Food quality assessment using chemoresistive gas sensors: achievements and future perspectives
Received
20th October 2023
, Accepted 13th December 2023
First published on 3rd January 2024
Abstract
Real-time monitoring of food freshness facilitates the supply of safe and high-quality food products to customers. Various gases, including C2H4, NH3, C3H9N, and H2S, can be generated during the spoilage or ripening process of food, providing insights into the state of food freshness. Chemoresistive gas sensors represent one of the reliable pathways to assess food quality due to their high sensitivity, fast response speed, and easy integration. In this paper, we review recent progress in chemoresistive gas sensors for applications in food quality monitoring. Diverse materials, such as metal oxides, carbon-based materials, transition metal dichalcogenides, and other emerging materials, have been proposed with discussions on their sensing mechanisms. This review primarily focuses on the key strategies to enhance the sensitivity and selectivity of specific food quality marker gases using the chemical properties of materials. Additionally, we address the remaining challenges hindering the practical application of chemoresistive gas sensors, such as water poisoning, power consumption, and sensor reliability, while proposing potential solutions. The chemoresistive sensor platform, encompassing functional sensing materials and data recognition systems, can pave the way for real-time food quality monitoring technology in the future.
Sustainability spotlight
Various gases generated during the food spoilage or ripening process are reliable indicators for food freshness assessment. In this perspective, chemoresistive gas sensors are plausible candidates for real-time food quality monitoring due to their advantages such as cost effectiveness, fast response, and facile miniaturization. Developing high performance chemoresistive gas sensors and combining them with machine learning technologies can be a sustainable strategy for ensuring the consistent delivery of high quality food products to consumers. We believe this review could pave the way for sustainable possibilities in agricultural and food packaging applications.
|
1. Introduction
To date, with the rapid growth of the food industry and expansion of the supply chain, real-time monitoring of food freshness is demanded to ensure the safety and quality of food products. The early-stage monitoring of food spoilage not only reduces the risk of waste products but also prevents food-borne disease. Furthermore, for foods such as fruits that undergo maturation, monitoring their ripening stages enables the large-scale cultivation of high-quality products.
Food products undergo various changes over time due to microbial growth or metabolism. Traditionally, diverse techniques have been used to detect such changes and assess overall food quality. For instance, a pH indicator, like a colorimetric sensor,1,2 can evaluate changes in food acidity caused by the production of organic acids or bases. This sensor allows real-time monitoring of food quality and can be integrated into food packaging. However, variations in temperature and illumination conditions may pose challenges in accurately determining the actual state of the product via visual means.3,4 Microbial sensors, on the other hand, measure the presence of microorganisms formed during food spoilage, providing an estimation of food quality.5,6 These sensors use fluidic devices containing antibodies that react with bacteria. While they offer real-time and large-scale measurement of microbial presence within food products, they require sophisticated equipment.
Recently, researchers have proposed an alternative method for monitoring food quality that involves detecting gases produced during the spoilage or ripening stages of food. For instance, trimethylamine (C3H9N, TMA) is generated during the spoilage process of fish and meats and its concentration gradually increases over time.7 Similarly, ethylene (C2H4) is a plant hormone gas produced during the growth and ripening of fruits.8 Therefore, the detection of these gases gives a reliable pathway for food freshness monitoring. In this regard, chemoresistive gas sensors can be suggested as a valuable technology for real-time food quality assessment. They take numerous advantages such as fast response speed, cost-effectiveness, and miniaturization capabilities.9,10 To date, several results have been reported on food-generated gas detection using chemoresistive gas sensors.11
In this review, we will explore the fundamental operating mechanism of chemoresistive gas sensors and introduce their research trends in food quality monitoring fields. The main focus of this review is the achievement of selective detections toward target gases for real-time application, where numerous gas species coexist simultaneously. Furthermore, the challenges, potential solutions, and future perspectives of employing chemoresistive gas sensors for food quality monitoring will be discussed.
2. Chemoresistive gas sensors: overview
For real-time detection of food freshness, it is necessary to develop a sensor platform with integrated sensor arrays (Fig. 1). To achieve this, single gas sensors with high sensitivity and selectivity to various food freshness marker gases, even at low concentrations, should be evolved. In this section, we will provide a detailed review of the operating mechanism, evaluation parameters, candidate materials, and design method for chemoresistive gas sensors.
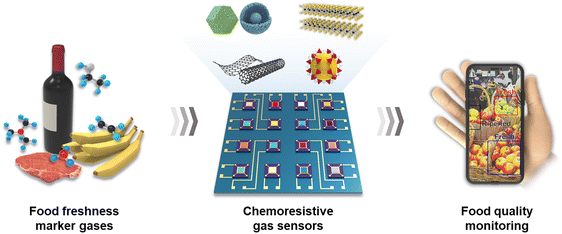 |
| Fig. 1 Schematic diagram of chemoresistive gas sensors for food quality monitoring. | |
2.1 Operating mechanism of chemoresistive gas sensors
The operating mechanism of chemoresistive gas sensors has been extensively reported.12 The general operating principle involves the injection or extraction of charge carriers within the material through redox reactions between active adsorbed oxygen species and the target gases. This mechanism is illustrated in Fig. 2.
