Identification of spectral responses of different plastic materials by means of multispectral imaging
Received
28th July 2023
, Accepted 16th November 2023
First published on 8th February 2024
Abstract
In this work, multispectral imaging (MSI) is introduced as an innovative, practical, and non-invasive solution capable of identifying and detecting (micro)plastics. MSI holds significant appeal for industry due to its flexibility, ease of implementation, and portability. The integration of MSI with Principal Components Analysis (PCA) enables precise identification of different plastics and differentiation of microplastics within mixtures. The technique successfully identifies and quantifies the pure spectral response (endmembers) of each microplastic in every pixel of the original image. As a result, the model excels in distinguishing specific plastic materials from their surrounding backgrounds. This novel approach facilitates the identification of randomly dispersed microplastics in water.
Environmental significance
The presented work provides immediate insight to environmental processes and impacts due to the identification of plastic materials in the environment, which has gained a high interest in the last years in particular when not recyclable. In addition we present methods and processes for the identification of overlaid transparent plastics which are definitely challenging. Sensing and identification of plastic contaminants are in fact major issues which, if properly handled, identified and categorized, will help to monitor environmental pollution and reduce its negative impacts on ecosystems. This aspect has created great concern among the scientific community due to its impact on flora and fauna and on human health.
|
1 Introduction
Environmental impact resulting from the release of plastic materials,1,2 particularly if not recyclable, has increased significantly in recent years, creating great concern among the scientific community due to its impact on flora, fauna, and human health. Therefore, the detection and identification of plastic contaminants are the main issues that, if addressed appropriately, could help monitor environmental pollution and mitigate its negative impacts on ecosystems. When plastics are dispersed in the form of microplastics (MPs), serious pollution effects have been detected. These effects include the contamination of marine and aquatic ecosystems, the absorption and accumulation of chemicals, habitat alteration, toxicological effects, bioaccumulation, microplastic transport, and potential risks to human health through the consumption of contaminated seafood.3–8 MPs can exhibit remarkable diversity in their characteristics. They can vary significantly in dimensions, encompassing a wide size range from 5 millimeters down to microscopic scales, such as nanometers. Their structures can take on various forms, including irregularly shaped fragments, spherical microbeads, fibers, or film-like particles, depending on their source and degradation process. Additionally, MPs may possess different densities, with some floating on water surfaces, others sinking to the ocean floor, and some remaining suspended in the water column. In terms of composition, MPs can be crafted from diverse types of polymers, such as polyethylene, polypropylene, polyvinyl chloride (PVC), and more, each with unique properties and environmental behaviors.9 The concentration of microplastics (MPs) in water ecosystems increases as plastic production gradually increases every year.10 Currently, almost 71% of plastic waste is directly absorbed by the environment, and the remaining waste is reused in a different format, leading to increased microplastic pollution.10 Apart from exhibiting different size, structure and polymer type, MPs concentrations are usually unknown11 when dispersed in mixtures of different materials, not only polymers, whose compositions typically influence the overall toxicity.12,13 Consequently, detecting MPs using a single detection method,10 especially when they are present in mixtures of different sizes and materials14 and overlapping, poses significant challenges. This challenge is particularly pronounced when dealing with fragments of transparent plastic labels covering transparent plastic objects.
Various multi-detection techniques have been proposed for MPs detection and can be categorized into two primary groups based on the analytical approach employed. These techniques either rely on physical properties (e.g., color, size) or chemical properties (e.g., composition, structure).15,16 Visual analysis is still used for identifying large (1–5 mm), colored MPs through imaging, but the spatial resolution of cameras limits the detection of particles below 1 mm. Other techniques have been explored, but their effectiveness remains unsatisfactory. Among these, the stereo-microscope was the first to be employed for morphological analysis and counting of MPs in the hundreds of micron range but is constrained by particles shape and size limitations. It is in fact difficult to distinguish between synthetic and natural (e.g., coloured cotton) fibres by microscopy alone.15 It has been estimated that up to 70% of measurement errors can occur during testing, with this error percentage increasing as particle size decreases.17 Scanning electron microscopy (SEM) has been utilized for MPs detection due to its ability to provide high-definition images.18 However, due to its time-consuming and expensive nature, it cannot be used routinely. Energy-dispersive X-ray spectroscopy (EDS), used to determine the chemical compositions of plastic particles, shares similar drawbacks. Accessing suitable facilities regularly and preparing samples require significant time and effort. Non-destructive methods such as Raman spectroscopy and FTIR have been proposed as alternatives, as well as destructive methods like liquid chromatography (LC), gas chromatography-mass spectrometry (GC-MS), including pyrolysis gas chromatography-mass spectrometry, and thermal desorption gas chromatography19–30 respectively. Unfortunately, these methods are limited in their ability to detect microplastics dispersed in water. The main impediments to their routine adoption stem from the lengthy sample preparation procedures and complex measurement protocols, which require skilled experts. They are all expensive and bulky, requiring therefore huge investments and dedicated personnel, especially when data analysis is involved. Furthermore, most of these methods struggle to distinguish both opaque and transparent plastics when partially overlapped. X-ray and hyperspectral imaging yielded recently interesting and promising results when dealing with overlapped plastics,31–33 with proposed techniques which are however unsuited for non-professional or brief trained personnel. Thus, there is a pressing need for a method that can effectively, intuitively, and clearly identify (micro)plastics among different materials in a generic sample while being easily implementable and reproducible.
This study proposes multispectral imaging (MSI) as a highly innovative and practical solution and a non-invasive technique to identify and sense (micro)plastics. It also appeals to industry due to its flexibility, ease of implementation, and portability and combines the benefit of imaging (i.e. spatial resolution) and spectral analysis (i.e. components' fingerprint and quantification). MSI is a technique which allows to acquire reflectance spectrum in a certain frequency interval associated to each pixel of an object image. Thus, it's possible to analyse the surface of an object in a non-invasive way, avoiding potential contamination, and providing at the same time both imaging data and spectral data. Spatially and spectral resolved mapping of the investigated materials are therefore obtained. To address the challenges of (micro)plastics identification and quantification, this study employs Multispectral Imaging (MSI) in combination with Principal Components Analysis (PCA).34 This synergistic approach allows to accurately distinguish between different plastics and identify various microplastics within unknown mixtures. In the process of identifying the “pure spectral responses” (endmembers) inherent to each microplastic and quantifying the abundance present within every pixel of the original image, the capability to effectively differentiate specific plastic materials from the surrounding background emerges. The innovative approach here proposed has found application in a critical case, where the task at hand involves the identification of mixtures composed of microplastics distributed randomly within a water medium.
