PASCAL: the perovskite automated spin coat assembly line accelerates composition screening in triple-halide perovskite alloys†
Received
13th March 2024
, Accepted 18th May 2024
First published on 22nd May 2024
Abstract
The Perovskite Automated Spin Coat Assembly Line – PASCAL – is introduced as a materials acceleration platform for the deposition and characterization of spin-coated thin films, with specific application to halide perovskites. We first demonstrate improved consistency of perovskite film fabrication by controlling process parameters, the influence of which is uniquely exposed under the automated experimental framework. Next, we report on an automated campaign of composition engineering to improve the durability of perovskite absorbers for tandem solar cell applications. We screen compositions spanning the triple-cation, triple-halide composition space, MAxFA0.78Cs0.22−xPb(I0.8−y−zBryClz)3. Data-driven clustering identifies four characteristic behaviors within this space regarding figures of merit for durability and open-circuit voltage, with data from each sample acquired automatically in PASCAL characterization line. Finally, a film composition durable to light and elevated temperature exposure is identified via a regression model trained on the high-throughput dataset. The approach, hardware, and data detailed herein highlight automated platforms as an opportunity to accelerate the identification and discovery of novel thin film materials and demonstrates the efficacy of PASCAL specifically for automation of solution-processed optoelectronic thin film research.
1 Introduction
The compositional flexibility of halide perovskites presents both opportunity for discovery of tailored materials for myriad optoelectronic applications and risk of superfluous efforts. Whether fine-tuning composition within known spaces, or searching in novel spaces (e.g., double perovskites,1–4 pseudocations/pseudohalides,5–9 new additives,10 chalcogenides11), the perovskite subfield and the optoelectronics community more broadly faces a number of hurdles in screening efforts. First is the enormity of design space. In the case of halide perovskites, nearly half12,13 of the periodic table can plausibly be incorporated into the structure, making an exhaustive search of the space intractable. Second is the sensitivity of the functional properties of optoelectronic materials to defects.14 In the particular case of halide perovskites, irreproducibility of the fabrication is also a substantial concern, owing in part to low (or even metastable15) formation energies and correspondingly a highly kinetically-driven synthesis process (e.g., spin coating with an antisolvent). These factors exacerbate the challenge in academic research and discovery efforts to achieve sample-to-sample, batch-to-batch, and interlaboratory experimental comparisons. In halide perovskites, even for the same operator,16 practices may deviate in seemingly small ways over time, e.g. the rate17 or timing18 of antisolvent dispensing during spin coating, leading to large changes in outcomes. Finally, the active material is only one component of the complete photovoltaic cell, and covariances between active material composition, interface passivation strategies, charge transport layers, and the process parameters associated with each step expand the scale of what is ideally a global optimization problem.
These risks inherent to bold compositional searches can be ameliorated by the use of automation.19 Robotic automation of halide perovskite experiments is growing in prevalence, improving experimental throughput and process precision.12,20–29 Such automation has been commonplace in drug discovery, facilitated by liquid handling hardware to execute solution-based experiments. Automation of thin film experiments is somewhat more difficult because solid samples require more advanced hardware to manipulate than liquids. Nevertheless, the significant benefits of materials automation12,30–33 have inspired pioneering efforts to automate spin coating platforms for thin film halide perovskite research.20,34–38 Furthermore, precision automation enables acceleration of materials discovery with machine learning by reducing noise that can slow learning. Already, the community has proven benefits of black-box models and physics informed models to facilitate materials screening39–41 and performance prediction.42–44
Halide perovskites hold particular promise for use in tandem solar cells, which may lower overall costs of solar electricity via a higher power conversion efficiency. Using a wide bandgap perovskite on silicon, researchers have achieved a power conversion efficiency of 33.9%,45 the record for any non-concentrator 2-terminal tandem solar cell.46 Perovskites are well positioned as candidates for a tandems because of their potential to have low production costs, tunable bandgaps, and sharp optical absorption edges.47–49 For perovskites to serve as a suitable two-terminal top-cell tandem partner with silicon, the bandgap must be tuned above 1.6 eV to allow for current matching between the top and bottom cell. Prior investigations50,51 have proved this challenging to achieve without sacrificing durability. It is challenging to maintain a microscopically single phase alloy perovskite when the bromine fraction is beyond 15–25% (depending on the A-site composition).52 At these wider bandgaps these perovskites typically suffer from phase segregation, leading to large open circuit voltage (VOC) deficits and rapid degradation of device performance.53 Thus, the community has sought a path to improved phase stability by tuning the perovskite primarily via alloying.54–60 Today, wide bandgap perovskites have made great strides in achieving improved stability, but still face phase instability when exposed to operational temperatures, illumination, and electrical biases.56,61–64
In this work, we report a robotic platform for spin coating and characterizing optoelectronic thin films. With a first focus on perovskites, we call the platform the Perovskite Automated Spin Coat Assembly Line (PASCAL). PASCAL automates fabrication of thin films by the standard spin coat procedures employed in manual processes, albeit with precision, control, and record keeping surpassing that of human operators. PASCAL additionally passes samples through a “characterization line”, generating a standard dataset of imaging (darkfield, brightfield, and photoluminescence intensity) and spectroscopy (transmittance and photoluminescence) for each sample. With PASCAL, we fabricate and measure samples at a rate of up to 430 depositions or measurements per day.
Using PASCAL, we first show reproducibility studies uncovering latent variables typically uncontrolled in the perovskite fabrication process and then, with controlled conditions, execute experimental screening and device prototyping of wide bandgap perovskite compositions. After validating reproducibility, as a proof of concept, we fabricate and test films of 58 unique compositions in the MAxFA0.78Cs0.22−xPb(I0.8−y−zBryClz)3 space with 4 repeats each in under 48 cumulative hours, evaluating the films on bandgap, photoluminescence (PL), and PL stability against intense illumination and thermal stress to establish the trade-offs made between these properties across the compositional space. From these results, we divide the compositional space into four distinct behavior regions using spectral clustering and identify a relatively superior composition in its spectral stability of PL emission through Gaussian process regression. Overall, we highlight the effectiveness of the robotic platform to design and validate absorber compositions for perovskites specifically and, more generally, thin films where functional properties or optima can be guided by optical characterization.
2 Experimental methods
2.1 Preparation of absorber stock solutions
For Sections 4.1.1 and 4.1.2 we used a Cs0.22FA0.78Pb(I0.85Br0.15)3 perovskite composition with 3% excess MAPbCl3. For Section 4.2, the case study on wide bandgap composition screening, 8 “endpoint” stock solutions were prepared by hand to reach a nominal composition of MAxFA0.78Cs0.22−xPb(I0.8−y−zBryClz)3 (Table 1). All solutions were prepared at 1.2 M in a 3
:
1 volume ratio of DMF
:
DMSO using MAI (GreatCell), FAI (GreatCell), CsI (Sigma, 99.999%), PbI2 (Sigma, 99.999%), PbBr2 (Sigma, 99.999%), and PbCl2 (Sigma, 99.999%). To produce individual compositions in the screening experiments, “endpoint” solutions were mixed in a polypropylene 96-well plate by an Opentrons OT2 liquid handler to achieve the target compositions for the screening experiments.
Table 1 Stock solutions to create MAxFA0.78Cs0.22−xPb(I0.8−y−zBryClz)3
x
|
y
|
z
|
0 |
0.1 |
0 |
0 |
0.2 |
0 |
0 |
0 |
0.1 |
0.1 |
0.1 |
0.1 |
0.1 |
0.1 |
0 |
0.1 |
0.2 |
0 |
0.1 |
0 |
0.1 |
0.1 |
0.1 |
0.1 |
For the target composition identified by the ML model and used in Section 4.3, the MA0.035FA0.78Cs0.185Pb(I0.863Br0.132Cl0.005)3 solution was made directly by hand.
