DOI:
10.1039/D4DD90010C
(Editorial)
Digital Discovery, 2024,
3, 1081-1085
Perspective on AI for accelerated materials design at the AI4Mat-2023 workshop at NeurIPS 2023
Abstract
Applications of advanced artificial intelligence (AI) methods in the materials science domain has grown significantly in recent years resulting in numerous research efforts spanning diverse aspects of materials design, materials synthesis, and materials characterization. The AI for Accelerated Materials Design (AI4Mat) workshop at NeurIPS 2023 featured many of the ongoing major research themes by bringing together an international interdisciplinary community of researchers and enthusiasts across academia, industry, and national labs. The goal of these discussions was to highlight cutting-edge work from active researchers in these fields and uncover major impactful research problems that the community can jointly address. In this article, the AI4Mat-2023 organizing committee showcases the major developments in the field as well as ongoing research challenges where innovative solutions can bring transformative changes to the state-of-the-art in applying AI for accelerated materials design. The editors of Digital Discovery are pleased to feature this overview, and a selection of these manuscripts, in a new themed collection.
1 AI for accelerated materials design (AI4Mat)
Advancements in the field of artificial intelligence (AI), particularly in deep learning,1 has made accelerating the discovery, development, and understanding of materials more and more tractable. This has been primarily achieved by furthering the research at the intersection of AI and materials, which has lead to the diversity and growth of both the domains. Exploring this intersection was the inspiration for the 1st AI for Accelerated Materials Design (AI4Mat) workshop held at NeurIPS 2022.2 AI4Mat-2022 first introduced the concept of self-driving materials laboratories – described in Section 2 – to the mainstream AI community, fostering deep technical discussion along the intersection of AI and materials science, both in a computational and real-world experimental setting. Some of the insights that emerged from AI4Mat-2022 included addressing the fragmented nature of data collection, processing and usage in materials design which creates major scaling challenges. Moreover, AI4Mat-2022 highlighted the major challenges associated with experimental workflows, as well as the need for further innovations to make experimental materials science amendable for AI integration. Discussion at AI4Mat-2022 suggested worthwhile future directions, such as focusing on sample efficiency for materials synthesis and building interpretable methods for materials characterization. The insights of AI4Mat-2022 helped inform many of the themes of AI4Mat-2023, which are described in more detail in Section 3, with increased emphasis on how AI can help drive materials design from simulation to physical experiments (Sim2Mat Lightning Talk Panel) and how large language models (LLMs) can serve as a platform for a plethora of materials challenges. As such, the focus areas and research presented at AI4Mat-2023 built upon the insights and research work of AI4Mat-2022 at a time of significant growth in the research community. While AI4Mat-2022 included 40 accepted posters, AI4Mat-2023 had over 80 posters representing a doubling in accepted and submitted work as well as a significantly greater number of workshop attendees representing both AI and materials science researchers.
1.1 Can AI design materials?
Recent research in the field, including much of the research presented at the workshop, has seen a significant number of new deep learning methods proposed for modeling materials properties3–5 and bringing the power of generative models to create previously unknown materials including small molecules,6–9 proteins10 and periodic crystal structures.11,12 While the aforementioned works represent great progress in deploying AI for outstanding challenges, much future work remains to truly leverage the power of advanced AI for accelerated materials design spanning in silico simulation & design, efficient chemical synthesis, and precise material characterization. The posters and spotlights presented in the workshop are already showing emerging work in those directions with methods being developed to address specific challenges, whether it be application-driven design, improving sample efficiency in synthesis or developing methods to characterize materials based on real-world experimental data sources. Additionally, as highlighted in workshop discussions there are still opportunities for future research in designing more complex materials systems, especially at the nanoscale, which more closely mimic the complexity of materials systems deployed today for diverse applications.
2 AI4Mat focus – closing the discovery loop
The unifying themes of AI4Mat centers on creating a positive feedback loop based on three interacting themes as shown in Fig. 1: (1) AI-guided design—which centers on automating what kind of materials should be made based on their desired application and how materials can be quickly evaluated in silico using advanced computational tools, including AI models; (2) automated chemical synthesis—which centers on bringing materials design into the physical world as efficiently as possible using AI-enhanced automation; (3) automated material characterization—which focuses on analyzing materials that have been synthesized to gain a comprehensive idea of their structure, properties and behavior.
