DOI:
10.1039/D3CE01306E
(Paper)
CrystEngComm, 2024,
26, 4782-4803
Quadrupolar NMR crystallography guided crystal structure prediction (QNMRX-CSP)†
Received
23rd December 2023
, Accepted 19th July 2024
First published on 22nd July 2024
Abstract
We describe a new quadrupolar NMR crystallography guided crystal structure prediction (QNMRX-CSP) protocol for the prediction and refinement of crystal structures, including its design, benchmarking, and application to seven organic HCl salts. Five HCl salts with a limited number of low-energy conformations were chosen as model systems for benchmarking: betaine HCl, glycine HCl, D-alanine HCl, guanidine HCl, and aminoguanidine HCl; two were chosen for blind tests: N,N′-dimethylglycine HCl and metformin HCl. The QNMRX-CSP protocol uses experimental 35Cl solid-state NMR (SSNMR) spectra and X-ray diffraction (XRD) data in tandem with Monte Carlo simulated annealing and dispersion-corrected plane-wave density functional theory (DFT-D2*) calculations. The protocol comprises three modules: (i) the assignment of motion groups, (ii) a Monte Carlo simulated annealing algorithm for generating tens of thousands of candidate structures, and (iii) DFT-D2* geometry optimizations of structural models and concomitant computation of 35Cl EFG tensors. Key benchmarked metrics are used for retaining the best candidate structures, including unit cell parameters, static lattice energies, and EFG distances (ΓEFG). The protocol is shown to generate structural models that are excellent matches with experimental crystal structures that have been DFT-D2* geometry optimized, as validated by crystallographic R-factors (R) and root-mean squared distances (RMSDs) of atomic positions that are well below 10% and 0.2 Å, respectively. Finally, consideration is given to the use of the QNMRX-CSP protocol as a standalone technique or in concert with other NMRX methods and Rietveld refinements, and possible applications employing other quadrupolar nuclei (i.e., 14N and 17O).
1. Introduction
Crystal structure prediction (CSP) methods are increasingly used to calculate the structures and properties of known materials, as well as for the discovery of new materials.1–7 CSP methods can be grouped into two general but complementary areas: (i) first principles calculations for structural prediction with limited or no experimental input and (ii) computational mining of databases such as the Cambridge Structural Database,8,9 Crystallography Open Database,10 and Inorganic Crystal Structure Database.11 CSP is critical for establishing structure–property-function relationships in materials; as such, the Cambridge Crystallographic Data Center9 (CCDC) has conducted a series of collaborative “blind tests” in which they solicited researchers to use CSP methods for the prediction and refinement of crystal structures.12–17
CSP methods are applied extensively to organic compounds18–23 and active pharmaceutical ingredients (APIs)24,25 to aid in screening of their potential solid forms (i.e., polymorphs, salts, hydrates, solvates, and cocrystals)26–29 and prediction of properties.30,31 CSP methods are not without their limitations, since they can be computationally expensive in the absence of key structural information, such as the unit cell parameters, the number of crystallographically distinct molecules (Z′), and/or the identities of multiple molecular conformations or electronic configurations (e.g., conformers, tautomers, zwitterions, etc.). Furthermore, the differences in energy among similar structures tend to be small and can depend on the computational methods, posing difficulties in evaluating and validating structures based solely on their energies.32–34 In view of this, CSP methods can benefit from data derived from powder X-ray diffraction (pXRD) (i.e., unit cell parameters, space group, Z′) and solid-state NMR (SSNMR) spectroscopy (i.e., Z′, molecular conformations, dynamics, disorder, internuclear distance measurements, and bonding).
In this regard, NMR crystallography (NMRX), which uses SSNMR data to aid in the prediction, validation, and/or refinement of crystal structures, has emerged as a fruitful area of research, complementing and enhancing CSP investigations.35–41 In the most simple form of NMRX, SSNMR has long been applied to aid in identifying the space group and/or number of crystallographically distinct sites in the unit cell.35,42–44 By contrast, more modern NMRX studies have featured the measurements and/or first principles calculations of 1H chemical shifts, homonuclear dipolar couplings, and spin diffusion rates to solve crystal structures of small organic molecules,45–50 or in other instances, 29Si chemical shifts and homonuclear dipolar couplings to determine structures of zeolites and silicate frameworks.51–53
More recently, contemporary NMRX-CSP methods have demonstrated impressive results for molecular organic crystals and pharmaceutical solids,54–60 usually combining computational methods and experimental data that usually include: (i) density function theory (DFT) or other ab initio calculations for geometry optimization and calculation of NMR parameters; (ii) SSNMR methods for the accurate measurement of chemical shifts; and (iii) the acquisition of high-quality pXRD data, for purposes of fingerprinting, structural validation, and/or Rietveld refinement.61–63 DFT calculations of magnetic shielding parameters, which largely involve comparison to 13C, 1H, or 15N isotropic chemical shifts,64–73 and to a lesser degree, chemical shift tensors,74–79 can be computationally demanding, depending on the choice of functional and basis set. Furthermore, modeling the influences of long-range inter- and intramolecular interactions (e.g., hydrogen bonding, π–π stacking, etc.) on NMR interaction tensors can be approached with methodologies involving periodic boundary conditions, cluster-based calculations, or fragment-based calculations. GIPAW calculations are widely used for the calculation of NMR tensor parameters from models of periodic solids, but are limited in the type of model chemistry that can be used efficiently (e.g., calculations using hybrid functionals are computationally expensive);72,80–82 in contrast, calculations using clusters and/or fragments to model the extended lattice structure permit the exploration of different classes of functionals in an efficient manner, allowing for increasingly accurate calculations of chemical shifts.64,79 Finally, since the successful prediction of chemical shifts has generally come with high computational expense, Emsley et al. and other researchers have developed machine-learning methods for rapidly predicting 1H, 13C, 15N, and 17O chemical shifts, with applications in NMRX-CSP.83–88
Quadrupolar nuclides (i.e., nuclear spins I > ½) have been utilized in NMRX studies to a much lesser degree,89–109 though we note there are numerous studies that employ quadrupolar SSNMR, XRD, and DFT methods that are not explicitly described as NMRX. To the best of our knowledge, there are no NMRX-CSP studies that feature the combined use of crystal packing algorithms, DFT calculations of electric field gradient (EFG) tensors, and quadrupolar NMR data for organic solids. This is somewhat surprising, given the many quadrupolar nuclides of elements of great import to organic solids (i.e., 14N, 17O, 23Na, 35Cl, etc.) and the exquisite sensitivity of EFGs at the nucleus to the local structural environment (this is especially true of the longer-range, weaker intermolecular interactions associated with crystal packing, which often have only minor influences on chemical shifts). Many studies by our group and others have shown the great utility of the relationships between EFG tensors and atomic environments in API solid forms110–119 for purposes of spectral fingerprinting,120,121 identifying impurities,122 observing molecular-level dynamics,123–127 and quantification,128–130 which are critical first steps towards applications in NMRX.
Crucial in quadrupolar NMRX methods is the computation of EFG tensors that match well with experiment. In organic solids, the positions of hydrogen atoms are well known to influence 14N, 17O, and 35Cl EFG tensors.111,131,132 We have found that DFT methods employing a dispersion correction (DFT-D2*) for the accurate refinement of atomic coordinates, particularly hydrogen atom positions, generally result in excellent agreement between calculated and measured EFG tensors.133–135 Notably, 35Cl EFG tensors of chloride ions, which are prevalent in the many HCl salts of APIs (nearly half of known solid forms of APIs),136 are very sensitive to the numbers, arrangements, and types of hydrogen bonds (under the broadest definition of H⋯Cl bonds of ≤2.6 Å,137–139 it is those of ca. 2.2 Å or less that most dramatically impact 35Cl EFG tensors).97 Hence, with the recent successes of DFT-D2* methodologies, SSNMR of quadrupolar nuclei can start to play an important role in modern NMRX-CSP studies of organic solids.
In this work, we present a new quadrupolar NMR crystallography guided crystal structure prediction (QNMRX-CSP) protocol that uses SSNMR and XRD data, along with a Monte Carlo simulated annealing algorithm and plane-wave DFT-D2* calculations, and its application to organic HCl salts (Scheme 1), with an emphasis on the role of 35Cl EFG tensors. The QNMRX CSP protocol (Scheme 2) is comprised of three modules, each of which features clearly delineated steps, and key metrics for retaining the best candidate structures (see §2.2), which include unit cell parameters, static lattice energies (Elat and Ecutoff), and the EFG distances (ΓEFG). Final candidate structures are validated using crystallographic R-factors (R) and comparison of atomic positions via calculations of root-mean squared distances (RMSDs).
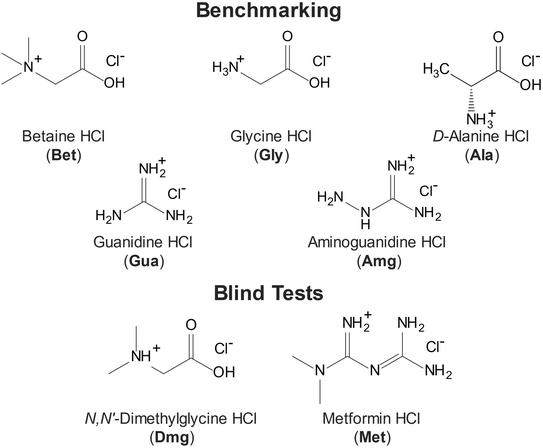 |
| Scheme 1 Molecular diagrams of the seven HCl salts of organic molecules. Five structural models are used for benchmarking calculations (betaine HCl (Bet), glycine HCl (Gly), D-alanine HCl (Ala), guanidine HCl (Gua), and aminoguanidine HCl (Amg)) and two are used in blind test cases (N,N′-dimethylglycine HCl (Dmg) and metformin HCl (Met)). | |
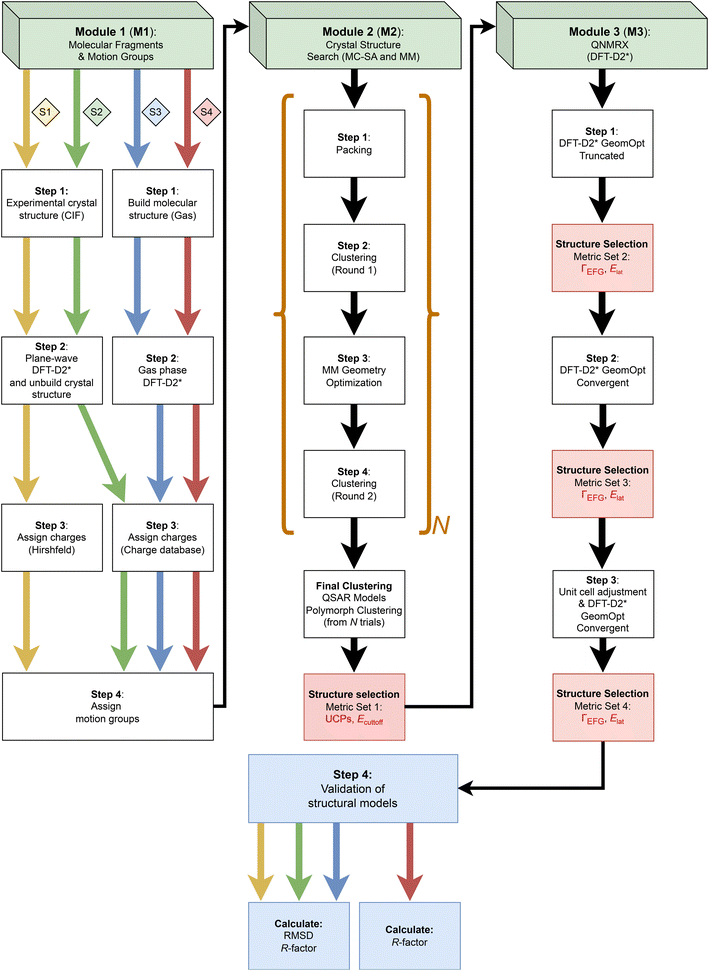 |
| Scheme 2 A flowchart of the QNMRX-CSP protocol. The protocol comprises three modules (M1, M2, and M3) indicated by the green boxes at the top of the figure, with each step indicated by a white box, benchmarked metrics used for selecting the best candidate structures indicated by red boxes, and structural validation steps indicated by blue boxes. The black arrows indicate progression through the protocol regardless of the stage, whereas the coloured arrows indicate the stage of the calculation (S1 yellow, S2 green, S3 blue, and S4 red) involved in benchmarking and blind tests. The polymorph prediction routine is indicated by the brown brackets and is repeated N times. Details are given in §3.2. | |
We discuss (i) the design of the QNMRX-CSP protocol; (ii) the restriction of the space group and the benchmarking of metrics for selecting candidate structures using structural models of five HCl salts of organic molecules (i.e., betaine HCl, glycine HCl, D-alanine HCl, guanidine HCl, and aminoguanidine HCl; Scheme 1), which have known crystal structures and 35Cl EFG tensors; and (iii) four different stages of calculations, including three benchmarking stages (Scheme 2: S1, S2, and S3, indicated by yellow, green, and blue arrows, respectively) and one stage for blind tests and/or predictions of unknown structures (Scheme 2: S4, red arrow). A detailed walkthrough of benchmarking is presented for the example of betaine HCl, and blind tests for the predictions of the structures of N,N′-dimethylglycine HCl and metformin HCl are considered. Finally, we discuss the use of the QNMRX-CSP protocol for molecules of greater size and flexibility, their implementation with other quadrupolar nuclides, and their general application as both standalone and complementary methods for refinement, prediction, and discovery of new crystal structures.
2. Methods
2.1 Computational details
Overview.
The following tools were used within BIOVIA Materials Studio 2020 R3:140 polymorph was used to assign motion groups and generate candidate crystal structures, quantitative structure activity relationship models polymorph clustering was used to cluster multiple polymorph trials, and CASTEP was used to run plane-wave DFT calculations82 on either structural models generated from polymorph or obtained from the CSD. See Supplement S1 in the ESI† for information on workstations.
Polymorph.
