DOI:
10.1039/D4AN00181H
(Paper)
Analyst, 2024,
149, 3152-3160
Thermal evaporation as sample preparation for silver–assisted laser desorption/ionization mass spectrometry imaging of cholesterol in amyloid tissues†
Received
1st February 2024
, Accepted 9th April 2024
First published on 9th April 2024
Abstract
Cholesterol plays an important biological role in the body, and its disruption in homeostasis and synthesis has been implicated in several diseases. Mapping the locations of cholesterol is crucial for gaining a better understanding of these conditions. Silver deposition has proven to be an effective method for analyzing cholesterol using mass spectrometry imaging (MSI). We optimized and evaluated thermal evaporation as an alternative deposition technique to sputtering for silver deposition in MSI of cholesterol. A silver layer with a thickness of 6 nm provided an optimal combination of cholesterol signal intensity and mass resolution. The deposition of an ultrathin nanofilm of silver enabled high-resolution MSI with a pixel size of 10 μm. We used this optimized method to visualize the distribution of cholesterol in the senile plaques in the brains of APP/PS1 mice, a model that resembles Alzheimer's disease pathology. We found that cholesterol was evenly distributed across the frontal cortex tissue, with no evidence of plaque-like accumulation. Additionally, we investigated the presence and distribution of cholesterol in myocardial sections of a human heart affected by wild-type ATTR amyloidosis. We identified the presence of cholesterol in areas with amyloid deposition, but complete colocalization was not observed.
1. Introduction
Cholesterol is an essential component of cell membranes that helps to maintain their structural integrity and fluidity. It serves as a precursor for various molecules, including steroid hormones and bile acids. Cholesterol is also an important signaling molecule and the major component of lipid rafts, which are structures that regulate the function of cell signaling pathways.1,2 Disruption of cholesterol homeostasis is associated with a wide range of diseases, including cancer, cardiovascular disease, and various amyloidoses. Amyloidosis is a protein deposition disease in which precursor proteins are pathologically misfolded from their regular physiological conformation into a beta-sheet structure. These misfolded proteins can aggregate into oligomers and later form insoluble amyloid fibrils that are deposited extracellularly in tissues.3 Over 30 different precursor proteins have been identified as amyloidogenic, meaning they have the ability to cause different amyloid diseases.4,5 Amyloid deposition can occur in a systemic or a localized manner. The main types of systemic amyloidosis are AL (primary, light-chain amyloidosis), AA (secondary, inflammation-related amyloidosis), and ATTR (transthyretin amyloidosis).5,6 Alzheimer's disease (AD) is an example of localized amyloidosis and is the most common type of human amyloidosis leading to dementia.7 One of the pathological hallmarks of AD is the accumulation of insoluble β-amyloid (Aβ) in the brain, leading to the formation of senile plaques. Several lines of evidence indicate that cholesterol plays a role in AD pathogenesis. Studies have shown that cholesterol modulates Aβ production by regulating γ-secretase activity.8–10 A recent study found that the targeted deletion of astrocyte cholesterol synthesis reduced Aβ and tau burden in a mouse model of AD.11 Research efforts focused on tracking the distribution and quantity of cholesterol in senile plaques during AD in both humans and animal models have yielded mixed results, and a consensus on this particular aspect of AD pathology has yet to emerge.12–17
Different analytical methods have been used for cholesterol analysis, especially liquid or gas chromatography coupled with mass spectrometry.18,19 To obtain spatially resolved information in tissues, mass spectrometry imaging (MSI) has been utilized in combination with various ionization techniques. These include secondary ion mass spectrometry (SIMS),20–25 desorption electrospray ionization (DESI),26–28 matrix-assisted laser desorption/ionization (MALDI), and laser desorption ionization (LDI).29–34 SIMS detects cholesterol as a dehydrated protonated molecule [M − H2O + H]+ and has been used in many biological applications.35 The intact cholesterol molecule can be directly imaged after adding metals to the experiment.
Recently, silver has gained popularity as a potent signal enhancer in MSI analysis of both cholesterol and unsaturated fatty acids.31 Silver and other metal salts of 2,5-dihydroxybenzoic acid have been used to characterize natural wax esters.36 Adding silver nitrate to DESI solvents increases the specificity and sensitivity of cholesterol and, in general, all double bond-containing molecules.28,37 SIMS and (MA)LDI require the deposition of a thin layer of silver or a silver salt on the sample surface. Two principal methods are used for silver deposition: dry deposition (typically sputtering)32,38–40 and wet deposition, which involves the spraying of silver nanoparticles (AgNPs)41–44 or AgNO3.31
Spraying AgNPs is a common method of wet silver deposition. A wide variety of protocols are available to achieve stable colloidal dispersion of AgNPs in water or organic solvents. Controlling the size and stability of metal NPs is crucial due to their tendency to aggregate in solution.45 During NP synthesis, capping agents such as polyvinylpyrrolidone (PVP),43,46,47 sodium citrate,42,48 and glutathione43,49 are commonly used for this purpose. AgNPs in MSI analysis have been particularly utilized for high-resolution MSI of unsaturated lipids.41–44 Yang et al. recently adopted an alternative approach to wet deposition, investigating the use of AgNO3 instead of AgNPs.31 Silver salt spray deposition is a sensitive and selective technique for analyzing unsaturated compounds, achieving a pixel size down to 10 μm. The main advantages of the method are its ease of implementation and affordability; no additional costly instrumentation or knowledge of AgNP preparation is required. The principal disadvantage is that the sprayer needs to be thoroughly cleaned after each deposition to prevent silver stains and contamination. Two spray runs of pure acetonitrile (ACN) with the addition of 1% trifluoroacetic acid (TFA) have proven to be an effective cleaning approach.31
Sputtering is a physical vapor deposition method used to deposit thin films. It is typically used in scanning electron microscopy to coat samples with conductive materials, usually metals. During the sputtering process, high-energy ions are deployed to displace species from the surface layer of a solid target onto a substrate (sample). Metal nanoparticles are then homogenously deposited onto samples in nanometer-thin layers.33,38 Dufresne et al. developed a silver–assisted laser desorption/ionization (AgLDI) method involving the deposition of silver by sputtering using a beam of charged argon molecules. The method proved effective for analyzing unsaturated compounds, such as cholesterol and unsaturated fatty acids, with a pixel size of 5 μm.32
In this study, we examined the thermal evaporation of silver as an alternative deposition technique in MSI for cholesterol analysis. Thermal evaporation involves heating a source material in a vacuum chamber using a resistive heat source until it evaporates. The vapor then condenses onto a substrate (sample), forming a thin film. We used this optimized method to examine cholesterol distribution in two types of amyloid tissues. First, we examined Aβ pathology in the brain of an APP/PS1 mouse, a widely used transgenic model that mimics AD-like pathology. Second, we obtained a tissue sample from a human heart affected by wild-type ATTR amyloidosis.
