Artificial intelligence-enabled optimization of battery-grade lithium carbonate production†
Received
18th June 2024
, Accepted 20th September 2024
First published on 14th October 2024
Abstract
By 2035, the need for battery-grade lithium is expected to quadruple. About half of this lithium is currently sourced from brines and must be converted from lithium chloride into lithium carbonate (Li2CO3) through a process called softening. Conventional softening methods using sodium or potassium salts contribute to carbon emissions during reagent mining and battery manufacturing, exacerbating global warming. This study introduces an alternative approach using carbon dioxide (CO2(g)) as the carbonating reagent in the lithium softening process, offering a carbon capture solution. We employed an active learning-driven high-throughput method to rapidly capture CO2(g) and convert it to lithium carbonate. The model was simplified by focusing on the elemental concentrations of C, Li, and N for practical measurement and tracking, avoiding the complexities of ion speciation equilibria. This approach led to an optimized lithium carbonate process that capitalizes on CO2(g) capture and improves the battery metal supply chain's carbon efficiency.
1. Introduction
Lithium carbonate is a critical precursor for the production of lithium-ion batteries which range from use in portable electronics to electric vehicles. In fact, battery applications account for over 80% of all lithium produced globally and demand a high purity level, with raw lithium carbonate (Li2CO3) requiring a purity above 99%.1,2 Typically, lithium is sourced from brines or clays in forms such as lithium chloride or sulfate which are then converted to lithium carbonate through a metathesis reaction. In this reaction, the chloride or sulphate anion is replaced by carbonate, precipitating the insoluble lithium carbonate product (see eqn (1)).1,3–5 This process, called softening, must be performed such that impurities in the brine are excluded from the product during crystallization, and the crystal size is sufficiently large to facilitate filtration and isolation.1,3,6 | Conventional method: 2LiCl(aq) + Na2CO3(aq) ⇌ 2NaCl(aq) + Li2CO3(s) | (1) |
| Current study: 2LiCl(aq) + 2NH4OH(aq) + CO2(g) ⇌ Li2CO3(s) + 2NH4Cl(aq) + H2O(l) | (2) |
The conventional approach to softening usually uses mined sodium carbonate (Na2CO3) as the carbonate source, producing soluble sodium chloride (NaCl), or sodium sulfate (Na2SO4) as the reaction byproduct.3,4 The presence of these sodium salts in the crystallization matrix can lead to inclusion within the Li2CO3 crystals, resulting in a lower purity of the final product.6,7 Moreover, the carbon atom sourced from mined sodium carbonate salt is released as carbon dioxide gas (CO2(g)) into the atmosphere during battery manufacturing. Additionally, the mining and transporting of sodium (or potassium) carbonate contributes to the overall carbon footprint of lithium carbonate production.7
In contrast, our proposed method aims to utilize onsite-produced CO2(g) as a carbonate source for softening. This approach offers the potential to reduce the carbon footprint associated with the softening process itself, remove sodium from the solution, and potentially enhance the purity of the final Li2CO3 product. One approach is to react CO2(g) with an aqueous solution of ammonium hydroxide (NH4OH) to produce ammonium carbonate in situ ((NH4)2CO3);8,9 solutions of lithium chloride (LiCl) can then be treated with this ammonium carbonate to produce lithium carbonate (the net equilibrium can be seen in eqn (2)). However, softening lithium brines with ammonium carbonate involves complex equilibria governing CO2(g) dissolution, Li2CO3 precipitation, and the reaction of carbonic acid (a weak acid) with ammonium hydroxide (a weak base).8,10,11 The reaction produces ammonium as a byproduct, which is a weak acid that can protonate carbonate, hindering Li2CO3 precipitation by converting dissolved carbonate ions into bicarbonate.10 A system of multiple inter-related equilibria describes this chemical system (Table 1). While this approach reduces the carbon footprint associated with the softening reaction itself, the overall environmental impact of the process still depends on the sourcing and production methods of all involved compounds, including ammonia. This highlights opportunities for future investigations and optimizations to further improve the process.
