DOI:
10.1039/D2PY01538B
(Review Article)
Polym. Chem., 2023,
14, 1690-1717
Review of quantitative and qualitative methods for monitoring photopolymerization reactions
Received
8th December 2022
, Accepted 28th February 2023
First published on 31st March 2023
Abstract
Photopolymerization processes are currently one of the leading methods used in various areas of the chemical industry. Despite the many advantages and the simplicity of the light-initiated polymerization process itself, there are a number of parameters that are crucial to the performance and efficiency of the process, and that also affect economic or environmental specifications. As a result, the ability to quickly control the process in real time is essential. There are a number of techniques that enable the on-line control of photopolymerization, and this paper provides a broad overview of the available methods, both for laboratory and industrial use, that allow qualitative and quantitative analysis of the process. This review provides a description of each research methodology along with a description of the parameters that can be monitored and controlled using the technique.
1. Introduction
In recent years, the real-time monitoring of chemical reactions has become a priority in ensuring the quality of the final product and optimization of the production process.1–3 This trend is caused by the growing market and legal requirements regarding product quality and environmental protection.4 The polymer industry needs fast, reliable, non-invasive, and cheap methods to control the polymerization processes.5 Many industries use photopolymerization processes and the possibility of continuous monitoring of the reactions allows efficient management of the process, enables its temporal and spatial control, and improves the properties of the final products. Knowing the nature of the ongoing process is also an environmental and economic gain, which is an additional argument for the necessity of monitoring methods for rapid light-initiated polymerization processes. The ability to on-line monitor the process is especially used in the light-cured paint and adhesives industry.6 Another industry that puts a significant focus on monitoring the processes is additive technology, otherwise known as 3D-VAT printing. Process monitoring makes it possible to respond to changes occurring in the light-sensitive resin while printing and allows for optimization of the printing parameters to ensure the fastest possible printing, while maintaining excellent optical resolutions of the finished 3D material.7 Other industries that benefit from online monitoring of polymerization processes are also the biomedical industries, such as the tissue engineering industry or dentistry.
Monitoring of the polymerization reaction allows, among others, for quantitative studies of reaction kinetics, tracking molar mass changes and polymer molecular weight distribution, viscosity, degrees of branching, and many other parameters characteristic of polymer systems.8–10 It also enables the optimization of reaction conditions and a critical evaluation of existing hypotheses and kinetic models used to describe polymerization reactions. Traditional off-line monitoring methods have many limitations, including time delay, mainly due to sampling and time-consuming qualitative and quantitative tests, which result in a significant time interval between sampling and obtaining the results of their analysis. This phenomenon is particularly problematic when dealing with fast UV-initiated polymerization processes. Another problem is, e.g., different properties of the small sample taken for analysis, and the final product in bulk. Sampling also affects the quality and efficiency of the process and product due to the sensitivity of free radical polymerization to inhibitory agents such as oxygen.11 The frequently appearing term on-line monitoring means tracking and controlling reactions/changes occurring in the reaction system in real-time, continuously, and directly from the polymerizing medium. Such on-line characterization is possible due to the use of selective sensors/labels of chemical or physical probes, an appropriate reactor or attachment design for automatic sample analysis, and modern and advanced control technology. Currently, the availability of high-speed computers, explicitly dedicated to processing data from on-line and in situ measurements, as well as fiber-optic connection technologies, offers new possibilities for monitoring polymerization processes (Fig. 1).12,13
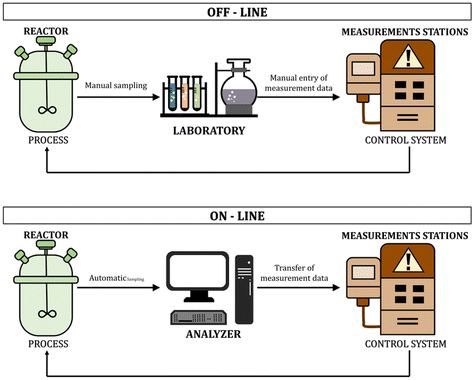 |
| Fig. 1 The idea of off-line and on-line measurements. | |
One of the key advantages of the photopolymerization reaction is its speed, which causes photopolymerization processes to occur as fast as ∼1000 kg mol−1 at a time of 5 min. During photopolymerization, many physicochemical parameters change, i.e., viscosity, polarity, and crosslinking density. Accurate tracking of the kinetics of changes occurring during ultrafast reactions is a difficult challenge when high-intensity radiation sources are additionally used in industrial practice. The overriding goal of monitoring the course of photopolymerization reactions, regardless of the mechanism, type of photoinitiator, light source, and other factors affecting the process is to determine the degree of conversion or another indicator of the reaction's progress in real-time. The amount of conversion achieved depends on many factors, among others the exposure time and intensity, composition, and homogeneity of the composition, ambient conditions (i.e., the inertia of the environment relative to the polymerizing coating), and temperature.14–17
The determination of the residue of unreacted monomer in the final product is particularly important in industrial production that is strictly regulated, among others by governmental organizations, as current quality standards require that the monomer content of the polymer is below 500 ppm and in some cases even 10 ppm.18,19 Hence, polymerization to high monomer conversion rates has become the dominant goal in optimizing polymerization processes, and developing methods to control and track the progress of polymerization reactions has become a way to achieve this goal. However, when describing methods of monitoring polymerization reactions in real-time, their usefulness should be separated depending on the scale of application (i.e., laboratory or industrial scale) due to completely different quality, quantitative and technical requirements.20–22
There are several requirements for monitoring the photopolymerization reaction in real-time. These methods must exhibit high sensitivity, as the measurement is carried out in thin layers with micron thicknesses (1–50 μm) and a high rate of data acquisition at short intervals, as the conversion of a liquid monomer into a cross-linked polymer can take just a few seconds. In addition, the repeatability and reliability of the results are essential features of all analytical methods (Fig. 2). Currently used real-time techniques for monitoring reactions differ in precision, sensitivity, speed, the complexity of the equipment and the amount of process data obtained per unit of time.23–25
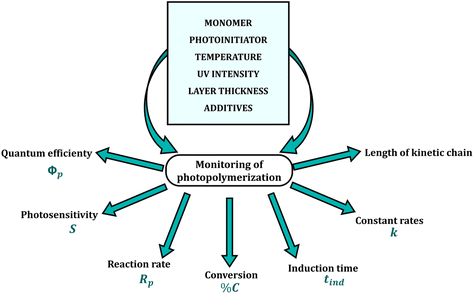 |
| Fig. 2 Key parameters and factors affecting photopolymerization kinetics. | |
Research methods, so far used to monitor the progress of photochemically initiated polymerization processes and the analysis of their kinetics, can be divided into two types:
• direct – based on the measurement of the amount of produced polymer or unreacted monomer;
• indirect – consisting of the measurement of changes in the physicochemical properties of the polymerizing composition, which are proportional to the degree of conversion of the monomer or the amount of the produced polymer26,27
In practice, there is also a division into periodical and continuous methods. Discrete (periodical) methods, provide results punctually, cyclically, at specified time intervals, or irregularly. Non-continuous methods include infrared spectroscopy (IR), photoacoustic spectroscopy (PAS), and gravimetry. These methods are useful for the determination of the rate of polymerization and the amount of unreacted monomer but are considered to be time-consuming and tedious methods that do not perform under industrial conditions.13,28
A valuable tool in monitoring photoinduced polymerization reactions is continuous methods, consisting of the real-time monitoring of chemical or physical changes under the influence of UV light. These include Fourier-transform infrared spectroscopy (FTIR), fluorescence spectroscopy, photodifferential scanning calorimetry (PDSC), dilatometry, interferometry, and radiometry. These methods provide both qualitative and quantitative information on the photopolymerization process, both in real-time and in situ.23,29
2. Quantitative methods for monitoring photopolymerization reactions
Nowadays, spectroscopic techniques (UV VIS, IR, NIR, FTIR, Raman) are the most useful to monitor the progress of the reaction both continuously and periodically. Furthermore, the combination of spectroscopic and optical fiber techniques brings new perspectives to in-process monitoring. Spectroscopy provides valuable information at the molecular level about the degree of reaction and product quality. The rapid development of electronics and information technology results in the design of spectroscopic equipment with increasing precision and sensitivity (i.e. with a high signal-to-noise ratio, necessary to detect even the smallest changes in the spectrum) and the appearance of so-called super-fast computers to perform complex calculations occurring in the multidimensional analysis. Spectroscopic techniques have several advantages over other traditional laboratory techniques, such as high speed and the reliability of measurements, fast and easy sample preparation, a multitude of analyses in one operation, and a non-destructive character. It should be noted, however, that spectroscopic techniques cannot be used as ad hoc monitoring techniques and therefore calibration and validation of the obtained experimental data are necessary, which requires chemometric methods to calibrate the measuring equipment.12,30,31
2.1. Fluorescence probe technology
The fluorescent probe technique (FPT) is classified as a non-destructive technique for monitoring polymerization processes in real time continuously, providing both qualitative and quantitative parameters. Appropriately selected in terms of stability and sensitivity, the fluorescent probe provides information on the progress of the reaction directly from the polymerization medium. This technique, due to its high sensitivity and time resolution of measurements, is particularly dedicated to monitoring fast photopolymerization processes.32–36
2.1.1. Principle of FPT technique.
FPT technology is based on the process of absorption of a specific wavelength by the fluorescent probe molecule used in the experiment and analysis of the resulting emission spectrum using a spectrophotometer. Monitoring of the changes occurring in the reaction environment is possible when the fluorescent probe is added to the polymerizing composition in a very small amount ranging from 0.01–0.1 wt%.37,38 The light source from the measurement system excites the probe molecule to higher energy levels, and the changes in polarity and microviscosity that occur during the polymerization process affect the stabilization of the excited states to varying extents.32,39 The effect of these changes can be a change in fluorescence intensity, a change in the position of the fluorescence spectrum of the probe, or a change in the shape of the spectrum (Fig. 3). The resulting kinetic profile of the polymerization reaction, with a typical sigmoidal shape, clearly represents each of its stages, i.e. the initial section of the curve corresponding to the induction time (tind), followed by the start of the actual monomer polymerization process (i.e. the section of the curve where a marked increase in the reaction rate occurs is the same as the increase in the polymer structure), and the so-called plateau, i.e. the flat range of the curve marking the end of the polymerization process (Fig. 3). The limitations of this method are also an important issue. They involve the need to select the appropriate probe in terms of emission characteristics so that it does not overlap with the other components of the composition, as well as have the appropriate sensitivity to a specific changing parameter during the polymerization process. In addition, the sensors should be characterized by high photostability, which in the case of unstable sensors falsifies the results. Another important parameter to pay attention to is the degree of homogeneity of the composition. The sensor only reacts to changes in its microenvironment. For this reason, the composition containing the sensor should be mixed as well as possible to ensure an even distribution of the sensor throughout the volume.
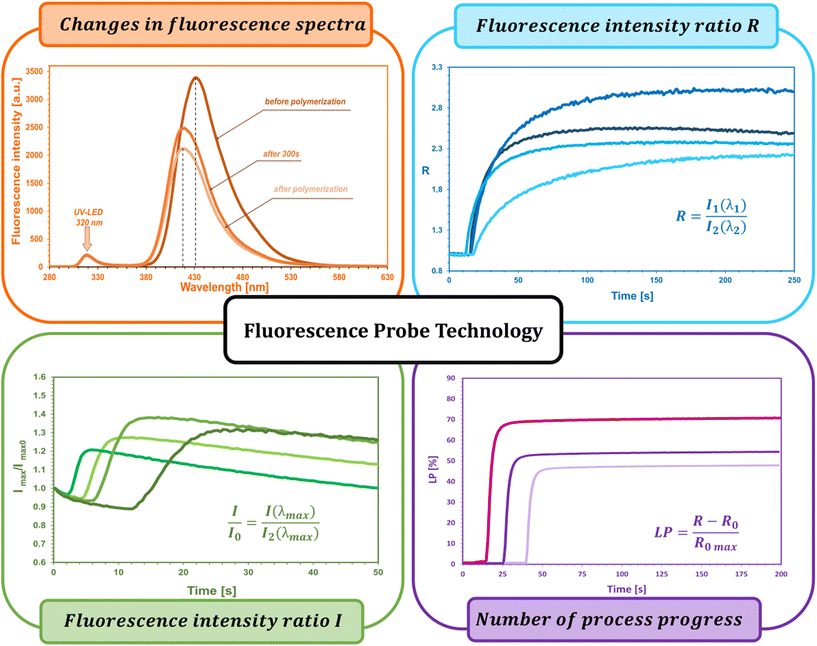 |
| Fig. 3 Characteristic parameters determined by the FPT method. | |
2.1.2. Parameters of the FPT method and their importance in photopolymerization processes.
In FPT technology, at least one of the parameters characterizing fluorescence must change according to changes in the environment. The basis of the presented FPT technology is to measure changes in the fluorescence characteristics of the fluorescent probe as the properties of the environment in which it is placed change. Examples of fluorescent probes used to monitor photopolymerization reactions include (Coumarin 1), 4-dimethylamino-4′-nitrostilbene (DMANS), 6-propionyl-2-(dimethylamino)naphthalene (Prodan) or 2(4-(4-(dimethylamino)phenyl)1,3-butadienyl)-3-ethylbentzothiatzole p-toluenesulfonate (Styryl 7), whose structures are shown in Fig. 4c.40–42 Among the parameters by which changes in the environment can be correlated with the fluorescence phenomenon are the monomer/excimer fluorescence intensity ratio, the wavelength corresponding to maximum emission (λmax), the intensity at maximum emission I(max), the fluorescence lifetime (τ) and the emission wavelength range. The choice of the appropriate parameter to best reflect changes in the reaction environment depends on the physicochemical properties of the system under study.
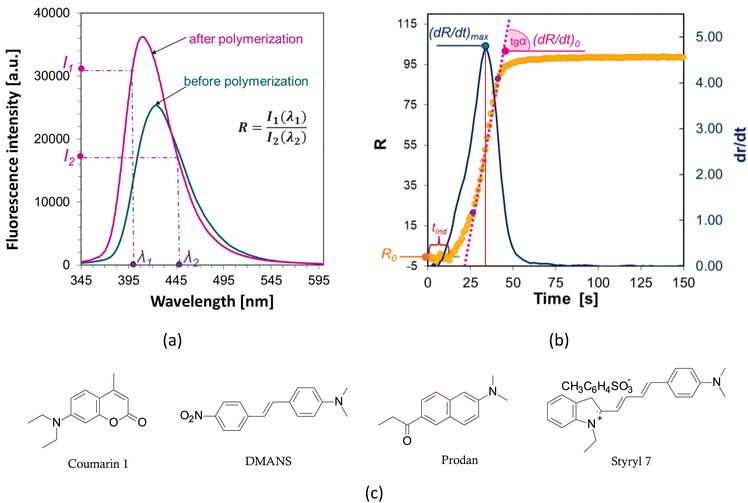 |
| Fig. 4 Graphical demonstration of how to determine key parameters, using the FPT method. (a) Fluorescence spectra of the probe before and after polymerization. (b) The polymerization progress rate R, values of induction times tind and degrees of the slope of kinetic profiles tgα. (c) Examples of common fluorescent probe molecules. | |
The kinetic parameters obtained directly from measurements and the kinetic profile in the FPT technique are the ratio of fluorescence intensity (R) at a given moment in time (t), initial fluorescence intensity ratio (R0), induction time (t0), and the slope of the kinetic curve (dR/dt)0. All the parameters are described in detail below and shown in Fig. 3.
The fluorescence intensity ratio (R) is usually measured as the ratio of the intensity (I1) at a shorter wavelength (λ1) to the intensity (I2) at a longer wavelength (λ2) (Fig. 4a). This ratio is an indicator of the changes in the position of the probe's fluorescence spectrum along the wavelength axis. As the spectrum shifts toward shorter wavelengths as polymerization progresses, the R-value increases and is independent of the probe concentration or geometric dimensions of the sample. As polymerization progresses, with the appropriate choice of wavelengths λ1 and λ2, the R parameter can be considered as a quantitative guide to the degree of conversion of the monomer's functional groups, which has been repeatedly confirmed by experimental studies through a linear correlation with the degree of curing.43,44 The FPT profiles, presented as R = f(t), can be converted into kinetic curves showing the conversion of the monomer as a function of time C = f(t) using appropriate calibration factors determined relative to the actual conversion values. Calibration can be done by correlating the rate (R) with the conversion (C), e.g., using a polynomial:32
| R = R0 + Aα + Bα2 + Cα3 + … + Nαn | (1) |
where:
R – fluorescence intensity ratio measured in real-time,
R
0 – initial fluorescence intensity ratio measured before polymerization,
α – conversion of functional groups in the monomer (determined, for example, by the RT-FTIR technique),
α
n
– coefficients of the polynomial determined empirically.