2 Materials & methods
In this work, multispectral images and corresponding data analysis of pure plastics were conducted under different critical conditions in order to identify and properly solve the main issues related to imaging and plastics detection, including: (i) delineation of plastic responses; (ii) characterization of background contributions; (iii) assessment of spatial and spectral resolutions; and (iv) mitigation of artifacts such as shadows and multiple reflections.
The methodology employed involves the recording of multispectral images, which are subsequently subjected to analysis through Principal Component Analysis.
2.1 Computational model and endmembers extraction
The process of acquiring data with a multispectral camera entails the creation of a Multispectral Cube (MSC).35,36 This cube combines the spatial and spectral characteristics of multiple congruent images captured at different wavelengths (K), resulting in a three-dimensional dataset. The MSC can be regarded as a tensor that encompasses the intensities of all pixels, with its dimensions denoted as M × N × K. Here, the index K refers to all the wavelengths employed by the camera while M and N, for a given K, refer to the coordinates of each pixel.
In order to understand how different materials react when probed with these K wavelengths, each MSC is decomposed into M·N K-dimensional vectors. Consequently, a single vector encompasses all K intensity values, corresponding to the K different wavelengths, associated with a specific pixel. The high dimensionality of the K-dimensional vectors in our data set (K = 8, M = 1280, N = 800, resulting in 1.024.000 vectors each one with 8 entries), can lead to a high degree of computational complexity. To address this issue, Principal Component Analysis (PCA) was used to reduce the dimension of the data set while preserving a significant degree of “variability”. This is possible thanks to the great redundancy (multicollinearity) which is present in the initial data set. By considering the M·N of a MSC and calculating the covariance matrix Γ of sections of the cube which correspond to different wavelengths, one obtains:
| 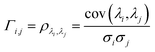 | (1) |
where
ρλi,λj is Pearson's correlation coefficient, cov(
λi,
λj) represents the covariance between section of the cube corresponding respectively to
λi and
λj and
σi and
σj the respective standard deviations. Covariance matrix
Γ can be written also as:
| 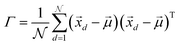 | (2) |
where

are the

vectors of the cube and

is their average. MSC values are real numbers, consequently the entries
Γi,j are also real values.
Γ is symmetric and real and therefore can be diagonalized:
with
C = diag(
c1,
c2,…,
cK), {
ci}
i=1,…,K eigenvalues of
Γ considered in decreasing order, namely
c1 ≥
c2 ≥…≥
cK, also in the construction of the unitary orthonormal matrix
U which contains the eigenvectors of
Γ. The principal components of the initial data set (
i.e. MSC) are obtained by projecting the latter on the obtained eigenvectors. Each principal component is connected to an eigenvalue, from which a “weight” in the computation of the total variance can be derived as:
37 | 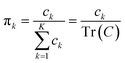 | (4) |
Since the eigenvalues are ordered decreasingly, most of the “variability” of the initial data set will be in the first D < K principal components. Given that
, in order to retain as much variability as possible from the original dataset, this study selected a number D < K of principal components such that
. The principal components can then be obtained by projecting
vectors on the first D orthonormalized eigenvectors, which are on the columns of the matrix W, sub matrix of U, of dimension K × D:
|  | (5) |
If the matrix Γ is computed again with eqn (1), Γi=j = 1 while Γi≠j ≈ 0 showing that the vectors
, which correspond to the
pixels, are completely uncorrelated. The last part of the analysis consists in finding the “spectral responses”38 of different materials. This work assumes that pixels composing the original image is a linear combination of pure spectral responses (endmembers, EMs) i.e. in first approximation one can apply a Linear Mixing Model (LMM). The coefficients of this linear combination are called abundances and represent the fraction in which EMs appear within each pixel. This approach assumes that at least one D-dimensional pixel represents a pure endmember spectrum in the MSC for each different material composing the mixture. The LMM model is applied under the hypotheses that the multiple scattering among individual EMs can be neglected and the surface is partitioned by fractional abundances. In this case in fact the composite spectrum of each pixel is well represented by a LMM.39 Furthermore, the linear mixing model has been applied in cases where photons impinging on the camera sensor interact with just one material. The validation of LMM in the microplastics mixture has become mandatory in this work because the size of the mixed element is microscopic and the detected photons undergo several reflections and refractions, interacting with different materials. As a consequence, a nonlinear mixing model could be guessed from a theoretical point of view.40
After undergoing PCA analysis, each pixel of the multispectral cube is represented by a D-dimensional vector
, the respective principal component. Under the assumptions of LMM, each
is a linear combination of L ≤ D endmembers, that we call
(j = 1, 2, …, L):
| 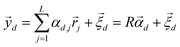 | (6) |
where

is the vector containing all the
L abundances
αd,j of the
d-th pixel,
R is the matrix having as column the
L EMs

while

is a shift accounting for possible noise sources. According to their physical meaning, abundances must be subjected to two constraints:
|  | (7) |
namely they must add up to one for each single pixel, accounting for its composition, and they must be positive numbers. Calling
Y the matrix

containing all the principal components

,
R the matrix
D ×
L of the EMs,
A the matrix

having as columns all the vectors

and
G the matrix

of the noise, we obtain the following equation:
In order to identify different microplastics in a mixture, the spectral responses must be measured. This request is equivalent to determine the abundances contained in A i.e. to invert eqn (9) after having obtained the L endmembers. To attain this result, the N-FINDR algorithm was used, which is founded on the work of Winter et al.41 It involves a spectral unmixing procedure based on a geometric method. The algorithm assumes that the L endmembers form the vertices of a convex simplex in the set
composed by all the data points and defined by the LMM as:
|  | (10) |
The endmembers extraction is nothing but the process of identification of the vertices of the associated convex simplex. The idea behind endmember extraction via the N-FINDR iterative algorithm lies in the assumption that the volume of the simplex whose vertices are determined by the spectral response of a single material (i.e. the purest pixels in the MSC) is always greater than the ones formed by any other combination of pixels.42 Therefore, the goal of N-FINDR algorithm is to find a simplex which can contain as many MSC data points as possible, assuming that each data sample can be described as a linear mixture of its vertices (LMM). In addition, N-FINDR assumes that, for each of the L endmembers there is at least one pixel containing only a single sample material spectral response. L is an integer chosen by the user, which must not exceed the number of spectral dimensions of the MSC. This means that among the pixels, at least L pure spectral responses must exist. Finally, matrix A is obtained solving a Fully Constrained Least Squares (FCLS) problem from an algorithm based on the use of the least-squares error to compute the optimal least-squares value of the abundances. This method, moreover, does not require to estimate the additional noise term.43,44
