Application of nanopore sequencing for accurate identification of bioaerosol-derived bacterial colonies
Received
26th December 2023
, Accepted 2nd May 2024
First published on 23rd May 2024
Abstract
Bioaerosol samples are characterized by very low biomass, so culture-based detection remains a reliable and acceptable technique to identify and quantify microbes present in these samples. The process typically involves the generation of bacterial colonies by inoculating the sample on an agar plate, followed by the identification of colonies through DNA sequencing of a PCR-amplified targeted gene. The Sanger method is often the default choice for sequencing, but its application might be limited in identifying multi-species microbial colonies that could potentially form from bacterial aggregates present in bioaerosols. In this work, we compared Sanger and MinION nanopore sequencing techniques in identifying bioaerosol-derived bacterial colonies using 16S rRNA gene analysis. We found that for five out of the seven colonies examined, both techniques indicated the presence of the same bacterial genus. For one of the remaining colonies, a noisy Sanger electropherogram failed to generate a meaningful sequence, but nanopore sequencing identified it to be a mix of two bacterial genera. For the other remaining colony, the Sanger sequencing suggested a single genus with a high sequence alignment and clean electropherogram; however, the nanopore sequencing suggested the presence of a second less abundant genus. These findings were further corroborated using mock colonies, where nanopore sequencing was found to be a superior method in accurately classifying individual bacterial components in mock multispecies colonies. Our results show the advantage of using nanopore sequencing over the Sanger method for culture-based analysis of bioaerosol samples, where direct inoculation to a culture plate could lead to the formation of multispecies colonies.
Environmental significance
Culture-based detection of microbes present in bioaerosols typically involves growing microorganisms into colonies and subsequent identification by targeted gene sequencing. Sanger sequencing of the 16S rRNA gene is most commonly used for the identification of bacterial colonies formed after inoculation of bioaerosol samples on agar media. In this study, the performance of Sanger sequencing was compared with MinION nanopore sequencing for the identification of bioaerosol-derived bacterial colonies. Nanopore sequencing outperformed Sanger sequencing by detecting bacteria at higher taxonomic resolution and identifying individual bacterial components in multispecies colonies. Our findings demonstrate the potential of nanopore sequencing for microbial identification in culture-based analysis of bioaerosol and other complex environmental samples.
|
Introduction
Monitoring the presence of pathogens in bioaerosols is crucial for assessing potential health risks associated with exposure to air. Bioaerosols include a rich and diverse community of microbes, only a small number of which may be pathogenic. The low biomass of bioaerosol complicates their detection and identification and often requires the use of culture-based enrichment methods prior to other analyses.1–5 One key advantage of the culture-based detection approach is that when successfully implemented, it is able to identify even a very small number of viable organisms in the sample and can help assess the health risk posed by the ambient air in an environment.6 Due to this high sensitivity for detection, the culture-based technique is not only employed for microbial analysis of air7,8 but also for a wide variety of samples, including clinical specimens, food, and environmental samples such as soil and water.2,9–12
A common workflow for culture-based studies is the generation of colonies from microorganisms present in a sample, followed by analysis of the colonies. This is based on the assumption that the colonies are homogeneous and are generated by aggregated growth from a single microorganism. However, for bioaerosol samples, the approach can encounter certain challenges in achieving an accurate identification of the colonies. Bioaerosols are distributed over a range of sizes with up to 30% of the total number being ≥4.7 μm in diameter, suggesting potential aggregation of microbes in these larger particles.13 Indeed, microbes in the air are reported to exist as aggregates of variable size, often tightly bound to particulate matters, and thus, can potentially form multispecies colonies when bioaerosol samples are inoculated on agar media.14–16 Culture-based detection is commonly conducted by capturing bioaerosol particles on an agar plate (e.g., by depositional sampling and impaction), followed by growing viable microbes.5 However, the particle-bound or aggregated microorganisms would remain in close physical proximity on the agar plate and could potentially lead to growth without a distinct colony boundary. Indeed, the existence of multispecies colonies is reported, where distant bacterial species associate in a single colony structure with specific interactions observed between them.17,18 Although information is lacking regarding the formation of multispecies colonies from inoculation of bioaerosols, the fact that airborne microbes often exist as aggregates raises such possibility and emphasizes the need for accurate identification of microbial colonies.
The classical approach to identify microbial colonies involves a battery of biochemical tests, but that is now mostly replaced by sequencing-based techniques, which offer a rapid, accurate, and sensitive method for bacterial identification. Targeted amplicon analyses are commonly used for taxonomic classification and for studying phylogenetic relationships due to the conserved nature of essential genes.19,20 The 16S ribosomal RNA gene (henceforth abbreviated as 16S) contains nine variable regions and is present universally in bacteria and archaea, providing a robust tool for the classification of bacteria and archaea.19–23 16S amplicon analysis is widely used for the identification of bacteria and archaea; however, the implementation of different sequencing technologies can influence the resolution of the results and the scope of application.
Sanger sequencing, first introduced more than four decades ago, is still widely used and remains as one of the primary sequencing tools for the identification of microbial colonies through targeted gene amplification. Sanger sequencing is highly accurate in sequencing reads up to ∼1000 bases and is often held as a reference or standard to compare the accuracy of other sequencing techniques.24–27 One major limitation of Sanger sequencing is that only one homogeneous DNA sequence can be read by this technique, and the presence of additional sequences in the sample will impact the output.12,13 Thus, Sanger sequencing is not suitable for sequencing 16S amplicons from a sample with a mixed microbial composition. For such applications, next-generation sequencing (NGS) such as Illumina, which employs massively parallel short-read sequencing, is commonly used to classify all bacterial taxa. However, the sequencing platform allows only short-reads with a sequence length of <500 bp, restricting the coverage of the 16S gene to a maximum of two variable regions and limiting the taxonomic classification up to the genus level.28–30
Third-generation sequencing, commonly referred to the sequencing platforms offered by Oxford Nanopore Sequencing (ONT) and Pacific Biosciences (PacBio), overcomes some of the major limitations of NGS by enabling long-read sequencing.31,32 Among these techniques, MinION nanopore sequencing from ONT utilizes a protein nanopore complex to guide a DNA strand to translocate through the pore and determines the sequence from the changes in ionic conductivities as different nucleotide bases pass through the pore.32 Nanopore sequencing has significantly advanced in the last decade with improvements in sequencing accuracy and capacity. Combined with packaging in an extremely portable, inexpensive sequencing device, and relatively simple library preparation procedures, applications of nanopore sequencing have grown tremendously in recent years.33–37 The long-read capability of nanopore sequencing allows for full-length 16S gene amplicon sequencing with the ability to discriminate up to the species level in a sample of mixed bacterial composition.30 Furthermore, multiplexing the samples by barcoding enables running multiple samples on a single run, enhancing the throughput and reducing the cost. Together, these features of nanopore sequencing make it a potentially attractive procedure for the identification of bacterial colonies through 16S amplicon analysis.
In this study, we compared Sanger and nanopore sequencing for the identification of bacterial colonies derived from bioaerosol samples and explored any advantages afforded by nanopore sequencing. Targeted amplification of full-length 16S genes was conducted for individual colonies, and the amplicons were sequenced using both techniques. We investigated the accuracy of these two techniques in colony identification, especially when there is potential for the existence of multispecies colonies. The findings were further corroborated with mock multispecies colony samples.
Methods
Bioaerosol sample collection
Bioaerosol samples were collected from the Clarkson University campus using an in-house developed, portable bioaerosol sampler called TracB (Trace Aerosol sensor and Collector for Bio-particles).38 The sampler is a low-power and low-pressure drop device that uses electrostatic precipitation to capture airborne particles on a removable collection plate along with real-time monitoring of air quality parameters. Unlike the common high-pressure drop aerosol samplers (e.g., impactors and impingers), the device operates without a pump and the airflow is driven by a 12 V DC fan mounted on the device. Operating at a sampling flow rate of 10 L min−1, the device is designed for particle collection over a wide size range of 0.01–10 μm, and the collection efficiency for bacterial aerosols was found to be over 50%.38 The design of the TracB device enables it to run for an extended period, from days to several weeks, capturing airborne particles throughout this time and being able to provide a reflection of airborne microbes in the sampling window. Our preliminary work suggested that the bioaerosol sample collection of two weeks provides sufficient biomass to run sequencing analysis. This has prompted us to set our sampling time window to two weeks for both sequencing and culture-based analysis, which was followed in this study as well. It is to be noted that any potential impact of the long sampling period on the properties and fate of captured bacteria has not been investigated in this study.