2. Experimental section
Samples
Male APP/PS1 mice on a C57Bl/6 background and WT male controls were obtained from the Biotechnology and Biomedicine Centre of the Academy of Sciences and Charles University (BIOCEV) (Vestec, Czech Republic) and housed in the animal facility of the Institute of Organic Chemistry and Biochemistry of the Czech Academy of Sciences (IOCB CAS) under a 12 h light/dark cycle (temperature 23 ± 2 °C). The mice were provided with free access to water and fed a standard rodent diet (ssniff Spezialdiäten GmbH, Soest, Germany). At 10 months of age, the mice were deeply anesthetized with pentobarbital at 170 mg per kg body weight (Sigma-Aldrich, St Louis, MO, USA) and then transcardially perfused with a 0.01 M solution of ice-cold PBS (pH 7.4) supplemented with heparin at 10 U ml−1 (Zentiva, Prague, Czech Republic).48 Mouse brains, including the frontal cortex from the left hemisphere, were dissected, frozen in dry ice-chilled isopentane, and stored at −80 °C. All animal experiments were performed according to the ethical guidelines for animal experiments outlined in Act no. 246/1992 of the Czech Republic and approved by the Committee for Experiments with Laboratory Animals of the Czech Academy of Sciences (CAS).
Human heart samples were obtained from cardiac surgery patients at the Institute for Clinical and Experimental Medicine (IKEM) in Prague. The first subject (a male, age 74 years), who exhibited wild-type ATTR cardiomyopathy, underwent excision from the left ventricle during the implantation of mechanical circulatory support. The second subject was a 46-year-old male donor with a normal echocardiographic examination contraindicated for transplantation due to coronary findings. Tissue samples underwent an initial macroscopic evaluation and were subsequently frozen in their native state for further analysis. All research was conducted following relevant guidelines and regulations of the Institute for Clinical and Experimental Medicine. The protocol was approved by the Ethics Committee of the Institute for Clinical and Experimental Medicine (G-16-06-28).
Sample preparation
Samples were sectioned at a thickness of 12 μm with a cryostat (CM1520, Leica Biosystems) at −18 °C for heart tissue and at −15 °C for brain tissue. The sections were thaw-mounted on precooled indium tin oxide (ITO) glass slides (Bruker, Bremen, Germany), placed in a desiccator for 60 min, and stored at −80 °C until further processing. Before applying the silver or MALDI matrix, the samples were placed in a desiccator at room temperature for 30 min to avoid water condensation on their surfaces.
Silver layer deposition
Silver films were deposited in a custom-made thermal evaporation system (Elettrorava S.p.A., Italy) operated at 5 × 10–6 mbar. The metal was evaporated from a silver wire (99.99% Ag). A quartz crystal microbalance-based thin film deposition controller (XTC/3, Inficon, USA) was used to monitor and control the growth of silver films at different thicknesses (6, 11, and 22 nm), setting the deposition rate to 5 Å s−1. All used settings can be found in ESI Table 1.† The morphology of the silver layer surface was observed using the Nova NanoSEM 450 scanning electron microscope (SEM) (FEI, Hillsboro, OR, USA) for secondary electron imaging and the Olympus IX83 optical microscope (Olympus Europa SE & Co. KG, Germany). The SEM was operated at 10−4 Pa with an acceleration voltage of 10 kV. The Everhart–Thornley detector (ETD) or a through-the-lens detector (TLD) were used to capture secondary electron images.
iMatrixSpray spraying
Before spraying, 10 mg mL−1 of 1,5-diaminonaphthalene (DAN; purity 97%) in 70% acetonitrile (ACN) was freshly prepared. The iMatrixSpray automatic sprayer (Tardo Gmbh, Switzerland) was employed for the spraying process using parameters for dry spraying as previously described.50 ACN and DAN were purchased from Merck (Massachusetts, USA). Water was obtained from a Milli-Q integral system (Merck Millipore, USA).
Mass spectrometry imaging
Experiments were performed on the ultrafleXtreme MALDI-TOF/TOF instrument (Bruker, Germany) equipped with a 1 kHz Smartbeam II Nd:YAG laser (355 nm) in a positive mode within a mass range of 100 to 1200 Da operating in reflectron mode. The source accelerating voltage was set at 25 kV in positive polarity. For each spectrum, 100 laser shots were applied with a laser intensity of 75%. The global laser attenuator offset was set to 36%, and the attenuator offset was set to 0% with an attenuator range of 40%. The spot size was set to small for the measurements. The detector gain voltage was set to 2200 V. Prior to each measurement, calibration was performed using silver clusters. The pixel size ranged from 10 to 50 μm.
Data analysis
Measurement and data evaluation were performed using flexControl and flexImaging software (Bruker, Germany). SCiLS Lab 2016b software (Bruker, Germany) was used to generate of figures and perform statistical analysis. Raw data were converted to SCiLS Lab format using TopHat baseline removal and total ion count (TIC) normalization. Principal component analysis (PCA) and receiver operator characteristic (ROC) analysis were used for statistical evaluation.
Congo red staining
Stokes’ modification of Highman's Congo red technique was employed to detect amyloid51 in heart histological samples. The process involved staining with a saturated Congo red solution for 25 minutes. After washing in distilled and tap water for 5 minutes, the nuclei were counterstained with Mayer's hematoxylin for 5 minutes. The stained sections were then dehydrated and mounted for further analysis using the Olympus BX41 fluorescence microscope (Olympus Europa SE & Co. KG, Germany).