2.2 Sample preparation
All the plastic samples employed in this work and described in the following section are prepared starting from standard commercially available products, that is, transparent PET water bottles and milk boxes (made of PET and PP) as well as the corresponding PP labels and HDPE/PP caps. In addition to the standard samples, two different mixtures were prepared. The first one was produced starting from a HDPE bottle cap and a PP envelope taken from a common commercial product. The two samples were sliced by using routine and standard mechanical techniques to mime real processes (including abrasion, cutting, fragmentation) so that to produce plastic pieces with size ranging roughly between 0.5 mm and 1 cm. Fragments have been preliminary inspected with optical microscopy. The second mixture is composed of HDPE fragments obtained by abrasion with sandpaper of HDPE's bottle cap and then added to MilliQ® water. The concentration of the obtained suspension is C ≃ 6.4 mg g−1 (wHDPE/wH2O). When sandpaper abrasion has been used, the bottle cap was placed in a cooler at −2 °C for two hours, then in a hot plate at 60 °C for two hours and finally exposed to a UV lamp (P = 9 mW cm−2) for the same amount of time. This process was chosen to facilitate samples fragmentation when wiped with sandpaper and to obtain smaller fragments accordingly.
To further test the sensitivity of the employed multispectral camera in terms of (micro)plastic size detection, a mixture of HDPE fragments and water was also prepared following the previously described procedure for the preparation of the fragments, with a concentration of C = 6.4 mg g−1 (wHDPE/wH2O). 3 mg of this suspension were poured between two microscope glass slides (25.4 mm × 76.2 mm) to obtain a thin film of water in order to reduce the contribution of electromagnetic absorption by water.
2.3 Experimental setup
In this work a commercial HI camera has been used such as Unispectral ColorIR EVK-UNS61000 multispectral camera. The block diagram representing the employed multispectral camera is presented in Fig. 3. It acquires K = 8 images, each one at a single different wavelength λ = 711, 741, 768, 797, 826, 855, 884, 918 nm in the near infrared. For each single λ, equivalent to fixing K, each image has dimensions M × N = 800 × 1280 pixels. Every cube's entry Im,n,k represents a single pixel intensity, with a precision of 10 bit in grey scale, namely 0 ≤ Im,n,k ≤ 1023 with Im,n,k taking only integer values. Before proceeding with the analysis, the intensity is calibrated by using eqn (11), as discussed in the following. The illumination of the sample can be carried out by means of external illumination systems, but the multispectral camera offers a 4-LED panel to light up the sample. The central wavelengths (CWLs) of the illumination LED are 730, 810, 850 and 910 nm. Only two different LEDs can be turned on at the same time.
The pictures of plastic materials, each taken with the 8 different wavelengths, have been acquired under the illumination of one of the four commercially available LEDs (the one at λ = 810 nm) which the camera allows to use while acquiring and a desk spot white LED lamp (IKEA, nominal power: 100 W, 1521 lm). The acquisition of the MSCs was performed at a fixed distance from the samples of ≃25 cm for a total covered area of ≃6 cm2. On the other hand, the duration for multispectral cubes varies in the range 2–15 seconds per multispectral band. This variance in time is attributed to variations in light exposure conditions during data acquisition. The experimental set-up has been minimized in order to be cheap, easy to be reproduced and compatible with any industrial environment. Plastic materials have been placed on a common white paper sheet (A4 – 80 g) which has been used to acquire the background white and dark images to perform a suitable calibration before applying the model previously described.
3 Results
Let I0(m,n,λ) be the acquired image intensity of the pixel at coordinates (m,n) taken at wavelength λ in grey scale and Iw(m,n,λ) the intensity of the white background (without any other object) in the same conditions of illumination. By defining Id(m,n,λ) as the intensity of the dark reference image, the calibrated intensity Im,n,λ is given by: | 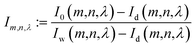 | (11) |
In Fig. 1 two pictures properly calibrated, both corresponding to λ = 797 nm, of two different plastic materials commonly available are shown. In Fig. 1a a transparent PET fragment and in Fig. 1b a HDPE bottle cap are reported as examples. In Fig. 2 the abundance map plot for the transparent PET fragment by setting L = 6 EMs is presented. Each pixel intensity represents the abundance of a given endmember, where darker colours (starting from blue) correspond to a low abundance of the given endmember, while the brighter ones signify a higher abundance, up to 1 for the red colour. The model is hence able to distinguish a certain plastic material with respect to the surrounding background. Fig. 1c and d show the abundance plot of the EM which highlights the plastic material with respect to the background. It is interesting to note that the analytical method proposed in this work is also able to recognize artifacts such as the presence of reflections and halos in the multispectral image (EM 2, EM 4, EM 6 in Fig. 1c) as well as lack of homogeneity in the external illuminations (EM 3 and EM 5 in the same figure). It is therefore possible, by visual inspection, to remove them and prevent biased plastics quantification. The employed model and subsequent analysis are also capable to distinguish between different materials in the same image. After obtaining the endmembers and the abundances are extracted, the abundance map allows to identify the components of a plastic mixture by observing the abundance map of the endmember highlighting one of the possible materials in the sample. An example with 2 different types of plastics, staked one above another, is indeed shown in Fig. 4. The latter is a picture of two bottle caps, a PP one (the one placed under the others) and a HDPE one, together with a fragment of a PP bottle label (Fig. 4a, corresponding to the calibrated picture acquired with λ = 768 nm). As it can be seen in Fig. 4c, only the HDPE cap is highlighted with respect to the background when one specific endmember is chosen. Some pixels in correspondence of the bottle label are of a brighter colour, but with lower intensity when compared to the surrounding cap. In Fig. 4b, the PP cap located under the others and the external borders of the PP label are highlighted. This is coherent with our expectations considering their composition as declared by the manufacturer. Also the border of the HDPE top is evident: this could be due to the fact that it is a transitional region between two different spectral response of the materials as suggested by the fact that in the central part of the cap the dark blue colour dominates. The remaining central part of the label is recognized in an abundance plot referring to another EM (Fig. 4d). When compared to Fig. 4b, it is possible to evidence the presence of reflections of the light source on the label. However, this confirms that our model is able to recognize reflection phenomena, as it can be seen in Fig. 4c on the right, where the circular shape of the stack of caps can be recognized.