Bacterial culture
After two weeks of sample collection using the TracB device, particles deposited on the collection plate were retrieved by wiping with a sterile, wet (by PBS) cotton swab. The sample was immediately inoculated on Tryptic Soy Agar (TSA, Difco) plates by gently spreading the swab over the agar media. Following sample inoculation, the TSA plates were incubated at 30 °C for 16–18 h to promote bacterial growth and colony formation before further analysis.
DNA extraction from bacterial colonies
Once bacterial colonies were formed on the agar plates inoculated with bioaerosol samples, seven visibly distinct colonies with no overlap of margins with nearby colonies were randomly selected for this study. Bacteria from a single colony were carefully picked up by a sterile inoculation loop, and DNA extraction was performed using a Fast DNA SPIN Kit for Soil (MP Biomedicals #116560200) following the manufacturer's protocol. The extraction process included a bead beating step using a MP Biomedicals FastPrep-24™ bead beater, operating at 6.0 m s−1 for 1 min. The DNA was eluted in 50 μL volume. The quality (260/280 ratio) and concentration of extracted DNA were measured using a Nanodrop spectrophotometer (Thermo Fisher Scientific) and a Quantus Fluorometer with Quantifluor ONE dsDNA dye (Promega #E4891).
Preparation of mock colony samples
The mock colony samples were prepared using pure genomic DNA from two known control bacterial taxa, namely Acinetobacter baumannii strain 2208 (ATCC 19606D-5) and Stenotrophomonas maltophilia strain 810-2 (ATCC 13637D-5). A. baumannii and S. maltophilia DNA (10 ng μL−1 in nuclease free water) were mixed at predetermined volumetric ratios (9
:
1, 1
:
1, and 1
:
9) to prepare mock multispecies colony samples with different proportional abundances of these two DNA. For example, a sample with 90% A. baumannii and 10% S. maltophilia was prepared by mixing 4.5 μL of A. baumannii DNA with 0.5 μL of S. maltophilia DNA. The mixed DNA or pure bacterial DNA were further used for Sanger and nanopore sequencing as mock colony samples.
Sanger sequencing
For Sanger sequencing, the full-length 16S gene was amplified from genomic DNA samples through the PCR using 27F (5′-GAGTTTGATCATGGCTCAG-3′) and 1492R (5′-ACGGCTACCTTGTTACGACTT-3′) primers. The PCR reaction mixture contained: 12.5 μL LUNA LongAmp Taq 2X Master Mix (NEB #M0287S), 5.5 μL Nuclease-Free Water (Thermo Fisher Scientific #AM9937), 5 μL of bacterial DNA, and 1 μL of the 27F and 1492R primers per reaction. The PCR cycling conditions used were as follows: initial denaturation at 95 °C for 1 min, followed by 26 cycles of denaturation at 95 °C for 20 s, annealing at 55 °C for 30 s, and extension at 65 °C for 2 min; this was followed by a single 5 min extension at 65 °C. After the completion of the PCR, the amplification products were run through 1.2% agarose gel electrophoresis to confirm the presence of a single band at ∼1.5 kb. 400–600 ng of the PCR amplification products were sent to Eurofins Genomics for Sanger sequencing using the tube sequencing format. The Sanger sequences returned by Eurofins Genomics in a FASTA file format were aligned against the NCBI 16S reference database for classification using the BLASTn algorithm. The electropherogram files were visualized using the SangerSeqR package in R (https://bioconductor.org/packages/release/bioc/html/sangerseqR.html).
Nanopore sequencing
16S amplification and barcoding for nanopore sequencing were performed using a 16S Barcoding Kit SQK-16S024 from ONT following a protocol recommended by the manufacturer. The kit contained barcoded full-length 16S primers (9/27F and 1492R) to be used for PCR amplification. The PCR reaction mixture contained 25 μL LUNA LongAmp Taq 2X Master Mix (NEB #M0287S), 5 μL Nuclease-Free Water (Thermo Fisher Scientific #AM9937), 10 μL bacterial DNA, and 10 μL of barcoded 16S primers. The following PCR cycling conditions were used: initial denaturation at 95 °C for 1 min; 26 cycles of denaturation at 95 °C for 20 s, annealing at 55 °C for 30 s, and extension at 65 °C for 2 min; a single 5 min extension at 65 °C. The PCR product (barcoded 16S amplicon) was cleaned up from the PCR reaction mixtures using AMPure XP Solid Phase Reversible Immobilization (SPRI) paramagnetic beads (Beckman–Coulter #A63880). 50 μL of the PCR reaction mixture containing the amplified product was mixed with an equal volume of SPRI bead suspension and a magnetic separation rack was used to separate DNA-bound beads from the rest of the solution. After two washes with 70% ethanol, the cleaned PCR product was eluted from the beads in 10 μL of buffer solution containing 10 mM Tris–HCl pH 8.0 with 50 mM NaCl and the DNA concentration was quantified on a Quantus Fluorometer using Quantifluor ONE dsDNA dye (Promega #E4891).
The nanopore 16S sequencing was performed using either a Flongle™ or a MinION™ flow cell (R.9.4.1) attached to a MinION MK1B device. The MinION™ flow cell is capable of generating greater sequencing output due to a larger number of pores, while Flongle™ is more suitable for sequencing a smaller number of samples in a single run. Flongle sequencing was performed as follows: the flow cell was first primed with a mix of 117 μL of flush buffer and 3 μL of flush tether to wash out the storage buffer solution. Once flushed, the flow cell was loaded with a solution containing 5 μL of DNA amplicon library (premixed with 0.5 μL rapid adapter protein), 15 μL of sequencing buffer, and 10 μL of library loading beads, after which the sequencing run was started. To conduct sequencing on a MinION flow cell, it was first primed by loading 800 μL of flush buffer/flush tether mix through the priming port and incubating for 5 min. Immediately before loading the DNA library another 200 μL of flush buffer/flush tether mix was added to the priming port with the Spot-On port open. Sequencing was started after adding through the Spot-On port a solution mix containing 11 μL of sample DNA library (previously mixed with 1 μL of the rapid adapter protein), 34 μL of sequencing buffer, 4.5 μL of nuclease-free water, and 25.5 μL of the loading beads (added immediately before use).
Basecalling and sequence identification
Basecalling was performed using Guppy Basecalling Software (version 5.0.7+2332e8d65) from ONT.39 The more recently released Bonito “super accuracy” basecaller model was used along with the Fast basecaller model to compare the basecalling performance on the accuracy of nanopore read sequences.
Nanopore read sequences were identified using the EPI2ME 16S analysis pipeline (EPI2ME Fastq 16S v21.03.05), which performs the Nucleotide Basic Local Alignment Search Tool (BLASTn) on each individual read against the NCBI 16S reference database. For taxonomical classification, minimum identity thresholds for species and the genus level were set at 99% and 95%, respectively.28 Additionally, only reads that returned the lowest common ancestor (lca) value of 0 during EPI2ME alignment were considered successfully classified, and sequences with a lca value of −1 or 1 were considered unclassified. For mock colony samples, the classified sequences were further separated into correctly classified and misclassified categories based on whether the classification correctly matched with known references.40 The classification of Sanger sequences was also performed by alignment against the NCBI 16S reference database using the BLASTn tool. The minimum identity threshold criteria used for taxonomic classification were the same as those used for nanopore sequences. Additionally, the top three alignment matches were examined for agreement to assign to a specific taxon (e.g., the top three alignment matches should be from the same genus for genus-level assignment). If an agreement was not reached, then the assignment was provided to the lowest common ancestor.
Construction of consensus sequences from nanopore reads
Consensus sequences from nanopore reads were constructed using the NGSpeciesID program.27 NGSpeciesID utilized fastq files from guppy basecalling to create one or a few highly accurate representative sequences from thousands of nanopore reads by using a combination of selective clustering and polishing strategies. Bonito basecalled fastq files of 16S reads were used as input and the intended target length parameter was set as 1500 (approximate length of 16S gene) along with the maximum deviation from the target parameter set as 500 to cluster and generate consensus sequences of 16S amplicons for all bacterial species present in the sample.