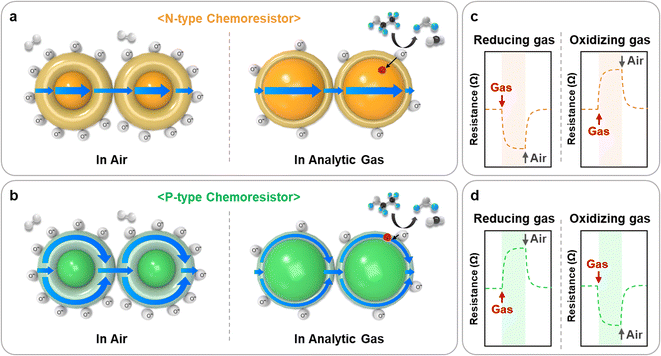 |
| Fig. 2 Gas sensing mechanism and resistance transients of (a), (c) n-type and (b), (d) p-type chemoresistive gas sensors. | |
In the ambient atmosphere, negatively charged oxygen species (O2−, O−, and O2−) adsorb onto the surface of the material, resulting in the formation of electron depletion layers (EDLs) in n-type materials (Fig. 2a) and hole accumulation layers (HALs) in p-type materials (Fig. 2b). Upon exposure to reducing gases such as CO, C2H5OH, and NH3, these gases react with adsorbed oxygen species and inject electrons into the EDLs or HALs of the material. Therefore, the resistance of the chemoresistor decreases (n-type) or increases (p-type) (Fig. 2c and d). On the other hand, oxidizing gases such as NO2 directly adsorb onto the surface of materials, extracting electrons and thereby expanding the EDLs or HALs. This leads to an increase (n-type) or a decrease (p-type) in the resistance of the material.
Some specific chemoresistors, especially two-dimensional (2D) nanomaterials, operate through an alternative sensing mechanism.13 Analytic gas molecules directly interact with material and exchange charge carriers. In p-type 2D materials, oxidizing gases (NO2) withdraw electrons, inducing a decrease in resistance. In contrast, reducing gases (CO and NH3) inject electrons, resulting in an increase in resistance. The n-type 2D materials exhibit the opposite phenomenon of chemoresistive variations.
2.2. Evaluation parameters of chemoresistive gas sensors
The gas sensing performance can be evaluated using several parameters, including response, sensitivity, selectivity, limit of detection, response/recovery times, and stability. These parameters play a vital role in choosing the appropriate solutions for specific applications. The response (S) of the sensor (n-type) is usually defined as RaRg−1 − 1 when the sensor is exposed to reducing gas and as RgRa−1 − 1 when exposed to oxidizing gas; where Ra and Rg represent the sensor resistance in air and analyte gas, respectively. Sensitivity refers to the change in response per unit concentration of analyte gas. This can be calculated as the slope of the plot showing analyte gas response vs. concentrations. Another crucial parameter for determining sensing performance is selectivity. Selectivity represents the ability of a sensor to detect a target gas despite the presence of interfering gasses. Typically, it is defined as response ratio, ST/SI, where ST and SI are the sensor responses to the target gas and interfering gas, respectively.
The limit of detection (LOD) is the lowest measurable concentration of analyte gases. Representative criteria for calculating the theoretical detection limit include RaRg−1 >1.2 (ref. 14 and 15) and a signal-to-noise ratio >3.16,17 Additionally, the dynamic behavior of the sensor can be evaluated by measuring the response and recovery times. The response time is the duration needed to achieve stable sensor resistance when the sensor is exposed to analyte gas. Conversely, recovery time is the time required for the sensor to return to its initial resistance in the absence of analyte gas. Typically, the time taken to reach 90% of the resistance change is referred to as the response and recovery time. The reliability of the sensor can be confirmed by measuring the repetitive sensor response over an extended period. Also, the stability of the sensor under varying humid conditions is a significant factor, and it will be discussed in detail in the following session.
2.3. Materials for chemoresistive gas sensors
The primary operation principle of chemoresistive gas sensors relies on the variations in the electrical resistance of sensing material upon exposure to the analytic gas. Various materials have been employed for chemoresistors, including metal oxide semiconductors (MOSs), carbon-based materials, and transition metal dichalcogenides (TMDs) (Table 1).
Table 1 Material type, materials, typical operating temperature, and major target gases of chemoresistive gas sensors
Material type |
Materials |
Operating temp. |
Target gases |
Ref. |
Metal oxide |
SnO2, WO3, In2O3, TiO2, Fe2O3, NiO, Co3O4 |
200–500 °C |
Most gases |
14, 18–20, 50, 52 |
Carbon-based materials |
CNTs, Gr, rGO |
RT–100 °C |
NO2, NH3 |
23–25
|
TMDs |
MoS2, SnS2, WS2, In2S3, MoSe |
RT–250 °C |
NO2, H2S, NH3 |
28–30
|
MOFs |
Cu3(HHTP)2, Ni3(HITP)2, Co3(HITP)2 |
RT–150 °C |
NO2, NH3, H2S |
39, 40 |
MXenes |
Ti3C2Tx, V2CTx, V4C3Tx |
RT–150 °C |
C2H5OH, CH3COCH3, H2, CH4 |
43–45
|
MOSs such as SnO2, WO3, In2O3, Co3O4, and NiO have been used as sensing materials for detecting various gases, including C2H5OH, NH3, H2S, and CO.18–21 These MOSs possess abundant adsorbed oxygen species, which play a crucial role in inducing resistance changes and contributes to their excellent sensing performance. However, their operating temperatures are relatively high (200–500 °C), resulting in high power consumption and poor long-term stability.
