DOI:
10.1039/D4AN00854E
(Paper)
Analyst, 2024,
149, 4862-4871
Characterization of vaginal Lactobacillus in biologically relevant fluid using surface-enhanced Raman spectroscopy†
Received
14th June 2024
, Accepted 4th August 2024
First published on 12th August 2024
Abstract
The native vaginal microbiome plays a crucial role in maintaining vaginal health and disruption can have significant consequences for women during their lifetime. While the composition of the vaginal microbiome is important, current methods for monitoring this community are lacking. Clinically used techniques routinely rely on subjective analysis of vaginal fluid characteristics or time-consuming microorganism culturing. Surface-enhanced Raman spectroscopy (SERS) can aid in filling this gap in timely detection of alterations in the vaginal microbiome as it can discriminate between bacterial species in complex solutions including bacterial mixtures and biofluids. SERS has not previously been applied to study variations in vaginal Lactobacillus, the most common species found in the vaginal microbiome, in complex solutions. Herein, the SERS spectra of Lactobacillus crispatus (L. crispatus) and Lactobacillus iners (L. iners), two of the most common vaginal bacteria, was characterized at physiologically relevant concentrations. Subsequently, the ability of SERS to detect L. crispatus and L. iners in both pure mixtures and when mixed with a synthetic vaginal fluid mimicking solution was determined. In both pure and complex solutions, SERS coupled with partial least squares regression predicted the ratiometric bacterial content with less than 10% error and strong goodness of prediction (Q2 > 0.9). This developed method highlights the applicability of SERS to predict the dominant Lactobacillus in the vaginal micro-environment toward the monitoring of this community.
Introduction
The vaginal microbiome is a robust community of microorganisms that are vital in maintaining the health of the reproductive system. This community is dominated by Lactobacillus species with other species present in low abundance.1–5Lactobacillus crispatus (L. crispatus) and Lactobacillus iners (L. iners) have been reported to be the most dominant species in numerous studies.1,6–10 While the biocomposition of the vaginal microbiome may change in relation to age, menarche, time in the menstrual cycle, use of and type of contraception, and frequency of sexual intercourse, the dominance of Lactobacillus has been consistently shown.1,2,11Lactobacillus functions in the vagina to inhibit pathogenic growth by reducing the pH of the vagina via the production of lactic acid, and by producing hydrogen peroxide (H2O2), bacteriocins, and other antimicrobial compounds.12 The reduction in Lactobacillus concentration in the vagina, known as vaginal dysbiosis, results in an increased risk of a multitude of negative health conditions. Vaginal dysbiosis can lead to bacterial vaginosis, vulvovaginal candidiasis (colloquially known as “yeast infection”), trichomoniasis, aerobic vaginitis, increased risk of acquiring sexually transmitted infections (STIs) and urinary tract infections (UTIs) and can reduce the conception rate during in vitro fertilization (IVF).1,13–15 Additionally, during pregnancy, vaginal dysbiosis can lead to preterm birth, premature rupture of membranes, and neonatal sepsis.16,17
Currently, three clinical techniques are used for assessing vaginal health and the vaginal microbiome: the Nugent criteria, the Amsel criteria, and culturing of vaginal fluid. The Nugent criteria is based on visual identification of bacteria morphotypes relating to the healthy microbiome and pathogenic species using microscopy and is generally considered the gold standard.18 The second commonly used method is Amsel scoring, which relies on identification of visual and olfactory characteristics of vaginal fluid.19,20 Finally, culturing of vaginal fluid for identification of microbes is used to analyze the presence of vaginal dysbiosis. Culturing includes streaking vaginal swabs on solid agar plates and incubating to promote microbial growth.21–24 All three methods exhibit numerous limitations. Both Nugent and Amsel scoring have shown significant subjectivity and variability, including interobserver, intraobserver, and intercenter variability.25–28 Microbial culture may fail to show any microbial growth or require a lengthy wait time (typically 5 days), and results may be misconstrued from culture growth of normal vaginal microbes.21,24 Despite the importance of Lactobacillus in the vaginal microbiome, current methods are lacking for rapid detection and monitoring of vaginal Lactobacillus. Vibrational spectroscopy, such as Raman spectroscopy and surface-enhanced Raman spectroscopy (SERS), shows great promise for filling the gap in the timely detection of vaginal Lactobacillus as these are label-free and nondestructive methods that can provide results in real-time.29 Additionally, the biochemical sensitivity of these techniques can allow for the detection of vaginal bacteria from vaginal fluid with minimal sample preparation.
Raman spectroscopy is an inelastic scattering technique that provides a spectral fingerprint representative of the chemical bonds present in a sample.30 Raman spectroscopy can be used in vivo or ex vivo, and biomedical Raman spectroscopy has shown the ability to detect diseased tissues and biofluids along with other medically relevant information.31–33 Raman scattering is an inherently weak phenomenon, and this weakness leads to the need for highly sensitive equipment or long exposure times, reducing the clinical usability of conventional Raman spectroscopy.34–37 The chemical sensitivity of Raman spectroscopy is retained in SERS while the signal strength is enhanced up to 1014 times by positioning the analytes near roughened metallic surfaces (e.g., gold or silver films) or metallic colloids (e.g., gold or silver nanoparticles).38 The sensitivity and biochemical specificity of SERS has resulted in vast research for the use of this technique in biomedical detection and diagnostics.