All perovskite solutions contained 9% excess PbI2. Solutions were vortexed until no solids were visible (15–20 min), heated to 85 °C for 10 min, allowed to cool down to room temperature, then filtered through a 0.20 μm filter. All steps were performed within a nitrogen-filled glovebox (≤1 ppm O2 and H2O).
2.2 Film fabrication
1 × 1 cm glass slides were cleaned via sequential sonication steps each with a duration of 15 min in the following order: 5 v/v% Hellmanax (Sigma) in DI water, DI water, acetone, ethanol, and isopropyl alcohol. After drying with a filtered compressed dry air gun, the samples were treated by UV-ozone for 20 min under an O2 flow of 5 scfm. Upon completion, the substrates were immediately moved into the glovebox containing the robotic platform for film deposition.
Films were fabricated by PASCAL using spincoating inside a glovebox with constant N2 purge. Specifically, 40 μL of absorber solution were dispensed statically, followed by spinning at 3000 rpm for 50 s. 80 μL of methyl acetate were dispensed from 2 mm above the spinning substrate at a rate of 100 μL s−1 with 22 s remaining in the 3000 rpm spin step. After the spin completes, the sample is immediately moved to a hotplate and annealed at 100 °C for 30 min by the gantry robot arm, and is finally moved to an aluminum-floored sample storage tray to cool to room temperature.
2.3 Robot precision
Robot precision was characterized by analysis of real time records of processing steps from a typical 45-sample experiment. Spincoater RPM, dispense timing, and anneal duration are all recorded during normal PASCAL operation. The custom spincoater achieved an absolute variation over time within 1% (Fig. S1a†). The liquid handler achieved an antisolvent drop timing standard deviation of 18 ms (Fig. S1b†). The gantry-gripper module within PASCAL achieves an annealing duration standard deviation of 10 ms (Fig. S1c†).
2.4 Custom spincoater
A custom spincoater was built using a hollow-shaft brushless 1700 kV motor (iFlight) with a rotary encoder (ODrive Robotics) for precise position control. Detailed build instructions are available online at https://github.com/fenning-research-group/PASCAL.
2.5 Photoluminescence
Photoluminescence (PL) spectra are acquired using a 0.9 W 632 nm laser (ThorLabs) as an excitation source. Spectra are collected through a 200 μm slit grating 8 nm resolution spectrometer (StellarNet) and fit by a single Gaussian (cts vs. eV) to extract PL peak intensity, energy, and full-width half-max. All PL characterization is performed at least 3 min after the glass slide has moved onto an aluminum tray to allow for cooling after annealing. Once cooled, the sample is transferred to a linear stage, which carries the sample through a series of measurement stations.
2.6 Photostability
Series of photoluminescence spectra are acquired at 2 s intervals using a focused 0.9 W 450 nm laser (ThorLabs) as an excitation source for a 2 min duration. The focused power is equivalent to about 4 suns intensity. Individual spectra are fitted by a single Gaussian (cts vs. eV) to extract PL peak intensity, energy, and full-width half-max, and these extracted parameters are compared over time to evaluate film stability.
2.7 Thermal stability
A subset of the films are measured by PL spectroscopy before and after heating at 85 °C for 4.5 h on a hotplate in a nitrogen glovebox. Films are moved to the aluminum storage tray for at least 5 min before each measurement to cool to room temperature.
2.8 Scanning electron microscopy
SEM (Apreo) measurement was taken using an in-lens detector at an accelerating current of 0.1 nA and 5 kV.
2.9 X-ray-diffraction
XRD (Anton Paar) was conducted in a parallel beam scanning mode at an incident angle of 10° ω.
2.10 Photoluminescence quantum yield
1 sun equivalent intensity photoluminescence quantum yield (PLQY) measurements were carried out on a homebuilt setup consisting of 532 nm laser for excitation, using lock in detection at 993 Hz and a photodetector signal. PLQY was calculated using the method developed by de Mello et al.,65 with the responsivity of the Si photodetector accounted for using a photoluminescence spectrum of each sample and the responsivity of the detector. To calculate the implied voltage, the following relationship was used, adapted from de Mello et al.:65 | iVoc = Vlim + kT ln(PLQY) | (1) |
where iVoc is the 1-sun implied voltage, Vlim is the detailed balance limited voltage at 1-sun, and kT is the thermal voltage at room temperature. The detailed balance limit voltage was calculated using the optical bandgap estimated from transmission. Changes in implied voltages were calculated using the relative change in the uncalibrated PL intensity in PASCL spectroscopy module.
2.11 K-means spectral clustering
Scalarized features were fed into sklearn's KMC function66 with a manually set cluster count of 4 and radial basis function kernel. See data availability statement for further details.
2.12 Gaussian process regression
The Euclidean distance from zero peak shift on axes of both thermal degradation and photodegradation was defined as a figure of merit for PL energy stability. A Gaussian process regression was fit on this metric and the composition within the triple-halide space predicted to have the least shift was we extracted. The model utilized a single objective GP from Botorch.67 See data availability statement for further details.
3 PASCAL overview
PASCAL can perform most steps of the typical perovskite thin film fabrication process (Fig. 1a), including liquid handling, spin coating, annealing, and thin film characterizations such as imaging and spectroscopy. Pioneering efforts in material acceleration platforms have often generated proxy samples in model architectures that are then used to drive experiments in standard architectures.25–27 PASCAL can fabricate standard thin films and sub-cells for direct investigation e.g. of perovskite materials and their interfaces with transport layers for direct integration into solar cells. To do so, the system can perform arbitrary sequences of operations, including the deposition of multiple thin film layers with intervening annealing and characterization steps. PASCAL (Fig. 1b) consists of six primary components: a liquid handler (Opentrons OT2) to mix and dispense solutions, a custom spin coater, an array of three hotplates to anneal samples and perform thermal degradation tests, a characterization train to measure optical spectra and take images of each sample, storage trays to hold substrates, and a rectilinear gantry and gripper to transfer samples between stations. The spincoater was custom built to fit within one of the deck slots of the liquid handler, such that the liquid handler can dispense directly onto it.68,69 All components are housed inside a nitrogen glovebox (≤1 ppm O2 and H2O) to enable work on air-sensitive materials such as perovskites, which are sensitive to both oxygen and humidity exposure.61 The overall system is controlled from one computer using a custom Python library. This library is publicly available,69 but is heavily tailored to PASCAL. Some of the more generalizable code, specifically those for planning solution mixing and job scheduling for parallel job execution, are available as standalone Python packages.70,71
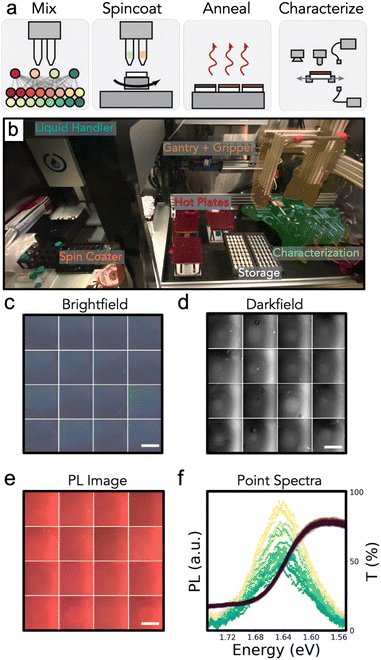 |