From this perspective, carbon-based materials such as carbon nanotube (CNTs), graphene (Gr), and reduced graphene oxide (rGO) have been suggested as another candidate for chemoresistors owing to their flexibility, low operating temperature (RT–100 °C), and high chemical tunability.22–27 Furthermore, TMDs such as MoS2, WS2, SnS2, and MoSe2 have received significant attention as sensing materials for detection of NH3, H2S, and NO2.28–31 Their 2D structures with high specific surface areas and abundant active edge sites enhance the adsorption of gas molecules, resulting in sensitive sensing performance.32,33 The unique characteristics of 2D TMDs, such as adjustable bandgap and flexibility, provide a diverse range of sensing materials with relatively low operating temperatures (RT–250 °C).
As emerging materials, metal–organic frameworks (MOFs) are being explored for their application in chemoresistive gas sensors due to their diverse advantages such as abundant mesopores, ultrahigh specific surface area, and high catalytic activities.34–36 Their porous structures provide high gas accessibility and abundant adsorption sites which facilitate the gas sensing reactions.37,38 Furthermore, some specific 2D structured conductive MOFs can be operated even at room temperature (RT) with high gas selectivity.39,40 Additionally, other materials such as covalent organic frameworks (COFs),41,42 MXene,43–45 or their hybrids46–49 are garnering intense attention as potential candidates for gas sensing applications.
2.4. Material design for chemoresistive gas sensors
Nanostructured materials with abundant active sites and high gas accessibility facilitate rapid and effective sensing reactions. The analytic gas permeates sensing materials through their pores and reacts with adsorbed oxygen species. Therefore, it is crucial to design the configuration of nanostructures such as size, porosity, and thickness to enhance gas sensing performance. For example, our coworkers prepared highly gas accessible Fe2O3 nanotube arrays via the glancing angle deposition (GAD) process.50 The vertically aligned Fe2O3 nanotube arrays exhibited improved acetone response compared to dense planar Fe2O3 thin film. Notably, the Fe2O3 nanotube arrays showed a rapid response time of less than 3 s. This can be attributed to their large specific surface area and the presence of nanosized narrow necks.16 Jo et al. reported hierarchical Co3O4 hollow nanocages prepared by MOF template.51 The shell of hollow nanocages was composed of thin nanosheet building blocks, facilitating the gas permeation within the nanostructure. The response to xylene increased as both the size and shell thickness of the nanocages decreased. However, when the size of nanocages became extremely small, the xylene response decreased significantly due to the reduced gas accessibility caused by the particle agglomeration. These findings suggest that optimal particle size and thickness should be considered when designing gas sensing materials. Numerous studies have focused on improving gas sensing ability via designing nanostructures such as nanowires, nanosheets, hollow, hierarchical, and porous films.52–55
Forming a heterostructure or heterojunction between sensing materials and catalysts such as metal and metal oxide can be a viable strategy to control sensing characteristics. This approach allows the modulation of the thickness of EDLs/HALs, tuning of catalytic activity, and the adjustment of chemical affinity to specific gases.56,57 First, the enlargement in EDLs/HALs increases sensor response due to the higher variation in charge carrier concentration upon exposure to analyte gases. Therefore, metal and metal oxide catalysts with high work functions have been loaded/doped onto sensing materials to achieve high gas response. For instance, our coworkers decorated Au nanoparticles (NPs) on WO3 to increase response to ethanol and NO2 by decreasing the background charge carrier concentration of WO3.58 Likewise, we also fabricated the Fe2O3 loaded NiO nanorods via GAD which exhibited ultrasensitive toluene sensing properties by forming a p–n junction.59 Second, the incorporation of additives with sensing materials can increase the number of active sites, thereby contributing to enhanced catalytic activity. Zhou et al. reported Rh-doped SnO2 as a highly selective acetone sensor.60 The Rh doping elevated the number of adsorbed oxygens, leading to a high acetone response. Also, Lee et al. fabricated a highly sensitive volatile organic compounds (VOCs) sensor by loading Au NPs onto the In2O3 inversed opal thin films.61 The Au NPs effectively promoted oxygen adsorption, resulting in increased VOCs response. Lastly, additives showing high chemical affinity to specific gases can be employed to enhance gas sensing properties. For example, Choi et al. reported the CuO-loaded SnO2 hollow spheres as an ultrasensitive H2S gas sensor.62 Compared to the pristine SnO2, the CuO-loaded SnO2 showed a 7.1 times higher response to H2S. This can be attributed to the high chemical affinity of CuO toward H2S, which converts CuO into CuS upon exposure to H2S, resulting in a significant decrease in sensor resistance.63 Also, loading Pd and PdO can increase H2 response, as Pd triggers the H2 spill-over effect; which decompose H2 molecules into H atoms. For instance, Zhou et al. fabricated a highly sensitive and selective H2 gas sensor by loading Pd NPs on W18O49.64 By loading Pd NPs on W18O49, the response to H2 increased preferentially. Also, Kim et al. reported a PdO-functionalized SnO2 as a selective H2 sensor.65 Compared to the pristine SnO2, the PdO-functionalized SnO2 exhibited a 6.4 times higher response to H2, attributable to the Pd-induced spillover effect. Overall, forming appropriate heterojunctions with sensing materials and additives can effectively enhance sensitivity and selectivity.