There is widespread interest in the use of SERS in the field of microbiology and infectious diseases. Specifically, SERS has been widely used to study the unique biochemical differences between bacterial species and strains.39 Liu et al. showed discrimination and identification of specific species based on their unique spectra.40 Strain level discrimination has also been achieved using nine strains of the foodborne pathogen Listeria monocytogenes.41 Previous reports also highlight the source of the SERS signal. When the plasmonic substrate or nanoparticles are extracellularly interacting with bacterial cells, cell wall components such as peptidoglycan, membrane proteins, small molecules such as adenine, and metabolites of purine degradation, are spectrally enhanced.39,41 This technique has also been reported to be advantageous over conventional Raman spectroscopy for the identification of bacteria due to higher diversity in the cell wall of the bacteria compared to the whole cells.42 Vaginal microbes and pathogens have previously been spectrally characterized using SERS.43 Numerous vaginal bacteria, including L. crispatus and L. iners, were characterized individually and partial least squares regression was utilized to investigate the potential of SERS to discriminate between vaginal bacterial species. While this work demonstrated discrimination of the vaginal bacteria, only a single bacterial concentration was characterized and provided no discrimination of the vaginal bacteria when mixed in co-cultures or from a complex biofluid matrix, such as the native vaginal fluid. These two factors will greatly influence the ability of specific species detection in clinically relevant settings. Therefore, investigation into the ability of SERS to measure Lactobacillus when present in complex environments, such as mixed bacterial solutions or vaginal fluid, is warranted toward the development of a SERS-based method for monitoring the health of the vaginal microbiome.
This work aims to utilize SERS to characterize the spectra of two key vaginal Lactobacillus species, L. crispatus and L. iners, at physiologically relevant concentrations and detect these species in both mixed cultures and complex media. Herein, we investigate spectral changes in the L. crispatus and L. iners spectra from 106 to 109 CFU mL−1. Further, we evaluated the ability of SERS to detect each species when mixed in pure solution and in complex media that mimics the vaginal fluid environment. Partial least squares regression was then utilized to predict the ratiometric content of L. crispatus and L. iners in mixtures based on the resulting SERS spectra. To the best of our knowledge, this work demonstrates the first discrimination of vaginal Lactobacillus in complex solutions using SERS.
Methods
Materials
Trisodium citrate, gold(III) chloride, agar powder, potassium hydroxide, bovine serum albumin, urea, glycerol, and nitric acid were purchased from Sigma-Aldrich (St. Louis, MO). De Man–Rogosa–Sharpe (MRS) broth powder, MRS agar powder, hydrochloric acid, (4-(2-hydroxyethyl)-1-piperazineethanesulfonic acid) (HEPES), proteose peptone, heat-inactivated horse serum, yeast extract, dextrose, calcium hydroxide, lactic acid, acetic acid, and sodium chloride were all purchased from Fisher Scientific (Hampton, NH). L. crispatus (strain VPI 7635, ATCC #33197) and L. iners (strain AB107, ATCC #55195) were purchased from American Type Culture Collection (ATCC) (Manassas, VA). Standard aluminum foil (Reynolds Wrap, Reynolds, Lake Forest, IL) was purchased from a local supermarket. All chemicals were of analytical grade and used with no further purification.
Gold nanoparticle synthesis
Citrate-stabilized gold nanoparticles (AuNPs) were synthesized for this study following previously published methods by Bastus et al.44 Briefly, 150 mL of trisodium citrate (1.8 mM) was heated to boiling in a round bottom flask outfitted with a condenser after cleaning with aqua regia (3
:
1 HCl
:
HNO3). After boiling, 1 mL of gold(III) chloride (25 mM) was injected into the flask while vigorously stirring. This was allowed to react for 15 minutes and a color change from clear to pale red was observed, indicating AuNPs seed formation. The temperature was reduced to 90 °C and the solution was allowed to react for one hour. Following the 1-hour reaction time, three growth steps were introduced to the AuNPs seeds by adding 1 mL of trisodium citrate (48 mM) and 1 mL of gold(III) chloride to the flask with a 30-minute incubation time between each growth step. The diameter of the AuNPs was determined by dynamic light scattering (DLS) (Malvern Panalytical Advance Series Ultra; United Kingdom). The AuNPs were further characterized by measuring the zeta potential (Malvern Panalytical Advance Series Ultra; United Kingdom) to determine the surface charge. The localized surface plasmon resonance (LSPR) peak, and nanoparticle concentration was determined using ultraviolet-visible-near-infrared (UV-Vis-NIR) spectrophotometry (Cary 7000, Agilent Technologies; Santa Clara, California).45 After three growth steps, the AuNPs had a final diameter of 45 nm, LSPR peak of 530 nm, and surface charge of −42.5 mV.
Bacterial culturing
L. crispatus was cultured from a frozen stock on MRS agar plates at 37 °C under 5% CO2 for 24 hours. Following the colony growth, a liquid culture was grown by transferring a single colony to 5 mL of MRS broth and was allowed to incubate for 24 hours (37 °C and 5% CO2). L. iners was cultured using NYC III media following the same procedure. The cultures were then washed with sterile deionized (DI) water twice using centrifugation at 3300 relative centrifugal force (RCF) for 8 min, and 1 mL of washed culture was collected for optical density (OD) readings at 600 nm to quantify the bacterial concentration using UV-vis-NIR spectrophotometry.
SERS characterization of L. crispatus and L. iners
To collect SERS spectra of L. crispatus and L. iners individually, liquid cultures of each species were grown and washed following the previously mentioned procedures. Using the OD, the washed cultures were adjusted to the desired physiological concentrations (106–109 CFU mL−1).46 The washed liquid cultures were mixed with AuNPs (1.4 nM) at a 1
:
4 volumetric ratio (bacteria
:
AuNPs), vortexed for 2–3 seconds to ensure adequate mixing of the two solutions and allowed to interact for 15 minutes. A 1 μL droplet of this solution was applied to an aluminum foil covered glass slide and allowed to dry. The final bacteria concentrations measured were 2 × 102–2 × 105 CFUs per droplet after taking into account bacteria dilution when mixing with AuNPs. After drying, SERS spectra were collected using a Renishaw inVia Raman microscope (Renishaw; United Kingdom) equipped with 785 nm laser excitation. Point spectra were taken under 5× magnification (NA = 0.12) with ∼40 mW of laser power measured at the sample and a total integration time of 10 seconds. Three droplets were measured from each bacterial solution and five spectra were taken from each droplet. All bacterial concentrations were measured in triplicate experiments (n = 45 spectra for each bacterial concentration).