The use of the fluorescence intensity ratio (R) to monitor the course of polymerization in real time eliminates the effect of variations in the fluorescence intensity of the probe, arising from external factors such as changes in the intensity of the probe excitation light source, the distance of the sample from the measuring head (e.g., as a result of sample vibration), or the concentration of the probe (e.g., as a result of its bleaching). Nevertheless, the range of applicability of the R parameter also has its limitations. Namely, depending on the type of probe used and the type of process monitored, the probe may be unsuitable for monitoring changes in its environment due to, for example, photolysis, photoisomerization, photochemical side reactions of the probe with components of the monitored system, or its non-specific interactions with neighboring molecules.45 As a result of probe side reactions, it is occasionally possible to observe a drop in the kinetic profile at the end of the monitored process, caused, inter alia, by an overlap of the probe photolysis by-product spectrum with the probe fluorescence spectrum used for monitoring, which affects the intensity values measured at one of the fixed wavelengths. An approach to such a situation is to use the parameter of normalized fluorescence intensity as an indicator of the progress of polymerization, i.e. I/I0, where I is the fluorescence intensity of the probe at a given moment, and I0 is the intensity measured before the beginning of the reaction. The normalized fluorescence intensity method is particularly useful in cationic photopolymerization, where the probe molecule happens to be protonated by acid generated by the photodissociation of the cationic photoinitiator. Protonated probe molecules exhibit different fluorescence characteristics than the unprotonated form, which sometimes disturbs the kinetic profile observed using the R parameter, and can result in longer polymerization induction times.46,47
Another parameter used in the FPT technique is the initial fluorescence intensity ratio (R0), which is measured before the polymerization reaction begins and the fluorescence spectrum of the probe is scanned continuously. The measurement is based on collecting a single scan of a recent composition. This parameter is a qualitative parameter and can be an indicator to identify the composition and “freshness” of photo-curable compositions.32,45 Namely, small changes in the measured value of R0 can be evidence of a change in its composition or spontaneous partial polymerization due to inadequate storage conditions, transportation, or deliberate action by the manufacturer to falsify the formulation. By measuring R0, it is easy to detect whether a new supply of a photocurable resin formulation meets the range of expected repeatable delivery.
Another important parameter is the induction time (tind), which is the time that elapses from the start of exposure of the composition to the start of polymerization. This quantity is a measure of monomer purity, which is proportional to the concentration of inhibitors and stabilizers dissolved in the monomers and inversely proportional to the concentration of the photoinitiator.48,49 Most monomers or coating formulations have a so-called “shelf life”, which is defined as the maximum storage time before polymerization begins. Reactive monomers usually contain a small amount of inhibitors to prevent spontaneous polymerization. To extend the life of the monomer, more inhibitor is added. Also, oxygen from the air, which diffuses into the monomer, is an effective inhibitor of radical polymerization.50–54 Hence, measuring the induction time gives a quick assessment of the purity of the composition. In addition, it has also been found that increasing the temperature can increase the induction time, because as the temperature increases, the viscosity of the composition decreases, thus facilitating the penetration of inhibitory oxygen molecules. A comparison of induction times can also be useful in comparing the quantum yield of photoinitiation by different photoinitiators for the same composition and at the same initiator concentration.55,56 This approach is justified by the lower or higher efficiency of the photoinitiator's generation of reactive radicals capable of consuming oxygen dissolved in the monomer.
The slope of the curve at the initial stage of polymerization (dR/dt)0 is a measure of the maximum rate of photopolymerization, which occurs soon after its start and depends on the concentration of photoinitiator and its initiation efficiency. A photopolymerization rate that is too high can be reduced, for example, by reducing the photoinitiator concentration. If the initial rate is too low, by adding more photoinitiator, the polymerization process can be accelerated. With the same irradiation conditions and identical concentrations of the series of photoinitiators tested, the relative initiation efficiency (Ereli) for each can be determined, allowing quantitative studies of the efficiency of the photoinitiation step.32,57,58 The slope of the (dR/dt)0 curve is usually determined at the intercept point of the kinetic profile as follows (Fig. 4b):
• the sigmoidal curve of photopolymerization (yellow curve in Fig. 4) is transformed into its first derivative (blue curve),
• the position of the inflection point on the kinetic curve is determined based on the position of the maximum of the first derivative,
• determination by the method of least squares fits the equation of a straight line, a tangent to the kinetic curve at its inflection point (pink line), the value of the slope of which is the sought value of (dR/dt)0. To determine the parameters of the tangent, the points on either side of the inflection point that form a straight segment on the kinetic curve are taken.
An example set for FPT is shown in Fig. 5.
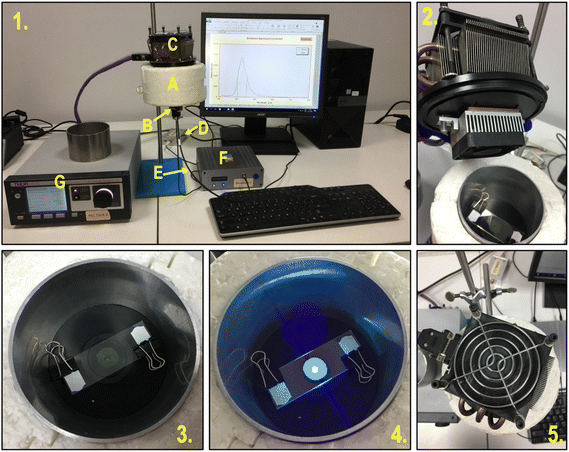 |
| Fig. 5 Measurement stand for monitoring the kinetics of photopolymerization by the FPT technique under isothermal conditions. 1. A – thermostatted measuring chamber; B – measuring head where the UV-LED diode was placed and the fiber optic cable connected; C – thermostatic head with Peltier module; D – power supplies for diodes with emission wavelengths of 320 nm or 365 nm, 469 nm, 501 nm, 595 nm, respectively; E – fiber optic cable; F – miniature spectrometer EPP2000C made by StellarNet Inc. (range: 200–700 nm); G – thermostatic head controller with temperature control in the range of 10–65 °C. 2. The appearance of the thermostatic head from the bottom. 3. The sample with a drop of the tested composition placed in the chamber. 4. The sample with a drop of the tested composition placed in the chamber and spotlighted with light from an LED. 5. The appearance of the thermostatic head from the top. | |
2.2. Fourier-transform real-time infrared spectroscopy (RT-FTIR)
The high potential of infrared spectroscopy in monitoring the kinetics and degree of conversion during the progress of the photopolymerization reaction is confirmed by numerous scientific publications.59–65 IR spectroscopy provides different measuring techniques for spectral registration depending on the optical properties and physical state of the sample, e.g., transmission, reflectance (ATR, HATR), and transflectance (DRIFT) techniques. In the case of rapid radical photopolymerization reactions of compositions containing acrylate and methacrylate monomers, two techniques are most commonly used:
• FTIR for performing routine, single measurements;
• RT-FTIR for real-time and in situ monitoring of the monomer conversion rate.
In addition, when curing liquid samples in the form of thin layers, with the application of various UV radiation sources, the ATR technique is used, which allows thin layers of the composition to be applied to the surface of the diamond crystal and exposed from different distances and angles. However, each of these techniques requires the use of special, expensive materials with the appropriate optical density and IR-beam permeability (e.g., KBr, FB2, CaF2, NaCl) that, when cured, are usually destroyed.66–70
Fourier-transform real-time infrared spectroscopy (RT-FTIR) has found particular appreciation in the quantitative monitoring of photopolymerization reaction progress and is widely regarded as an effective and universal method for determining the degree of conversion over time, reaction rate, and quantum efficiency of photoinitiation and polymerization under varying conditions. The high importance of the RT-FTIR technique has been proved by numerous studies in photopolymerization systems.71–78
RT-FTIR spectroscopy, according to numerous literature sources, has been and remains a useful technique for studying the kinetics of photopolymerization reactions according to radical, cationic, and hybrid mechanisms, in addition to various exposure conditions, types of light source used, coating thickness, monomer and oligomer reactivity or efficiency of newly developed photoinitiating systems.79–85
For free-radical photopolymerization, RT-FTIR measurement consists of repeated scanning of the sample at short intervals and observing in real-time changes in the IR absorption spectrum, for example, changes in both the intensity and position characteristic of (meta)acrylic groups, i.e.:86–89
• a band at 810–816 cm−1 characteristic of a twisting vibration of the –CH
CH2 group,
• a band at 1405 cm−1 characteristic of a bending vibration of the
CH2 group,
• a band at 1620–1635 cm−1, characteristic of a stretching vibration of the C
C group, which additionally influences the growth and shift towards larger wavelengths (i.e., up to 1700–1750 cm−1) of the oscillation of the carbonyl group C
O, to which these bonds are conjugated, as a result of the gradual disappearance of double bonds C
C during the polymerization process.
The crucial parameters determined by the RT-FTIR method are the conversion (%CFTIR) and reaction rate (Rp). The conversion rate is calculated as the ratio of the peak height or area at a given time of the reaction to the initial peak height or area before the polymerization process begins (eqn (2)), whereas the rate of polymerization is the result of the differentiation of the conversion curve from the reaction time (Fig. 6).
| 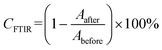 | (2) |
where:
CFTIR is the conversion of a given monomer/functional group of a monomer, determined using the real-time FTIR technique,
Aafter is an area of the absorbance peak characteristic of a given monomer at the end of the photopolymerization process, and
Abefore is an area of the absorbance peak characteristic of a given monomer at the beginning of the photopolymerization process.
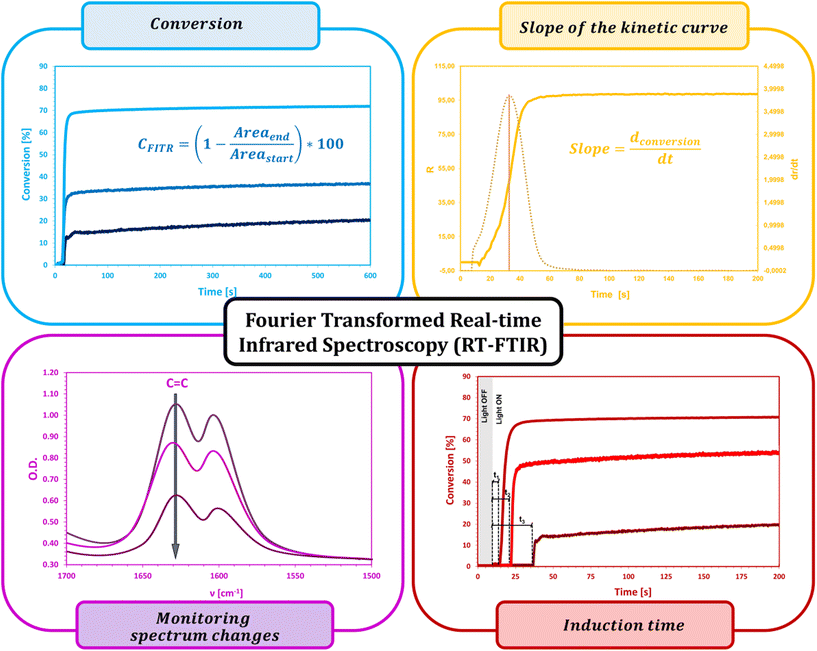 |
| Fig. 6 Characteristic parameters determined by the RT-FTIR method. | |
In this technique, due to the high time resolution, on-line measurements can be carried out on both laboratory and industrial scales in the coating and printing processes. The high measurement sensitivity allows the detection of extremely small changes in monomer concentration.90–94 One of the unique features of RT-FTIR spectroscopy is the ability to evaluate the amount of unreacted functional groups (residual monomer) trapped in a three-dimensional polymer network formed from multifunctional monomers and linear polymers with a high glass transition temperature (Tg). Compared with other methods, RT-FTIR provides an accurate and fast evaluation of the essential kinetic parameters of the reaction – even during so-called dark photopolymerization, after the removal of the radiation source. Despite the numerous advantages of RT-FTIR, this method is not suitable for samples containing IR beam-scattering pigments, and the film thickness, which should be in the range of 1–100 μm, is also a limiting factor (Fig. 6).95–99 Therefore, this method is only applicable to thin films, so when using RT-FTIR, it is not possible to obtain information, e.g., about the kinetics of rheological changes in the composition, changes in volume due to the expansion or contraction of the polymer network or changes in crosslinking density along with the sample thickness profile. Hence, RT-FTIR spectroscopy is often combined with other techniques in so-called combined techniques, i.e., rheological measurements,100–102 dielectric analysis (DEA),103–106 fluorescence spectroscopy,107–110 photocalorimetry (DSC),111–113 thermogravimetry (TGA) and others.114–117
ATR-FTIR (attenuated total reflectance Fourier-transform infrared) spectroscopy has also been utilized to successfully examine the conversion of the reaction and composition during polymerization monitoring. It is a contacting sampling technique that uses a crystal with a high refractive index and low IR absorption in the target IR region as an internal reflection element (Fig. 7). When the IR beam enters the internal reflection element below an angle that exceeds the critical angle for total internal reflection, an evanescent wave is set up which penetrates a small distance beyond the crystal surface into space. It is possible to identify the infrared spectrum of a sample by bringing it into contact with the crystal, where it will interact with the evanescent wave and absorb infrared radiation. The absorbance of the sample attenuates the evanescent wave. For the evanescent wave to enter the sample, there must be close contact between the sample and the crystal. The evanescent wave is appropriate for the quantitative investigation of small samples since the range of its penetration depth is typically 1 to 10 mm. Due to its insensitivity to strongly absorbing substances (like water) and sample thickness, ATR spectroscopy is a potent tool. Additionally, when acquiring structural and kinetic data on a polymerization process in real-time, ATR-FTIR can provide a wealth of information about the reaction system at the molecular level. For a range of systems, including the batch homopolymerizations of butyl acrylate, methyl methacrylate, and vinyl acetate as well as styrene/1,3-butadiene emulsion copolymerization, the use of ATR-FTIR for monitoring polymerizations has been reported.118–123
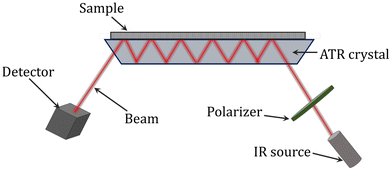 |
| Fig. 7 Schematic representation of an ATR-FTIR system. | |
2.3. Raman spectroscopy
Compared with infrared spectroscopy, Raman spectroscopy is based on the emission process associated with the effect of scattering of photons by vibrations of the particles of the examined sample occurring as a result of changes in polarizability (i.e., deformation of the distribution of the particle charge by an external electric field). It was first predicted by Smekal in 1923 and observed experimentally by Raman and Krishnan in 1928.124,125 Raman spectroscopy has several advantages over IR spectroscopy, e.g., the ability to study lower frequency vibrations, and has a higher spatial resolution due to the greater penetration of the light wavelength used in Raman spectroscopy into the sample. However, the Raman technique is slower and more expensive. Despite this, Raman spectroscopy is one of the most popular techniques, within which can distinguish more than 25 different types of known Raman spectroscopy technique, such as, for instance, hyper-Raman scattering, Raman-induced Kerr effect spectroscopy, stimulated/coherent Raman scattering, spontaneous Raman, and Fourier-transform Raman scattering.126,127 Nevertheless, infrared spectroscopy and Raman spectroscopy are considered to be complementary techniques.128–130
The polarizability of the electrons in a molecule corresponds with the intensity of the Raman effect. The molecule is briefly in a virtual energy state as a result of this excitation before the photon is released. When inelastic scattering occurs, the energy of the photon that is emitted is either lower or higher than the energy of the photon that was incident. The sample is in a changed rotational or vibrational state following the scattering event. After the molecule switches to a new rovibronic (rotational-vibrational-electronic) state, the scattered photon shifts to a different energy and, consequently, a different frequency in order for the system's total energy to stay constant. The energy difference between the molecule's first and final rovibronic states is the same as this one. The scattered photon will be moved to a lower frequency (lower energy) if the final state has more energy than the original state, maintaining the overall energy constant. A Stokes shift, sometimes known as a downshift, denotes this change in frequency. The dispersed photon will undergo an anti-Stokes shift, also known as an upshift, if the end state has lower energy. A change in a molecule's electric dipole–electric dipole polarizability with respect to the vibrational coordinate corresponding to the rovibronic state is required for the molecule to display a Raman effect. This polarizability shift affects how strongly the Raman scattering occurs. As a result, the rovibronic states of the molecule determine the Raman spectrum, which is the scattering strength as a function of the frequency shifts.131–133
| 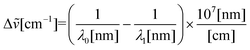 | (3) |
where Δ
![[small nu, Greek, tilde]](https://www.rsc.org/images/entities/i_char_e0e1.gif)
is the Raman shift expressed in wavenumber,
λ0 is the excitation wavelength, and
λ1 is the Raman spectrum wavelength.
