DOI:
10.1039/D3SC03725H
(Edge Article)
Chem. Sci., 2023,
14, 11727-11736
Metal organic layers enabled cell surface engineering coupling biomembrane fusion for dynamic membrane proteome profiling†
Received
19th July 2023
, Accepted 30th September 2023
First published on 5th October 2023
Abstract
Systematically dissecting the highly dynamic and tightly communicating membrane proteome of living cells is essential for the system-level understanding of fundamental cellular processes and intricate relationship between membrane-bound organelles constructed through membrane traffic. While extensive efforts have been made to enrich membrane proteins, their comprehensive analysis with high selectivity and deep coverage remains a challenge, especially at the living cell state. To address this problem, we developed the cell surface engineering coupling biomembrane fusion method to map the whole membrane proteome from the plasma membrane to various organelle membranes taking advantage of the exquisite interaction between two-dimensional metal–organic layers and phospholipid bilayers on the membrane. This approach, which bypassed conventional biochemical fractionation and ultracentrifugation, facilitated the enrichment of membrane proteins in their native phospholipid bilayer environment, helping to map the membrane proteome with a specificity of 77% and realizing the deep coverage of the HeLa membrane proteome (5087 membrane proteins). Furthermore, membrane N-phosphoproteome was profiled by integrating the N-phosphoproteome analysis strategy, and the dynamic membrane proteome during apoptosis was deciphered in combination with quantitative proteomics. The features of membrane protein N-phosphorylation modifications and many differential proteins during apoptosis associated with mitochondrial dynamics and ER homeostasis were found. The method provided a simple and robust strategy for efficient analysis of membrane proteome, offered a reliable platform for research on membrane-related cell dynamic events and expanded the application of metal–organic layers.
Introduction
As the fundamental feature of living cells, biomembranes are the basic barrier between intracellular and extracellular environments, which also serve to divide and separate the organelles.1,2 The membrane proteins (MPs) embedded in or attached to the phospholipid bilayer are encoded by approximately 30% of the genome3 and are essential for various biological processes,4,5 such as cellular signaling,6,7 trafficking,8,9 pathogenesis10 and adhesion,11 thus motivating the choice for over 60% of drug targets.12,13 Since biomembranes are highly dynamic, MPs can be transferred in different membrane-bound organelles at membrane contact sites or through vesicle transport, allowing their coordinate response to different environmental and biological conditions.1,14–17 Therefore, the deep coverage proteome analysis of the whole membrane, including the plasma membrane, organelle membrane, and the membrane contact sites, helps expound the dynamical but highly ordered cell events, and understand the process of life activities more comprehensively and systematically.
Unfortunately, the complex physicochemical properties including low abundance and high hydrophobicity make MP detection extremely challenging.5,18,19 Traditional density gradient centrifugation and ultracentrifugation methods were first used to isolate membrane components for subsequent proteome analysis.20–22 The obtained membrane components were often treated with high-salt and high-pH buffers for membrane washing23–26 to further remove the contaminants adhering to MPs, or extracted through different detergents27–29 to improve the efficiency. However, the multi-step centrifugation and washing made the whole preparation procedure very tedious and inefficient. Alternatively, a solid phase extraction strategy was also developed for MP extraction mainly through hydrophobic interaction between different segments of proteins in lysate and the selected solid matrix such as graphene,30 zeolitic imidazolate frameworks31 and nanodiamond particles.32 The approach simplified the process but proteins with less interaction with the matrix were easily lost, resulting in low coverage. Meanwhile, both methods carried out MP enrichment in the lysate, disengaging from the native lipid-membrane environment and causing protein conformational changes and loss of numerous weakly bound proteins on biomembranes. Accordingly, there is still an urgent demand to develop the enrichment methods of MPs with high selectivity and deep coverage, to achieve the dynamic membrane proteome analysis in any biological process of interest.
The cell surface engineering strategy,33–36 coating the bioactive molecules or functional materials over the cell surface to regulate cell activity and function, provides us with new insights into biomembrane enrichment. Furthermore, two-dimensional metal–organic layers (MOLs) possess unique features including flexible sheet structure, high surface area, abundant accessible active sites and tunable biocompatibility,37–39 making them an ideal candidate for surface engineering of living cells. Herein, in this work we propose the cell surface engineering coupling biomembrane fusion method, termed E-fusion, to cope with the challenge (Fig. 1A). Specifically, the ultra-thin flexible structure of MOL was first prepared for living cell wrapping. The outer plasma membrane was then bound to MOL through multiple interactions, while the inner organelle membranes were then specifically absorbed to the plasma membrane by biomembrane fusion after mild cell lysis. Finally, global analysis of the dynamic membrane proteome was achieved after extraction of MPs from the biomembranes. The strategy enabled MP profiling in intact cells with high selectivity and deep coverage, and was applicable to various cell samples and extracellular vesicles. The combination of E-fusion and post-translational modification analysis technology provided modification information with membrane specificity. By integrating with quantitative proteomics based on pseudo-isobaric dimethyl labeling, we could realize dynamic delineation of MPs in a variety of physiological and pathological processes to discover critical MP functions and elucidate molecular mechanisms of diseases. In general, the developed E-fusion strategy was proven to be a robust and universal approach with high selectivity and deep coverage for membrane proteome analysis.
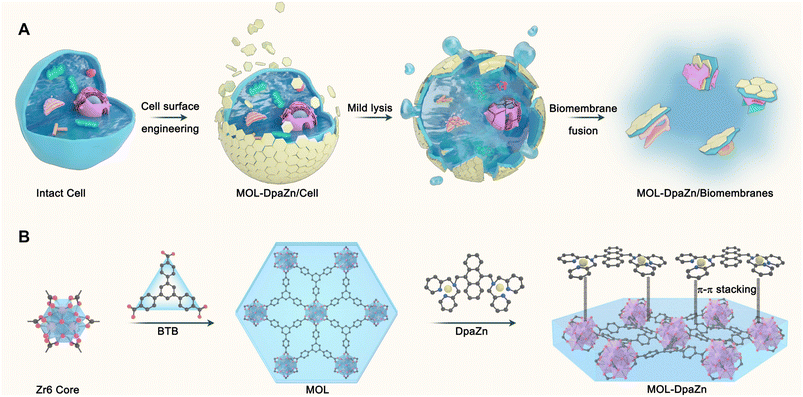 |
| Fig. 1 Schematic illustration of the E-fusion method and preparation of two-dimensional functional metal organic layers (MOL-DpaZn). | |
Results
Development of the E-fusion method
Essential to achieving global MP enrichment using the E-fusion method was the engineering of the cell surface. To establish strong interaction with the cell membrane, we set our sight on ultra-thin flexible MOL materials which showed superior properties at the living system interface due to their low cytotoxicity and multiple interactions. Furthermore, 2Zn(II)-chelated bis (dipicolylamine) complex, denoted as DpaZn, showed a high binding constant (around 10−7 M) with the phosphate on the phospholipid bilayer.40 Therefore, we envisaged that the functionalization of MOL with DpaZn (MOL-DpaZn) would effectively enhance the interaction with the cell membrane. In our experiment, we first synthesized the MOL [Zr6O4(OH)4(BTB)2(OH)6(H2O)6; BTB = 1,3,5-benzenetribenzoate] as the matrix for cell surface engineering due to the low cytotoxicity and high stability of the constituting zirconium clusters, and then DpaZn was deposited on its surface mainly by strong π–π stacking (Fig. 1B). Atomic force microscopy (AFM) images (Fig. S1†) of the material gave a thickness of about 10 nm suggesting the multilayer nanosheet structure. Theoretically speaking, MOL-DpaZn has the potential to realize cell surface engineering.