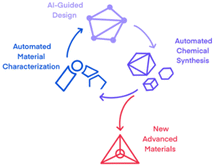 |
| Fig. 1 Self-driving materials laboratories leveraging (1) AI-guided design; (2) automated chemical synthesis; (3) automated material characterization. | |
While there have been recent advances in showcasing end-to-end automation of self-driving materials laboratories,13 including research work at AI4Mat-2022 for thin-film coatings by Rupnow et al.,14 much work remains to make the self-driving laboratories framework accessible to a broad range of materials design, synthesis, and characterization cases. Additionally, AI-focused venues like NeurIPS often focus primarily on in silico based methods that mostly fit into the AI-guided design category while materials science communities often focus on concrete synthesis and characterization procedures for very targeted applications. This trend was also observed in AI4Mat-2022 where the vast majority of accepted papers focused on AI-guided design given the representation of the AI-focused research at NeurIPS. With the additional focus on growing all research themes in AI4Mat-2023, Automated Materials Characterization with real-world experimental data showed significantly more representation suggesting a promising direction for more integration of interdisciplinary research.
2.1 AI4Mat-2022 – what remains unsolved?
The AI4Mat-2022 workshop discussion, spotlight presentations and posters identified major gaps at the fragmented nature of data collection, which is particularly pronounced when trying to jointly understand experimental and simulation data.15 In addition to data scarcity, the discussions emphasized the potential benefit of having human scientists in the loop to accelerate diverse types of discovery and analysis procedures. The research needs uncovered at AI4Mat-2022 help inform the discussion and research efforts presented at AI4Mat-2023 to advance the state-of-the-art while also growing the interdisciplinary community needed to tackle these complex challenges.
3 AI4Mat-2023
AI4Mat-2023 aimed to build upon the insights from AI4Mat-2022, to showcase new research challenges given the advances in the field and to highlight recent work drawing from a diverse set of established and early career researchers including graduate students. Given the strong need to connect in silico computational materials design with real-world experiments through synthesis and characterization, AI4Mat-2023 started with a focused discussion on this topic during the Sim2Mat Lightning Talk Panel. The second focus area centered on the role of LLMs in materials design due to the emergence of LLMs as powerful tools for technological challenges, including initial work on materials design.16–19
3.1 Sim2Mat lightning talk panel
The Sim2Mat Lightning Talk Panel included researchers from national labs (Rama Vasudevan – Oak Ridge National Laboratory, Maria K Chan – Argonne National Laboratory) and industry (Vijay Narasimhan – Merck KGaA, Darmstadt, Germany) with the lightning talks providing concrete examples for real-world applications of AI in materials design and suggestions for future research work. While the panel presentations and discussion had numerous interesting insights, some joint themes emerged from multiple panel members:
• Success of AI-infused materials design involves closing the loop between design, simulation and synthesis + characterization experiments leading to end-to-end verification and agreement of all the modalities. AI techniques have the potential to expand the capabilities of each of the constituent processes for a given case while also providing connective tissue with digital tools that can be applied at large scale.
• A toolbox of AI-inspired ideas can enable continuous discovery of new materials that act as scalable platforms for new technologies, such as new semiconductors for computer hardware, small molecules and protein macromolecules for healthcare and biotechnology, and electrochemical materials for energy generation and storage. Targeted algorithms with end-to-end digital integration enable materials innovations to scale to production more quickly leading to faster deployment of new materials systems. This can be particularly useful when designing materials that span multiple scales, such as nanoscale materials, where different physics and chemistry become relevant at different stages of the design process.
• At the intersection of AI and materials science, both fields can enable each other. While materials science can inspire the creation of new targeted AI methods, such as diffusion models based on the physics of mass transport and new symmetry-informed data representations and neural network architectures, AI methods can inspire the development of new materials science workflow such as designing materials based on human feedback similar to reinforcement learning from human feedback (RLHF) that was used to train commercial LLMs.