The Raman effect is based on the interaction of an electron cloud in a sample with the external electric field of monochromatic light. Depending on the polarizability of the molecule, this interaction might cause a dipole moment within the molecule. There cannot actually be a change in energy level because the laser light does not stimulate the molecule. The emission (fluorescence or phosphorescence) of a molecule in an excited electronic state emitting a photon and returning to the ground electronic state, frequently to a vibrationally excited state on the ground electronic state potential energy surface, should not be confused with the Raman effect. Additionally, IR absorption, where the energy of the absorbed photon corresponds to the energy discrepancy between the initial and final rovibronic states, differs from Raman scattering.134–136
Raman differs from IR spectroscopy in that it depends on the electric dipole–electric dipole polarizability derivative rather than the electric dipole moment derivative or the atomic polar tensor (APT). Raman spectroscopy can be used to investigate rovibronic transitions that might not be visible in the infrared, as demonstrated by the law of mutual exclusion in centrosymmetric molecules. Large Raman intensity transitions frequently have weak IR intensity transitions, and vice versa. If a bond is highly polarized, polarization is only slightly affected by a minor change in the bond's length, such as that caused by vibration. Therefore, polar bond vibrations (such as those between C–O, N–O, and O–H) are relatively weak Raman scatterers. However, because such polarized bonds carry their electrical charges during the vibrational motion (unless they are canceled out by symmetry factors), the net dipole moment change is greater, which results in a stronger IR absorption band. On the other hand, relatively neutral bonds (such as C–C, C–H, and C
C) experience significant polarizability changes during a vibration. Although vibrations involving primarily this sort of link are significant Raman scatterers, they are feeble in the IR because the dipole moment is not similarly impacted. Inelastic incoherent neutron scattering (IINS), a third vibrational spectroscopy method, can be utilized to identify the vibrational frequencies of highly symmetric molecules that may be both IR and Raman inactive. The three techniques are complementary because the IINS selection rules, or allowable transitions, differ from those of IR and Raman. For a particular vibrational transition, they all yield the same frequency, but the relative intensities convey different information since the molecule interacts with the entering particles differently depending on whether they are photons for IR and Raman or neutrons for IINS.127,131,137
Chemistry uses Raman spectroscopy to identify molecules, investigate chemical bonding, and examine intramolecular interactions. Since a molecule's chemical bonding and symmetry determine the vibrational frequencies that are produced, Raman offers a fingerprint that can be used to recognize substances. Raman spectroscopy is used in solid-state physics to characterize materials, gauge temperature, and determine a sample's crystallographic orientation. Similar to single molecules, distinctive phonon modes can be used to identify a solid material. The ratio of the Stokes and anti-Stokes intensities of the spontaneous Raman signal provides information on the population of a phonon mode. Other low-frequency excitations of a material, like plasmons, magnons, and superconducting gap excitations, can also be seen using Raman spectroscopy.138,139
Numerous uses of Raman spectroscopy may be found in biology and medicine. It has encouraged research into low-frequency collective motion in proteins and DNA and their biological activities, helping to demonstrate the existence of low-frequency phonons in proteins and DNA. For tissue imaging with SERS-labeled antibodies, olefin or alkyne-modified Raman reporter molecules are being produced. Another non-invasive method for the in situ, real-time biochemical evaluation of wounds has been Raman spectroscopy. The creation of a numerical indicator for the advancement of wound healing was made possible by the multivariate analysis of Raman spectra.140–142
Raman spectroscopy also found use in polymer analysis in a lab environment or on a process line. By the Raman spectrum, it is possible to follow the degree of polymerization. Polymers tend to “orient” when extruded. In other words, the molecular axis lines up with the direction of extrusion. One can infer information about the orientation of the polymer in the component by orienting the sample in the coordinate system of the instrument and examining the polarization of the Raman light. The mechanical and other physical properties of the polymer may be affected by this.141,143–145
2.4. Near-infrared spectroscopy
Near-infrared spectroscopy (NIR) has also found recognition in monitoring the progress and kinetics of curing and crosslinking reactions. This technique is also used in the control of industrial processes as it has sufficient time resolution and high measurement sensitivity as well as a comprehensive and versatile analytical potential.146–149 Furthermore, effective analysis of the recorded data stream can be supported by advanced chemometric methods. Dedicated NIR analyzers, supported by multichannel detectors, are compact, robust, and relatively inexpensive. In addition, they are usually equipped with optical fibers, which allow the spectrometer and measuring head to be spatially separated, allowing the device to be easily integrated into a working production line. The high time resolution and high sensitivity of the measurement, non-destructive character, simplicity, and speed of analysis are satisfactory features for monitoring conversions in fast photopolymerization reactions, with the additional advantage of inexpensive and reliable spectrophotometers.150–156 However, in quantitative terms, the NIR technique requires the use of chemometric methods, due to the multidimensionality of data, photometric noise, and significant influence of many external factors such as the temperature, humidity, thickness, and optical properties of the sample on the shape and intensity of NIR spectra. In addition, when testing thin films, the molecular absorption coefficients in near-infrared are quite small, requiring a larger sample volume to obtain an unintended signal.157–166
The basic analysis of the reflectivity suitability of NIR spectroscopy for on-line monitoring of the conversion rate of very thin, both transparent and pigment-containing, acrylate coatings on various substrates (i.e., PP film, paper, wood, MDF) was performed by Scherzer et al.30,167,168,311 This technique can be successfully used to monitor the conversion of double acrylate bonds in thin layers around a few μm under UV exposure, both on a laboratory scale and on a semi-technical coating line.169–171 In addition, a very good linear correlation has been found between the results of the NIR technique and those of the FTIR spectroscopy and the HPLC technique for the determination of monomer conversions, although chromatographic methods show higher conversion values than spectroscopic methods.30,168,172
2.5. Photoacoustic spectroscopy
Photoacoustic spectroscopy (PAS), also called optoacoustic spectroscopy, classified as photothermal spectroscopy, is a non-invasive and non-destructive technique for monitoring changes in the thermal and optical properties of a material as a result of energy absorption.173–175 The possibility of using any source of monochromatic radiation (e.g., IR, UV-VIS) makes it an attractive technique for investigating changes in a sample at the molecular level. During measurement, the modulated radiation intensity interacts with the absorbing sample, and a photoacoustic signal is generated that is proportional to the absorbance of the sample.175–177 Radiation-free relaxation processes generate a heatwave of the same frequency as the incident modulated radiation. The periodic change of temperature on the sample surface generates acoustic waves in the gas atmosphere, which are detected by a microphone or other piezoelectric detector.177–179 Since the signal intensity is directly related to the absorbance of the coating, UV-VIS or IR spectra can be recorded, depending on the type of selected incident radiation.175,177 Compared with other techniques, e.g., IR, PAS has a lower measurement sensitivity, but in combination with FTIR or fluorescence spectroscopy, it is a reliable and accurate tool for the quantitative monitoring of photopolymerization kinetics. The limitation of PAS in quantitative analysis is the need to define a reference peak because the intensity of the photoacoustic signal depends on the depth of penetration and heat diffusion in the composition layer.173,180
The PAS technique is particularly suitable for evaluating the cure along with the thickness profile of the coating, which is difficult to analyze by other techniques, in particular with regard to the thickness of paint and varnish samples. The intensity of the photoacoustic signal also depends on the amount of heat emitted during the photoinitiated polymerization process, which is related to the rate of polymerization, which allows the analysis of the process kinetics.173,177,181 This technique has proved itself in the study of UV or electron beam (EB) cured acrylate coatings, where monomer or photoinitiator degradation was monitored, in the study of the kinetics and degree of polymerization of dental resins and epoxy-graphite composites. PAS also provides information on the energy state and quantum efficiency of the excited state of the molecule and the lifetime of intermediate products in photochemical reactions.173,175,182
2.6. Photodifferential scanning calorimetry
A widespread method of quantitative monitoring of photoinduced polymerization processes in real-time is one of the thermal analysis methods – differential scanning calorimetry (PDSC). Isothermal measurement of the amount of heat released as a function of time (ΔH in W g−1), in an inert gas atmosphere during the photopolymerization reaction, is often used in the study of process rate (Fig. 8). At a constant thermal capacity of the polymeric composition, the degree of transformation of the monomer into the polymer is proportional to the amount of generated heat if no other events like crystallization proceed. The heat formed is a report of the entire sample in which the light shines.183,184 The usefulness of this technique for polymerization monitoring is due to the exothermic nature of the monomers to polymer reaction. Mole enthalpy polymerizations of typical monomers are in the range 50–200 kJ mol−1 and acrylate monomers in the range 78–86 kJ mol−1.184,185
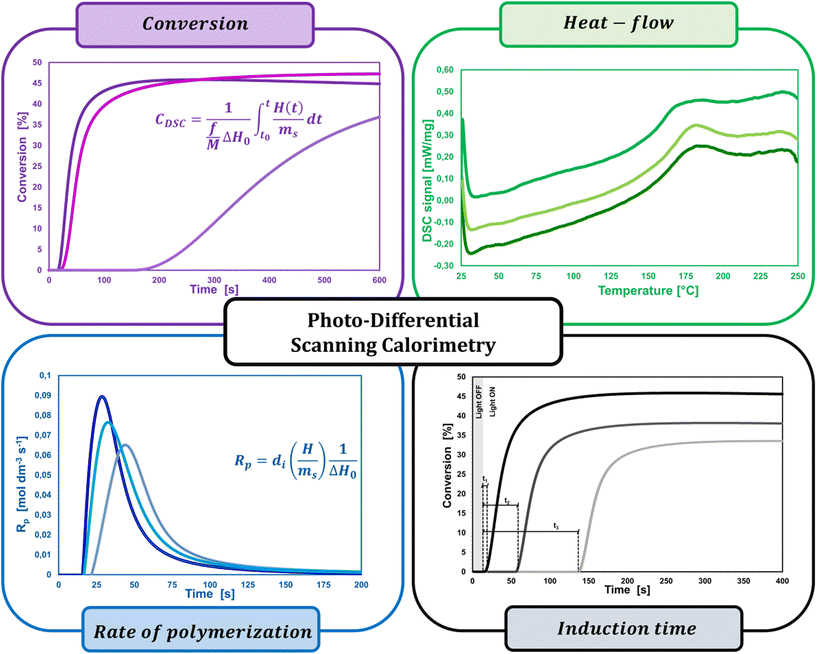 |
| Fig. 8 Characteristic parameters determined by the differential scanning calorimetry method. | |
Photo-DSC measurements are used to study the kinetics of photopolymerization, and to determine the influence of physicochemical factors such as monomer structure, temperature, light intensity, and inhibitor concentration on its mechanism and efficiency.16,186 Photo-DSC temperature studies were used to investigate the diffusion phenomena of polymer chain segments and auto-acceleration or auto-deceleration phenomena in polymerizing acrylate systems.16,30,187 These phenomena are related to the dynamic in the reaction environment, which is limited due to diffusion processes, the viscosity of the polymerizing system, and sterile obstacles (Fig. 8). As the temperature increases, the viscosity of the system decreases, increasing the mobility of the radicals.16,188 The Photo-DSC is a useful tool for determining different states and transitions in polymers and soft matter in general. Important parameters from photo-DSC measurements include the reaction heat and heat transfer coefficient. These values, in combination with numerical methods and appropriate algorithms, allow us to calculate among others: the total monomer conversion rate (CDSC), maximum polymerization rate (Rp DSC), inhibition time (tind), and activation energy (Ea) (Fig. 8).30,184,189 In addition, a lot of research has been done to prove the feasibility of determining the ratio of propagation to termination rate constants (kp/kt) using the photo-DSC technique. A detailed analysis for these two kinetic parameters has been widely discussed by Jakubiak and Rabek.190
The main parameters determined with this technique, in addition to the heat change during the reaction given directly from the experiment, are the rate of photopolymerization (Rp DSC) and the conversion of the reacting monomer/monomers (CDSC). The mentioned parameters can be calculated by applying the following formulas (4) and (5):117,191
| 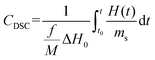 | (4) |
where:
ms is a mass of the sample,
M is the molecular mass of the monomer,
f is the functionality of the monomer, and Δ
H0 is the enthalpy of the polymerization of the given functional group.
| 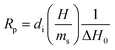 | (5) |
where:
ms is the mass of the sample,
M is the molecular mass of the monomer, Δ
H0 is the enthalpy of the polymerization of the given functional group,
di is the density of i-composition, and
H is a heat flow.
Although the PDSC technique makes a significant contribution to the quantitative and qualitative monitoring of photopolymerization reaction kinetics, it also has its limitations and disadvantages. Photocalorimetry is characterized by long response times due to the relatively low thermal conductivity of the sample, which limits the time resolution of the apparatus. In the case of fast photopolymerization reactions, the heat dissipation efficiency of the polymerizing sample per unit of time through the device is too low.186,192,193 Reliable data can only be obtained for systems where the maximum curing speed occurs after at least 5 seconds of curing, with a total curing time of not less than 1 minute. This limitation requires the use of UV radiation, which is two or three orders of magnitude less intense than in practical systems, and consequently requires action in anaerobic media, as the inhibiting effect of oxygen becomes more visible at low light intensity. In addition, the thickness of the sample layer weighed into the DSC crucible is never reliable, because, in the case of, e.g., low-viscosity (meta)acrylate monomers, the monomer does not cover the entire surface of the aluminum crucible uniformly, but flows down to the edges of the crucible under surface tension forces. Therefore obtained measurement data are not always verifiable and repeatable. Furthermore, due to the small sample size for PDSC (approx. 1 mg), it is not possible to measure some important composition properties after curing, such as the hardness, flexibility, and gloss.186,194 A lot of work has been devoted to the determination of protocols allowing for a fast and most precise investigation of the photopolymerization processes of multicomponent polymer compositions by the team of Bachmann et al.195
The PDSC method was used to study the kinetics of photopolymerization depending on the function of the monomers and oligomers, concentration and type of photoinitiator, UV light intensity and wavelength, temperature, type of gas surrounding the sample, and sample thickness. PDSC is not limited to the curing of (meta)acrylate monomers but also applies to thiol-ene systems, hybrids, unsaturated polyesters, and cationally polymerized epoxy resins. It can also occur in combination with RT-FTIR tandem and fluorescence spectroscopy.30,196,197
2.7. Photorheology
During photopolymerization or photocuring processes, there are rapid changes in the rheological behavior of uncured resin that can be measured with the help of photorheology. Without influencing or harming the target sample, rheological characteristics can be assessed in situ by applying small-amplitude, sinusoidal deformations while also being exposed to light. When combined with RT-FTIR, this method makes it possible to track changes in the modulus as well as light-induced sol-to-gel transitions, which can be used to connect mechanical qualities to the degree of chemical conversion (Fig. 9).191,198,199
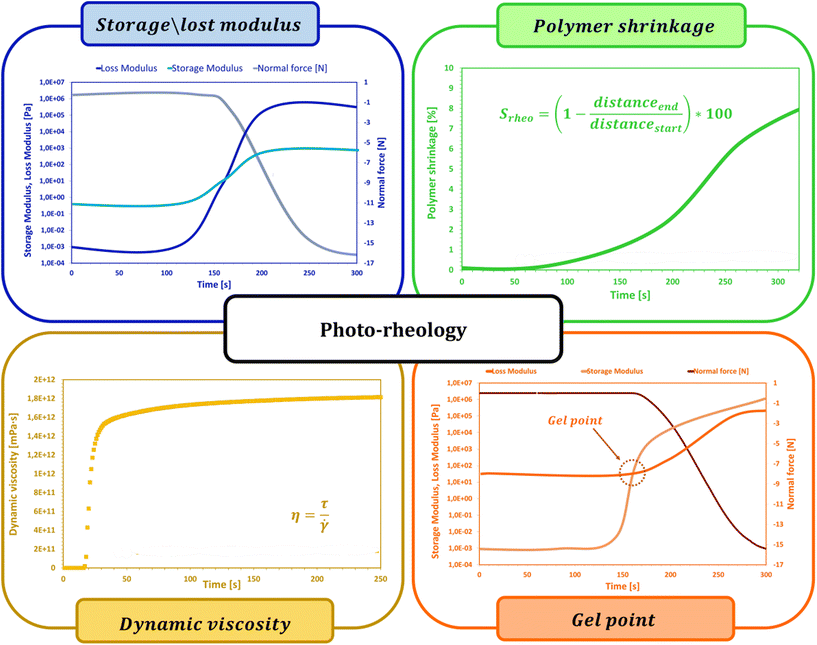 |
| Fig. 9 Characteristic parameters determined by photo-rheology. | |
Khan et al. conducted one of the earliest chemorheology studies on UV-curing thiol–ene complexes.200 To maintain the curing rate low enough for the rheometer to record any changes in rheological properties, single UV pulses were used in this study. Further research revealed that this technique could monitor the formation of structures in UV polymerizing resins and determine the gel point.201,202 Again, because of the modest UV intensity used in this research (0.2 mW cm−2), the modulus build-up was somewhat gradual, increasing by five orders of magnitude in just 500 seconds. The photorheology was improved by Lee et al. to explore the rapid curing of a polyester acrylate with much higher intensities (15 mW cm−2).203 Lee et al. developed a method whereby the input strain signal and the output stress signal were recorded externally, separated into small time intervals (0.2 to 0.5 cycles of stimulation), and fitted with sinusoidal functions because the sampling rate of the equipment was restricted to approximately 1 s. Thus, the phase shift between stress and strain as well as the storage and loss moduli were computed. With a time precision of up to 50 data points per second, they were able to track a rise in the complicated viscosity of three orders of magnitude in just five seconds. However, this method is insufficiently sensitive to analyze monomers with viscosities less than roughly 100 Pa s.