Table 1 Detailed equilibrium reactions in lithium brine softening using ammonium hydroxide and carbon dioxide gas
Vapor–liquid |
Solid formation |
CO2(g) ⇌ CO2(aq) |
Eqn (3) |
NH4(aq)+ + HCO3(aq)− ⇌ NH4HCO3(s) |
Eqn (11) |
NH3(g) ⇌ NH3(aq) |
Eqn (4) |
NH4(aq)+ + NH2COO(aq)− ⇌ NH2COONH4(s) |
Eqn (12) |
H2O(g) ⇌ H2O(l) |
Eqn (5) |
3NH4(aq)+ + CO3(aq)2− + HCO3(aq)− + 2H2O(l) + NH3(aq) ⇌ 2(NH4)2CO3·H2O(s) |
Eqn (13) |
4NH4(aq)+ + CO3(aq)2− + 2HCO3(aq)− ⇌ (NH4)2CO3·2NH4HCO3(s) |
Eqn (14) |
![[thin space (1/6-em)]](https://www.rsc.org/images/entities/char_2009.gif) |
Liquid speciation |
Carbonate formation |
NH3(aq) + H2O(l) ⇌ NH4(aq)+ + OH(aq)− |
Eqn (6) |
H2CO3(aq) ⇌ H(aq)+ + HCO3(aq)− |
Eqn (15) |
CO2(aq) + H2O(l) ⇌ H(aq)+ + HCO3(aq)− |
Eqn (7) |
HCO3(aq)− ⇌ H(aq)+ + CO3(aq)2− |
Eqn (8) |
2Li(aq)+ + CO3(aq)2− ⇌ Li2CO3(s) |
Eqn (16) |
H2O(l) ⇌ H(aq)+ + OH(aq)− |
Eqn (9) |
NH3(aq) + HCO3(aq)− ⇌ NH2COO(aq)− + H2O(l) |
Eqn (10) |
The goal of this work was to identify a set of reaction conditions that maximizes lithium carbonate formation (eqn (16)). Modeling this complex system of equilibria through traditional thermodynamic approaches is challenged by the multitude of independent chemical species, the numerous inter-related reactions, and the possible importance of kinetics.7 Experimental approaches to optimize this system were similarly challenged by the complexity of the search space and its potential nonlinearities. Among the few examples of Li2CO3 production with CO2(g), M. Tian et al. observed that increasing ammonium hydroxide concentration from 200 g L−1 to 400 g L−1 raised the yield from 43.0% to 49.6%.12 The highest reported yield in similar systems (using LiOH) is about 73% with 2 mol L−1 LiOH solution at 40 °C.13 In spite of the significance of Li2CO3 production and growing environmental concerns emphasizing the development of carbon capture reactions, studies on Li2CO3 production using CO2(g) remain limited.
In recent years, high-throughput experimentation (HTE) has been widely adopted for materials discovery and optimization across various fields.14–18 HTE accelerates discovery and optimization processes by conducting multiple small-scale experiments simultaneously. However, utilizing HTE without the intelligent selection of parameters and experiments can still be both costly and challenging. The integration of artificial intelligence (AI) tools, particularly active learning, has greatly advanced the field of materials science.19–26 This integration has given rise to AI-enabled HTE platforms, which offer an effective way to intelligently navigate chemical space using active learning techniques.15,19,27–29 Gaussian Process Regression (GPR) models are among the most popular machine learning tools for materials process optimization and active learning platforms.30–34 Their popularity stems from their flexibility, predictive power, and Bayesian nature, which enables effective uncertainty analysis.35–37
In this study, we propose a Bayesian active learning-driven high-throughput workflow to optimize the CO2(g)-based lithium brine softening method for producing solid lithium carbonate, tailored for the battery industry. Using a simplified representation of the system that only included the chemical nature of the compounds, we were able to monitor changes in the system and define traceable optimization parameters. This approach, focusing on elemental compositions rather than engaging with complex species space and detailed reaction mechanisms, enhances the speed and practical applicability of our models. We believe this method is well-suited to the demands of industrial contexts, prioritizing rapid process adjustments to meet operational needs. In the following, the design of this workflow and our key findings are discussed in detail.
2. Methods
The active learning cycle, illustrated in Fig. 1, began with high throughput experiments conducted at 66 °C. This temperature was chosen to maximize the Li2CO3 crystallization rate.7,8,12 Increasing the temperature beyond 70 °C results in loss of NH3 and CO2 gas from the system (see Section 2.1, Experimental procedures). Decreasing the temperature below 66 °C only results in a slower reaction rate, and thus a longer experiment time. Lower temperatures (25 °C and 48 °C) were explored in our experimental setup, but it was observed that within this specific context, these did not affect the yield of Li2CO3 (see Fig. S3 and Table S2†). The stable Li2CO3 yield observed across various temperatures can be attributed to the temperature-dependent equilibria involving NH3, NH4+, CO32−, HCO3−, and H2CO3. Temperature shifts induce changes in these equilibria, which regulate the availability of carbonate ions necessary for Li2CO3 formation, thereby maintaining an almost consistent Li2CO3 saturation concentration despite fluctuations in temperature.