SERS of Lactobacillus mixtures
L. crispatus and L. iners were cultured following procedures outlined previously. The OD of each bacteria culture was used to adjust the concentration of each washed bacteria solution to 1 × 108 CFU mL−1 then were mixed at volumetric ratios (v
:
v) to achieve the following mixtures as shown in Table 1. Each bacteria solution was vortexed for 2–3 seconds to ensure adequate sample mixing. After mixing the two bacteria, AuNPs (1.4 nM) were added to the mixtures at a 1
:
4 volumetric ratio (bacteria
:
AuNPs) by pipetting and solutions were again vortexed for 2–3 seconds; solutions were allowed to sit for 15 minutes to allow time for AuNPs and bacteria interaction. Then, three 1 μL droplets of the final solution were dropped on a glass slide coated with aluminum foil and allowed to fully dry. The total concentration of bacteria in each droplet was 2 × 104 CFUs per droplet. After drying, SERS spectra were collected using the same parameters described previously. All bacterial solutions were made in triplicate and spectra were recorded from each experiment (n = 45 spectra for each ratio).
Table 1 Ratios of L. crispatus and L. iners used for mixture analysis
L. crispatus
|
L. iners
|
100% |
0% |
95% |
5% |
90% |
10% |
75% |
25% |
50% |
50% |
25% |
75% |
10% |
90% |
5% |
95% |
0% |
100% |
SERS of Lactobacillus in complex media
To determine the ability of SERS to discriminate Lactobacillus from a complex solution that mimics biologically relevant fluid, a synthetic vaginal fluid solution (SVF) that contains the major components found in human vaginal fluid was chosen. The SVF solution was adapted from a previously reported SVF recipe.47 The components of the SVF and concentrations can be found in Table 2. The SERS spectra of SVF was collected by mixing SVF with AuNPs (1.4 nM) at a 1
:
4 volumetric ratio (SVF
:
AuNPs). The same sample volume and SERS spectral collection protocol as above was utilized. Toward determining the ability of SERS to detect L. crispatus and L. iners in a complex fluid, the Lactobacillus was mixed with SVF as follows. L. crispatus and L. iners were grown from frozen stocks and washed with DI water according to protocols detailed above. The OD of each washed cultured was measured and adjusted to 1 × 108 CFU mL−1. Finally, the adjusted bacteria solutions were centrifuged at 3300 RCF for 8 min and the supernatant was replaced with an equal volume of SVF. After suspending each species in SVF, the bacteria in SVF solutions were mixed at the following volumetric ratios using the same mixing protocols previously described: 100
:
0, 75
:
25 50
:
50, 25
:
75, and 0
:
100 (% L. crispatus
:
% L. iners). SERS spectra were collected in the same manner as described previously. Experiments were carried out in triplicate with SERS spectra being collected from each experiment (n = 45 spectra for each ratio).
Table 2 Chemical components and concentrations utilized for making SVF solution
Chemical |
Concentration (g L−1) |
Potassium hydroxide |
1.4 |
Calcium hydroxide |
0.222 |
Bovine serum albumin |
10.0 |
Lactic acid |
2.0 |
Acetic acid |
1.0 |
Urea |
0.4 |
Glucose |
5.0 |
Glycerol |
0.16 |
Spectral processing & data analysis
All spectra were processed using a Savitsky-Golay filter (2nd order, window size of 7) for noise smoothing and asymmetric least squares regression (p = 0.001, λ = 7500) for removal of the fluorescent background.48,49 Spectra were then normalized via standard normal variate normalization.50 The limit of detection (LOD) of each species using this experimental design was calculated using the 734/1435 cm−1 peak ratio (L. crispatus) and 1025/1541 cm−1 peak ratio (L. iners) and performing a linear fit. Equations reported by Armbruster and Pry were utilized to calculate the LOD for each species.51 The ability to determine the ratio of L. crispatus to L. iners in pure solutions and in complex media was evaluated using partial least squares regression (PLSR).52 All spectra were truncated to 700–1650 cm−1 prior to being input into the regression model. Next, two dedicated PLSR models were built, one for pure solution and one for complex media due to the numerous spectral features noted from the SVF solution. For each model, the number of loading vectors used was determined via stabilization of the root mean square error of calibration; 9 loading vectors for the pure solution dataset and 19 loading vectors for the complex media were utilized. Each regression model was trained using leave-one-ratio-out cross validation, which leaves all spectra from a certain ratio out while training the model then applies the model to the left-out ratio spectra. Model performance was evaluated using goodness of prediction (Q2) between the predicted and known bacteria ratio of the cross validation set (designated between values of 0 to 1) and the root mean square error of prediction (RMSEP) for each model. Spectral features that contributed significantly to the PLSR model were determined via variable importance in projection (VIP) which is derived from the PLS weights and the amount of variance explained by each predictor.52,53 Spectral processing, normalization, and PLSR were performed using MATLAB (MathWorks; Natick, MA). Curve fitting to determine the LOD was performed in Excel (Microsoft; Redmond, WA) and Origin (OriginLab; Northampton, MA) was utilized for all spectral plotting and figure generation.