To integrate mechanical data with chemical knowledge, Steeman's team204 combined photorheology with real-time Fourier-transform infrared spectroscopy to derive the modulus as a function of double bond conversion. Their investigation was conducted at a UV intensity of 28 mW cm−2. By dividing the data into manageable intervals and applying a Fourier transformation, rheological information was acquired. At around the same time, Botella et al. conducted a study of a similar nature.205,206
Systems undergoing simultaneous photopolymerization and chemical cross-linking are the main applications of photorheology.198,207,208 One significant exception is the research of Higham et al.209 on the UV-activated ionic cross-linking of alginate hydrogels by released Ca2+ ions, which only caused physical gelation without any additional alginate polymerization. A range of materials, such as optical adhesives,200 acrylates,198,207 epoxies,208,210 dental resins, and photoresponsive hydrogels,209,211 have been characterized using this method.209
During polymerization, photorheology tracks rheological modifications.212Eqn (6) can be used to fit the relationship between the suspension viscosity and time using a modified Boltzmann sigmoidal model:213
|  | (6) |
where
t is the response time and
η0 and
η∞ are, respectively, the initial and ultimate viscosities before and after illumination. The relationship between the induction time
t0 and the time spent in illumination
tillum is given by the formula
t =
tillum −
t0. Using
eqn (6) to fit the photorheological data specifies the induction time
t0, the half-reaction time
t50, and the period Δ
t as three parameters. The time needed to raise the viscosity from its starting value log
η0 to (log
η0 + log
η∞)/2 is known as the half-reaction time, or
t50. As the viscosity deviates from
η0 in the semi-log linear domain, the period Δ
t corresponds to the time associated with the sigmoidal transition zone. The induction time
t0, which was calculated from the moment at which the viscosity rise becomes obvious, stands for the amount of time required to start the reaction. The treatment parameters that were determined using these techniques can be understood using theoretical models.
214 Sensitivity
Dp data can be interpreted in terms of the absorption model for sensitivity,
214 which describes how the UV beam is attenuated by the photoinitiator (PI) and dyes as well as by scattering.
In addition to determining the main parameters of the photopolymerization process mentioned above, photorheology also makes it possible to measure the linear shrinkage of photosensitive samples based on a method developed by Shah's team,215 since, in addition to the standard parameters such as viscosity, storage modulus or loss modulus, which are monitored during photopolymerization using a rheometer equipped with a photocuring unit, the height of the gap between the upper and lower geometries of the photorheometer in which the sample is placed is also tracked. Shrinkage of the sample, or swelling, leads to a change in the height of the gap, and by using eqn (7) it is possible to convert changes in the height of the gap into polymerization shrinkage over time:191,216
| 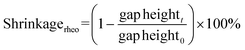 | (7) |
where gap height
0 and gap height
t are, respectively, the height of the gap between the upper and lower geometries of the photorheometer at the beginning of the measurement (at time zero) and during the measurement (at time
t).
The process known as gelation is one of the most important phenomena occurring during the crosslinking of polymer chains by photopolymerization. At the gel point, there is a rapid change in the viscosity of the investigated sample caused by the polymerization reaction of individual chains into a two- and then three-dimensional polymer network. Photorheology is also one of the methods that allow a determination of the gel point. As the photopolymerization reaction progresses, both loss and storage moduli increase, while as the growing polymer network permeates the entire volume of the sample, the storage modulus increases much faster than the loss modulus, and the intersection point of the two moduli is considered as the gel point. Determination of the gelation point using a photorheometer is possible only for samples in which at the beginning of the reaction the storage modulus is lower than the initial loss modulus. As proved in the literature, the composition of the cured sample not only affects the process itself, but also influences the possibility of determining the gel point. Lepcio et al.199,217 proved that the addition of a filler to the resin increases the viscosity of the solution sufficiently that at onset of the reaction the storage modulus exceeds the loss modulus, due to the formation of a physical network capable of bearing the load, making the determination of the gel point impossible.
Liska et al.218 described the coupling of an infrared spectrometer with a photorheometer. A parallel plate rheometer with a UV- and IR-translucent window can be used to get the rheological data. Monitoring the decline of the relevant IR-peak for the reactive monomer unit (e.g., C
C double bond peak for (meth)acrylates, C
C double bond peak in thiol–ene systems, C–O epoxy peak for epoxy resins) provides chemical information (i.e., the conversion at the gel point and ultimate conversion). The conversion can be observed in the near-infrared region (for example, acrylate double bonds, epoxy groups), or the MIR region, depending on the relative concentration of reactive functional groups in the sample volume and the strength of the IR signal (e.g. thiol signal). The interested reader can find a detailed description of this set in Lisk's publication.218 Additionally, the Stansbury research team has demonstrated the in situ monitoring of photopolymerization-induced phase separation by NIR linked turbidity measurements, utilizing a UV/VIS spectrometer.219
3. Qualitative methods for monitoring photopolymerization reactions
3.1. Dynamic mechanical analysis
Dynamic mechanical analysis (DMA) is a valuable source of information regarding the physical and mechanical properties of photosetting materials. It is used to investigate viscoelastic properties by applying an oscillating force (tensile, shear, compression, etc.) on a sample of known size as a function of temperature and time, and to monitor changes (deformations) in the sample geometry.220–227 This technique provides the following parameters: flow tendency (viscosity), shear modulus, glass transition temperature (Tg), storage modulus (E′), loss modulus (E′′), and mechanical loss angle tangent (tgδ). The storage modulus measures the stored energy, representing the elastic portion, and the loss modulus measures the energy dissipated as heat, representing the viscous portion. The response of the sample to the applied force allows us to determine the gelation point and the glass transition temperature as a function of temperature.221,222,226–230
Long molecular chain polymers have special viscoelastic properties that combine the traits of Newtonian fluids with elastic solids. The mechanical characteristics of elastic solids, where stress is proportional to strain in tiny deformations, are described by the classical theory of elasticity. Such a stress response is not dependent on the pace of strain. The characteristics of a viscous fluid, for which the response of stress depends on the strain rate, are described by the classical theory of hydrodynamics. Combinations of springs and dashpots can be used to mechanically represent the solid and liquid behavior of polymers. Dynamic mechanical analysis, which applies a sinusoidal force (stress) to a material and measures the resulting displacement (strain), is used to study a polymer's viscoelastic property. The resulting strain and tension will be exactly in phase for a solid that is perfectly elastic. There will be a 90 degree phase lag between strain and stress for a totally viscous fluid. Viscoelastic polymers have properties in the middle, where some phase lag will happen during DMA experiments. The following equations hold when strain is applied and stress follows behind:
• Stress:
| σ = σ0 sin(tω + δ) | (8) |
• Strain:
| ε = ε0 sin(tω) | (9) |
where
ω – frequency of strain oscillation,
t – time, and
δ – phase lag between stress and strain.
DMA can be applied in tandem with either FTIR or DSC techniques. It has proved useful in the determination of the mechanical and volume relaxation properties of photochemically crosslinked multifunctional (meth)acrylate-based compositions intended as materials for data storage systems, as well as in rheological studies of isothermally crosslinked epoxy resins, viscoelastic properties of thiol–ene/acrylate systems, photocured dental prosthesis resins, and microscopic phase separation studies in epoxy-acrylate hybrid resins.231–234 DMA can be used to characterize or monitor reactions analogous to coupling mass spectrometers to monitor reactions by a switching valve with a sample loop.235 Moreover, modifications to the method are possible, such as coupling it with another, as described by Zwartkruis et al. They introduced a hyphenated time-resolved technique that allows the measurement of reactive group conversion (polymerization) while simultaneously monitoring network formation by the development of mechanical properties with real-time dynamic mechanical analysis near-infrared spectroscopy (RT-DMA/NIRS).236
3.2. Dielectric analysis
Dielectric analysis (DEA) measurements have been applied during the curing of mainly thermoset materials. The analysis of dielectric properties involves the application of a sinusoidal voltage between electrodes placed in direct contact with the sample, which, during curing, due to changes in viscosity and the mobility of ions, electrons, or charged atoms present in the resin, induces changes in the measured current.237–240 If parameters such as the frequency of the excitation voltage, the area of the electrodes, and the distance between them are known, the changes in the phase and amplitude of the current allow the calculation of characteristic electrical quantities such as the dielectric constant (dielectric permeability), dielectric loss factor and dielectric strength, which are correlated with the degree of monomer conversion. The DEA method was also used to monitor the changes in other parameters of the cured sample i.e., diffusion properties, viscosity, Tg, and shrinkage (Fig. 9).237,238,241 A scheme of the equipment setup used for DEA experiments is shown in Fig. 10.
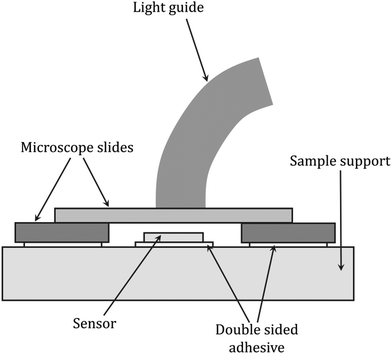 |
| Fig. 10 Scheme of the dielectric analysis setup for monitoring light-initiated polymerization reactions, based on Steinhaus et al.243 | |
Decker's team, during both radical and cationic photopolymerization, monitored real-time changes in the dielectric properties of the photocurable compositions using a combination of FTIR and DEA techniques. This approach allowed a determination of the polymerization rate and degree of cure by correlating the changes in the dielectric properties of the compositions with the monomer conversion. Nevertheless, some limitations have been found in the application of dielectric techniques to monitor in situ reactions, such as the curing of epoxy resins. It should be kept in mind that during curing, where gelation and vitrification processes occur, the concentration of mobile ions decreases as the reaction progresses, which is the reason for the inconsistency between the time dependence of the ionic conductivity and viscosity. Hence, the use of rheological measurements by DMA and electrical techniques has some limitations in monitoring the reaction progress, especially when the gel point is reached and for high conversion values.30,237,242
3.3. Dilatometry
In particular, the technique is designed to measure polymerization shrinkage due to changes in the sample volume or density during photopolymerization. Under the assumption that the amount of shrinkage is directly proportional to the degree of curing, the rate of reaction can be determined from the slope of the measurement curve, where the rate of shrinkage development is calculated from the first derivative of the shrinkage value concerning time and is considered to be a measure of the reaction rate (Fig. 13).244–247
Dilatometric studies of vinyl monomer polymerization have shown that monomer conversion is linearly related to density change.248,249 In thermoset systems, an approximately linear relationship was found between the volume change during resin curing and the monomer conversion until vitrification occurred. Similarly, for epoxy resin curing processes, there is a linear correlation between volume and conversion in the range of 65–95% conversion, while the found linear relationship between the specific volume and Tg during the thermal curing of thermoset compositions may suggest a nonlinear relationship between volume and conversion since the correlation of Tg with conversion generally shows a nonlinear trend.246,247,250 Hence, dilatometry is of limited use due to the lack of unambiguous correlation and reliability of the determined measurement parameters, while there are other more valuable techniques for determining shrinkage, which are discussed in the next subsection of the paper. A scheme of the equipment setup used for dilatometric experiments is shown in Fig. 11.
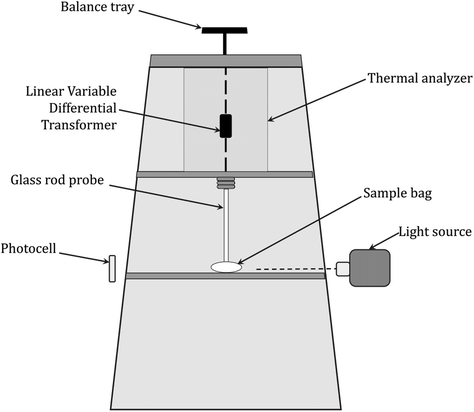 |
| Fig. 11 Scheme of a recording dilatometer for monitoring light-initiated polymerization reactions, based on McGinniss and Holsworth.251 | |
3.4. Interferometry
Interferometry is an alternative method for measuring, among other things, shrinkage in real-time and provides greater accuracy and precision compared with traditional methods of measuring polymerization shrinkage such as dilatometry described above. The basic principle of this method is to use two intersecting laser beams to create an interference pattern on the photocured film. The bright areas become cross-linked, while in the dark areas the liquid resin remains unchanged. The interference pattern is formed under laser irradiation due to the increase in the refractive index of the composition during its photopolymerization. When exposed to only one laser beam (i.e., the reference beam), the resulting pattern causes a diffraction of incident light with an efficiency that is directly related to the degree of polymerization (Fig. 13). The operational wavelength of the laser beam depends on the refractive index of the air through which it passes and this alters with the air temperature, air pressure and relative humidity. Therefore, the wavelength of the beam needs to be altered (compensated) to incorporate any changes in these parameters.252–256
The advantages of interferometry include the inherent sensitivity and accuracy of interferometric measurements offered by an instrument that does not need to be calibrated because the wavelength of the laser light source provides a precisely known wavelength. In addition, the ability to collect data at high speed allows for the real-time characterization of rapid photopolymerization reactions, among others. The low cost and relative ease of use associated with the device are also advantages. The interferograms obtained from the sample curing measurements can be converted into, among other things, kinetic profiles representing the percent linear shrinkage over time, which provide information about the relative shrinkage rate and the total degree of shrinkage. Data extraction from interferograms is straightforward compared with dilatometric measurements, where significant temperature corrections and calibration procedures are required to achieve high accuracy and precision.257–259 A scheme of the equipment setup used for laser interferometric experiments is shown in Fig. 12.
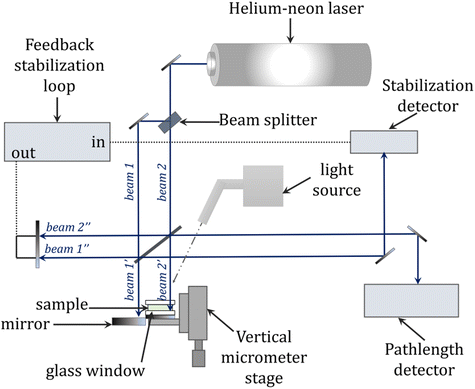 |
| Fig. 12 General schematic of the laser interferometry setup for monitoring photopolymerization reactions, based on Fogleman et al.257 | |
The use of optical fibers, laser light sources, and numerous modifications of typical Michelson and Mach–Zehnder interferometers have greatly expanded the range of applications of interferometry. It proved to be useful in studies of the kinetics of the photopolymerization of acrylates, where interferometric measurements provided information on changes in the refractive index and thickness of the composition layer accompanying the curing process. A detailed analysis of the interferometric data allowed characterization of various aspects of the curing process including the degree and profile of curing along with the layer depth, gel point, shrinkage and oxygen inhibition, intensity, and time of radiation dose. Moreover, as an optical method, interferometry has found practical applications in stereolithography, and holography, for the study of photo-cured dental fillings, composite materials, and others.124,257,260,261
4. Alternative radical photopolymerization monitoring techniques and specific examples of their applications
Among the applications of the photopolymerization reaction are dentistry and light-cured fillings. Adequate polymerization of acrylate composite resin, which is the main component of currently used dental fillings, has a significant impact on the success of cavity restoration while the degree of curing affects the mechanical and physicochemical properties of the cured material, i.e., abrasion resistance, bonding strength to dentin, solubility in saliva and biocompatibility. Uneven polymerization along the depth profile of the restoration may lead to inadequate curing at the interface between the composite and the tooth surface. Thus, it is highly desirable to monitor the degree of composite cure in real-time for optimal performance and the quality of the final material.262–264
In assessing the degree of cure of dental compositions, destructive techniques that require sample preparation, such as the scraping technique in assessing the depth of cure, microhardness tests or differential scanning calorimetry (DSC) are commonly used.265,266 Techniques are currently being sought to non-destructively monitor the degree of hardening. The techniques of reflectance infrared spectroscopy and Raman spectroscopy are among the non-destructive methods to investigate the degree of curing of dental compositions. However, the disadvantages of these methods are only surface monitoring of the polymerization reaction and a lack of information about the changes inside the restoration.267,268 Other techniques encountered are microcomputed tomography (μCT) and magnetic resonance imaging (MRI) (Fig. 14).269
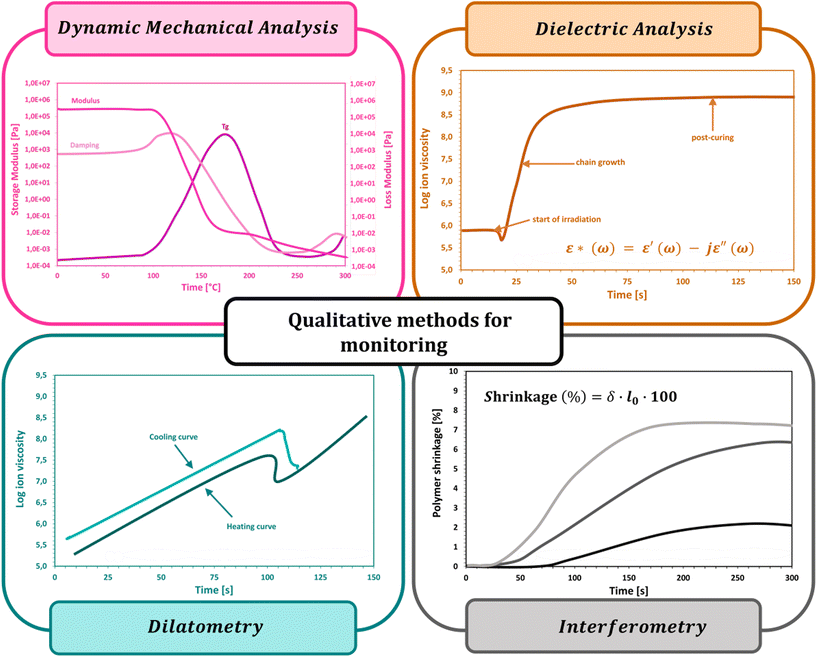 |
| Fig. 13 Characteristic parameters determined by photorheology. | |
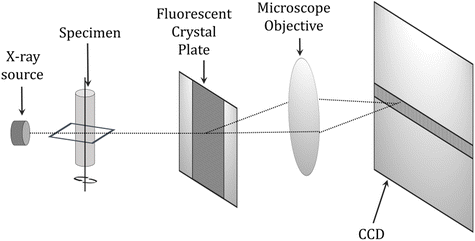 |
| Fig. 14 Schematic diagram of a microcomputed tomography (μCT) scanner.270 | |
The μCT technique can accurately measure changes in composition volume due to polymerization, but cannot be used to monitor these changes on an ongoing basis during cavity restoration. MRI studies are useful for studying photocuring processes, but the ability to monitor the complete curing of the composition is limited by the very short relaxation time, known as the T2 time constant.269 Dynamic measurements by optical coherence tomography (OCT) and low coherence interferometry (LCI) are also performed in measuring the degree of cure of photocurable resins.269,271 In these techniques, the composition's refractive index and layer thickness correlate with the degree of cure. However, these measurements are limited to compositions that do not contain fillers and to layer depths of up to 1 mm due to the limited penetration of near-infrared light. One technique that appears in the literature as a method for monitoring the degree of curing is the terahertz (THz) technique, where terahertz radiation in the wavelength range 3 mm–3 μm and frequency range 0.1–10 THz is used to study dental materials, e.g., to measure demineralization and damage to enamel and dentin.269 The THz technique exploits changes in the dielectric parameters of the composition components during photocuring. The advantage of this method is that there is no limitation on the thickness of the cured layers due to the much higher transmittance of terahertz radiation by organic materials, which allows the examination of composition layers over a cross-section of several millimeters (Fig. 15).