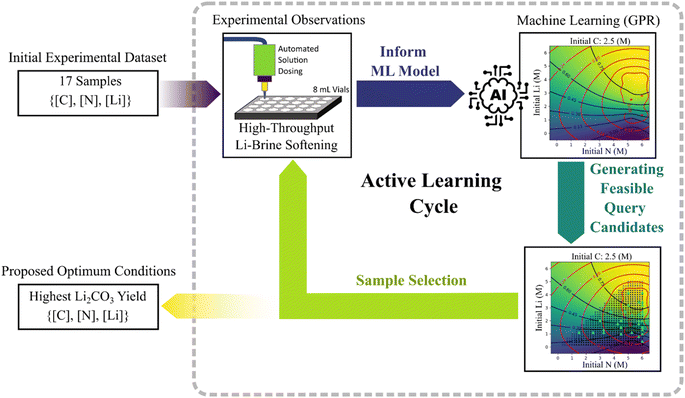 |
| Fig. 1 The active learning workflow for high-throughput Li-brine softening. | |
To effectively implement the HTE, it was necessary to extensively monitor system progress, including tracking the concentration of various species. However, considering the complexity of the system of equilibria in this study, this process required significant resources and automation, leading to increased cost and time. To mitigate these challenges, we narrowed our exploration scope to focus on major elements in the system – lithium (Li), nitrogen (N), and carbon (C) – allowing for more efficient and swift monitoring of their concentrations before and after reactions. Details of the characterization methods and related measurements are thoroughly described in Section 2.1, Experimental procedures.
Each HTE batch consisted of 24 miniature experiments, each with an 8 mL volume, and focused on the softening reaction between NH4OH, CO2(g), and LiCl. The experimental procedure, as shown in Fig. 2, involved bubbling CO2(g) into an ammonium hydroxide solution, followed by mixing it with a LiCl solution in different ratios to initiate crystallization. We conducted initial measurements of C, N, and Li concentrations in the input batches using infrared (IR) spectroscopy and ion chromatography. Subsequent determination of dissolved Li+ ion concentrations in the final solution allowed for calculating sedimented Li2CO3 and the overall yield in each vial. Further details of the HTE procedures can be found in Section 2.1, Experimental procedures.
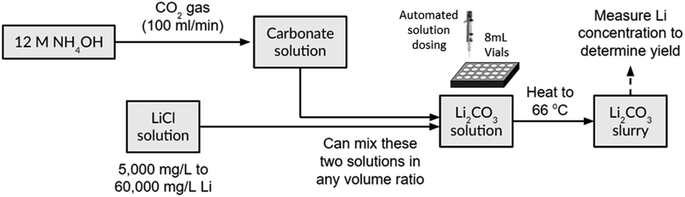 |
| Fig. 2 Block flow diagram of the high-throughput experimentation workflow and softening process for converting LiCl to Li2CO3 using CO2(g) and aqueous ammonium hydroxide (NH4OH). | |
A Gaussian Process Regression (GPR) model was used as the core of the predictive analytics tool. The model, trained using all available experimental data at each iteration, predicts lithium yields within a defined chemical space of nitrogen, carbon, and lithium (N–C–Li). This N–C–Li space, meshed within a range of 0 to 6 mol L−1, informed our data acquisition strategy, which segmented the data pool into three tiers: high lithium carbonate yield, large uncertainty in GPR predictions, and random exploration. ESI Fig. S4 and Table S3† detail the experimental limitations and the conditions viable for data acquisition.
2.1. Experimental procedures
2.1.1. Materials.
All chemicals were purchased from Sigma Aldrich (purity > 98%). DI water (>13 Mohm) was sourced from an in-house reverse osmosis unit. CO2 gas was obtained from Praxair.
2.1.2. Instrumentation.
Ion chromatography (IC) data was recorded on a Dionex ICS-6000 equipped with a CS16-4 μM, 4 × 250 mm column. Eluent consisted of isocratic 35 mM methane sulfonic acid with a flow rate of 0.640 mL min−1. Liquid dosing for high throughput experiments was carried out with an Unchained Labs Junior pipette robot. Samples were mixed and heated with an IKA ThermoShaker orbital mixer. Infrared (IR) spectroscopy measurements were made using an MT ReactIR probe.
2.1.3. Procedure for 8 mL vial experiments.
The experiments were carried out in 8 mL vials in a 24 well plate and the data that was collected is tabled in Table S1.† Solutions were made by mixing aqueous LiCl with a solution of NH4OH that has been carbonated by bubbling CO2(g) into it (see below for details on this solution). Aliquots from the NH4OH/CO2 solution were removed at various time intervals of elapsed CO2(g) bubbling time in order to collect data at different carbonation levels (measured as total carbon concentration). Another variable that was altered was the ratio between the volume of LiCl solution added and the volume of the NH4OH/CO2 solution added (this effectively varies the total nitrogen concentration in each sample). The final variable that was altered was the Li concentration in the LiCl solution. All three of these variables were varied in each sample to give rise to solutions that contained different concentrations of Li, C, and N (see Fig. 3).