| Fig. 1 (a) Typical workflow schematic of PASCAL (left to right). (b) Photograph of PASCAL inside controlled glovebox environment. Color labels depict various components. Example demonstration of PASCAL in-line characterization suite on 1.64 eV bandgap perovskite sub-cells (Glass/ITO/MeO-2PACz/perovskite) including (c) brightfield imaging, (d) darkfield imaging, (e) PL imaging, and (f) point spectra of PL (yellow-green) and of transmission (dark blue). Scale bar 1.5 mm. | |
A typical compositional screening experiment begins with the interpolation of stock solutions into target solutions and mixed in a 96-well plate by the liquid handler. Next, a substrate is transferred to the spincoater chuck by the robot arm. The spincoater incorporates an encoder such that it can be rotated to a known position, which is necessary for the parallel-plate gripper to pick up the square sample substrates. During spincoating, PASCAL affords greater precision and recording of this critical step than is possible with human operation. The dispense position, height, and rate are precisely controlled, and the dispense timing is repeatable with a standard deviation of 18 ms (Fig. S1b†). After spin coating, the sample is transferred to one of three hot plates (depending on the set temperature), annealed, and then transferred to a storage tray to cool to room temperature prior to characterization. PASCAL characterization line consists of cameras, LED, and laser excitation sources, a halogen lamp, and a spectrometer to allow for a standard-set of images (brightfield, darkfield, PL) and spectroscopy (PL and transmission) measurements (Fig. 1c–f). Additionally, the characterization line has a linear stage to carry one sample between the various stations, allowing sample transfers between stations during the characterization step (which can take many minutes) to be decoupled from transfers between the fabrication steps (which can occur every ≈10 s when operating at maximum capacity). PASCAL can work on many samples in parallel, with typical experiments requiring about 3.5 h for fabrication and characterization of 45 sub-cell samples (2 thin film depositions) in parallel processing mode or 10 h in sequential processing mode (example job schedule in Fig. 2a and b). To complete devices, after PASCAL completes all tasks, sub-cells (e.g., Glass/ITO/MeO-2PACz/perovskite) are manually transferred to a glovebox-attached evaporation chamber for physical vapor deposition of contact layers to complete the device and finally to an automated illuminated current–voltage testing platform in the glovebox for device measurement without any air exposure.72
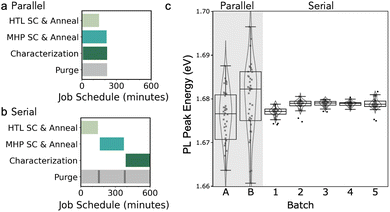 |
| Fig. 2 (a) Typical 45 sub-cell (Glass/ITO/MeO-2PACz/perovskite) job schedule with parallel processing and (b) serial processing. (c) Batch-to-batch PL peak energy box plots, batches A–B are fabricated in parallel processing mode, and batches 1–5 are fabricated in serial processing mode. | |
4 Results and discussion
With an introduction to the PASCAL platform complete, the remainder of this work presents the application of PASCAL to perovskite film fabrication toward development of a photo- and thermally-stable perovskite composition relevant to perovskite on silicon tandem solar cells. We present results on: (1) identifying and controlling hidden variables in perovskite processing and verifying PASCAL repeatability in sub-cell and cell fabrication, (2) exploiting PASCAL to screen the complex triple-cation, triple-halide composition space for wide-bandgap solar absorbers, applying machine learning to assist discovery of robust compositions and (3) prototyping devices using durable compositions identified to have a stable PL emission energy under thermal and illuminated accelerated test conditions.
4.1 Addressing reproducibility
4.1.1 Uncovering hidden variables.
Leveraging PASCAL mixing and timing repeatability we identified glovebox solvent partial pressure as having a negative effect on the repeatability of PL peak energy. PASCAL can operate all deposition and characterization tasks in parallel to optimize run time (Fig. 2a). However, parallel deposition of a hole transport layer (HTL, MeO-2PACz) in ethanol and perovskite in 3DMF
:
1DMSO with MeOAc anti-solvent reveals a wide distribution in the PL emission energy of the resulting thin films (Fig. 2c batches A–B). In comparison, we use serial processing of each layer to separate the solvent atmospheres (Fig. 2b), which involves an isolated 15 min N2 purge of the glovebox and removal of prior processing materials/waste prior to subsequent deposition or characterization to decrease the residual partial pressure of solvent vapors. This change resulted in a significant drop in the coefficient of variation (CV) of the PL peak energy from 3.92% for batches A–B, which were parallel processed, to 0.08% for batches 1–5, which were serial processed.
4.1.2 Batch-to-batch control.
In serial processing mode, using a similar triple-halide composition to Xu et al.,56 (Cs0.22FA0.78Pb(I0.85Br0.15)3) with 3% excess MAPbCl3 and 9% excess PbI2, we produced 5 batches of sub-cells (Glass/ITO/MeO-2PACz/perovskite) and then compared their performance to device. Exemplary XRD and SEM measurement of standalone films on glass are shown in Fig. S2.† The sub-cells are finished with thermal evaporation of C60/BCP/Ag outside of PASCAL in a separate glovebox, with the full device schematic shown in Fig. 3a.
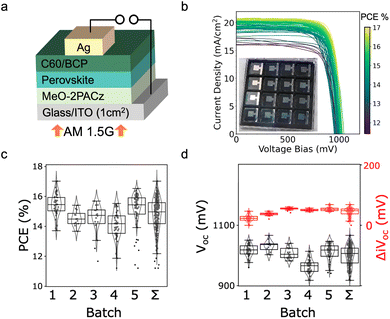 |
| Fig. 3 (a) Architecture of devices employed in this paper. (b) Reverse JV scan curves of devices (Cs0.22FA0.78Pb(I0.85Br0.15)3) from 5 combined batches, PCE of curves depicted by color bar. Bottom left inset example photo of 16 PASCAL fabricated 1 cm2 devices inside 5 cm2 container. (c) Box plots of device reverse scan PCE, and combined summary distribution. (d) Box plots of sub-cell delta implied open-circuit voltage (red, above) and device open-circuit voltage (black, below). Batches are labeled in order of fabrication. | |
In the device JV curves (Fig. 3b), average FF of all 5 batches were 75.1 ± 2.7%. Batch-wise median PCE (Fig. 3c) fluctuates, with the combined distribution having a median PCE of 15.0% and IQR of 1.4%. The champion device achieved 17% PCE. Loss analysis by PLQY reveals a Voc deficit of ≈400 mV, with a combined ≈200 mV lost at the selective contacts in these prototype devices (Fig. S3†). This analysis identifies promise for improved performance with a dedicated passivation strategy and improved band alignment.61
Regarding reproducibility, the distribution of device open voltage, shown in Fig. 3d, shows an interquartile range of about 50 mV (32.2 mV standard deviation). Interestingly, as seen in Fig. 3d, the variation in the implied open circuit voltage for the sub-cell fabrication wholly automated by PASCAL was significantly less, as quantified by the change in PL intensity of the films within the batches. The interquartile range for the populations of sub-cell ΔiVoc is 16.2 mV with a standard deviation of 12.7 mV. The coefficient of variation in the implied Voc is only ≈1.1%. Moving to the finished device involves increasing architectural complexity, and in the end the coefficient of variation of cell voltages increases, but remains relatively small at ≤3.2%.