Results
16S sequencing and colony identification: Sanger method
Bioaerosol samples collected with a portable bioaerosol sampler were plated on Tryptic Soy Agar for the generation of colonies. From these colonies, seven distinct colonies with no visible overlap with the nearby colonies were randomly chosen for this study. Genomic DNA was extracted from each of these colonies and Sanger sequencing was performed on full-length 16S PCR amplification products (Fig. 1). The results of Sanger sequencing are summarized in Fig. 2. The sequencing generated a single consensus sequence for each colony. The sequences were identified by aligning against the NCBI 16S database using the nucleotide Basic Local Alignment Search Tool (BLASTn). The top match from the search was used for taxonomic classification and a genus-level identification was assigned when there was at least 95% identity.28 Using this criterion, six out of seven colonies (colony 2–7, Fig. 2) were successfully identified at the genus level; however, no classification was possible for colony 1. To understand why the sequence from colony 1 failed to provide a taxonomic classification, we looked at the electropherograms. The electropherograms from Sanger sequencing were used to assess the quality of the consensus sequence generated. A peak in an electropherogram represents the signal from a nucleotide base and is used to determine the base at a specific location of the consensus sequence. An electropherogram with high quality will consist of single, discrete peaks, while areas of poorer quality contain multiple overlapping peaks. We found that the electropherogram for colony 1 is considerably noisier than the electropherograms for other colonies with many overlapping peaks distributed throughout. Well-resolved electropherogram peaks are an important prerequisite for the data processing software to determine Sanger sequences with high read accuracy.41 The noisy electropherogram of colony 1 explains why a consensus sequence could not be obtained by the Sanger method.
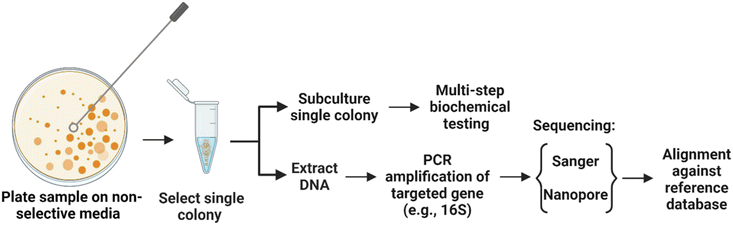 |
| Fig. 1 Schema outlining the main approaches for the identification of bacterial colonies. Both Sanger and nanopore sequencing techniques can be used for identification by targeted gene amplification and are focused on this work. | |
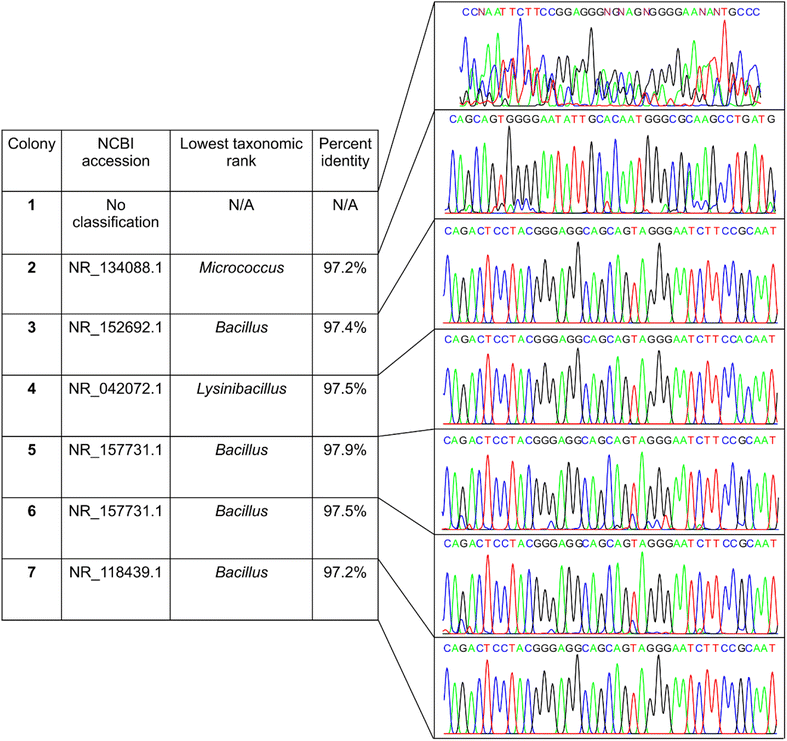 |
| Fig. 2 Classification of bacterial colonies by Sanger sequencing of 16S amplicons. The sequence obtained for each colony (designated 1–7) was compared against the NCBI database and the top match was used for taxonomic classification. For each colony, subsections of electropherograms (corresponding to base pairs 256–297) are shown on the right. Colony 1 was not successfully classified. The electropherograms were visualized using the R package SangerSeqR. | |
16S sequencing and colony identification: nanopore
Next, we conducted MinION nanopore sequencing of full-length 16S amplicons obtained from the same seven bacterial colonies. First, we compared the performance between the standard Fast basecalling model and the more recently introduced, highly discriminatory Bonito (“super accuracy”) basecalling model in converting the flow cell-generated ionic current data into sequences of nucleotide bases. Fast and Bonito basecalling of ionic current data generated a total of 133
149 and 163
552 read sequences, respectively. Table 1 summarizes the outcome for genus-level classification of both basecalling methods, including the number of reads, the percentage of total reads that were successfully classified, and the mean percent identity (Ī) of all sequences for each colony. We observed that switching from Fast to Bonito basecalling while maintaining a constant Quality Score (Q-score) of 13 leads to an increase in the number of total reads and Ī, but the percentage of correct classification remains comparable. However, implementing the Bonito basecalling at a Q-score threshold of 13 and increasing the identity threshold (I) to 95%, considered to be optimal for taxonomic identification at the genus level,28 we noticed a substantial improvement in the percentage of correct classification (≥99.4% reads were correctly classified). It is to be noted that this improvement in classification accuracy was associated with a considerable drop in the total read number.25 The Bonito-basecalled sequences (Q ≥ 13 and I ≥ 95%) were taxonomically identified using the EPI2ME 16S workflow, which utilizes the NCBI 16S database as a reference database (Fig. 3). The calculation of relative abundances from these classified sequences showed a single bacterial genus for colonies 3–7 (relative abundance ≥ 99.5%) and their identities matched well with the findings from Sanger sequencing (Fig. 3). In the case of colony 1, for which Sanger sequencing failed to assign a taxonomic classification with an inferior quality electropherogram, nanopore sequencing indicated the presence of two dominant taxa, namely Alkalihalobacillus (87.1%) and Kocuria (10.9%). For colony 2 also, nanopore sequencing showed the presence of two bacteria, namely Micrococcus (68.4%) and Paraburkholderia (27.7%). Interestingly, Sanger sequencing of the colony classified it as Micrococcus with a high 97.4% identity and had an electropherogram with clean, distinct peaks. Thus, not only the less abundant bacteria in colony 2 were not identified by Sanger sequencing but also the potential presence of a second bacterial species was not suggested by the electropherogram, or the sequence obtained.
Table 1 Genus-level classification of 16S amplicons for bacterial colonies 1–7 after nanopore sequencing. The outcomes for Fast and Bonito basecalling and assignment of different Q-scores are compareda
Colony |
Fast basecalling Q ≥ 7 |
Bonito basecalling Q ≥ 7 |
Bonito basecalling Q ≥ 13, I ≥ 95% |
Total |
Classified (%) |
Ī (%) |
Total |
Classified (%) |
Ī (%) |
Total |
Classified (%) |
Ī (%) |
The total number of reads, the percentage of reads successfully classified, and the mean percent identities are presented in the table. Q thresholds of 7 and 13 correspond to an 85% and a 95% chance that each base is accurate, respectively. The classification was performed using the NCBI 16S database for reference. Abbreviations: quality score (Q), percent identity (I), and mean percent identity (Ī).