Fluorescein labeled MOL (F-MOL) was co-incubated with HeLa cells to evaluate the engineering ability. As visualized using confocal laser scanning microscopy in Fig. 2A and S2A,† F-MOL achieved relatively complete coverage of the whole cell, and was increasingly selectively adsorbed on the cell membrane with the extension of incubation time, without entering the cells at all. Based on this success, we tested whether the MOL–plasma membrane system would further adsorb organelle membranes mediated by biomembrane fusion, and therefore, allowing global MP identification. We found the co-localization of F-MOL with fluorescent mitochondrial membranes and with fluorescent nuclear membranes after mild lysis of the pre-engineered cells (Fig. 2B and S2B†), which validated the occurrence of fusion. Transmission electron microscopy (TEM) and scanning electron microscopy (SEM) were used to observe the interaction of MOL with biomembranes. The cells were co-incubated with MOL-DpaZn in culture medium, and after mild lysis, the MOL-DpaZn/biomembrane system was obtained. Compared with MOL-DpaZn, the MOL-DpaZn/biomembrane system maintained the wrinkled layered structure but exhibited lower transparency and a rougher surface (Fig. 2C–F), corresponding to the change in the surface properties of the material caused by biomembrane adsorption.41 Notably, the MOL-DpaZn/biomembrane system showed the presence of P element (Fig. 2G and H), which strongly proved the adsorption of biomembranes to MOL-DpaZn. Finally, the MOL-DpaZn/biomembrane system was generated ready for global MP enrichment.
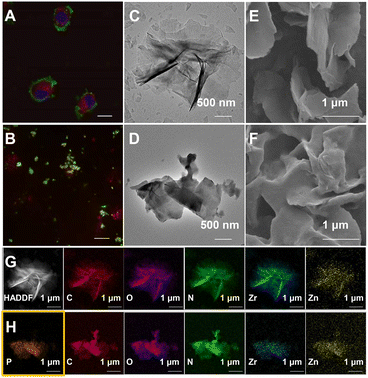 |
| Fig. 2 Development and validation of E-fusion as a valuable approach for MP enrichment. (A) Confocal microscopic image of HeLa cells incubated with F-MOL (0.1 mg mL−1, 3 h). DiI (1,1′-dioctadecyl-3,3,3′,3′ tetramethylindocarbocyanine perchlorate) (10 μg mL−1, 25 min) and Hoechst 33342 (bisBenzimide H 33342) (5 μg mL−1, 30 min) were for cytoplasm and nucleus stain, respectively. Scale bar: 10 μm. (B) Confocal microscopic image showing the co-localization of F-MOL with mitochondrial membranes. Mito Tracker Deep Red (100 nM, 25 min) was for mitochondria stain. Scale bar: 10 μm. (C) TEM images of MOL-DpaZn and (D) MOL-DpaZn/biomembranes. (E) SEM images of MOL-DpaZn and (F) MOL-DpaZn/biomembranes. (G) TEM mapping of MOL-DpaZn and (H) MOL-DpaZn/biomembranes. | |
Exploration of the cell surface engineering mechanism
We continued to make efforts to elucidate the interactions between MOL-DpaZn and plasma membrane. The contact angle of MOL and MOL-DpaZn indicated hydrophilicity, suggesting the hydrophilic interaction with the hydrophilic periphery of phospholipid bilayers caused by the soluble proteins, glycan components and phospholipid hydrophilic head. After incubation, we found the enhanced hydrophobicity of the material with the contact angle increasing from 31.2° to 58.8° (Fig. 3A), verifying the successful engineering and fusion of the overall hydrophobic biomembranes. In addition, the immobilization of the DpaZn complex on the one hand increases the positive charge of the material, and on the other hand it can enhance the coordination between the material and the phospholipid bilayer. As shown in Fig. 3B, compared with MOL, MOL-DpaZn exhibited more positive surface potential, while the transition from positive to negative potential occurred after biomembrane adsorption, indicating strong electrostatic interaction. Furthermore, X-ray photoelectron spectroscopy (XPS) intensities of Zr 3d and Zn 2p were significantly reduced, which might be attributed to the formation of metal–phosphate coordination (Fig. 3C and D) on the cell surface. Taken together, the material might first be adsorbed to the cell surface through hydrophilic interaction, followed by electrostatic and coordinative interactions between the material and the phospholipid bilayer, enabling cell surface engineering. After mild lysis, the cell membrane was bonded on the material surface, which provided basis for the fusion of organelle membranes. The feasibility of the strategy would be further validated by the following MP identification results.
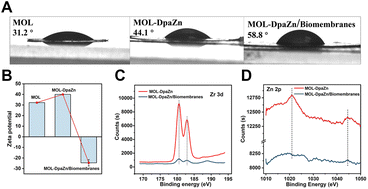 |
| Fig. 3 Exploration of the cell surface engineering mechanism. (A) Contact angle of MOL, MOL-DpaZn and MOL-DpaZn/biomembrane system. (B) Zeta potential of MOL, MOL-DpaZn and MOL-DpaZn/biomembrane system. (C) Zr 3d spectra and Zn 2p spectra (D) obtained by the XPS of MOL-DpaZn and MOL-DpaZn/biomembrane system. | |
Optimization of MP enrichment workflow
We systematically optimized the MP enrichment workflow to maximize the selectivity and coverage. When referring to incubation concentration and time of MOL-DpaZn, cell viability and protein perturbation were first considered. As shown in Fig. 4A, there was a negligible influence on cell viability when cells were incubated with 0.1 mg mL−1 MOL-DpaZn for 3 h, but the cell viability dropped to 80% when the concentration was further increased to 0.2 mg mL−1. Further proteomic perturbation data presented in Fig. S3† show that proteins with at least 2.0-fold abundance change between the MOL-DpaZn treated samples (0.1 mg mL−1, 3 h) and the control accounted for less than 5.0%, suggesting the negligible proteomic perturbation of MOL-DpaZn under these conditions. We tried to reduce the incubation time to 1 or even 0.5 h to shorten the workflow, however, proteome data showed less MP identification number (Fig. 4B). Thus, the incubation conditions of 0.1 mg mL−1 and 3 h were chosen as our optimal conditions for MP enrichment.