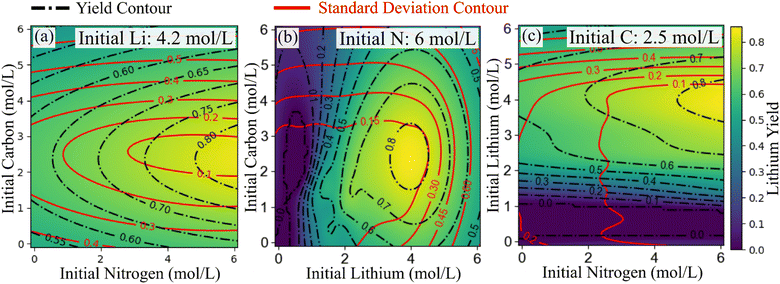 |
| Fig. 3 Lithium carbonate yield predictions for varying lithium brine softening conditions using the GPR model. This figure showcases the predicted yield across different slices of the chemical landscape, leveraging both historic and newly acquired data from the active learning cycles. Each panel visualizes the yield for a set initial concentration: (a) 4.2 mol L−1 lithium, (b) 6 mol L−1 nitrogen, and (c) 2.5 mol L−1 carbon, with other elements varying from 0 to 6 mol L−1. The color gradient indicates the lithium yield, while black contours emphasize differences in yield regions and red contours delineate areas of standard deviation within the GPR predictions, thereby representing the model's uncertainty. | |
The vials were then capped and heated to 66 °C, while mixing vigorously in an orbital mixer. Note that heating is required to speed up the crystallization – even though Li2CO3 solubility does not change with temperature in this system, the vials are still heated to complete the experiment within 24 hours. In situ IR spectroscopy was employed to determine the maximum temperature suitable for our experiments. An IR probe was inserted into an uncapped solution containing 0.5 M (NH4)2CO3 and 2 M NH4OH, and the temperature of the solution was gradually increased from 20 °C to 90 °C. The solution's temperature was held for 5 minutes at each 10-degree interval (i.e., 40 °C, 50 °C, etc.). At 70 °C, the IR signals for carbonate (1395 cm−1) and ammonia (1108 cm−1) remained stable, showing no decrease during the isothermal hold. However, at 80 °C, a decrease in the intensities of these peaks was observed, indicating the loss of ammonia and CO2(g). Consequently, the maximum temperature for the experiments was set slightly below 70 °C, at 66 °C, to prevent any loss of CO2(g) or ammonia from the system.
After 24 hours of mixing at 66 °C, a 1 mL aliquot was taken from each sample, often presenting as a slurry of Li2CO3, and filtered through a syringe filter. Cooling during filtration did not impact our results, as Li2CO3 solubility was observed to be stable across the tested temperatures in our experimental conditions, as evidenced by Fig. S3 and Table S2.† The filtered solution was then diluted and analyzed for lithium concentration using ion chromatography.
2.1.4. Preparation of the NH4OH/CO2 solutions and IR measurements.
The NH4OH/CO2 solution was prepared as follows: a 50 mL solution of 12 M NH4OH, taken directly from the stock bottle provided by Sigma, was added to a stirred reactor using a Rushton turbine impeller to enhance CO2(g) dissolution. CO2(g) was bubbled into this solution through a Tygon tube at a rate of 100 mL min−1. The solution temperature was maintained at 25 °C with a stirring rate of 500 rpm. Every 15 minutes, 100 μL aliquots were taken from the reactor and diluted into a tube containing 2.5 mL of 1 M NaOH and 7.4 mL DI water (totaling 10 mL, 100× dilution). This dilution ensured that all dissolved carbon converted to carbonate, allowing accurate measurement by the IR probe. The total carbon concentration in each 10 mL diluted sample was determined by ReactIR, specifically using the peak height of the carbonate band at approximately 1395 cm−1 (refer to Fig. S2†) via a calibration curve created with K2CO3. The carbonate concentration in the diluted sample was then multiplied by 100 to calculate the total carbon concentration in the original NH4OH/CO2 solution at the time of aliquot sampling. This data is visually represented in Fig. S1.† After 120 minutes of CO2(g) bubbling, the C to N ratio stabilized at 1
:
2 (6 M C and 12 M N), indicating stoichiometric reaction between ammonia and CO2(g), with no further addition of CO2(g) required beyond this point.