|
1 |
19 270 |
89.5 |
86.2 |
24 753 |
89.6 |
91.4 |
2271 |
99.4 |
95.9 |
2 |
14 037 |
90.7 |
86.9 |
18 517 |
91.1 |
92.0 |
1894 |
99.7 |
96.2 |
3 |
12 063 |
96.0 |
87.5 |
15 469 |
93.9 |
92.5 |
1961 |
99.6 |
96.6 |
4 |
24 741 |
91.9 |
86.6 |
28 734 |
92.7 |
92.1 |
3345 |
99.9 |
96.3 |
5 |
15 598 |
96.0 |
87.4 |
19 182 |
93.9 |
92.5 |
2543 |
99.7 |
96.6 |
6 |
18 471 |
96.0 |
87.3 |
21 534 |
93.7 |
92.4 |
2910 |
99.8 |
96.6 |
7 |
28 969 |
94.3 |
87.0 |
35 363 |
92.6 |
92.5 |
4022 |
99.8 |
96.6 |
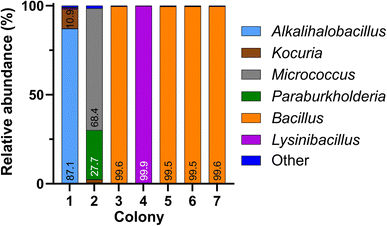 |
| Fig. 3 Relative abundances of bacterial genera in colonies 1–7, obtained by nanopore sequencing of 16S rDNA amplicons (Bonito basecalling; Q-score ≥ 13 and percent identity ≥ 95%). | |
Evaluation on mock multispecies colonies constructed with pure bacterial DNA
Bioaerosol-derived colonies, identified through sequencing, indicated the potential existence of multispecies colonies. Although Sanger sequencing of the 16S gene successfully identified typical colonies formed by a single bacterial species, we found inconsistent outcomes (percent identity and electropherogram quality) for multispecies colonies identified by nanopore sequencing. To further understand how 16S Sanger sequences change when more than one bacterial genomic DNA is present in the sample, we designed a setup of mock multispecies colonies by mixing pure DNA samples of two bacterial species at known proportions. Pure genomic DNAs of Acinetobacter baumannii (A. baumannii, ATCC 19606D-5) and Stenotrophomonas maltophilia (S. maltophilia, ATCC 13637D-5) were mixed at 3 different ratios (w/w) of 9
:
1, 1
:
1, and 1
:
9. These proportions were selected to compare the performance of the two sequencing techniques under scenarios where two bacterial species within a colony exist at a similar level of abundance and where the abundance of one species dominates over the other. Sanger sequencing was conducted in these samples and the results were compared against the pure DNA controls of A. baumannii and S. maltophilia (Fig. 4). The sequences from pure DNA controls of A. baumannii (sample A) and S. maltophilia (sample E) were classified correctly when aligned against the NCBI 16S reference database, although identity matches were 95.5% and 97.4%, respectively, allowing for genus level identification. The Sanger sequence generated from the sample with 90% A. baumannii and 10% S. maltophilia (sample B, Fig. 4) was classified as A. baumannii with 96.8% identity match, while the sequence generated from the sample with 10% A. baumannii and 90% S. maltophilia (sample D) had the closest alignment with S. maltophilia with 81.3% identity. For the sample with 50% of both A. baumannii and S. maltophilia (control sample C), the Sanger sequence was found to have the closest alignment with A. baumannii with a low 79.3% identity. Unlike samples A, B, and E, the lower identity match for samples C and D would allow the lowest taxonomic classification to the phylum level only. The electropherograms also showed distinct changes to different levels of DNA mixing (Fig. 4). The electropherogram of pure A. baumannii DNA (sample A) had clear and separated peaks, while pure S. maltophilia DNA (sample E) showed some peak overlap even though the alignment of the Sanger sequence showed a high percentage identity. In mixed samples, the presence of 10% S. maltophilia DNA made only a minimal change to the electropherogram signal of A. baumannii (sample B); however, the presence of 10% A. baumannii caused substantial degradation of the electropherogram signal of S. maltophilia (sample D). Counterintuitively though, equal mixing of each of these two DNA (sample C) resulted in a relatively clean electropherogram, although the identity match of the Sanger sequence obtained from the electropherogram was lower than that of the other two mixed samples.
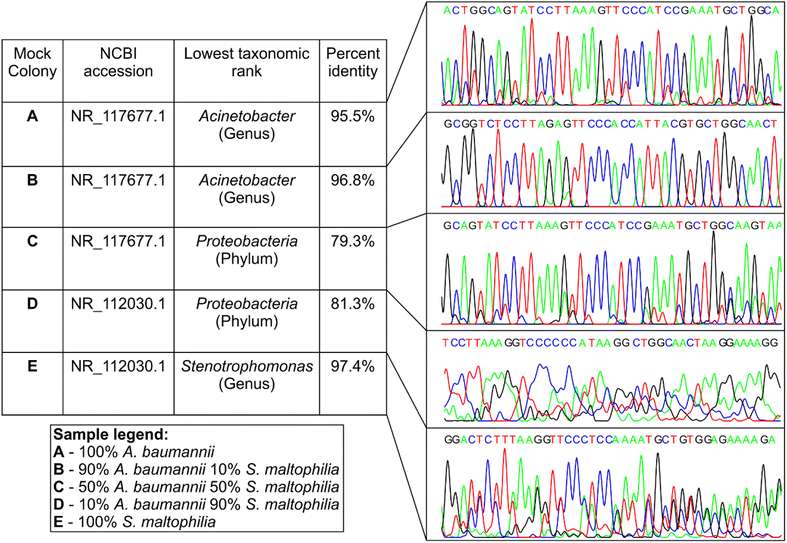 |
| Fig. 4 Classification results of the mock colony samples A–E by Sanger sequencing of 16S amplicons. Electropherogram segments corresponding to each sample are shown. Taxonomic classification was obtained by the best match of a sequence against the NCBI 16S database. The electropherograms were obtained using the R package SangerSeqR. | |
In parallel to Sanger sequencing, we performed nanopore sequencing of the samples from mock colonies. The Bonito-basecalled reads correctly classified >99% of reads at the genus level (Q-score ≥ 13 and I ≥ 95%) and the performance was comparable for all samples irrespective of different proportions of DNA mixing. We found that the nanopore sequencing was able to successfully classify pure DNA samples (samples A and E) as well as mixed DNA samples (samples B, C, and D) with the relative abundances reflecting the proportion of mixing (Fig. 5). It is to be noted that for samples C–E, where the proportions of S. maltophilia were relatively higher, a small fraction of total reads was identified as Xanthomonas. Although S. maltophilia is phenotypically distinct from Xanthomonas species, at the rRNA gene level a high degree of sequence similarity exists between them;42 indeed, due to such sequence similarity, S. maltophilia was previously classified as Xanthomonas maltophilia.43 Their closeness in the rRNA gene sequence combined with the read accuracy limits for nanopore sequencing potentially resulted in the observed appearance of a small population of Xanthomonas in samples containing S. maltophilia genomic DNA. Since the species-level information of pure DNA samples was known for the control experiment, we also attempted species-level identification for the sequences raising the threshold of I to ≥99%.44 The new threshold criteria reduced the total number of passed reads to 5148 (Table 2). We found that for the pure A. baumannii and S. maltophilia samples, 97.4% and 92.1% of the correctly classified sequence reads from nanopore sequencing accurately matched to the species level. A similar degree of accuracy was maintained in the species-level identification of mixed samples.
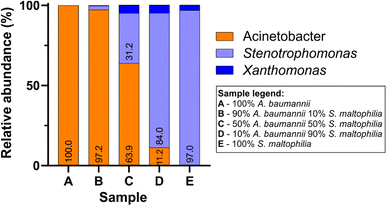 |
| Fig. 5 Relative abundances of bacterial genera in mock colony samples A–E, obtained by nanopore sequencing of 16S amplicons (Bonito basecalling; Q-score ≥ 13 and percent identity ≥ 95%). | |
Table 2 Summary classification of 16S amplicons for mock colony samples A–E sequenced using nanopore sequencing (Bonito basecalling; Q-score ≥ 13). For genus and species-level classification, the thresholds used for I were set at ≥95% and ≥99%, respectively. Mock colony composition: A, 100% A. baumannii; B, 90% A. baumannii and 10% S. maltophilia; C, 50% A. baumannii and 50% S. maltophilia; D, 10% A. baumannii and 90% S. maltophilia; E, 100% S. maltophilia.a
Mock colony |
Bonito genus-level Q ≥ 13 and I ≥ 95% |
Bonito species-level Q ≥ 13 and I ≥ 99% |
Total |
CC (%) |
MC (%) |
UC (%) |
Ī (%) |
Total |
CC (%) |
MC (%) |
UC (%) |
Ī (%) |
The table shows the total number of reads along with the percentage of reads Correctly Classified (CC), Misclassified (MC), and Unclassified (UC) for both genus and species-level classification. The classification was performed using the NCBI 16S database for reference. Abbreviations: Q-score (Q), percent identity (I), and mean percent identity (Ī).