3.2 Large language models (LLMs) for materials design fireside chat
The second panel of AI4Mat-2023 focused on the role, capabilities, and limitations of LLMs in the context of materials design. The panel discussion featured experts from both academic (Gabe Gomes – Carnegie Mellon University) and industry research organizations, including startups (Andrew White – Future House) and large corporations (Gowoon Cheon – Google Research). The panel discussed various applications for LLMs, how they can be adapted for practical materials science along with a lengthy discussion on the role of data representation in concrete applications of AI tools in materials science not necessarily limited to the various modalities of language and LLMs. Throughout the discussion, a set of insights emerged that highlighted the complex evolving role of LLMs and their interconnected application to materials design:
• LLMs are challenging the notion that structured data is the most effective representation for various fields, including materials science. Given that most human knowledge in materials science, chemistry, and physics has been recorded in natural language and that language enables communication of real-world actions, such as materials synthesis and analysis procedures, the panel advocated that language and other less structured data types deserve greater exploration to better understand their utility.
• LLMs can greatly accelerate tasks related to science by providing scientists with easier access to knowledge and accelerating the ability for humans and machines to use tools more effectively. Given LLMs' ability to understand and produce functional code for many applications, LLMs could provide useful interfaces for human–machine and machine–machine interactions in materials science. Additionally, LLMs might also provide a platform to distil and preserve expert scientist knowledge into text, thereby enabling a greater range of scientists to access expert knowledge more efficiently.
• The development of LLMs in materials science can serve as a point of proof for the applications of LLMs in other domains given the availability of reliable measurements in materials science. The availability of these concrete measurements can serve as a point of proof to mitigate common issues of LLMs, such as hallucinations and unpredictable fragility for various applications. While hallucinations themselves continue to be a major challenge for LLMs, especially in scientific domains, the panel also raised the potential of leveraging controlled hallucinations for exploration in materials design applications.
3.3 Spotlight
AI4Mat-2023 included 18 ‘spotlight’ submitted papers that served to highlight the emerging work of primarily junior researchers, in this emerging interdisciplinary research community. Thematically, the spotlights spanned new proposed benchmarks and methods for training supervised models,20,21 accelerating chemical synthesis and materials characterization by infusing AI,22–27 showcasing the capabilities of LLMs and generative models for materials science,28–34 as well as new developments on machine learning potentials for in silico simulations.35–37
The spotlights touched on, and in some cases expanded, upon many of the themes that were uncovered in the panel sessions. Examples include spotlights proposing new benchmarks that can address impactful challenges in materials science, spotlights showcasing the capabilities of LLMs for various materials science tasks and new research introducing the capabilities of state-of-the-art generative models into materials design for diverse applications. On generative methods in particular, the spotlights in AI4Mat-2023 provided new ideas beyond the recent development of the large-scale generative models described in Section 1, such as GFlowNets and LLMs as autoregressive generative models.
3.4 Digital Discovery themed collection
In partnership with the Royal Society of Chemistry’s Digital Discovery journal, AI4Mat-2023 launched a themed collection of research papers drawn from some of the most exciting and high-quality AI4Mat-2023 paper submissions. AI4Mat-2023 included the opportunity and requirements for the themed collection in its call for submissions inviting authors to contribute to both the workshop and the themed collection. In their submissions, paper authors specified whether they wanted their paper to be considered as part of the themed collection, which was indicated to AI4Mat-2023 reviewers. Based on a round of double-blind reviews from AI4Mat reviewers, the AI4Mat-2023 committee invited a subset of accepted workshop papers to submit a revised version of their manuscript to the themed collection. The authors of the invited papers then revised their manuscripts based on the initial reviewer feedback by the workshop camera-ready deadline. Following the workshop, the invited papers underwent a second round of single-blind reviews by relevant domain experts. Ultimately, based on the reviews from the first double-blind round and the second single-blind round, the AI4Mat-2023 committee and Digital Discovery editorial team, jointly selected the final set of papers for the themed collection. Lastly, the selected papers underwent a data review in line with the journal's policies before publication, assessing the supporting code and data provided. Journal submission dates for manuscripts are set to the date they were selected for the collection.