For high throughput experiments, the above procedure was repeated, except aliquots were instead removed at various other time intervals (other than every 15 minutes) based on what the predicted total carbon concentration would be at that time (see Fig. S1†). The total C concentration in these aliquots was extrapolated from the data shown in Fig. S1.†
3. Results and discussions
The AI-enhanced HTE approach facilitated a comprehensive exploration of the lithium brine softening process, effectively predicting lithium carbonate yields within the initial N–C–Li parameter space, as depicted in Fig. S5.† Remarkably, just two iterations of AI-driven HTE cycles were sufficient to identify yield values of 83% surpassing the 65% yield reported from traditional lab techniques. This yield is 10% higher than the highest yield reported by Y. Sun et al. for Li2CO3 production using CO2(g).38 Notably, the optimized 83% Li2CO3 yield achieved in this study not only matches or exceeds yields obtained using combinations of CO2(g) with sodium- or potassium-hydroxide but also, does so at 20 to 30 degrees Celsius lower.7,39–41 This lower temperature approach not only reduces energy consumption but also attains yields that are either higher or comparable, while efficiently removing excess sodium and potassium impurities from the material matrix and further minimizing the carbon footprint.
Slices of the explored chemical space (Fig. 3) highlight conditions for maximizing lithium carbonate yields as predicted by our AI-enhanced HTE approach. Despite the potential for even higher yields suggested by active learning, experimental constraints, such as pipe blockages and sedimentation in the vials due to high lithium carbonate concentrations, limited further exploration. However, these observations hinted at further potential for improvement.
As illustrated in Fig. 3, our active learning model underscores the significance of high initial lithium concentrations for achieving superior yields. It suggests that optimal lithium concentrations are slightly below the stoichiometric ratio of lithium to carbon in Li2CO3, ideally at 4.2 molar for initial lithium and approximately 2.5 molar for initial carbon, suggesting an excess of carbon. In addition, Fig. 3 shows that achieving high yields requires nitrogen concentrations to significantly exceed stoichiometric expectations for CO2(g) capture, contrary to the initial chemical intuition of equivalent nitrogen and carbon concentrations. Interestingly, higher initial carbon concentrations reduced yield, defying the expectation of increased yield from higher carbonate concentration as per Le Chatelier's principle. This reduction in yield is likely due to a drop in pH following CO2(g) dissolution, where each mole of carbon releases two moles of protons during carbonic acid neutralization.
To evaluate the performance of our Gaussian process regression models, we monitored the uncertainty of predictions across iterations. The evolving landscape of the predicted yield space is presented in Fig. S6.† A notable decrease in prediction uncertainty after the first active learning cycle is evident in Fig. S6,† underscoring the method's ability to rapidly identify optimal initial reaction conditions in the space of N–C–Li. The second iteration did not show a significant reduction in prediction uncertainty, indicating that this cycle focused more on refining and validating the model's initial predictions. This is corroborated by trends in Fig. S6,† where the area with low prediction uncertainty expands, but the yield landscape remains relatively unchanged. Furthermore, we detail the contribution of each tier of our data acquisition strategy in enhancing model performance and information gain in Section 6 of the ESI (Fig. S8).† Section 7 of the ESI† explores the performance comparison between the GPR model and other commonly used models across various iterations of our study, as illustrated in Fig. S9 and S10.†
4. Conclusions
In summary, this study demonstrates that active learning can accelerate the optimization of chemical reactions occurring in complex systems of equilibria. Reducing the chemical space to key elements has proven instrumental in enabling swift and effective system optimization. By simplifying the chemical space, we were able to define and track reaction parameters, which led to the development of our AI-enhanced HTE workflow. Aligned with the established performance of GPR models reported in materials optimization processes,20,31–34,37 our active learning workflow also benefited from this powerful methodology. This innovative method facilitated efficient exploration and optimization of the chemical space, culminating in lithium carbonate yields exceeding 83% in just a single iteration of our AI-boosted HTE cycle. This yield marks a substantial improvement over existing yields reported in the literature for Li2CO3 production from LiCl brines using NH4OH and CO2(g).7,38 Looking ahead, the methodologies and insights from this study hold wide applicability, extending to various reactions and fields within material science. Future endeavors will focus on further refining these AI-driven techniques, applying them to other complex chemical processes, and enhancing their scalability and adaptability to meet diverse industrial requirements.
Data availability
The experimental data supporting this article are detailed in Table S1 of the ESI,† which also includes additional information on experimental procedures and complementary analyses. All experimental data, AI-generated experiment suggestions, executable Python scripts, and resources necessary for reproducing the paper's figures and analyses are hosted on our dedicated GitHub repository and archived on Zenodo. For full access to these materials and more details, please visit our GitHub Repository at https://github.com/shmouses/AI-enabled-HTE-Li-Production and the Zenodo Archive with DOI: https://doi.org/10.5281/zenodo.11661727.