The polymorph prediction routine includes a Monte Carlo simulated annealing algorithm to search a phase space and generate candidate crystal structures. All space groups can be searched during the prediction routine; however, we chose to search space groups of the crystal structures from which the structural models were developed (§3.1). The polymorph prediction routine (steps 1–4 in M2, the Crystal Structure Search module) consists of four steps: (i) packing, (ii) a first round of clustering, (iii) geometry optimization, and (iv) a second round of clustering, which are all performed using the default “ultra-fine quality” settings. In the packing step (step 1), the maximum number of candidate structures to generate is set to 10
000, with maximum and minimum temperatures of 1.5 × 105 K and 300 K, respectively, heating and cooling factors of 0.025 and 0.0005, respectively, and a minimum move factor of 1.0 × 10−10. Heating during the packing step is carried out until either the maximum temperature is reached or a minimum of 14 consecutive structures are accepted (i.e., 14 structures, each lower in energy than the previous), at which point cooling begins. A preliminary geometry optimization is included in the packing step (vide infra). For the first clustering step (step 2), similar structures are grouped together and the lowest energy representative is retained based on the forcefield type (in this case, the Dreiding force field)141 using the following default set of parameters: a radial distribution cut-off of 7.0 Å (maximum interatomic distance cutoff for electrostatic interactions between pairs of atoms), a tolerance of 0.13 (the minimum similarity measure value for a structure to be included in a cluster, this value ranges between 0 and 1, with 1 being an exact match) and 140 bins (the maximum number of structures that are evaluated in a cluster). The geometry optimization step (step 3) is performed using the following convergence thresholds: 2 × 10−5 kcal mol−1 for energy, 10−3 kcal mol−1 Å−1 for forces, 10−3 GPa for stress, and 10−5 Å for structural displacement. During this step, the positions of the motion groups and unit cell parameters are allowed to vary over 500 iterations (the relative positions of the atoms within the motion groups are kept constant). To calculate the energies of the structural models, an atom-based summation method for all interaction energies (i.e., electrostatic, Van der Waals, and hydrogen bonding) involving the Dreiding forcefield was applied.124 Hirshfeld charges used in this step were obtained from a protocol described in the ESI† (see Table S1 and Supplement S2 for information on the Charge Database). Finally, the second clustering step (step 4) is performed with the same parameters used in the first clustering step, retaining the lowest energy structures. The polymorph prediction routine is repeated over N trials (in this study, N varies between 10 and 20, vide infra).
QSAR models polymorph clustering.
After performing N trials, the QSAR models polymorph clustering module142 was used to group any similar structures across different trials (e.g., trials 1 through 10), retaining the lowest energy structures and discarding the rest. The parameters used are identical to those involved in the clustering steps outlined in §2.1. The final structures from the Crystal Structure Search module (M2) are selected on the basis of unit cell parameters that best match experiment (§3.2) and the lowest static lattice energies (§2.2).
CASTEP.
Plane-wave DFT-D2* geometry optimizations133,134,143 employed the low-memory BFGS scheme,144 with structural refinements proceeding for five cycles (i.e., herein designated as the truncated BFGS scheme) or to convergence. Maximum values for structural convergence were set as follows: energy change (5 × 10−6 eV per atom), displacement (5 × 10−4 Å per atom), and Cartesian force (10−2 eV Å−1). The RPBE functional was used for all calculations, along with ultrasoft pseudopotentials generated on-the-fly,72 a plane-wave cutoff energy of 800 eV, evaluation of integrals over the Brillouin zone using a Monkhorst–Pack grid with a k-point spacing of 0.05 Å−1, and semi-empirical dispersion corrections using a modified version of Grimme's two-body atomic-pairwise model.143 Following all geometry optimizations, 35Cl EFG tensors were computed using a nuclear quadrupole moment of Q(35Cl) = −8.112 fm2.145
2.2 Metrics used in QNMRX-CSP
Several key metrics are used to assess agreement between experimentally determined structures and NMR parameters with their counterparts obtained from calculations.
Powder diffraction.
Diffraction patterns from known crystal structures or candidate structures were simulated using the powder pattern module within the Mercury program.146 Simulations included a Cu Kα radiation source with a wavelength of 1.54056 Å, a 2θ range of 5–50° with a step size of 0.02°, Bragg–Brentano geometry, and a full-width half maximum peak width of (0.1)θ. The crystallographic R-factors (R), which can be used as a measure of disagreement between observed (Fo) and calculated (Fc) signal amplitudes, are given by: | 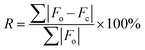 | (1) |
Crystal packing similarity.
The crystal packing similarity function147 in the CSD-Materials module within Mercury146 is used to compare crystal structures and calculate root-mean squared distances (RMSDs) of the atomic positions relative to a reference structure. Default options are used, including a packing shell size of 15 molecules with geometric tolerances of 20% for distances and 20° for angles.
Static lattice energy.
The static lattice energies (Elat) of structural models are used both after the M2 step 3 geometry optimization (Ecutoff) and each M3 DFT-D2* geometry optimization. In M2 step 3, extensive benchmarking calculations across all model systems determined that those structural models with values of Elat that are higher than Elow by a factor of 0.135·|Elow| are retained. Since Elat values are negative, an equation defining the value of Ecutoff for structural models retained by this metric is: | Ecutoff ≤ Elow − 0.135·Elow | (2) |
In M3 steps 1–3, all structures are retained with static lattice energies falling under the lowest value of Elat determined from benchmarking calculations (§3.2).
EFG distance.
The degree of similarity between calculated and experimental EFG tensors with principal components Vkk, k = 1, 2, 3, which are defined such that |V33| ≥ |V22| ≥ |V11|, is evaluated using the EFG distance (ΓEFG) metric,134 which is analogous to the chemical shift distance introduced by Grant et al.148ΓEFG is defined as: |  | (3) |
| Δkk = |Vcalckk − Vexpkk| | (4) |
N.B.: because the sign of CQ (and V33) cannot be determined experimentally, only the absolute magnitudes of the values of Vkk are used in this expression.
3. Results and discussion
The development, testing, and application of the QNMRX-CSP protocol involved refinement of the steps within each module in terms of their position, function, and computational expense, as well as benchmarking key metrics over several computational stages that are used to select structural candidates that best match with experiment. We describe: (i) in §3.1 our rationale and methodology for choosing certain simple organic hydrochloride salts as model systems (Scheme 1); (ii) in §3.2 the three modules (M1, M2, and M3) that constitute the protocol, including the individual steps in each module (Scheme 2); (iii) in §3.3 the four stages of calculations (S1, S2, S3, and S4, as indicated by the yellow, green, blue, and red arrows, respectively, in Scheme 2) involved in benchmarking the metrics via comparisons to known structures (S1, S2, and S3) or for “blind” tests (S4), with the goal of enabling the prediction of hitherto unknown structures; (iv) in §3.4 a walkthrough of the application of the QNMRX-CSP protocol (in S1) to predict a structural model of betaine HCl and presentation of similar data for other model systems; and (v) in §3.5 the application of QNMRX-CSP to two blind tests (in S4) to solve the structures of N,N′-dimethylglycine HCl and metformin HCl.
3.1 Choice of model systems
We highlight five organic HCl salts (Scheme 1) that were used to benchmark the QNMRX-CSP protocol through three stages (S1–S3), including Gly, Bet, Ala, Gua, and Amg, with Dmg and Met used in S4 for “blind” tests. These systems were selected using the following criteria: (i) they are crystalline solids of with a limited number of low-energy conformations; (ii) they have known crystal structures (see Table 1, N.B.: there are two polymorphs for Bet and Met, which were not attempted and do not have their crystal structure information listed);149,150 (iii) they feature a single chemical formula unit per asymmetric unit (i.e., Z′ = 1);151 and (iv) they have accurately measured 35Cl EFG tensors (see Table 2) that are in good agreement with those obtained from structural models refined using the DFT-D2* method.133,134 Although these molecules are expected to have varying, small degrees of conformational flexibility, their structural models are treated as rigid bodies during the Monte Carlo simulated annealing routines in M2, but are allowed to vary conformationally during the plane-wave DFT-D2* geometry optimizations.
Table 1 Crystallographic information for organic HCl salts
Compounda |
Space group |
a (Å) |
b (Å) |
c (Å) |
α (°) |
β (°) |
γ (°) |
Volume (Å3) |
Z
|
Crystallographic structure database reference codes for crystal structures glycine HCl (GLYHCL), betaine HCl (BETANC01), D-alanine HCl (ALAHCL), guanidine HCl (GUANIDC01), aminoguanidine HCl (AMGUAC02), N,N′-dimethylglycine (BUTNIN), and metformin HCl (JAMRIY01).
All structures have Z′ = 1. See main text for discussion.
|
Betaine HCl |
P21/c |
7.428 |
9.108 |
11.550 |
90.00 |
96.71 |
90.00 |
776.054 |
4 |
Glycine HCl |
P21/c |
7.117 |
5.234 |
13.745 |
90.00 |
97.25 |
90.00 |
507.913 |
4 |
D-Alanine HCl |
P212121 |
7.148 |
17.590 |
5.202 |
90.00 |
90.00 |
90.00 |
654.065 |
4 |
Guanidine HCl |
Pbcn
|
7.614 |
9.015 |
13.051 |
90.00 |
90.00 |
90.00 |
895.941 |
8 |
Aminoguanidine HCl |
P21/n |
6.038 |
7.809 |
11.103 |
90.00 |
90.00 |
77.07 |
510.241 |
4 |
N,N′-Dimethylglycine HCl |
P21/c |
7.061 |
7.194 |
13.932 |
90.00 |
98.88 |
90.00 |
699.219 |
4 |
Metformin HCl |
P21/c |
7.923 |
13.894 |
7.923 |
90.00 |
114.49 |
90.00 |
793.797 |
4 |
Table 2 Experimentally measured EFG tensor and chemical shift parameters for organic HCl salts
Compound |
C
Q
(MHz) |
η
Q
|
δ
iso
(ppm) |
Ref. |
The experimental uncertainty in the last digit(s) for each value is indicated in parentheses. CQ = eQV33/h, where the principal components of the EFG tensor are defined such that |V33| ≥ |V22| ≥ |V11|. The sign of CQ cannot be determined from the experimental 35Cl NMR spectra, but can be determined from first principles calculations. ηQ = (V11 − V22)/V33. δiso = (δ11 + δ22 + δ33)/3. |
Bet |
5.95(8) |
0.1(2) |
95(3) |
128
|
Gly |
6.42(5) |
0.61(3) |
101(5) |
113
|
Ala |
6.4(1) |
0.75(6) |
106(5) |
118
|
Gua |
2.65(8) |
0.78(4) |
101(4) |
128
|
Amg |
2.0(2) |
0.76(4) |
50(3) |
111
|
Dmg |
4.74(6) |
0.74(2) |
103(5) |
128
|
Met |
2.44(4) |
0.61(5) |
60(2) |
129
|
3.2 Quadrupolar NMR crystallography guided crystal structure prediction
The QNMRX-CSP protocol (Scheme 2) includes three modules: (i) M1: molecular fragments and motion groups; (ii) M2: Crystal Structure Search; and M3: quadrupolar NMR crystallography (QNMRX). Each module was designed to include well delineated steps, some of which use key metrics, determined by numerous benchmarking trials, to retain candidate structures. We reiterate that the numerical values of these metrics were benchmarked on several structural models of HCl salts, which are used as upper limits for structural retention. They include unit cell parameters (i.e., a, b, c, α, β, and γ), static lattice energies (i.e., Ecutoff and Elat, §2.2 for definitions), and comparisons of experimental and calculated 35Cl EFG tensors, as described by EFG distances (ΓEFG, §2.2 for definitions).
The QNMRX-CSP protocol proceeds as follows: M1 provides the molecular fragments and motion groups, where molecular fragments are defined as chemically sensible groupings of atoms into molecules and/or ions with independent formula units, and motion groups are defined as independent formula units that are allowed to move during M2. We note that there may be multiple motion groups in a molecular fragment; however, for the benchmarking cases described herein, the molecular fragments and motion groups are identical for all intents and purposes. In M2, these molecular fragments are input into a Monte Carlo simulated annealing algorithm, which generates thousands of candidate structures with distinct unit cells and packing arrangements. Structural models are retained based on unit cell parameters and Ecutoff. In M3, a series of three distinct plane-wave DFT geometry optimizations are applied, along with calculations of 35Cl EFG tensors and Elat, all of which are used as metrics for retention of structures. Finally, the structural models are validated based on comparison to pXRD patterns by calculating R and RMSDs (see §2.2 for definitions).
We now turn our attention to the details of these modules, beginning with benchmarking calculations, which feature comparison of computationally derived structural models and NMR parameters with known experimental data (i.e., a QNMRX-CSP computation in S1, following the pathway indicated by the yellow arrows in Scheme 1). Here, the objective is to start with a known molecular fragment and execute the QNMRX-CSP protocol while considering the functions of the individual steps and benchmarking of metrics, in order to obtain one or more structural models for validation.
Module 1: molecular fragments and motion groups.
The objective of M1 is to obtain suitable molecular fragments with appropriate atomic charges and well-defined motion groups. First, for purposes of benchmarking calculations in S1, a crystal structure is obtained from the CSD and a structural model based on the CIF is subjected to a DFT-D2* geometry optimization. Next, the crystal structure is unbuilt to obtain the molecular fragments (i.e., the chloride anion and organic cation) to which the calculated Hirshfeld charges are applied (Tables S2–S8†). In the initial stages of developing our QNMRX-CSP protocol, the assignments of charges using both Hirshfeld152 and Mulliken153 population analysis were explored (Table S9†); we proceeded with Hirshfeld charges, since this led to valid structural solutions with greater frequency. Finally, the chloride anion and organic cation are set as independent motion groups.
Module 2: Crystal Structure Search.
M2 generates thousands of candidate structures with distinct unit cells and arrangements of molecular fragments using Monte Carlo simulated annealing, with the fragments obtained from M1 as a starting point. We chose the polymorph prediction routine as implemented in BIOVIA Materials Studio,140 which is reported in CSP studies154–159 and CCDC blind tests of organic molecules.12–17
The polymorph prediction routine in M2 consists of four steps: packing, a first round of clustering, geometry optimization, and a second round of clustering, where (i) packing randomly generates crystal structures and minimizes their energies with unit cell and geometry optimizations; (ii) a first round of clustering aggregates similar structures into groups and retains the lowest energy representatives; (iii) geometry optimizations minimize the energies of the remaining structures, and (iv) a second round of clustering removes any remaining duplicate structures (§2.1). For a given structural model, this routine is executed N times (the value of N is subjective, and largely determined by the retention of structural models that pass structural validation in M3, vide infra).
There are several parameters in M2 that can be adjusted. First, the algorithm in M2 can be executed with multiple clustering and geometry optimization steps, as demonstrated by Park et al., where a routine consisting of six individual steps was used (i.e., packing, clustering, geometry optimization, clustering, geometry optimization, and clustering) to generate candidate crystal structures of a contorted-hexabenzocoronene.159 We found the extra steps of a second geometry optimization and third round of clustering unnecessary. Second, each step has its own settings, which strikes a balance between computational cost and confidence in sampling all possible candidate structures.154–159 Third, the choice of force field and summation method for interaction energies is critically important. For organic molecules, two reliable classes of force fields are Dreiding141 and variations of COMPASS (i.e., I, II, and III),160,161 which were used in previous CCDC blind tests.12–17 The summation methods for these force fields can be chosen as atom-based, group-based, Ewald, or PPPM.162–164 In this work, we used the Dreiding force field with an atom-based summation, as this combination provides the best balance between quality and computational cost. Finally, we selected the space group(s) of interest. It is common practice to search the nine most common space groups that occur for ca. 95% of organic crystalline solids, including P21/c, P
, P212121, P21, C2/c, Pbca, Pnma, Pna21, and Pbcn (of these, the first 5 comprise 75% of known structures).165 Herein, the choice of space group in M2 is restricted to that of the reported crystal structure (i.e., P21/c, P212121, or Pbcn) in our benchmarking calculations and blind tests as this greatly reduces the computational expense and enable us to validate our metrics of static lattice energies and EFG tensors for structural selection and not space group selection. N.B.: in the case of an unknown structure, indexing of a high-quality pXRD pattern could provide information on the space group; however, it is possible to explore different space groups, albeit at increased computational expense (this is beyond the scope of the current work).