Results & discussion
L. crispatus & L. iners spectral characterization
Lactobacillus in the vaginal microbiome is reported to be present at a physiological concentration range of 107–108 CFU mL−1. Herein, we characterized the SERS spectra of the two dominant vaginal Lactobacillus, L. crispatus and L. iners, within the concentration range of 106–109 CFU mL−1 to include one concentration above and below the physiological concentration.46Fig. 1 shows the SERS spectra of both species were dominated by commonly reported bacterial peaks including the 734, 1025, and 1465 cm−1 peaks at the highest bacterial concentration. The peak at 734 cm−1 is reported in literature as C–N stretching, glycosidic ring vibrations, or in plane breathing mode of adenine. This peak may be indicative of adenine containing compounds (FAD, NAD, NADH, DNA) or peptidoglycan in the bacterial cell wall.39,43,54,55 The peak at 1025 cm−1, arising from C–N or C–C stretching, or C–H in plane bending, may stem from biochemical components such as phospholipids, carbohydrates, and amino acids.43 Finally, the 1465 cm−1 peak showing CH2 and C–H vibrations may indicate protein, lipid, and phospholipid content.43 Additionally, spectral differences are also evident between the two bacteria. Of note, the 734 cm−1 peak is not the most dominant feature in the L. iners spectrum, which is a common feature in SERS spectra of bacteria. This is hypothesized to result from the thinness of the L. iners cell wall previously studied using transmission electron microscopy (TEM).56
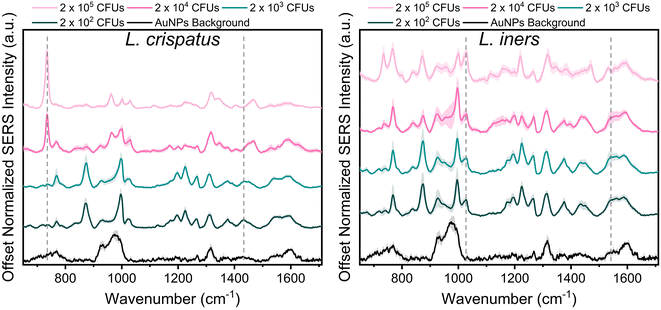 |
| Fig. 1 SERS spectra of L. crispatus (left) and L. iners (right) from 2 × 105 CFUs per droplet to 2 × 102 CFUs per droplet. Each spectra is the mean and standard deviation (SD) (represented by shaded error bars) of 3 experimental replicates [n = 45 spectra]. Dashed grey lines show peaks chosen for LOD calculation via peak ratios (L. crispatus: 734/1435 cm−1, L. iners: 1025/1541 cm−1). Spectra are vertically offset for clarity. | |
When reducing the bacterial concentration, we identified a shift in the relative intensity of certain peaks in the SERS spectra of both species (Fig. 1). The spectra of L. crispatus shows a reduction in the height of the 734 cm−1 and 1470 cm−1 features and an increase in the intensity of numerous peaks such as 768, 875, 1195, 1225, 1375, and 1435 cm−1 as the bacterial concentration decreases. A similar trend is identified in the spectra of L. iners at decreasing bacterial concentrations. Additionally, we identified an increase in peaks from the nanoparticle background between 920–995, 1317, and 1590 cm−1 at lower bacterial concentrations. The LOD of L. crispatus was calculated as 3.4 × 103 CFUs per droplet (1.7 × 107 CFU mL−1) using the ratio of 734 cm−1/1435 cm−1 (ESI Fig. 1A†). The L. iners LOD was calculated to be 7.9 × 103 CFUs per droplet (3.94 × 107 CFU mL−1) using the ratio of the 1025 cm−1/1541 cm−1 peak (ESI Fig. 1B†). The shift in peak intensities as the bacterial concentration decreased are hypothesized to be due to differences in AuNPs binding and density on the bacterial cell wall at the different bacterial concentrations. Transmission electron microscopy was performed with L. crispatus at low (2 × 103 CFUs per droplet) and high (2 × 105 CFUs per droplet) bacterial loads to confirm this hypothesis. These images show much higher AuNPs density on the L. crispatus cell wall at low bacterial concentrations as compared to the higher bacterial concentration (ESI Fig. 2A and B†). This is due to the AuNPs concentration being held constant as bacterial concentration was altered, resulting in less bacteria for the AuNPs to bind to. Therefore, as the bacterial concentration decreased, the bacterial cell wall became saturated with AuNPs and no additional AuNPs could attach to the cell wall, limiting the lower value of bacteria that could be measured. Furthermore, this hypothesis explains why an increase in AuNPs background is seen at lower concentrations as there may be an accumulation of unbound AuNPs after the bacteria cell wall is saturated. While this could limit the lower bacterial concentrations that can be measured, the AuNPs concentration or ratio can be further optimized to shift the measurable bacterial concentration range for specific applications. However, the bacterial LOD measured here is near the lower limit of the physiological concentration of Lactobacillus in the healthy microbiome, so it is satisfactory for this application. Finally, the SERS spectra of L. iners was found to have less signal to noise than the spectra collected from L. crispatus (Fig. 1). Transmission electron microscopy imaging was used to investigate the source of this and revealed differences in the size distribution of the two bacteria. L. crispatus displayed long rod morphology while L. iners showed short rod morphology (ESI Fig. 2C†). These differences in bacteria size and shape could result in differing AuNPs coverage of the two bacteria, influencing the signal strength and noise.