3. Results and discussion
AgLDI mass spectrometry imaging optimization
Silver layer optimization and characterization.
Silver layer thicknesses were optimized on brain tissue sections of control mice. The thermal evaporation method was tested at decreasing Ag film thicknesses of 22 nm, 11 nm, and 6 nm. A quartz crystal microbalance-based thin film deposition controller was used to monitor and control the growth of silver films of different thicknesses at a deposition rate of 5 A/s. The deposition time was approximately 12 s for 6 nm-thin film, 22 s for 11 nm, and 44 s for 22 nm. Cholesterol provided adducts with silver ions at m/z 493.2 [M + 107Ag]+ and m/z 495.2 [M + 109Ag]+. This characteristic isotopic pattern originates from two stable silver isotopes in nature, 107Ag and 109Ag, with atomic abundances of 52% and 48%, respectively. Fig. 1 shows representative AgLDI spectra in the cortex region of a mouse brain section in positive ion mode. When examining cholesterol mass resolution with sufficient signal intensity in relation to different silver layer thicknesses, we observed that greater silver thickness resulted in lower resolution (22 nm, R = 4000; 11 nm, R = 9500; 6 nm, R = 11
500). The best mass resolution was achieved with a silver film thickness of 6 nm.
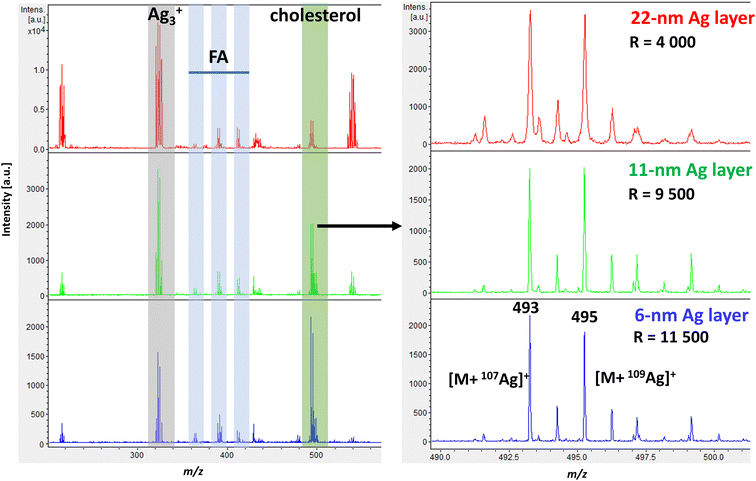 |
| Fig. 1 Representative spectra from AgLDI of a mouse brain section showing the cortex region in positive ion mode. Sections with Ag films deposited using thermal evaporation are given in decreasing thicknesses (22 nm – red, 11 nm – green, 6 nm – blue). Highlighted ions show a silver cluster [Ag3]+, fatty acids [M + 107Ag]+: palmitoleic acid – m/z 363, oleic acid – m/z 389, arachidonic acid – m/z 411, and cholesterol at m/z 493.2 [M + 107Ag]+ and m/z 495.2 [M + 109Ag]+. | |
Similarly, a study by Hansen et al., who investigated sputter-coated metal screening in the small-molecule analysis,38 found that optimal metal layers were relatively thin for all metals analyzed while increasing layer thickness led to poorer quality images. However, the optimal layer thickness can vary slightly depending on the mass spectrometer instrumentation, the analyte of interest, and the type of tissue. Tissues with higher levels of cholesterol and lipids typically require thicker silver layers for effective ionization.32
Additional experiments where the spot size was kept constant and only the laser intensity was varied for different Ag thicknesses are shown in ESI Fig. 1† as ion yield and mass resolution plots. Both 11 nm and 6 nm Ag thicknesses gave similar results in cholesterol signal intensity, with 6 nm showing better resolution. Dufresne et al. investigated the sputtering of silver and AgLDI to analyze olefins. At an optimal silver thickness, they observed a change in ion intensity between the dominant silver cluster (Ag3+) and the cholesterol silver adduct.32 In our case, the change became visible when a 6 nm ultrathin Ag nanofilm was used, as shown in Fig. 1.
As shown in Fig. 1, the representative spectrum of a mouse brain section in the cortex region in positive ion mode at a thickness of 6 nm illustrates the effectiveness of AgLDI in detecting cholesterol. In addition to cholesterol, fatty acids were also detected with lower intensities in the region of m/z 300 to 500. Specifically, palmitoleic acid (PA, m/z 363, m/z 365), oleic acid (OA, m/z 389, m/z 391), and arachidonic acid (AA, m/z 411, m/z 413), were identified as corresponding silver ion adducts. As shown in ESI Fig. 2,† a significant part of the spectrum was occupied by silver cluster ions (Ag2+, Ag3+, Ag3+, etc.). These clusters can be used for mass calibration before measurement or as an internal standard.
Scanning electron microscopy revealed the morphology of silver nanolayers deposited on ITO glass using thermal evaporation (Fig. 2, left). The layer with an optimal thickness of 6 nm was not a continuous film but rather a system of coalesced nanoislands. A similar morphology was previously documented for a silver sputtered on resist glass.52 This coalescence behavior is typical for noble metal films on oxide surfaces.53
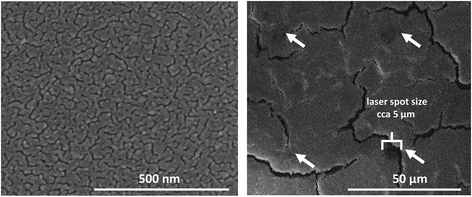 |
| Fig. 2 SEM characterization of ultrathin Ag film (6 nm) deposited using thermal evaporation. Off-tissue image of an ITO glass slide at 120 000× magnification (left). On-tissue image at 800× magnification showing the laser spot size (right). | |
AgLDI MSI reproducibility and pixel size.
The reproducibility of the sample preparation process was evaluated using three consecutive sections of a mouse brain. Silver was deposited separately by thermal evaporation, and each sample was measured individually. The distribution and intensity of cholesterol in these three consecutive sections were very similar (ESI Fig. 3†). The average mean intensity of cholesterol was 3.47 a.u. ± 0.12 a.u., and the average maximum intensity was 63.24 a.u. ± 2.69 a.u. from these sections.
