DOI:
10.1039/D2SD00137C
(Paper)
Sens. Diagn., 2023,
2, 390-397
Removal of hemolysis interference in serum Raman spectroscopy by multivariate curve resolution analysis for accurate classification of oral cancers†
Received
8th August 2022
, Accepted 3rd January 2023
First published on 10th January 2023
Abstract
Serum is an important biofluid that accurately reflects pathology and is used for early diagnosis of a wide range of diseases. Like most serological assays, Raman spectroscopic measurements also require serum samples free of hemolysis, i.e., breakdown of red blood cells, as their strong contribution to the Raman spectrum can mask subtle biomolecular changes in serum leading to inaccurate results. Therefore, hemolyzed samples are routinely rejected for serum Raman studies. However, in most cases, it would be difficult to get fresh samples, thereby reducing the sample size for chemometric analysis. In this work, we employed multivariate curve resolution-alternating least squares (MCR-ALS) analysis to extract pure biomolecular components and tried to digitally remove interference due to hemolysis in serum Raman spectroscopy. We demonstrate its application using hemolyzed/non-hemolyzed serum from control hamsters (untreated) and hamsters with 7,12-dimethylbenz[a]anthracene (DMBA)-induced oral tumors. Our results clearly show that even Raman spectra of hemolyzed serum samples can be pre-processed and used to make a differential diagnosis with high accuracy. We believe that the proposed method has huge potential in Raman diagnostics as it can be employed for the so-called routinely used non-hemolyzed serum samples to further improve diagnostic accuracies as shown in this work.
Introduction
Serum as a systemic biofluid is a routinely used biospecimen in clinical analysis. It is composed of an aqueous solution (around 95% water) containing proteins, peptides, amino acids (such as albumins, globulins, lipoproteins, enzymes, and hormones), carbohydrates, lipids, electrolytes, and other small molecules.1 Moreover, twenty-two abundant proteins constitute 99% of the human serum.2–4
In general clinical practice, serum is derived from blood samples by centrifugation steps. Even though the use of serum for disease diagnostics is frequent, it is prone to a serious problem called hemolysis. Hemolysis in blood samples (plasma and serum) is defined as the release of intracellular components (hemoglobin) due to the rupture of red blood cells (RBCs). It may occur because of certain diseases like inherited or acquired hemolytic anemia (in vivo hemolysis), or improper procedures during phlebotomy (in vitro hemolysis).5 Hemolysis in serum causes major interference in disease diagnosis/testing due to which hemolyzed serum samples are rejected for further analysis as they may affect test outcomes.5,6 It can have other practical consequences as repeating sample collection is not always feasible. Furthermore, rejecting a sample means subjecting a patient to another invasive test that can delay diagnosis.7
Visual inspection of hemolysis requires expertise and increases the chances of ambiguity which in turn increases the unreliability of the results.8,9 Recently, many researchers tried to solve the problem by identifying and evaluating the degree of hemolysis. Liu, Y. et al. made use of copper shells anchored on magnetic nanoparticles for the removal of highly abundant histidine-rich proteins from human blood.10 F. Marques-Garcia et al. proposed integral management of strategies based on methods such as sample freezing, osmotic shock, and shear stress to detect the degree of interference by hemolysis.11 Zhang, WZ and Price, DJ proposed a statistical model for in vitro hemolyzed samples to restore potassium which gets wrongly predicted due to hemolysis.12 C. Yang et al. used a deep learning-based system to assess the serum quality for usage in clinical laboratories.13 L. Heireman et al. suggested formalizing the factors responsible for hemolysis so that risk assessment can be done to avoid false clinical reporting.14 M. Bosma et al. used the H-index which aids in the detection of hemolysis in patients with extracorporeal life support.15 All the previous studies reveal mainly strategies to avoid hemolyzed blood samples for clinical analysis. On the other hand, a few proposed methods aim to minimize the hemolyzed content in samples, but are uneconomical, tedious, and practically unviable. Therefore, a unified approach or method is required which can not only detect hemolysis precisely irrespective of its origin but also allow the use of hemolyzed serum samples otherwise considered as biological waste.