The implementation of PASCAL has demonstrated a significant reduction in process variability, primarily attributable to the elimination of operator bias and errors in critical stages such as anti-solvent timing.16 The robot precision in handling sensitive processes improves consistency, a factor often compromised in manual operations. This is most evident in PASCAL serial processing mode where a tight batch-to-batch variation in PL peak energy and intensity was achieved in the complex triple-cation, triple-halide perovskite synthesis. We note that PASCAL begins operation with manually prepared ink stocks, where care must be taken lest error associated with precursor mass (here ±0.1 mg) and solution volume (here ±0.1 μL) propagate across batches.
4.1.3 Outlook on reproducibility.
The standardized data set generated by PASCAL facilitates a nuanced batch-to-batch comparison, enabling identification of subtler trends and anomalies. For instance, variations linked different solvent partial pressure incompatibilities, and steps external to PASCAL, like the evaporation of contacts, are now more readily identifiable.
While PASCAL fabricated sub-cells show tight distributions, the magnitude of variation in the device Voc was greater than in the sub-cell iVoc. The HTL/perovskite are just 2 layers of the full device architecture, and subsequent deposition now take place outside of PASCAL tight control. Future work will investigate irreproducibility in sample transfer and PVD deposition of contact layers.
4.2 Wide bandgap screening
Having established expected variance for a single composition, we screened a subset of the vast compositional perovskite landscape (MAxFA0.78Cs0.22−xPb(I0.8−y−zBryClz)3) fabricating 58 (details in methods) unique compositions to find 4 distinct behavior regions based on features of high importance to photovoltaic performance (i.e., initial PL spectrum and intensity, PL spectral changes upon illumination, PL spectral changes upon elevated temperature exposure). Thin film data for each feature is provided in the SI (Fig. S4–S9†) and their results are discussed below.
The bandgaps of thin films across the compositional screening range from 1.56 eV to 1.70 eV (Fig. S4†), covering the spread of both spectrally ideal and empirically tested ideal bandgaps of absorbers atop silicon in tandem devices.50,51,73 The dominant trends in bandgap arise, as expected from variations in the halide composition at the X-site.74,75 Most evident is the widening bandgap with increasing Br fraction (moving towards the top corner of the ternary diagrams). This contrasts with the non-monotonic relationship between Cl content (moving towards the bottom right corner), which initially widens until Cl comprises ≈7% of the halide content, after which the bandgap redshifts. This result is consistent with previous reports of the limited Cl solubility in the perovskite lattice in similar composition spaces, where, past a certain percentage, Cl addition causes compositional segregation into lower and higher Cl-containing regions and a net redshift in emission.56 This redshift is caused by the formation of iodine-rich phases, which act as sinks for carriers.52,76–78 Interestingly, we observe this redshift in all 10% Cl compositions except those with the highest Br content (10% Br) regardless of A-site composition, suggesting that the solubility limit of Cl in this system is modestly increased by Br addition. The composition of the A-site also affects the material bandgap. As we increase MA content (and accordingly decrease Cs content), the bandgap narrows slightly likely due to octahedral tilt as the effective A-site radius is manipulated.58
The photoluminescence (PL) intensity of films across the compositional space is shown in Fig. S5.† PL intensity is logarithmically proportional to the open-circuit voltage expected from a photovoltaic cell,79,80 and we can use PL data as a figure of merit to identify promising absorbers for future cell development. Broadly, compositions with ≥10% Br content display relatively lower PL intensity, and compositions with ≥7% Cl display anomalously high PL intensity indicative of phase splitting.56
We test the photostability of our thin films by recording changes in PL spectra over 2 min of constant irradiation by a blue laser (405 nm), with power equivalent to ≈4 sun. From these spectra, we extract the changes in both PL peak energy and intensity to evaluate a composition photostability (Fig. S6 and S7†).
Films across the composition space were seen to either “photodarken” (PL intensity decreases) or “photobrighten” (increases), with final PL intensities ranging from 10% to 200% of the initial intensity (Fig. S5†). We refer to this metric as the photointensity change. Broadly, compositions with ≥14% Br mostly lose emission intensity under photoexposure. All but one photobrightening composition contain Cl, with the largest increases in emission intensity occurring in compositions with ≥5% Cl.
Films were seen to either blueshift or redshift in emission energy under photoexposure, with all films shifting by less than 25 meV in either direction (Fig. S7†). Broadly, we observe that compositions with higher Cl fractions tend to redshift more after photoexposure.
We test the thermal stability of our thin films by recording changes in PL spectra before and after exposure to 85 °C in a nitrogen environment and observe that PL intensity reduces for nearly all films (Fig. S8†). At 0% and 5% MA content, the films with the highest Br fractions showed the most loss of PL intensity, dropping by up to three orders of magnitude (Fig. S8†). At 10% MA content, however, there is no clear relationship between halide composition and intensity loss. Across all halide compositions, higher MA content leads to greater losses in PL intensity after thermal degradation.
PL emission energy is seen to either redshift or blueshift after thermal testing, with all films shifting by less than 40 meV in either direction (Fig. S9†). Most films blueshifted, with those with 5% or higher Cl loading show the largest blueshift up to 40 meV after testing. Films with over 10% Br and under 5% Cl slightly redshifted (up to 7 meV after 4.5 h). Within this same space, MA content seems to increase the magnitude of redshift after exposure to 85 °C.
Notably, the screening study identified compositions that spanned red-to blueshifts upon photo- and thermal-stability testing. This span raises the possibility that within this space lies compositions that may show no shift.
4.2.1 Learning from the screening data.
We reduce the dimensionality in the screening data by using k-means spectral clustering (KMC)66 to segment perovskite compositions into four regions of distinct behavior, allowing for effective comparison across multiple dimensions. Specifically, we select 5 screening metrics which capture the stability and potential performance of the absorbers: thermal stability (by changes in PL emission energy and intensity, with no change desired), photostability (by changes in PL emission energy and intensity, with no change desired), and the initial PL intensity (brighter is better).
KMC performs clustering on the combined latent space of these 5 dimensions (Fig. 4a), with the number of clusters having been selected by trial-and-error – fewer than four clusters grouped disparate compositions together, while greater than four clusters only divided clusters in spurious ways that were not meaningful in our context of absorber screening. Note that the composition was not used for clustering, and that KMC does not require clusters to be contiguous in the compositional space.
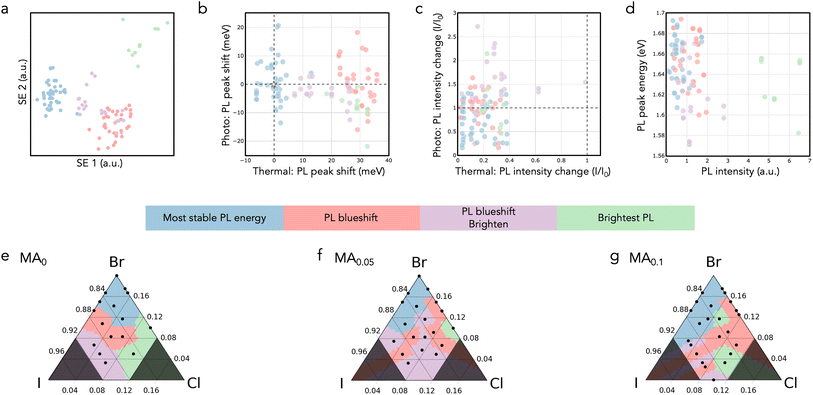 |
| Fig. 4 (a) Spectral clustering latent space, with arbitrary spectral embedding units. (b–d) Feature space plots of characterized samples, with each sample colored according to spectral cluster. Design goals in dashed black line. Yellow star depicts GPR assisted composition. (e–g) Ternary plots of tested compositions MAxFA0.78Cs0.22−xPb(I0.8−y−zBryClz)3, MA content above ternary. Spectral clustered and manually labeled distinct regions shaded in with nearest neighbor interpolation according to colorbar below. Areas of no experiment, I- and Cl-rich corners, are shaded out. | |
After the 4 unique clusters were generated, we manually labeled their distinct performance based on each cluster average features (Table S1†) and provide the following labels: (1) “most stable PL energy”, (2) “PL blueshift”, (3) “PL blueshift & brighten”, and (4) “brightest PL”. The “most stable PL energy” cluster is particularly interesting for photovoltaic development.