To evaluate high spatial resolution (i.e., pixel size) capabilities, we performed MSI of both the mouse brain and mouse brain cerebellum at decreasing pixel sizes down to 10 μm (Fig. 3). We chose the cerebellum for testing purposes because of its histological features and numerous subregions.54 We detected the highest signal intensity of cholesterol in white matter, particularly in the fiber tracks of the cerebellum and corpus callosum. MSI was adept at distinguishing variations in cholesterol intensity between different layers of the cerebellum, namely the molecular layer, granular layer, and fiber tracks. We also took an optical microscope image of the mouse cerebellum after analysis with laser ablation spots. Even at a pixel size of 10 μm, free space was discernible between the laser ablation spots. Inspection of the spots using a scanning electron microscope (SEM) revealed an average ablation spot of 5 μm (Fig. 2), demonstrating the potential to reach pixel size up to 5 μm.
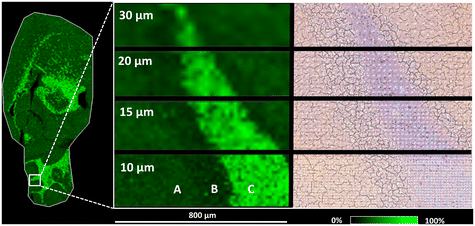 |
| Fig. 3 AgLDI MSI (Ag 6 nm) analysis of cholesterol (m/z 493.2, [M + 107Ag]+). The whole brain section (left) at a spatial resolution of 50 μm. The mouse cerebellum (middle) at spatial resolutions of 30 μm, 20 μm, 15 um, and 10 μm illustrates the distinct variations in cholesterol intensity between (A) the lobule/molecular layer, (B) the lobule/granular layer, and (C) fiber tracks. An optical microscope image (right) of the mouse cerebellum shows the section after analysis with laser ablation spots. | |
Two factors significantly impact pixel size in MALDI: the laser (given that the diameter of the laser spot determines the ablation area) and the size and homogeneity of the matrix crystals on the tissue surface.55,56 In our case, thermal evaporation resulted in a nanolayer of silver. Therefore, the pixel size was primarily dependent on the laser, in particular the diameter of the laser spot. As previously mentioned, Dufresne et al. developed an AgLDI method for imaging cholesterol and other unsaturated analytes involving the deposition of silver using a sputter coating system.32 They found that a nano silver layer with a thickness of 23 ± 2 nm was optimal for analyzing brain tissue. Using laser oversampling, they achieved high resolutions down to a cellular range of 5 μm. We obtained similar results using the thermal evaporation method for silver deposition, achieving a pixel size of 10 μm without the need for laser oversampling. This indicates that thermal evaporation can be a viable alternative deposition method to sputtering in MSI analysis.
Cholesterol distribution in the brain of a mouse model of Alzheimer's disease pathology
Several studies have implicated cholesterol in the pathogenesis of Alzheimer's disease.8–11 However, its presence in senile plaques has only been investigated by a few studies, with mixed results.12–16,57 To examine the distribution of cholesterol in senile plaques, we utilized the APP/PS1 mouse model, a widely used transgenic model of Alzheimer's disease pathology. These double transgenic mice express mutated human amyloid precursor protein (APP) and mutated human presenilin (PS1), which are both linked to early-onset AD and the accumulation of neurotoxic Aβ. Even at six months of age, Aβ deposition in the form of senile plaques is discernible in specific brain regions, such as the hippocampus and cortex, and is accompanied by neuroinflammation and memory impairments.58,59
In our previous studies, we documented the altered distribution of several membrane lipids in the brains of APP/PS1 mice compared with WT controls.50,60 These lipids exhibited plaque-like accumulation in the brain. Using multimodal imaging experiments involving MSI and immunohistochemical staining, we colocalized these lipids with Aβ plaques and neuroinflammation.60 These conclusions are consistent with previously published reports in mouse models and humans, demonstrating the accumulation or depletion of various lipids in AD.16,57,61–65 The gangliosides GM2 and GM3 have also been found to be elevated in the amyloid-like plaques observed in Hunter's disease.66
ROC analysis was employed to detect changes in m/z values. In line with our previous MALDI MSI lipidomic studies, both of which employed 1,5-diaminonaphthalene as the MALDI matrix,50,60 the most notable changes in intensity and distribution applied to gangliosides (GM2 36:1, GM3 36:1) and phosphatidylinositol (PI 38:4). As shown in Fig. 4 (left), plaque-like accumulation of GM3 36:1 is visible in the frontal cortices of APP/PS1 mice compared to wild-type controls. However, this result was obtained using MALDI MSI, which is not sensitive enough for cholesterol analysis. In this study, we aimed to investigate whether cholesterol would accumulate in the same Aβ plaque regions. To that end, we applied an optimized AgLDI mass spectrometry imaging method. Using this technique, we examined cholesterol distribution in a consecutive section of the frontal cortex. As shown in Fig. 4 (right), cholesterol appeared evenly distributed across the frontal cortex tissue, with no evidence of plaque-like accumulation. ROC analysis revealed no significant differences between the APP/PS1 mice and wild-type controls. For additional images from the analysis, see ESI Fig. 4 and 5.†
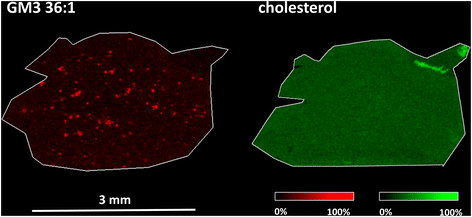 |
| Fig. 4 MALDI (left) and AgLDI (right, Ag 6 nm) MSI analysis of an APP/PS1 mice frontal cortex section. Ion images of ganglioside (GM3 36:1) (red, m/z 1179.7 [M – H]−) and cholesterol (green, m/z 493.2, [M + 107Ag]+) at a spatial resolution of 20 μm. | |