Vibrational spectroscopy, in particular, Raman spectroscopy (RS), is one of the most suitable techniques to obtain detailed biochemical and molecular information from any biological sample including cells, tissues and body fluids.16–18 In general, it is a non-invasive, cost-effective, rapid and label-free method with great potential in disease diagnostics.19–21 Indeed, many researchers including us have studied high and low molecular weight serum constituents in both qualitative manner and quantitative manner using RS.22–24 In addition, RS with the help of statistical analyses has the potential for real-time reporting of the degree of hemolysis.25 Further, Raman spectroscopy assisted with chemometric approaches has been proven to increase the sensitivity of blood-based diagnostics and showed the possibility of clinical translation.26 However, for diagnostic purposes, clinical laboratories still require non-hemolyzed samples.27–29
To obtain hemolyzed and non-hemolyzed serum samples for our study, the experimental hamster buccal pouch (HBP) model was utilized. The 7,12-dimethylbenz[a]anthracene (DMBA)-induced oral carcinogenesis HBP model is the most widely used experimental model to study oral cancer progression.30,31 DMBA is a polycyclic aromatic hydrocarbon and a proven pro-carcinogen.32 Oxidation of DMBA by cytochrome P450 enzymes produces electrophilic metabolites that form covalent adducts with DNA and cause DNA damage.33 14 week DMBA treatment is known to induce exogenous tumors in the HBP model.34 Therefore, in this study, we aim to resolve the problem of interference of hemolysis in serum samples during Raman spectroscopic measurements using multivariate curve resolution-alternating least squares (MCR-ALS) analysis.
Materials and methods
Serum samples
Serum samples were obtained from both control hamsters and hamsters with DMBA-induced oral tumors. The hamster buccal pouch (HBP) model is the most widely used experimental model to study oral cancer progression as mentioned earlier. 14 week DMBA (carcinogen) treatment is known to induce exogenous tumors in the HBP model. The animal study was approved by the Institutional Animal Ethics Committee (Project no. 09/2021). The hamsters were housed under standard laboratory conditions in the animal facility at ACTREC, TMC, Kharghar, Navi Mumbai, India, with a commercial diet and with water provided ad libitum. The experiment was performed on six to eight weeks old female golden Syrian hamsters (Mesocricetus auratus). The hamsters were randomly grouped into a control group (untreated) and a DMBA treated group, wherein the right buccal pouch in each hamster was topically treated with 0.5% DMBA (Sigma-Aldrich, MO, USA) using a paintbrush (Camlin, no. 4, Mumbai, India), thrice a week, for 14 weeks. The retro-orbital blood collection technique was used to collect blood samples once every week during the study starting from ‘Week 0’ termed ‘W0’ before the animal experiment commenced. All the blood samples were allowed to clot at room temperature for 2 hours, followed by centrifugation at 3000 rpm for 15 min to separate the serum (supernatant).
For ease of discussion, serum samples belonging to ‘W0’ (untreated) are termed ‘Control’ and samples exhibiting frank carcinogenic changes through weeks 11–14 indicated as ‘W11–W14’ are termed ‘Tumor’ group. Table 1 lists the details of the number of hamster serum samples included in the study.
Table 1 Summary of the number of control and tumor induced hamsters whose serum samples are included in this study
Control group (‘W0’) |
Tumor group (‘W11–W14’) |
DMBA untreated hamsters at week 0 |
DMBA treated hamsters through weeks 11–14 |
Hemolyzed |
Non-hemolyzed |
Hemolyzed |
Non-hemolyzed |
8 |
7 |
10 |
10 |
Raman spectroscopy
Raman spectra of serum samples were recorded using a WITec alpha300R (WITec, GmbH, Germany) confocal Raman microscope. The experimental parameters were as follows: laser excitation wavelength – 532 nm, laser power – 8 mW, grating – 600 grooves per mm, spectral range – 400 to 4000 cm−1, acquisition time 5 s × 10, and objective lens – 50×. All serum samples used in this study were measured in their liquid form after passive thawing on ice as a drop of 8 μl on a CaF2 window. As the serum is a heterogeneous sample, for every serum sample, at least 10 spectra were recorded. Each spectrum was interpolated in the biological fingerprint region (600–1800 cm−1), corrected for cosmic ray signals, and background interference was reduced using a smoothing filter (Savitzky–Golay method; window size 3), followed by vector normalization. Each of the above pre-processed spectra could be used as an individual entity for multivariate analysis, referred to as a spectra-wise approach, or the spectra acquired from each serum sample could be averaged to obtain mean spectra for multivariate analyses, referred to as a patient-wise or sample-wise approach. As the patient-wise approach is preferable to use to avoid misclassifications due to sample heterogeneity,18,24 in the current study, the pre-processed spectra of each serum sample were averaged to obtain a mean representative spectrum.