Conflicts of interest
The authors declare that there are no competing financial interests or conflicts of interest.
Acknowledgements
The authors gratefully acknowledge funding from the Critical Minerals Research, Development, and Demonstration (CMRDD) Program administered by Natural Resources Canada. We also extend our gratitude to Standard Lithium for their financial support and valuable discussions that have guided the project's design. Additionally, Telescope Innovations thanks the Mining Innovation Commercialization Accelerator (MICA) for financial support related to this project. We sincerely appreciate the reviewers for their constructive feedback, which has contributed to the refinement of the manuscript.
References
- F. Meng, J. McNeice, S. S. Zadeh and A. Ghahreman, Review of Lithium Production and Recovery from Minerals, Brines, and Lithium-Ion Batteries, Miner. Process. Extr. Metall. Rev., 2021, 42(2), 123–141, DOI:10.1080/08827508.2019.1668387.
- L. Li, V. G. Deshmane, M. P. Paranthaman, R. Bhave, B. A. Moyer and S. Harrison, Lithium Recovery from Aqueous Resources and Batteries: A Brief Review, Johnson Matthey Technol. Rev., 2018, 62(2), 161–176 CrossRef CAS.
- Y. Ma, M. Svärd, X. Xiao, J. M. Gardner, R. T. Olsson and K. Forsberg, Precipitation and Crystallization Used in the Production of Metal Salts for Li-Ion Battery Materials: A Review, Metals, 2020, 10(12), 1609 CrossRef CAS.
- W. Yang, L. Zhou, J. Dai, L. Zhou, M. Zhang, C. Xie, H. Hao, B. Hou, Y. Bao and Q. Yin, Crystallization of Lithium Carbonate from Aqueous Solution: New Insights into Crystal Agglomeration, Ind. Eng. Chem. Res., 2019, 58(39), 18448–18455, DOI:10.1021/acs.iecr.9b03644.
- S. Zhao, J. Gao, S. Ma, C. Li, Y. Ma, Y. He, J. Gong, F. Zhou, B. Zhang and W. Tang, Mechanism and Modelling of Reactive Crystallization Process of Lithium Carbonate, Processes, 2019, 7(5), 248 CrossRef CAS.
-
J. E. Hein and J. P. Kennepohl, Process and Method for Refining Lithium Carbonate Starting from an Impure Lithium Chloride Solution, US Pat., US20210180153A1, 2024, https://patents.google.com/patent/US20210180153A1/en Search PubMed.
- S. Kim, H. Yoon, T. Min, B. Han, S. Lim and J. Park, Carbon Dioxide Utilization in Lithium Carbonate Precipitation: A Short Review, Environ. Eng. Res., 2024, 29(3), 230553 CrossRef.
- M. Tian, Z. Wang, J. Cao, J. Guo and X. Gong, Insight into Lithium Carbonate Crystallization in the Mild Reaction System LiCl-NH3·H2O-CO2 by Stabilizing the Solution with NH3·H2O, J. Cryst. Growth, 2019, 520, 46–55 CrossRef CAS.
-
Y. Tanimura, K. Mitsuhashi, R. Kawarabuki, M. Kawata and Y. Yamaguchi, Method for Producing Lithium Carbonate, US Pat., US8920763B2, 2024, https://patents.google.com/patent/US8920763B2/en Search PubMed.
- A. D. Ryabtsev, L. T. Menzheres, A. A. Kurakov and E. P. Gushchina, Interaction of Ammonium Bicarbonate with Lithium Chloride Solutions, Theor. Found. Chem. Eng., 2006, 40(6), 649–654, DOI:10.1134/S0040579506060157.
- R. Zevenhoven, S. Eloneva and S. Teir, Chemical Fixation of CO2 in Carbonates: Routes to Valuable Products and Long-Term Storage, Catal. Today, 2006, 115(1–4), 73–79 Search PubMed.
- M. Tian, J. Guo, Z. Wang, J. Cao and X. Gong, Synergetic Effect of Secondary Nucleation and Growth on the Lithium Carbonate Particle Size in the Gas–Liquid Reactive Crystallization of LiCl–NH3·H2O–CO2, Particuology, 2020, 51, 10–17, DOI:10.1016/j.partic.2019.10.006.
- Z. Zhou, F. Liang, W. Qin and W. Fei, Coupled Reaction and Solvent Extraction Process to Form Li2CO3: Mechanism and Product Characterization, AIChE J., 2014, 60(1), 282–288, DOI:10.1002/aic.14243.