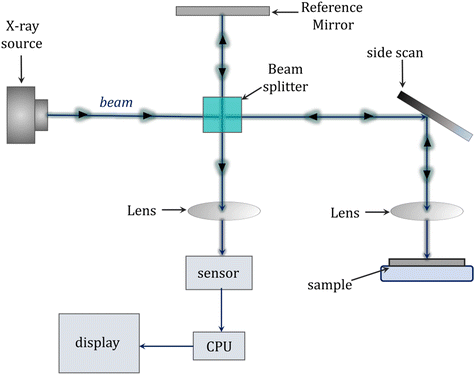 |
| Fig. 15 Schema of optical coherence tomography (OCT). | |
The availability and usefulness of techniques for monitoring the degree of cure of dental restorations are limited, so new solutions are constantly being sought to directly monitor the degree of cure in the volume of the entire restorative composition during tooth structure restoration.
In addition, one of the problems encountered during the photopolymerization of compositions containing acrylates is high polymerization shrinkage associated with the intramolecular transformation of weak van der Waals interactions into strong covalent carbon–carbon bonds. Shrinkage can impair the mechanical properties, cause surface irregularities of the coating, and cause poor adhesion of the coating to the substrate and depends on, among other things, the composition, monomers and resins used, and the presence of fillers, as well as the conversion and degree of crosslinking. This is particularly troublesome in the case of photocured dental restorations, where due to shrinkage, a gap is formed between the tooth and the composite allowing the penetration of bacteria. Hence, it is reasonable to monitor and determine the degree of polymerization shrinkage of monomers and oligomers during the photopolymerization reaction.264,272 For this purpose, many experimental methods have been tested for the quantification of polymerization shrinkage, where the main test material was acrylate dental compositions. Among others, dilatometry, interferometry, refractometry, gas pycnometer methods, strain gauges, special laser techniques, optical measurements using video-microscopes, and many others were used.271,273 The aforementioned methods were often a source of accurate and reproducible data on the degree and kinetics of polymerization shrinkage; however, their disadvantages were primarily the labor intensity and the fact that the determined shrinkage values could not be related to the instantaneous conversion rate, which required further combination with another technique. The polymerization of light-cured fillings is not the only area using batch-process analysis techniques. These areas also include 3D printing process control or the production of UV-curable coatings. These areas benefit from the use of these techniques by reducing the exposure time and real-time quality control or selecting the most optimal postcuring times in 3D printing.274,275
Spectroscopic methods have proved to be the optimal approach to simultaneously monitor conversion and shrinkage in real-time. Examples of such combined techniques include the combination of a laser sensor-detector, mounted over a diamond crystal of an ATR attachment, in an FTIR spectrometer, which allowed the quantitative measurement of shrinkage concerning conversion.228 Another interesting solution among the combined techniques is the combination of photorheometry, more specifically dynamic mechanical analysis (DMA) with near-infrared spectroscopy (FT-NIR), proposed by Schmidt and Scherzer.228 The combination of the two measurement techniques provided the ability to directly correlate shrinkage and conversion and their dependence on time, as well as the exposure conditions of the composition.276–279
The photorheometry technique itself is used to monitor in real-time the viscoelastic properties of the cured materials. This technique has proved itself more than once in studies of the photopolymerization kinetics of (meth)acrylate compositions, thiol–ene compositions, and sol–gel systems.228,280 The quantitative shrinkage values measured by this technique showed a very good correlation with the data determined by other methods reported in the literature. In addition to shrinkage, other measurable parameters also correlated with conversion, including the viscosity, mechanical properties, and shear modulus.
Slopek et al. used micro-rheological measurements to quantitatively monitor the in situ gelation step in the free radical photopolymerization process.281,282 Brownian motions of inert rhodamine-labeled silicon particles of micrometric size were observed during the UV-beam irradiation of photosensitive acrylate resins and hydrogel compositions and the use of an optical videomicroscope. Statistical analysis of the velocity and position of these particles as a function of time, conditioned by the changing rheological properties of their surroundings, made it possible to study the influence of numerous parameters on the photopolymerization process and gelation phase. Monitoring using micro-rheological studies allowed the determination of three-dimensional gelation profiles and the investigation of the influence of such parameters as the photoinitiator concentration, the formulation of the composition, and the radiation intensity on the course of photopolymerization. Significant changes in gelation time were observed with changes in the intensity of the UV beam and the depth of light penetration into the sample. The inhibitory effect of oxygen on the rate of gelation and curing of the resin was also found. During these experiments, an additional pulsed UV source was used, which allowed for quantitative studies of dark reactions. Based on the above experiments, the initiation (ki), propagation (kp), and termination (kt) rate constants, among others, were determined.281,282
Another solution proposed by several other authors, including Chiou, Lange, Botella, et al. was to monitor the conversion and rheological changes in the sample during photopolymerization by combining the FTIR technique with a rheometer. However, this method did not take into account the spatial changes in the sample, since the rheometer measures the averaged mechanical properties throughout the sample volume (Fig. 16).283,284,308–310
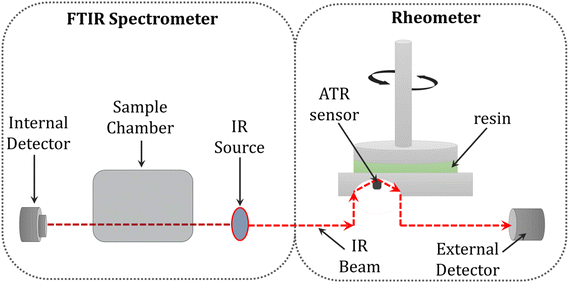 |
| Fig. 16 Schematic illustration of the combination of FTIR spectroscopy with an ATR crystal and rheology.285 | |
To get a thorough picture of reactions, it is beneficial to combine a number of strategies. The most well-known example of polymerizations is the so-called ACOMP system.286,287 ACOMP (automatic continuous online monitoring of polymerization) performs continuous sample withdrawal and dilution from a reactor into a tiny stream of reactor fluid that passes through a number of inline detectors, primarily a refractometer (RI), ultraviolet absorbance spectrometer (UV), time-dependent static light scattering (TDSLS) device, and perhaps also a viscometer, to perform online monitoring of the absolute weight-average molecular weight of the polymers. Comparative measurements of monomer conversions were made using in situ NIR and the ACOMP detection train, and they showed good agreement.288 Only batch and semi-batch processes used ACOMP.289,290 The ACOMP system is entirely automated and interfaces with the distributed control system (DCS) of the plant to track the output of the studied data.291,292
Other methods, in addition to spectroscopic ones, provide important information about how a polymerization is developing. In this regard, mass spectrometry is significant. To examine the mechanisms of radical, photochemical, electrochemical, and organometallic or polymerization reactions, there are numerous additional analysis instruments for batch processing.293,294
Since spectroscopy only provides a small amount of information on macromolecular structures and end-group chemistry, monitoring polymerizations by spectroscopic approaches frequently yields very limited information. Unique product patterns, data on polymer end-groups, and block copolymer compositions can all be ascertained using mass spectrometry (MS). Thus, a common strategy for monitoring reaction products continuously involves connecting a mass spectrometer to a reaction tank.295,296 Santos described a straightforward online linking of microreactor devices to mass spectrometers.297 In certain circumstances, samples were transferred from the reactor outlet into the mass spectrometer using a six-port switching valve with a sample loop, a mass rate attenuator, or other automated sampling techniques.298,299 A low-volume mixing tee that is directly connected to an electrospray mass spectrometer's ionization probe was developed by Sam and his colleagues.300 There are many instances of comparable setups in the literature that examine reaction mechanisms, reactive intermediates, and the kinetics of chemical reactions. By adjusting the length of the transfer capillary, the mixing tee is typically connected to the ionization probe with a fused-silica capillary that offers residence periods from 0.7 to 28 s in a continuous flow mode.301,302 Santos and Metzger were the first to make continuous online MS monitoring of polymerization reactions available. Early in the polymerization process, they looked at the mechanisms of the homogeneously catalyzed Ziegler–Natta polymerization of ethane and Brookhart polymerization of alkenes.303 A first micromixer, which also functions as a microreactor to start the polymerization reaction, is used to combine the reactants. The capillary that carried the reactive solution to the second micromixer, where it was quenched with the ESI solvent and then delivered straight to the ESI nozzle, is where the polymerization process took place. Even though it has an easy-to-understand design, this method only allows for a little amount of screening of reaction (residence) timings. Ley developed a tiny electrospray ionization mass spectrometer that is connected to a device for preparative flow chemistry via a switching valve.304 With this technique, it is able to screen starter materials, monitor intermediates and competing reaction pathways, and improve reaction conditions. In microfluidic devices, Belder and colleagues recently introduced on-chip MS reaction monitoring.305 With this technique, a single quick prototype microfluidic device integrates electrochromatography separation, electrospray ionization, and chemical reactions. This setup investigated the Passerini three-component reaction (Passerini-3CR) to show the high-throughput-screening capability of continuous microreactors for macromolecular design, more especially the synthesis of diblock copolymer conjugates.306 The kinetics of n-butyl acrylate's reversible addition fragmentation chain transfer polymerization were investigated using the same method. Time-sweep studies can be performed to track a whole polymerization over time in a single experiment by adjusting flow rates in the reactor.307
5. Conclusions
There are numerous automatable characterization techniques available for monitoring photopolymerization operations. In addition to gathering data on specific polymers such as molecular weight distributions and polymer end-groups, a wide range of characteristics can be evaluated. Additional technique integration will present hurdles in the future, particularly in the area of flow chemistry. Inline and online characterization approaches are fundamentally best suited to monitor flow procedures. No system that would provide direct feedback to process control and thus enable a fully automated synthesis routine has yet been established, with the exception of the ACOMP system, which is extremely focused on industrial polymerization processes. A major improvement of research in synthetic fields can be anticipated by combining a number of online monitoring approaches (chosen based on the specific type of response) with computerized feedback and reactor management. As full remote control becomes accessible at the same time, reactors can be fully integrated into the IT infrastructure, making them suitable for industry 4.0.
Conflicts of interest
The authors declare no conflict of interest.
Acknowledgements
One of the authors, W. T., would like to thank the National Science Centre (NCN, Poland), PRELUDIUM 19, Project No: 2020/37/N/ST5/03161 and Foundation for Polish Science (Warsaw, Poland), project START, Grant No. START 087.2022 for the financial support of the principal investigator. Also, another author, P. Sz., would like to thank the Ministry of Science and Higher Education (MNiSW) under the Diamond Grant project, contract number 0072/DIA/2020/49 for the financial support of the principal investigator. The authors would like to thank the National Science Centre (NCN, Poland), OPUS-LAP, Project No: UMO-2020/39/I/ST5/03556 for funding this publication (open access).
References
- J. Litster and I. D. L. Bogle, Engineering, 2019, 5, 1003–1009 CrossRef CAS.
- A. J. S. Hammer, A. I. Leonov, N. L. Bell and L. Cronin, JACS Au, 2021, 1, 1572–1587, DOI:10.1021/JACSAU.1C00303.
- L. Rogers and K. F. Jensen, Green Chem., 2019, 21, 3481–3498 RSC.
- C. Hepburn, Oxford Rev. Econ. Policy, 2010, 26, 117–136 CrossRef.
- M. A. Dubé and S. Salehpour, Macromol. React. Eng., 2014, 8, 7–28 CrossRef.
- M. Topa, J. Ortyl, A. Chachaj-Brekiesz, I. Kamińska-Borek, M. Pilch and R. Popielarz, Spectrochim. Acta, Part A, 2018, 199, 430–440 CrossRef CAS PubMed.
- M. Topa, F. Petko, M. Galek, M. Jankowska, R. Popielarz and J. Ortyl, Eur. Polym. J., 2021, 156(5), 110612, DOI:10.1016/j.eurpolymj.2021.110612.
- S. Liu, Y. Cheng, H. Zhang, Z. Qiu, R. T. K. Kwok, J. W. Y. Lam and B. Z. Tang, Angew. Chem., Int. Ed., 2018, 57, 6274–6278 CrossRef CAS PubMed.
- H. Algamaiah, N. Silikas and D. C. Watts, Dent. Mater., 2020, 36, 1266–1274 CrossRef CAS PubMed.
- B. Sullivan, D. Kalliecharan, I. Kostylev, G. Earle, J. W. Stansbury, R. B. Price and D. Labrie, J. Mech. Behav. Biomed. Mater., 2021, 124, 104884, DOI:10.1016/j.jmbbm.2021.104884.
-
W. F. Reed, Monitoring Polymerization Reactions : From Fundamentals to Applications, Wiley, 2013 Search PubMed.
- E. Frauendorfer, A. Wolf and W. D. Hergeth, Chem. Eng. Technol., 2010, 33, 1767–1778 CrossRef CAS.
- A. M. Alb and W. F. Reed, Macromol. React. Eng., 2010, 4, 470–485 CrossRef CAS.
- M. Topa, J. Ortyl, A. Chachaj-Brekiesz, I. Kamińska-Borek, M. Pilch and R. Popielarz, Spectrochim. Acta, Part A, 2018, 199, 430–440 CrossRef CAS PubMed.
- I. Kamińska, J. Ortyl and R. Popielarz, Polym. Test., 2015, 42, 99–107 CrossRef.
- J. Ortyl, P. Milart and R. Popielarz, Polym. Test., 2013, 32, 708–715 CrossRef CAS.
- N. Zaquen, M. Rubens, N. Corrigan, J. Xu, P. B. Zetterlund, C. Boyer and T. Junkers, Prog. Polym. Sci., 2020, 107, 101256, DOI:10.1016/j.progpolymsci.2020.101256.
- D. Lithner, A. Larsson and G. Dave, Sci. Total Environ., 2011, 409, 3309–3324 CrossRef CAS PubMed.
- P. H. H. Araujol, C. Sayfcr’, J. G. R. Poco2 and R. Giudici’, Polym. Eng. Sci., 2002, 42(7), 1442–1468 CrossRef.
- The International Organization for Standardization ISO 1628-2-2020.
- C. Sarosi, M. Moldovan, A. Soanca, A. Roman, T. Gherman, A. Trifoi, A. M. Chisnoiu, S. Cuc, M. Filip, G. F. Gheorghe and R. M. Chisnoiu, Polymers, 2021, 13(24), 4415, DOI:10.3390/polym13244415.
- T. Junkers, Macromol. Chem. Phys., 2016, 218(2), 1600421, DOI:10.1002/macp.201600421.
- P. Garra, A. H. Bonardi, A. Baralle, A. al Mousawi, F. Bonardi, C. Dietlin, F. Morlet-Savary, J. P. Fouassier and J. Lalevée, J. Polym. Sci., Part A: Polym. Chem., 2018, 56, 889–899 CrossRef CAS.
- B. B. Guo, C. Liu, J. H. Xin, C. Y. Zhu and Z. K. Xu, Polym. Chem., 2021, 12, 4332–4336 RSC.
- Y. Pan, T. Li, Y. Zhou and L. Li, Polymer, 2022, 242, 124613, DOI:10.1016/j.polymer.2022.124613.
- O. Kammona, E. G. Chatzi and C. Kiparissides, J. Macromol. Sci., Rev. Macromol. Chem. Phys., 1999, 39, 57–134 CrossRef.
-
I. Alig, D. Lellinger, S. Agarwal and H. Oehler, in Reactive and Functional Polymers, 2013, vol. 73, pp. 316–322 Search PubMed.
- G. E. Fonseca, M. A. Dubé and A. Penlidis, Macromol. React. Eng., 2009, 3, 327–373 CrossRef CAS.
- M. Bouzrati-Zerelli, J. Kirschner, C. P. Fik, M. Maier, C. Dietlin, F. Morlet-Savary, J. P. Fouassier, J. M. Becht, J. E. Klee and J. Lalevée, Macromolecules, 2017, 50, 6911–6923 CrossRef CAS.
- R. Hardis, J. L. P. Jessop, F. E. Peters and M. R. Kessler, Composites, Part A, 2013, 49, 100–108 CrossRef CAS.