Multivariate analysis
A common approach was used for every case considered in this study. For each case, at first, the principal component based linear discriminant analysis (PC-LDA) method was employed for evaluating classification performance on the original dataset using commercially available software (Unscrambler). After the preliminary evaluation, we performed singular value decomposition (SVD) analysis to determine the number of significant components contributing to the dataset. SVD was performed using Igor Pro software from Wavemetrics. Based on the results from SVD for each dataset, we typically identified 2 or 3 significant contributions to the data. We further employed the MCR-ALS method with identified parameters to resolve the components into spectral and concentration profiles. Based on the biomolecular inspection, a suitable component was selected and was used to reconstruct the new dataset by matrix multiplication of the respective spectral and concentration profiles. Finally, PC-LDA was performed again on the vector normalized reconstructed dataset and classification accuracies were compared to that of the original dataset to understand the extent of removal of the effects of hemolysis.
Particularly, MCR-ALS with non-negative constraints was used to extract pure components such as heme contributions. Briefly, MCR-ALS is a matrix approximation method that helps to resolve meaningful spectral components along with their concentration profiles as follows.
where
A is a non-negative data matrix of dimensions
m ×
n comprising a number of Raman spectra (
n) and their respective intensities in the form of wavenumbers (
m),
W represents a resolved and pure spectral matrix of dimensions
m ×
k and
H represents the concentration profiles for pure spectral components with dimensions
k ×
n. The number of components
k is generally decided by the user based on singular value decomposition (SVD) analysis. The refinement of the components is done until convergence of the Frobenius norm, which is given as:
In addition to non-negative constraints, regularization parameters in the form of the L1-norm (Lasso regression) between 0 and 0.005 was applied for better optimization of ALS which can be given as:
| (WTW + α2E)H ≈ WTA(HHT + α2E)W ≈ HAT | (3) |
where
E is a
k ×
k all-ones matrix. A Python program designed for spectroscopic studies was used to employ the MCR-ALS method analysis.
35,36
Results and discussion
Raman spectra of hemolyzed and non-hemolyzed serum
Serum is known to be rich in proteins such as albumin and globulins and a Raman spectrum of serum is dominated by protein vibrations. It is important to note that serum also contains biomolecules such as fatty acids, urea, creatinine and various other important metabolites whose subtle changes indicate underlying pathological conditions.2,37 As the norm, hemolyzed samples are generally considered biological waste and are always discarded to avoid any cross-reaction during biochemical testing. The same routine is also usually followed for Raman spectroscopic research in the development of diagnostic applications. In this study, we considered both hemolyzed and non-hemolyzed serum samples from hamsters for Raman spectroscopic measurements and their average spectra from control and DMBA-induced oral cancer groups are presented in Fig. 1.
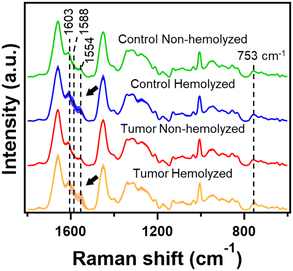 |
| Fig. 1 Average spectra (solid line) with standard deviation (shaded region) of hemolyzed and non-hemolyzed samples from the control and DMBA induced tumor groups. Solid arrows indicate large variation in signals in the hemoglobin marker region. | |
Overall, as expected, all four spectra were dominated by protein signatures such as the tyrosine doublet at 830 cm−1 and 850 cm−1, phenylalanine ring breathing mode at 1004 cm−1, C–H bending at 1340 cm−1 and 1450 cm−1 and amide I band at 1656 cm−1 in addition to contributions from other metabolites. Interestingly, we can also observe heme-related bands such as pyr breathing at 753 cm−1, ν(CC)asym modes at 1588 cm−1 and 1603 cm−1 and ν(Cββ) at 1554 cm−1 in hemolyzed serum from both groups of hamsters indicative of the presence of hemoglobin and its contribution to serum Raman spectra.38,39 Though their intensities seem not so significant in Fig. 1, it is important to keep in mind that they represent average spectra from each sample group and that a large deviation was observed only in Hb marker regions (shaded region indicating standard deviation in Fig. 1). Therefore, we investigated how the random presence of hemolyzed serum samples in the control and DMBA treated group of hamsters affect chemometric analysis and eventually classification accuracies.