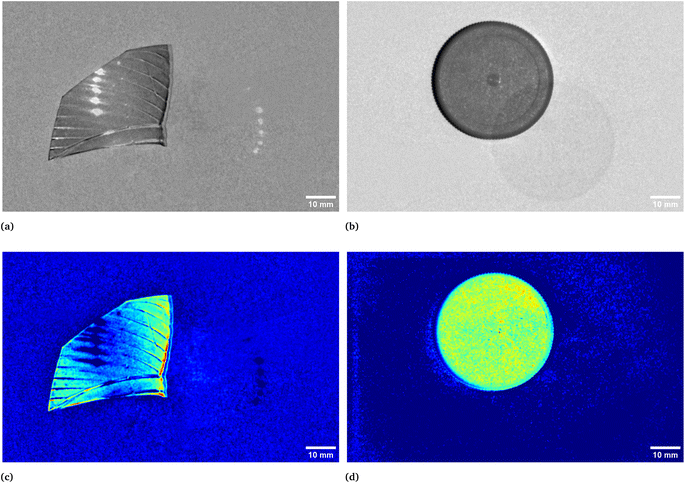 |
| Fig. 1 Calibrated images in grey scale of a transparent bottle PET fragment (a) and of a HDPE bottle cap (b), both acquired at λ = 797 nm. In (c) and (d), respectively, the correspondent abundances plots which in turns correspond to the endmembers representing their spectral responses. The two materials are hence highlighted with respect to the background. In particular, the complete set of abundances plots for the PET fragment is reported in Fig. 2. | |
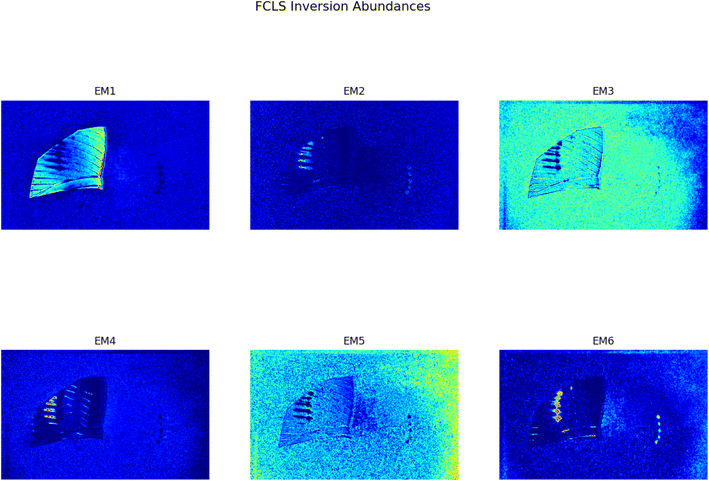 |
| Fig. 2 Set of abundances plots for a transparent PET fragment obtained through the FCLS algorithm and the analysis proposed in this work. The initial calibrated image is reported in Fig. 1a. | |
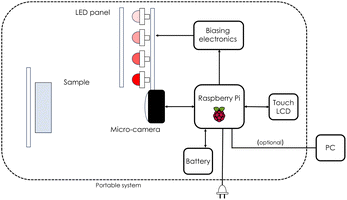 |
| Fig. 3 Block diagram representing the ColorIR EVK-UNS61000 multispectral camera exploited in this work. Diagram adapted from Lopez-Ruiz et al.45 | |
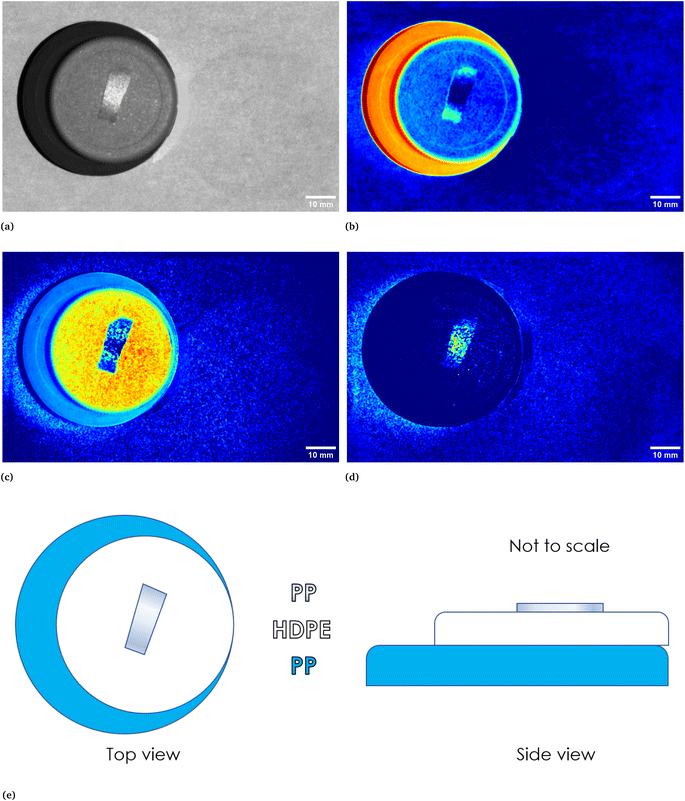 |
| Fig. 4 In (a), calibrated image in grey scale of a stack of three different plastic objects, namely, starting from the bottom, a PP bottle cap, a HDPE bottle cap and a piece of a PP bottle label. In (e) a sketch is reported to show how the three objects were stacked. In (b)–(d) abundances plots corresponding to three different endmembers. In particular, (b) identifies the PP cap at the bottom of the stack and the borders of the upper placed PP label, (c) identifies the HDPE cap while (d) identifies the central part of the PP label, which could be ascribed to a reflection phenomena if compared with the original calibrated image. | |
This analysis proves to be capable of distinguish between two different kind of plastic materials also when the two are reduced to smaller pieces (dimensions ranging between cm and mm). Fig. 5 shows the abundance plots of the L = 6 EMs corresponding to a mixture of PP and HDPE fragments.