SERS of Lactobacillus mixtures
Following spectral characterization of L. crispatus and L. iners at four physiologically relevant concentrations, mixtures of the two Lactobacillus were measured in order to investigate the ability of SERS to determine the dominant microbe in complex environments. The two bacteria were mixed in pure solution (DI water) and the resulting spectral changes were determined. Fig. 2 shows that the 734 cm−1 peak is the most prominent feature in the SERS spectra when L. crispatus was the dominant species in the mixture. As the amount of L. crispatus decreased and L. iners increased, peaks including the 768 cm−1 and 1540 cm−1, assigned to ring breathing and amide carbonyl group vibrations, respectively, increase which may indicate variation in amino acid and protein content.30,57,58 Additionally, a peak shift in the region between 1420–1490 cm−1 is observed, with spectra from mixtures dominated by L. crispatus having a peak centered at 1465 cm−1 (CH2 and C–H vibrations) while mixtures with more L. iners content exhibited a more blue-shifted peak centered at 1435 cm−1 (CH2 scissoring and deformation). This spectral change may highlight differences in the protein and lipid cell wall content of the two bacterial species.30,43
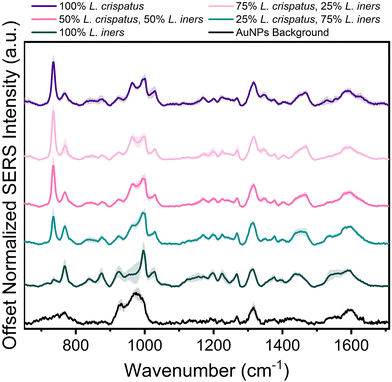 |
| Fig. 2 SERS spectra of L. crispatus and L. iners mixtures. Each spectra is the mean and SD (represented by shaded error bars) of 3 experimental replicates [n = 45 spectra]. Spectra are vertically offset for clarity. | |
Additional analysis was performed via PLSR with the goal of predicting the ratio of L. crispatus to L. iners based on the resulting SERS spectra. This is a chemometric technique that combines feature decomposition and linear regression and is commonly used to analyze Raman and SERS spectra. It is advantageous for this application because it has been shown to perform well using colinear datasets and datasets that have high dimensionality, two common features of spectroscopic data.52,59,60 A PLSR model was generated in this study with 45 spectra for each ratio of L. crispatus
:
L. iners using leave-one-ratio-out cross validation. High goodness of prediction is found between the PLSR model and the known ratio values (Q2 = 0.9535) with a RMSEP of 8.19% when predicting the ratiometric amount of L. crispatus in each mixture, denoted as a value between 0–1 representing 0% to 100% (Fig. 3A). Similar performance is found when predicting L. iners ratiometric content with a Q2 value of 0.9506 and RMSEP of 8.45%. The spectral features that were most utilized for determining the ratio of L. crispatus and L. iners were identified using the VIP of the PLSR model (Fig. 3B). Features with a VIP score greater than 1 contribute significantly to the model prediction.61,62 The feature that is most heavily weighted is 734 cm−1, which shows a significant decrease in intensity as L. crispatus content decreases in the ratiometric mixtures (Fig. 2). Other peaks identified by the VIP plot that contributed substantially to the model predictions include 962, 995, 1320, 1340, 1435, 1535, and 1560 cm−1 which are representative of proteins, lipids, phospholipids, amino acids, and carbohydrate content (ESI Table 1†). Further, to test the model on unseen data, a model was generated while leaving 10% of the dataset out of the training and cross-validation datasets. The withheld spectra were then used to test the model to investigate overfitting of the model. For the withheld dataset, the RMSEP for L. crispatus was 7.31% and 8.02% for L. iners. These results demonstrate that SERS coupled with PLSR can predict the ratiometric values of L. crispatus and L. iners when mixed in pure solution with low error. This is promising because the ability to determine the dominant Lactobacillus species in the vaginal microbiome is clinically relevant as it has been reported that there are differences in the protection capabilities of L. crispatus and L. iners. L. crispatus has been correlated with a stable microbiome, whereas L. iners has been reported to be the most prevalent Lactobacillus associated with vaginal dysbiosis.63,64 Identifying the dominant species or transition from L. crispatus dominance to L. iners dominance using a spectroscopic approach such as that shown here may aid in vaginal microbiome monitoring or the early detection of the transition to vaginal dysbiosis.
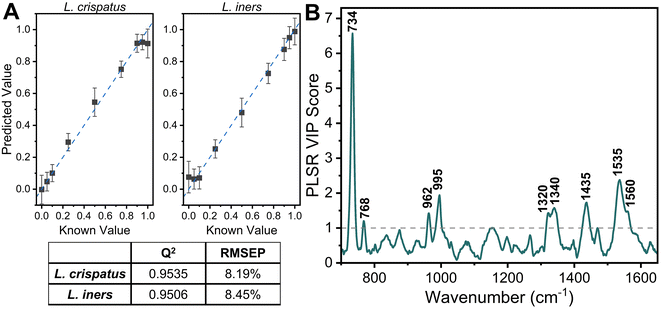 |
| Fig. 3 PLSR of L. crispatus and L. iners mixtures. (A) Scatter plot of PLSR predictions vs. known values (mean and SD) showing a high goodness of fit with known values [dashed line represents perfect prediction] and Q2 and RMSEP of PLSR model. (B) Variable of importance plot highlight features with a score greater than 1, indicating importance to the PLSR model. | |
SERS of Lactobacillus mixtures in complex media
The applicability of SERS to determine the bacterial content in complex media, such as vaginal fluid, without the need for sample purification or separation, is clinically relevant. Toward this goal, synthetic vaginal fluid mimicking solution (Table 2) was utilized to investigate the ability of SERS to monitor Lactobacillus. The SERS profile of the SVF was first characterized prior to mixing the Lactobacillus and SVF (ESI Fig. 3†). In both the spectra of SVF and SVF + Lactobacillus, the most dominant spectral feature is at 870 cm−1, reported to be from indole ring scissoring, indole N–H displacement, indole ring vibration with N–H bending, C–H stretching. This peak may be representative of amino acids and glucose in the SVF65–67 (Fig. 4 and ESI Fig. 2†). Although Fig. 4 shows substantial overlap between bacterial and SVF spectral features, there are still identifiable peaks relating to the presence of the bacteria, such as the 734 and 1435 cm−1 peaks. The 734 cm−1 peak, a peak that is unique to bacteria and not found in the spectra of SVF, shows an observable change in intensity as the bacterial content in the mixtures was varied. This peak can be identified in the spectra of 100% L. crispatus in SVF and steadily decreases as the L. crispatus content in the mixtures decreases. This correlates with our previous results where this feature was dominant in the L. crispatus spectra but not as strong in the L. iners spectra (Fig. 1). The 1435 cm−1 peak (CH2 scissoring and deformation43) is more prominent at higher L. iners ratios, as seen when the bacteria are mixed in pure solution when the peak shifts from 1470 cm−1 to 1435 cm−1 (Fig. 2). Therefore, identifiable features from the different mixture components (e.g. L. crispatus, L. iners, and SVF) are evident, despite the significant overlap between the biochemical profiles of Lactobacillus and SVF. Further analysis of the Lactobacillus mixtures in SVF spectra was performed using PLSR to improve the identification of the two species in complex environments.