- S. Asmussen, W. Schroeder, I. Dell'Erba and C. Vallo, Polym. Test., 2013, 32, 1283–1289 CrossRef CAS.
- S. Hu, R. Popielarz and D. C. Neckers, Macromolecules, 1998, 51, 4107–4113 CrossRef.
- J. Ortyl, K. Sawicz and R. Popielarz, J. Polym. Sci., Part A: Polym. Chem., 2010, 48, 4522–4528 CrossRef CAS.
- J. Ortyl, M. Galek, P. Milart and R. Popielarz, Polym. Test., 2012, 31, 466–473 CrossRef CAS.
- R. Popielarz and O. Vogt, J. Polym. Sci., Part A: Polym. Chem., 2008, 46(11), 3531 CrossRef.
- J. Ortyl, P. Fiedor, A. Chachaj-Brekiesz, M. Pilch, E. Hola and M. Galek, Sensors, 2019, 19(7), 1668, DOI:10.3390/s19071668.
- J. Paczkowski and D. C. Neckers, Macromolecules, 1992, 25, 548–553 CrossRef CAS.
- J. Ortyl, K. Sawicz and R. Popielarz, J. Polym. Sci., Part A: Polym. Chem., 2010, 48, 4522–4528 CrossRef CAS.
- I. Kamińska, J. Ortyl and R. Popielarz, Polym. Test., 2016, 55, 310–317 CrossRef.
-
C. Peinado, E. F. Salvador, F. Catalina and A. E. Lozano, Solvatochromic and rigidochromic fluorescent probes based on D±p-A diaryl ethylene and butadiene derivatives for UV-curing monitoring Search PubMed.
- R. Vatanparast, S. Li, K. Hakala and H. Lemmetyinen, Macromolecules, 2000, 33, 438–443 CrossRef CAS.
- J. Ortyl, K. Sawicz-Kryniger, J. Wirski and R. Popielarz, Przem. Chem., 2011, 9, 431370–431374 Search PubMed.
- W. F. Jager, A. A. Volkers and D. C. Neckers, Macromolecules, 1995, 28(24), 8153–8158 CrossRef CAS.
- J. C. Song and D. C. Neckers, Multidimensional Spectroscopy of Polymers, 1995, 28, 472–487 CrossRef.
- Z. Wang and J. Song, J. Polym., 1996, 34, 325–333 CAS.
- O. Okay, D. Kaya and O. Pekcan, Polymer, 1999, 40, 6179–6187 CrossRef CAS.
- J. Ortyl, M. Galek, P. Milart and R. Popielarz, Polym. Test., 2012, 31, 466–473 CrossRef CAS.
- T. Scherzer and H. Langguth, Nucl. Instrum. Methods Phys. Res., Sect. B, 2001, 185, 276–282 CrossRef CAS.
- J. Ortyl, M. Galica, R. Popielarz and D. Bogdał, Pol. J. Chem. Technol., 2014, 16, 75–80 CrossRef CAS.
- M. K. Aromaa and P. K. Vallittu, Dent. Mater., 2018, 34, 1247–1252 CrossRef CAS PubMed.
- N. Li and X. C. Pan, Chin. J. Polym. Sci. (Engl. Ed.), 2021, 39, 1084–1092 CrossRef CAS.
- N. B. Cramer, C. P. O'Brien and C. N. Bowman, Polymer, 2008, 49, 4756–4761 CrossRef CAS.
- S. C. Ligon, B. Husár, H. Wutzel, R. Holman and R. Liska, Chem. Rev., 2014, 114, 577–589 CrossRef PubMed.
- R. Simič, J. Mandal, K. Zhang and N. D. Spencer, Soft Matter, 2021, 17, 6394–6403 RSC.
- C. Peinado, E. F. Salvador, J. Baselga and F. Catalina, Macromol. Chem. Phys., 2001, 202, 1924–1934 CrossRef CAS.
- J. Eric Dietz and N. a. Peppas, Polymer, 1997, 38, 3767–3781 CrossRef.
- J. Ortyl, M. Topa, I. Kamińska-Borek and R. Popielarz, Eur. Polym. J., 2019, 116, 45–55 CrossRef CAS.
- J. Ortyl, J. Wilamowski, P. Milart, M. Galek and R. Popielarz, Polym. Test., 2015, 48, 151–159 CrossRef CAS.
- X. He, W. Jia, Y. Gao, S. Jiang, J. Nie and F. Sun, Eur. Polym. J., 2022, 167, 111066, DOI:10.1016/j.eurpolymj.2022.111066.
- T. L. Huang and Y. C. Chen, J. Photochem. Photobiol., A, 2022, 167, 111066, DOI:10.1016/j.jphotochem.2022.113900.
- P. B. F. Soares, D. Magalhães, J. Silverio, J. R. W. Silva, J. L. Gonçalves Junior and C. J. Soares, Dent. Mater., 2012, 28, e58 CrossRef.
- M. Trujillo, S. M. Newman and J. W. Stansbury, Dent. Mater., 2004, 20, 766–777 CrossRef CAS PubMed.
- E. Hola, A. Gruchała, R. Popielarz and J. Ortyl, Eur. Polym. J., 2021, 160, 110802, DOI:10.1016/j.eurpolymj.2021.110802.
- M. Topa, E. Hola, M. Galek, F. Petko, M. Pilch, R. Popielarz, F. Morlet-Savary, B. Graff, J. Laleveé and J. Ortyl, Polym. Chem., 2020, 11, 5261–5278 RSC.
- P. Fiedor, M. Pilch, P. Szymaszek, A. Chachaj-Brekiesz, M. Galek and J. Ortyl, Catalysts, 2020, 10(3), 284, DOI:10.3390/catal10030284.
- X. Xu, C. Du, F. Ma, Y. Shen and J. Zhou, Forensic Sci. Int., 2020, 310, 110222, DOI:10.1016/j.forsciint.2020.110222.
- I. Soares, S. França de Sá and J. L. Ferreira, Spectrochim. Acta, Part A, 2020, 240, 118548, DOI:10.1016/j.saa.2020.118548.
- C. C. Zhang, J. Shi, S. Hartlaub, J. P. Palamara, I. Petrovic and B. Yilmaz, Catal. Commun., 2021, 150, 106273, DOI:10.1016/j.catcom.2020.106273.
- P. Fiedor, M. Pilch, P. Szymaszek, A. Chachaj-Brekiesz, M. Galek and J. Ortyl, Catalysts, 2020, 10(3), 284, DOI:10.3390/catal10030284.
- W. Tomal, D. Krok, A. Chachaj-Brekiesz and J. Ortyl, Eur. Polym. J., 2021, 156, 110603, DOI:10.1016/j.eurpolymj.2021.110603.
- K. F. Fazende, D. P. Gary, J. D. Mota-Morales and J. A. Pojman, Macromol. Chem. Phys., 2020, 221(6), 1900511, DOI:10.1002/macp.201900511.
- T. Xue, L. Tang, R. Tang, Y. Li, J. Nie and X. Zhu, Dyes Pigm., 2021, 188, 109212, DOI:10.1016/j.dyepig.2021.109212.
- H. Chen, G. Noirbent, Y. Zhang, K. Sun, S. Liu, D. Brunel, D. Gigmes, B. Graff, F. Morlet-Savary, P. Xiao, F. Dumur and J. Lalevée, Dyes Pigm., 2021, 188, 109213, DOI:10.1016/j.dyepig.2021.109213.
- W. Tomal, T. Świergosz, M. Pilch, W. Kasprzyk and J. Ortyl, Polym. Chem., 2021, 12, 3661–3676 RSC.
- A. C. Scanone, U. Casado, W. F. Schroeder and C. E. Hoppe, Eur. Polym. J., 2020, 134, 109841, DOI:10.1016/j.eurpolymj.2020.109841.
- M. Topa and J. Ortyl, Materials, 2020, 13(18), 4093, DOI:10.3390/ma13184093.
- M. Topa, F. Petko, M. Galek and J. Ortyl, Sensors, 2020, 20(11), 3043, DOI:10.3390/s20113043.
- E. Hola, M. Pilch, M. Galek and J. Ortyl, Polym. Chem., 2020, 11, 480–495 RSC.
- T. Borjigin, G. Noirbent, D. Gigmes, P. Xiao, F. Dumur and J. Lalevée, Eur. Polym. J., 2022, 162, 110885, DOI:10.1016/j.eurpolymj.2021.110885.
- C. Bignardi, L. F. Oliveira, N. M. Pesqueira, B. A. Riga-Rocha, A. E. H. Machado, V. P. Carvalho-Jr and B. E. Goi, J. Photochem. Photobiol., A, 2022, 423, 113595, DOI:10.1016/j.jphotochem.2021.113595.
- S. Krishnamurthy, Y. Yoshida and T. Endo, Polym. Chem., 2022, 13, 267–274 RSC.
- X. Allonas, M. Lecompère, B. Gachet, A. Criqui, D. Maréchal and C. Croutxé-Barghorn, Polym. Chem., 2021, 12, 6846–6853 RSC.
- K. Toyota, Polymer, 2021, 218, 123490, DOI:10.1016/j.polymer.2021.123490.
- M. Topa and J. Ortyl, J. Photopolym. Sci. Technol., 2021, 34(3), 259–262 CrossRef CAS.
- M. Topa, E. Hola, M. Galek, F. Petko, M. Pilch, R. Popielarz, F. Morlet-Savary, B. Graff, J. Laleveé and J. Ortyl, Polym. Chem., 2020, 11, 5261–5278 RSC.
- C. Ni, K. Feng, X. Li, H. Zhao and L. Yu, Prog. Org. Coat., 2020, 148, 105824, DOI:10.1016/j.porgcoat.2020.105824.
- K. I. Hadjiivanov, D. A. Panayotov, M. Y. Mihaylov, E. Z. Ivanova, K. K. Chakarova, S. M. Andonova and N. L. Drenchev, Chem. Rev., 2021, 121, 1286–1424 CrossRef CAS PubMed.
- W. Tomal, M. Pilch, A. Chachaj-Brekiesz, M. Galek, F. Morlet-Savary, B. Graff, C. Dietlin, J. Lalevée and J. Ortyl, Polym. Chem., 2020, 11, 4604–4621 RSC.
- S. Wierzbicki, K. Mielczarek, M. Topa-Skwarczyńska, K. Mokrzyński, J. Ortyl and S. Bednarz, Eur. Polym. J, 2021, 161, 110836, DOI:10.1016/j.eurpolymj.2021.110836.
- R. Hoffmann, H. Naatz and A. Hartwig, J. Mater. Sci., 2022, 57, 1755–1777 CrossRef CAS.
- A. Tsuneishi, S. Uchiyama, R. Hayashi, K. Taki and T. Kozawa, Jpn. J. Appl. Phys., 2018, 57(9), 096501, DOI:10.7567/JJAP.57.096501.
- I. M. Barszczewska-Rybarek, J. Appl. Polym. Sci., 2012, 123, 1604–1611 CrossRef CAS.
- E. Hola, M. Topa, A. Chachaj-Brekiesz, M. Pilch, P. Fiedor, M. Galek and J. Ortyl, RSC Adv., 2020, 10, 7509–7522 RSC.
- A. al Mousawi, C. Dietlin, B. Graff, F. Morlet-Savary, J. Toufaily, T. Hamieh, J. P. Fouassier, A. Chachaj-Brekiesz, J. Ortyl and J. Lalevée, Macromol. Chem. Phys., 2016, 217, 1955–1965 CrossRef.
- J. J. Schwartz, D. S. Jakob and A. Centrone, Chem. Soc. Rev., 2022, 51, 5248–5267 RSC.
- M. Miyazaki, T. Kamiya, M. Wohlgemuth, K. Chatterjee, R. Mitrić, O. Dopfer and M. Fujii, Phys. Chem. Chem. Phys., 2022, 24, 73–85 RSC.
- C. K. Akhgar, J. Ebner, O. Spadiut, A. Schwaighofer and B. Lendl, Anal. Chem., 2022, 94, 5583–5590 CrossRef CAS PubMed.
- K. M. Fahelelbom, A. Saleh, M. M. A. Al-Tabakha and A. A. Ashames, Rev. Anal. Chem., 2022, 41, 21–33 CrossRef CAS.
- Z. Czech, A. Kowalczyk, J. Ortyl and J. Swiderska, Pol. J. Chem. Technol., 2013, 15, 12–14 CrossRef CAS.
- M. Z. I. Mollah, M. R. I. Faruque, D. A. Bradley, M. U. Khandaker and S. al Assaf, Radiat. Phys. Chem., 2023, 202, 110500, DOI:10.1016/j.radphyschem.2022.110500.
- G. Sun, X. Wu and R. Liu, Prog. Org. Coat., 2021, 155, 106229, DOI:10.1016/j.porgcoat.2021.106229.
- T. Revathi and R. Jeyalakshmi, Constr. Build. Mater., 2021, 267, 120965, DOI:10.1016/j.conbuildmat.2020.120965.
- A. Rasool and T. Z. Rizvi, Phys. B, 2022, 646, 414291, DOI:10.1016/j.physb.2022.414291.
- J. I. Viegas, S. Thomas, R. N. Gontijo, A. Righi, R. L. Moreira and A. Dias, Mater. Res. Bull., 2022, 146, 111616, DOI:10.1016/j.materresbull.2021.111616.
- K. C. Maurya, A. I. K. Pillai, M. Garbrecht and B. Saha, Mater. Today Phys., 2022, 27, 100797, DOI:10.1016/j.mtphys.2022.100797.
- L. El-Adel, A. Ouasri, A. Rhandour and L. Hajji, Solid State Commun., 2021, 340, 114541, DOI:10.1016/j.ssc.2021.114541.
- N. Baden, H. Kobayashi and N. Urayama, Int. J. Polym. Anal. Charact., 2020, 25, 1–7 CrossRef CAS.
- M. He, X. Chen, J. Zhang, J. Li, D. Zhao, Y. Huang, D. Huo, X. Luo and C. Hou, Food Chem., 2023, 400, 134064, DOI:10.1016/j.foodchem.2022.134064.
- S. Phan, J. L. Padilla-Gamiño and C. K. Luscombe, Polym. Test., 2022, 116, 107752, DOI:10.1016/j.polymertesting.2022.107752.
- M. I. Rumaling, F. P. Chee, A. Bade, N. H. Hasbi, S. Daim, F. Juhim, M. Duinong and R. Rasmidi, Heliyon, 2022, 8, e10472 CrossRef CAS PubMed.
- M. S. Horpan, N. Şahan, H. Paksoy, O. Sivrikaya and M. Günes, Sol. Energy Mater. Sol. Cells, 2019, 195, 346–352 CrossRef CAS.
- B. Ghosh, N. Roy, D. Roy, S. Mandal, S. Ali, P. Bomzan, K. Roy and M. Nath Roy, J. Mol. Liq., 2021, 344, 117977, DOI:10.1016/j.molliq.2021.117977.
- W. Tomal and J. Ortyl, Eur. Polym. J., 2022, 111588 CrossRef CAS.
- K. S. Etsè, K. D. Etsè, G. Zaragoza and A. Mouithys-Mickalad, J. Mol. Struct., 2022, 1269, 133731, DOI:10.1016/j.molstruc.2022.133731.
- V. Enev, P. Sedláček, S. Jarábková, T. Velcer and M. Pekař, Colloids Surf., A, 2019, 575, 1–9 CrossRef CAS.
- D. Lörchner, D. Dittmann, U. Braun, L. W. Kroh and R. Köppen, J. Anal. Appl. Pyrolysis, 2019, 141, 104635, DOI:10.1016/j.jaap.2019.104635.
- W. Tomal, A. Chachaj-Brekiesz, R. Popielarz and J. Ortyl, RSC Adv., 2020, 10, 32162–32182 RSC.
- M. A. Dubé and L. Li, Polym.-Plast. Technol. Eng., 2010, 49, 648–656 CrossRef.
- J. C. Steinbach, M. Schneider, O. Hauler, G. Lorenz, K. Rebner and A. Kandelbauer, Polymers, 2020, 12, 1–13 CrossRef PubMed.
- D. Fischer, K. Sahre, M. Abdelrhim, B. Voit, V. B. Sadhu, J. Pionteck, H. Komber and J. Hutschenreuter, C. R. Chim., 2006, 9, 1419–1424 CrossRef CAS.
- M. A. Dubé and L. Li, Polym.-Plast. Technol. Eng., 2010, 49, 648–656 CrossRef.
- S. Salehpour and M. A. Dubé, Macromol. React. Eng., 2012, 6, 85–92 CrossRef CAS.
- A. Ausili, M. Sánchez and J. C. Gómez-Fernández, Biomed. Spectrosc. Imaging, 2015, 4, 159–170 CAS.
- C. Hu, Q. Liu, H. Zhang and K. Cui, Opt. Lasers Eng., 2023, 160, 107313, DOI:10.1016/j.optlaseng.2022.107313.
- C. Raman and K. Krishnan, Nature, 1928, 121, 501–502 CrossRef CAS.
-
D. A. Derek and A. Long, The Raman effect : a unified treatment of the theory of Raman scattering by molecules, Wiley, 2002 Search PubMed.
- T. Lilo, C. L. M. Morais, C. Shenton, A. Ray and N. Gurusinghe, Photodiagn. Photodyn. Ther., 2022, 38, 102785 CrossRef CAS PubMed.