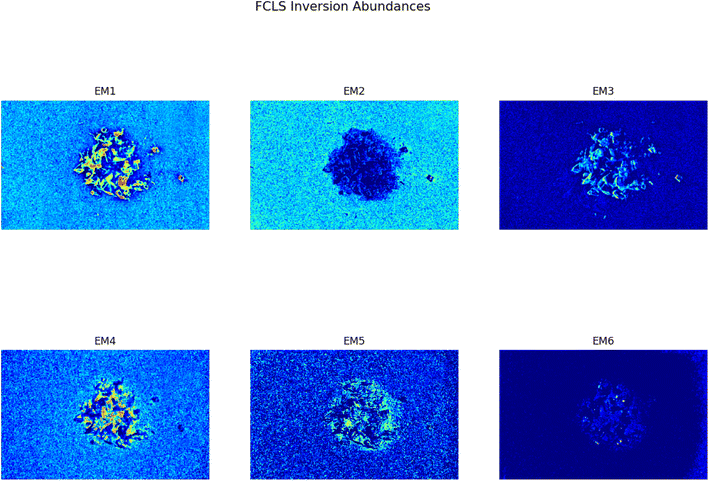 |
| Fig. 5 Set of abundances plots (L = 6) obtained analyzing an image of a mixture of two different plastics fragments, HDPE and PP. As it can be noticed EM 1 and EM 4 correctly identifies the two different materials; these EMs are reported respectively in Fig. 6a and b. The model proposed in this work is capable of distinguish also the background (EM 2) and reflection phenomena as well as halos (EM 3, EM5, EM 6). | |
As it can be seen, two EMs, EM 1 (Fig. 6a) and EM 4 (Fig. 6b), highlight with respect to the background each one a specific part of the mixture. Moreover, EM 1 emphasizes the two isolated small fragments on the right, which are HDPE fragments and which were slightly displaced from the mixture core in order to ease the identification of HDPE fraction after the analysis. The two fragments are in fact depicted with much darker colours in EM 4. Confirming the interpretation that EM 1 shows the abundance corresponding to the spectral response of HDPE, while EM 4 is instead referred to PP. In fact, if the two EMs are superimposed by making EM 4 slightly transparent and filtering out the background of the two EMs, namely setting to dark blue most of the “graininess” pixels region in the background, EM 1 non highlighted region match with the corresponding highlighted region of EM 4, as can be seen in Fig. 7. It is necessary to clarify that the aforementioned filtering out is a forced re-assignment of RGB pixels values which in no way alters the pixels emphasized with brighter colours in the abundance plots in the region of the mixture. Concerning the other EMs of the mixture (Fig. 5), it was observed that the proposed model is able also in this case to identify the background (in EM 2) and halos as well as other reflection phenomena (EM 3, EM 5 and EM 6), as observed in the previous cases. The light blue “graininess” observed in EM 1 and EM 4 is present also in EM 2 (the background) and, partially, in EM 5. This phenomenon, given the great number of objects placed in front of the camera objective, may be due to multiple interactions of light with the plastic materials. In this case photons impinging on the camera sensor could have been interacting with more than only one material and, consequently the LMM is strictly not the best. Its simplicity with respect to a non linear mixing model (LNMM) is however a strong benefit and the results here obtained clearly indicate that it is possible to recognize the regions where LNMM could provide more accurate results. Interestingly, a way to avoid the noisy background in at least one of the two EMs that correctly identifies the two materials is forcing the number of EMs to be L = 8, which yields the result reported in Fig. 8. In these abundances plots, EM 1, reported also in Fig. 6c to ease the visualization, identifies correctly HDPE while EM 7, shown in Fig. 6d, identifies PP. In Fig. 5 it is possible to appreciate the difference between corresponding EMs: imposing L = 8 allows a better identification of HDPE with respect to the background and the other material if compared to the case L = 6, but provides however a less precise recognition of PP. Nevertheless, both values of L allow accurate identification of the two materials composing the mixture, confirming once again the validity and effectiveness of the proposed here model.