On average, one trial of the polymorph prediction routine in M2 (i.e., one iteration of packing, clustering, geometry optimization, and clustering—indicated by the square brackets in Scheme 2) for a small organic HCl model generates between ca. 3000 and 5000 candidate structures. The total number of trials ranges between N = 10 and 20.
After these trials, the first set of benchmarked metrics involving Ecutoff (i.e., structures are retained that fall within 13.5% of the lowest energy structure) and unit cell parameters (i.e., a, b, and c, and the unique angle β, which are within ±20% of those of the known crystal structure with α and γ fixed at 90° for the space groups herein), are applied. One final clustering cycle was performed to remove any remaining duplicate structures between trials.
Module 3: quadrupolar NMR crystallography.
M3 consists of four steps. Steps 1, 2, and 3 entail three consecutive DFT-D2* geometry optimizations (under different conditions, vide infra) and concomitant calculations of 35Cl EFG tensors. The metrics, ΓEFG and Elat, were progressively adjusted downwards with each consecutive calculation, for selecting the best candidate structures. In step 4, the structural models are validated by calculating the R and positional RMSD values (N.B.: this type of structural validation is only made in benchmarking calculations when comparison to a refined crystal structure is possible; for blind tests or unknown structures, only the R is calculated for comparison against experimentally derived pXRD data).
The details of the DFT-D2* geometry optimization steps and metrics used in structural selection in M3 are as follows: first, DFT-D2* truncated BFGS geometry optimizations (see §2.1) are performed and the 35Cl EFG tensors are calculated. These rapidly eliminate many unreasonable structural models without the need for fully-converged geometry optimizations. Benchmarked metrics of ΓEFG ≤ 0.7 MHz and Elat ≤ 135 kJ mol−1 are used to retain candidate structures (typically, 10% or less are retained). Second, convergent DFT-D2* geometry optimizations are performed and 35Cl EFG tensors are calculated, and ΓEFG ≤ 0.49 MHz and Elat ≤ 50 kJ mol−1 are used to retain the best candidates. Finally, the remaining structural models have their unit cell parameters adjusted to match those of the known crystal structures, followed by a convergent DFT-D2* geometry optimization and calculation of 35Cl EFG tensors. At this point, the benchmarked metrics are the most stringent, with ΓEFG ≤ 0.49 MHz and Elat ≤ 1 kJ mol−1. N.B.: the adjustment of the unit cell is not possible unless there are unit cell parameters available from a known crystal structure (in benchmarking) or from indexed pXRD data (in blind tests and for unknown structures), which is a limitation of the protocol at this point. This limitation arises, in part, from the tendency of DFT-D2* geometry optimizations to overestimate the unit cell volumes;166,167 this may be rectified in future studies, as routines for higher-order dispersion corrections become available in the CASTEP platform (e.g., D3 and D3(BJ)).168–170
In step 4, comparisons of R (experimental vs. theoretical pXRD patterns) and RMSDs of atomic positions (when known structures are available) are used to validate the final structural models. In protein and pharmaceutical crystallography, refined structures that are deemed acceptable have R ≤ 25% and R ≤ 10%, respectively.171–174 In recent CCDC blind tests, submitted structures are reported as being valid with RMSDs ≤ 0.80 Å; however, this large RMSD is dependent on the size and flexibility of the molecule, with the best structures typically ≤0.20 Å.17 In this work, the candidate structural models that passed our final set of metrics consistently yielded values of R ≤ 9.2% and RMSD ≤ 0.12 Å, with most well below the thresholds of the abovementioned standards (see Tables S10–S14†).
M3 is the most computationally demanding module in the QNMRX-CSP protocol; as such, we have explored numerous measures to reduce computational times while not sacrificing the selection of suitable candidate structures. The first DFT-D2* geometry optimization and calculation of EFG tensors is computationally inexpensive; however, the second and third DFT-D2* geometry optimizations and EFG tensor calculations take considerably more time (N.B.: these calculations are run in parallel to reduce computational time). The net computational time depends on several factors: (i) the size of the unit cell; (ii) the numbers and types of atoms in the molecular units; (iii) increased numbers of motion groups (including separate molecular fragments and/or conformational flexibility); (iv) the number of candidate structures remaining after the application of a given metric; and (v) computational power. The structural models we have chosen for this study are ideal in the sense that they have relatively few atoms and small unit cells; however, some initial studies in our group on systems of increasing size and complexity are indicative of success, albeit with longer net computational times (this is beyond the scope of the current work). Of the factors above, an increased number of motion groups presents interesting problems that must be dealt with in both M1 and M3. It is possible that careful selection of multiple low-energy conformers in M1 could greatly accelerate this protocol for calculations on structures of increasing complexity and degrees of motional freedom. These aspects are given preliminary exploration in the discussion of blind tests (§3.5).
3.3 QNMRX-CSP benchmarking stages
The QNMRX-CSP protocol and its three modules described in §3.2 are used for purposes of designing and refining the protocol and benchmarking its metrics. As discussed above, this initial benchmarking stages is referred to as S1 (Scheme 2, yellow arrows). To progress toward blind or de novo CSP, three more stages were explored, including two other benchmarking stages, S2 and S3, and a series of blind tests in S4. These stages each start with different types of structural models and/or assignments of Hirshfeld charges, as described below.
Stage 1: known crystal structure and calculated Hirshfeld charges.
S1 (Scheme 2, yellow arrows, §3.2), serves as the initial test of the QNMRX-CSP protocol, where the goal is to predict a structure using geometry-optimized fragments and concomitant Hirshfeld charges with the known crystal structure as the starting point.
Stage 2: known crystal structure and charge database.
S2 (Scheme 2, green arrows) is identical to S1 in all respects, except for the use of a database of Hirshfeld charges to make charge assignments on the molecular fragments. The motivation for this benchmarking stage is (i) to test the QNMRX-CSP protocol with pre-determined Hirshfeld charges; and (ii) to ensure that when QNMRX-CSP is applied to unknown structures, for which no computationally derived Hirshfeld charges are available, that a reliable database of charges can be used in M1 calculations. Details on the development of the database of Hirshfeld charges, are found in the ESI,† Supplement S1, and Table S1.
Stage 3: gas-phase structural model and Hirshfeld charge database.
In S3 (Scheme 2, blue arrows), molecular fragments are not taken from crystal structures, but rather, derived from gas-phase geometry optimizations. First, a structural model of the organic cation is built (net charge of +1), confined within the center of a (15 × 15 × 15) Å3 unit cell with P1 symmetry, and subjected to a convergent DFT-D2* geometry optimization. This new geometry-optimized organic cation is assigned Hirshfeld charges as described in S2. This organic cation and a chloride ion are subsequently used in calculations in the M1 module. S3 is still considered a benchmarking stage, since (i) structural validation in M3 includes comparison of both R and RMSD values, the latter of which depends on knowledge of the crystal structure; and (ii) the results of these calculations, which closely resemble the approach to be used for blind tests and unknown structures, were used to refine the QNMRX-CSP protocol and its associated metrics.
Stage 4: blind tests and unknown structures.
S4 (Scheme 2, red arrows) is identical in almost every respect to S3; however, it is reserved for the application to systems with unknown crystal structures. This includes blind tests, in which the structure is known to a third party who validates the final structures via comparison of RMSDs (i.e., the best structural candidates are submitted to the third party, with only R used for final structural validation in step 4). Furthermore, in S4, the unit cell parameters and EFG tensors are derived solely from experiment, and the final candidate structures are validated using benchmarked values of ΓEFG and R derived from S1 to S3 (N.B.: after the successful prediction of the crystal structure in a S4 blind test, it is possible to use the resulting data for overall refinement of the QNMRX-CSP protocol and improvement of metrics).
3.4 QNMRX-CSP of structural models: a walkthrough for betaine HCl
A walkthrough of the application of QNMRX-CSP in S1 is described below using Bet as the example (Fig. 1–3). The numbers of structural models generated by M2 and M3 steps 1–3, along with the structural candidates selected by application of the benchmarked metrics, are summarized in Table 3.
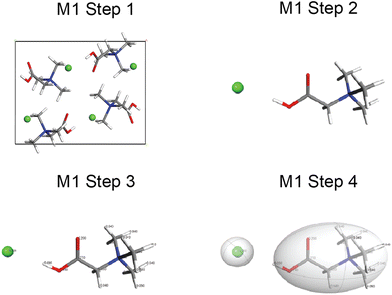 |
| Fig. 1 Schematic diagrams for the walkthrough of the QNMXR-CSP benchmarking protocol for betaine HCl in Module 1, stage 1 (molecular fragments and motion groups). M1 step 1: obtain a known crystal structure (BETANC01). M1 step 2: perform a DFT-D2* geometry optimization and unbuild the crystal structure. M1 step 3: assign the Hirshfeld charges to the atoms. M1 step 4: assign the motion groups. | |
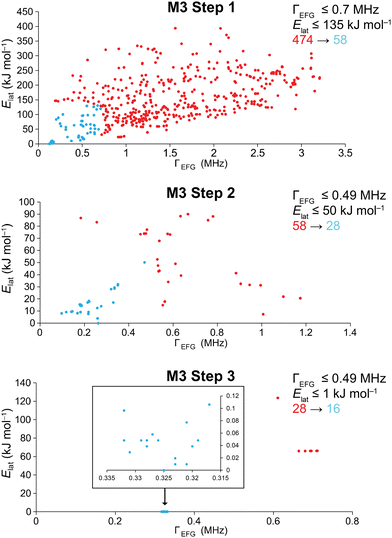 |
| Fig. 2 Scatter plots of Elatvs. ΓEFG for the walkthrough of the QNMXR-CSP protocol in Module 3, stage 1, steps 1–3 (QNMRX) for betaine HCl: red and blue points denote discarded and retained candidate structures, respectively. The numbers of structures before (red) and after (blue) the application of benchmarked metrics are shown to the right. Shown in the inset of the scatter plot in M3 step 3 are the structures that have Elat ≤ 1 kJ mol−1. | |
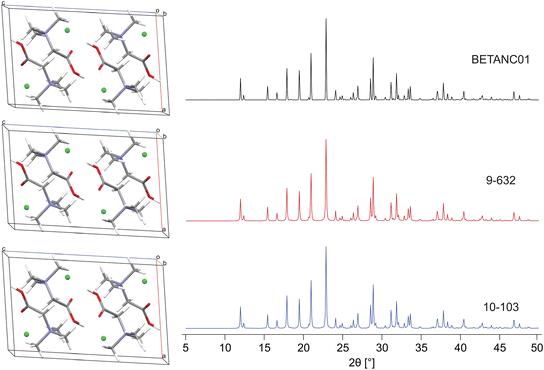 |
| Fig. 3 A comparison of the DFT-D2* geometry-optimized structural model of betaine HCl derived from its known crystal structure (BETANC01) with two (from a set of 16) validated structural models from S1 benchmarking trials, 9-632 and 10-103 with a ΓEFG = 0.321 and 0.317 MHz, Elat = 0.010 and 0.106 kJ mol−1, R = 1.135 and 1.737%, and RMSD = 0.003 and 0.113 Å, respectively. | |
Table 3 The numbers of structural models retained from the QNMRX-CSP protocol in stage 1 as applied in benchmarking calculations on betaine HCl
QNMRX-CSP protocola |
Number of structures |
Initial |
|
Retained |
Benchmarked metrics for structure selection in modules M2 and M3 are listed in the leftmost column, including the uncertainty in unit cell parameters, a, b, c, α, β, and γ; and threshold values for the static lattice energies, Ecutoff and Elat; and the EFG distance, ΓEFG (see text for more details).
|
M2: ±20% a, b, c, α, β, γ; Ecutoff ≤ 13.5% |
49 451 |
→ |
474 |
M3 step 1: ΓEFG ≤ 0.70 MHz; Elat ≤ 135 kJ mol−1 |
474 |
→ |
51 |
M3 step 2: ΓEFG ≤ 0.49 MHz; Elat ≤ 50 kJ mol−1 |
51 |
→ |
28 |
M3 step 3: ΓEFG ≤ 0.49 MHz; Elat ≤ 1 kJ mol−1 |
28 |
→ |
16 |
In M1 (Fig. 1), step 1 consists of obtaining the known crystal structure (i.e., CIF) of Bet (BETANC01) from the CSD. In step 2, a DFT-D2* geometry optimization is conducted on a structural model derived from the CIF to refine the atomic coordinates and calculate the Hirshfeld charges. In step 3, the Hirshfeld atomic charges are assigned to atoms (Scheme SA and Table S2†) on the organic cation and chloride anion. In step 4, the organic cation and chloride anion are assigned as motion groups.
In M2, the motion groups of Bet are subjected to N = 10 trials of the four steps of the polymorph prediction routine, which generates 49
451 structures (Table 3). The first set of benchmarked metrics are applied at this point, eliminating the majority of structural candidates; the 474 structural candidates that are retained (ca. 1% of the total) are passed to M3.
In M3 steps 1–3 (Fig. 2), DFT-D2* geometry optimizations and increasingly stringent metrics are used to retain the best candidate structures, progressing from 474 → 58 → 28 → 16 (Table 3). In M3 step 4, these final candidates are validated via their R and RMSDs, which range from 1.1% to 2.7% and 0.002 to 0.119 Å, respectively. We note that R ≤ 3%, in line with R reported for numerous structures deposited in the CSD, in which ca. 90% of structures have R ≤ 10%.171–174 Two of the sixteen best structural candidates for Bet, 9-632 and 10-103 (i.e., the 632nd and 103rd structure generated during the polymorph prediction routine from trials 9 and 10), are compared to the reported structure refined at the DFT-D2* level in Fig. 3. The final ΓEFG, Elat, R, and RMSD values for the validated structures are in Table 4.
Table 4 Validated structural models of betaine HCl from the benchmarking of the QNMRX-CSP protocol in stage 1
Structural modela |
Γ
EFG (MHz)b |
E
lat (kJ mol−1)c |
R (%)d |
RMSD (Å)e |
The structural model notation is defined as the trial number-structure number.
Γ
EFG is the EFG distance; see §2.5 and eqn (2) and (3) for further information.
E
lat is the static lattice energy of the structural model, normalized to that of the lowest energy structure, which is assigned a value of Elat = 0 kJ mol−1.
R is the R-factor, R = ∑|Fo − Fc|/∑|Fo| × 100%.
