Natl. Acad. Sci. U. S. A., 2016, 113, 11208–11213 CrossRef CAS PubMed.
- D. Sun, Z. Chen, M. Wu and Y. Zhang, Nanotheranostics, 2017, 1, 389–402 CrossRef PubMed.
- T. Bøgelund Kristensen, M. L. T. Knutsson, M. Wehland, B. Elmedal Laursen, D. Grimm, E. Warnke and N. E. Magnusson, Int. J. Mol. Sci., 2014, 15, 23024–23041 CrossRef PubMed.
- S. Lee, S. Kim, D. J. Koo, J. Yu, H. Cho, H. Lee, J. M. Song, S. Y. Kim, D. H. Min and N. L. Jeon, ACS Nano, 2021, 15, 338–350 CrossRef CAS PubMed.
- Y. Y. Chen, A. M. Syed, P. MacMillan, J. V. Rocheleau and W. C. W. Chan, Adv. Mater., 2020, 32, 1906274 CrossRef CAS PubMed.
- A. Albanese, A. K. Lam, E. A. Sykes, J. V. Rocheleau and W. C. W. Chan, Nat. Commun., 2013, 4, 2718 CrossRef PubMed.
- H. F. Wang, R. Ran, Y. Liu, Y. Hui, B. Zeng, D. Chen, D. A. Weitz and C. X. Zhao, ACS Nano, 2018, 12, 11600–11609 CrossRef CAS PubMed.
- D. Sun, S. Zhou and W. Gao, ACS Nano, 2020, 14, 12281–12290 CrossRef CAS PubMed.
- V. Brancato, A. Garziano, F. Gioiella, F. Urciuolo, G. Imparato, V. Panzetta, S. Fusco and P. A. Netti, Acta Biomater., 2017, 47, 1–13 CrossRef PubMed.
- V. Brancato, F. Gioiella, G. Imparato, D. Guarnieri, F. Urciuolo and P. A. Netti, Acta Biomater., 2018, 75, 200–212 CrossRef CAS PubMed.
- K. L. Swetha and A. Roy, Drug Delivery Transl. Res., 2018, 8, 1508–1526 CrossRef CAS PubMed.
- K. M. Lugo-Cintrón, M. M. Gong, J. M. Ayuso, L. Tomko, D. J. Beebe, M. Virumbrales-Muñoz and S. M. Ponik, Cancers, 2020, 12, 1173 CrossRef PubMed.
- L. K. Huynh, C. J. Hipolito and P. Ten Dijke, Biomolecules, 2019, 9, 743 CrossRef CAS PubMed.
- A. Ahmadi, M. Najafi, B. Farhood and K. Mortezaee, J. Cell. Physiol., 2019, 234, 12173–12187 CrossRef CAS PubMed.
- B. Zhang, K. Jin, T. Jiang, L. Wang, S. Shen, Z. Luo, Y. Tuo, X. Liu, Y. Hu and Z. Pang, Sci. Rep., 2017, 7, 1–12 CrossRef PubMed.
- M. Kanapathipillai, A. Mammoto, T. Mammoto, J. H. Kang, E. Jiang, K. Ghosh, N. Korin, A. Gibbs, R. Mannix and D. E. Ingber, Nano Lett., 2012, 12, 3213–3217 CrossRef CAS PubMed.
|
This journal is © The Royal Society of Chemistry 2023 |
Click here to see how this site uses Cookies. View our privacy policy here.