Functional overlayers, when deposited onto the sensing layer, possess the capability to enhance sensitivity and selectivity toward target gases. One approach involves employing a physical filtering method, utilizing the difference in molecular sizes of gases to selectively detect small-sized gases. For instance, Jo et al. suggested a selective formaldehyde sensor using a ZIF-7/PEBA mixed matrix membrane (MMM) coated TiO2 sensor.14 The pristine TiO2 sensor showed a high response to both ethanol and formaldehyde. However, upon applying the MMM coating on the sensing layer, larger ethanol molecules were filtered out, allowing only smaller formaldehyde molecules to penetrate the sensing layer. As a result, this sensor exhibited remarkable selectivity towards formaldehyde. Alternatively, catalytic filtering layers can be applied, considering the reforming/oxidation reactions of the analyte gases. Moon et al. designed a Rh–TiO2 overlaid SnO2 sensor that selectively detected benzene over xylene and toluene.66 The catalytic Rh–TiO2 layer facilitated the conversion of stable benzene into reactive species (e.g., aldehydes), while the interfering gases were completely oxidized (e.g., CO2 and H2O). As demonstrated in the previous reports, the application of overlayers with physical or chemical filtering abilities can enhance the selectivity and sensitivity of chemoresistive gas sensors.
3. Chemoresistive gas sensors for food quality monitoring
Chemoresistive gas sensors are currently employed in various fields such as breath analysis, environmental, and industrial applications.67,68 In particular, the detection of specific gases generated during the process of food spoilage, fermentation, and ripening, can provide information about the freshness stage of food items. The number of publications and citations related to this application have been significantly increased and accounted for a large proportion compared to other fields (Fig. 3) In this section, we will focus on the development trends in chemoresistive gas sensors for detecting various food freshness marker gases and their underlying mechanisms. Table 2 provides the summary of various chemoresistive gas sensors to detect food quality marker gases.
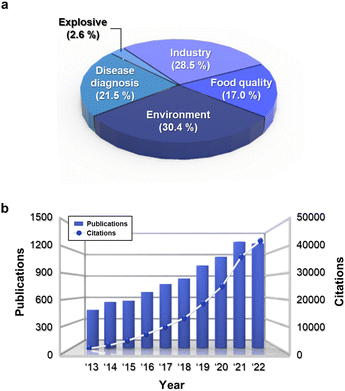 |
| Fig. 3 (a) Diverse applications of chemoresistive gas sensors reported in the literature, (b) the number of publications and citations on chemoresistive sensors for food quality monitoring. The data was collected using Web of Science – Web of Science Core Collection Search: keywords of (gas sens* or chemiresis* or chemoresis*) and environment* or industry* or breath* or health* or food or explosive* were used. | |
Table 2 Summary of chemoresistive gas sensors for food quality marker gases (response was defined as RaRg−1, RaRg−1 − 1, RgRa−1, RgRa−1 − 1, IaIg−1, IaIg−1 − 1, IgIa−1, or IgIa−1 − 1)
Gas |
Materials |
Conc. [ppm] |
Response |
Operating temp. [°C] |
LOD [ppb] |
Food |
Ref. |
C2H4 |
Cr2O3–SnO2 hollow spheres |
2.5 |
16.8 |
350 |
24 |
Banana |
69
|
Pd–V2O5–TiO2/In2O3 bilayer |
1 |
18.6 |
325 |
7.3 |
Banana |
70
|
Cu-SWNT |
50 |
1.7 |
RT |
— |
Banana, avocado, apple, pear, orange |
71
|
Pd–SnO2 nanoparticle |
100 |
11.1 |
250 |
50 |
Banana, lemon, apple, pear |
72
|
ZnO nanosheet |
200 |
6.4 |
500 |
1.0 × 104 |
Banana |
73
|
NH3 |
WS2 thin film |
0.2 |
2.1% |
30 |
100 |
— |
74
|
MoS2 thin film |
30 |
0.1% |
30 |
300 |
— |
75
|
MoS2 flakes |
50 |
6.58 |
150 |
— |
Fish (stingray) |
76
|
PTS-doped PANI |
5 |
2.25 |
30 |
— |
Fish, pork, chicken |
77
|
CuBr film |
5 |
30 |
RT |
0.2 |
— |
78
|
Fluorinated GO |
500 |
121% |
RT |
6.1 |
— |
25
|
TiO2/MXene |
30 |
41.0% |
RT (UV) |
0.2 |
Fish, pork, shrimp |
79
|
CNT/metalloporphyrin |
20 |
4.4% |
RT |
500 |
Cod, salmon, pork, chicken |
80
|
C3H9N |
WO3 hollow sphere |
5 |
56.9 |
450 |
11.8 |
— |
81
|
α-MoO3 nanorod |
200 |
413 |
RT |
1000 |
— |
82
|
Pr-doped Ce4W9O33 |
20 |
20.1 |
350 |
— |
— |
83
|
MoO3 nanoplate |
5 |
374.7 |
300 |
45 |
— |