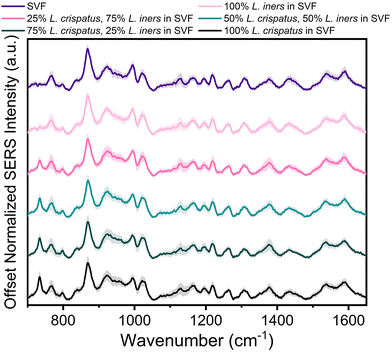 |
| Fig. 4 SERS spectra of L. crispatus and L. iners spiked in SVF. Each spectra is the mean and SD (represented by shaded error bars) of 3 experimental replicates [n = 45 spectra]. Spectra are vertically offset for clarity. | |
The PLSR modeling results in Q2 values greater than 0.90 (L. crispatus = 0.9492, L. iners = 0.9301) when predicting the ratiometric value of L. crispatus and L. iners despite the addition of the complex media (Fig. 5A). Additionally, the RMSEP values remain low with the L. crispatus RMSEP equal to 8.46% and the L. iners RMSEP equal to 9.91%. We believe that the L. iners RMSEP was higher than the L. crispatus RMSEP due to more distinct spectral features being present when L. crispatus is dominant in the mixtures (Fig. 4). Also, previous discussion of L. crispatus spectra having higher enhancement due to its shape and size could potentially lead to lower error when predicting L. crispatus. To identify features that significantly contributed to the PLSR model predictions, the variable importance plot was again utilized (Fig. 5B). Similar to before, the 734 cm−1 peak is the dominant feature for model prediction. Seven other features have VIP values greater than 1 and may describe variations in protein, lipid, and amino acid contributions (ESI Table 1†). Two of these features, 865, and 1435 cm−1, were visually identifiable and discussed previously, and the model was able to further extract additional peaks that differ across the six mixtures.
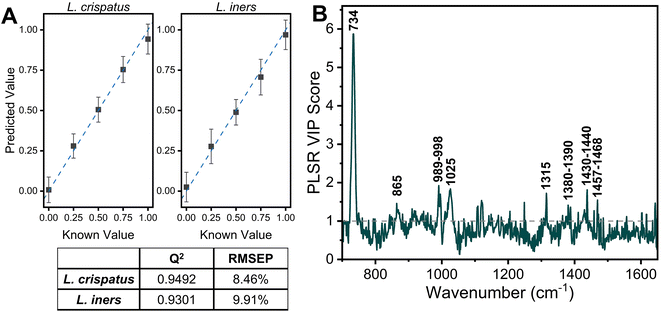 |
| Fig. 5 PLSR of L. crispatus and L. iners in SVF. (A) Scatter plot of PLSR predictions and known values (mean and SD) showing a high goodness of fit with known values and Q2 and RMSEP of PLSR model. (B) Variable of importance plot highlight features with a score greater than 1, indicating importance to the PLSR model. | |
The impact of the complex media in predicting the ratio of L. crispatus and L. iners was determined by comparing the Q2 and RMSEP values for the two PLSR models developed (Fig. 3 and 5). There is less than a 3% decrease in the Q2 values when moving to the complex media (0.38% [L. crispatus] and 2.16% [L. iners]), and all values remain above 0.90, indicating strong goodness of prediction for the PLSR model. Additionally, the RMSEP of the predicted L. crispatus and L. iners ratio values show minimal change when in pure or complex media (0.33% [L. crispatus], 1.73% [L. iners] increase). Moreover, despite a higher spectral overlap of L. iners with the SVF resulting in a larger increase in the RMSEP for L. iners, there is still a strong goodness of prediction and a low RMSEP. The VIP plots from each PLSR model also highlight many of the same spectral features that contributed significantly to the models in both pure solution and complex fluid. Overall, four spectral features, relating to cell wall components, adenine, amino acids, and protein content, had significant contributions for both models (ESI Table 1†).
Herein, SERS coupled with PLSR has been shown to perform well (Q2 > 0.9, RMSEP < 10%) for identifying the underlying spectral changes related to the differences in the two bacterial species and their relative content in solution, despite the significant spectral overlap. This highlights the strength of SERS in determining the ratiometric content of L. crispatus and L. iners in complex environments. Utilizing SERS can be beneficial for the detection or monitoring of vaginal bacteria without the need for separation from the complex biofluid, thereby simplifying sample preparation procedures and highlights the potential for future use of SERS in clinical settings to detect and monitor the vaginal microbiome. Future work will aim to ensure the addition of other bacterial species does not significantly affect the ability of SERS to determine the dominant Lactobacillus in both mixed samples and in human vaginal fluid.
Conclusion
In this work, the concentration dependent SERS spectra of L. crispatus and L. iners have been characterized across physiologically relevant concentrations in which these bacteria would be found in the vaginal microbiome. Further, SERS coupled with PLSR performs with high goodness of prediction and low error to predict the ratiometric value of each bacteria when mixed in solution and in complex media mimicking human vaginal fluid. To the best of our knowledge, this is the first published report to characterize the SERS spectra of two key vaginal Lactobacillus species at physiological concentration. Further, the ability of SERS to detect these two Lactobacillus in both pure solutions and complex media has not previously been reported in the literature. Overall, this study highlights the potential of SERS to detect the dominant Lactobacillus in the vaginal microbiome without the need for excessive sample preparation, thereby increasing the potential for timely monitoring of vaginal dysbiosis.
Data availability
Data supporting this article have been included as part of the ESI.†
Conflicts of interest
The authors declare no conflicts of interest.
Acknowledgements
This research was supported by startup funds allocated to A.L by the Department of Biomedical Engineering and the Department of Chemistry at Vanderbilt University. The authors would like to thank the Vanderbilt Institute for Nanoscale Science and Engineering (VINSE) for access to analytical tools used in this work.
References
- X. Zhou, S. J. Bent, M. G. Schneider, C. C. Davis, M. R. Islam and L. J. Forney, Microbiology, 2004, 150, 2565–2573 CrossRef CAS PubMed.
- S. R. Johnson, C. R. Petzold and R. P. Galask, Am. J. Reprod. Immunol. Microbiol., 1985, 9, 1–5 CrossRef CAS PubMed.
- R. Bhujel, S. K. Mishra, S. K. Yadav, K. D. Bista and K. Parajuli, BMC Infect. Dis., 2021, 21, 1–6 CrossRef PubMed.