- A. Hole, P. Jadhav, K. Pansare, H. Noothalapati, A. Deshmukh, V. Gota, P. Chaturvedi and C. M. Krishna, Vib. Spectrosc., 2022, 122, 103414, DOI:10.1016/j.vibspec.2022.103414.
- J. Sun, X. Xu, S. Feng, H. Zhang, L. Xu, H. Jiang, B. Sun, Y. Meng and W. Chen, Talanta, 2023, 253, 123807, DOI:10.1016/j.talanta.2022.123807.
- H. J. Ojeda-Galván, A. C. Hernández-Arteaga, M. C. Rodríguez-Aranda, J. F. Toro-Vazquez, N. Cruz-González, S. Ortíz-Chávez, M. Comas-García, A. G. Rodríguez and H. R. Navarro-Contreras, Spectrochim. Acta, Part A, 2023, 285, 121941 CrossRef PubMed.
- Q. Zeng, C. Chen, C. Chen, H. Song, M. Li, J. Yan and X. Lv, Spectrochim. Acta, Part A, 2023, 286, 122000, DOI:10.1016/j.saa.2022.122000.
- M. Akram, M. I. Majeed, H. Nawaz, N. Rashid, M. R. Javed, M. Z. Ali, A. Raza, M. Shakeel, H. M. ul Hasan, Z. Ali, U. Ehsan and M. Shahid, Photodiagn. Photodyn. Ther., 2022, 103199 CrossRef CAS PubMed.
- Y.-H. Huang, J.-S. Lin, F.-L. Zhang, Y.-J. Zhang, X.-M. Lin, S.-Z. Jin and J.-F. Li, Curr. Opin. Colloid Interface Sci., 2022, 61, 101622 CrossRef CAS.
- Y. J. Liu, M. Kyne, S. Wang, S. Wang, X. Y. Yu and C. Wang, Spectrochim. Acta, Part A, 2022, 282, 121686, DOI:10.1016/j.saa.2022.121686.
- N. George, H. Singh, R. Jotaniya and S. R. Pandya, Forensic Sci. Int., 2015, 252, e10–e16 CrossRef PubMed.
- R. Hinojosa-Nava, E. v. Mejía-Uriarte, A. R. Vázquez-Olmos and R. Y. Sato-Berrú, Spectrochim. Acta, Part A, 2023, 284, 121776 CrossRef CAS PubMed.
- R. R. Jones, D. C. Hooper, L. Zhang, D. Wolverson and V. K. Valev, Nanoscale Res. Lett., 2019, 14, 231 CrossRef PubMed.
-
G. G. Hammes, Spectroscopy for the Biological Sciences, 2005 Search PubMed.
- Y. Itoh and T. Hasegawa, J. Phys. Chem. A, 2012, 116, 5560–5570 CrossRef CAS PubMed.
- A. Kołodziej, A. Wesełucha-Birczyńska, M. Świętek, Ł. Skalniak and M. Błażewicz, J. Mol. Struct., 2020, 1212, 128135, DOI:10.1016/j.molstruc.2020.128135.
- P. Talik, P. Moskal, L. M. Proniewicz and A. Wesełucha-Birczyńska, J. Mol. Struct., 2020, 1210, 128062, DOI:10.1016/j.molstruc.2020.128062.
- E. Turan, A. Zengin, Z. Suludere, N. Ö. Kalkan and U. Tamer, Talanta, 2022, 237, 122926, DOI:10.1016/j.talanta.2021.122926.
-
C. M. Snively and J. L. Koenig, Polymer Applications of IR and Raman Spectroscopy, 1999 Search PubMed.
-
B. H. Stuart, (Vibrational S CTROSO)In/Review Polymer crystallinity studied using Raman spectroscopy, 1996, vol. 10 Search PubMed.
- A. Biselli, A. Echtermeyer, R. Reifsteck, P. Materla, A. Mitsos, J. Viell and A. Jupke, J. Chromatogr. A, 2022, 1675, 463140, DOI:10.1016/j.chroma.2022.463140.
- Y. Su, Y. Miao, Y. Zhu, W. Zou, B. Yu, Y. Shen and H. Cong, Polym. Chem., 2021, 12, 4707–4713 RSC.
- Y. He, Y. Pan, X. Zhao, W. Fan, Y. Cai and X. Mou, Acta Biomater., 2022, 152, 546–561, DOI:10.1016/j.actbio.2022.07.045.
- B. Sana, A. Finne-Wistrand and D. Pappalardo, Mater. Today Chem., 2022, 25, 100963 CrossRef CAS.
- X. Men, X. Geng, Z. Zhang, H. Chen, M. Du, Z. Chen, G. Liu, C. Wu and Z. Yuan, Mater. Today Bio, 2022, 16, 100383, DOI:10.1016/j.mtbio.2022.100383.
- M. A. de Oliveira, M. Guimarães Carvalho Machado, S. E. Dias Silva, T. Leite Nascimento, E. Martins Lima, G. Pound-Lana and V. C. F. Mosqueira, Eur. Polym. J., 2019, 120, 109255, DOI:10.1016/j.eurpolymj.2019.109255.
- A. Bonardi, F. Bonardi, G. Noirbent, F. Dumur, C. Dietlin, D. Gigmes, J. P. Fouassier and J. Lalevée, Polym. Chem., 2019, 10, 6505–6514 RSC.
- G. Noirbent, Y. Xu, A. H. Bonardi, D. Gigmes, J. Lalevée and F. Dumur, Eur. Polym. J., 2020, 139, 110019, DOI:10.1016/j.eurpolymj.2020.110019.
- Q. Li, Y. Ran, W. Shi, M. Qin, Y. Sun, J. Kuang, H. Wang, H. Chen, Y. Guo and Y. Liu, Appl. Mater. Today, 2021, 22, 100899, DOI:10.1016/j.apmt.2020.100899.
- S. Bose, J. R. Summers, B. B. Srivastava, V. Padilla-Gainza, M. Peredo, C. M. Trevino De Leo, B. Hoke, S. K. Gupta and K. Lozano, Opt. Mater., 2022, 123, 111866, DOI:10.1016/j.optmat.2021.111866.
- L. Hu, Q. Hao, L. Wang, Z. Cui, P. Fu, M. Liu, X. Qiao and X. Pang, Polym. Chem., 2021, 12, 545–553 RSC.
- H. Zhang, S. Tian, M. Li, J. Xie, H. Dai, L. Hu and L. Yan, Biomacromolecules, 2022, 23, 3243–3256 CrossRef CAS PubMed.
- C. Malegori, E. Alladio, P. Oliveri, C. Manis, M. Vincenti, P. Garofano, F. Barni and A. Berti, Talanta, 2020, 215, 120911, DOI:10.1016/j.talanta.2020.120911.
- A. R. da Silva Bruni, V. M. A. T. de Oliveira, A. S. T. Fernandez, O. A. Sakai, P. H. Março and P. Valderrama, Food Chem., 2021, 365, 130466, DOI:10.1016/j.foodchem.2021.130466.
- W. Song, S. Zhao, Y. Zhang, C. Ruan, A. Huang, X. Hu, M. Zhao, W. Zhou, J. Wang, X. Wang, H. Wang, Z. Hou and Z. Wang, Constr. Build. Mater., 2022, 325, 126773, DOI:10.1016/j.conbuildmat.2022.126773.
- P. F. Ndlovu, L. S. Magwaza, S. Z. Tesfay and R. R. Mphahlele, J. Food Compos. Anal., 2021, 102, 104035, DOI:10.1016/j.jfca.2021.104035.
- C. T. Kucha, L. Liu, M. Ngadi and C. Gariépy, J. Food Compos. Anal., 2022, 111, 104633, DOI:10.1016/j.jfca.2022.104633.
- R. Kapoor, A. Malvandi, H. Feng and M. Kamruzzaman, Lebensm.-Wiss. Technol., 2022, 154, 112602, DOI:10.1016/j.lwt.2021.112602.
- J. M. Amigo, A. del Olmo, M. M. Engelsen, H. Lundkvist and S. B. Engelsen, Food Chem., 2019, 297, 124946, DOI:10.1016/j.foodchem.2019.06.013.
- J. Simon, O. Tsetsgee, N. A. Iqbal, J. Sapkota, M. Ristolainen, T. Rosenau and A. Potthast, Carbohydr. Polym., 2022, 278, 118887, DOI:10.1016/j.carbpol.2021.118887.
- H. K. Angeyo and S. Gari, Appl. Radiat. Isot., 2022, 186, 110274, DOI:10.1016/j.apradiso.2022.110274.
- C. Sringarm, S. Numthuam, R. Singanusong, S. Jiamyangyuen, S. Kittiwatchana, S. Funsueb and S. Rungchang, Lebensm.-Wiss. Technol., 2022, 167, 113876 CrossRef CAS.
-
T. Tamir, Springer Series in Optical Sciences, vol. 69 Search PubMed.
- R. M. Dittmar, R. A. Palmer and R. O. Carter, Appl. Spectrosc. Rev., 1994, 29, 171–231 CrossRef CAS.
- G. Mirschel, O. Daikos, K. Heymann, U. Decker, T. Scherzer, C. Sommerer, B. Genest and C. Steckert, Prog. Org. Coat., 2014, 77, 1682–1687 CrossRef CAS.
- M. Cheng, Q. Fu, B. Tan, Y. Ma, L. Fang, C. Lu and Z. Xu, Prog. Org. Coat., 2022, 167, 5404 CrossRef.
- Y. Hattori, M. Sugata, H. Kamata, M. Nagata, T. Nagato, K. Hasegawa and M. Otsuka, J. Drug Delivery Sci. Technol., 2018, 46, 111–121 CrossRef CAS.
-
K. S. Anseth, C. Decker and C. N. Bowman, Communications to the Editor Real-Time Infrared Characterization of Reaction Diffusion during Multifunctional Monomer Polymerizations, 1995, vol. 28 Search PubMed.
- F. de S. de Borges, F. J. H. Moraes, M. A. Pereira Neto, J. R. M. d’Almeida, A. C. Bento and N. Cella, Polym. Adv. Technol., 2022, 33(12), 4341–4354, DOI:10.1002/pat.5864.
- A. Karlas, N. A. Fasoula, K. Paul-Yuan, J. Reber, M. Kallmayer, D. Bozhko, M. Seeger, H. H. Eckstein, M. Wildgruber and V. Ntziachristos, Photoacoustics, 2019, 14, 19–30 CrossRef PubMed.
- J. Hayden, M. Giglio, A. Sampaolo, V. Spagnolo and B. Lendl, Photoacoustics, 2022, 25, 100330, DOI:10.1016/j.pacs.2022.100330.
- S. v. Ovsepian, I. Olefir, G. Westmeyer, D. Razansky and V. Ntziachristos, Neuron, 2017, 96, 966–988 CrossRef CAS PubMed.
-
T. Gensch, C. Viappiani and S. E. Braslavsky, in Encyclopedia of Spectroscopy and Spectrometry, Elsevier, 2016, pp. 539–547 Search PubMed.
- T. Schmid, Anal. Bioanal. Chem., 2006, 384, 1071–1086 CrossRef CAS PubMed.
- R. C. M. Sales, M. F. Diniz, R. C. L. Dutra, G. P. Thim and D. Dibbern-Brunelli, J. Mater. Sci., 2011, 46, 1814–1823 CrossRef CAS.
- M. L. Mckelvy, T. R. Britt, B. L. Davis, J. K. Gillie, L. A. Lentz, A. Leugers, R. A. Nyquist and C. L. Putzig, Infrared Spectrosc., 1996, 93–160 CAS.
-
N. Chattopadhyay, M. van der Auweraer and F. C. de Schryver, Determination of the nature of the lowest triplet state of the intramolecular charge-transfer probes DMABN and DMABA by laser-induced optoacoustic spectroscopy, 1997, vol. 279 Search PubMed.
- M. Topa and J. Ortyl, Materials, 2020, 13(18), 4093, DOI:10.3390/ma13184093.
-
P. Gill, T. T. Moghadam and B. Ranjbar, Differential Scanning Calorimetry Techniques: Applications in Biology and Nanoscience, 2010 Search PubMed.
- E. Habib and X. X. Zhu, Polymer, 2018, 135, 178–184 CrossRef CAS.
- J. M. Antonucci and E. E. Toth, J. Dent. Res., 1983, 62, 121–125 CrossRef CAS PubMed.
- J. Drzeżdżon, D. Jacewicz, A. Sielicka and L. Chmurzyński, TrAC, Trends Anal. Chem., 2019, 110, 51–56 CrossRef.
- D. S. Esen, F. Karasu and N. Arsu, Prog. Org. Coat., 2011, 70, 102–107 CrossRef CAS.
- X. Zhang, N. Li, Z. Wei, B. Dai and S. Han, Renewable Energy, 2022, 196, 737–748 CrossRef CAS.
- R. Chakraborty and M. D. Soucek, Eur. Polym. J., 2008, 44, 3326–3334 CrossRef CAS.
- J. Jakubiak and J. F. Rabek, POLIMERY, 2000, 45, nr–7 Search PubMed.
- W. Tomal and J. Ortyl, Eur. Polym. J., 2022, 180, 111588, DOI:10.1016/j.eurpolymj.2022.111588.
- M. Yamato, A. Imai and H. Kawakami, Polymer, 2022, 259, 125339, DOI:10.1016/j.polymer.2022.125339.
- C. Capitain, J. Ross-Jones, S. Möhring and N. Tippkötter, Int. Biodeterior. Biodegrad., 2021, 265, 128731, DOI:10.1016/j.ibiod.2020.104914.
- T. W. Jarrells and E. J. Munson, J. Pharm. Sci., 2022, 111, 2765–2778 CrossRef CAS PubMed.
- J. Bachmann, E. Gleis, S. Schmölzer, G. Fruhmann and O. Hinrichsen, Anal. Chim. Acta, 2021, 1153, 338268, DOI:10.1016/j.aca.2021.338268.
- E. Andrzejewska, E. Socha and M. Andrzejewski, Polymer, 2006, 47, 6513–6523 CrossRef CAS.
- J. Kabatc, J. Ortyl and K. Kostrzewska, RSC Adv., 2017, 7, 41619–41629 RSC.
- C. Gorsche, R. Harikrishna, S. Baudis, P. Knaack, B. Husar, J. Laeuger, H. Hoffmann and R. Liska, Anal. Chem., 2017, 89, 4958–4968 CrossRef CAS PubMed.
- W. Tomal, D. Krok, A. Chachaj-Brekiesz, P. Lepcio and J. Ortyl, Addit. Manuf., 2021, 48, 102447, DOI:10.1016/j.addma.2021.102447.
-
S. A. Khan, I. M. Plitz, R. A. Frantz, B. C. Research and R. Bank, In situ technique for monitoring the gelation of UV curable polymers, 1992, vol. 31 Search PubMed.
- B.-S. Chiou, R. J. English and S. A. Khan, Macromolecules, 1996, 29(16), 5368–5374 CrossRef CAS.
- B. Sen Chiou, R. J. English and S. A. Khan, ACS Symp. Ser., 1997, 673, 150–166 CrossRef.
-
S. S. Lee, A. Luciani and J.-A. E. Månson, A rheological characterisation technique for fast UV-curable systems, 2000, vol. 38 Search PubMed.
- P. A. M. Steeman, A. A. Dias, D. Wienke and T. Zwartkruis, Macromolecules, 2004, 37, 7001–7007 CrossRef CAS.
- A. Botella, J. Dupuy, A. A. Roche, H. Sautereau and V. Verney, Macromol. Rapid Commun., 2004, 25, 1155–1158 CrossRef CAS.
- W. D. Cook, S. Chausson, F. Chen, L. le Pluart, C. N. Bowman and T. F. Scott, Polym. Int., 2008, 57, 469–478 CrossRef CAS.
- L. E. Schmidt, Y. Leterrier, J. M. Vesin, M. Wilhelm and J. A. E. Månson, Macromol. Mater. Eng., 2005, 290, 1115–1124 CrossRef CAS.
- B. Golaz, V. Michaud, Y. Leterrier and J. A. E. Mnson, Polymer, 2012, 53, 2038–2048 CrossRef CAS.
- A. K. Higham, C. A. Bonino, S. R. Raghavan and S. A. Khan, Soft Matter, 2014, 10, 4990–5002 RSC.
-
L. G. Lovell’, S. M. Newman2 and C. N. Bowmanl, The Effects of Light Intensity, Temperature, and Comonomer Composition on the Polymerization Behavior of Dimethacrylate Dental Resins, 1999, vol. 78 Search PubMed.
- J. Wang and V. M. Ugaz, Electrophoresis, 2006, 27, 3349–3358 CrossRef CAS PubMed.
-
L. E. Schmidt, D. Schmäh, Y. Leterrier and J. A. E. Månson, in Rheologica Acta, 2007, vol. 46, pp. 693–701 Search PubMed.
- B. J. Love, T. Savart and C. Dove, Macromol. Mater. Eng., 2010, 295, 146–152 CrossRef.
- V. Tomeckova and J. W. Halloran, J. Eur. Ceram. Soc., 2010, 30, 2833–2840 CrossRef CAS.
- D. U. Shah and P. J. Schubel, Polym. Test., 2010, 29, 629–639 CrossRef CAS.
- A. Nejadebrahim, M. Ebrahimi, X. Allonas, C. Croutxé-Barghorn, C. Ley and B. Métral, RSC Adv., 2019, 9, 39709–39720 RSC.