The evidence to date on changes in cholesterol during AD is contradictory. One study utilizing fluorescence microscopy highlighted the accumulation of cholesterol in Aβ plaques.67 However, subsequent research revealed this finding was likely due to false-positive staining.13 Another study, which employed an LC-MS lipidomic approach involving the microdissection of Aβ plaques, reported a two-fold increase in cholesterol in human Aβ plaques compared with tissue without Aβ.17 Time-of-flight secondary ion mass spectrometry (TOF-SIMS) analysis has been used to investigate cholesterol distribution in AD animal models15,16 and humans.14 One of these studies identified cholesterol granules surrounding Aβ plaques in mouse brain tissue overexpressing a mutant form of APP.15 However, a subsequent study using the same model did not confirm these results.16 A study on the human cortex reported an increase in the mean cholesterol signal compared to controls but did not find any cholesterol increase in or around Aβ plaques.14 It's possible that previously reported cholesterol accumulation17 may fall below the sensitivity thresholds of currently available TOF-SIMS imaging methods. A study on AD human brain tissues employing coherent anti-Stokes Raman scattering (CARS) and fluorescence microscopy showed lipid accumulation in fibrillar Aβ plaques. The main components were lipids with long acyl chains, suggesting fatty acids and ceramides. However, the presence of cholesterol could not be excluded due to the partial overlapping of its spectral features with other lipids.12
Cholesterol distribution in the human heart with transthyretin amyloidosis
Cardiac amyloidosis is characterized by abnormal deposition of β-amyloid in the heart, which can lead to heart damage and dysfunction. Of the many amyloid diseases, two types account for more than 95% of all cases of cardiac amyloidosis. These are immunoglobulin light-chain amyloidosis (AL) and transthyretin amyloidosis (ATTR).5 ATTR is caused by misfolding of the liver-derived precursor protein transthyretin (TTR). There are two main types of transthyretin amyloidosis: hereditary (ATTRm) and wild-type (ATTRwt).68,69In vitro studies of amyloidogenic proteins have shown that these proteins bind to lipids in plasma membranes, creating a favorable local environment for protein misfolding and aggregation.70–72 Another study specifically focusing on TTR found that cholesterol and anionic phospholipids increase the binding of TTR to lipid membranes.73
The presence and localization of cholesterol in the heart during ATTR amyloidosis have yet to be examined. In this study, human heart samples, specifically myocardial excisions of the left ventricle, were obtained during surgical intervention. ESI Fig. 6† shows sections of a control human heart and a heart affected by wild-type ATTR amyloidosis after Congo red staining, a common technique used to identify amyloid deposits. When examined under a light microscope, ATTR deposits exhibit in histologic sections orange-red staining.74,75 To investigate the distribution of cholesterol in serial sections of myocardial samples, we utilized the AgLDI mass spectrometry imaging technique. ESI Fig. 7† shows the distribution of cholesterol in the myocardium of a control human heart and a heart with wild-type ATTR amyloidosis. Cholesterol is unevenly distributed in both heart tissues, with certain small spots exhibiting high intensity. The spots in serial sections of the ATTR-affected heart were further examined after Congo red amyloid staining. Fig. 5 and ESI Fig. S8† show that some regions of high cholesterol intensity colocalized with amyloid deposits, but others did not. To test whether these inconsistencies were caused by differences in the serial sections, we compared two images of non-serial sections from the same ATTR-affected heart after Congo red staining (the three sections in between were analyzed by AgLDI). As shown in ESI Fig. 9,† the amyloid deposits in the non-serial sections display a close resemblance to each other. This indicates that differences in the distribution of amyloid and cholesterol between the serial sections were not caused by variations in the sections themselves.
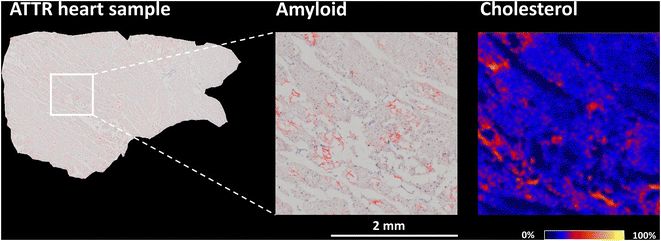 |
| Fig. 5 Congo red amyloid staining (left) and AgLDI MSI analysis (right, Ag 6 nm) of an ATTR-affected myocardial section. Ion image cholesterol (m/z 493.2, [M + 107Ag]+) at a spatial resolution of 20 μm. | |
In vitro studies have shown a correlation between the concentration of cholesterol and the binding of Aβ to membrane lipids.73,76,77 The composition of the lipid membrane plays an important role in binding. Microdomains known as lipid rafts are specialized lipid membrane regions enriched in cholesterol and sphingolipids. These membrane parts are denser and less fluid than the surrounding membrane.78,79 Binding amyloidogenic proteins to lipid rafts may contribute to amyloidosis by inducing conformational changes and aggregation.80,81 In our study, we confirmed the presence of cholesterol in areas containing ATTR deposits. However, complete colocalization with regions of high cholesterol intensity was not found.
4. Conclusions
We optimized the thermal evaporation of silver intending to apply the method to tissue samples for AgLDI analysis of mouse brain tissue sections. An optimal combination of cholesterol signal intensity and mass resolution was achieved using an ultrathin Ag nanofilm with a thickness of 6 nm. We evaluated the reproducibility of the sample preparation process and demonstrated high-resolution MSI with a pixel size of 10 μm with the possibility of decreasing it down to 5 μm. Our results indicate that thermal evaporation is a suitable silver deposition technique for AgLDI analysis, providing comparable results to the sputtering technique. This makes it a promising alternative deposition method for MSI analysis.
Additionally, we investigated the distribution of cholesterol in amyloidosis. First, we investigated cholesterol distribution in senile plaques in the brain of an APP/PS1 transgenic mouse, a model resembling Alzheimer's disease pathology. Using an optimized AgLDI mass spectrometry imaging technique, we found no evidence of plaque-like accumulation of cholesterol in the frontal cortex tissue. In contrast, up-regulated lipids, particularly gangliosides (GM2 36:1, GM3 36:1) and phosphatidylinositol (PI 38:4), showed plaque-like accumulation in the frontal cortices of APP/PS1 mice compared to wild-type controls, in agreement with our previous MSI lipidomic studies.