RMSD is the root-mean squared distance, which is a measure of the distance between corresponding atomic positions and bond angles from the reported crystal structure and candidate structural model(s).
|
2-320 |
0.325 |
0.000 |
1.387 |
0.112 |
2-486 |
0.323 |
0.010 |
1.461 |
0.113 |
9-632 |
0.321 |
0.010 |
1.135 |
0.003 |
9-158 |
0.323 |
0.019 |
1.936 |
0.111 |
2-330 |
0.331 |
0.029 |
2.253 |
0.110 |
2-494 |
0.32 |
0.039 |
1.549 |
0.112 |
4-272 |
0.328 |
0.039 |
2.017 |
0.002 |
2-313 |
0.328 |
0.048 |
2.035 |
0.119 |
4-46 |
0.326 |
0.048 |
2.059 |
0.003 |
7-75 |
0.332 |
0.048 |
2.534 |
0.002 |
10-105 |
0.329 |
0.048 |
2.024 |
0.114 |
8-152 |
0.319 |
0.048 |
1.765 |
0.114 |
10-47 |
0.327 |
0.058 |
1.843 |
0.113 |
5-98 |
0.321 |
0.077 |
1.952 |
0.004 |
2-326 |
0.332 |
0.096 |
1.955 |
0.113 |
10-103 |
0.317 |
0.106 |
1.737 |
0.113 |
The QNMRX-CSP protocol in S1 was similarly applied to Gly, Amg, Ala, and Gua, with validated structural models shown in Fig. S1–S12.† All benchmarking calculations in stages 1, 2, and 3 resulted in successful predictions of the known crystal structure, with all culminating in the selection of valid structural models (Table 5), thereby demonstrating the robustness of QNMRX-CSP for these systems. Visual comparisons and structural validations via comparison of the RMSDs and R are shown in Fig. S13–S17 and Tables S10–S14,† respectively.
Table 5 The numbers of structures retained from the QNMRX-CSP protocol in S1, S2, and S3, as applied in benchmarking calculations on the five structural models
Structural model |
QNMRX-CSP protocol |
M2a |
M3b |
M3b |
M3b |
Step 1 |
Step 2 |
Step 3 |
Initial |
Selected |
Selected |
Selected |
Selected |
Benchmarked metrics for structure selection in M2 include ±20% a, b, c, α, β, and γ and Ecutoff ≤ 13.5%.
Benchmarked metrics for structure selection in M3 include ΓEFG ≤ 0.70 MHz and Elat ≤ 135 kJ mol−1 in step 1, ΓEFG ≤ 0.49 MHz and Elat ≤ 50 kJ mol−1 in step 2, and ΓEFG ≤ 0.49 MHz and Elat ≤ 1 kJ mol−1 in step 3.
|
Bet |
S1 |
49 451 |
474 |
51 |
28 |
16 |
S2 |
51 855 |
239 |
41 |
24 |
10 |
S3 |
59 323 |
276 |
40 |
25 |
12 |
Gly |
S1 |
55 172 |
70 |
9 |
4 |
2 |
S2 |
59 348 |
123 |
24 |
15 |
6 |
S3 |
102 860 |
70 |
21 |
3 |
1 |
Ala |
S1 |
42 440 |
305 |
63 |
22 |
10 |
S2 |
49 045 |
99 |
19 |
5 |
3 |
S3 |
38 427 |
259 |
55 |
17 |
4 |
Gua |
S1 |
50 496 |
386 |
81 |
41 |
19 |
S2 |
53 659 |
398 |
96 |
48 |
47 |
S3 |
43 937 |
149 |
19 |
6 |
6 |
Amg |
S1 |
61 414 |
334 |
63 |
23 |
3 |
S2 |
58 380 |
86 |
20 |
8 |
1 |
S3 |
104 280 |
84 |
21 |
7 |
1 |
Crucially, part of the benchmarking process also involved the exploration of structural models with only one of ΓEFG or Elat falling below their threshold values, in order to gauge the importance of having both of these metrics for structural retention. In numerous instances, structural models produced by M3 step 2 that had ΓEFG and/or Elat values above the thresholds were tested in M3 step 3 to see if convergence and agreement with experiment could be obtained. The vast majority of the structural models of this type failed on both of these counts (examples for Bet are given in Table S15 and Supplement S3†), confirming the importance of both ΓEFG or Elat for retaining the structural models that agree best with experiment.
3.5 QNMRX-CSP stage 4: blind tests
After thorough benchmarking, the QNMRX-CSP protocol was executed in blind tests (i.e., S4 calculations, Scheme 2, red arrows) on two structural models, Dmg and Met (Scheme 1). In the case of this work, the experimental scXRD structures were concealed by a third party, and we were provided with the following: (i) a molecular diagram, (ii) crystallographic information (i.e., space group and unit cell parameters), (iii) experimental EFG tensor parameters, and (iv) pXRD diffraction data.
N,N′-Dimethylglycine HCl.
In this first blind test involving Dmg, two individuals executed QNMRX-CSP in S4 (Scheme 2, red arrows) following identical procedures, with the goal of independently testing the protocol and to see if both runs converge to similar candidate structures. In M1, the motion groups generated by the individuals (Fig. S18A†) led to similar cationic conformers (denoted as Dmg1 and Dmg2). In M2, the motion groups of Dmg1 and Dmg2 were subjected to a total of N = 20 trials each of the polymorph prediction routine (steps 1–4), resulting in totals of 96
606 and 100
040 structures, respectively (Table 6). After applying the first set of benchmarked metrics, 204 and 421 candidate structures, respectively, are passed to M3. In M3, truncated and convergent DFT-D2* geometry optimizations and selection of the best candidate structures based on benchmarked metrics (steps 1–3, Table 6), both led to 2 candidate structures (for a total of 4) that were validated using R (stage 4, Table 7), which are effectively all identical to one another. QNMRX-CSP was successful in correctly predicting the crystal structure of Dmg in this first blind test Fig. 4, as evidenced by the RMSDs and R-factors that are well within the uncertainties acceptable for structural models obtained from Rietveld refinements, validation of crystal structures using pXRD data, and NMR crystallographic refinements.171–174
Table 6 The numbers of structures retained from the QNMRX-CSP protocol in stage 4 as applied in two independent blind tests for two starting conformers of N,N′-dimethylglycine HCl, Dmg1 and Dmg2 and metformin HCl, Met1 and Met2
Conformer |
QNMRX-CSP protocola |
Number of structures |
Initial |
|
Retained |
See the footnotes in Table 3 for definitions.
|
Dmg1 |
M2: ±20% a, b, c, α, β, γ; Ecutoff ≤ 13.5% |
96 606 |
→ |
204 |
M3 step 1: ΓEFG ≤ 0.70 MHz; Elat ≤ 135 kJ mol−1 |
204 |
→ |
31 |
M3 step 2: ΓEFG ≤ 0.49 MHz; Elat ≤ 50 kJ mol−1 |
31 |
→ |
4 |
M3 step 3: ΓEFG ≤ 0.49 MHz; Elat ≤ 1 kJ mol−1 |
4 |
→ |
2 |
Dmg2 |
M2: ±20% a, b, c, α, β, γ; Ecutoff ≤ 13.5% |
100 040 |
→ |
421 |
M3 step 1: ΓEFG ≤ 0.70 MHz; Elat ≤ 135 kJ mol−1 |
421 |
→ |
72 |
M3 step 2: ΓEFG ≤ 0.49 MHz; Elat ≤ 50 kJ mol−1 |
72 |
→ |
9 |
M3 step 3: ΓEFG ≤ 0.49 MHz; Elat ≤ 1 kJ mol−1 |
9 |
→ |
2 |
Met1 |
M2: ±20% a, b, c, α, β, γ; Ecutoff ≤ 13.5% |
54 278 |
→ |
175 |
M3 step 1: ΓEFG ≤ 0.70 MHz; Elat ≤ 135 kJ mol−1 |
175 |
→ |
26 |
M3 step 2: ΓEFG ≤ 0.49 MHz; Elat ≤ 50 kJ mol−1 |
26 |
→ |
5 |
M3 step 3: ΓEFG ≤ 0.49 MHz; Elat ≤ 1 kJ mol−1 |
5 |
→ |
0 |
Met2 |
M2: ±20% a, b, c, α, β, γ; Ecutoff ≤ 13.5% |
59 420 |
→ |
212 |
M3 step 1: ΓEFG ≤ 0.70 MHz; Elat ≤ 135 kJ mol−1 |
212 |
→ |
55 |
M3 step 2: ΓEFG ≤ 0.49 MHz; Elat ≤ 50 kJ mol−1 |
55 |
→ |
7 |
M3 step 3: ΓEFG ≤ 0.49 MHz; Elat ≤ 1 kJ mol−1 |
7 |
→ |
4 |
Table 7 Validated structural models of N,N′-dimethylglycine HCl and metformin HCl in two independent blind tests of the QNMRX-CSP protocol in stage 4
Conformer |
Structural modela |
Γ
EFG (MHz)b |
E
lat (kJ mol−1)c |
R (%)d |
RMSD (Å)e |
The structural model notation is defined as the trial number-structure number.
Γ
EFG is the EFG distance; see §2.2 and eqn (3) and (4) for further information.
E
lat is the static lattice energy of the structural model, normalized to that of the lowest energy structure, which is assigned a value of Elat = 0 kJ mol−1.
R is the R-factor, R = ∑|Fo − Fc|/∑|Fo| × 100%.
RMSD is the root-mean squared distance, which is a measure of the distance between corresponding atomic positions and bond angles from the reported crystal structure and candidate structural model(s).
|
Dmg1 |
17-199 |
0.116 |
0.056 |
1.189 |
0.013 |
Dmg1 |
18-730 |
0.083 |
0.015 |
0.655 |
0.007 |
Dmg2 |
11-1746 |
0.080 |
0 |
0.484 |
0.004 |
Dmg2 |
14-1079 |
0.090 |
0.059 |
0.197 |
0.015 |
Met2 |
7-14 |
0.067 |
0 |
0.086 |
0.007 |
Met2 |
8-24 |
0.056 |
0.019 |
1.075 |
0.009 |
Met2 |
1-15 |
0.060 |
0.019 |
1.42 |
0.011 |
Met2 |
3-19 |
0.059 |
0.067 |
0.962 |
0.007 |
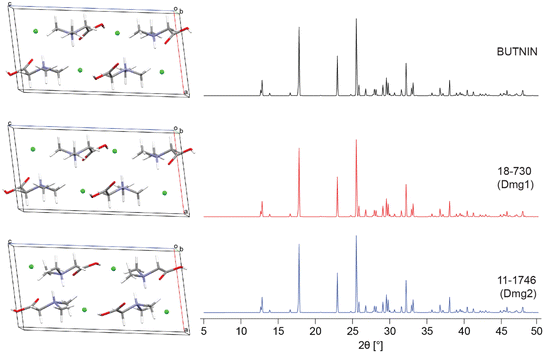 |
| Fig. 4 A comparison of the DFT-D2* geometry-optimized structural model of N,N′-dimethylglycine HCl derived from its known crystal structure (BUTNIN) with the validated structural models from S4 blind tests, 18-730 (Dmg1) and 11-1746 (Dmg2), with a ΓEFG = 0.083 and 0.080 MHz, Elat = 0.015 and 0 kJ mol−1, R = 0.655 and 0.484%, and RMSD = 0.007 and 0.004 Å, respectively. | |
Metformin HCl.
Two considerations were made prior to the application of the QNMRX-CSP protocol in S4 to Met. First, the protonation site is unclear, since there are two possibilities: a primary ketimine or secondary ketimine (Scheme 3). Hence, initial DFT-D2* gas-phase geometry optimizations were performed on both molecular fragments, where it was found that the primary ketimine structure is 50 kJ mol−1 lower in energy than the secondary ketimine; therefore, only the primary ketimine was subjected to QNMRX-CSP. Second, there are two possible low-energy conformers of the primary ketimine, Met1 and Met2; gas-phase geometry optimizations reveal that Met1 has an energy ca. 3 kJ mol−1 lower than that of Met2.
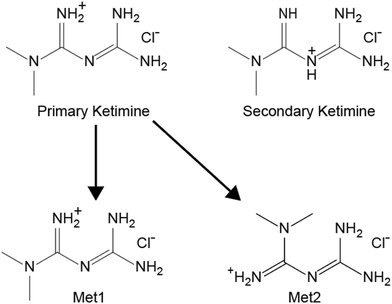 |
| Scheme 3 Molecular diagrams of the two protonation sites for metformin HCl (top), the primary ketimine (left) and the secondary ketimine (right). Gas phase calculations reveal the former to have an energy ca. 50 kJ mol−1 lower than that of the latter, only the two conformers of the primary ketimine (bottom) were subjected to the full QNMRX-CSP protocol in S4. | |
The remainder of the QNMRX-CSP S4 protocol was carried out using the two conformers of Met1 and Met2 as starting points. In M1 steps 3 and 4, the Hirshfeld charges were applied to the atoms and motion groups were selected (Fig. S18B†). In M2, 10 trials of the polymorph prediction routine were performed for each conformer, leading to 54
278 and 59
420 structural models, with benchmarked metrics yielding 175 and 212 structural candidates for Met1 and Met2, respectively. In M3, steps 1–3 reduced the total number of suitable candidate structures to 0 and 4 for Met1 and Met2, respectively (Table 6). The final structural candidates were given to a third party, who confirmed their validity. Two of the four validated structures are compared to the known structure in Fig. 5 and a summary of the final ΓEFG, Elat, R, and RMSD values are given in Table 7.
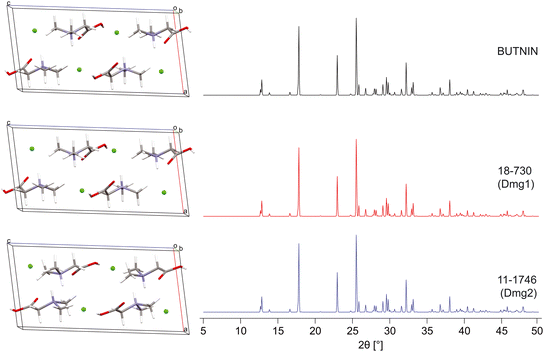 |
| Fig. 5 A comparison of the DFT-D2* geometry-optimized structural model of metformin HCl derived from its known crystal structure (JAMRIY01) with two (from the set of 4) validated structural models from S4 blind tests, 3-19 and 7-14 with a ΓEFG = 0.059 and 0.067 MHz, Elat = 0.067 and 0 kJ mol−1, R = 0.962 and 0.086%, and RMSD = 0.007 and 0.007 Å, respectively. | |
The two blind tests resulted in final structural models matching the reported crystal structures (with R ≤ 1.5% and RMSD ≤ 0.5 Å), demonstrating that QNMRX-CSP can yield structures comparable to those obtained from diffraction methods. This is very promising for future predictions of unknown crystal structures of HCl salts of pharmaceuticals and other organic molecules. A complete summary of the culmination of the results for both benchmarking across three Stages (i.e., S1, S2, and S3) and the two blind tests representing S4 that are discussed (vide supra) can be found in Table 8.
Table 8 Summary of key statistics during M2 and M3 and average of final metrics for validated benchmarked and blind tests structures for the QNMRX-CSP protocol
Compound |
Stage |
Number of trials (N) |
Initial number of structuresa |
Final number of structuresb |
Average ΓEFG (MHz) |
Average Elat (kJ mol−1) |
Average R (%) |
Average RMSD (Å) |
Number of structures after N trials of M2.