In inverting the latent space (Fig. 4b and d), we see that the “most stable PL energy” has PL peak shifts that is uniquely distinguishable from rest of the clusters. Similarly, the “brightest PL” cluster is clearly distinguishable on the PL intensity axis.
These clusters are mapped back to the compositional space in Fig. 4e–g. In 0% MA compositions below 7% Cl (Fig. 4e), the space separates into 3 distinct behaviors (blue, red, purple) that suggest there may be compositional boundaries where targeted features can be achieved. The MA content on the absorber A-site plays a significant role in shifting the boundaries of these functional behaviors (Fig. 4e–g). Most notably, the stable blue region grows toward richer I fraction with increasing MA (10%) content. The green “brightest PL” region broadly remains within high Cl% with MA loading, suggesting that this bright PL effect is more a consequence of the X-site fraction than the A-site fraction (ABX3).
KMC effectively uncovered latent structures within the dataset, demonstrating its strength in differentiating regions of distinct behavior. Notably, “brightest PL” and “most stable PL energy” clusters exhibited distinct separations in the latent space, illustrating KMC ability to identify clear differences in features. However KMC revealed less distinct separation for the “PL blueshift” and “PL blueshift & brighten” clusters, likely due to overlaps in their feature spaces. This overlap manifested as ambiguous fluctuating boundaries in the 5% and 10% MA compositional spaces, suggesting an ambiguity between these distinct behaviors at these halide compositions.
4.3 Prototyping a durable composition
Armed with a clustered compositional space, we use the promising “most stable PL energy” cluster to construct a Gaussian process regression (GPR) model67 that correlates composition to PL peak shift stability. The model is fit using each sample euclidean distance from the origin on the thermal and photo PL peak shift axis to predict a composition with no shift.
The relevant halide space is defined in Fig. 5a, with 3 MA slices showing the GPR fits in Fig. 5b–d (model full space with finer increments of MA slices provided as a Video in the ESI†). The GPR predicted that no shift would be seen upon photo- or thermal stability testing of a film with composition MA0.035FA0.78Cs0.185Pb(I0.866Br0.134)3. For synthesis considering this target composition, we added a small fraction of methylammonium chloride (MACl) to the precursor ink as a crystallization additive that is known to volatilize out of the film during annealing.56,81 The nominal precursor composition selected for device prototyping was thus: MA0.035FA0.78Cs0.185Pb(I0.863Br0.132Cl0.005)3. This composition is shown as a star symbol in Fig. 5c.
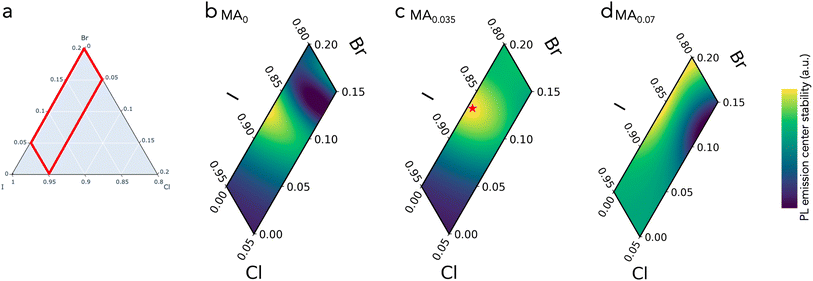 |
| Fig. 5 (a) GPR model relevant halide space inside red outline. Fitted GPR slices of (b) MA 0%, (c) MA 3.5%, (d) MA 7% composition. Bright yellow depicts a thermal-/photo PL peak shift stable composition. Red star depicts composition chosen (MA0.035FA0.78Cs0.185Pb(I0.863Br0.132Cl0.005)3). | |
PASCAL thin films produced at this composition resulted in ≤1 meV (Table S2†) PL peak shift under heat and photoexposure. Prototype devices were fabricated with PCEs reaching above 15.5% with no interface or bulk passivation. The devices Voc distribution showed an IQR of 30 mV (Fig. S10†). While our focus was on rapid screening and development of the bare perovskite thin film stability, we anticipate that improvements in stability of the absorber layer will likely contribute positively to overall device durability.
In short, the GPR model successfully assisted in down selection of a composition with minimal PL peak shift. However, we do advise caution in the blanket application of GPR across the full composition space here or in wider searches in perovskites. Specifically, instances of pronounced contrasts, like the distinct PL peak energy (Fig. S4†) and intensity (Fig. S5†) observed at high Cl fractions, corresponding to underlying phase separation, may be better suited to other models such as random forest regressions,82 ridge regressions,83 or physics injected Bayesian models12,39 that may enable prediction of sharp phase boundaries.
Furthermore, the photo- and thermal stability tests on bare perovskite thin films are of course preliminary. Further development requires the ultimate application of more comprehensive standardized tests, such as the ISOS consensus tests84 or the IEC terrestrial photovoltaic standards.85 Further testing of promising compositions in final device architectures could establish more full correlations with relevant photovoltaic durability metrics and perhaps identify early film-level tests like those used here that may predict downstream device durability.
5 Conclusions
In this work, we introduce PASCAL, the Perovskite Automated Spin Coating Assembly Line, a high-throughput automated platform designed for accelerating the spin coating and analysis of thin films. PASCAL provides significantly enhanced control over spin coated thin film fabrication, surpassing the limitations of manual methods without compromise on thin film architecture. We leverage PASCAL's repeatability to address sensitive processing parameters known to challenge halide perovskite fabrication, such as anti-solvent drop timings. With PASCAL, we identify and minimize the influence of previously hidden variables, such as solvent partial pressure, thereby achieving a high level of repeatability. This advancement facilitates extensive compositional screening where we reproducibly analyze 58 unique compositions within the complex triple-halide, triple-cation composition space. With this screening dataset, we leverage machine learning to develop a durable composition that displays nearly zero photoluminescence peak shifts under both 85 °C and approximately 4-sun photoexposure. Finally, we prototype this composition in single-junction devices.
PASCAL's capabilities to efficiently screen in composition and process space for development of solution-processed films and multilayer devices are demonstrated here with a focus on halide perovskites. More broadly, the automation of solution processing of thin films permits applications across early-stage research leveraging the rapid prototyping enabled by spin coating and non-contact optoelectronic characterization. Moreover, ink design for transferability to scalable meniscus coating can be rapidly prototyped within PASCAL (e.g. antisolvent free deposition). Looking forward, the automation, repeatability and high-throughput of PASCAL paves the way for future high-dimensional film deposition studies, rapid optimization, and interlaboratory control.
Data availability
The data and analysis code can be found at https://github.com/fenning-research-group/PASCAL.