|
A |
41 158 |
99.9 |
<0.1 |
0.1 |
97.3 |
573 |
94.1 |
2.5 |
3.4 |
99.2 |
B |
79 618 |
99.7 |
<0.1 |
0.3 |
97.2 |
1419 |
86.2 |
13.6 |
2.2 |
99.3 |
C |
73 554 |
99.6 |
<0.1 |
0.4 |
97.3 |
1266 |
88.2 |
10.2 |
1.6 |
99.3 |
D |
84 019 |
99.9 |
<0.1 |
0.1 |
97.3 |
1233 |
91.3 |
4.0 |
4.7 |
99.2 |
E |
40 366 |
99.6 |
<0.1 |
0.4 |
97.3 |
657 |
85.5 |
5.0 |
9.5 |
99.2 |
Generation of consensus amplicon sequences from nanopore data
Although nanopore sequencing demonstrates an advantage over the Sanger method in amplicon-based identification of bacteria from multispecies colonies, one criticism of this technique is the relatively lower read accuracy of individual amplicons. For a DNA sample from a single species, this limitation is addressed through the generation of a consensus sequence from the read sequences with read accuracy being comparable to that obtained through the Sanger method.27,45 Such an approach has recently been expanded in the NGSpeciesID workflow for mixed DNA samples through implementation of appropriate clustering and polishing strategies.27 Implementing this method on nanopore sequence data obtained from pure DNA controls of A. baumannii and S. maltophilia generated consensus sequences that accurately matched the NCBI database sequence with >99.9% identity (Table 3). Furthermore, the alignment to the source strain sequences (ATCC 19606D-5 and ATCC 13637D-5 for A. baumannii and S. maltophilia, respectively) matched by 99.93% for both samples, indicating a deviation of only one nucleotide in the entire 16S region. For the mock multispecies colony with 50% presence of each of these two bacterial DNA (sample C), two consensus sequences were generated with one having a 100% match with A. baumannii and the other having a 98.04% match with S. maltophilia. When applied for mock colony samples with 90% DNA from A. baumannii (sample B) or S. maltophilia (sample D), the technique returned a single consensus sequence for A. baumannii and S. maltophilia, respectively, with an identity match of >99.9%. These results confirm that highly accurate consensus sequences of 16S amplicons can be obtained from nanopore reads even in mixed colony samples, except when the relative abundance of a bacterial species is disproportionately lower than that of the dominant species.
Table 3 Classification of consensus sequences generated from nanopore reads of samples from mock and bioaerosol-derived colonies (Bonito basecalling; Q-score ≥ 13), obtained by alignment against the NCBI 16S database using the BLASTn tool
|
NCBI accession no. |
Taxonomic classification |
Percent identity (%) |
Mock colony |
A |
NR_113 237.1 |
Acinetobacter baumannii
|
100 |
B |
NR_113 237.1 |
Acinetobacter baumannii
|
100 |
C |
NR_113 237.1 |
Acinetobacter baumannii
|
100 |
|
NR_112 030.1 |
Stenotrophomonas maltophilia
|
98.04 |
D |
NR_112 030.1 |
Stenotrophomonas maltophilia
|
99.93 |
E |
NR_112 030.1 |
Stenotrophomonas maltophilia
|
99.93 |
Bioaerosol-derived colony |
1 |
NR_108 311.1 |
Alkalihalobacillus rhizosphaerae
|
99.1 |
|
NR_025 723.1 |
Kocuria marina
|
99.86 |
2 |
NR_116 578.1 |
Micrococcus yunnanensis
|
99.65 |
|
NR_025 058.1 |
Paraburkholderia fungorum
|
99.73 |
3 |
NR_115 526.1 |
Bacillus cereus
|
100 |
4 |
NR_112 628.1 |
Lysinibacillus fusiformis
|
99.66 |
5 |
NR_114 581.1 |
Bacillus thuringiensis
|
100 |
6 |
NR_114 581.1 |
Bacillus thuringiensis
|
99.93 |
7 |
NR_042 337.1 |
Bacillus altitudinis
|
99.87 |
After successful construction of consensus sequences of 16S amplicons from mock colonies, we aimed to implement the tool on the data from bioaerosol-derived colonies. The consensus sequences obtained for bioaerosol samples were found to have >99% identity match with sequences in the NCBI 16S database, enabling species-level identification (Table 3). For example, the top three matches of the consensus sequences for colonies 3, 5, and 6 were found to be strains of B. cereus, B. thuringiensis, and B. thuringiensis, respectively with the percent identity ranging from 100% to 99.73, which correspond to a mismatch of 0–4 bases for ∼1.5 kb amplicon. Moreover, for colonies 1 and 2, consensus sequences of both bacteria that contributed to each of these mixed colonies could be generated maintaining a similarly high identity match to the NCBI database. It is to be noted that the total number of nanopore sequence reads for bioaerosol-derived colonies was substantially lower than the reads from the mock colonies (compare the Q ≥ 13 and I ≥ 95% read numbers in Tables 1 and 2) but that didn't adversely impact the quality of the consensus sequence. Taken together, our results indicate that 16S reads from nanopore sequencing could be utilized not only to identify individual bacteria from mixed bacterial colonies and estimate their proportional abundance but also to construct a full-length amplicon sequence with high accuracy.
Discussion
Using full-length 16S amplicons, we found that both Sanger and nanopore sequencing techniques are effective and corroborate well with genus-level identification of bacteria when the colonies have a homogeneous composition of a single taxon. Additionally, consensus sequences constructed from the nanopore reads yielded highly accurate sequence alignment (≥99%), enabling species-level identification. For the multispecies colonies, nanopore sequencing was able to identify individual bacterial components with an estimate of their compositional representation; however, Sanger sequencing either identified the dominant bacterial taxa or failed to identify any taxa. Furthermore, we found that the sequence identity match from Sanger sequencing and electropherogram quality were less informative metrics to rule out the potential existence of multiple bacterial species in a colony.
While several studies have compared nanopore sequencing with NGS such as Illumina sequencing,40,46,47 relatively few studies are directed toward the comparison of MinION sequencing and Sanger sequencing.48 The interest in using nanopore sequencing as a potential alternative sequencing tool to Sanger sequencing is primarily for amplicon-based assays, commonly used in forensic genetics or tracking species in the field.45,48 Since Sanger and nanopore sequencing are based on completely different technologies and generate a different form of output, the comparison is not straightforward and done through the generation of a consensus sequence from nanopore read sequences.27 Among the few comparisons being made for targeted amplicon analysis, Vasiljevic et al. reported using nanopore sequencing to identify animal species via the species-diagnostic region of the mitochondrial cytochrome b (mtDNA cyt b) gene with an amplicon length of approximately 421 bp.49 Their results showed that the consensus sequences derived from nanopore sequencing were remarkably close to Sanger sequences with a deviation of not more than 1 bp. It is to be noted that the performance of Sanger sequencing starts to decrease for longer sequence lengths (>1000 bases), and therefore, could have less accuracy in sequencing the full-length 16S gene (∼1.5 kb).50 Not surprisingly, we found that Sanger method-derived 16S sequences had <98% maximum identity match against the NCBI database for both pure bacterial DNA and cultured colonies, preventing a species-level classification. The nanopore sequencing technology, however, is not constrained by such limitations as the read accuracy is independent of the length of DNA fragments sequenced. This is further supported by our findings that 16S consensus sequences generated from nanopore reads of pure DNA samples of A. baumannii and S. maltophilia strains deviate by only one base from the maximally aligned sequences in the NCBI 16S database.