- T. C. Malig, L. P. E. Yunker, S. Steiner and J. E. Hein, Online High-Performance Liquid Chromatography Analysis of Buchwald–Hartwig Aminations from within an Inert Environment, ACS Catal., 2020, 10(22), 13236–13244, DOI:10.1021/acscatal.0c03530.
- Y. Wang, B. Goh, P. Nelaturu, T. Duong, N. Hassan, R. David, M. Moorehead, S. Chaudhuri, A. Creuziger, J. Hattrick-Simpers, D. J. Thoma, K. Sridharan and A. Couet, Integrated High-Throughput and Machine Learning Methods to Accelerate Discovery of Molten Salt Corrosion-Resistant Alloys, Adv. Sci., 2022, 9(20), 2200370, DOI:10.1002/advs.202200370.
- M. L. Green, C. L. Choi, J. R. Hattrick-Simpers, A. M. Joshi, I. Takeuchi, S. C. Barron, E. Campo, T. Chiang, S. Empedocles and J. M. Gregoire, Fulfilling the Promise of the Materials Genome Initiative with High-Throughput Experimental Methodologies, Appl. Phys. Rev., 2017, 4(1), 011105 Search PubMed.
- B. DeCost, H. Joress, S. Sarker, A. Mehta and J. Hattrick-Simpers, Towards Automated Design of Corrosion Resistant Alloy Coatings with an Autonomous Scanning Droplet Cell, JOM, 2022, 74(8), 2941–2950 CrossRef.
- M. Shevlin, Practical High-Throughput Experimentation for Chemists, ACS Med. Chem. Lett., 2017, 8(6), 601–607 CrossRef CAS PubMed.
- R. K. Vasudevan, K. Choudhary, A. Mehta, R. Smith, G. Kusne, F. Tavazza, L. Vlcek, M. Ziatdinov, S. V. Kalinin and J. Hattrick-Simpers, Materials Science in the Artificial Intelligence Age: High-Throughput Library Generation, Machine Learning, and a Pathway from Correlations to the Underpinning Physics, MRS Commun., 2019, 9(3), 821–838 CrossRef CAS PubMed.
- B. L. DeCost, J. R. Hattrick-Simpers, Z. Trautt, A. G. Kusne, E. Campo and M. L. Green, Scientific AI in Materials Science: A Path to a Sustainable and Scalable Paradigm, Mach. Learn.: Sci. Technol., 2020, 1(3), 033001 Search PubMed.
- J. Li, K. Lim, H. Yang, Z. Ren, S. Raghavan, P.-Y. Chen, T. Buonassisi and X. Wang, AI Applications through the Whole Life Cycle of Material Discovery, Matter, 2020, 3(2), 393–432 CrossRef.
- C. López, Artificial Intelligence and Advanced Materials, Adv. Mater., 2023, 35(23), 2208683, DOI:10.1002/adma.202208683.
- S. Sun, N. T. P. Hartono, Z. D. Ren, F. Oviedo, A. M. Buscemi, M. Layurova, D. X. Chen, T. Ogunfunmi, J. Thapa, S. Ramasamy, C. Settens, B. L. DeCost, A. G. Kusne, Z. Liu, S. I. P. Tian, I. M. Peters, J.-P. Correa-Baena and T. Buonassisi, Accelerating Photovoltaic Materials Development via High-Throughput Experiments and Machine-Learning-Assisted Diagnosis, Joule, 2019, 3(6), 1437–1451, DOI:10.1016/j.joule.2019.05.014.
- S. Back, A. Aspuru-Guzik, M. Ceriotti, G. Gryn'ova, B. Grzybowski, G. Ho Gu, J. Hein, K. Hippalgaonkar, R. Hormázabal, Y. Jung, S. Kim, W. Youn Kim, S. Mohamad Moosavi, J. Noh, C. Park, J. Schrier, P. Schwaller, K. Tsuda, T. Vegge, O. A. v. Lilienfeld and A. Walsh, Accelerated Chemical Science with AI, Digital Discovery, 2024, 3(1), 23–33, 10.1039/D3DD00213F.
- S. S. Mousavi M, A. Pofelski and G. Botton, EELSpecNet: Deep Convolutional Neural Network Solution for Electron Energy Loss Spectroscopy Deconvolution, Microsc. Microanal., 2021, 27(S1), 1626–1627, DOI:10.1017/S1431927621005997.
- S. S. Mousavi M, A. Pofelski, H. Teimoori and G. A. Botton, Alignment-Invariant Signal Reality Reconstruction in Hyperspectral Imaging Using a Deep Convolutional Neural Network Architecture, Sci. Rep., 2022, 12(1), 17462 Search PubMed.