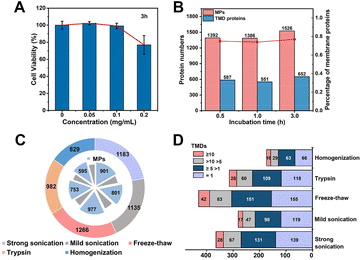 |
| Fig. 4 Optimization of MP enrichment workflow. (A) Cytotoxicity test of the MOL-DpaZn material at different incubation concentrations. (B) Total number of MPs and TMD proteins identified with three LC-MS/MS runs after incubation with MOL-DpaZn for different times. (C) Identified proteins and MPs with different cell lysis methods. (D) Comparison of TMD proteins identified with different cell lysis methods, including homogenization, trypsin, freeze–thaw, mild and strong sonication. | |
We reasoned that the lysis of MOL-DpaZn engineered cells would destroy the relative integrity of biomembranes and perturb the interaction between material and biomembranes, causing the loss of MPs from the MOL-DpaZn/biomembrane system. We compared the proteome data of HeLa cells identified under five different lysis methods (Fig. 4C and D). The repeated freezing and thawing resulted in the identification of more MPs and especially transmembrane domain (TMD) containing proteins, proving our hypothesis that the mild lysis helps the construction of the MOL-DpaZn/biomembrane system for subsequent MP extraction. For extraction and solubilization of MPs, the ionic liquid 1-dodecyl-3-methylimidazolium chloride (C12Im-Cl) buffer (10%, m/v) was used due to its superiority in low abundance MP identification according to previous studies.27,42,43 The addition of pyrophosphate in the extraction buffer, enabling competitive extraction theoretically, did not increase the identification, indicating the sufficient extraction strength of C12Im-Cl (Fig. S4†). Taken together, we determined the relatively optimal conditions for the E-fusion method.
Identification of membrane proteome in intact HeLa cells
Based on the optimized conditions, we performed membrane proteome analysis with intact HeLa cells. Generally, MPs were extracted from the obtained MOL-DpaZn/biomembrane system and analyzed by LC-MS/MS (LTQ Orbitrap Velos mass spectrometer) (Fig. 5A). As a result, we identified 1985 proteins and 1526 of them were annotated as MPs through the Gene Ontology (GO) database and TMHMM algorithm (a predictor of protein transmembrane helix). The MP ratio was as high as 77%, demonstrating excellent selectivity (Fig. 5B, Data 1). In particular, out of the top 100 proteins of PSM, 91 (91%) were MPs and additional 8 (8.0%) proteins were membrane-related proteins with the GO cellular component term of ‘extracellular’ (Data 1). Furthermore, numerous organelle MPs were identified in addition to the plasma membrane proteins after the mild disruption of MOL-DpaZn pre-engineered cells. The subcellular distribution of MPs is shown in Fig. 5B (Data 1). In particular, we noticed the marker MPs of different organelles. For example, CD 44, EGFR, and IGF2R of the plasma membrane, GOLM1/2 and GOLGB1 of Golgi, CANX and EMC complex in the endoplasmic reticulum, COX and TOM complex in the mitochondrion, SUN1/2 and NUP complex in the nucleus and LAMP1/2 in the lysosome were positively enriched. In addition to these typical organelles, the MPs of vesicles were captured, supported by the identification of the core component of COPI and COPII vesicle including the TMED complex, and the SNARE complex which mediates membrane fusion at the vesicle membrane.44 More importantly, membrane contact sites (MCS),14,45 as the cellular signaling hub to link organelles to coordinate various cellular processes, are mediated by protein complexes and crucial for cell homeostasis.17 Our method could enrich the MPs in MCS including endoplasmic reticulum–Golgi intermediate compartment (ERGIC) and mitochondria-associated membrane (MAM), suggesting the potential to explore dynamic membrane trafficking. To further explore the characteristics of the identified 1526 MPs, we performed GO enrichment analysis using DAVID. It was found that proteins were highly involved in various transport biological processes (Fig. 5C), suggesting the ability to strongly enrich hydrophobic transporters. Focusing on non-MPs, we noticed that 150 membrane-related proteins with cellular component annotations of ‘extracellular’ which might possess weak interaction with the plasma membrane were also identified, demonstrating the superiority in identification selectivity and coverage.
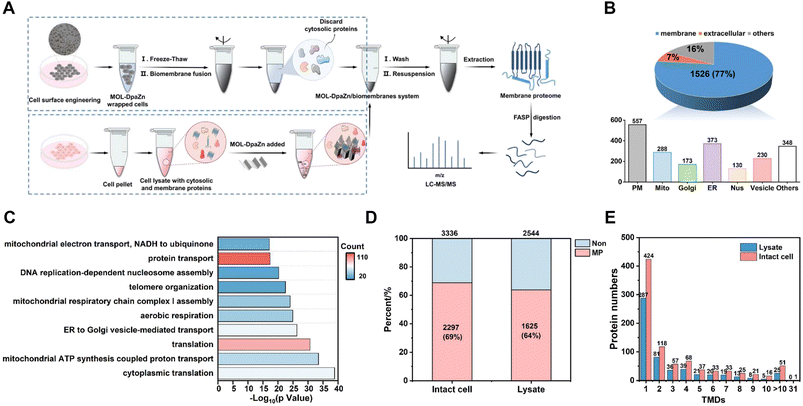 |
| Fig. 5 Identification of membrane proteome in intact HeLa cells. (A) Workflow of membrane proteome profiling in intact cell or in lysate. (B) Distribution of the identified proteins using the E-fusion method with three LC-MS/MS runs. (C) Biological processes in GO enrichment analysis for 1526 MPs identified using the E-fusion method. Comparison of the enrichment using MOL-DpaZn in intact cell and lysate from the identified membrane (D) and TMD (E) proteins. | |
We further compared the data with that of whole cell lysate to verify the efficiency of the method. As shown in Fig. S5A and B,† the E-fusion method resulted in the higher selectivity and deeper coverage identification of MPs (1358, 78%), while 1007 (58%) MPs were detected in the whole cell lysate. For both proteins identified, E-fusion treatment enhanced the intensity of MPs and inhibited the identification of non-MPs according to label-free MS quantitative analysis (Fig. S5C†). And hydrophobic proteins with positive GRAVY values increased from 143 (8.2%) to 325 (19%) after E-fusion treatment (Fig. S5D†), indicating the feasibility of this strategy.