84
|
Co3O4@ZnO hollow cage |
33 |
41 |
250 |
130 |
— |
85
|
Au–Cr2O3 yolk shell |
5 |
200.9 |
225 |
4.3 |
— |
86
|
5,5′′-Di(naphthalen-1-yl)-2,2′:5′,2′′-terthiophene (NA-3T-NA) |
2.1 × 105 |
4.1 |
RT |
2.2 × 104 |
Fish, pork, chicken |
87
|
H2S |
Ordered mesoporous WO3 |
50 |
269 |
250 |
— |
— |
88
|
Bi2S3/SnS2 nanoparticle |
0.5 |
12.3 |
RT |
— |
— |
89
|
CdS-ATP nanowire |
10 |
9.99% |
RT (visible light) |
0.1 |
— |
90
|
Ag-doped graphene |
0.5 |
37% |
RT |
100 |
— |
91
|
MIL-88B (Fe) nanoparticle |
100 |
1056 |
RT |
170 |
— |
92
|
Ni3(HHTP)2 thin film |
5 |
20.85 |
RT |
3 |
— |
93
|
SnSe2/WO3 nanoparticle |
10 |
33.8% |
RT |
28 |
Egg |
94
|
NiO-doped CuO composite |
1 |
106% |
180 |
1 |
Fish, pork |
95
|
Macroporous ZnO@ZIF-8/Pt |
5.5 |
118 |
310 |
40 |
Fish, shrimp |
96
|
Inverse opal ZnCoOx@BM-ZIF |
5 |
260 |
180 |
70 |
Chicken |
97
|
CH3CHO |
Al-doped ZnO |
0.4 |
50.6 |
200 |
— |
— |
98
|
Hierarchical SnO2 nanoparticle |
100 |
178.3 |
243 |
500 |
— |
99
|
CH3COOH |
Hierarchical SnO2 nanoflowers |
100 |
47.7 |
260 |
— |
— |
100
|
Mesoporous CuO nanoparticle |
100 |
27.2 |
200 |
— |
— |
101
|
Peptide encapsulated SWCNTs |
0.4 × 104 |
21% |
RT |
— |
Wine |
102
|
3.1. Ethylene (C2H4) sensors
Ethylene (C2H4) is a plant hormone gas that evolves during ripening, and it has numerous effects on the growth, senescence, seed germination, ripening, browning, and softening of fruits and vegetables.103,104 As fruits ripen, the emitted concentration of ethylene increases, accelerating the ripening process and ultimately leading to fruit spoilage. Thus, detecting ethylene in a sensitive and selective manner can be a useful strategy to determine the freshness of fruits and vegetables.
In general, most oxide semiconductors generally exhibit low response and selectivity to ethylene, as it is the simplest alkene with non-polarity and strong bonds. To overcome this limitation, pretreatment of gases such as the chemical oxidation of interference gases into less reactive or non-reactive species (e.g., CO2 and H2O) and the ethylene reforming reaction into more reactive intermediates(e.g., aldehydes) have been suggested. Jeong et al. reported a sensitive and selective ethylene sensor based on a Cr2O3–SnO2 bilayer consisting of hollow SnO2 spheres as a sensing layer and a nanoscale catalytic Cr2O3 overlayer (Fig. 4a).69 The SnO2 sensing layer was prepared using the screen-printing method and the catalytic Cr2O3 overlayer (∼300 nm) was coated via electron-beam evaporation. The Cr2O3–SnO2 sensor exhibited a significant increase in ethylene selectivity after coating the Cr2O3 layer (Sethylene/SI >3.3 to 2.5 ppm analytic gases at 375 °C), which was 8.0 times higher than that of the pristine SnO2. The underlying mechanisms of these results can be explained as the chemical filtration of Cr2O3 which induces the catalytic oxidation of more reactive interfering gases into non- or less-reactive species (e.g., CO2 and H2O) while maintaining the response to relatively stable ethylene. They further evaluated the potential of the Cr2O3–SnO2 sensor for fruit quality monitoring applications by investigating the time-dependent changes in the sensor response during fruit ripening. Moon et al. fabricated the Pd–V2O5–TiO2 yolk–shell coated In2O3 bilayer sensor for ethylene detection (Fig. 4b).70 They used the Pd–V2O5–TiO2 yolk–shell overlayer as a heterogeneous Wacker oxidation catalyst, which reforms less reactive ethylene into highly reactive acetaldehyde. This reforming reaction improved the response to ethylene, as acetaldehyde is a well-known reactive species. The conversion of ethylene to acetaldehyde through the Pd–V2O5–TiO2 overlayer was confirmed using proton transfer quadrupole mass spectroscopy. The Pd–V2O5–TiO2/In2O3 bilayer sensor exhibited 15.5 times higher sensitivity to ethylene (RaRg−1 − 1 = 18.6 to 5 ppm at 300 °C) compared to that of the pristine In2O3 sensor and 41.4 times higher selectivity owing to the catalytic filtering of Pd–V2O5–TiO2.