Number of structures after M3 step 3.
Total number of trials across Dmg1 and Dmg2 (see §3.5).
Sum of structures from Dmg1 and Dmg2 after N trials of M2 (see §3.5).
Total number of trials across Met1 and Met2 (see §3.5).
Sum of structures from Met1 and Met2 after N trials of M2 (see §3.5).
|
Bet |
1 |
10 |
49 451 |
16 |
0.325 |
0.045 |
1.853 |
0.079 |
2 |
10 |
51 855 |
10 |
0.328 |
0.033 |
1.643 |
0.057 |
3 |
10 |
59 323 |
12 |
0.326 |
0.037 |
1.693 |
0.085 |
Gly |
1 |
10 |
55 172 |
2 |
0.462 |
0.015 |
1.855 |
0.020 |
2 |
10 |
59 348 |
6 |
0.444 |
0.010 |
1.478 |
0.019 |
3 |
20 |
102 860 |
1 |
0.455 |
0.000 |
2.173 |
0.021 |
Ala |
1 |
10 |
42 440 |
10 |
0.200 |
0.022 |
1.175 |
0.010 |
2 |
10 |
49 045 |
3 |
0.202 |
0.015 |
1.689 |
0.013 |
3 |
10 |
38 427 |
4 |
0.224 |
0.131 |
9.10 |
0.065 |
Gua |
1 |
10 |
50 496 |
19 |
0.423 |
0.414 |
4.476 |
0.041 |
2 |
10 |
53 659 |
47 |
0.427 |
0.053 |
1.968 |
0.013 |
3 |
10 |
43 937 |
6 |
0.424 |
0.030 |
1.693 |
0.009 |
Amg |
1 |
10 |
61 414 |
3 |
0.304 |
0.003 |
1.156 |
0.006 |
2 |
10 |
58 380 |
1 |
0.301 |
0.000 |
1.060 |
0.006 |
3 |
20 |
104 280 |
1 |
0.317 |
0.000 |
1.271 |
0.009 |
Dmg |
4 |
40c |
196 646d |
4 |
0.093 |
0.033 |
0.631 |
0.010 |
Met |
4 |
20e |
113 698f |
4 |
0.061 |
0.026 |
0.886 |
0.009 |
4. Conclusions
We have designed a novel protocol, QNMRX-CSP, for the prediction of crystal structures that utilizes quadrupolar parameters determined from SSNMR data (i.e., 35Cl EFG tensors) and unit cell parameters obtained from diffraction methods. The QNMRX-CSP protocol was benchmarked using reported 35Cl EFG tensors and scXRD data from five HCl salts of small organic molecules, via a process of assigning motion groups, Monte Carlo simulated annealing for crystal packing, and DFT-D2* calculations for structural refinement. Key metrics for selecting the best candidate structures were determined from this process and applied in two blind tests, from which the structures of N,N′-dimethylglycine HCl and metformin HCl were predicted. For all systems, the QNMRX-CSP protocol predicted structural models that compare well with experiment, featuring average RMSDs < 0.2 Å and R < 10% (Table 8).171–174
The QNMRX-CSP protocol, in this nascent stage, has only targeted the use of 35Cl EFG tensor parameters and a limited set of structures; however, there are a plethora of interesting frontiers for its extension. First, it is possible that the protocol may function with other quadrupolar nuclei commonly found in APIs and other organic molecules, such as 14N and/or 17O, since calculations of their EFG tensors from structural models obtained from DFT-D2* geometry optimizations have shown excellent agreement with experiment.133–135 Unlike chloride ion sites, the oxygen and nitrogen atoms are almost always involved in covalent bonding schemes, which narrows the potential range of EFG tensors that can be investigated for different structural moieties; however, hydrogen bonding is known to dramatically impact these EFG tensors,99,111,131 suggesting possible use of 14N and/or 17O NMRX-CSP protocols as either a standalone or complementary methodology. Second, the two blind tests, along with ongoing work in our laboratory (beyond the scope of the current work), suggest that this protocol may be useful for the prediction of structures of increasing complexity (i.e., an increased number of motion groups and/or more torsional flexibility in the organic cations). Third, as the sizes of the organic cations are increased, it is possible that the QNMRX-CSP protocol, with EFG tensors alone, may not provide valid structural predictions; however, if the protocol is used in tandem with current NMRX-CSP methods (e.g., involving either the first principles calculations of chemical shifts or their prediction with machine learning algorithms like ShiftML),83–87 it is possible that valid structural models may be obtained in greater frequency, accuracy, rapidity than by the independent application of only one of these methods.
The QNMRX-CSP protocol, in its current form, is limited due to the reliance upon unit cell parameters determined from diffraction methods (this is especially noted in Module 2 where unit cell parameters are a metric, and in Module 3 step 3, where the unit cell parameters of the candidate structural models are adjusted to match those of the reported crystal structure). We believe as methods for dispersion corrections (e.g., DFT-D3*, DFT-D3/BJ)168–170 continue to develop and the treatment of unit cell expansion during geometry optimizations155 becomes more reliable, their implementation in software packages like CASTEP may be able to better predict the unit cell parameters, thereby reducing dependence on diffraction data. Another pathway for improving and expanding the QNMRX-CSP protocol is the implementation of crystal packing algorithms other than the Monte Carlo simulated annealing routine in Materials Studio/Polymorph; fortunately, there are several options available.175,176 This would also expand the use of the protocol to a wider range of researchers with access to different software packages. Furthermore, the calculation of anisotropic displacement parameters must also be a future objective, in order to provide estimates of uncertainties in the atomic positions, akin to those obtained from diffraction studies (preliminary work has already been reported from NMRX-CSP studies reliant upon chemical shifts).177,178
Finally, the QNMRX-CSP protocol was designed with three key points in mind: (1) the predicted and refined structures are obtained after extensive benchmarking, taking into account efficiency and reproducibility; (2) the modules, steps, stages, and metrics are carefully delineated, so that those who are interested in applying the protocol for solving unknown structures have a reliable path forward; and (3) the protocol may be of enormous value in situations where single-crystal structures are not available, since it may be possible to index pXRD data and obtain potential sets of unit cell parameters and space groups, which can be narrowed down using complementary NMR data and QNMRX methods, and ultimately be of use in aiding Rietveld analyses.
Data availability
The data sets supporting this article have been uploaded as part of the ESI.† The CASTEP data manager (CDM) is an executable application developed in-house to automate the execution and processing of truncated and converged DFT-D2* calculations on candidate structures generated through the polymorph prediction routine and is available on the web https://github.com/rschurko.
Author contributions
A. A. Peach, C. H. Fleischer III, K. Levin, and J. E. Sanchez participated in the execution of the QNMRX-CSP protocol on benchmarked systems. A. A. Peach and C. H. Fleischer III participated in the blind tests of the QNMRX-CSP protocol. K. Levin developed the CASTEP data manager used for queueing and running calculations in parallel. A. A. Peach, C. H. Fleischer III, K. Levin, S. T. Holmes, and R. W. Schurko contributed to the design of the QNMRX-CSP protocol. A. A. Peach, C. H. Fleischer III, S. T. Holmes, and R. W. Schurko contributed to the writing and editing of the manuscript.
Conflicts of interest
There are no conflicts to declare.
Acknowledgements
R. W. S. is grateful for research support from The Florida State University and the National High Magnetic Field Laboratory (NHMFL), which is funded by the National Science Foundation Cooperative Agreement (DMR-1644779, DMR-2128556) and by the State of Florida. This work was supported in part by the U.S. Department of Energy, Office of Science, Office of Basic Energy Sciences, under Award Number DE-SC0022310, covering expenses related to postdoctoral wages. We also thank Genentech and the Natural Sciences and Engineering Research Council of Canada (NSERC, RGPIN-2016_06642 Discovery Grant) for funding early stages of this research.
References
-
A. R. Oganov, Modern Methods of Crystal Structure Prediction, Wiley, Weinheim, Germany, 2010 Search PubMed.
-
Computational Materials Discovery, ed. A. R. Oganov, G. Saleh and A. G. Kvashnin, The Royal Society of Chemistry, Cambridge, 2018 Search PubMed.
- A. R. Oganov, C. J. Pickard, Q. Zhu and R. J. Needs, Structure prediction drives materials discovery, Nat. Rev. Mater., 2019, 4, 331–348 CrossRef.
-
Prediction and Calculation of Crystal Structures, ed. S. Atahan-Evrenk and A. Aspuru-Guzik, Springer International Publishing, Cham, 2014, vol. 345 Search PubMed.
- A. R. Oganov, Crystal structure prediction: reflections on present status and challenges, Faraday Discuss., 2018, 211, 643–660 RSC.
- D. H. Bowskill, I. J. Sugden, S. Konstantinopoulos, C. S. Adjiman and C. C. Pantelides, Crystal Structure Prediction Methods for Organic Molecules: State of the Art, Annu. Rev. Chem. Biomol. Eng., 2021, 12, 593–623 CrossRef CAS PubMed.
- S. L. Price, Control and prediction of the organic solid state: a challenge to theory and experiment, Proc. R. Soc. A, 2018, 474, 20180351 CrossRef PubMed.
- R. Taylor and P. A. Wood, A Million Crystal Structures: The Whole Is Greater than the Sum of Its Parts, Chem. Rev., 2019, 119, 9427–9477 CrossRef CAS PubMed.
- C. R. Groom, I. J. Bruno, M. P. Lightfoot and S. C. Ward, The Cambridge Structural Database, Acta Crystallogr., Sect. B: Struct. Sci., Cryst. Eng. Mater., 2016, 72, 171–179 CrossRef CAS PubMed.
- S. Gražulis, D. Chateigner, R. T. Downs, A. F. T. Yokochi, M. Quirós, L. Lutterotti, E. Manakova, J. Butkus, P. Moeck and A. Le Bail, Crystallography Open Database – an open-access collection of crystal structures, J. Appl. Crystallogr., 2009, 42, 726–729 CrossRef PubMed.
- A. Belsky, M. Hellenbrandt, V. L. Karen and P. Luksch, New developments in the Inorganic Crystal Structure Database (ICSD): accessibility in support of materials research and design, Acta Crystallogr., Sect. B: Struct. Sci., 2002, 58, 364–369 CrossRef PubMed.
- J. P. M. Lommerse, W. D. S. Motherwell, H. L. Ammon, J. D. Dunitz, A. Gavezzotti, D. W. M. Hofmann, F. J. J. Leusen, W. T. M. Mooij, S. L. Price, B. Schweizer, M. U. Schmidt, B. P. van Eijck, P. Verwer and D. E. Williams, A test of crystal structure prediction of small organic molecules, Acta Crystallogr., Sect. B: Struct. Sci., 2000, 56, 697–714 CrossRef CAS PubMed.
- W. D. S. Motherwell, H. L. Ammon, J. D. Dunitz, A. Dzyabchenko, P. Erk, A. Gavezzotti, D. W. M. Hofmann, F. J. J. Leusen, J. P. M. Lommerse, W. T. M. Mooij, S. L. Price, H. Scheraga, B. Schweizer, M. U. Schmidt, B. P. van Eijck, P. Verwer and D. E. Williams, Crystal structure prediction of small organic molecules: a second blind test, Acta Crystallogr., Sect. B: Struct. Sci., 2002, 58, 647–661 CrossRef PubMed.
- G. M. Day, W. D. S. S. Motherwell, H. L. Ammon, S. X. M. M. Boerrigter, R. G. Della Valle, E. Venuti, A. Dzyabchenko, J. D. Dunitz, B. Schweizer, B. P. van Eijck, P. Erk, J. C. Facelli, V. E. Bazterra, M. B. Ferraro, D. W. M. M. Hofmann, F. J. J. J. Leusen, C. Liang, C. C. Pantelides, P. G. Karamertzanis, S. L. Price, T. C. Lewis, H. Nowell, A. Torrisi, H. A. Scheraga, Y. A. Arnautova, M. U. Schmidt and P. Verwer, A third blind test of crystal structure prediction, Acta Crystallogr., Sect. B: Struct. Sci., 2005, 61, 511–527 CrossRef CAS PubMed.
- G. M. Day, T. G. Cooper, A. J. Cruz-Cabeza, K. E. Hejczyk, H. L. Ammon, S. X. M. Boerrigter, J. S. Tan, R. G. Della Valle, E. Venuti, J. Jose, S. R. Gadre, G. R. Desiraju, T. S. Thakur, B. P. van Eijck, J. C. Facelli, V. E. Bazterra, M. B. Ferraro, D. W. M. Hofmann, M. A. Neumann, F. J. J. Leusen, J. Kendrick, S. L. Price, A. J. Misquitta, P. G. Karamertzanis, G. W. A. Welch, H. A. Scheraga, Y. A. Arnautova, M. U. Schmidt, J. van de Streek, A. K. Wolf and B. Schweizer, Significant progress in predicting the crystal structures of small organic molecules – a report on the fourth blind test, Acta Crystallogr., Sect. B: Struct. Sci., 2009, 65, 107–125 CrossRef CAS PubMed.
- D. A. Bardwell, C. S. Adjiman, Y. A. Arnautova, E. Bartashevich, S. X. M. Boerrigter, D. E. Braun, A. J. Cruz-Cabeza, G. M. Day, R. G. Della Valle, G. R. Desiraju, B. P. van Eijck, J. C. Facelli, M. B. Ferraro, D. Grillo, M. Habgood, D. W. M. Hofmann, F. Hofmann, K. V. J. Jose, P. G. Karamertzanis, A. V. Kazantsev, J. Kendrick, L. N. Kuleshova, F. J. J. Leusen, A. V. Maleev, A. J. Misquitta, S. Mohamed, R. J. Needs, M. A. Neumann, D. Nikylov, A. M. Orendt, R. Pal, C. C. Pantelides, C. J. Pickard, L. S. Price, S. L. Price, H. A. Scheraga, J. van de Streek, T. S. Thakur, S. Tiwari, E. Venuti and I. K. Zhitkov, Towards crystal structure prediction of complex organic compounds – a report on the fifth blind test, Acta Crystallogr., Sect. B: Struct. Sci., 2011, 67, 535–551 CrossRef CAS PubMed.