Generally, MPs, especially multi-transmembrane proteins, tend to degrade without the phospholipid bilayer environment. The E-fusion method was based on the interaction between MOL-DpaZn and the phospholipid bilayer, enabling MP enrichment while the phospholipid bilayer remains relatively intact. For an intuitive comparison, the MOL-DpaZn was incubated with intact cells and cell lysate, respectively. MOL-DpaZn was added into the cell culture medium for E-fusion treatment, while equal amounts of material were incubated with the cell lysate obtained by ultrasound as the control group (Fig. 5A). As a result, we identified 1625 MPs containing 554 TMD proteins from the lysate sample, whereas 2297 MPs including 884 TMD proteins were identified from the intact cell sample (Fig. 5D and E), which is nearly 1.5 times improvement. Furthermore, the E-fusion method improved the quality of the mass spectra from MPs according to the higher PSM and sequence coverage of co-identified 1395 MPs as distributed in Fig. S6A and B.† The higher intensity towards co-identified MPs and especially TMD proteins also proved the above conclusion (Fig. S6C and D†). More importantly, many MPs perform specific cellular functions, such as signal transduction and ion transport, through the formation of protein complexes. However, the direct lysis treatment may lead to the loss of certain components of MP complexes, especially those with weak or transient binding, suggesting the urgency and necessity of the in situ strategy. We were encouraged by the results (Fig. S6E and F†) that 313 complexes/clusters from 1588 nodes and 8910 PPIs were found in the intact cell sample, whereas the lysate sample showed only 229 complexes/clusters from 1149 nodes and 7251 PPIs. From the specific analysis of the unique proteins identified in the intact cell sample, we observed some representative MP complexes such as Golgi-associated COPI and COPII vesicle complex, G-protein complex and mitochondrial respiratory chain complex I (Fig. S6G†). Therefore, significant improvements in identification coverage and data quality were obtained by the E-fusion method taking advantage of the relatively intact biomembrane structure, especially for TMD proteins and MP complexes. Furthermore, by contrast with the commercial MP extraction kit (Invent Biotechnologies, Inc), we found better TMD protein and MP complex identification results, and more extraction yields by the E-fusion method (Table S1†).
Reproducibility and universality of MP identification
The above results demonstrated the excellent selectivity and coverage of the E-fusion method. Motivated by this progress, we proceeded to examine whether this technique allowed for identification with excellent reproducibility and universality. We first repeated the whole enrichment process in the HeLa cell with three batches of the MOL-DpaZn material, which were prepared in different months. As shown in Fig. 6A, the numbers of identified proteins from three runs of each batch 1, 2, and 3 sample were 3658, 3336, and 3360 proteins, respectively, and of those, 2471, 2297, and 2299 were MPs, respectively. We found that approximately 70% of MPs were repeatedly identified in three batches, demonstrating the excellent qualitative reproducibility. Furthermore, label-free identification was then performed to further validate the quantitative reproducibility. For the identified proteins, quantitative values across every 2 batches were used to calculate the coefficient of variation (CV). As visualized in Fig. 6B, every 2 batches showed very low variation with a median CV of 11%, 12%, 8.6%, respectively. Furthermore, we created a matrix of correlation coefficients comparing the protein quantities in all MS runs against each other (Fig. 6C). We found high correlations above 0.95 between different batches. Accordingly, both qualitative and quantitative results unequivocally demonstrated the excellent reproducibility of the E-fusion method, which might provide reliable results for biological research.
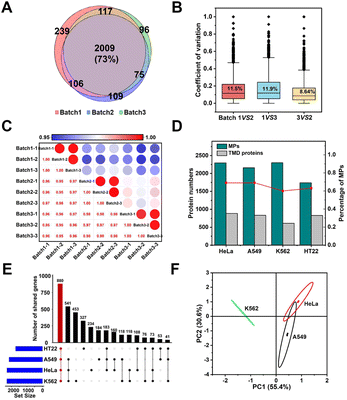 |
| Fig. 6 Reproducibility and universality of the E-fusion method. (A) Overlap of MPs identified using the MOL-DpaZn material of three batches with three LC-MS/MS runs. (B) Quantification CVs between the two batches. (C) Pearson correlation of all quantified protein abundance values per groups. (D) Bar graphs indicated the number and ratio of MPs identified in different biological samples. (E) Distribution of the identified membrane genes in different biological samples visualized by upset plot. The bar chart on top visualized the number of proteins contained within each intersection as defined in the lower part. (F) Principal component analysis of quantified MPs in human biological samples. | |
In addition to the excellent reproducibility, we further sought to investigate the universality among different biological samples. We incubated the material with living adherent cells HeLa and A549, suspension cells K562 and neuron cells HT22, respectively. As shown in Fig. 6D, we successfully mapped the membrane proteomic landscapes of all these cells, and the MPs were identified with similar selectivity and coverage with a common intersection of 880 genes (Fig. 6E). Through Principal Component Analysis (PCA) (Fig. 6F), we were encouraged to find that samples originating from the same cell type clustered separately, suggesting the relatively unique membrane signature for each cell type. Therefore, E-fusion was capable of mapping membrane proteome in different cells with high reproducibility.
In depth analysis of HeLa cell membrane proteome
The well-characterized enrichment performance demonstrated the suitability of our method to map membrane proteome in intact cells with high coverage and robustness. To further improve the coverage of HeLa membrane proteome analysis, high pH reverse phase liquid chromatography (Hp-RP) fractionation technology was further applied. After dividing into 10 fractions, we identified 9692 proteins containing 5087 MPs (corresponding to 5087 genes) and 1962 TMD proteins distributed in different organelles (Fig. S7A,† Data 2) by using more powerful Exploris™ 480 MS. Especially, 42 GPCRs, 117 CD antigens (corresponding to 130 MPs) and 358 transport proteins (including 182 solute carrier transporters and 70 ion channels) were positively identified (Data 2). As far as we know, this is currently the largest dataset of HeLa cell MPs. GO analysis showed that the identified MPs mainly participated in the following biological functions (Fig. S7B†), such as vesicle mediated transport, endocytosis and mitochondrial ATP synthesis coupled protein transport. In summary, this map of the HeLa membrane proteome provides resources for further functional research through various membrane associated signaling pathways.