Second, we investigated cholesterol distribution in a heart affected by wild-type transthyretin amyloidosis. We identified the presence of cholesterol in areas of amyloid deposition, but complete colocalization with regions of high cholesterol intensity was not observed.
Author contributions
Conceptualization: V. V.; funding acquisition: L. M., J. C. and V. M.; investigation: S. S., O. F.; methodology: S. S., V. V. and J. R.; project administration: V. V.; resources: A. M., O. F., M. K. and L. M.; visualization: S. S. and O. F.; writing – original draft: S. S.; writing – review & editing: V. V., O. F., J. R., M. K., V. M., L. M. and J. C; all authors have read and agreed to the published version of the manuscript.
Data availability
The mass spectrometry imaging datasets have been deposited in the public repository Zenodo (https://zenodo.org) under https://doi.org/10.5281/zenodo.8183543.
Conflicts of interest
The authors declare that they have no known competing financial interests or personal relationships that could have appeared to influence the work reported in this paper.
Acknowledgements
Supported by the project National Institute for Research of Metabolic and Cardiovascular Diseases (Programme EXCELES, ID Project No. LX22NPO5104) - Funded by the European Union – Next Generation EU.
We sincerely thank Michael FitzGerald for proofreading the article.
References
- Z. Korade and A. K. Kenworthy, Neuropharmacology, 2008, 55, 1265–1273 CrossRef CAS PubMed.
- R. Vona, E. Iessi and P. Matarrese, Front. Cell Dev. Biol., 2021, 9, 622908 CrossRef PubMed.
- B. P. Hazenberg, Rheum. Dis. Clin. North Am., 2013, 39, 323–345 CrossRef PubMed.
- J. D. Sipe, M. D. Benson, J. N. Buxbaum, S. Ikeda, G. Merlini, M. J. Saraiva and P. Westermark, Amyloid, 2014, 21, 221–224 CrossRef PubMed.
- J. P. Donnelly and M. Hanna, Cleve. Clin. J. Med., 2017, 84, 12 CrossRef PubMed.
- E. Muchtar, A. Dispenzieri, H. Magen, M. Grogan, M. Mauermann, E. D. McPhail, P. J. Kurtin, N. Leung, F. K. Buadi, D. Dingli, S. K. Kumar and M. A. Gertz, J. Intern. Med., 2021, 289, 268–292 CrossRef CAS PubMed.
- J. Ghiso and B. Frangione, Adv. Drug Delivery Rev., 2002, 54, 1539–1551 CrossRef CAS PubMed.
- Q. Liu, C. V. Zerbinatti, J. Zhang, H. S. Hoe, B. Wang, S. L. Cole, J. Herz, L. Muglia and G. Bu, Neuron, 2007, 56, 66–78 CrossRef CAS.
- Q. Liu and J. Zhang, Neurosci. Bull., 2014, 30, 331–345 CrossRef CAS PubMed.
- P. Osenkowski, W. Ye, R. Wang, M. S. Wolfe and D. J. Selkoe, J. Biol. Chem., 2008, 283, 22529–22540 CrossRef CAS PubMed.
- H. Wang, J. A. Kulas, C. Wang, D. M. Holtzman, H. A. Ferris and S. B. Hansen, Proc. Natl. Acad. Sci., 2021, 118, e2102191118 CrossRef CAS PubMed.
- J. Kiskis, H. Fink, L. Nyberg, J. Thyr, J.-Y. Li and A. Enejder, Sci. Rep., 2015, 5, 13489 CrossRef CAS PubMed.
- T. Lebouvier, C. Perruchini, M. Panchal, M.-C. Potier and C. Duyckaerts, Acta Neuropathol., 2009, 117, 31–34 CrossRef CAS PubMed.
- A. N. Lazar, C. Bich, M. Panchal, N. Desbenoit, V. W. Petit, D. Touboul, L. Dauphinot, C. Marquer, O. Laprévote, A. Brunelle and C. Duyckaerts, Acta Neuropathol., 2013, 125, 133–144 CrossRef CAS.
- S. Solé-Domènech, P. Sjövall, V. Vukojević, R. Fernando, A. Codita, S. Salve, N. Bogdanović, A. H. Mohammed, P. Hammarström, K. P. R. Nilsson, F. M. LaFerla, S. Jacob, P.-O. Berggren, L. Giménez-Llort, M. Schalling, L. Terenius and B. Johansson, Acta Neuropathol., 2013, 125, 145–157 CrossRef.
- L. Carlred, A. Gunnarsson, S. Solé-Domènech, B. Johansson, V. Vukojević, L. Terenius, A. Codita, B. Winblad, M. Schalling, F. Höök and P. Sjövall, J. Am. Chem. Soc., 2014, 136, 9973–9981 CrossRef CAS.
- M. Panchal, J. Loeper, J.-C. Cossec, C. Perruchini, A. Lazar, D. Pompon and C. Duyckaerts, J. Lipid Res., 2010, 51, 598–605 CrossRef CAS.
- S. Becker, S. Röhnike, S. Empting, D. Haas, K. Mohnike, S. Beblo, U. Mütze, R. A. Husain, J. Thiery and U. Ceglarek, Anal. Bioanal. Chem., 2015, 407, 5227–5233 CrossRef CAS.
- J. Blondelle, J. P. Pais de Barros, F. Pilot-Storck and L. Tiret, Methods Mol. Biol., 2017, 1668, 39–60 CrossRef CAS PubMed.
- A. Brunelle, D. Touboul and O. Laprévote, J. Mass Spectrom., 2005, 40, 985–999 CrossRef CAS PubMed.
- A. Brunelle and O. Laprévote, Anal. Bioanal. Chem., 2009, 393, 31–35 CrossRef CAS PubMed.
- B. Cillero-Pastor, G. Eijkel, A. Kiss, F. J. Blanco and R. M. Heeren, Anal. Chem., 2012, 84, 8909–8916 CrossRef CAS.
- M. Brulet, A. Seyer, A. Edelman, A. Brunelle, J. Fritsch, M. Ollero and O. Laprévote, J. Lipid Res., 2010, 51, 3034–3045 CrossRef CAS PubMed.