Author contributions
D. N. C., R. E. K., and D. P. F. conceived PASCAL. D. N. C., R. E. K. contributed to building PASCAL's hardware and software. R. E. K. wrote critical software to orchestrate modules within PASCAL. D. N. C., R. E. K., and J. R. P., maintained PASCAL. D. N. C. led experiments surrounding parallel/serial processing, repeatability studies, durable prototype films/devices and assisted with wide bandgap screening. R. E. K. and M. K. led wide bandgap screening experiments. E. O. assisted with all sample fabrication. A. G. assisted with physical vapor depositions. R. E. K., D. N. C., M. K., and K. K. conceived/built an automated device tester, K. K. measured devices with it. J. R. P. built a custom PLQY system, H. M. V. took PLQY measurements on it. D. N. C. conducted all data curation and formal analysis with R. E. K. collaboration on wide bandgap screening experiments. D. N. C. built all visualizations. D. P. F. led the research team, acquired funding, and supervised the research. D. N. C. and R. E. K. wrote original draft, while all authors contributed to the review and editing of the manuscript.
Conflicts of interest
There are no conflicts to declare.
Acknowledgements
We would like to thank University of California, San Diego MAE156B course team members Kieu Huynh, Manuel Saldana, Alanna Bailey, and Angela Yu for development of and early beta testing of the gripper and gantry system. We also would like to thank Justin Skaggs for early development and beta testing of the automated device tester. The compositional screening and solar cell fabrication in this work was supported by the California Energy Commission, EPC-19-004. R.E.K. acknowledges the support of a UC San Diego Materials Science and Engineering Dissertation Year Fellowship.
References
- A. W. Sleight and R. Ward, Inorg. Chem., 1963, 1963 Search PubMed.
- H.-J. Feng, W. Deng, K. Yang, J. Huang and X. C. Zeng, J. Phys. Chem. C, 2017, 121, 4471–4480 CrossRef CAS.
- A. H. Slavney, T. Hu, A. M. Lindenberg and H. I. Karunadasa, J. Am. Chem. Soc., 2016, 138, 2138–2141 CrossRef CAS PubMed.
- S. Vasala and M. Karppinen, Prog. Solid State Chem., 2015, 43, 1–36 CrossRef CAS.
- F. Bella, C. Gerbaldi, C. Barolo and M. Grätzel, Chem. Soc. Rev., 2015, 44, 3431–3473 RSC.
- W. Zhang, M. Saliba, D. T. Moore, S. K. Pathak, M. T. Hörantner, T. Stergiopoulos, S. D. Stranks, G. E. Eperon, J. A. Alexander-Webber, A. Abate, A. Sadhanala, S. Yao, Y. Chen, R. H. Friend, L. A. Estroff, U. Wiesner and H. J. Snaith, Nat. Commun., 2015, 6, 6142 CrossRef CAS PubMed.
- Q. Jiang, D. Rebollar, J. Gong, E. L. Piacentino, C. Zheng and T. Xu, Angew. Chem., Int. Ed., 2015, 54, 7617–7620 CrossRef CAS PubMed.
- L. Chu, Matter, 2021, 4, 1762–1764 CrossRef CAS.
- J. Wang, M. A. Uddin, B. Chen, X. Ying, Z. Ni, Y. Zhou, M. Li, M. Wang, Z. Yu and J. Huang, Adv. Energy Mater., 2023, 13, 1–8 Search PubMed.
- M. A. Uddin, P. J. S. Rana, Z. Ni, G. Yang, M. Li, M. Wang, H. Gu, H. Zhang, B. D. Dou and J. Huang, Nat. Commun., 2024, 15, 1355 CrossRef CAS PubMed.
- Y.-Y. Sun, M. L. Agiorgousis, P. Zhang and S. Zhang, Nano Lett., 2015, 15, 581–585 CrossRef CAS PubMed.
- M. Ahmadi, M. Ziatdinov, Y. Zhou, E. A. Lass and S. V. Kalinin, Joule, 2021, 5, 2797–2822 CrossRef CAS.
- M. Saliba, Adv. Energy Mater., 2019, 9, 1803754 CrossRef.
- R. A. Kerner, E. D. Christensen, S. P. Harvey, J. Messinger, S. N. Habisreutinger, F. Zhang, G. E. Eperon, L. T. Schelhas, K. Zhu, J. J. Berry and D. T. Moore, ACS Appl. Energy Mater., 2023, 6, 295–301 CrossRef CAS.
- G. P. Nagabhushana, R. Shivaramaiah and A. Navrotsky, Proc. Natl. Acad. Sci. U. S. A., 2016, 113, 7717–7721 CrossRef CAS PubMed.
- K. P. Goetz and Y. Vaynzof, ACS Energy Lett., 2022, 7, 1750–1757 CrossRef CAS.
- A. Taylor, Q. Sun, K. Goetz, Q. An, T. Schramm, Y. Hofstetter, M. Litterst, F. Paulus and Y. Vaynzof, Nat. Commun., 2021, 12, 1878 CrossRef CAS PubMed.
- K. Wang, M. Tang, H. Dang, R. Munir, D. Barrit, M. De Bastiani, E. Aydin, D. Smilgies, S. De Wolf and A. Amassian, Adv. Mater., 2019, 31, e1808357 CrossRef PubMed.
- R. E. Kumar, A. Tiihonen, S. Sun, D. P. Fenning, Z. Liu and T. Buonassisi, Matter, 2022, 5, 1353–1366 CrossRef.
- B. P. MacLeod, F. G. Parlane, T. D. Morrissey, F. Häse, L. M. Roch, K. E. Dettelbach, R. Moreira, L. P. Yunker, M. B. Rooney, J. R. Deeth, V. Lai, G. J. Ng, H. Situ, R. H. Zhang, M. S. Elliott, T. H. Haley, D. J. Dvorak, A. Aspuru-Guzik, J. E. Hein and C. P. Berlinguette, Sci. Adv., 2020, 6, eaaz8867 CrossRef CAS PubMed.
- R. W. Epps, M. S. Bowen, A. A. Volk, K. Abdel-Latif, S. Han, K. G. Reyes, A. Amassian and M. Abolhasani, Adv. Mater., 2020, 32, 2001626 CrossRef CAS PubMed.
- Z. Li, M. A. Najeeb, L. Alves, A. Z. Sherman, V. Shekar, P. Cruz Parrilla, I. M. Pendleton, W. Wang, P. W. Nega, M. Zeller, J. Schrier, A. J. Norquist and E. M. Chan, Chem. Mater., 2020, 32, 5650–5663 CrossRef CAS.
- P. W. Nega, Z. Li, V. Ghosh, J. Thapa, S. Sun, N. T. P. Hartono, M. A. N. Nellikkal, A. J. Norquist, T. Buonassisi, E. M. Chan and J. Schrier, Appl. Phys. Lett., 2021, 119, 041903 CrossRef CAS.
- H. Zhao, W. Chen, H. Huang, Z. Sun, Z. Chen, L. Wu, B. Zhang, F. Lai, Z. Wang, M. L. Adam, C. H. Pang, P. K. Chu, Y. Lu, T. Wu, J. Jiang, Z. Yin and X.-F. Yu, Nat. Synth., 2023, 2, 505–514 CrossRef.
- T. Wang, R. Li, H. Ardekani, L. Serrano-Luján, J. Wang, M. Ramezani, R. Wilmington, M. Chauhan, R. W. Epps, K. Darabi, B. Guo, D. Sun, M. Abolhasani, K. Gundogdu and A. Amassian, Matter, 2023, 6, 2963–2986 CrossRef CAS.
- K. Higgins, S. M. Valleti, M. Ziatdinov, S. V. Kalinin and M. Ahmadi, ACS Energy Lett., 2020, 5, 3426–3436 CrossRef CAS.
- E. Gu, X. Tang, S. Langner, P. Duchstein, Y. Zhao, I. Levchuk, V. Kalancha, T. Stubhan, J. Hauch, H. J. Egelhaaf, D. Zahn, A. Osvet and C. J. Brabec, Joule, 2020, 4, 1806–1822 CrossRef CAS.