Evaluating the effect of hemolysis in Raman spectroscopic analysis of serum
Hemolysis in serum is a major hindrance influencing spectral quality in Raman spectroscopic analysis as Hb bands appear in the same spectral window and significantly overlap with bands from other important biomolecules as mentioned earlier. To understand its effect, we performed analyses based on both the disease state (control/tumor) and hemolysis state (hemolyzed/non-hemolyzed) leading to four combinations mentioned below.
1. Control group containing both non-hemolyzed and hemolyzed serum samples.
2. Tumor group containing both non-hemolyzed and hemolyzed serum samples.
3. Hemolyzed group containing both control and tumor serum samples.
4. Non-hemolyzed group containing both control and tumor serum samples.
Control: hemolyzed vs. non-hemolyzed
In order to understand the effect of hemolysis in serum Raman spectroscopy, we first evaluated hemolyzed and non-hemolyzed serum samples from untreated hamsters taken at week 0, i.e., the control group. In this particular case, we can safely assume that the biomolecular composition of both hemolyzed and non-hemolyzed serum samples is similar except for the contributions such as hemoglobin and others due to the rupture of RBCs. Therefore, in terms of disease state, we should treat both of them as one category irrespective of the degree of hemolysis as they were obtained from the control group. If indeed hemolysis did not affect the serum Raman spectral analysis, one would expect no classification between the two types. However, PC-LDA of the data showed a classification accuracy of 99.3% and the confusion matrix in Table 2 (labelled ‘Before MCR’) indicated differentiability between the two types despite being from the same group. Inspection of the PCA results along with MCR-ALS components clearly indicates that the PC1 component, with significant heme-related features as shown in PC1 loadings which contribute to about 84% of the data, is the main discriminator (ESI† Fig. S1). Therefore, we set out to remove the contribution from hemolysis in the data employing MCR-ALS analysis. The results of the two component MCR-ALS model are presented in Fig. 2. Component 1 showed Raman features such as 1003 cm−1, 1340 cm−1, 1440 cm−1, and 1650 cm−1 primarily from proteins which are commonly observed under non-hemolyzed conditions. Therefore, this component is most likely to retain the characteristic biomolecular content of serum. On the other hand, component 2 showed a Raman spectral profile characteristic of hemoglobin with prominent marker bands at 1603 cm−1, 1588 cm−1, 1554 cm−1, and 753 cm−1.38,39 In short, component 1 is the desired component while the other is not. So, we reconstructed the data considering the spectral and concentration profiles of component 1 only and reevaluated using discriminant analysis. After MCR reconstruction, the classification accuracy dropped to 60% suggesting that the unique heme-related features which interfered in the analysis earlier have been removed successfully. Indeed, the confusion matrix in Table 2 (labelled ‘After MCR’) revealed that half of the hemolyzed samples were misclassified implying randomness. This indicates that the MCR reconstructed data are now homogeneous, contain essential biomolecular information of control serum and can be considered as a single group irrespective of the degree of hemolysis in each sample.
Table 2 Summary of the PC-LDA results before and after MCR analysis for the control group
Control: hemolyzed vs. non-hemolyzed |
Classification by PC-LDA |
|
Before MCR |
After MCR |
Accuracy (%) |
99.3 |
60 |
Confusion matrix |
Predicted →/actual ↓ |
Hemolyzed |
Non-hemolyzed |
Hemolyzed |
Non-hemolyzed |
Hemolyzed |
7 |
0 |
4 |
2 |
Non-hemolyzed |
1 |
7 |
4 |
5 |
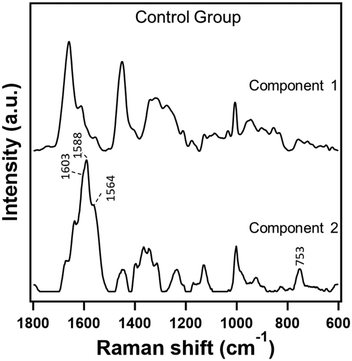 |
| Fig. 2 Raman spectra extracted in a two component MCR-ALS model from hemolyzed and non-hemolyzed serum Raman spectra from the control group. | |
Tumor: hemolyzed vs. non-hemolyzed
With encouraging results from the control group, we tried a similar approach to homogenize hemolyzed and non-hemolyzed serum Raman spectra from the tumor group.