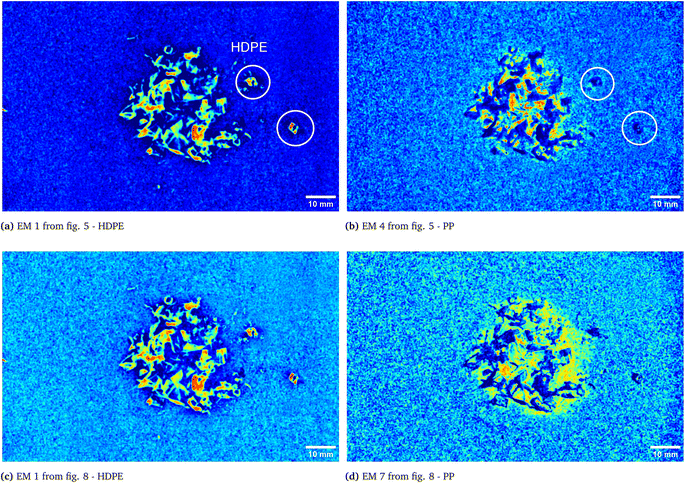 |
| Fig. 6 Abundances plots corresponding to the EMs which identifies the two components fragments, HDPE and PP, of the analyzed mixture. In (a) and (b) EM 1 and EM 4, respectively, from Fig. 5, obtained from the analysis with L = 6, are reported. The displaced HDPE fragments are indicated with white circles. Abundances plots obtained forcing L = 8 are reported in the remaining two pictures, (c) and (d). | |
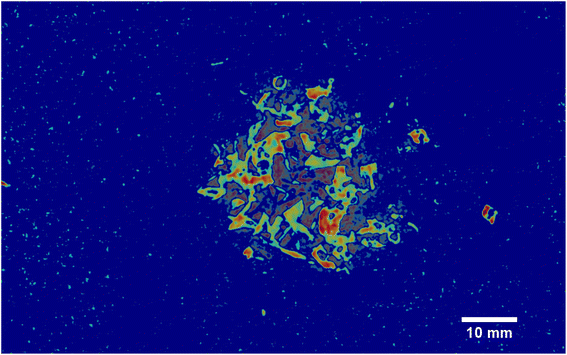 |
| Fig. 7 Overlap of the EMs 1 and 4 from Fig. 5. EM 4 transparency has been increased in order to allow the overlapping with EM 1. Moreover, to avoid the noisy background, the light blue “graininess” pixels have been set to dark blue when possible, without altering in any way the regions highlighted with brighter colours, namely the ones corresponding to the two components. | |
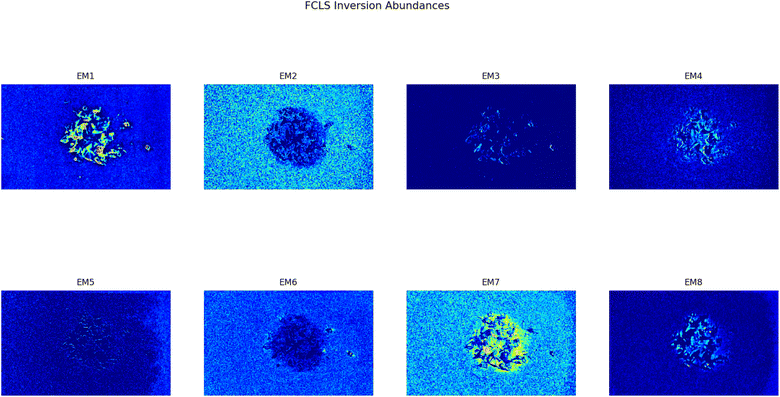 |
| Fig. 8 Set of abundances plots (L = 8) obtained analyzing an image of a mixture of two different plastics fragments, HDPE and PP. As it can be noticed EM 1 and EM 7 correctly identifies the two different materials; these EMs are reported respectively in Fig. 6c and d to ease the comparison with the case L = 6, Fig. 6a and b. | |
To further assess the efficacy of our method, we tested it with a sample consisting in HDPE fragments dispersed in a thin film of water between two glass slides (as shown in Fig. 9, see Section 2.2 for additional details on the preparation of the sample). Fig. 9c shows the EM which highlights the HDPE fragments dispersed in the thin film of water between the two slides and, as can be seen from the reported scale, with the employed device it is possible to detect HDPE fragments down to approximately 0.5 mm. Fig. 9a corresponds to the calibrated picture of the two slides containing the mixture acquired at λ = 797 nm, while Fig. 9b shows the EM which identifies the border of the water film, clearly evident also in Fig. 9a, and some halos in part of the background. This behaviour, as in the case of HDPE and PP mixture, may be attributed to multiple interactions of light with the materials. Nevertheless, the adopted technique allows to distinguish the HDPE fragments from the composite background and it is able to recognize the presence of a thin film of water in the surrounding of the plastic material, together with what can be reasonably interpreted as reflections and halos.
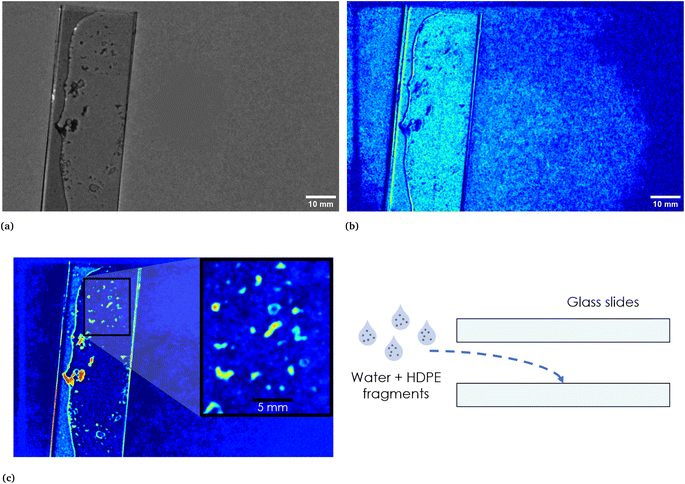 |
| Fig. 9 In (a), calibrated image in grey scale of the thin film of water and HDPE fragments between two microscope glass slides. A sketch of the sample is reported on the right in (c). In the same figure on the left, the EM highlighting the HDPE fragments is reported. The inset shows the scale. In (b), EM which identifies the area corresponding to the water film and the two glass slides and which recognizes reflections and halos present in part of the background. As it can be seen, the darker region in correspondence of the thin film of water corresponds to the HDPE fragments highlighted in the EM reported in (c). | |
4 Conclusions
In this work multispectral infrared imaging and principal components analysis were successfully combined to obtain the spectral responses of different types of polymers starting from a Multispectral Cube (MSC), namely the combination of K congruent images each one acquired at a different wavelength. The MSC in each case was acquired with a commercial multispectral camera (Unispectral ColorIR EVK-UNS61000 multispectral camera) and then analyzed with the model developed in this study. The method proposed in this work proved to be able to identify single polymers alone (HDPE, PET) or stacked together (HDPE, PP) on a white background (a common white paper sheet, A4 – 80 g). The employed technique is moreover capable of distinguishing between two different types of plastics when the latter are reduced to fragments (dimensions ranging between mm to cm) and mixed together in unknown fractions. Artifacts such as the presence of reflections and halos in the multispectral image are also effectively recognized. In terms of (micro)plastics size detection, the proposed analysis, along with the employed multispectral camera, allows the detection of HDPE fragments dispersed in a thin film of water down to 0.5 mm. These promising results open new interesting possibilities in (micro)plastics detection down to 0.5 mm, even in the case of polymers dispersed in small volumes of waters. The required analysis, starting from the MSC, could be easily implemented in a routine software in order to be carried out almost automatically, without the needing of specialised personnel nor bulky and expensive instruments. In fact, in this work, apart from the commercial camera, only a common white paper sheet and a table lamp have been employed to obtain the images at K different wavelengths (the MSC cube). The inherent integration potential of the here proposed technique, its flexibility and portability make it a very robust alternative for plastics sensing in almost every situation in which a fast and effective analysis is required.