- A. M. Reilly, R. I. Cooper, C. S. Adjiman, S. Bhattacharya, A. D. Boese, J. G. Brandenburg, P. J. Bygrave, R. Bylsma, J. E. Campbell, R. Car, D. H. Case, R. Chadha, J. C. Cole, K. Cosburn, H. M. Cuppen, F. Curtis, G. M. Day, R. A. DiStasio Jr, A. Dzyabchenko, B. P. van Eijck, D. M. Elking, J. A. van den Ende, J. C. Facelli, M. B. Ferraro, L. Fusti-Molnar, C.-A. Gatsiou, T. S. Gee, R. de Gelder, L. M. Ghiringhelli, H. Goto, S. Grimme, R. Guo, D. W. M. Hofmann, J. Hoja, R. K. Hylton, L. Iuzzolino, W. Jankiewicz, D. T. de Jong, J. Kendrick, N. J. J. de Klerk, H.-Y. Ko, L. N. Kuleshova, X. Li, S. Lohani, F. J. J. Leusen, A. M. Lund, J. Lv, Y. Ma, N. Marom, A. E. Masunov, P. McCabe, D. P. McMahon, H. Meekes, M. P. Metz, A. J. Misquitta, S. Mohamed, B. Monserrat, R. J. Needs, M. A. Neumann, J. Nyman, S. Obata, H. Oberhofer, A. R. Oganov, A. M. Orendt, G. I. Pagola, C. C. Pantelides, C. J. Pickard, R. Podeszwa, L. S. Price, S. L. Price, A. Pulido, M. G. Read, K. Reuter, E. Schneider, C. Schober, G. P. Shields, P. Singh, I. J. Sugden, K. Szalewicz, C. R. Taylor, A. Tkatchenko, M. E. Tuckerman, F. Vacarro, M. Vasileiadis, A. Vazquez-Mayagoitia, L. Vogt, Y. Wang, R. E. Watson, G. A. de Wijs, J. Yang, Q. Zhu and C. R. Groom, Report on the sixth blind test of organic crystal structure prediction methods, Acta Crystallogr., Sect. B: Struct. Sci., Cryst. Eng. Mater., 2016, 72, 439–459 CrossRef CAS.
- G. J. O. Beran, I. J. Sugden, C. Greenwell, D. H. Bowskill, C. C. Pantelides and C. S. Adjiman, How many more polymorphs of ROY remain undiscovered, Chem. Sci., 2022, 13, 1288–1297 RSC.
- S. L. Price, Predicting crystal structures of organic compounds, Chem. Soc. Rev., 2014, 43, 2098–2111 RSC.
- S. L. Price, Computational prediction of organic crystal structures and polymorphism, Int. Rev. Phys. Chem., 2008, 27, 541–568 Search PubMed.
- S. L. Price, Computed Crystal Energy Landscapes for Understanding and Predicting Organic Crystal Structures and Polymorphism, Acc. Chem. Res., 2009, 42, 117–126 CrossRef CAS PubMed.
- S. L. Price, Why don't we find more polymorphs?, Acta Crystallogr., Sect. B: Struct. Sci., Cryst. Eng. Mater., 2013, 69, 313–328 CrossRef CAS PubMed.
-
S. L. Price and J. G. Brandenburg, in Non-Covalent Interactions in Quantum Chemistry and Physics, ed. G. A. D. Alberto Otero de la Roza, Elsevier, Amsterdam, 2017, pp. 333–363 Search PubMed.
- S. L. Price, D. E. Braun and S. M. Reutzel-Edens, Can computed crystal energy landscapes help understand pharmaceutical solids?, Chem. Commun., 2016, 52, 7065–7077 RSC.
- S. Price, The computational prediction of pharmaceutical crystal structures and polymorphism, Adv. Drug Delivery Rev., 2004, 56, 301–319 CrossRef CAS.
- Y. A. Abramov, Virtual hydrate screening and coformer selection for improved relative humidity stability, CrystEngComm, 2015, 17, 5216–5224 RSC.
- J. Yuan, X. Liu, S. Wang, C. Chang, Q. Zeng, Z. Song, Y. Jin, Q. Zeng, G. Sun, S. Ruan, C. Greenwell and Y. A. Abramov, Virtual coformer screening by a combined machine learning and physics-based approach, CrystEngComm, 2021, 23, 6039–6044 RSC.
- I. J. Sugden, D. E. Braun, D. H. Bowskill, C. S. Adjiman and C. C. Pantelides, Efficient Screening of Coformers for Active Pharmaceutical Ingredient Cocrystallization, Cryst. Growth Des., 2022, 22, 4513–4527 CrossRef CAS.
- R. S. Payne, R. J. Roberts, R. C. Rowe and R. Docherty, Examples of successful crystal structure prediction: polymorphs of primidone and progesterone, Int. J. Pharm., 1999, 177, 231–245 CrossRef CAS PubMed.
- G. J. O. Beran, J. D. Hartman and Y. N. Heit, Predicting Molecular Crystal Properties from First Principles: Finite-Temperature Thermochemistry to NMR Crystallography, Acc. Chem. Res., 2016, 49, 2501–2508 CrossRef CAS PubMed.
- G. J. O. Beran, Modeling Polymorphic Molecular Crystals with Electronic Structure Theory, Chem. Rev., 2016, 116, 5567–5613 CrossRef CAS PubMed.
- J. Nyman and G. M. Day, Static and lattice vibrational energy differences between polymorphs, CrystEngComm, 2015, 17, 5154–5165 RSC.
- C. R. Taylor and G. M. Day, Evaluating the Energetic Driving Force for Cocrystal Formation, Cryst. Growth Des., 2018, 18, 892–904 CrossRef CAS.
- G. M. Day, J. Chisholm, N. Shan, W. D. S. Motherwell and W. Jones, An Assessment of Lattice Energy Minimization for the Prediction of Molecular Organic Crystal Structures, Cryst. Growth Des., 2004, 4, 1327–1340 CrossRef CAS.
- F. Taulelle, NMR crystallography: crystallochemical formula and space group selection, Solid State Sci., 2004, 6, 1053–1057 CrossRef CAS.
- K. D. M. Harris, NMR Crystallography as a Vital Tool in Assisting Crystal Structure Determination from Powder XRD Data, Crystals, 2022, 12, 1277 CrossRef CAS.
-
R. K. Harris, R. E. Wasylishen and M. J. Duer, NMR Crystallography, WILEY-VCH Verlag, Hoboken, New Jersey, 2009 Search PubMed.
- D. L. Bryce, NMR crystallography: structure and properties of materials from solid-state nuclear magnetic resonance observables, IUCrJ, 2017, 4, 350–359 CrossRef CAS PubMed.
- P. Hodgkinson, NMR crystallography of molecular organics, Prog. Nucl. Magn. Reson. Spectrosc., 2020, 118–119, 10–53 CrossRef CAS PubMed.
- C. Martineau, NMR crystallography: Applications to inorganic materials, Solid State Nucl. Magn. Reson., 2014, 63–64, 1–12 CrossRef CAS PubMed.
- S. E. Ashbrook and D. McKay, Combining solid-state NMR spectroscopy with first-principles calculations – a guide to NMR crystallography, Chem. Commun., 2016, 52, 7186–7204 RSC.
- R. K. Harris, NMR crystallography: the use of chemical shifts, Solid State Sci., 2004, 6, 1025–1037 CrossRef CAS.
- C. M. Widdifield, S. O. Nilsson Lill, A. Broo, M. Lindkvist, A. Pettersen, A. Svensk Ankarberg, P. Aldred, S. Schantz and L. Emsley, Does Z′ equal 1 or 2? Enhanced powder NMR crystallography verification of a disordered room temperature crystal structure of a p38 inhibitor for chronic obstructive pulmonary disease, Phys. Chem. Chem. Phys., 2017, 19, 16650–16661 RSC.
-
F. Taulelle, in Encyclopedia of Magnetic Resonance, ed. R. K. Harris, R. E. Wasylishen and M. J. Duer, John Wiley & Sons, Ltd, Chichester, UK, 2009, pp. 1–14 Search PubMed.
- E. Salager, R. S. Stein, C. J. Pickard, B. Elena and L. Emsley, Powder NMR crystallography of thymol, Phys. Chem. Chem. Phys., 2009, 11, 2610 RSC.
- B. Elena, G. Pintacuda, N. Mifsud and L. Emsley, Molecular Structure Determination in Powders by NMR Crystallography from Proton Spin Diffusion, J. Am. Chem. Soc., 2006, 128, 9555–9560 CrossRef CAS PubMed.
- B. Elena and L. Emsley, Powder Crystallography by Proton Solid-State NMR Spectroscopy, J. Am. Chem. Soc., 2005, 127, 9140–9146 CrossRef CAS PubMed.
- E. Salager, G. M. Day, R. S. Stein, C. J. Pickard, B. Elena and L. Emsley, Powder Crystallography by Combined Crystal Structure Prediction and High-Resolution 1 H Solid-State NMR Spectroscopy, J. Am. Chem. Soc., 2010, 132, 2564–2566 CrossRef CAS PubMed.
- M. Baias, J.-N. Dumez, P. H. Svensson, S. Schantz, G. M. Day and L. Emsley, De Novo Determination of the Crystal Structure of a Large Drug Molecule by Crystal Structure Prediction-Based Powder NMR Crystallography, J. Am. Chem. Soc., 2013, 135, 17501–17507 CrossRef CAS PubMed.
- M. Baias, C. M. Widdifield, J.-N. N. Dumez, H. P. G. G. Thompson, T. G. Cooper, E. Salager, S. Bassil, R. S. Stein, A. Lesage, G. M. Day and L. Emsley, Powder crystallography of pharmaceutical materials by combined crystal structure prediction and solid-state 1H NMR spectroscopy, Phys. Chem. Chem. Phys., 2013, 15, 8069–8080 RSC.
- D. H. Brouwer and J. Van Huizen, NMR crystallography of zeolites: How far can we go without diffraction data?, Magn. Reson. Chem., 2019, 57, 167–175 CrossRef CAS PubMed.
- D. H. Brouwer and M. Horvath, A simulated annealing approach for solving zeolite crystal structures from two-dimensional NMR correlation spectra, Solid State Nucl. Magn. Reson., 2015, 65, 89–98 CrossRef CAS PubMed.
- D. H. Brouwer, S. Cadars, J. Eckert, Z. Liu, O. Terasaki and B. F. Chmelka, A general protocol for determining the structures of molecularly ordered but noncrystalline silicate frameworks, J. Am. Chem. Soc., 2013, 135, 5641–5655 CrossRef CAS PubMed.
- A. S. Tatton, H. Blade, S. P. Brown, P. Hodgkinson, L. P. Hughes, S. O. N. N. Lill and J. R. Yates, Improving Confidence in Crystal Structure Solutions Using NMR Crystallography: The Case of β-Piroxicam, Cryst. Growth Des., 2018, 18, 3339–3351 CrossRef CAS.
- J. Czernek, M. Urbanova and J. Brus, NMR Crystallography of the Polymorphs of Metergoline, Crystals, 2018, 8, 378 CrossRef.
- J. Struppe, C. M. Quinn, S. Sarkar, A. M. Gronenborn and T. Polenova, Ultrafast 1 H MAS NMR Crystallography for Natural Abundance Pharmaceutical Compounds, Mol. Pharmaceutics, 2020, 17, 674–682 CAS.
- M. K. Dudek, P. Paluch, J. Śniechowska, K. P. Nartowski, G. M. Day and M. J. Potrzebowski, Crystal structure determination of an elusive methanol solvate – hydrate of catechin using crystal structure prediction and NMR crystallography, CrystEngComm, 2020, 22, 4969–4981 RSC.
- M. K. Dudek, P. Paluch and E. Pindelska, Crystal structures of two furazidin polymorphs revealed by a joint effort of crystal structure prediction and NMR crystallography, Acta Crystallogr., Sect. B: Struct. Sci., Cryst. Eng. Mater., 2020, 76, 322–335 CrossRef CAS PubMed.
- F. Bravetti, R. E. Russo, S. Bordignon, A. Gallo, F. Rossi, C. Nervi, R. Gobetto and M. R. Chierotti, Zwitterionic or Not? Fast and Reliable Structure Determination by Combining Crystal Structure Prediction and Solid-State NMR, Molecules, 2023, 28, 1876 CrossRef CAS PubMed.
- F. Bravetti, S. Bordignon, E. Alig, D. Eisenbeil, L. Fink, C. Nervi, R. Gobetto, M. U. Schmidt and M. R. Chierotti, Solid-State NMR-Driven Crystal Structure Prediction of Molecular Crystals: The Case of Mebendazole, Chem. – Eur. J., 2022, 28, e202103589 CrossRef CAS PubMed.
- J. K. Harper and D. M. Grant, Enhancing Crystal-Structure Prediction with NMR Tensor Data, Cryst. Growth Des., 2006, 6, 2315–2321 CrossRef CAS.
- K. Kalakewich, R. Iuliucci and J. K. Harper, Establishing Accurate High-Resolution Crystal Structures in the Absence of Diffraction Data and Single Crystals—An NMR Approach, Cryst. Growth Des., 2013, 13, 5391–5396 CrossRef CAS.
- J. K. Harper, D. H. Barich, J. Z. Hu, G. A. Strobel and D. M. Grant, Stereochemical Analysis by Solid-State NMR: Structural Predictions in Ambuic Acid, J. Org. Chem., 2003, 68, 4609–4614 CrossRef CAS PubMed.
- S. T. Holmes, R. J. Iuliucci, K. T. Mueller and C. Dybowski, Critical Analysis of Cluster Models and Exchange-Correlation Functionals for Calculating Magnetic Shielding in Molecular Solids, J. Chem. Theory Comput., 2015, 11, 5229–5241 CrossRef CAS PubMed.
- S. T. Holmes, O. G. Engl, M. N. Srnec, J. D. Madura, R. Quiñones, J. K. Harper, R. W. Schurko and R. J. Iuliucci, Chemical Shift Tensors of Cimetidine Form A Modeled with Density Functional Theory Calculations: Implications for NMR Crystallography, J. Phys. Chem. A, 2020, 124, 3109–3119 CrossRef CAS PubMed.
- J. D. Hartman, R. A. Kudla, G. M. Day, L. J. Mueller and G. J. O. Beran, Benchmark fragment-based 1H, 13C, 15N and 17O chemical shift predictions in molecular crystals, Phys. Chem. Chem. Phys., 2016, 18, 21686–21709 RSC.
- J. D. Hartman, A. Balaji and G. J. O. Beran, Improved Electrostatic Embedding for Fragment-Based Chemical Shift Calculations in Molecular Crystals, J. Chem. Theory Comput., 2017, 13, 6043–6051 CrossRef CAS PubMed.
- J. L. McKinley and G. J. O. Beran, Improving Predicted Nuclear Magnetic Resonance Chemical Shifts Using the Quasi-Harmonic Approximation, J. Chem. Theory Comput., 2019, 15, 5259–5274 CrossRef CAS PubMed.
- D. Zhao, X. Shen, Z. Cheng, W. Li, H. Dong and S. Li, Accurate and Efficient Prediction of NMR Parameters of Condensed-Phase Systems with the Generalized Energy-Based Fragmentation Method, J. Chem. Theory Comput., 2020, 16, 2995–3005 CrossRef CAS PubMed.
- P. A. Unzueta and G. J. O. Beran, Polarizable continuum models provide an effective electrostatic embedding model for fragment-based chemical shift prediction in challenging systems, J. Comput. Chem., 2020, 41, 2251–2265 CrossRef CAS PubMed.
- C. Poidevin, G. L. Stoychev, C. Riplinger and A. A. Auer, High Level Electronic Structure Calculation of Molecular Solid-State NMR Shielding Constants, J. Chem. Theory Comput., 2022, 18, 2408–2417 CrossRef CAS PubMed.