Unlike the control group samples, the tumor group samples are expected to show variation in their biomolecular composition due to both DMBA-induction and degree of hemolysis. Despite belonging to the same group, PC-LDA of the data showed clear classification with a 90% accuracy. Inspection of the confusion matrix (Table 3) along with the results from PCA and MCR-ALS analyses again revealed heme-related components contributing to about 73% of the data to be the primary reason for classification as observed earlier (ESI† Fig. S2). Therefore, we carried out MCR-ALS analysis and constructed a two component model whose resolved components are presented in Fig. 3. Component 1 showed pure protein signatures indicating a heme-free serum composition while component 2 contained significant heme-contributions along with protein Raman features. Reconstruction of the data with heme-free component 1 resulted in successful homogenization of the data with a reduced discrimination accuracy of only 65%. A closer look into the confusion matrix in Table 3 reveals the introduction of randomness in classification of these samples between the two types due to digital removal of the contribution from hemolysis.
Table 3 Summary of the PC-LDA results before and after MCR analysis for the tumor group
Tumor: hemolyzed vs. non-hemolyzed |
Classification by PC-LDA |
|
Before MCR |
After MCR |
Accuracy (%) |
90 |
65 |
Confusion matrix |
Predicted →/actual ↓ |
Hemolyzed |
Non-hemolyzed |
Hemolyzed |
Non-hemolyzed |
Hemolyzed |
9 |
1 |
6 |
3 |
Non-hemolyzed |
1 |
9 |
4 |
7 |
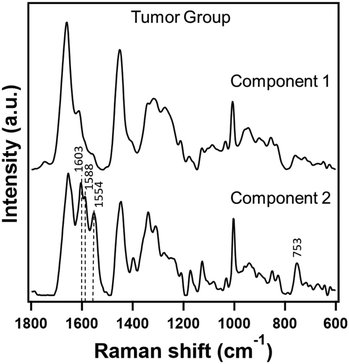 |
| Fig. 3 Raman spectra extracted in a two component MCR-ALS model from hemolyzed and non-hemolyzed serum Raman spectra from the tumor group. | |
Hemolyzed: control vs. tumor
In the two cases discussed above, the category of the dataset was the same, i.e., either control or tumor, and MCR-ALS analysis helped us to remove the interference due to hemolysis and homogenize the data. However, the main goal is to use hemolyzed serum Raman spectral data to make a diagnosis. Therefore, we employed a similar approach to evaluate only hemolyzed data from both control and tumor groups.
It is understandable that the biochemical profiles of serum from these two groups would be different and that one would expect good discrimination by traditional multivariate analyses of Raman spectra. However, contrary to our understanding, the classification accuracy obtained by PC-LDA between the control and tumor groups was only 50% indicating hemolyzed samples to be unreliable for differential diagnosis. Particularly, a close look into the confusion matrix revealed misclassification of 7 out of 10 tumor samples as control (Table 4). Inspection of the PCA loadings and MCR-ALS components (ESI† Fig. S3) revealed that principal component 1 containing heme features contributed significantly to about 73% of the data suggesting its role in the misclassification. Borrowing the idea from two previous cases, we anticipated that removal of the contribution of hemolysis from serum Raman spectra by the MCR-ALS reconstruction approach might improve classification of the two groups. Therefore, keeping the complexity of current data in mind, we constructed a three component MCR-ALS model and the results are shown in Fig. 4. Among the three extracted components, component 1 was heme-free and the other two (components 2 and 3) showed significant heme-related Raman spectral features as discussed earlier. Therefore, to eliminate the effect of hemolysis, we discarded components 2 and 3 and reconstructed the data with only component 1.
Table 4 Summary of PC-LDA results before and after MCR analysis for hemolyzed data from both control and tumor groups
Hemolyzed: control vs. tumor |
Classification by PC-LDA |
|
Before MCR |
After MCR |
Accuracy (%) |
50 |
83.3 |
Confusion matrix |
Predicted →/actual ↓ |
Control |
Tumor |
Control |
Tumor |
Control |
6 |
7 |
7 |
2 |
Tumor |
2 |
3 |
1 |
8 |
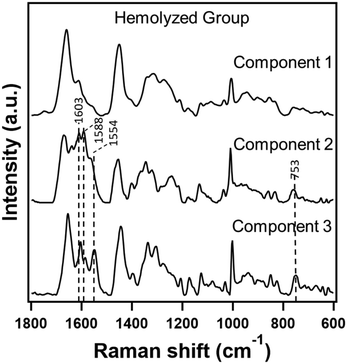 |
| Fig. 4 Raman spectra extracted in a three component MCR-ALS model from hemolyzed serum Raman spectra from both control and tumor groups. | |
Such a simple approach resulted in a marked improvement in classification with about an 83.3% accuracy by PC-LDA. The confusion matrix after MCR reconstruction revealed 8 out of 10 tumor samples now being rightly classified as tumors, demonstrating the effectiveness of our method. In other words, by using MCR-ALS, we can obtain the only component which shows characteristic biomolecular information of control/tumor groups which can be effectively used to make differential diagnosis after removing the unreliable hemolyzed components.