- P. Lepcio, F. Ondreas, K. Zarybnicka, M. Zboncak, O. Caha and J. Jancar, Soft Matter, 2018, 14, 2094–2103 RSC.
- C. Gorsche, R. Harikrishna, S. Baudis, P. Knaack, B. Husar, J. Laeuger, H. Hoffmann and R. Liska, Anal. Chem., 2017, 89, 4958–4968 CrossRef CAS PubMed.
- C. R. Szczepanski, C. S. Pfeifer and J. W. Stansbury, Polymer, 2012, 53, 4694–4701 CrossRef CAS PubMed.
- N. Venkatesh, H. G. Hanumanthraju, K. P. Prashanth, B. K. Venkatesha and L. Yuvaraj, Mater. Today: Proc., 2022, 54, 421–427 CAS.
- K. Ramraji, K. Rajkumar, K. L. Harikrishna and P. Sarmaji Kumar, Mater. Today: Proc., 2022, 62, 1342–1346 CAS.
- J. Schalnat, D. G. Gómez, L. Daelemans, I. de Baere, K. de Clerck and W. van Paepegem, Polym. Test., 2020, 91, 106799, DOI:10.1016/j.polymertesting.2020.106799.
- J. Schalnat, L. Daelemans, I. de Baere, K. de Clerck and W. van Paepegem, Polym. Test., 2021, 103, 107368, DOI:10.1016/j.polymertesting.2021.107368.
- S. Ebrahimi, M. Meunier and A. Soldera, Polym. Test., 2022, 111, 107585, DOI:10.1016/j.polymertesting.2022.107585.
-
K. Arunprasath, M. Vijayakumar, M. Ramarao, T. G. Arul, S. P. Pauldoss, M. Selwin, B. Radhakrishnan and V. Manikandan, in Materials Today: Proceedings, Elsevier Ltd, 2021, vol. 50, pp. 1559–1562 Search PubMed.
- H. Ulus, H. B. Kaybal, F. Cacık, V. Eskizeybek and A. Avcı, Eng. Fract. Mech., 2022, 268, 108507, DOI:10.1016/j.engfracmech.2022.108507.
- J. Jyoti and A. K. Arya, Polym. Test., 2020, 91, 106839, DOI:10.1016/j.polymertesting.2020.106839.
- C. Schmidt and T. Scherzer, J. Polym. Sci., Part B: Polym. Phys., 2015, 53, 729–739 CrossRef CAS.
- H. Ulus, Int. J. Adhes. Adhes., 2022, 114, 103120, DOI:10.1016/j.ijadhadh.2022.103120.
- J. Y. Choi, K. Yanamandra, A. Shetty and N. Gupta, Polymer, 2022, 242, 124562, DOI:10.1016/j.polymer.2022.124562.
-
S. Ravichandran, E. Vengatesan and A. Ramakrishnan, in Materials Today: Proceedings, Elsevier Ltd, 2020, vol. 27, pp. 177–180 Search PubMed.
- A. Azevedo do Nascimento, F. Fernandez, F. S. da Silva, E. P. C. Ferreira, J. D. José and A. P. Cysne Barbosa, Composites, Part A, 2020, 137, 106016, DOI:10.1016/j.compositesa.2020.106016.
- X. Li, W. Huang, Y. Sui, G. Wang, L. Xiao and D. Zhang, Compos. Commun., 2021, 28, 100932, DOI:10.1016/j.coco.2021.100932.
- J. Cheng, X. Yang, L. Dong, Z. Yuan, W. Wang, S. Wu, S. Chen, G. Zheng, W. Zhang, D. Zhang and H. Wang, Polym. Test., 2017, 59, 371–376 CrossRef CAS.
-
K. P. Menard and N. R. Menard, Dynamic Mechanical Analysis, 3rd edn, 2020 Search PubMed.
- P. A. M. Steeman, A. A. Dias, D. Wienke and T. Zwartkruis, Macromolecules, 2004, 37, 7001–7007 CrossRef CAS.
- M. Afsharian, H. Ahn and S. Kamali, Decis. Anal. J., 2022, 4, 100079 CrossRef.
- B. Hilker, K. B. Fields, A. Stern, B. Space, X. P. Zhang and J. P. Harmon, Polymer, 2010, 51, 4790–4805 CrossRef CAS.
- U. Müller, C. Pretschuh, E. Zikulnig-Rusch, E. Dolezel-Horwath, M. Reiner and S. Knappe, Prog. Org. Coat., 2016, 90, 277–283 CrossRef.
- M. Sernek and F. A. Kamke, Int. J. Adhes. Adhes., 2007, 27, 562–567 CrossRef CAS.
- A. K. Higham, L. A. Garber, D. C. Latshaw, C. K. Hall, J. A. Pojman and S. A. Khan, Macromolecules, 2014, 47, 821–829 CrossRef CAS.
- U. Müller, C. Pretschuh, R. Mitter and S. Knappe, Int. J. Adhes. Adhes., 2017, 73, 45–50 CrossRef.
- J. Steinhaus, B. Hausnerova, T. Haenel, M. Großgarten and B. Möginger, Dent. Mater., 2014, 30, 372–380 CrossRef CAS PubMed.
- K. Ariyoshi and Y. Yamamoto, J. Power Sources, 2022, 533, 231360, DOI:10.1016/j.jpowsour.2022.231360.
- A. I. B. Rondão, J. P. F. Grilo, M. Starykevich and F. M. B. Marques, Thermochim. Acta, 2022, 709, 179147, DOI:10.1016/j.tca.2022.179147.
- D. Drdlik, V. Marak, K. Maca and K. Drdlikova, Ceram. Int., 2022, 48, 24599–24608 CrossRef CAS.
- A. Korolev, M. Mishnev and D. V. Ulrikh, Polymers, 2022, 14, 4281 CrossRef CAS PubMed.
-
F. Ramsteiner, Test Apparatus Fluid Dilatometer to Measure the Volume Change of Polymeric Materials During Tensile Tests, 1996, vol. 15 Search PubMed.
- A. Garton and M. H. George, Polymer, 1975, 16, 934–935 CrossRef CAS.
-
M. Hunkel, H. Surm and M. Steinbacher, in Handbook of Thermal Analysis and Calorimetry, Elsevier B.V., 2018, vol. 6, pp. 103–129 Search PubMed.
-
V. D. Mcginniss and R. M. Holsworth, The Use of a Recording Dilatometer to Study the Photopolymerization of Ethylene Glycol Dimethacrylate Using the Isobutyl Ether of Benzoin as Pho t oinit iat or, 1975, vol. 19 Search PubMed.
- R. E. Schwerzel, A. S. Jariwala and D. W. Rosen, J. Appl. Polym. Sci., 2013, 129, 2653–2662 CrossRef CAS.
- T. Z. N. Sokkar, M. A. El-Bakary, M. I. Raslan, M. A. Sewidan and A. A. Hamza, Optik, 2022, 251, 168485, DOI:10.1016/j.ijleo.2021.168485.
- O. J. Kio, J. Yuan, A. J. Brooks, G. L. Knapp, K. Ham, J. Ge, D. van Loo and L. G. Butler, Addit. Manuf., 2018, 24, 364–372 CAS.
- N. Demoli, A. Knežević, Z. Tarle, A. Meniga, J. Šutalo and G. Pichler, Opt. Commun., 2004, 231, 45–51 CrossRef CAS.
- K. Manoli, D. Goustouridis, S. Chatzandroulis, I. Raptis, E. S. Valamontes and M. Sanopoulou, Polymer, 2006, 47, 6117–6122 CrossRef CAS.
- E. A. Fogleman, M. T. Kelly and W. T. Grubbs, Dent. Mater., 2002, 18(4), 324–330 CrossRef CAS PubMed.
- D. T. Van-Pham, K. Sorioka, T. Norisuye and Q. Tran-Cong-Miyata, Polymer, 2011, 52, 739–745 CrossRef CAS.
- W. Shang, S. Li, B. Li, G. Zhang and J. Liu, Measurement, 2022, 198, 111367, DOI:10.1016/j.measurement.2022.111367.
-
N. A. Davidenko, I. I. Davidenko, V. A. Pavlov, N. G. Chuprina and E. v. Mokrinskaya, in Handbook of Nanomaterials for Manufacturing Applications, Elsevier, 2020, pp. 345–417 Search PubMed.
- D. Chen, Z. Yin, Z. Chen, S. Valyukh and Y. Yu, Opt. Lasers Eng., 2022, 157, 107114, DOI:10.1016/j.optlaseng.2022.107114.
-
T. J. Byerley, J. D. Eick, G. P. Chen, C. C. Chappelow and F. Millich, Synthesis and polymerization of new expanding dental monomers, 1992, vol. 8 Search PubMed.
-
A. Maffezzoli and R. Terzi, Cure behaviour of visible light activated dental composites Part II Non-isothermal kinetics, 1995, vol. 6 Search PubMed.
- J. L. Ferracane, Crit. Rev. Oral Biol. Med., 1995, 6, 302–318 CrossRef CAS PubMed.
- N. B. Cramer, J. W. Stansbury and C. N. Bowman, J. Dent. Res., 2011, 90, 402–416 CrossRef CAS PubMed.
- C. S. Pfeifer, Z. R. Shelton, R. R. Braga, D. Windmoller, J. C. MacHado and J. W. Stansbury, Eur. Polym. J., 2011, 47, 162–170 CrossRef CAS PubMed.
- J. W. Stansbury and S. H. Dickens, Dent. Mater., 2001, 17(1), 71–79 CrossRef CAS PubMed.
- S. Imazato, J. F. Mccabe, H. Tarumi, A. Ehara and S. Ebisu, Dent. Mater., 2001, 17(2), 178–183 CrossRef CAS PubMed.
-
M. Schwerdtfeger, S. Lippert, M. Koch, A. Berg, S. Katletz and K. Wiesauer, Terahertz time-domain spectroscopy for monitoring the curing of dental composites, 2000, vol. 22 Search PubMed.
- M. D. Bentley, M. C. Ortiz, E. L. Ritman and J. Carlos Romero, Am. J. Physiol.: Regul., Integr. Comp. Physiol., 2002, 282, R1267–R1279 CrossRef CAS PubMed.
- H. Lu, J. W. Stansbury and C. N. Bowman, Dent. Mater., 2004, 20, 979–986 CrossRef CAS PubMed.
- H. Lu, J. W. Stansbury, S. H. Dickens, F. C. Eichmiller and C. N. Bowman, J. Biomed. Mater. Res., Part B, 2004, 71, 206–213 CrossRef PubMed.
-
C. L. Davidson and A. J. Feilzer, Polymerization shrinkage and polymerization shrinkage stress in polymer-based restoratives, 1997, vol. 25 Search PubMed.
- G. Pignatelli, A. Strasse, A. Gumenyuk and I. Gornushkin, Mater. Test., 2022, 64(1), 24–32, DOI:10.1515/mt-2021-2082.
-
S. Shohan, J. Harm, M. Hasan, B. Starly and R. Shirwaiker, in Procedia Manufacturing, Elsevier B.V., 2021, vol. 53, pp. 636–643 Search PubMed.
- V. Darcos, S. Monge and D. M. Haddleton, J. Polym. Sci., Part A: Polym. Chem., 2004, 42, 4933–4940 CrossRef CAS.
-
N. Sheibat-Othman, D. Peycelon and G. Févotte, in Industrial and Engineering Chemistry Research, American Chemical Society, 2004, vol. 43, pp. 7383–7391 Search PubMed.
- P. B. Zetterlund, H. Yamazoe, B. Yamada, D. J. T. Hill and P. J. Pomery, Macromolecules, 2001, 34(22), 7686–7691 CrossRef CAS.
- J. J. Haven and T. Junkers, Eur. J. Org. Chem., 2017, 2017, 6474–6482 CrossRef CAS.
-
I. Alig, D. Lellinger, S. Agarwal and H. Oehler, in Reactive and Functional Polymers, 2013, vol. 73, pp. 316–322 Search PubMed.
- R. P. Slopek, H. K. McKinley, C. L. Henderson and V. Breedveld, Polymer, 2006, 47, 2263–2268 CrossRef CAS.
- A. Boddapati, S. B. Rahane, R. P. Slopek, V. Breedveld, C. L. Henderson and M. A. Grover, Polymer, 2011, 52, 866–873 CrossRef CAS.
- A. Botella, J. Dupuy, A. A. Roche, H. Sautereau and V. Verney, Macromol. Rapid Commun., 2004, 25, 1155–1158 CrossRef CAS.
- Y. Y. Chiu and J. Lee, Polym. Chem., 1995, 33, 269–283 CrossRef CAS.
- M. Özparpucu, T. Wolfrum, E. Windeisen-Holzhauser, M. Knorz and K. Richter, Eur. J. Wood Wood Prod., 2020, 78, 85–91 CrossRef.
- C. A. McFaul, A. M. Alb, M. F. Drenski and W. F. Reed, Polymer, 2011, 52, 4825–4833 CrossRef CAS.
- J. S. Siqueira, F. H. Florenzano and W. F. Reed, Polymer, 2021, 226, 123798, DOI:10.1016/j.polymer.2021.123798.
- F. H. Florenzano, P. Enohnyaket, V. Fleming and W. F. Reed, Eur. Polym. J., 2005, 41, 535–545 CrossRef CAS.
- C. A. McFaul, M. F. Drenski and W. F. Reed, Polymer, 2014, 55, 4899–4907 CrossRef CAS.
- A. M. Alb and W. F. Reed, Macromol. React. Eng., 2010, 4, 470–485 CrossRef CAS.
-
W. Reed and A. M. Alb, Monitoring Polymerization Reactions: From Fundamentals to Applications, 2014 Search PubMed.
- J. J. Haven, J. Vandenbergh and T. Junkers, Chem. Commun., 2015, 51, 4611–4614 RSC.
- J. H. Vrijsen, I. A. Thomlinson, M. E. Levere, C. L. Lyall, M. G. Davidson, U. Hintermair and T. Junkers, Polym. Chem., 2020, 11, 3546–3550 RSC.
- J. van Herck, I. Abeysekera, A.-L. Buckinx, K. Cai, J. Hooker, K. Thakur, E. van de Reydt, P.-J. Voorter, D. Wyers and T. Junkers, Digital Discovery, 2022, 1, 519–526 RSC.
- D. Fabris, Mass Spectrom. Rev., 2005, 24, 30–54 CrossRef CAS PubMed.
- T. Gruendling, S. Weidner, J. Falkenhagen and C. Barner-Kowollik, Polym. Chem., 2010, 1, 599–617 RSC.
- L. S. Santos, Eur. J. Org. Chem., 2008, 235–253 CrossRef CAS.
- T. W. T. Bristow, A. D. Ray, A. O'Kearney-Mcmullan, L. Lim, B. McCullough and A. Zammataro, J. Am. Soc. Mass Spectrom., 2014, 25, 1794–1802 CrossRef CAS PubMed.
- S. Stockinger, J. Gmeiner, K. Zawatzky, J. Troendlin and O. Trapp, Chem. Commun., 2014, 50, 14301–14309 RSC.
-
J. W. Sam, X.-J. Tang, R. S. Magliozzo and J. Peisach, Electrospray Mass Spectrometry of Iron Bleomycin II: Investigation of the Reaction of Fe(III)-Bleomycin with Iodosylbenzene, 1995, vol. 117 Search PubMed.
- B. v. Silva, F. A. Violante, A. C. Pinto and L. S. Santos, Rapid Commun. Mass Spectrom., 2011, 25, 423–428 CrossRef CAS PubMed.
- H. Y. Wang, W. L. Yim, Y. L. Guo and J. O. Metzger, Organometallics, 2012, 31, 1627–1634 CrossRef CAS.
- L. S. Santos and J. O. Metzger, Angew. Chem., Int. Ed., 2006, 45, 977–981 CrossRef PubMed.
- D. L. Browne, S. Wright, B. J. Deadman, S. Dunnage, I. R. Baxendale, R. M. Turner and S. v. Ley, Rapid Commun. Mass Spectrom., 2012, 26, 1999–2010 CrossRef CAS PubMed.
- C. Dietze, S. Schulze, S. Ohla, K. Gilmore, P. H. Seeberger and D. Belder, Analyst, 2016, 141, 5412–5416 RSC.
- J. J. Haven, E. Baeten, J. Claes, J. Vandenbergh and T. Junkers, Polym. Chem., 2017, 8, 2972–2978 RSC.
- J. J. Haven, N. Zaquen, M. Rubens and T. Junkers, Macromol. React. Eng., 2017, 11(4), 1700016, DOI:10.1002/mren.201700016.
- J. Lange, N. Altmann, C. T. Kelly and P. J. Halley, Polymer, 2000, 41(15), 5949–5955, DOI:10.1016/S0032-3861(99)00758-2.
- B. Chiou and S. Khan, Macromolecules, 1997, 30(23), 7322–7328, DOI:10.1021/ma9708656.
- A. Botella, J. Dupuy, A. A. Roche, H. Sautereau and V. Verney, Macromol. Rapid Commun., 2004, 25(12), 1155–1158, DOI:10.1002/marc.200400087.
- T. Scherzer, R. Mehnert and H. Lucht, Macromol. Symp., 2004, 205(1), 151–162, DOI:10.1002/masy.200450114.
Footnote |
† This paper is dedicated to the memory of Tomasz Świergosz who passed away on June 30, 2022, at the age of 36. |
|
This journal is © The Royal Society of Chemistry 2023 |
Click here to see how this site uses Cookies. View our privacy policy here.