Similar to the AI4Mat spotlit articles, the collection of papers in this themed collection includes high-quality research drawn from AI4Mat submissions that detail the diverse research areas at the intersection of advanced AI and materials science. On the whole, the papers in the themed collection form a broad perspective on the intersection of AI and materials science, which we hope will continue to expand in the future. Some of the themed collection papers outline new methods for modeling materials, drawing from solid-state structure representation (https://doi.org/10.1039/D4DD00018H), machine learning methods to characterize molecules based on molecular spectra experiments (https://doi.org/10.1039/D4DD00021H), and a new molecular representation that spans modeling and generation of a diverse of molecular design cases (https://doi.org/10.1039/D4DD00019F). Additionally, themed collection papers propose generative methods to solve practical materials design challenges, including: offline reinforcement for crystal structure discovery (https://doi.org/10.1039/D4DD00024B), applying GFlowNets for discovering diverse equilibrium molecular structures (https://doi.org/10.1039/D4DD00023D), as well as new metal–organic framework compounds (https://doi.org/10.1039/D4DD00020J). Lastly, the themed collection continues to advance the field by proposing new benchmarks that span a wide range of open problems in machine-learning potentials (https://doi.org/10.1039/D4DD00027G), continuum-scale materials systems (https://doi.org/10.1039/D4DD00028E) and information extraction from various modalities included in scientific articles (https://doi.org/10.1039/D4DD00032C). Taken together, the themed collection papers represent a subset of the scope of defining practical challenges for AI to solve in materials, while also continuing to push the state-of-the-art in developing and evaluating new AI methods.
4 What's next?
As we look to further grow the research intersection of AI and materials science along with the community of researchers working on this emerging and impactful work, we believe that there is a lot of exciting work to be continued both from a technical and a community building perspective. Concretely, for future AI4Mat workshops, we aim to address the following:
• Increase the representation of the materials synthesis community given that it was the least represented community in AI4Mat-2023. Additionally, we aim to grow the global reach of AI4Mat to ensure that diversity remains a priority as AI4Mat and the community of researchers at the intersection of AI and materials science continues to grow.
• While AI for materials design is still in its infancy and many challenges remain, there are clear examples of how the field is having a growing impact on both research and industrial applications. Digital tools are becoming an increasingly important part of the corporate ecosystem while researchers at academic, government, and corporate institutions continue to push the boundary of end-to-end systems for materials discovery and production. As such, integration of digital tools and system level automation solutions is an important effort to push the state-of-the-art in accelerated materials design. Given the ever-growing complexity of the interface of various experimental and simulation systems, as well as the continued development of data structures and data representations, we believe that data management systems will become essential for developing scalable and generalizable systems for AI-infused materials discovery.
• As the intersection of materials science and AI continue to grow, it will be interesting to see how ideas from both fields continue to cross-pollinate. Similar to how materials modeling problems have inspired the design of new AI models in geometric deep learning, AI has inspired the application of novel experimental and simulation methodologies in materials such as materials design by RLHF. We encourage researchers to pay special attention to cross-pollinations that can potentially disrupt these fields.
• The interfaces of AI, materials simulations, and experimental equipment including both synthesis and characterization are becoming more accessible in part due to the power of LLMs which have the potential to become a potent tool in human–machine and machine–machine communications. Thus, the role of emerging AI disciplines such as human-in-the-loop AI, and human-centered AI in materials discovery is likely to emerge as an important area for future materials science research.
Conflicts of interest
There are no conflicts of interest to declare.
Acknowledgements
We acknowledge the contribution of invited speakers to AI4Mat-2023 at NeurIPS 2023. For the Sim2Mat Lightning Panel, we acknowledge: Rama Vasudevan from Oak Ridge National Laboratory, Maria K. Chan from Argonne National Laboratory, and Vijay Narasimhan from EMD Electronics, all of whom gave talks and participated in a panel discussion. For the LLM Fireside Chat we acknowledge: Andrew White from the University of Rochester & Future House, Gowoon Cheon from Google Research, and Gabe Gomes from Carnegie Mellon University, all of whom participated in a panel discussion. We would also like to acknowledge AI4Mat-2023 advisor Alán Aspuru-Guzik who provided valuable suggestions for designing various aspects of the workshop.