- H. Nygren, K. Börner, B. Hagenhoff, P. Malmberg and J. E. Månsson, Biochim. Biophys. Acta, 2005, 1737, 102–110 CrossRef CAS PubMed.
- H. Nygren, B. R. Johansson and P. Malmberg, Microsc. Res. Tech., 2004, 65, 282–286 CrossRef CAS PubMed.
- L. S. Eberlin, A. L. Dill, A. B. Costa, D. R. Ifa, L. Cheng, T. Masterson, M. Koch, T. L. Ratliff and R. G. Cooks, Anal. Chem., 2010, 82, 3430–3434 CrossRef CAS PubMed.
- C. Wu, D. R. Ifa, N. E. Manicke and R. G. Cooks, Anal. Chem., 2009, 81, 7618–7624 CrossRef CAS PubMed.
- A. U. Jackson, T. Shum, E. Sokol, A. Dill and R. G. Cooks, Anal. Bioanal. Chem., 2011, 399, 367–376 CrossRef CAS PubMed.
- Y. Schober, S. Guenther, B. Spengler and A. Römpp, Anal. Chem., 2012, 84, 6293–6297 CrossRef CAS PubMed.
- A. Roux, L. Muller, S. N. Jackson, J. Post, K. Baldwin, B. Hoffer, C. D. Balaban, D. Barbacci, J. A. Schultz, S. Gouty, B. M. Cox and A. S. Woods, J. Neurosci. Methods, 2016, 272, 19–32 CrossRef CAS PubMed.
- E. Yang, F. Fournelle and P. Chaurand, J. Mass Spectrom., 2020, 55, e4428 CrossRef CAS PubMed.
- M. Dufresne, A. Thomas, J. Breault-Turcot, J.-F. Masson and P. Chaurand, Anal. Chem., 2013, 85, 3318–3324 CrossRef CAS PubMed.
- L. Xu, M. Kliman, J. G. Forsythe, Z. Korade, A. B. Hmelo, N. A. Porter and J. A. McLean, J. Am. Soc. Mass Spectrom., 2015, 26, 924–933 CrossRef CAS PubMed.
- J. Wang, Z. Wang, F. Liu, Y. Mo, L. Cai, C. Sun, S. Zhang, R. Zhang, Z. Abliz and X. Zhang, Int. J. Mass Spectrom., 2018, 432, 9–13 CrossRef CAS.
- S. M. Cologna, Adv. Exp. Med. Biol., 2019, 1115, 155–166 CrossRef CAS PubMed.
- V. Vrkoslav, R. Míková and J. Cvačka, J. Mass Spectrom., 2009, 44, 101–110 CrossRef CAS PubMed.
-
J. Lillja and I. Lanekoff, in Mass Spectrometry Imaging of Small Molecules: Methods and Protocols, ed. Y.-J. Lee, Springer US, New York, NY, 2022, pp. 241–249, DOI:10.1007/978-1-0716-2030-4_17.
- R. L. Hansen, M. E. Dueñas and Y. J. Lee, J. Am. Soc. Mass Spectrom., 2019, 30, 299–308 CrossRef CAS PubMed.
- T. M. Guinan, O. J. R. Gustafsson, G. McPhee, H. Kobus and N. H. Voelcker, Anal. Chem., 2015, 87, 11195–11202 CrossRef CAS PubMed.
- N. Lauzon, M. Dufresne, V. Chauhan and P. Chaurand, J. Am. Soc. Mass Spectrom., 2015, 26, 878–886 CrossRef CAS PubMed.
- A. Płaza, A. Kołodziej, J. Nizioł and T. Ruman, ACS Meas. Sci. Au, 2022, 2, 14–22 CrossRef PubMed.
- A. S. Mohammadi, J. S. Fletcher, P. Malmberg and A. G. Ewing, Surf. Interface Anal., 2014, 46, 379–382 CrossRef CAS.
- M. Guan, Z. Zhang, S. Li, J. a. Liu, L. Liu, H. Yang, Y. Zhang, T. Wang and Z. Zhao, Talanta, 2018, 179, 624–631 CrossRef CAS PubMed.
- L. Muller, K. Baldwin, D. C. Barbacci, S. N. Jackson, A. Roux, C. D. Balaban, B. E. Brinson, M. I. McCully, E. K. Lewis, J. A. Schultz and A. S. Woods, J. Am. Soc. Mass Spectrom., 2017, 28, 1716–1728 CrossRef CAS PubMed.
- G. B. Yagnik, R. L. Hansen, A. R. Korte, M. D. Reichert, J. Vela and Y. J. Lee, Anal. Chem., 2016, 88, 8926–8930 CrossRef CAS PubMed.
- P.-Y. Silvert, R. Herrera-Urbina, N. Duvauchelle, V. Vijayakrishnan and K. T. Elhsissen, J. Mater. Chem., 1996, 6, 573–577 RSC.
- Y. Yin, Z. Y. Li, Z. Zhong, B. Gates, Y. Xia and S. Venkateswaran, J. Mater. Chem., 2002, 12, 522–527 RSC.
- J. Kimling, M. Maier, B. Okenve, V. Kotaidis, H. Ballot and A. Plech, J. Phys. Chem. B, 2006, 110, 15700–15707 CrossRef CAS.
- W. Ding, S. Huang, L. Guan, X. Liu and Z. Luo, RSC Adv., 2015, 5, 64138–64145 RSC.
- Š. Strnad, V. Pražienková, D. Sýkora, J. Cvačka, L. Maletínská, A. Popelová and V. Vrkoslav, Talanta, 2019, 201, 364–372 CrossRef PubMed.
- G. Stokes, Med. Lab. Sci., 1976, 33, 79–80 CAS.
- S. Goetz, M. Bauch, T. Dimopoulos and S. Trassl, Nanoscale Adv., 2020, 2, 869–877 RSC.
-
M. Ohring, Characterization of Thin Films and Surfaces, Academic Press, San Diego, 2002 Search PubMed.
- M. Dufresne, N. H. Patterson, J. L. Norris and R. M. Caprioli, Anal. Chem., 2019, 91, 12928–12934 CrossRef CAS PubMed.