- J. Li, J. Li, R. Liu, Y. Tu, Y. Li, J. Cheng, T. He and X. Zhu, Nat. Commun., 2020, 11, 1–10 CrossRef CAS PubMed.
- A. Dubey, S. L. Sanchez, J. Yang and M. Ahmadi, Chem. Mater., 2024, 36, 2165–2176 CrossRef CAS.
- R. J. R. Jones, Y. Lai, D. Guevarra, K. Kan, J. A. Haber and J. M. Gregoire, Digital Discovery, 2024 10.1039/D4DD00061G.
- F. Rahmanian, S. Fuchs, B. Zhang, M. Fichtner and H. S. Stein, Digital Discovery, 2024 10.1039/D3DD00257H.
- N. J. Szymanski, B. Rendy, Y. Fei, R. E. Kumar, T. He, D. Milsted, M. J. McDermott, M. Gallant, E. D. Cubuk, A. Merchant, H. Kim, A. Jain, C. J. Bartel, K. Persson, Y. Zeng and G. Ceder, Nature, 2023, 624, 86–91 CrossRef CAS PubMed.
- M. Kodera and K. Sayama, Digital Discovery, 2023, 2, 1683–1687 RSC.
- J. Wagner, C. G. Berger, X. Du, T. Stubhan, J. A. Hauch and C. J. Brabec, J. Mater. Sci., 2021, 56, 16422–16446 CrossRef CAS.
- J. Zhang, B. Liu, Z. Liu, J. Wu, S. Arnold, H. Shi, T. Osterrieder, J. A. Hauch, Z. Wu, J. Luo, J. Wagner, C. G. Berger, T. Stubhan, F. Schmitt, K. Zhang, M. Sytnyk, T. Heumueller, C. M. Sutter-Fella, I. M. Peters, Y. Zhao and C. J. Brabec, Adv. Energy Mater., 2023, 13, year Search PubMed.
- Y. Zhao, J. Zhang, Z. Xu, S. Sun, S. Langner, N. Hartono, T. Heumueller, Y. Hou, J. Elia, N. Li, G. Matt, X. Du, W. Meng, A. Osvet, K. Zhang, T. Stubhan, Y. Feng, J. Hauch, E. Sargent, T. Buonassisi and C. Brabec, Nat. Commun., 2021, 12, 2191 CrossRef CAS PubMed.
- Z. Xu, Y. Zhao, J. Zhang, K. Chen, C. J. Brabec and Y. Feng, Phys. Rev. Mater., 2020, 4, 095401 CrossRef CAS.
- T. Osterrieder, F. Schmitt, L. Lüer, J. Wagner, T. Heumüller, J. Hauch and C. J. Brabec, Energy Environ. Sci., 2023, 16, 3984–3993 RSC.
- M. A. Ziatdinov, A. Ghosh and S. V. Kalinin, Mach. learn.: sci. technol., 2022, 3, 015003 Search PubMed.
- L. Pereira Diaz, C. J. Brown, E. Ojo, C. Mustoe and A. J. Florence, Digital Discovery, 2023, 2, 692–701 RSC.
- A. Mannodi-Kanakkithodi and M. K. Chan, Energy Environ. Sci., 2022, 1930–1949 RSC.
- J. Yang, P. Manganaris and A. Mannodi-Kanakkithodi, J. Chem. Phys., 2024, 160, 064114 CrossRef CAS PubMed.
-
B. N. Slautin, Y. Liu, H. Funakubo, R. K. Vasudevan, M. A. Ziatdinov and S. V. Kalinin, Bayesian Co-navigation: Dynamic Designing of the Materials Digital Twins via Active Learning, 2024 Search PubMed.
- M. H. Rahman, P. Gollapalli, P. Manganaris, S. K. Yadav, G. Pilania, B. DeCost, K. Choudhary and A. Mannodi-Kanakkithodi, APL mach. learn., 2024, 2, 016122 CrossRef.
-
L. LONGi Green Energy Technology Co., LONGi sets a new world record of 33.9% for the efficiency of crystalline silicon-perovskite tandem solar cells, 2023, accessed: 2024-03-04, https://www.longi.com/en/news.
-
Best research-cell efficiency chart, 2024, https://www.nrel.gov/pv/cell-efficiency.html.
- J. S. Manser, M. I. Saidaminov, J. A. Christians, O. M. Bakr and P. V. Kamat, Acc. Chem. Res., 2016, 49, 330–338 CrossRef CAS PubMed.
- D. P. McMeekin, G. Sadoughi, W. Rehman, G. E. Eperon, M. Saliba, M. T. Hörantner, A. Haghighirad, N. Sakai, L. Korte, B. Rech, M. B. Johnston, L. M. Herz and H. J. Snaith, Science, 2016, 351, 151–155 CrossRef CAS PubMed.
- S. De Wolf, J. Holovsky, S. J. Moon, P. Löper, B. Niesen, M. Ledinsky, F. J. Haug, J. H. Yum and C. Ballif, J. Phys. Chem. Lett., 2014, 5, 1035–1039 CrossRef CAS PubMed.
- K. A. Bush, S. Manzoor, K. Frohna, Z. J. Yu, J. A. Raiford, A. F. Palmstrom, H.-P. Wang, R. Prasanna, S. F. Bent, Z. C. Holman and M. D. McGehee, ACS Energy Lett., 2018, 3, 2173–2180 CrossRef CAS.
- E. Aydin, T. G. Allen, M. De Bastiani, L. Xu, J. Ávila, M. Salvador, E. Van Kerschaver and S. De Wolf, Nat. Energy, 2020, 5, 851–859 CrossRef CAS.
- E. T. Hoke, D. J. Slotcavage, E. R. Dohner, A. R. Bowring, H. I. Karunadasa and M. D. McGehee, Chem. Sci., 2015, 6, 613–617 RSC.
- J. Tong, Q. Jiang, F. Zhang, S. B. Kang, D. H. Kim and K. Zhu, ACS Energy Lett., 2021, 6, 232–248 CrossRef CAS PubMed.
- T. Matsui, T. Yokoyama, T. Negami, T. Sekiguchi, M. Saliba, M. Grätzel and H. Segawa, Chem. Lett., 2018, 47, 814–816 CrossRef CAS.
- M. Saliba, T. Matsui, J.-Y. Seo, K. Domanski, J.-P. Correa-Baena, M. K. Nazeeruddin, S. M. Zakeeruddin, W. Tress, A. Abate, A. Hagfeldt and M. Grätzel, Energy Environ. Sci., 2016, 9, 1989–1997 RSC.
- J. Xu, C. Boyd, Z. Yu, A. Palmstrom, D. Witter, B. Larson, R. France, J. Werner, S. Harvey, E. Wolf, W. Weigand, S. Manzoor, M. van Hest, J. Berry, J. Luther, Z. Holman and M. McGehee, Science, 2020, 367, 1097–1104 CrossRef CAS PubMed.
- K. A. Bush, A. F. Palmstrom, Z. J. Yu, M. Boccard, R. Cheacharoen, J. P. Mailoa, D. P. McMeekin, R. L. Hoye, C. D. Bailie, T. Leijtens, I. M. Peters, M. C. Minichetti, N. Rolston, R. Prasanna, S. Sofia, D. Harwood, W. Ma, F. Moghadam, H. J. Snaith, T. Buonassisi, Z. C. Holman, S. F. Bent and M. D. McGehee, Nat. Energy, 2017, 2, 1–7 Search PubMed.