Profiling of membrane N-phosphoproteome
MP phosphorylation plays a critical role in signal transduction that mediate various physiological processes including proliferation, apoptosis, inflammation, subcellular transport and so on.46–48 However, N-phosphorylation research is seriously lagging behind O-phosphorylation due to the relative instability. Our group has previously developed bis(zinc(II)-dipicolylamine)-functionalized sub-2 μm core–shell silica microspheres (SiO2@DpaZn) for HeLa cell lysate N-phosphorylation peptide enrichment.49 Therefore, we expected to integrate this method with E-fusion for preliminary exploration of membrane N-phosphoproteome to enhance signal transduction and drug mechanism studies. MPs extracted from MOL-DpaZn/HeLa biomembranes were reduced, alkylated and digested for SiO2@DpaZn based N-phosphorylation peptide enrichment. After removing the pLys/pArg peptides localized to the C-terminus, 118 N-phosphorylation sites (34 pHis, 45 pLys and 39 pArg, Fig. S8A,† Data 3) assigned to 95 MPs with localization probability over 0.75 were identified. The N-phosphorylated MPs were significantly enriched in endoplasmic reticulum organization, rRNA processing, protein folding and cell–cell adhesion according to GO analysis (Fig. S8B†). We further explored the uniqueness of N-phosphorylation on different organelle membranes, as shown in Fig. S8C.† We identified relatively more N-phosphorylated plasma membrane proteins and sites (Data 3), including pLys of CD36, pArg of SLC4A2, pHis of SLC4A7, ATP2B1 and CAV2. Interestingly, an insulin receptor tyrosine kinase substrate protein was found carrying 3 N-phosphorylated sites (1 pHis and 2 pLys), which might expand our understanding of related signal transduction. Many pLys sites were also identified on the endoplasmic reticulum membrane and nuclear membrane, including pLys of PGRMC1/2 receptors and Nesprin-1/2. The identified N-phosphorylated sites on the mitochondrial membrane were relatively few, and assigned to HK1(Hexokinase), BAD and CDS2, respectively. Amino acid motif analysis using the WebLogo of the 118 N-phosphorylation sites (Fig. S8D†) revealed that acidic amino acids (D, E) were highly enriched around N-phosphorylation sites, and serine was also found nearby, indicating that N-phosphorylation and O-phosphorylation might have a crosstalk. The possible biological functions of N-phosphorylation modification could be inferred from these motif results. In addition, these motifs could be used as clues to identify potential kinases, phosphorylases and other binding proteins and provide guidance for the preparation of specific antibodies to further reveal the function of N-phosphorylation. Taken together, the E-fusion method provided a sample pre-processing technique for enrichment analysis of post translational modifications such as unstable N-phosphorylation.
Dynamic change of the membrane proteome during apoptosis
Apoptosis, as a highly regulated form of cell death, plays vital roles in cell growth and the homeostasis of multicellular organisms,50 during which extensive membrane remodeling was found to sense stress and transport apoptotic signals51–54 including membrane permeabilization,55 vesicular processes,53,56 membrane blebbing57 and so on. To gain insights into these dynamic membrane changes, we utilized human neuroblastoma cell line SY5Y to implement the E-fusion method under different stages of etoposide stimulation (Fig. 7A). We first examined the etoposide-induced apoptosis caused by DNA damage. The cell viability and morphological changes proved the occurrence of apoptosis (Fig. S9A and B†). And the immunoblot analysis of marker proteins (Fig. 7B) made the apoptosis reliable. For membrane proteome dynamics analysis during apoptosis, the incubation of 100 μM etoposide for 6 h and 18 h of SY5Y cells, corresponding to the early and late apoptosis, was chosen.
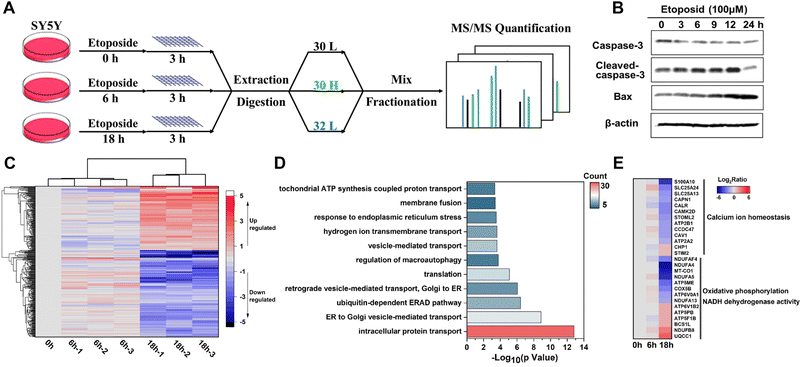 |
| Fig. 7 Revealing the membrane proteome dynamics in the etoposide induced apoptosis of SY5Y cells. (A) Workflow of dynamic membrane proteome mapping in etoposide induced SY5Y cells. (B) Immunoblotting analysis of Caspase-3 and Bax protein in SY5Y cells under exposure of etoposide (100 μM). (C) The heat map shows the dynamic changes of 353 identified MPs during etoposide treatment. (D) Biological processes in GO enrichment analysis for the identified 283 differential MPs in the group treated with etoposide for 18 h compared with those in the control group. (E) The heat map shows the dynamic changes of some typical mitochondrial and ER MPs. | |
With the quantitative analysis of pseudo-isobaric dimethyl labeling with advantages of high quantification accuracy,58 the dynamic changes of 496 proteins at 3 time points were identified, among which 353 were annotated as MPs (Data 4). High biological reproducibility was achieved, as a quantitative ratio from three independent biological replicates correlated well in each time point (Fig. 7C). The heatmap analysis also revealed that 0 h and 6 h results were more closely related, corresponding to the relatively slight changes in the early apoptosis process. We focused on the data with 18 h treatment. GO analysis of differential MPs (fold change > 2, P < 0.05) throughout the apoptosis process indicated that ubiquitin-dependent ERAD pathway, vesicle-mediated transport, endoplasmic reticulum stress and hydrogen ion transport might have potential implications for apoptosis (Fig. 7D). Of these differential MPs (283), 40 were also quantified in the whole proteome data, and the dynamic changes during apoptosis were completely consistent (Data 4).
Furthermore, we could understand the apoptosis process from the perspective of different organelle membranes. We found the change of many mitochondrial MPs, which agreed with the close connection between mitochondrial dynamics and apoptosis.59–61 The upregulation of OMM (outer mitochondrial membrane) proteins61 NLRX1 and MYO19 and downregulation of YME1L1 (refs. 62 and 63) and OPA1 (ref. 64) (key proteins that mediate inner mitochondrial membrane fusion) were reported to increase mitochondrial fission. Endophilin B1,65 as a protein that directly links mitochondrial morphogenesis processes with apoptosis induction, could induce extensive mitochondrial fragmentation and was also up-regulated. Some differential proteins were highly enriched in biological processes related to oxidative phosphorylation and NADH dehydrogenase (ubiquinone) activity (Fig. 7E), which might be due to the mitochondrial dysfunction caused by abnormal mitochondrial dynamics. We also found the involvement of endoplasmic reticulum (ER) MPs in apoptosis. As shown in Fig. 7E, many calcium homeostasis related proteins were downregulated after a long time of etoposide treatment, which might suggest imbalance of Ca2+ metabolism66,67 and perturbations in ER homeostasis.68,69 The downregulation of unfolded protein response (UPR) related proteins (ERO1A, VCP)70 and BAP31 (refs. 71 and 72) (a broad-specificity MP chaperone and quality control factor which could support ER and mitochondrial homeostasis) also suggested ER stress and occurrence of apoptosis. Notably, many differential ER membranes and Golgi MPs participated in the vesicle-mediated transport between ER and Golgi; their upregulation might indicate extensive trafficking between these compartments to integrate stress signals sensed in apoptosis,56 such as SEC24C and SEC31A, and the downregulation might be caused by damaged organelle membrane structure or cleaved by caspases,73 such as COPB2, SEC16A and VAPA. Besides, expressions of several proteins associated with nucleocytoplasmic transport (RAN, SCE1L, IPO9, NUP50, NUP153), endocytosis (SMAP1, CHMP6, EPS15R, TRFC, CAV1, ITSN1), and transmembrane signal transduction (plexin D1/B2, IGF1R) were significantly changed, but related studies are still patchy and their functions in apoptosis remain poorly characterized.