- M. Amir, J. A. Brown, S. L. Rager, K. Z. Sanidad, A. Ananthanarayanan and M. Y. Zeng, Microorganisms, 2020, 8, 1–21 Search PubMed.
- V. Florova, R. Romero, A. L. Tarca, J. Galaz, K. Motomura, M. M. Ahmad, C. D. Hsu, R. Hsu, A. Tong, J. Ravel, K. R. Theis and N. Gomez-Lopez, Cytokine, 2021, 137, 155316 CrossRef CAS PubMed.
- L. Donati, A. Di Vico, M. Nucci, L. Quagliozzi, T. Spagnuolo, A. Labianca, M. Bracaglia, F. Ianniello, A. Caruso and G. Paradisi, Arch. Gynecol. Obstet., 2010, 281, 589–600 CrossRef PubMed.
- M. I. Petrova, M. van den Broek, J. Balzarini, J. Vanderleyden and S. Lebeer, FEMS Microbiol. Rev., 2013, 37, 762–792 CrossRef CAS PubMed.
- A. Vásquez, T. Jakobsson, S. Ahrné, U. Forsum and G. Molin, J. Clin. Microbiol., 2002, 40, 2746–2749 CrossRef PubMed.
- R. Verhelst, H. Verstraelen, G. Claeys, G. Verschraegen, J. Delanghe, L. Van Simaey, C. De Ganck, M. Temmerman and M. Vaneechoutte, BMC Microbiol., 2004, 4, 1–11 CrossRef PubMed.
- M. Tärnberg, T. Jakobsson, J. Jonasson and U. Forsum, APMIS, 2002, 110, 802–810 CrossRef PubMed.
- M. J. Redelinghuys, J. Geldenhuys, H. Jung and M. M. Kock, Front. Cell. Infect. Microbiol., 2020, 10, 354 CrossRef PubMed.
- A. A. Aroutcheva, J. A. Simoes and S. Faro, Infect. Dis. Obstet. Gynecol., 2001, 9, 33–39 CrossRef CAS PubMed.
- F. Losa, S. Palacios, S. P. G. Rodríguez, L. Baquedano, D. Khorsandi and M. J. Muñoz, Obstet. Gynaecol. Cases Rev., 2022, 9, 222 Search PubMed.
- A. Lev-Sagie, F. De Seta, H. Verstraelen, G. Ventolini, R. Lonnee-Hoffmann and P. Vieira-Baptista, J. Low. Genit. Tract Dis., 2022, 26, 79 Search PubMed.
- L. O. Eckert, D. E. Moore, D. L. Patton, K. J. Agnew and D. A. Eschenbach, Infect. Dis. Obstet. Gynecol., 2003, 11, 11–17 CrossRef PubMed.
- N. Zheng, R. Guo, Y. Yao, M. Jin, Y. Cheng and Z. Ling, BioMed Res. Int., 2019, 2019, 6079734 Search PubMed.
- H. L. Brown, D. D. Fuller, L. T. Jasper, T. E. Davis and J. D. Wright, Infect. Dis. Obstet. Gynecol., 2004, 12, 17 CrossRef CAS PubMed.
- R. P. Nugent, M. A. Krohn and S. L. Hillier, J. Clin. Microbiol., 1991, 29, 297–301 CrossRef CAS PubMed.
- R. L. Cook, G. Reid, D. G. Pond, C. A. Schmitt and J. D. Sobel, J. Infect. Dis., 1989, 160, 490–496 CrossRef CAS PubMed.
- R. Amsel, P. A. Totten, C. A. Spiegel, K. C. S. Chen, D. Eschenbach and K. K. Holmes, Am. J. Med., 1983, 74, 14–22 CrossRef CAS PubMed.
- D. Nenadić and M. D. Pavlović, Vojnosanit. Pregl., 2015, 72, 523–528 CrossRef PubMed.
-
K. G. Kelly, Clinical Methods: The History, Physical, and Laboratory Examinations, Butterworths, 3rd edn, 1990 Search PubMed.
- Y. Li, S. Wang, H. Li, X. Song, H. Zhang, Y. Duan, C. Luo, B. Wang, S. Ji, Q. Xie and Z. Zhang, BMC Infect. Dis., 2020, 20, 319 CrossRef CAS PubMed.
- C. Han, W. Wu, A. Fan, Y. Wang, H. Zhang, Z. Chu, C. Wang and F. Xue, Arch. Gynecol. Obstet., 2015, 291, 251–257 Search PubMed.
- B. E. Sha, H. Y. Chen, Q. J. Wang, M. R. Zariffard, M. H. Cohen and G. T. Spear, J. Clin. Microbiol., 2005, 43, 4607–4612 CrossRef PubMed.
- P. Vieira-Baptista, A. R. Silva, M. Costa, R. Figueiredo, C. Saldanha and C. Sousa, Int. J. Gynecol. Obstet., 2022, 156, 552–559 CrossRef PubMed.
- R. Bhujel, S. K. Mishra, S. K. Yadav, K. D. Bista and K. Parajuli, BMC Infect. Dis., 2021, 21, 1–6 CrossRef PubMed.
- J. C. Lagier, S. Edouard, I. Pagnier, O. Mediannikov, M. Drancourt and D. Raoult, Clin. Microbiol. Rev., 2015, 28, 208 CrossRef CAS PubMed.
- L. F. D. C. E. S. de Carvalho and M. S. Nogueira, Photodiagn. Photodyn. Ther., 2020, 30, 101765 CrossRef PubMed.
- Z. Movasaghi, S. Rehman and I. U. Rehman, Appl. Spectrosc. Rev., 2007, 42, 493–541 CrossRef CAS.
- K. Liu, Q. Zhao, B. Li and X. Zhao, Front. Bioeng. Biotechnol., 2022, 10, 354 Search PubMed.
- A. F. García-Flores, L. Raniero, R. A. Canevari, K. J. Jalkanen, R. A. Bitar, H. S. Martinho and A. A. Martin, Theor. Chem. Acc., 2011, 130, 1231–1238 Search PubMed.