Notes and references
- Y. LeCun, Y. Bengio and G. Hinton, Nature, 2015, 521, 436–444 CrossRef CAS PubMed.
-
S. Miret, M. Skreta, B. Sanchez-Lengelin, S. P. Ong, Z. Morgan-Chan and A. Aspuru-Guzik, AI4MAT – NeurIPS 2022, https://sites.google.com/view/ai4mat Search PubMed.
-
A. Duval, S. V. Mathis, C. K. Joshi, V. Schmidt, S. Miret, F. D. Malliaros, T. Cohen, P. Lio, Y. Bengio and M. Bronstein, arXiv, 2023, preprint, arXiv:2312.07511 Search PubMed.
-
S. Miret, K. L. K. Lee, C. Gonzales, M. Nassar and M. Spellings, Transactions on Machine Learning Research, 2023 Search PubMed.
- L. Chanussot, A. Das, S. Goyal, T. Lavril, M. Shuaibi, M. Riviere, K. Tran, J. Heras-Domingo, C. Ho and W. Hu,
et al.
, ACS Catal., 2021, 11, 6059–6072 CrossRef CAS.
-
Z. Zhu, C. Shi, Z. Zhang, S. Liu, M. Xu, X. Yuan, Y. Zhang, J. Chen, H. Cai, J. Lu, et al., arXiv, 2022, preprint, arXiv:2202.08320 Search PubMed.
- Z. Wu, B. Ramsundar, E. N. Feinberg, J. Gomes, C. Geniesse, A. S. Pappu, K. Leswing and V. Pande, Chem. Sci., 2018, 9, 513–530 RSC.
-
R. Ghugare, S. Miret, A. Hugessen, M. Phielipp and G. Berseth, arXiv, 2023, preprint, arXiv:2310.02902 Search PubMed.
-
E. Hoogeboom, V. G. Satorras, C. Vignac and M. Welling, International conference on machine learning, 2022, pp. 8867–8887 Search PubMed.
- J. L. Watson, D. Juergens, N. R. Bennett, B. L. Trippe, J. Yim, H. E. Eisenach, W. Ahern, A. J. Borst, R. J. Ragotte and L. F. Milles,
et al.
, Nature, 2023, 620, 1089–1100 CrossRef CAS PubMed.
- A. Merchant, S. Batzner, S. S. Schoenholz, M. Aykol, G. Cheon and E. D. Cubuk, Nature, 2023, 1–6 Search PubMed.
-
C. Zeni, R. Pinsler, D. Zügner, A. Fowler, M. Horton, X. Fu, S. Shysheya, J. Crabbé, L. Sun, J. Smith, et al., arXiv, 2023, preprint, arXiv:2312.03687 Search PubMed.
-
M. Sim, M. G. Vakili, F. Strieth-Kalthoff, H. Hao, R. Hickman, S. Miret, S. Pablo-García and A. Aspuru-Guzik, 2023.
- C. C. Rupnow, B. P. MacLeod, M. Mokhtari, K. Ocean, K. E. Dettelbach, D. Lin, F. G. Parlane, H. N. Chiu, M. B. Rooney and C. E. Waizenegger,
et al.
, Cell Rep. Phys. Sci., 2023, 4 CAS.
-
S. Miret, B. Sanchez-Lengelin, M. Skreta, S. P. Ong, Z. Morgan-Chan and A. Aspuru-Guzik, AI4Mat NeurIPS 2022 Workshop Recap, https://sites.google.com/view/ai4mat/ai4mat-2022 Search PubMed.
-
A. M. Bran, S. Cox, A. D. White and P. Schwaller, arXiv, 2023, preprint, arXiv:2304.05376 Search PubMed.
- D. A. Boiko, R. MacKnight, B. Kline and G. Gomes, Nature, 2023, 624, 570–578 CrossRef CAS PubMed.
-
Y. Song, S. Miret, H. Zhang and B. Liu, Findings of the Association for Computational Linguistics: EMNLP 2023, 2023, pp. 5724–5739 Search PubMed.