Analysis of the quantitative data revealed the general consistency between the changes of MPs and their expected apoptosis-related function and also disclosed proteins without clear roles in apoptosis which may provide us a new direction to explore the mechanism of etoposide-induced apoptosis in human neuroblastoma cells. Taken together, our results demonstrated the utility and advantage of the E-fusion method to delineate the orderly changes in cell response to environmental apoptosis stimulus signals from the perspective of various types of MPs and provided some meaningful findings to help understand molecular mechanisms of DNA damage induced apoptosis.
Discussion
Our initial goal aimed at the cell surface engineering via MOL to capture plasma membrane proteins. And we identified 2207 plasma membrane proteins using Exploris™ 480 mass spectrometry. Interestingly, we found that organelle MPs were also efficiently identified. We deduced that the plasma membrane modified MOL was obtained after mild lysis, and then the organelle membranes were rapidly adsorbed to them possibly through biomembrane fusion. Therefore, the cell surface engineering coupling biomembrane fusion method was developed for MP profiling in intact cells. It was found that the phospholipid bilayer was crucial for surface engineering and identification. For Gram-negative bacteria lacking teichoic acid, it was difficult for MOL-DpaZn to form an effective wrapping, resulting in poor identification (Fig. S10†). We added urea in the washing buffer during the washing process. Even though the concentration was increased to 1.0 M, the number remained stable (Fig. S4†), which suggested that the membrane was tightly bound to the material. As shown in Fig. 5 and S7,† our work exhibited excellent selectivity and coverage. By comparison with lysate level enrichment, enhanced identification coverage and data quality was achieved, especially for TMD proteins and MP complexes.
In addition to various cells, our method is also applicable to vesicles with similar membrane structures. In total, 438 MPs (75%) and 131 TMD proteins were identified from extracellular vesicles (EVs) obtained from the SH-SY5Y cell culture medium (Fig. S10†). The results further proved the excellent universality of the E-fusion method. Furthermore, the method achieved excellent reproducibility and robustness due to the reduction of reaction steps. Therefore, the E-fusion method could provide a reliable platform for studying protein–protein interaction, proteoform, and post-translational modifications of MPs. For example, in combination with the SiO2@DpaZn microspheres prepared in our group, we can study the N-phosphorylation (phosphorylation at histidine, arginine and lysine) of MPs because many histidines involved in transport proteins are phosphorylated, such as KCa3.1 and TRPV5. In situ enrichment of MPs can greatly reduce sample background, thereby increasing the number of low-abundance N-phosphorylation sites.
Regarding the limitations of the new method, one should mention the indistinguishable subcellular organelle localization of the enriched membrane proteins. All the membrane proteins attached to the material were extracted together, making it impossible to obtain each organelle membrane protein separately. Given the mounting concern about plasma membrane proteins for medical research, it may be possible to achieve the enrichment of plasma membrane proteins by utilizing the cell surface targeting ability of materials and combining photocatalytic proximity labeling technology, since the MOL material is easily functionalized by photosensitizers. Furthermore, our method showed poorer MP identification towards Gram-negative bacteria and was a longer enrichment process compared with the kit method. Materials with stronger and more diverse interactions with cell membrane may achieve rapid targeting and efficient enrichment. Overall, the work provided advanced technology for the comprehensive study of MPs.
Conclusions
In summary, we disclosed here the cell surface engineering coupling biomembrane fusion method for MP enrichment in intact cells. This method exhibited excellent selectivity and deep coverage, making it more suitable for dynamic changes of MP study. We identified 1526 (77%) MPs in HeLa cells including marker proteins in various membrane-bound organelles and realized the deep coverage of the HeLa membrane proteome (5087 MPs). And we proved the advantage of enrichment in intact cell compared with that in lysate through the analysis of the identification data of MPs, TMD proteins and MP complexes. With different batches of material, 73% of MPs could be identified repeatedly by this method and the quantitative variation is around 10%, suggesting the qualitative and quantitative reproducibility. We also successfully profiled the membrane proteome of A549, K562, HT22 cells and EVs, portrayed the membrane N-phosphoproteome and revealed membrane proteome dynamics in the etoposide induced apoptosis of SY5Y cells through differential expression of MPs of various organelles. Together, this methodology represents a significant advance toward developing the cell surface engineering coupling biomembrane fusion method for elucidating comprehensive MPs.
Data availability
The proteomics data have been deposited to the ProteomeXchange Consortium via the PRIDE partner repository with the dataset identifier PXD044945.
Author contributions
Conceptualization: Bo Jiang, Lihua Zhang and Yukui Zhang; investigation: Qianqian Jiang, Zichun Qiao, and Yutong Hou; methodology: Qianqian Jiang and He Wang; resources: Zhigang Sui; supervision: Bo Jiang, Zhen Liang, Lihua Zhang and Yukui Zhang; validation: Qianqian Jiang; visualization: Qianqian Jiang and He Wang; writing-original draft: Qianqian Jiang; writing-review & editing: Baofeng Zhao, Bo Jiang, Zhen Liang and Lihua Zhang.
Conflicts of interest
There are no conflicts to declare.
Acknowledgements
This work was supported by the National Natural Science Foundation (21834006, 22074140, 21725506, 32088101), National Key R&D Program of China (2020YFE0202200) and Talent innovation support program of Dalian (2019CT07).
Notes and references
- T. Tamura and I. Hamachi, Curr. Opin. Chem. Biol., 2022, 70, 102182 CrossRef CAS PubMed.
- A.-N. Bondar, Chem. Rev., 2019, 119, 5535–5536 CrossRef CAS PubMed.
- L. Fagerberg, K. Jonasson, G. von Heijne, M. Uhlén and L. Berglund, Proteomics, 2010, 10, 1141–1149 CrossRef CAS PubMed.
- R. S. Hegde and R. J. Keenan, Nat. Rev. Mol. Cell Biol., 2022, 23, 107–124 CrossRef CAS PubMed.