- P. Chaurand, J. Proteomics, 2012, 75, 4883–4892 CrossRef CAS PubMed.
- A. R. Buchberger, K. DeLaney, J. Johnson and L. Li, Anal. Chem., 2018, 90, 240–265 CrossRef CAS PubMed.
- M. Blank and C. Hopf, J. Neurochem., 2021, 159, 330–342 CrossRef CAS PubMed.
- M. Kilgore, C. A. Miller, D. M. Fass, K. M. Hennig, S. J. Haggarty, J. D. Sweatt and G. Rumbaugh, Neuropsychopharmacology, 2010, 35, 870–880 CrossRef CAS PubMed.
- J. L. Jankowsky, D. J. Fadale, J. Anderson, G. M. Xu, V. Gonzales, N. A. Jenkins, N. G. Copeland, M. K. Lee, L. H. Younkin, S. L. Wagner, S. G. Younkin and D. R. Borchelt, Hum. Mol. Genet., 2004, 13, 159–170 CrossRef CAS PubMed.
- Š. Strnad, V. Pražienková, M. Holubová, D. Sýkora, J. Cvačka, L. Maletínská, B. Železná, J. Kuneš and V. Vrkoslav, Analyst, 2020, 145, 4595–4605 RSC.
- I. Kaya, D. Brinet, W. Michno, S. Syvänen, D. Sehlin, H. Zetterberg, K. Blennow and J. Hanrieder, ACS Chem. Neurosci., 2017, 8, 347–355 CrossRef CAS PubMed.
- I. Kaya, D. Brinet, W. Michno, M. Başkurt, H. Zetterberg, K. Blenow and J. Hanrieder, ACS Chem. Neurosci., 2017, 8, 2778–2790 CrossRef CAS PubMed.
- W. Michno, P. M. Wehrli, H. Zetterberg, K. Blennow and J. Hanrieder, Biochim. Biophys. Acta, Proteins Proteomics, 2019, 1867, 458–467 CrossRef CAS.
- E. Gónzalez de San Román, I. Manuel, M. T. Giralt, I. Ferrer and R. Rodríguez-Puertas, Biochim. Biophys. Acta, Biomembr., 2017, 1859, 1604–1614 CrossRef PubMed.
- L. Ferré-González, A. Lloret and C. Cháfer-Pericás, Prog. Lipid Res., 2023, 90, 101223 CrossRef PubMed.
- M. Dufresne, D. Guneysu, N. H. Patterson, M. M. Marcinkiewicz, A. Regina, M. Demeule and P. Chaurand, Anal. Bioanal. Chem., 2017, 409, 1425–1433 CrossRef CAS PubMed.
- T. Mori, D. Paris, T. Town, A. M. Rojiani, D. L. Sparks, A. Delledonne, F. Crawford, L. I. Abdullah, J. A. Humphrey, D. W. Dickson and M. J. Mullan, J. Neuropathol. Exp. Neurol., 2001, 60, 778–785 CrossRef CAS PubMed.
- V. Musetti, F. Greco, V. Castiglione, A. Aimo, C. Palmieri, D. Genovesi, A. Giorgetti, M. Emdin, G. Vergaro, L. A. McDonnell and A. Pucci, Biomedicines, 2022, 10, 3054 CrossRef CAS PubMed.
- E. Wieczorek and A. Ożyhar, Cells, 2021, 10, 1768 CrossRef CAS PubMed.
- G. P. Gorbenko and P. K. J. Kinnunen, Chem. Phys. Lipids, 2006, 141, 72–82 CrossRef CAS PubMed.
- C. M. Yip, A. A. Darabie and J. McLaurin, J. Mol. Biol., 2002, 318, 97–107 CrossRef CAS PubMed.
- G. P. Eckert, C. Kirsch, S. Leutz, W. G. Wood and W. E. Müller, Pharmacopsychiatry, 2003, 36(Suppl 2), S136–S143 CAS.
- X. Hou, A. Mechler, L. L. Martin, M. I. Aguilar and D. H. Small, Biochim. Biophys. Acta, 2008, 1778, 198–205 CrossRef CAS PubMed.
- E. I. Yakupova, L. G. Bobyleva, I. M. Vikhlyantsev and A. G. Bobylev, Biosci. Rep., 2019, 39, BSR20181415 CrossRef PubMed.
-
R. Linke, Congo Red Staining of Amyloid: Improvements and Practical Guide for a More Precise Diagnosis of Amyloid and the Different Amyloidoses, 2006, pp. 239–276, DOI:10.1007/0-387-25919-8_12.
- A. Kakio, S.-i. Nishimoto, K. Yanagisawa, Y. Kozutsumi and K. Matsuzaki, Biochemistry, 2002, 41, 7385–7390 CrossRef CAS PubMed.
- S. Subasinghe, S. Unabia, C. J. Barrow, S. S. Mok, M.-I. Aguilar and D. H. Small, J. Neurochem., 2003, 84, 471–479 CrossRef CAS PubMed.
- K. Simons and R. Ehehalt, J. Clin. Invest., 2002, 110, 597–603 CrossRef CAS PubMed.
-
D. A. Brown, in Encyclopedia of Biological Chemistry, ed. W. J. Lennarz and M. D. Lane, Academic Press, Waltham, 2nd edn, 2013, pp. 741–744, DOI:10.1016/B978-0-12-378630-2.00185-7.
- G. P. Gellermann, T. R. Appel, A. Tannert, A. Radestock, P. Hortschansky, V. Schroeckh, C. Leisner, T. Lütkepohl, S. Shtrasburg, C. Röcken, M. Pras, R. P. Linke, S. Diekmann and M. Fändrich, Proc. Natl. Acad. Sci. U. S. A., 2005, 102, 6297–6302 CrossRef CAS PubMed.
- A. Kakio, S.-i. Nishimoto, Y. Kozutsumi and K. Matsuzaki, Biochem. Biophys. Res. Commun., 2003, 303, 514–518 CrossRef CAS PubMed.
|
This journal is © The Royal Society of Chemistry 2024 |
Click here to see how this site uses Cookies. View our privacy policy here.