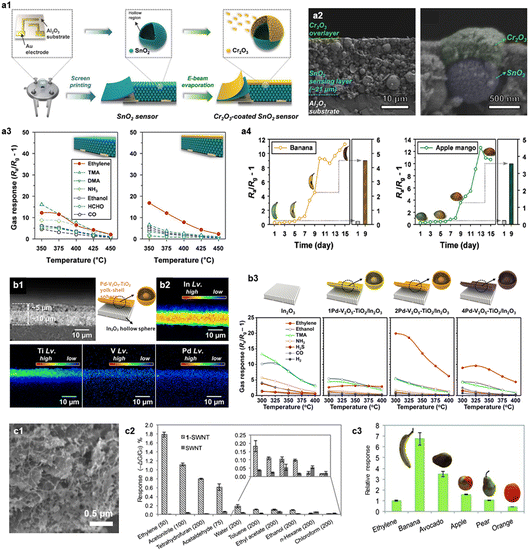 |
| Fig. 4 Ethylene sensors. (a1) Illustration of Cr2O3–SnO2bilayer sensor fabrication process, (a2) cross-section scanning electron microscopy (SEM) images of Cr2O3–SnO2, (a3) gas sensing characteristics of the pristine SnO2 sensor and the Cr2O3–SnO2 bilayer sensor at the temperature range of 350–450 °C (concentration: 2.5 ppm), (a4) gas sensing properties of the Cr2O3–SnO2 bilayer sensor to banana and apple mango. Reproduced with permission from ref. 69 Copyright 2019, Wiley. (b1) Cross-sectional backscattered image of Pd–V2O5–TiO2/In2O3 bilayer sensor, (b2) electron probe micro analysis (EPMA) elemental (In, Ti, V and Pd) images of bilayer, (b3) gas sensing properties of the pristine In2O3 sensor and the mPd–V2O5–TiO2/In2O3 (m = 1, 2, 4) bilayer sensor. Reproduced with permission from ref. 70 Copyright 2023, The Royal Society of Chemistry. (c1) SEM image of 1-SWNT, (c2) gas response of the 1-SWNT and the SWNT sensor to various analyte gases at RT (concentration: 50 ppm), (c3) relative response of the 1-SWNT sensor to 100 g of different fruit to 20 ppm of ethylene. Reproduced with permission from ref. 71 Copyright 2012, Wiley. | |
Ethylene sensors using carbon-based materials have been also reported. Esser et al. prepared a single-wall carbon nanotube with Cu(I) complexes (1-SWNT) for an ethylene sensor.71 They proposed the Cu(I) complex as an ethylene receptor, as Cu(I) has been found to be a cofactor of ethylene receptor (ETR1) in plants during the ripening process (Fig. 4c). The 1-SWNT showed significantly enhanced ethylene response and selectivity compared to those of the pristine SWNT. They further confirmed the feasibility of 1-SWNT sensor to real-time fruit freshness quality monitoring by measuring the time-dependent changes in the sensor response upon exposure to various fruits (banana, avocado, apple, pear, and orange).
3.2. Ammonia (NH3) sensors
Ammonia (NH3) is a toxic and harmful gas emitted from fish and meat, resulting from the bacterial degradation of protein in foods.105 Certain types of fish, including fermented skates, possess a strong ammonia odor, which is intensified as spoilage advances.106 Consequently, the detection of NH3 can serve as a reliable indicator of the freshness or spoilage state of fish and meat.
Several research studies were conducted to detect NH3 at low concentration levels in a highly selective and sensitive manner. Especially, sulfide-based TMD materials, including MoS2, exhibited a high sensitivity to NH3 due to the their chemical affinity.74,75 Our group reported a highly selective NH3 sensor with low operating power (19 mW) by integrating the MoS2 nanoflakes onto a MEMS (micro-electromechanical systems) platform (Fig. 5a).76 The pristine MoS2 exhibited a high response and selectivity to NH3 (RaRg−1 − 1 = 4.7, SNH3/SI >8.0 to 50 ppm at 150 °C). It is attributable to the strong binding energy of the MoS2 edge site to NH3, which was confirmed by density functional theory (DFT) calculations. The sensing performance of this sensor was further examined under exposure to a fresh skate for 5 days at RT. At fresh state, the sensor showed a negligible response, however, after 5 days, the response reached 26.5. The sensor response exhibited a linear correlation with fermentation time, suggesting that this sensor has the potential as a reliable food quality monitoring application.