Sanger sequencing is still a default choice in many fields for the identification and comparison of homogeneous genetic material, particularly when amplicons of sub-thousand base pairs are used for characterization.26 The technique can also be applied to mixed microbial samples through colony culture and isolation of individual taxa from distinct colonies. In this work, we found that the Sanger sequence of 16S amplicons from colony 1 has a low identity match and a noisy electropherogram with overlapping peaks, raising the suspicion of the presence of more than one bacterial species. Standard microbiological practice can address this by subculturing the culture isolate, followed by Sanger sequencing of the pure colonies generated. Sanger sequencing for colonies 2–7 demonstrated a clean electropherogram with distinct peaks and the sequences had an identity match of ≥97% against the NCBI 16S database. For colonies 3–7, the genus level identification from Sanger sequencing matched accurately with the nanopore sequencing result. However, for colony 2, which was identified as Micrococcus by Sanger sequencing, nanopore sequencing revealed that almost one third of the amplicons are from taxonomically distant Paraburkholderia. The mock colony experiment using pure bacterial DNA further confirmed that the presence of additional taxa in a sample has a variable impact on electropherogram quality and percent identity match for the dominant taxa, and such effects are taxa specific. We observed that the presence of 10% S. maltophilia in A. baumannii genomic DNA had minimum impact on the percent identity match for A. baumannii; however, the presence of 10% A. baumannii in S. maltophilia genomic DNA resulted in a drastic reduction in the identity match for S. maltophilia (97.4% to 81.3%) along with a conspicuous drop in the electropherogram quality. These results together suggest that the Sanger method has a limited ability to discriminate from 16S amplicons whether a bacterial colony is a true homogeneous colony (as in colonies 3–7) or a multispecies colony (as in colony 2). This uncertainty can pose a serious limitation on the applicability of Sanger sequencing in colony identification when there is potential for multispecies colony formation.42
Nanopore sequencing technology is emerging fast in the landscape of sequencing and is being applied for an increasing range of applications, including whole genome sequencing, microbiome analysis, and transcriptome analysis.34,36,37,51 Underlying the rapid growth and increased acceptance of this technology is a continual advancement in the sequencing platform and basecalling algorithm, increased availability of protocols and bioinformatics tools for analysis, along with benchmarking studies confirming the robustness and reliability of performance.52 Indeed, when comparing the preexisting Fast basecalling with the more recently introduced Bonito basecalling, we observed a substantial improvement in the quality of sequence reads (Ī increased by ∼5%) along with a larger number of reads passing a preset quality threshold. Implementing an appropriate quality threshold (Q-score ≥ 13, I ≥ 95%), we were able to achieve accurate genus-level classification. However, the biggest advantage of nanopore sequencing over the Sanger method in the context of colony identification comes from its ability to identify all bacterial taxa present in the colony as in the case of colonies 1 and 2. Additionally, a control experiment with pure genomic DNA demonstrated that the relative abundance of bacteria observed by the nanopore sequencing reasonably reflects their proportion in a mixed sample, although some deviation can result from the variability in 16S gene copies present among bacterial species.22,53
The capability of long-read sequencing by the MinION nanopore or PacBio sequencing platform offers a clear advantage over short-read sequencing technologies for applications in taxonomical identification or classification through targeted gene amplification. For the 16S gene, sequences longer than 1300 bases are considered to be suitable for reliable results.20 However 16S taxonomical classification by Illumina-based sequencing usually targets the hypervariable regions V4, V3–V4, or V4–V5 of the 16S gene due to the limitation of this technique to read only a short span of the 16S sequence.29,30 Such a restriction imposed on the amplicon length allows identification only up to the genus level. While near full-length 16S sequencing on the Illumina platform has been achieved by using unique, random sequences to tag individual 16S gene templates, the long, complex procedure is not practical for routine implementation.54 The long-read sequencing enables the analysis of full-length 16S gene amplicons and such coverage has been shown to successfully identify microbiota to species-level resolution.30 A recent study using the PacBio long-read sequencing platform achieved a read accuracy to the single nucleotide level through the construction of circular consensus sequences (CCSs), followed by the implementation of an advanced algorithm for analysis that enabled strain level identification.55 Although this result is highly accurate, the need for expensive equipment, and a relatively complex sample preparation and analysis process along with the higher cost associated with sequencing make it less suitable for routine identification of bacterial colonies.
In our work, we found that the implementation of Bonito basecalling followed by selection of high-quality reads (Q-score ≥ 13 and I ≥ 99%) enabled successful species-level classification of 97.4% and 92.1% of the amplicon sequences derived from pure DNA samples of A. baumannii and S. maltophilia, respectively. It is to be noted that an identity threshold of ∼99% is recommended for species-level identification from the full-length 16S sequence, and such a high threshold leads to the rejection of a significant proportion of reads, and therefore, requires a larger number of raw reads for analysis. Our results show that the construction of consensus sequences would be an attractive alternative strategy for species-level identification, where a highly accurate (>99%) identity match was observed even for mixed colony samples.44 Interestingly, this high accuracy match with the NCBI 16S database (only 0–4 base mismatch for top matches) enabled the species-level classification of Bacillus colonies, which is otherwise known to be an extremely challenging task to accomplish through the 16S sequencing approach. Moreover, the quality of the consensus sequence was maintained even with a smaller number of reads per sample (as observed with bioaerosol-derived colonies), which could be highly advantageous in reducing the sequencing cost by enabling a larger number of samples to be sequenced per flow cell or utilization of Flongle, a more affordable option for nanopore sequencing. One limitation of this consensus sequence-based identification approach would be potentially missing a bacterial species having a very low abundance in a multispecies culture, similar to what we observed for mock mixed colonies B and D, where only A. baumannii and S. maltophilia containing 90% of total DNA, respectively, were being identified.
Emerging bodies of work suggest that airborne microbes can pose multiple health risks that include transmission of infectious diseases, triggering of chronic diseases, and the spread of antimicrobial resistance genes.16,56 Microbes in the air exist both as single organisms and as aggregates, often bound to particulate matter. Due to their low numbers in the air, bioaerosol samples are usually plated directly on agar media for culture-based assays to study viable microorganisms. Since microbes can exist as aggregates in bioaerosols,14,15 such an aggregated form can potentially lead to the formation of multispecies colonies, and therefore, such possibilities should be considered when analyzing single colonies. While adjusting the culture conditions (e.g., temperature and incubation period) and subculturing could help in the generation of pure isolates and subsequent identification by the Sanger sequencing method, our results show that nanopore sequencing could enable fast and accurate identification of bacterial taxa in such samples. It is to be noted that even though nanopore sequencing costs can be substantially reduced by multiplexing samples to run on a single flow cell, the classical microbiological approach of subculturing to generate pure colonies of single bacterial species followed by Sanger sequencing would remain a more cost-effective approach for routine colony identification. However, with continued advancements and innovations in nanopore sequencing technology, the cost might come down substantially in the near future to be competitive with Sanger sequencing cost. Furthermore, the remarkable improvements in nanopore sequencing accuracy in recent years, the availability of a streamlined protocol for 16S analysis, and the ability to conduct sequencing experiments in the lab could make this technology a powerful tool for culture-based assays of bioaerosol or other complex environmental samples.
Conclusions
Microbial colonies are routinely detected by targeted gene sequencing utilizing the Sanger method. In this study, we compared nanopore sequencing against the Sanger technique for the identification of bioaerosol-derived microbial colonies. Using full-length 16S sequence data, we found that Sanger sequencing provides a consistent genus-level classification for single-species colonies; for multispecies colonies, this sequencing method is not only ineffective for identification but also cannot reliably indicate such a possibility. Nanopore sequencing successfully identified both single and multispecies colonies along with providing an approximate relative abundance of the bacterial taxa in the multispecies colonies. Furthermore, species-level identification was accomplished by the construction of highly accurate consensus sequences from a small number of full-length 16S reads. Thus, our findings suggest that nanopore sequencing could be an attractive alternative to accurate identification of bacterial colonies with resolution up to the species level, especially for complex environmental samples such as bioaerosols.
Data availability
All data used in this work are freely available to download at https://zenodo.org/record/7813856#.ZDSwpXbMIQ8. The datasets include nanopore and Sanger sequencing data as well as EPI2ME and NGSpeciesID analysis.
Author contributions
AM: conceptualization, methodology, investigation, formal analysis, and writing – original draft. DF: conceptualization, methodology, formal analysis, and writing – original Draft. PD: formal analysis. KK: investigation. SD: supervision, writing – review & editing, and funding acquisition. SS: conceptualization, supervision, project administration, writing – review & editing, and funding acquisition.
Conflicts of interest
There are no conflicts of interest to declare.
Acknowledgements
This study was supported by funding from the National Science Foundation (NSF STTR Phase II, Award No. 1853522) and the New York State Department of Economic Development (NYSDEC, Award No. C180132) through the Center for Advanced Materials Processing (CAMP). Daniel T. Fuller received support from the Lawrence ‘57’ and Antoinette Delaney Ignite Research Fellowship. Cartoons in Fig. 1 and table of contents (TOC) entry were created with https://BioRender.com.
References
- R. Urbano, B. Palenik, C. J. Gaston and K. A. Prather, Detection and phylogenetic analysis of coastal bioaerosols using culture dependent and independent techniques, Biogeosciences, 2011, 8, 301–309 CrossRef CAS.
- A. Tiwari, D. M. Oliver, A. Bivins, S. P. Sherchan and T. Pitkänen, Bathing Water Quality Monitoring Practices in Europe and the United States, Int. J. Environ. Res. Public Health, 2021, 18, 5513 CrossRef CAS PubMed.