- G. Von Heijne, J. Intern. Med., 2007, 261, 543–557 CrossRef CAS PubMed.
- S. Chen, Z. Xu, S. Li, H. Liang, C. Zhang, Z. Wang, J. Li, J. Li and H. Yang, Angew. Chem., Int. Ed., 2022, 61, e202113795 CrossRef CAS PubMed.
- Y. Chen and W. Weckwerth, Trends Plant Sci., 2020, 25, 930–944 CrossRef CAS PubMed.
- M. M. Kozlov and J. W. Taraska, Nat. Rev. Mol. Cell Biol., 2022, 24, 63–78 CrossRef PubMed.
- L. Zhang, X. Liang, T. Takáč, G. Komis, X. Li, Y. Zhang, M. Ovečka, Y. Chen and J. Šamaj, Plant Biotechnol. J., 2023, 21, 250–269 CrossRef PubMed.
- Y. Zhao, D. Hu, R. Wang, X. Sun, P. Ropelewski, Z. Hubler, K. Lundberg, Q. Wang, D. J. Adams, R. Xu and X. Qi, Nat. Commun., 2022, 13, 1121 CrossRef CAS.
- J. F. Pinello, Y. Liu and W. J. Snell, Dev. Cell, 2021, 56, 3380–3392.e3389 CrossRef CAS PubMed.
- E. Gulezian, C. Crivello, J. Bednenko, C. Zafra, Y. Zhang, P. Colussi and S. Hussain, Trends Pharmacol. Sci., 2021, 42, 657–674 CrossRef CAS PubMed.
- J. Gong, Y. Chen, F. Pu, P. Sun, F. He, L. Zhang, Y. Li, Z. Ma and H. Wang, Curr. Drug Targets, 2019, 20, 551–564 CrossRef CAS PubMed.
- S. Cohen, A. M. Valm and J. Lippincott-Schwartz, Curr. Opin. Cell Biol., 2018, 53, 84–91 CrossRef CAS PubMed.
- P. Lujan, J. Angulo-Capel, M. Chabanon and F. Campelo, Curr. Opin. Cell Biol., 2021, 71, 95–102 CrossRef CAS PubMed.
- F. Aniento, V. Sánchez de Medina Hernández, Y. Dagdas, M. Rojas-Pierce and E. Russinova, Plant Cell, 2022, 34, 146–173 CrossRef PubMed.
- K. C. Cook, E. Tsopurashvili, J. M. Needham, S. R. Thompson and I. M. Cristea, Nat. Commun., 2022, 13, 4720 CrossRef CAS PubMed.
- J. Pauwels, D. Fijalkowska, S. Eyckerman and K. Gevaert, Mass Spectrom. Rev., 2022, 41, 804–841 CrossRef CAS PubMed.
- O. Vit and J. Petrak, J. Proteomics, 2017, 153, 8–20 CrossRef CAS PubMed.
- P. G. Sadowski, T. P. J. Dunkley, I. P. Shadforth, P. Dupree, C. Bessant, J. L. Griffin and K. S. Lilley, Nat. Protoc., 2006, 1, 1778–1789 CrossRef CAS PubMed.
- C. C. Wu, M. J. MacCoss, K. E. Howell and J. R. Yates, Nat. Biotechnol., 2003, 21, 532–538 CrossRef CAS PubMed.
- J. M. Gilmore and M. P. Washburn, J. Proteomics, 2010, 73, 2078–2091 CrossRef CAS PubMed.
- Y. Zhao, W. Zhang, Y. Kho and Y. Zhao, Anal. Chem., 2004, 76, 1817–1823 CrossRef CAS PubMed.
- P. Kongpracha, P. Wiriyasermkul, N. Isozumi, S. Moriyama, Y. Kanai and S. Nagamori, Mol. Cell. Proteomics, 2022, 21, 100206 CrossRef CAS PubMed.
- M. M. Weldemariam, C.-L. Han, F. Shekari, R. B. Kitata, C.-Y. Chuang, W.-T. Hsu, H.-C. Kuo, W.-K. Choong, T.-Y. Sung, F.-C. He, M. C. M. Chung, G. H. Salekdeh and Y.-J. Chen, J. Proteome Res., 2018, 17, 4138–4151 CrossRef CAS PubMed.
- O. Vit, K. Harant, P. Klener, P. Man and J. Petrak, J. Proteomics, 2019, 204, 103411 CrossRef CAS PubMed.
- Q. Zhao, F. Fang, Y. Shan, Z. Sui, B. Zhao, Z. Liang, L. Zhang and Y. Zhang, Anal. Chem., 2017, 89, 5179–5185 CrossRef CAS PubMed.
- Y. Zhou, J. Gao, H. Zhu, J. Xu, H. He, L. Gu, H. Wang, J. Chen, D. Ma, H. Zhou and J. Zheng, Anal. Chem., 2018, 90, 2434–2439 CrossRef CAS PubMed.
- F. Fang, Q. Zhao, X. Li, Z. Liang, L. Zhang and Y. Zhang, Anal. Chim. Acta, 2016, 945, 39–46 CrossRef CAS PubMed.
- A. Uzzaman, Z. Shang, Z. Qiao, C.-X. Cao and H. Xiao, Microchim. Acta, 2018, 185, 123 CrossRef PubMed.
- B. Zhang, M. Guo, H. Wang, Z. Wang, L. Zhang, Y. Zhang, C. Cao and H. Xiao, Anal. Chem., 2021, 93, 15922–15930 CrossRef CAS PubMed.
- M. D. Pham, S. S. F. Yu, C.-C. Han and S. I. Chan, Anal. Chem., 2013, 85, 6748–6755 CrossRef CAS PubMed.
- M. C. Arno, Macromol. Rapid Commun., 2020, 41, 2000302 CrossRef CAS.
- H.-R. Jia, Y.-X. Zhu, Q.-Y. Duan and F.-G. Wu, Chem. Soc. Rev., 2021, 50, 6240–6277 RSC.
- H. R. Jia, Z. Zhang, X. Fang, M. Jiang, M. Chen, S. Chen, K. Gu, Z. Luo, F. G. Wu and W. Tan, Mater. Today Nano, 2022, 18, 100188 CrossRef CAS.
- Y. Wang, G. Huang, Q. Hou, H. Pan and L. Cai, Wiley Interdiscip. Rev.: Nanomed. Nanobiotechnology., 2022, e1875 Search PubMed.
- A. Murali, G. Lokhande, K. A. Deo, A. Brokesh and A. K. Gaharwar, Mater. Today, 2021, 50, 276–302 CrossRef CAS.
- J. Liang and K. Liang, Nano Today, 2021, 40, 101256 CrossRef CAS.
- D. Yu, H. Zhang, Z. Liu, C. Liu, X. Du, J. Ren and X. Qu, Angew. Chem., Int. Ed., 2022, 61, e202201485 CrossRef CAS PubMed.