Non-hemolyzed: control vs. tumor
It is important to note that these non-hemolyzed samples were identified as such based on visual inspection. Technically, this means that hemolysis could be to a degree not identifiable in these samples. Given the context, we decided to apply our method to non-hemolyzed serum samples from both control and tumor groups to check if such an approach would help in improving discrimination efficiency. To begin with, PC-LDA of these two groups of data showed moderately good classification with a 76.5% accuracy. Further, we constructed a three component MCR-ALS model whose results are presented in Fig. 5.
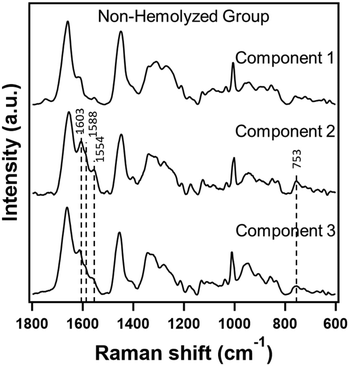 |
| Fig. 5 Raman spectra extracted in a three component MCR-ALS model from non-hemolyzed serum Raman spectra from both control and tumor groups. | |
Though not as pronounced compared to hemolyzed data in the previous sub-section, two among the three contained heme-related spectral signatures. Therefore, following a similar approach, we reconstructed the data with component 1 only and evaluated its performance. Surprisingly, discriminant analysis of MCR reconstructed data outperformed by ∼12% achieving a classification accuracy of 88.2%. In fact, one spectrum from each group which were misclassified earlier has been rightly classified after removing heme contributions as shown in the confusion matrix in Table 5.
Table 5 Summary of the PC-LDA results before and after MCR analysis for non-hemolyzed data from both control and tumor groups
Non-hemolyzed: control vs. tumor |
Classification by PC-LDA |
|
Before MCR |
After MCR |
Accuracy (%) |
76.5 |
88.2 |
Confusion matrix |
Predicted →/actual ↓ |
Control |
Tumor |
Control |
Tumor |
Control |
5 |
2 |
6 |
1 |
Tumor |
2 |
8 |
1 |
9 |
Conclusions
We attempted to make use of hemolyzed serum samples which are generally considered as biological waste in clinical biochemistry. With Raman spectroscopy being a label-free technique, Raman spectra of such hemolyzed serum, though masked by hemoglobin, still contain necessary biomolecular information to make a disease diagnosis. In this work, we introduce a pre-processing method based on MCR-ALS analysis to specifically remove interference due to hemolysis to a reasonable extent with which we can make an accurate diagnosis of oral cancers. The proposed method being a digital remedy effectively has multiple advantages. While on the one hand it can get rid of unwanted components without losing essential biomolecular information from the original dataset, on the other hand, it can also be viewed as a way to make use of the so-called biological waste to make a diagnosis especially in a cost-effective manner when collecting another sample is not feasible. Therefore, we believe that such an approach has huge potential in removing undesired components from serum and even other body fluids and may become an essential step during Raman spectroscopic measurements for reliable diagnosis in the future.
Author contributions
Ajinkya Anjikar: investigation, formal analysis, and writing – original draft. Priyanka Jadhav: investigation, formal analysis, and writing – original draft. Arti Hole: formal analysis. Rajapandian Paneerselvam: methodology and writing – review and editing. Arvind Ingle: resources. Tatsuyuki Yamamoto: supervision and writing – review and editing. Hemanth Noothalapati: conceptualization, methodology, supervision, writing – original draft, writing – review and editing, and funding acquisition. Murali Krishna C: conceptualization, supervision, and writing – review and editing.
Conflicts of interest
There are no conflicts to declare.