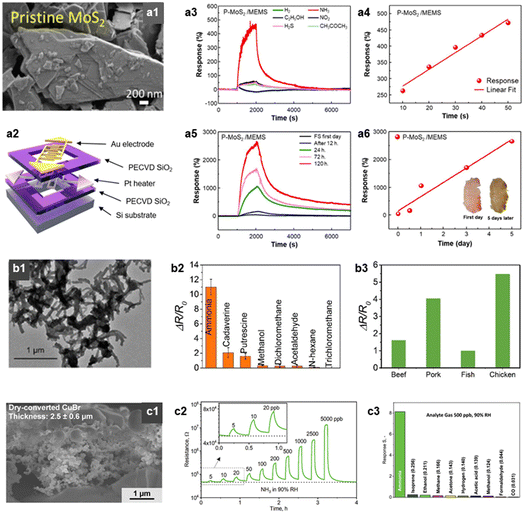 |
| Fig. 5 NH3 sensors. (a1) SEM image of MoS2 nanoflakes, (a2) schematic illustration of MEMS sensor platform (operating power = 19 mW), (a3) sensor response transients of MoS2-MEMS gas sensor to various analytic gases (concentration: 50 ppm), (a4) MoS2-MEMS sensor response to various concentrations of NH3 (concentration: 10–50 ppm), (a5 and a6) MoS2-MEMS sensor response after exposure to skate depending on the fermentation time. Reproduced with permission.74 Copyright 2023, American Chemical Society. (b1) Transmission electron microscope (TEM) image of PTS-PANI, gas responses (ΔRR0−1) of PTS-PANI sensor to (b2) various analytic gases and (b3) four kinds of raw meats (beef, pork, fish, and chicken). Reproduced with permission.75 Copyright 2018, American Chemical Society. (c1) Cross-sectional SEM image of CuBr films, (c2) resistance change transient of CuBr gas sensor upon exposure to various concentration of NH3 (concentration: 5–5000 ppb, RT, 90% RH), (c3) response (RgRa−1 − 1) of CuBr sensor to various analytic gases (concentration: 500 ppb). Reproduced with permission.76 Copyright 2020, Wiley. | |
Furthermore, NH3 was selectively detected using conducting polymer or ionic conductor thin films.107 Ma et al. reported a highly sensitive and wireless meat spoilage sensor using polyaniline (PANI) based material (Fig. 5b).77 They doped iron(III) p-toluene sulfonate hexahydrate (PTS) in PANI to enhance the sensing performance, and this sensor exhibited a high response (ΔRR0−1 = 2.25) toward 5 ppm NH3. Furthermore, the author measured the sensor response to various meat products (pork, beef, chicken, and fish) at 30 °C for 20 h. The sensor showed a high response (RgRa−1 − 1 = 1.0–5.0) which varied with the type of meat, due to the different protein levels. Güntner et al. reported a rapid and selective NH3 sensor using a porous CuBr film (Fig. 5c).78 The sensor discriminated NH3 by forming a stable amine complex (Cu(NH3)2+), resulting in a significant decrease in conductivity. The porous film structure (porosity = 78%) facilitated the efficient NH3 diffusion and interaction with the CuBr surface. Therefore, this sensor exhibited rapid response speed (2.2 min), high selectivity (SNH3/SI > 30), and low detection limit (LOD = 210 ppt with the criterion of signal-to-ratio >3) at RT and highly humid atmosphere (90% RH; relative humidity). Additionally, there are several reports on the development of highly selective, flexible, and RT operating NH3 sensors using emerging materials such as graphene, carbon nanotube, and Mxene.25,79,80
3.4. Trimethylamine (C3H9N) sensors
Most marine mammal products contain trimethylamine oxide (TMAO), which is converted into TMA gas through the metabolism process of anaerobic bacteria.108,109 Moreover, various volatile basic nitrogen gases, including TMA, are generated during the deterioration of meat.110,111 The concentration of TMA increases as fishery products or meat products undergo spoilage, therefore, it can serve as a criterion for assessing the freshness of seafood and meat products.112,113 For example, reported literature suggested a correlation between fish freshness and TMA concentration (fresh: 110 ppm, early corrosion: 10–50 ppm, rotting: >60 ppm).114
TMA is a representative amine gas that exhibits a high chemical affinity to oxides with a highly acidic surface. Cho et al. prepared a high-performance TMA sensor using WO3 hollow spheres (Fig. 6a).81 This sensor showed a high gas response (RaRg−1 = 56.9 at 450 °C) to 5 ppm TMA and a high selectivity (STMA/SI > 1.7) over other interfering gases. Additionally, Srinivasan et al. proposed a MoO3 nanorod gas sensor with high response to 200 ppm TMA (RaRg−1 = 413 at 30 °C; Fig. 6b).82 This sensor also showed excellent sensing performance to other amine gases (Ra/Rg−1 = 63 and 39 for dimethylamine and monomethylamine, respectively.) Considering that both WO3 and MoO3 have relatively high surface acidity,115 utilizing the acid–base interaction can be served as a promising pathway for a high-performance TMA sensor.83,84
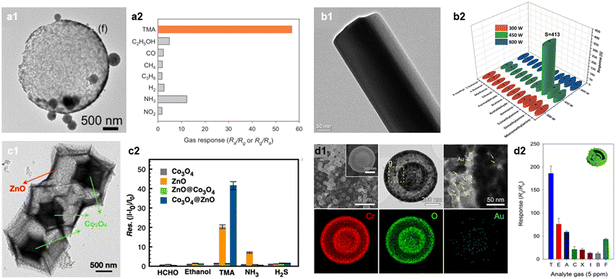 |
| Fig. 6 TMA sensors. (a1) SEM image of WO3 hollow spheres, (a2) gas response (RaRg−1) of WO3 hollow sphere sensor to various analytic gases (concentration: 5 ppm). Reproduced with permission.81 Copyright 2020, Elsevier. (b1) TEM image of α-MoO3 nanorod, (b2) gas response (RaRg−1) of α-MoO3 nanorod sensor to various gases at RT. Reproduced with permission (concentration: 200 ppm).82 Copyright 2021, Elsevier. (c1) TEM image of Co3O4@ZnO nanocage, (c2) gas response (II0−1 − 1) of Co3O4@ZnO sensor to various analytic gases at 250 °C (concentration: 100 ppm). Reproduced with permission.85 Copyright 2021, American Chemical Society. (d1) SEM, TEM, and EDS elemental mapping images of Au–Cr2O3 yolk–shell spheres, (d2) gas response (RgRa−1) of Au–Cr2O3 yolk–shell gas sensor to various analytic gases at 225 °C (concentration: 5 ppm). Reproduced with permission.86 Copyright 2017, Elsevier. | |
Furthermore, considering that TMA has a large molecular size, increasing the adsorption of oxygen species by adding an oxidative catalyst and designing nanostructures are the effective strategies to enhance the TMA sensing ability. Yan et al. reported a MEMS based TMA sensor using a Co3O4@ZnO hollow cage synthesized with a MOF template (Fig. 6c).85 The Co3O4@ZnO hollow cage exhibited enhanced TMA sensing performance (IgIa−1 − 1 = 41 to 33 ppm) compared to that of the bare ZnO (IgIa−1 − 1 = 20). The hollow cage structure with large surface area (48.4 m2 g−1) and high porosity provided excellent gas accessibility and abundant active sites for oxygen species to adsorb, resulting in a high gas response. Also, the formation of p(Co3O4)–n(ZnO) junction increased the thickness of EDLs, inducing the higher variation in sensor resistance upon exposure to TMA. Kim et al. prepared an Au-loaded Cr2O3 yolk–shell nanostructure via spray pyrolysis for its use as a TMA sensor (Fig. 6d).86 This sensor exhibited a high gas response (RgRa−1 = 200.9) and selectivity (STMA/SI > 2.1) to 5 ppm of TMA at 250 °C. Furthermore, the calculated LOD (using RgRa−1 >1.2 as a criterion) was as low as 4.3 ppb, indicating the potential use of this sensor for seafood freshness assessment. The synergistic combination of the highly gas accessible nanostructure and catalytic promotion effect of Au nanoparticles results in the superior TMA sensing performance.