- G. Banerjee, S. Agarwal, A. Marshall, D. H. Jones, I. M. Sulaiman, S. Sur and P. Banerjee, Application of advanced genomic tools in food safety rapid diagnostics: challenges and opportunities, Curr. Opin. Food Sci., 2022, 47, 100886 CrossRef CAS.
- N. Peker, N. Couto, B. Sinha and J. W. Rossen, Diagnosis of bloodstream infections from positive blood cultures and directly from blood samples: recent developments in molecular approaches, Clin. Microbiol. Infect., 2018, 24, 944–955 CrossRef CAS PubMed.
- I. Gandolfi, V. Bertolini, R. Ambrosini, G. Bestetti and A. Franzetti, Unravelling the bacterial diversity in the atmosphere, Appl. Microbiol. Biotechnol., 2013, 97, 4727–4736 CrossRef CAS PubMed.
- S. Zhang, Z. Liang, X. Wang, Z. Ye, G. Li and T. An, Bioaerosols in an industrial park and the adjacent houses: Dispersal between indoor/outdoor, the impact of air purifier, and health risk reduction, Environ. Int., 2023, 172, 107778 CrossRef PubMed.
- J. Fröhlich-Nowoisky, C. J. Kampf, B. Weber, J. A. Huffman, C. Pöhlker, M. O. Andreae, N. Lang-Yona, S. M. Burrows, S. S. Gunthe, W. Elbert, H. Su, P. Hoor, E. Thines, T. Hoffmann, V. R. Després and U. Pöschl, Bioaerosols in the Earth system: Climate, health, and ecosystem interactions, Atmos. Res., 2016, 182, 346–376 CrossRef.
- R. M. W. Ferguson, S. Garcia-Alcega, F. Coulon, A. J. Dumbrell, C. Whitby and I. Colbeck, Bioaerosol biomonitoring: Sampling optimization for molecular microbial ecology, Mol. Ecol. Resour., 2019, 19, 672–690 CrossRef CAS PubMed.
- E. Blagodatskaya and Y. Kuzyakov, Active microorganisms in soil: Critical review of estimation criteria and approaches, Soil Biol. Biochem., 2013, 67, 192–211 CrossRef CAS.
- V. Velusamy, K. Arshak, O. Korostynska, K. Oliwa and C. Adley, An overview of foodborne pathogen detection: In the perspective of biosensors, Biotechnol. Adv., 2010, 28, 232–254 CrossRef CAS PubMed.
- S. E. Dowd, Y. Sun, P. R. Secor, D. D. Rhoads, B. M. Wolcott, G. A. James and R. D. Wolcott, Survey of bacterial diversity in chronic wounds using Pyrosequencing, DGGE, and full ribosome shotgun sequencing, BMC Microbiol., 2008, 8, 43 CrossRef PubMed.
- R. L. Marsh, M. J. Binks, H. C. Smith-Vaughan, M. Janka, S. Clark, P. Richmond, A. B. Chang and R. B. Thornton, Prevalence and subtyping of biofilms present in bronchoalveolar lavage from children with protracted bacterial bronchitis or non-cystic fibrosis bronchiectasis: a cross-sectional study, Lancet Microbe, 2022, 3, e215–e223 CrossRef PubMed.
- Z. Liang, Y. Yu, Z. Ye, G. Li, W. Wang and T. An, Pollution profiles of antibiotic resistance genes associated with airborne opportunistic pathogens from typical area, Pearl River Estuary and their exposure risk to human, Environ. Int., 2020, 143, 105934 CrossRef CAS PubMed.
- K. W. Tham and M. S. Zuraimi, Size relationship between airborne viable bacteria and particles in a controlled indoor environment study, Indoor Air, 2005, 15, 48–57 CrossRef PubMed.
- W. Eduard, Measurement methods and strategies for non-infectious microbial components in bioaerosols at the workplace, Analyst, 1996, 121, 1197–1201 RSC.
- F. Shen and M. Yao, Bioaerosol nexus of air quality, climate system and human health, J. Nat. Sci., 2023, 2, 20220050 Search PubMed.
- L. M. McCully, A. S. Bitzer, S. C. Seaton, L. M. Smith and M. W. Silby, Interspecies Social Spreading: Interaction between Two Sessile Soil Bacteria Leads to Emergence of Surface Motility, Msphere, 2019, 4, e00696 CrossRef CAS PubMed.
- L. Xiong, Y. Cao, R. Cooper, W.-J. Rappel, J. Hasty and L. Tsimring, Flower-like patterns in multi-species bacterial colonies, Elife, 2020, 9, e48885 CrossRef CAS PubMed.
- C. R. Woese and G. E. Fox, Phylogenetic structure of the prokaryotic domain: The primary kingdoms, Proc. Natl. Acad. Sci. U. S. A., 1977, 74, 5088–5090 CrossRef CAS PubMed.
- P. Yarza, P. Yilmaz, E. Pruesse, F. O. Glöckner, W. Ludwig, K.-H. Schleifer, W. B. Whitman, J. Euzéby, R. Amann and R. Rosselló-Móra, Uniting the classification of cultured and uncultured bacteria and archaea using 16S rRNA gene sequences, Nat. Rev. Microbiol., 2014, 12, 635–645 CrossRef CAS PubMed.
- J. M. Janda and S. L. Abbott, 16S rRNA Gene Sequencing for Bacterial Identification in the Diagnostic Laboratory: Pluses, Perils, and Pitfalls▽, J. Clin. Microbiol., 2007, 45, 2761–2764 CrossRef CAS PubMed.
- T. Větrovský and P. Baldrian, The Variability of the 16S rRNA Gene in Bacterial Genomes and Its Consequences for Bacterial Community Analyses, PLoS One, 2013, 8, e57923 CrossRef PubMed.
- J. S. Johnson, D. J. Spakowicz, B.-Y. Hong, L. M. Petersen, P. Demkowicz, L. Chen, S. R. Leopold, B. M. Hanson, H. O. Agresta, M. Gerstein, E. Sodergren and G. M. Weinstock, Evaluation of 16S rRNA gene sequencing for species and strain-level microbiome analysis, Nat. Commun., 2019, 10, 5029 CrossRef PubMed.
- B. L. Karger and A. Guttman, DNA sequencing by CE, Electrophoresis, 2009, 30, S196–S202 CrossRef PubMed.
- L. M. Baudhuin, S. A. Lagerstedt, E. W. Klee, N. Fadra, D. Oglesbee and M. J. Ferber, Confirming Variants in Next-Generation Sequencing Panel Testing by Sanger Sequencing, J. Mol. Diagn., 2015, 17, 456–461 CrossRef CAS PubMed.
- P. D. N. Hebert, T. W. A. Braukmann, S. W. J. Prosser, S. Ratnasingham, J. R. deWaard, N. V. Ivanova, D. H. Janzen, W. Hallwachs, S. Naik, J. E. Sones and E. V. Zakharov, A Sequel to Sanger: amplicon sequencing that scales, BMC Genomics, 2018, 19, 219 CrossRef PubMed.
- K. Sahlin, M. C. W. Lim and S. Prost, NGSpeciesID: DNA barcode and amplicon consensus generation from long-read sequencing data, Ecol. Evol., 2021, 11, 1392–1398 CrossRef PubMed.
- R. C. Edgar, Accuracy of taxonomy prediction for 16S rRNA and fungal ITS sequences, Peerj, 2018, 6, e4652 CrossRef PubMed.
- M. C. Nelson, H. G. Morrison, J. Benjamino, S. L. Grim and J. Graf, Analysis, Optimization and Verification of Illumina-Generated 16S rRNA Gene Amplicon Surveys, PLoS One, 2014, 9, e94249 CrossRef PubMed.
- Y. Matsuo, S. Komiya, Y. Yasumizu, Y. Yasuoka, K. Mizushima, T. Takagi, K. Kryukov, A. Fukuda, Y. Morimoto, Y. Naito, H. Okada, H. Bono, S. Nakagawa and K. Hirota, Full-length 16S rRNA gene amplicon analysis of human gut microbiota using MinION™ nanopore sequencing confers species-level resolution, BMC Microbiol., 2021, 21, 35 CrossRef CAS PubMed.
- J. Shendure, S. Balasubramanian, G. M. Church, W. Gilbert, J. Rogers, J. A. Schloss and R. H. Waterston, DNA sequencing at 40: past, present and future, Nature, 2017, 550, 345–353 CrossRef CAS PubMed.