- J. R. Yates, C. J. Pickard and F. Mauri, Calculation of NMR chemical shifts for extended systems using ultrasoft pseudopotentials, Phys. Rev. B: Condens. Matter Mater. Phys., 2007, 76, 1–11 CrossRef.
- C. M. Widdifield, J. D. Farrell, J. C. Cole, J. A. K. K. Howard and P. Hodgkinson, Resolving alternative organic crystal structures using density functional theory and NMR chemical shifts, Chem. Sci., 2020, 11, 2987–2992 RSC.
- L. Wang and J. K. Harper, Refining crystal structures using 13 C NMR chemical shift tensors as a target function, CrystEngComm, 2021, 23, 7061–7071 RSC.
- J. K. Harper, R. Iuliucci, M. Gruber and K. Kalakewich, Refining crystal structures with experimental 13C NMR shift tensors and lattice-including electronic structure methods, CrystEngComm, 2013, 15, 8693 RSC.
- K. Kalakewich, R. Iuliucci, K. T. Mueller, H. Eloranta and J. K. Harper, Monitoring the refinement of crystal structures with 15N solid-state NMR shift tensor data, J. Chem. Phys., 2015, 143, 194702 CrossRef PubMed.
- J. K. Harper, A. E. Mulgrew, J. Y. Li, D. H. Barich, G. A. Strobel and D. M. Grant, Characterization of Stereochemistry and Molecular Conformation Using Solid-State NMR Tensors, J. Am. Chem. Soc., 2001, 123, 9837–9842 CrossRef CAS PubMed.
- S. E. Soss, P. F. Flynn, R. J. Iuliucci, R. P. Young, L. J. Mueller, J. Hartman, G. J. O. Beran and J. K. Harper, Measuring and Modeling Highly Accurate 15 N Chemical Shift Tensors in a Peptide, ChemPhysChem, 2017, 18, 2225–2232 CrossRef CAS PubMed.
- S. T. Holmes, R. J. Iuliucci, K. T. Mueller and C. Dybowski, Density functional investigation of intermolecular effects on 13C NMR chemical-shielding tensors modeled with molecular clusters, J. Chem. Phys., 2014, 141, 164121 CrossRef PubMed.
- C. J. Pickard and F. Mauri, All-electron magnetic response with pseudopotentials: NMR chemical shifts, Phys. Rev. B: Condens. Matter Mater. Phys., 2001, 63, 245101 CrossRef.
- J. R. Yates, C. J. Pickard, M. C. Payne and F. Mauri, Relativistic nuclear magnetic resonance chemical shifts of heavy nuclei with pseudopotentials and the zeroth-order regular approximation, J. Chem. Phys., 2003, 118, 5746–5753 CrossRef CAS.
- C. Bonhomme, C. Gervais, F. Babonneau, C. Coelho, F. Pourpoint, T. Azaïs, S. E. Ashbrook, J. M. Griffin, J. R. Yates, F. Mauri and C. J. Pickard, First-Principles Calculation of NMR Parameters Using the Gauge Including Projector Augmented Wave Method: A Chemist's Point of View, Chem. Rev., 2012, 112, 5733–5779 CrossRef CAS PubMed.
- M. Cordova, M. Balodis, A. Hofstetter, F. Paruzzo, S. O. Nilsson Lill, E. S. E. Eriksson, P. Berruyer, B. Simões de Almeida, M. J. Quayle, S. T. Norberg, A. Svensk Ankarberg, S. Schantz and L. Emsley, Structure determination of an amorphous drug through large-scale NMR predictions, Nat. Commun., 2021, 12, 2964 CrossRef CAS PubMed.
- M. Balodis, M. Cordova, A. Hofstetter, G. M. Day and L. Emsley, De Novo Crystal Structure Determination from Machine Learned Chemical Shifts, J. Am. Chem. Soc., 2022, 144, 7215–7223 CrossRef CAS PubMed.
- F. M. Paruzzo, A. Hofstetter, F. Musil, S. De, M. Ceriotti and L. Emsley, Chemical shifts in molecular solids by machine learning, Nat. Commun., 2018, 9, 4501 CrossRef PubMed.
- F. Musil, M. J. Willatt, M. A. Langovoy and M. Ceriotti, Fast and Accurate Uncertainty Estimation in Chemical Machine Learning, J. Chem. Theory Comput., 2019, 15, 906–915 CrossRef CAS PubMed.
- M. Cordova, E. A. Engel, A. Stefaniuk, F. Paruzzo, A. Hofstetter, M. Ceriotti and L. Emsley, A Machine Learning Model of Chemical Shifts for Chemically and Structurally Diverse Molecular Solids, J. Phys. Chem. C, 2022, 126, 16710–16720 CrossRef CAS PubMed.
- J. B. Kleine Büning and S. Grimme, Computation of CCSD(T)-Quality NMR Chemical Shifts via Δ-Machine Learning from DFT, J. Chem. Theory Comput., 2023, 19, 3601–3615 CrossRef PubMed.
- S. E. Ashbrook, D. M. Dawson, Z. Gan, J. E. Hooper, I. Hung, L. E. Macfarlane, D. McKay, L. K. McLeod and R. I. Walton, Application of NMR Crystallography to Highly Disordered Templated Materials: Extensive Local Structural Disorder in the Gallophosphate GaPO-34A, Inorg. Chem., 2020, 59, 11616–11626 CrossRef CAS PubMed.
- F. A. Perras and D. L. Bryce, Multinuclear Magnetic Resonance Crystallographic Structure Refinement and Cross-Validation Using Experimental and Computed Electric Field Gradients: Application to Na 2 Al 2 B 2 O 7, J. Phys. Chem. C, 2012, 116, 19472–19482 CrossRef CAS.
- C. Leroy, T.-X. Métro, I. Hung, Z. Gan, C. Gervais and D. Laurencin, From Operando Raman Mechanochemistry to “NMR Crystallography”: Understanding the Structures and Interconversion of Zn-Terephthalate Networks Using Selective 17 O-Labeling, Chem. Mater., 2022, 34, 2292–2312 CrossRef CAS PubMed.
- E. Papulovskiy, S. D. Kirik, D. F. Khabibulin, A. A. Shubin, V. M. Bondareva, A. S. Samoilo and O. B. Lapina, Condensation of ammonium niobium oxalate studied by NMR crystallography and X-ray powder diffraction, Catal. Today, 2020, 354, 26–35 CrossRef CAS.
- D. Carnevale, M. Hollenstein and G. Bodenhausen, Self-Assembly of DNA and RNA Building Blocks Explored by Nitrogen-14 NMR Crystallography: Structure and Dynamics, ChemPhysChem, 2020, 21, 1044–1051 CrossRef CAS PubMed.
- C. M. Widdifield, F. A. Perras and D. L. Bryce, Solid-state 185/187 Re NMR and GIPAW DFT study of perrhenates and Re 2 (CO) 10 : chemical shift anisotropy, NMR crystallography, and a metal–metal bond, Phys. Chem. Chem. Phys., 2015, 17, 10118–10134 RSC.
- C. P. Romao, F. A. Perras, U. Werner-Zwanziger, J. A. Lussier, K. J. Miller, C. M. Calahoo, J. W. Zwanziger, M. Bieringer, B. A. Marinkovic, D. L. Bryce and M. A. White, Zero Thermal Expansion in ZrMgMo 3 O 12 : NMR Crystallography Reveals Origins of Thermoelastic Properties, Chem. Mater., 2015, 27, 2633–2646 CrossRef CAS.
- D. M. Dawson, L. E. Macfarlane, M. Amri, R. I. Walton and S. E. Ashbrook, Thermal Dehydrofluorination of GaPO-34 Revealed by NMR Crystallography, J. Phys. Chem. C, 2021, 125, 2537–2545 CrossRef CAS.
- F. A. Perras, H. Thomas, P. Heintz, R. Behera, J. Yu, G. Viswanathan, D. Jing, S. A. Southern, K. Kovnir, L. Stanley and W. Huang, The Structure of Boron Monoxide, J. Am. Chem. Soc., 2023, 145, 14660–14669 CrossRef CAS PubMed.
- C. Huang, M. Mutailipu, F. Zhang, K. J. Griffith, C. Hu, Z. Yang, J. M. Griffin, K. R. Poeppelmeier and S. Pan, Expanding the chemistry of borates with functional [BO2]− anions, Nat. Commun., 2021, 12, 2597 CrossRef CAS PubMed.
- J. Shen, V. Terskikh, J. Struppe, A. Hassan, M. Monette, I. Hung, Z. Gan, A. Brinkmann and G. Wu, Solid-state 17 O NMR study of α- d -glucose: exploring new frontiers in isotopic labeling, sensitivity enhancement, and NMR crystallography, Chem. Sci., 2022, 13, 2591–2603 RSC.
- S. T. Holmes, W. D. Wang, G. Hou, C. Dybowski, W. Wang and S. Bai, A new NMR crystallographic approach to reveal the calcium local structure of atorvastatin calcium, Phys. Chem. Chem. Phys., 2019, 21, 6319–6326 RSC.
- S. Radhakrishnan, K. Lauwers, C. V. Chandran, J. Trébosc, S. Pulinthanathu Sree, J. A. Martens, F. Taulelle, C. E. A. Kirschhock and E. Breynaert, NMR Crystallography Reveals Carbonate Induced Al-Ordering in ZnAl Layered Double Hydroxide, Chem. – Eur. J., 2021, 27, 15944–15953 CrossRef CAS PubMed.
- B. Bouchevreau, C. Martineau, C. Mellot-Draznieks, A. Tuel, M. R. Suchomel, J. Trébosc, O. Lafon, J. Amoureux and F. Taulelle, An NMR-Driven Crystallography Strategy to Overcome the Computability Limit of Powder Structure Determination: A Layered Aluminophosphate Case, Chem. – Eur. J., 2013, 19, 5009–5013 CrossRef CAS PubMed.
- S. Sene, B. Bouchevreau, C. Martineau, C. Gervais, C. Bonhomme, P. Gaveau, F. Mauri, S. Bégu, P. H. Mutin, M. E. Smith and D. Laurencin, Structural study of calcium phosphonates: a combined synchrotron powder diffraction, solid-state NMR and first-principle calculations approach, CrystEngComm, 2013, 15, 8763 RSC.
- K. E. Johnston, J. M. Griffin, R. I. Walton, D. M. Dawson, P. Lightfoot and S. E. Ashbrook, 93Nb NMR and DFT investigation of the polymorphs of NaNbO3, Phys. Chem. Chem. Phys., 2011, 13, 7565 RSC.
- C. M. Rice, Z. H. Davis, D. McKay, G. P. M. M. Bignami, R. G. Chitac, D. M. Dawson, R. E. Morris and S. E. Ashbrook, Following the unusual breathing behaviour of 17 O-enriched mixed-metal (Al,Ga)-MIL-53 using NMR crystallography, Phys. Chem. Chem. Phys., 2020, 22, 14514–14526 RSC.
- C. Martineau, A. Cadiau, B. Bouchevreau, J. Senker, F. Taulelle and K. Adil, SMARTER crystallography of the fluorinated inorganic–organic compound Zn3Al2F12·[HAmTAZ]6, Dalton Trans., 2012, 41, 6232 RSC.
- P. Klein, J. Dedecek, H. M. Thomas, S. R. Whittleton, J. Klimes, J. Brus, L. Kobera, D. L. Bryce and S. Sklenak, NMR Crystallography of Monovalent Cations in Inorganic Matrices: Na + Siting and the Local Structure of Na + Sites in Ferrierites, J. Phys. Chem. C, 2022, 126, 10686–10702 CrossRef CAS.
- C. Martineau-Corcos, NMR Crystallography: A tool for the characterization of microporous hybrid solids, Curr. Opin. Colloid Interface Sci., 2018, 33, 35–43 CrossRef CAS.
- T. Whewell, V. R. Seymour, K. Griffiths, N. R. Halcovitch, A. V. Desai, R. E. Morris, A. R. Armstrong and J. M. Griffin, A structural investigation of organic battery anode materials by NMR crystallography, Magn. Reson. Chem., 2022, 60, 489–503 CrossRef CAS PubMed.
- K. M. N. Burgess, Y. Xu, M. C. Leclerc and D. L. Bryce, Alkaline-earth metal carboxylates characterized by 43Ca and 87Sr solid-state NMR: Impact of metal-amine bonding, Inorg. Chem., 2014, 53, 552–561 CrossRef CAS PubMed.
- H. Hamaed, J. M. Pawlowski, B. F. T. T. Cooper, R. Fu, S. Holger, R. W. Schurko, S. H. Eichhorn, R. W. Schurko, S. Holger, R. W. Schurko, S. H. Eichhorn and R. W. Schurko, Application of Solid-State 35 Cl NMR to the Structural Characterization of Hydrochloride Pharmaceuticals and their Polymorphs, J. Am. Chem. Soc., 2008, 130, 11056–11065 CrossRef CAS PubMed.
- M. Hildebrand, H. Hamaed, A. M. Namespetra, J. M. Donohue, R. Fu, I. Hung, Z. Gan and R. W. Schurko, 35 Cl solid-state NMR of HCl salts of active pharmaceutical ingredients: structural prediction, spectral fingerprinting and polymorph recognition, CrystEngComm, 2014, 16, 7334–7356 RSC.
- C. M. Widdifield and D. L. Bryce, Solid-State 79/81 Br NMR and Gauge-Including Projector-Augmented Wave Study of Structure, Symmetry, and Hydration State in Alkaline Earth Metal Bromides, J. Phys. Chem. A, 2010, 114, 2102–2116 CrossRef CAS PubMed.
- D. L. Bryce, G. D. Sward and S. Adiga, Solid-State 35/37 Cl NMR Spectroscopy of Hydrochloride Salts of Amino Acids Implicated in Chloride Ion Transport Channel Selectivity: Opportunities at 900 MHz, J. Am. Chem. Soc., 2006, 128, 2121–2134 CrossRef CAS PubMed.
- D. L. Bryce, M. Gee and R. E. Wasylishen, High-Field Chlorine NMR Spectroscopy of Solid Organic Hydrochloride Salts: A Sensitive Probe of Hydrogen Bonding Environment, J. Phys. Chem. A, 2001, 105, 10413–10421 CrossRef CAS.
- Y. Kazuhiko, D. Shuan and G. Wu, Solid-state 17O NMR investigation of the carbonyl oxygen electric-field-gradient tensor and chemical shielding tensor in amides, J. Am. Chem. Soc., 2000, 122, 11602–11609 CrossRef.
- X. Kong, Y. Dai and G. Wu, Solid-state 17O NMR study of 2-acylbenzoic acids and warfarin, Solid State Nucl. Magn. Reson., 2017, 84, 59–64 CrossRef CAS PubMed.
- F. G. Vogt, G. R. Williams and R. C. B. Copley, Solid-State NMR Analysis of a Boron-Containing Pharmaceutical Hydrochloride Salt, J. Pharm. Sci., 2013, 102, 3705–3716 CrossRef CAS PubMed.