- R. Wolthuis, M. van Aken, K. Fountas, J. S. Robinson, H. A. Bruining and G. J. Puppels, Anal. Chem., 2001, 73, 3915–3920 CrossRef CAS PubMed.
- S. P. Mulvaney and C. D. Keating, Anal. Chem., 2000, 72, 145–158 CrossRef CAS PubMed.
- Y. Zhang, H. Hong and W. Cai, Curr. Pharm. Biotechnol., 2010, 11, 654 CAS.
- C. Krafft and J. Popp, Anal. Bioanal. Chem., 2015, 407, 8263–8264 CrossRef CAS PubMed.
-
E. Smith and G. Dent, Modern Raman spectroscopy—a practical approach, John Wiley & Sons, 2005, vol. 36 Search PubMed.
- R. Pilot, R. Signorini, C. Durante, L. Orian, M. Bhamidipati and L. Fabris, Biosensors, 2019, 9, 1–99 CrossRef PubMed.
- W. R. Premasiri, J. C. Lee, A. Sauer-Budge, R. Theberge, C. E. Costello, L. D. Ziegler and D. Fraunhofer, Anal. Bioanal. Chem., 2016, 408, 4631–4647 CrossRef CAS PubMed.
- S. Liu, Q. Hu, C. Li, F. Zhang, H. Gu, X. Wang, S. Li, L. Xue, T. Madl, Y. Zhang and L. Zhou, ACS Sens., 2021, 6, 2911–2919 Search PubMed.
- E. Witkowska, D. Korsak, A. Kowalska, A. Janeczek and A. Kamińska, Anal. Bioanal. Chem., 2018, 410, 5019–5031 Search PubMed.
- A. Kudelski, Talanta, 2008, 76, 1–8 Search PubMed.
- S. M. Berus, M. Adamczyk-Popławska, K. Goździk, G. Przedpełska, T. R. Szymborski, Y. Stepanenko and A. Kamińska, Int. J. Mol. Sci., 2022, 23, 12576 CrossRef CAS PubMed.
- N. G. Bastús, J. Comenge and V. Puntes, Langmuir, 2011, 27, 11098–11105 Search PubMed.
- W. Haiss, N. T. K. Thanh, J. Aveyard and D. G. Fernig, Anal. Chem., 2007, 79, 4215–4221 Search PubMed.
- S. L. Hillier, M. A. Krohn, L. K. Rabe, S. J. Klebanoff and D. A. Eschenbach, Clin. Infect. Dis., 1993, 16, S273–S281 CrossRef PubMed.
- D. H. Owen and D. F. Katz, Contraception, 1999, 59, 91–95 CrossRef CAS PubMed.
- C. A. Lieber and A. Mahadevan-Jansen, Appl. Spectrosc., 2003, 57, 1363–1367 Search PubMed.
- S. He, W. Zhang, L. Liu, Y. Huang, J. He, W. Xie, P. Wu and C. Du, Anal. Methods, 2014, 6, 4402–4407 Search PubMed.
- R. Gautam, S. Vanga, F. Ariese and S. Umapathy, EPJ Tech. Instrum., 2015, 2, 1–38 Search PubMed.
- D. A. Armbruster and T. Pry, Clin. Biochem. Rev., 2008, 29, S49 Search PubMed.
- S. Wold, M. Sjöström and L. Eriksson, Chemom. Intell. Lab. Syst., 2001, 58, 109–130 CrossRef CAS.
-
S. Wold, E. Johansson and M. Cocchi, in 3D QSAR in Drug Design; Theory, Methods, and Applications, ed. H. Kubinyi, Kluwer/Escom, 2000, vol. 1 Search PubMed.
- S. Efrima and L. Zeiri, J. Raman Spectrosc., 2009, 40, 277–288 CrossRef CAS.
- P. Kubryk, R. Niessner and N. P. Ivleva, Analyst, 2016, 141, 2874 Search PubMed.
- H. Kim, T. Kim, J. Kang, Y. Kim and H. Kim, Microorganisms, 2020, 8, 969 Search PubMed.
- I. A. Boginskaya, E. A. Slipchenko, M. V. Sedova, J. Y. Zvyagina, A. D. Maximov, A. S. Baburin, I. A. Rodionov, A. M. Merzlikin, I. A. Ryzhikov and A. N. Lagarkov, Chemosensors, 2023, 11, 321 CrossRef CAS.
- S. Stewart and P. M. Fredericks, Spectrochim. Acta, Part A, 1999, 55, 1641–1660 Search PubMed.
-
H. Abdi, in Encyclopedia of Social Sciences Research Methods, ed. M. Lewis-Beck, A. Bryman and T. Liao, Sage Publications, 2003 Search PubMed.
- S. Guo, J. Popp and T. Bocklitz, Nat. Protoc., 2021, 16, 5426–5459 CrossRef CAS PubMed.
- I.-G. Chong and C.-H. Jun, Chemom. Intell. Lab. Syst., 2005, 78, 103–112 Search PubMed.
- R. A. V. Rossel and T. Behrens, Geoderma, 2010, 158, 46–54 CrossRef CAS.
- M. Vaneechoutte, Res. Microbiol., 2017, 168, 826–836 CrossRef PubMed.
- H. Verstraelen, R. Verhelst, G. Claeys, E. De Backer, M. Temmerman and M. Vaneechoutte, BMC Microbiol., 2009, 9, 1–10 Search PubMed.
- G. P. Szekeres and J. Kneipp, Front. Chem., 2019, 7, 434849 Search PubMed.
- T. T. B. Quyen, W. N. Su, K. J. Chen, C. J. Pan, J. Rick, C. C. Chang and B. J. Hwang, J. Raman Spectrosc., 2013, 44, 1671–1677 CrossRef CAS.
- G. Pezzotti, J. Raman Spectrosc., 2021, 52, 2348–2443 CrossRef CAS.
|
This journal is © The Royal Society of Chemistry 2024 |
Click here to see how this site uses Cookies. View our privacy policy here.