- M. Jain, H. E. Olsen, B. Paten and M. Akeson, The Oxford Nanopore MinION: delivery of nanopore sequencing to the genomics community, Genome Biol., 2016, 17, 239 CrossRef PubMed.
- R. R. Zascavage, K. Thorson and J. V. Planz, Nanopore sequencing: An enrichment-free alternative to mitochondrial DNA sequencing, Electrophoresis, 2019, 40, 272–280 CrossRef CAS PubMed.
- T. Mantere, S. Kersten and A. Hoischen, Long-Read Sequencing Emerging in Medical Genetics, Front. Genet., 2019, 10, 426 CrossRef CAS PubMed.
- R. M. Leggett, C. Alcon-Giner, D. Heavens, S. Caim, T. C. Brook, M. Kujawska, S. Martin, N. Peel, H. Acford-Palmer, L. Hoyles, P. Clarke, L. J. Hall and M. D. Clark, Rapid MinION profiling of preterm microbiota and antimicrobial-resistant pathogens, Nat. Microbiol., 2020, 5, 430–442 CrossRef CAS PubMed.
- L. Ciuffreda, H. Rodríguez-Pérez and C. Flores, Nanopore sequencing and its application to the study of microbial communities, Comput. Struct. Biotechnol. J., 2021, 19, 1497–1511 CrossRef CAS PubMed.
- R. E. Workman, A. D. Tang, P. S. Tang, M. Jain, J. R. Tyson, R. Razaghi, P. C. Zuzarte, T. Gilpatrick, A. Payne, J. Quick, N. Sadowski, N. Holmes, J. G. de Jesus, K. L. Jones, C. M. Soulette, T. P. Snutch, N. Loman, B. Paten, M. Loose, J. T. Simpson, H. E. Olsen, A. N. Brooks, M. Akeson and W. Timp, Nanopore native RNA sequencing of a human poly(A) transcriptome, Nat. Methods, 2019, 16, 1297–1305 CrossRef CAS PubMed.
- H. Priyamvada, K. Kumaragama, A. Chrzan, C. Athukorala, S. Sur and S. Dhaniyala, Design and evaluation of a new electrostatic precipitation-based portable low-cost sampler for bioaerosol monitoring, Aerosol Sci. Technol., 2021, 55, 24–36 CrossRef CAS.
- R. R. Wick, L. M. Judd and K. E. Holt, Performance of neural network basecalling tools for Oxford Nanopore sequencing, Genome Biol., 2019, 20, 129 CrossRef PubMed.
- R. Winand, B. Bogaerts, S. Hoffman, L. Lefevre, M. Delvoye, J. V. Braekel, Q. Fu, N. H. Roosens, S. C. D. Keersmaecker and K. Vanneste, Targeting the 16S rRNA Gene for Bacterial Identification in Complex Mixed Samples: Comparative Evaluation of Second (Illumina) and Third (Oxford Nanopore Technologies) Generation Sequencing Technologies, Int. J. Mol. Sci., 2019, 21, 298 CrossRef PubMed.
- B. Ewing, L. Hillier, M. C. Wendl and P. Green, Base-Calling of Automated Sequencer Traces UsingPhred. I. Accuracy Assessment, Genome Res., 1998, 8, 175–185 CrossRef CAS PubMed.
- E. R. Moore, A. S. Krüger, L. Hauben, S. E. Seal, M. J. Daniels, R. D. Baere, R. D. Wachter, K. N. Timmis and J. Swings, 16S rRNA gene sequence analyses and inter- and intrageneric relationships of Xanthomonas species and Stenotrophomonas maltophilia, FEMS Microbiol. Lett., 1997, 151, 145–153 CrossRef CAS PubMed.
- N. J. Palleroni and J. F. Bradbury, Stenotrophomonas, a New Bacterial Genus for Xanthomonas maltophilia (Hugh 1980) Swings et al. 1983, Int. J. Syst. Evol. Microbiol., 1993, 43, 606–609 CAS.
- R. C. Edgar, Updating the 97% identity threshold for 16S ribosomal RNA OTUs, Bioinformatics, 2018, 34, 2371–2375 CrossRef CAS PubMed.
- S. Maestri, E. Cosentino, M. Paterno, H. Freitag, J. M. Garces, L. Marcolungo, M. Alfano, I. Njunjić, M. Schilthuizen, F. Slik, M. Menegon, M. Rossato and M. Delledonne, A Rapid and Accurate MinION-Based Workflow for Tracking Species Biodiversity in the Field, Genes, 2019, 10, 468 CrossRef CAS PubMed.
- A. P. Heikema, D. Horst-Kreft, S. A. Boers, R. Jansen, S. D. Hiltemann, W. de Koning, R. Kraaij, M. A. J. de Ridder, C. B. van Houten, L. J. Bont, A. P. Stubbs and J. P. Hays, Comparison of Illumina versus Nanopore 16S rRNA Gene Sequencing of the Human Nasal Microbiota, Genes, 2020, 11, 1105 CrossRef CAS PubMed.
- S. M. Karst, M. S. Dueholm, S. J. McIlroy, R. H. Kirkegaard, P. H. Nielsen and M. Albertsen, Retrieval of a million high-quality, full-length microbial 16S and 18S rRNA gene sequences without primer bias, Nat. Biotechnol., 2018, 36, 190–195 CrossRef CAS PubMed.
- R. Ogden, N. Vasiljevic and S. Prost, Nanopore sequencing in non-human forensic genetics, Emerging Top. Life Sci., 2021, 5, 465–473 CrossRef CAS PubMed.
- N. Vasiljevic, M. Lim, E. Humble, A. Seah, A. Kratzer, N. V. Morf, S. Prost and R. Ogden, Developmental validation of Oxford Nanopore Technology MinION sequence data and the NGSpeciesID bioinformatic pipeline for forensic genetic species identification, Forensic Sci. Int.: Genet., 2021, 53, 102493 CrossRef CAS PubMed.
- R. Krishnakumar, A. Sinha, S. W. Bird, H. Jayamohan, H. S. Edwards, J. S. Schoeniger, K. D. Patel, S. S. Branda and M. S. Bartsch, Systematic and stochastic influences on the performance of the MinION nanopore sequencer across a range of nucleotide bias, Sci. Rep., 2018, 8, 3159 CrossRef PubMed.
- M. Jain, S. Koren, K. H. Miga, J. Quick, A. C. Rand, T. A. Sasani, J. R. Tyson, A. D. Beggs, A. T. Dilthey, I. T. Fiddes, S. Malla, H. Marriott, T. Nieto, J. O'Grady, H. E. Olsen, B. S. Pedersen, A. Rhie, H. Richardson, A. R. Quinlan, T. P. Snutch, L. Tee, B. Paten, A. M. Phillippy, J. T. Simpson, N. J. Loman and M. Loose, Nanopore sequencing and assembly of a human genome with ultra-long reads, Nat. Biotechnol., 2018, 36, 338–345 CrossRef CAS PubMed.
- R. M. Leidenfrost, D.-C. Pöther, U. Jäckel and R. Wünschiers, Benchmarking the MinION: Evaluating long reads for microbial profiling, Sci. Rep., 2020, 10, 5125 CrossRef CAS PubMed.
- S. W. Kembel, M. Wu, J. A. Eisen and J. L. Green, Incorporating 16S Gene Copy Number Information Improves Estimates of Microbial Diversity and Abundance, PLoS Comput. Biol., 2012, 8, e1002743 CrossRef CAS PubMed.
- C. M. Burke and A. E. Darling, A method for high precision sequencing of near full-length 16S rRNA genes on an Illumina MiSeq, Peerj, 2016, 4, e2492 CrossRef PubMed.
- B. J. Callahan, J. Wong, C. Heiner, S. Oh, C. M. Theriot, A. S. Gulati, S. K. McGill and M. K. Dougherty, High-throughput amplicon sequencing of the full-length 16S rRNA gene with single-nucleotide resolution, Nucleic Acids Res., 2019, 47, e103 CrossRef CAS PubMed.
- D. Wu, J. Xie, Y. Liu, L. Jin, G. Li and T. An, Metagenomic and Machine Learning Meta-Analyses Characterize Airborne Resistome Features and Their Hosts in China Megacities, Environ. Sci. Technol., 2023, 57, 16414–16423 CrossRef CAS PubMed.
Footnote |
† These authors contributed equally to this work. |
|
This journal is © The Royal Society of Chemistry 2024 |
Click here to see how this site uses Cookies. View our privacy policy here.