- F. Ren, L. Ward, T. Williams, K. J. Laws, C. Wolverton, J. Hattrick-Simpers and A. Mehta, Accelerated Discovery of Metallic Glasses through Iteration of Machine Learning and High-Throughput Experiments, Sci. Adv., 2018, 4(4), eaaq1566, DOI:10.1126/sciadv.aaq1566.
- J. K. Bunn, S. Han, Y. Zhang, Y. Tong, J. Hu and J. R. Hattrick-Simpers, Generalized Machine Learning Technique for Automatic Phase Attribution in Time Variant High-Throughput Experimental Studies, J. Mater. Res., 2015, 30(7), 879–889, DOI:10.1557/jmr.2015.80.
- A. Wang, H. Liang, A. McDannald, I. Takeuchi and A. G. Kusne, Benchmarking Active Learning Strategies for Materials Optimization and Discovery, Oxford Open Mater. Sci., 2022, 2(1), itac006 Search PubMed.
-
C. K. Williams and C. E. Rasmussen, Gaussian Processes for Machine Learning, MIT Press, Cambridge, MA, 2006, vol. 2 Search PubMed.
- J. Chen, L. Kang and G. Lin, Gaussian Process Assisted Active Learning of Physical Laws, Technometrics, 2021, 63(3), 329–342, DOI:10.1080/00401706.2020.1817790.
- M. M. Noack, G. S. Doerk, R. Li, J. K. Streit, R. A. Vaia, K. G. Yager and M. Fukuto, Autonomous Materials Discovery Driven by Gaussian Process Regression with Inhomogeneous Measurement Noise and Anisotropic Kernels, Sci. Rep., 2020, 10(1), 17663, DOI:10.1038/s41598-020-74394-1.
- E. Uteva, R. S. Graham, R. D. Wilkinson and R. J. Wheatley, Active Learning in Gaussian Process Interpolation of Potential Energy Surfaces, J. Chem. Phys., 2018, 149(17), 174114, DOI:10.1063/1.5051772.
- V. L. Deringer, A. P. Bartók, N. Bernstein, D. M. Wilkins, M. Ceriotti and G. Csányi, Gaussian Process Regression for Materials and Molecules, Chem. Rev., 2021, 121(16), 10073–10141, DOI:10.1021/acs.chemrev.1c00022.
- A. E. Gongora, B. Xu, W. Perry, C. Okoye, P. Riley, K. G. Reyes, E. F. Morgan and K. A. Brown, A Bayesian Experimental Autonomous Researcher for Mechanical Design, Sci. Adv., 2020, 6(15), eaaz1708, DOI:10.1126/sciadv.aaz1708.
-
A. Biswas, Y. Liu, N. Creange, Y.-C. Liu, S. Jesse, J.-C. Yang, S. V. Kalinin, M. A. Ziatdinov and R. K. Vasudevan, A Dynamic Bayesian Optimized Active Recommender System for Curiosity-Driven Human-in-the-Loop Automated Experiments, arXiv, 2023, preprint, arXiv:2304.02484, DOI:10.48550/arXiv.2304.02484.
- M. Ziatdinov, Y. Liu, K. Kelley, R. Vasudevan and S. V. Kalinin, Bayesian Active Learning for Scanning Probe Microscopy: From Gaussian Processes to Hypothesis Learning, ACS Nano, 2022, 16(9), 13492–13512, DOI:10.1021/acsnano.2c05303.
- Y. Sun, X. Song, J. Wang and J. Yu, Preparation of Li2CO3 by Gas-Liquid Reactive Crystallization of LiOH and CO2, Cryst. Res. Technol., 2012, 47(4), 437–442, DOI:10.1002/crat.201100571.
- G. Battaglia, L. Berkemeyer, A. Cipollina, J. L. Cortina, M. Fernandez De Labastida, J. Lopez Rodriguez and D. Winter, Recovery of Lithium Carbonate from Dilute Li-Rich Brine via Homogenous and Heterogeneous Precipitation, Ind. Eng. Chem. Res., 2022, 61(36), 13589–13602, DOI:10.1021/acs.iecr.2c01397.
- B. Han, R. A. U. Haq and M. Louhi-Kultanen, Lithium Carbonate Precipitation by Homogeneous and Heterogeneous Reactive Crystallization, Hydrometallurgy, 2020, 195, 105386 CrossRef CAS.
- Y. Jiang, C. Liu, X. Zhou, P. Li, X. Song and J. Yu, Toward CO2 Utilization: Gas–Liquid Reactive Crystallization of Lithium Carbonate in Concentrated KOH Solution, Energy Sources, Part A, 2021, 43(24), 3332–3344, DOI:10.1080/15567036.2019.1587068.
|
This journal is © The Royal Society of Chemistry 2024 |
Click here to see how this site uses Cookies. View our privacy policy here.