- G. E. Eperon, K. H. Stone, L. E. Mundt, T. H. Schloemer, S. N. Habisreutinger, S. P. Dunfield, L. T. Schelhas, J. J. Berry and D. T. Moore, ACS Energy Lett., 2020, 5, 1856–1864 CrossRef CAS.
- A. J. Ramadan, R. D. Oliver, M. B. Johnston and H. J. Snaith, Nat. Rev. Mater., 2023, 8, 822–838 CrossRef CAS.
- D. P. McMeekin, P. Holzhey, S. O. Fürer, S. P. Harvey, L. T. Schelhas, J. M. Ball, S. Mahesh, S. Seo, N. Hawkins, J. Lu, M. B. Johnston, J. J. Berry, U. Bach and H. J. Snaith, Nat. Mater., 2023, 22, 73–83 CrossRef CAS PubMed.
- S. P. Dunfield, L. Bliss, F. Zhang, J. M. Luther, K. Zhu, M. F. A. M. Hest, M. O. Reese and J. J. Berry, Adv. Energy Mater., 2020, 10, 1904054 CrossRef CAS.
- H. Meng, K. Mao, F. Cai, K. Zhang, S. Yuan, T. Li, F. Cao, Z. Su, Z. Zhu, X. Feng, W. Peng, J. Xu, Y. Gao, W. Chen, C. Xiao, X. Wu, M. D. McGehee and J. Xu, Nat. Energy, 2024, 9, 536–547 CrossRef CAS.
- J. Liu, E. Aydin, J. Yin, M. De Bastiani, F. H. Isikgor, A. U. Rehman, E. Yengel, E. Ugur, G. T. Harrison, M. Wang, Y. Gao, J. I. Khan, M. Babics, T. G. Allen, A. S. Subbiah, K. Zhu, X. Zheng, W. Yan, F. Xu, M. F. Salvador, O. M. Bakr, T. D. Anthopoulos, M. Lanza, O. F. Mohammed, F. Laquai and S. De Wolf, Joule, 2021, 5, 3169–3186 CrossRef CAS.
- H. Zhu, S. Teale, M. N. Lintangpradipto, S. Mahesh, B. Chen, M. D. McGehee, E. H. Sargent and O. M. Bakr, Nat. Rev. Mater., 2023, 8, 569–586 CrossRef.
- J. C. de Mello, H. F. Wittmann and R. H. Friend, Adv. Mater., 1997, 9, 230–232 CrossRef CAS.
- A. V. Knyazev, SIAM J. Sci. Comput., 2001, 23, 517–541 CrossRef.
- M. Balandat, B. Karrer, D. R. Jiang, S. Daulton, B. Letham, A. G. Wilson and E. Bakshy, Adv. Neural Inf. Process. Syst., 2020, 21524–21538 Search PubMed.
-
M. Stefik, Spin Coater, 2024, accessed: 2024-03-12, https://www.stefikgroup.com/spin-coater/.
-
D. N. Cakan, R. E. Kumar, J. R. Palmer, and K. C. Wong, fenning-research-group/PASCAL, v1.0.0, p. 2024, https://github.com/fenning-research-group/PASCAL.
-
R. E. Kumar, rekumar/roboflo: v0.2.1, 2024, https://github.com/rekumar/roboflo/tree/v0.2.1 Search PubMed.
-
R. E. Kumar, rekumar/mixsol: Release for Zenodo Citation, 2024, https://github.com/rekumar/mixsol/tree/v0.5.1 Search PubMed.
-
D. N. Cakan, R. E. Kumar, S. P. Dunfield, K. Kaushal and H. M. Vossler, fenning-research-group/jvbot: v1.0.0, 2024, https://github.com/fenning-research-group/jvbot.
- M. De Bastiani, E. Van Kerschaver, Q. Jeangros, A. Ur Rehman, E. Aydin, F. H. Isikgor, A. J. Mirabelli, M. Babics, J. Liu, S. Zhumagali, E. Ugur, G. T. Harrison, T. G. Allen, B. Chen, Y. Hou, S. Shikin, E. H. Sargent, C. Ballif, M. Salvador and S. De Wolf, ACS Energy Lett., 2021, 6, 2944–2951 CrossRef CAS.
- Z. Xiao, Y. Zhou, H. Hosono, T. Kamiya and N. P. Padture, Chem.–Eur. J., 2018, 24, 2305–2316 CrossRef CAS PubMed.
-
S. Gholipour and M. Saliba, Characterization Techniques for Perovskite Solar Cell Materials, Elsevier, 2020, pp. 1–22 Search PubMed.
- M. C. Brennan, A. Ruth, P. V. Kamat and M. Kuno, Trends Chem., 2020, 2, 282–301 CrossRef CAS.
- D. J. Slotcavage, H. I. Karunadasa and M. D. McGehee, ACS Energy Lett., 2016, 1, 1199–1205 CrossRef CAS.
- K. Frohna, M. Anaya, S. Macpherson, J. Sung, T. A. S. Doherty, Y.-h. Chiang, A. J. Winchester, K. W. P. Orr, J. E. Parker, P. D. Quinn, K. M. Dani, A. Rao and S. D. Stranks, Nat. Nanotechnol., 2022, 17, 190–196 CrossRef CAS PubMed.
- S. Siebentritt, T. P. Weiss, M. Sood, M. H. Wolter, A. Lomuscio and O. Ramirez, J. Phys.: Mater., 2021, 4, 042010 CAS.
- T. Kirchartz, J. A. Márquez, M. Stolterfoht and T. Unold, Adv. Energy Mater., 2020, 1904134 CrossRef CAS.
- H. Yu, F. Wang, F. Xie, W. Li, J. Chen and N. Zhao, Adv. Funct. Mater., 2014, 24, 7102–7108 CrossRef CAS.
- P. V. Balachandran, A. A. Emery, J. E. Gubernatis, T. Lookman, C. Wolverton and A. Zunger, Phys. Rev. Mater., 2018, 2, 043802 CrossRef CAS.
-
Z. Li, Q. Xu, Q. Sun, Z. Hou and W.-J. Yin, Stability Engineering of Halide Perovskite via Machine Learning, 2018 Search PubMed.
- M. V. Khenkin, E. A. Katz, A. Abate, G. Bardizza, J. J. Berry, C. Brabec, F. Brunetti, V. Bulović, Q. Burlingame, A. Di Carlo, R. Cheacharoen, Y.-B. Cheng, A. Colsmann, S. Cros, K. Domanski, M. Dusza, C. J. Fell, S. R. Forrest, Y. Galagan, D. Di Girolamo, M. Grätzel, A. Hagfeldt, E. von Hauff, H. Hoppe, J. Kettle, H. Köbler, M. S. Leite, S. Liu, Y.-L. Loo, J. M. Luther, C.-Q. Ma, M. Madsen, M. Manceau, M. Matheron, M. McGehee, R. Meitzner, M. K. Nazeeruddin, A. F. Nogueira, Ç. Odabaşı, A. Osherov, N.-G. Park, M. O. Reese, F. De Rossi, M. Saliba, U. S. Schubert, H. J. Snaith, S. D. Stranks, W. Tress, P. A. Troshin, V. Turkovic, S. Veenstra, I. Visoly-Fisher, A. Walsh, T. Watson, H. Xie, R. Yıldırım, S. M. Zakeeruddin, K. Zhu and M. Lira-Cantu, Nat. Energy, 2020, 5, 35–49 CrossRef.
- P. Holzhey and M. Saliba, J. Mater. Chem. A, 2018, 6, 21794–21808 RSC.
|
This journal is © The Royal Society of Chemistry 2024 |
Click here to see how this site uses Cookies. View our privacy policy here.