Acknowledgements
This work was funded in part by the Grant-in-Aid for Scientific Research of JSPS for H. N. (21K18081), the JSPS International Research Fellowship for M. K. C. (S21114) and support from the Faculty of Life and Environmental Sciences, Shimane University, Japan. P. J. would like to thank CSIR, New Delhi for a fellowship. The authors would like to thank Ms. Aishwarya Naidu and Mr. Monish Joshi for assistance in animal experiments. The proposal no. 09/2021 entitled “Biophysical studies of experimental carcinogenesis in hamster buccal pouch model: deciphering sequential changes” for use of animals for this proposal was approved by the Institutional Animal Ethics Committee of ACTREC, Navi Mumbai and all animal experiments were conducted complying with the guidelines of the Committee for the Purpose of Control and Supervision of Experiments on Animals (CPCSEA), Government of India.
Notes and references
- I. Beheshti, L. M. Wessels and J. H. Eckfeldt, Clin. Chem., 1994, 40, 2088–2092 CrossRef CAS.
- N. Psychogios, D. D. Hau, J. Peng, A. C. Guo, R. Mandal, S. Bouatra, I. Sinelnikov, R. Krishnamurthy, R. Eisner, B. Gautam, N. Young, J. Xia, C. Knox, E. Dong, P. Huang, Z. Hollander, T. L. Pedersen, S. R. Smith, F. Bamforth, R. Greiner, B. McManus, J. W. Newman, T. Goodfriend and D. S. Wishart, PLoS One, 2011, 6(2), e16957 CrossRef CAS.
- P. Gangadharan, A. Sivagnanam, B. Thangasamy, A. Pushparaj, P. Karunakaran, J. Raja, M. Jayaraman and P. Arunachalam, Eur. J. Mol. Clin. Med., 2021, 8, 740–749 Search PubMed.
- N. L. Anderson and N. G. Anderson, Mol. Cell. Proteomics, 2002, 1, 845–867 CrossRef CAS PubMed.
- W. N. W. Azman, J. Omar, T. S. Koon and T. S. T. Ismail, Oman Med. J., 2019, 34, 94–98 CrossRef PubMed.
- G. Lippi, P. Avanzini and G. Cervellin, Clin. Biochem., 2013, 46, 561–564 CrossRef CAS PubMed.
- L. Barbato, M. D. Campelo, S. Pigozzo, N. Realdon, A. Gandini, R. Barbazza, M. L. Coelho, C. Bovo, P. Marini and G. Lima-Oliveira, eJIFCC, 2020, 31, 15–20 Search PubMed.
- R. Hawkins, Ann. Clin. Biochem., 2002, 39, 521–522 CrossRef CAS PubMed.
- M. R. Glick, K. W. Ryder, S. J. Glick and J. R. Woods, Clin. Chem., 1989, 35, 837–839 CrossRef CAS.
- Y. Liu, Y. Wang, M. Yan and J. Huang, BioMed Res. Int., 2017, 2017, 7309481–7309481 Search PubMed.
- F. Marques-Garcia, Electron. J. Int. Fed. Clin. Chem. Lab. Med., 2020, 31, 85–97 CAS.
- W. Z. Zhang and D. J. Price, Clin. Chim. Acta, 2019, 497, 137–140 CrossRef CAS PubMed.
- C. Yang, D. Li, D. Sun, S. Zhang, P. Zhang, Y. Xiong, M. Zhao, T. Qi, B. Situ and L. Zheng, Clin. Chim. Acta, 2022, 531, 254–260 CrossRef CAS PubMed.
- L. Heireman, P. Van Geel, L. Musger, E. Heylen, W. Uyttenbroeck and B. Mahieu, Clin. Biochem., 2017, 50, 1317–1322 CrossRef CAS PubMed.
- M. Bosma, F. Waanders, H. P. Van Schaik, D. Van Loon, S. Rigter, E. Scholten and C. M. Hackeng, J. Crit. Care, 2019, 51, 29–33 CrossRef CAS PubMed.
- N. Hemanth, U. Suguru, O. Naoki, K. Yoshikazu, A. Masahiro, H. Hiro-o and Y. Tatsuyuki, Vib. Spectrosc., 2016, 85, 7–10 CrossRef.
- R. Mojidra, A. Hole, K. Iwasaki, H. Noothalapati, T. Yamamoto, M. K. Chilakapati and R. Govekar, Cell, 2021, 10(10), 2506 CrossRef CAS.
- A. K. Sahu, S. Dhoot, A. Singh, S. S. Sawant, N. Nandakumar, S. Talathi-Desai, M. Garud, S. Pagare, S. Srivastava, S. Nair, P. Chaturvedi and C. Murali Krishna, J. Biomed. Opt., 2015, 20, 115006 CrossRef PubMed.