Conflicts of interest
There are no conflicts to declare.
Acknowledgements
This work has been supported by Programma Operativo Nazionale “Ricerca e Innovazione” 2014–2020 (CCI 2014IT16M2OP005), risorse FSE REACT-EU, Azione IV.4 “Dottorati e contratti di ricerca su tematiche dell'innovazione” and Azione IV.5 “Dottorati su tematiche Green”. The authors kindly acknowledge Unispectral Ltd. for providing the device employed in this work and for the technical support.
References
- J. N. Hahladakis, C. A. Velis, R. Weber, E. Iacovidou and P. Purnell, An overview of chemical additives present in plastics: Migration, release, fate and environmental impact during their use, disposal and recycling, J. Hazard. Mater., 2018, 344, 179–199 CrossRef CAS PubMed.
- I. E. Napper and R. C. Thompson, Plastic debris in the marine environment: history and future challenges, Global Challenges, 2020, 4, 1900081 CrossRef PubMed.
- J. C. Prata, J. P. da Costa, I. Lopes, A. C. Duarte and T. Rocha-Santos, Environmental exposure to microplastics: An overview on possible human health effects, Sci. Total Environ., 2020, 702, 134455 CrossRef CAS PubMed.
- F. Barbosa, J. A. Adeyemi, M. Z. Bocato, A. Comas and A. Campiglia, A critical viewpoint on current issues, limitations, and future research needs on micro-and nanoplastic studies: From the detection to the toxicological assessment, Environ. Res., 2020, 182, 109089 CrossRef CAS PubMed.
- D. Elkhatib and V. Oyanedel-Craver, A critical review of extraction and identification methods of microplastics in wastewater and drinking water, Environ. Sci. Technol., 2020, 54, 7037–7049 CrossRef CAS PubMed.
- L. Van Cauwenberghe and C. R. Janssen, Microplastics in bivalves cultured for human consumption, Environ. Pollut., 2014, 193, 65–70 CrossRef CAS PubMed.
- C. Espinosa, J. M. G. Beltrán, M. A. Esteban and A. Cuesta, In vitro effects of virgin microplastics on fish head-kidney leucocyte activities, Environ. Pollut., 2018, 235, 30–38 CrossRef CAS PubMed.
- C. Campanale, C. Massarelli, I. Savino, V. Locaputo and V. F. Uricchio, A detailed review study on potential effects of microplastics and additives of concern on human health, Int. J. Environ. Res. Public Health, 2020, 17, 1212 CrossRef CAS PubMed.
- P. Samanta, S. Dey, D. Kundu, D. Dutta, R. Jambulkar, R. Mishra, A. R. Ghosh and S. Kumar, An insight on sampling, identification, quantification and characteristics of microplastics in solid wastes, Trends Environ. Anal. Chem., 2022, 36, e00181 CrossRef CAS.
- T. K. Dey, M. E. Uddin and M. Jamal, Detection and removal of microplastics in wastewater: evolution and impact, Environ. Sci. Pollut. Res., 2021, 28, 16925–16947 CrossRef CAS PubMed.
- N. P. Ivleva, Chemical analysis of microplastics and nanoplastics: challenges, advanced methods, and perspectives, Chem. Rev., 2021, 121, 11886–11936 CrossRef CAS PubMed.
- E. Davarpanah and L. Guilhermino, Are gold nanoparticles and microplastics mixtures more toxic to the marine microalgae Tetraselmis chuii than the substances individually?, Ecotoxicol. Environ. Saf., 2019, 181, 60–68 CrossRef CAS PubMed.
- L. Guilhermino, L. R. Vieira, D. Ribeiro, A. S. Tavares, V. Cardoso, A. Alves and J. M. Almeida, Uptake and effects of the antimicrobial florfenicol, microplastics and their mixtures on freshwater exotic invasive bivalve Corbicula fluminea, Sci. Total Environ., 2018, 622, 1131–1142 CrossRef PubMed.
- F. Caputo, R. Vogel, J. Savage, G. Vella, A. Law, G. Della Camera, G. Hannon, B. Peacock, D. Mehn and J. Ponti,
et al., Measuring particle size distribution and mass concentration of nanoplastics and microplastics: addressing some analytical challenges in the sub-micron size range, J. Colloid Interface Sci., 2021, 588, 401–417 CrossRef CAS PubMed.
- W. J. Shim, S. H. Hong and S. E. Eo, Identification methods in microplastic analysis: a review, Anal. Methods, 2017, 9, 1384–1391 RSC.
- J. Sun, X. Dai, Q. Wang, M. C. Van Loosdrecht and B.-J. Ni, Microplastics in wastewater treatment plants: Detection, occurrence and removal, Water Res., 2019, 152, 21–37 CrossRef CAS PubMed.
- V. Hidalgo-Ruz, L. Gutow, R. C. Thompson and M. Thiel, Microplastics in the marine environment: a review of the methods used for identification and quantification, Environ. Sci. Technol., 2012, 46, 3060–3075 CrossRef CAS PubMed.
- D. A. Cooper and P. L. Corcoran, Effects of mechanical
and chemical processes on the degradation of plastic beach debris on the island of Kauai, Hawaii, Mar. Pollut. Bull., 2010, 60, 650–654 CrossRef CAS PubMed.
- M. A. Browne, P. Crump, S. J. Niven, E. Teuten, A. Tonkin, T. Galloway and R. Thompson, Accumulation of microplastic on shorelines worldwide: sources and sinks, Environ. Sci. Technol., 2011, 45, 9175–9179 CrossRef CAS PubMed.