- R. P. Chapman and D. L. Bryce, A high-field solid-state 35/37Cl NMR and quantum chemical investigation of the chlorine quadrupolar and chemical shift tensors in amino acid hydrochlorides, Phys. Chem. Chem. Phys., 2007, 9, 6219–6230 RSC.
- F. G. Vogt, H. Yin, R. G. Forcino and L. Wu, 17O solid-state NMR as a sensitive probe of hydrogen bonding in crystalline and amorphous solid forms of diflunisal, Mol. Pharmaceutics, 2013, 10, 3433–3446 CrossRef CAS PubMed.
- E. Pindelska, A. Sokal and W. Kolodziejski, Pharmaceutical cocrystals, salts and polymorphs: Advanced characterization techniques, Adv. Drug Delivery Rev., 2017, 117, 111–146 CrossRef CAS PubMed.
- F. J. King, A. D. Searle and M. W. Urquhart, Ranitidine—Investigations into the Root Cause for the Presence of N-Nitroso- N, N -dimethylamine in Ranitidine Hydrochloride Drug Substances and Associated Drug Products, Org. Process Res. Dev., 2020, 24, 2915–2926 CrossRef CAS.
- R. K. Harris, NMR studies of organic polymorphs & solvates, Analyst, 2006, 131, 351 RSC.
- X. Kong, L. A. O'Dell, V. Terskikh, E. Ye, R. Wang and G. Wu, Variable-Temperature 17 O NMR Studies Allow Quantitative Evaluation of Molecular Dynamics in Organic Solids, J. Am. Chem. Soc., 2012, 134, 14609–14617 CrossRef CAS PubMed.
- P. M. J. Szell, J. R. Lewandowski, H. Blade, L. P. Hughes, S. O. Nilsson Lill and S. P. Brown, Taming the dynamics in a pharmaceutical by cocrystallization: investigating the impact of the coformer by solid-state NMR, CrystEngComm, 2021, 23, 6859–6870 RSC.
- I. Goldberga, N. Patris, C. H. Chen, E. Thomassot, J. Trébosc, I. Hung, Z. Gan, D. Berthomieu, T. X. Métro, C. Bonhomme, C. Gervais and D. Laurencin, First Direct Insight into the Local Environment and Dynamics of Water Molecules in the Whewellite Mineral Phase: Mechanochemical Isotopic Enrichment and High-Resolution 17O and 2H NMR Analyses, J. Phys. Chem. C, 2022, 126, 12044–12059 CrossRef CAS PubMed.
- C. Chen, I. Goldberga, P. Gaveau, S. Mittelette, J. Špačková, C. Mullen, I. Petit, T. Métro, B. Alonso, C. Gervais and D. Laurencin, Looking into the dynamics of molecular crystals of ibuprofen and terephthalic acid using 17 O and 2 H nuclear magnetic resonance analyses, Magn. Reson. Chem., 2021, 59, 975–990 CrossRef CAS PubMed.
- D. A. Hirsh, Y. Su, H. Nie, W. Xu, D. Stueber, N. Variankaval and R. W. Schurko, Quantifying Disproportionation in Pharmaceutical Formulations with 35 Cl Solid-State NMR, Mol. Pharmaceutics, 2018, 15, 4038–4048 CrossRef CAS PubMed.
- S. T. Holmes, J. M. Hook and R. W. Schurko, Nutraceuticals in Bulk and Dosage Forms: Analysis by 35 Cl and 14 N Solid-State NMR and DFT Calculations, Mol. Pharmaceutics, 2022, 19, 440–455 CrossRef CAS PubMed.
- A. M. Namespetra, D. A. Hirsh, M. P. Hildebrand, A. R. Sandre, H. Hamaed, J. M. Rawson and R. W. Schurko, 35 Cl solid-state NMR spectroscopy of HCl pharmaceuticals and their polymorphs in bulk and dosage forms, CrystEngComm, 2016, 18, 6213–6232 RSC.
- S. L. Veinberg, K. E. Johnston, M. J. Jaroszewicz, B. M. Kispal, C. R. Mireault, T. Kobayashi, M. Pruski and R. W. Schurko, Natural abundance14N and15N solid-state NMR of pharmaceuticals and their polymorphs, Phys. Chem. Chem. Phys., 2016, 18, 17713–17730 RSC.
- G. Wu, Solid-State 17O NMR studies of organic and biological molecules: Recent advances and future directions, Solid State Nucl. Magn. Reson., 2016, 73, 1–14 CrossRef CAS PubMed.
- S. T. Holmes and R. W. Schurko, Refining Crystal Structures with Quadrupolar NMR and Dispersion-Corrected Density Functional Theory, J. Phys. Chem. C, 2018, 122, 1809–1820 CrossRef CAS.
- S. T. Holmes, C. S. Vojvodin and R. W. Schurko, Dispersion-Corrected DFT Methods for Applications in Nuclear Magnetic Resonance Crystallography, J. Phys. Chem. A, 2020, 124, 10312–10323 CrossRef CAS PubMed.
- S. T. Holmes, R. J. Iuliucci, K. T. Mueller and C. Dybowski, Semi-empirical refinements of crystal structures using 17 O quadrupolar-coupling tensors, J. Chem. Phys., 2017, 146, 064201 CrossRef PubMed.
- G. S. Paulekuhn, J. B. Dressman and C. Saal, Trends in Active Pharmaceutical Ingredient Salt Selection based on Analysis of the Orange Book Database, J. Med. Chem., 2007, 50, 6665–6672 CrossRef CAS PubMed.
-
G. Desiraju and T. Steiner, The Weak Hydrogen Bond, Oxford University Press, Oxford, England, 2001, vol. 9 Search PubMed.
- T. Steiner, The Hydrogen Bond in the Solid State, Angew. Chem., Int. Ed., 2002, 41, 48–76 CrossRef CAS.
- T. Steiner, Hydrogen-Bond Distances to Halide Ions in Organic and Organometallic Crystal Structures: Up-to-date Database Study, Acta Crystallogr., Sect. B: Struct. Sci., 1998, 54, 456–463 CrossRef.
- S. J. Clark, M. D. Segall, C. J. Pickard, P. J. Hasnip, M. I. J. Probert, K. Refson and M. C. Payne, First principles methods using CASTEP, Z. Kristallogr. - Cryst. Mater., 2005, 220, 567–570 CrossRef CAS.
- S. L. Mayo, B. D. Olafson and W. A. Goddard, DREIDING: A generic force field for molecular simulations, J. Phys. Chem., 1990, 94, 8897–8909 CrossRef CAS.
- E. L. Willighagen, R. Wehrens, P. Verwer, R. De Gelder and L. M. C. Buydens, Method for the computational comparison of crystal structures, Acta Crystallogr., Sect. B: Struct. Sci., 2005, 61, 29–36 CrossRef CAS PubMed.
- S. Grimme, Semiempirical GGA-type density functional constructed with a long-range dispersion correction, J. Comput. Chem., 2006, 27, 1787–1799 CrossRef CAS PubMed.
- B. G. Pfrommer, M. Côté, S. G. Louie and M. L. Cohen, Relaxation of Crystals with the Quasi-Newton Method, J. Comput. Phys., 1997, 131, 233–240 CrossRef CAS.
- P. Pyykkö, Year-2017 nuclear quadrupole moments, Mol. Phys., 2018, 116, 1328–1338 CrossRef.
- C. F. MacRae, I. Sovago, S. J. Cottrell, P. T. A. Galek, P. McCabe, E. Pidcock, M. Platings, G. P. Shields, J. S. Stevens, M. Towler and P. A. Wood, Mercury 4.0: From visualization to analysis, design and prediction, J. Appl. Crystallogr., 2020, 53, 226–235 CrossRef CAS PubMed.
- J. A. Chisholm and S. Motherwell, COMPACK: A program for identifying crystal structure similarity using distances, J. Appl. Crystallogr., 2005, 38, 228–231 CrossRef.
- D. W. Alderman, M. H. Sherwood and D. M. Grant, Comparing, Modeling, and Assigning Chemical-Shift Tensors in the Cartesian, Irreducible Spherical, and Icosahedral Representations, J. Magn. Reson., Ser. A, 1993, 101, 188–197 CrossRef CAS.
- S. L. Childs, L. J. Chyall, J. T. Dunlap, D. A. Coates, B. C. Stahly and G. P. Stahly, A Metastable Polymorph of Metformin Hydrochloride: Isolation and Characterization Using Capillary Crystallization and Thermal Microscopy Techniques, Cryst. Growth Des., 2004, 4, 441–449 CrossRef CAS.
- W.-H. Yip, R.-J. Wang and T. C. W. Mak, Structure of a new form of betaine hydrochloride, Acta Crystallogr., Sect. C: Cryst. Struct. Commun., 1990, 46, 717–719 CrossRef.
- T. Steiner, Frequency of Z′ values in organic and organometallic crystal structures, Acta Crystallogr., Sect. B: Struct. Sci., 2000, 56, 673–676 CrossRef CAS PubMed.
- F. L. Hirshfeld, Bonded-atom fragments for describing molecular charge densities, Theor. Chim. Acta, 1977, 44, 129–138 CrossRef CAS.
- R. S. Mulliken, Electronic Population Analysis on LCAO–MO Molecular Wave Functions. I, J. Chem. Phys., 1955, 23, 1833–1840 CrossRef CAS.
-
F. J. J. Leusen and J. Kendrick, in Pharmaceutical Salts and Co-crystals, The Royal Society of Chemistry, 2011, pp. 44–88 Search PubMed.
- F. J. J. Leusen, Crystal Structure Prediction of Diastereomeric Salts: A Step toward Rationalization of Racemate Resolution, Cryst. Growth Des., 2003, 3, 189–192 CrossRef CAS.
- J. Kendrick, F. J. J. Leusen, M. A. Neumann and J. Van De Streek, Progress in crystal structure prediction, Chem. – Eur. J., 2011, 17, 10736–10744 CrossRef CAS PubMed.
- W. I. Cross, N. Blagden, R. J. Davey, R. G. Pritchard, M. A. Neumann, R. J. Roberts and R. C. Rowe, A Whole Output Strategy for Polymorph Screening, Cryst. Growth Des., 2003, 3, 151 CrossRef CAS.
- J. A. R. P. Sarma and G. R. Desiraju, The Supramolecular Synthon Approach to Crystal Structure Prediction, Cryst. Growth Des., 2002, 2, 93–100 CrossRef CAS.
- J. Park, C. W. Lee, S. H. Joo, J. H. Park, C. Hwang, H. K. Song, Y. S. Park, S. K. Kwak, S. Ahn and S. J. Kang, Contorted polycyclic aromatic hydrocarbon: Promising Li insertion organic anode, J. Mater. Chem. A, 2018, 6, 12589–12597 RSC.
- H. Sun, Compass: An ab initio force-field optimized for condensed-phase applications - Overview with details on alkane and benzene compounds, J. Phys. Chem. B, 1998, 102, 7338–7364 CrossRef CAS.
- H. Sun, Z. Jin, C. Yang, R. L. C. Akkermans, S. H. Robertson, N. A. Spenley, S. Miller and S. M. Todd, COMPASS II: extended coverage for polymer and drug-like molecule databases, J. Mol. Model., 2016, 22, 1–10 CrossRef PubMed.
- I. Fukuda and H. Nakamura, Non-Ewald methods: Theory and applications to molecular systems, Biophys. Rev., 2012, 4, 161–170 CrossRef CAS PubMed.
- N. Kamiya, I. Fukuda and H. Nakamura, Application of zero-dipole summation method to molecular dynamics simulations of a membrane protein system, Chem. Phys. Lett., 2013, 568–569, 26–32 CrossRef CAS.
- B. A. Wells and A. L. Chaffee, Ewald Summation for Molecular Simulations, J. Chem. Theory Comput., 2015, 11, 3684–3695 CrossRef CAS PubMed.
- C. P. Brock and J. D. Dunitz, Towards a Grammar of Crystal Packing, Chem. Mater., 1994, 6, 1118–1127 CrossRef CAS.
- G. A. Dolgonos, O. A. Loboda and A. D. Boese, Development of Embedded and Performance of Density Functional Methods for Molecular Crystals, J. Phys. Chem. A, 2018, 122, 708–713 CrossRef CAS PubMed.
- Y. N. Heit and G. J. O. Beran, How important is thermal expansion for predicting molecular crystal structures and thermochemistry at finite temperatures?, Acta Crystallogr., Sect. B: Struct. Sci., Cryst. Eng. Mater., 2016, 72, 514–529 CrossRef CAS PubMed.
- E. Caldeweyher, C. Bannwarth and S. Grimme, Extension of the D3 dispersion coefficient model, J. Chem. Phys., 2017, 147, 034112 CrossRef PubMed.
- S. Grimme, S. Ehrlich and L. Goerigk, Effect of the damping function in dispersion corrected density functional theory, J. Comput. Chem., 2011, 32, 1456–1465 CrossRef CAS PubMed.
- S. Grimme, J. Antony, S. Ehrlich and H. Krieg, A consistent and accurate ab initio parametrization of density functional dispersion correction (DFT-D) for the 94 elements H-Pu, J. Chem. Phys., 2010, 132, 154104 CrossRef PubMed.
-
D. E. McRee, in Practical Protein Crystallography, Elsevier, 2nd edn, 1999, vol. 3, pp. 91–269 Search PubMed.
-
J.-M. Rondeau and H. Schreuder, in The Practice of Medicinal Chemistry, Elsevier, 2015, pp. 511–537 Search PubMed.
- F. H. Allen, The Cambridge Structural Database: a quarter of a million crystal structures and rising, Acta Crystallogr., Sect. B: Struct. Sci., 2002, 58, 380–388 CrossRef PubMed.
-
G. Barr, W. Dong, C. J. Gilmore, A. Kern, A. Parkin and C. C. Wilson, Using the Cambridge Structural Database to validate powder structures, 2007, vol. 26 Search PubMed.
- M. A. Neumann, Tailor-Made Force Fields for Crystal-Structure Prediction, J. Phys. Chem. B, 2008, 112, 9810–9829 CrossRef CAS PubMed.
- M. Habgood, I. J. Sugden, A. V. Kazantsev, C. S. Adjiman and C. C. Pantelides, Efficient Handling of Molecular Flexibility in Ab Initio Generation of Crystal Structures, J. Chem. Theory Comput., 2015, 11, 1957–1969 CrossRef CAS PubMed.
- L. Wang, F. J. Uribe-Romo, L. J. Mueller and J. K. Harper, Predicting anisotropic thermal displacements for hydrogens from solid-state NMR: a study on hydrogen bonding in polymorphs of palmitic acid, Phys. Chem. Chem. Phys., 2018, 20, 8475–8487 RSC.
- A. Hofstetter and L. Emsley, Positional Variance in NMR Crystallography, J. Am. Chem. Soc., 2017, 139, 2573–2576 CrossRef CAS PubMed.
|
This journal is © The Royal Society of Chemistry 2024 |
Click here to see how this site uses Cookies. View our privacy policy here.