- D. R. Rice, K. J. Clear and B. D. Smith, Chem. Commun., 2016, 52, 8787–8801 RSC.
- Y. Kang, J. Liu, Y. Jiang, S. Yin, Z. Huang, Y. Zhang, J. Wu, L. Chen and L. Shao, Adv. Drug Delivery Rev., 2021, 175, 113820 CrossRef CAS PubMed.
- F. Fang, Q. Zhao, H. Chu, M. Liu, B. Zhao, Z. Liang, L. Zhang, G. Li, L. Wang, J. Qin and Y. Zhang, Mol. Cell. Proteomics, 2020, 19, 1724–1737 CrossRef CAS PubMed.
- Q. Zhao, H. Chu, B. Zhao, Z. Liang, L. Zhang and Y. Zhang, TrAC, Trends Anal. Chem., 2018, 108, 239–246 CrossRef CAS.
- T.-Y. Yoon and M. Munson, Curr. Biol., 2018, 28, R397–R401 CrossRef CAS.
- Y. Elbaz and M. Schuldiner, Trends Biochem. Sci., 2011, 36, 616–623 CrossRef CAS PubMed.
- K. Pang, W. Wang, J.-X. Qin, Z.-D. Shi, L. Hao, Y.-Y. Ma, H. Xu, Z.-X. Wu, D. Pan, Z.-S. Chen and C.-H. Han, MedComm, 2022, 3, e175 CrossRef CAS PubMed.
- F. Ardito, M. Giuliani, D. Perrone, G. Troiano and L. Lo Muzio, Int. J. Mol. Med., 2017, 40, 271–280 CrossRef CAS PubMed.
- B. C. Orsburn, L. H. Stockwin and D. L. Newton, Expert Rev. Proteomics, 2011, 8, 483–494 CrossRef CAS PubMed.
- Y. Hu, B. Jiang, Y. Weng, Z. Sui, B. Zhao, Y. Chen, L. Liu, Q. Wu, Z. Liang, L. Zhang and Y. Zhang, Nat. Commun., 2020, 11, 6226 CrossRef CAS PubMed.
- L. Gibellini and L. Moro, Cells, 2021, 10, 1765 CrossRef PubMed.
- J. Kale, Q. Liu, B. Leber and D. W. Andrews, Cell, 2012, 151, 1179–1184 CrossRef CAS PubMed.
- S. Bleicken, G. Hofhaus, B. Ugarte-Uribe, R. Schröder and A. J. García-Sáez, Cell Death Dis., 2016, 7, e2121 CrossRef CAS PubMed.
- R. Kakarla, J. Hur, Y. J. Kim, J. Kim and Y.-J. Chwae, Exp. Mol. Med., 2020, 52, 1–6 CrossRef CAS PubMed.
- J. E. Aslan and G. Thomas, Traffic, 2009, 10, 1390–1404 CrossRef CAS PubMed.
- C. Bogner, B. Leber and D. W. Andrews, Curr. Opin. Cell Biol., 2010, 22, 845–851 CrossRef CAS PubMed.
- R. S. Maag, S. W. Hicks and C. E. Machamer, Curr. Opin. Cell Biol., 2003, 15, 456–461 CrossRef CAS PubMed.
- S. Franz, K. Herrmann, B. Führnrohr, A. Sheriff, B. Frey, U. S. Gaipl, R. E. Voll, J. R. Kalden, H. M. Jäck and M. Herrmann, Cell Death Differ., 2007, 14, 733–742 CrossRef CAS PubMed.
- J. Liu, Y. Zhou, Y. Shan, B. Zhao, Y. Hu, Z. Sui, Z. Liang, L. Zhang and Y. Zhang, Anal. Chem., 2019, 91, 3921–3928 CrossRef CAS PubMed.
- S. Hoppins and J. Nunnari, Science, 2012, 337, 1052–1054 CrossRef CAS PubMed.
- K. Cao, J. S. Riley, R. Heilig, A. E. Montes-Gómez, E. Vringer, K. Berthenet, C. Cloix, Y. Elmasry, D. G. Spiller, G. Ichim, K. J. Campbell, A. P. Gilmore and S. W. G. Tait, Dev. Cell, 2022, 57, 1211–1225.e1216 CrossRef CAS PubMed.
- D. F. Suen, K. L. Norris and R. J. Youle, Genes Dev., 2008, 22, 1577–1590 CrossRef CAS PubMed.
- K. Ando, T. Yokochi, A. Mukai, G. Wei, Y. Li, S. Kramer, T. Ozaki, Y. Maehara and A. Nakagawara, Mol. Carcinog., 2019, 58, 1134–1144 CrossRef CAS PubMed.
- Y. Ruan, H. Li, K. Zhang, F. Jian, J. Tang and Z. Song, Cell Death Dis., 2013, 4, e896 CrossRef CAS PubMed.
- C. Frezza, S. Cipolat, O. Martins de Brito, M. Micaroni, G. V. Beznoussenko, T. Rudka, D. Bartoli, R. S. Polishuck, N. N. Danial, B. De Strooper and L. Scorrano, Cell, 2006, 126, 177–189 CrossRef CAS PubMed.
- B. Wang, M. Nguyen, N. C. Chang and G. C. Shore, EMBO J., 2011, 30, 451–452 CrossRef CAS PubMed.
- R. Rizzuto, P. Pinton, D. Ferrari, M. Chami, G. Szabadkai, P. J. Magalhães, F. D. Virgilio and T. Pozzan, Oncogene, 2003, 22, 8619–8627 CrossRef CAS PubMed.
- N. Demaurex and C. Distelhorst, Science, 2003, 300, 65–67 CrossRef CAS PubMed.
- R. Iurlaro and C. Muñoz-Pinedo, EMBO J., 2016, 283, 2640–2652 CAS.
- A. Merighi and L. Lossi, Int. J. Mol. Sci., 2022, 23, 15186 CrossRef CAS PubMed.
- G. Bonsignore, S. Martinotti and E. Ranzato, Int. J. Mol. Sci., 2023, 24, 1566 CrossRef CAS PubMed.
- E. M. Quistgaard, Biochimie, 2021, 186, 105–129 CrossRef CAS PubMed.
- T. Namba, F. Tian, K. Chu, S.-Y. Hwang, K. W. Yoon, S. Byun, M. Hiraki, A. Mandinova and S. W. Lee, Cell Rep., 2013, 5, 331–339 CrossRef CAS PubMed.
- L. E. Araya, I. V. Soni, J. A. Hardy and O. Julien, ACS Chem. Biol., 2021, 16, 2280–2296 CrossRef CAS PubMed.
|
This journal is © The Royal Society of Chemistry 2023 |
Click here to see how this site uses Cookies. View our privacy policy here.