-
K. Iwasaki, H. Noothalapati and T. Yamamoto, in Vibrational Spectroscopy in Protein Research, ed. Y. Ozaki, M. Baranska, I. K. Lednev and B. R. Wood, Academic Press, 2020, pp. 435–459, DOI:10.1016/B978-0-12-818610-7.00015-3.
- H. Noothalapati, K. Iwasaki and T. Yamamoto, Spectrochim. Acta, Part A, 2021, 258, 119818 CrossRef CAS PubMed.
- A. Hole, P. Jadhav, K. Pansare, H. Noothalapati, A. Deshmukh, V. Gota, P. Chaturvedi and C. M. Krishna, Vib. Spectrosc., 2022, 103414, DOI:10.1016/j.vibspec.2022.103414.
- H. J. Byrne, F. Bonnier, J. McIntyre and D. R. Parachalil, Clinical Spectroscopy, 2020, 2, 100004–100004 CrossRef.
- A. Sahu, N. Nandakumar, S. Sawant and C. M. Krishna, Analyst, 2015, 140, 2294–2301 RSC.
- A. Sahu, S. Sawant, H. Mamgain and C. M. Krishna, Analyst, 2013, 138, 4161–4174 RSC.
- R. Gautam, J. Y. Oh, M. B. Marques, R. A. Dluhy and R. P. Patel, Lab. Med., 2018, 49, 298–310 CrossRef PubMed.
- D. R. Parachalil, J. McIntyre and H. J. Byrne, Anal. Bioanal. Chem., 2020, 412, 1993–2007 CrossRef PubMed.
- G. Lippi, M. Plebani, S. Di Somma and G. Cervellin, Crit. Rev. Clin. Lab. Sci., 2011, 48, 143–153 CrossRef PubMed.
- G. Lippi, N. Blanckaert, P. Bonini, S. Green, S. Kitchen, V. Palicka, A. J. Vassault and M. Plebani, Clin. Chem. Lab. Med., 2008, 46, 764–772 CAS.
- P. Gupta, M. Thomas, N. Sbetan, G. Chacko, I. Savarimuthu, P. Cherian, A. Abas, S. Shiju, S. Karim, A. Kanaan, G. Bautista, N. Elsalasiny, S. A. Balushi, A. E. Haga and M. E. Hassan, Jt. Comm. J. Qual. Patient Saf., 2021, 47, 519–525 Search PubMed.
- J. J. Salley, J. Dent. Res., 1954, 33, 253–262 CrossRef CAS PubMed.
- D. Kanojia and M. M. Vaidya, Oral Oncol., 2006, 42, 655–667 CrossRef CAS PubMed.
- M. Miyata, M. Furukawa, K. Takahashi, F. J. Gonzalez and Y. Yamazoe, Jpn. J. Pharmacol., 2001, 86, 302–309 CrossRef CAS PubMed.
- T. Sugiyama, M. Osaka, K. Koami, S. Maeda and N. Ueda, Leuk. Res., 2002, 26, 1053–1068 CrossRef CAS PubMed.
- B. D. Martinez, P. A. Barato Gomez, C. A. Iregui Castro and J. E. Rosas Perez, BioMed Res. Int., 2020, 2020, 1470868 Search PubMed.
- K. Iwasaki, A. Kaneko, Y. Tanaka, T. Ishikawa, H. Noothalapati and T. Yamamoto, Biotechnol. Biofuels, 2019, 12(128), 1–10 CAS.
- K. Iwasaki, A. Araki, C. M. Krishna, R. Maruyama, T. Yamamoto and H. Noothalapati, Int. J. Mol. Sci., 2021, 22(800), 1–14 Search PubMed.
- S. Giansante, H. E. Giana, A. B. Fernandes and L. Silveira, Jr., Lasers Med. Sci., 2022, 37, 287–298 CrossRef PubMed.
-
B. R. Wood, K. Kochan and K. M. Marzec, in Vibrational Spectroscopy in Protein Research, ed. Y. Ozaki, M. Baranska, I. K. Lednev and B. R. Wood, Academic Press, 2020, pp. 375–414 Search PubMed.
- C. G. Atkins, K. Buckley, M. W. Blades and R. F. B. Turner, Appl. Spectrosc., 2017, 71, 767–793 CrossRef CAS PubMed.
|
This journal is © The Royal Society of Chemistry 2023 |
Click here to see how this site uses Cookies. View our privacy policy here.