-
Y. Song, S. Miret and B. Liu, Proceedings of the 61st Annual Meeting of the Association for Computational Linguistics (Volume 1: Long Papers), Toronto, Canada, 2023, pp. 3621–3639 Search PubMed.
-
K. L. K. Lee, C. Gonzales, M. Nassar, M. Spellings, M. Galkin and S. Miret, AI for Accelerated Materials Design – NeurIPS 2023 Workshop, 2023 Search PubMed.
-
F. Ottomano, G. D. Felice, R. Savani, V. Gusev and M. Rosseinsky, AI for Accelerated Materials Design – NeurIPS 2023 Workshop, 2023 Search PubMed.
-
A. M. Bran, C.-H. Huang and P. Schwaller, AI for Accelerated Materials Design – NeurIPS 2023 Workshop, 2023 Search PubMed.
-
T. Nguyen, S. Agrawal and A. Grover, AI for Accelerated Materials Design – NeurIPS 2023 Workshop, 2023 Search PubMed.
-
X. Hua, R. Ahmad, J. Blanchet and W. Cai, AI for Accelerated Materials Design – NeurIPS 2023 Workshop, 2023 Search PubMed.
-
M. Schwarzer, J. Farebrother, J. Greaves, K. Roccapriore, E. Cubuk, R. Agarwal, A. Courville, M. Bellemare, S. Kalinin, I. Mordatch and P. Castro, AI for Accelerated Materials Design – NeurIPS 2023 Workshop, 2023 Search PubMed.
-
K. Shibata and T. Mizoguchi, AI for Accelerated Materials Design – NeurIPS 2023 Workshop, 2023 Search PubMed.
-
A. H. Cheng, A. Lo, S. Miret, B. Pate and A. Aspuru-Guzik, AI for Accelerated Materials Design – NeurIPS 2023 Workshop, 2023 Search PubMed.
-
N. Gruver, A. Sriram, A. Madotto, A. G. Wilson, C. L. Zitnick and Z. W. Ulissi, AI for Accelerated Materials Design – NeurIPS 2023 Workshop, 2023 Search PubMed.
-
Y. Song, S. Miret, H. Zhang and B. Liu, AI for Accelerated Materials Design – NeurIPS 2023 Workshop, 2023 Search PubMed.
-
E. Soares, V. Sharma, E. V. Brazil, R. Cerqueira and Y.-H. Na, AI for Accelerated Materials Design – NeurIPS 2023 Workshop, 2023 Search PubMed.
-
S. Yang, K. Cho, A. Merchant, P. Abbeel, D. Schuurmans, I. Mordatch and E. D. Cubuk, AI for Accelerated Materials Design – NeurIPS 2023 Workshop, 2023 Search PubMed.
-
F. Cipcigan, J. Booth, R. N. B. Ferreira, C. R. D. Santos and M. B. Steiner, AI for Accelerated Materials Design – NeurIPS 2023 Workshop, 2023 Search PubMed.
-
G. Vogel, P. Sortino and J. M. Weber, AI for Accelerated Materials Design – NeurIPS 2023 Workshop, 2023 Search PubMed.
-
Mistal, A. Hernández-García, A. Volokhova, A. A. Duval, Y. Bengio, D. Sharma, P. L. Carrier, M. Koziarski and V. Schmidt, AI for Accelerated Materials Design – NeurIPS 2023 Workshop, 2023 Search PubMed.
-
V. Bihani, U. Pratiush, S. Mannan, T. Du, Z. Chen, S. Miret, M. Micoulaut, M. M. Smedskjaer, S. Ranu and N. M. A. Krishnan, AI for Accelerated Materials Design – NeurIPS 2023 Workshop, 2023 Search PubMed.
-
J. M. Stevenson, AI for Accelerated Materials Design – NeurIPS 2023 Workshop, 2023 Search PubMed.
-
X. Fu, A. Musaelian, A. Johansson, T. Jaakkola and B. Kozinsky, AI for Accelerated Materials Design – NeurIPS 2023 Workshop, 2023 Search PubMed.
|
This journal is © The Royal Society of Chemistry 2024 |
Click here to see how this site uses Cookies. View our privacy policy here.