- E. Fries, J. H. Dekiff, J. Willmeyer, M.-T. Nuelle, M. Ebert and D. Remy, Identification of polymer types and additives in marine microplastic particles using pyrolysis-GC/MS and scanning electron microscopy, Environ. Sci.: Processes Impacts, 2013, 15, 1949–1956 RSC.
- J. H. Dekiff, D. Remy, J. Klasmeier and E. Fries, Occurrence and spatial distribution of microplastics in sediments from Norderney, Environ. Pollut., 2014, 186, 248–256 CrossRef CAS PubMed.
- M.-T. Nuelle, J. H. Dekiff, D. Remy and E. Fries, A new analytical approach for monitoring microplastics in marine sediments, Environ. Pollut., 2014, 184, 161–169 CrossRef CAS PubMed.
- I. Hintersteiner, M. Himmelsbach and W. W. Buchberger, Characterization and quantitation of polyolefin microplastics in personal-care products using high-temperature gel-permeation chromatography, Anal. Bioanal. Chem., 2015, 407, 1253–1259 CrossRef CAS PubMed.
-
M. G. Löder and G. Gerdts, Methodology used for the detection and identification of microplastics—a critical appraisal, Marine Anthropogenic Litter, 2015, pp. 201–227 Search PubMed.
- E. Dümichen, P. Eisentraut, C. G. Bannick, A.-K. Barthel, R. Senz and U. Braun, Fast identification of microplastics in complex environmental samples by a thermal degradation method, Chemosphere, 2017, 174, 572–584 CrossRef PubMed.
- A. M. Elert, R. Becker, E. Duemichen, P. Eisentraut, J. Falkenhagen, H. Sturm and U. Braun, Comparison of different methods for MP detection: what can we learn from them, and why asking the right question before measurements matters?, Environ. Pollut., 2017, 231, 1256–1264 CrossRef CAS PubMed.
- G. Erni-Cassola, M. I. Gibson, R. C. Thompson and J. A. Christie-Oleza, Lost, but found with Nile red: a novel method for detecting and quantifying small microplastics (1 mm to 20 μm) in environmental samples, Environ. Sci. Technol., 2017, 51, 13641–13648 CrossRef CAS PubMed.
- S. M. Mintenig, I. Int-Veen, M. G. Löder, S. Primpke and G. Gerdts, Identification of microplastic in effluents of waste water treatment plants using focal plane array-based micro-Fourier-transform infrared imaging, Water Res., 2017, 108, 365–372 CrossRef CAS PubMed.
- C. F. Araujo, M. M. Nolasco, A. M. Ribeiro and P. J. Ribeiro-Claro, Identification of microplastics using Raman spectroscopy: Latest developments and future prospects, Water Res., 2018, 142, 426–440 CrossRef CAS PubMed.
- M. Lares, M. C. Ncibi, M. Sillanpää and M. Sillanpää, Occurrence, identification and removal of microplastic particles and fibers in conventional activated sludge process and advanced MBR technology, Water Res., 2018, 133, 236–246 CrossRef CAS PubMed.
- A. Brambilla, A. Gorecki, A. Potop, C. Paulus and L. Verger, Basis material decomposition method for material discrimination with a new spectrometric X-ray imaging detector, J. Instrum., 2017, 12, P08014 CrossRef.
-
G. Martinez, M. Aghaei, M. Dijkstra, B. Nagarajan, F. Jaarsma, J. van de Loosdrecht, P. Radeva and K. Dijkstra, 2022 IEEE International Conference on Image Processing (ICIP), 2022, pp. 2331–2335 Search PubMed.
-
F. Hollstein, M. Wohllebe and S. Arnaiz, Identification and sorting of plastics film waste by NIR-Hyperspectral-Imaging, Proceedings of the ICNIRS, 2015 Search PubMed.
-
I. T. Jollyfe, Principal Component Analysis, Springer, 2002 Search PubMed.
-
H. Grahn and P. Geladi, Techniques and Applications of Hyperspectral Image Analysis, John Wiley & Sons, 2007 Search PubMed.
- C. Pasquini, Near infrared spectroscopy: A mature analytical technique with new perspectives–A review, Anal. Chim. Acta, 2018, 1026, 8–36 CrossRef CAS PubMed.
-
G. R. Naik, Advances in Principal Component Analysis: Research and Development, Springer, 2017 Search PubMed.
-
S. Prasad and J. Chanussot, Hyperspectral Image Analysis: Advances in Machine Learning and Signal Processing, Springer Nature, 2020 Search PubMed.
- J. M. Bioucas-Dias, A. Plaza, N. Dobigeon, M. Parente, Q. Du, P. Gader and J. Chanussot, Hyperspectral unmixing overview: Geometrical, statistical, and sparse regression-based approaches, IEEE J. Sel. Top. Appl. Earth Obs. Rem. Sens., 2012, 5, 354–379 Search PubMed.
- J. Wei and X. Wang, An overview on linear unmixing of hyperspectral data, Math. Probl. Eng., 2020, 2020, 1–12 Search PubMed.
-
M. E. Winter, Imaging Spectrometry V, 1999, pp. 266–275 Search PubMed.
-
A. Plaza and C.-I. Chang, Algorithms and Technologies for Multispectral, Hyperspectral, and Ultraspectral Imagery XI, 2005, pp. 298–306 Search PubMed.
- N. Keshava and J. F. Mustard, Spectral unmixing, IEEE Signal Process. Mag., 2002, 19, 44–57 CrossRef.
- B. Rasti, B. Koirala, P. Scheunders and P. Ghamisi, How hyperspectral image unmixing and denoising can boost each other, Remote Sens., 2020, 12, 1728 CrossRef.
- N. Lopez-Ruiz, F. Granados-Ortega, M. A. Carvajal and A. Martinez-Olmos, Portable multispectral imaging system based on Raspberry Pi, Sens. Rev., 2017, 37, 322–329 CrossRef.
|
This journal is © The Royal Society of Chemistry 2024 |
Click here to see how this site uses Cookies. View our privacy policy here.