3.5. Hydrogen sulfide (H2S) sensors
Hydrogen sulfide (H2S) is a colorless, flammable, and corrosive gas with the distinctive smell of rotten eggs. H2S is easily produced during meat spoilage, resulting from the decomposition of sulfur-containing amino acids.116–118 Hence, detecting H2S can be a useful strategy to monitor meat freshness quality.
Various H2S sensors are suggested using MOSs and TMDs. For instance, Li et al. prepared a well-ordered mesoporous WO3 using the template-carbonization method for the H2S sensor (Fig. 7a).88 The high mesoporosity and surface area of WO3 resulted in a high H2S response (RaRg−1 = 269 to 50 ppm at 250 °C) and selectivity (SH2S/SI > 6). Also, Wang et al. synthesized the Bi2S3/SnS2 heterostructure via a solvothermal method and evaluated its sensing properties at RT.89 The Bi2S3/SnS2 sensor exhibited a high H2S sensitivity (RaRg−1 = 12.3 to 0.5 ppm) and selectivity (SH2S/SI > 3.0), attributable to the enlargement of EDLs and increased active sites after forming the heterojunctions. The CdS-based metal chalcogenide nanowire has been also reported by Jin et al. to detect H2S sensitively.90 The author functionalized the surface of CdS nanowires with an amino group (ATP = 4-aminothiophenol), as the amino group can selectively interact with H2S via hydrogen bonding (Fig. 7b). The ATP functionalized CdS (Cd-ATP) nanowire showed an excellent response (RaRg−1 = 9.99 to 10 ppm), selectivity, and response/recovery time to H2S under visible light irradiation at RT. Considering those reports, H2S can be effectively detected by designing highly, gas accessible structures with abundant active sites.
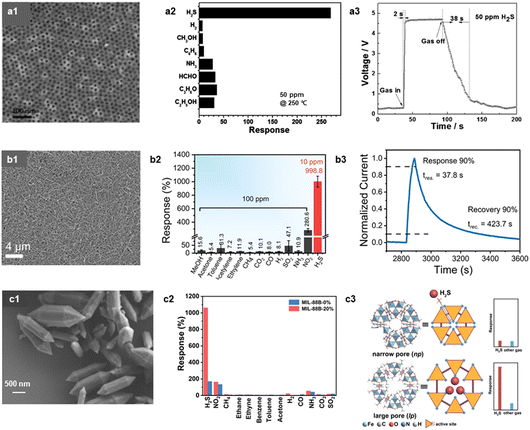 |
| Fig. 7 H2S sensors. (a1) SEM image of the ordered mesoporous WO3, (a2) gas response (RaRg−1) of the ordered mesoporous WO3 to various analyte gases at 250 °C (concentration: 50 ppm), (a3) the response and recovery curve of the ordered mesoporous WO3 to 50 ppm of H2S at 250 °C. Reproduced with permission.88 Copyright 2014, Wiley. (b1) SEM image of the Cd-ATP nanowires, (b2) gas response (RaRg−1) of the Cd-ATP sensor to various analyte gases under visible light at RT, (b3) response and recovery curve of the Cd-ATP nanowire sensor to 100 ppm H2S. Reproduced with permission.90 Copyright 2023, The Royal Society of Chemistry. (c1) SEM image of the MIL-88B-20%, (c2) gas response (II0−1 − 1) of the MIL-88B-0% and the MIL-88B-20% to different analyte gases at RT (concentration: 100 ppm), (c3) illustration of pore-sized dependent gas sensing properties. Reproduced with permission.92 Copyright 2023, Wiley. | |
Recently, carbon-based materials and MOF have garnered interest as emerging sensing materials. For instance, Ovsianytskyi et al. reported an Ag-doped Gr sensor, fabricated via the wet chemical method and measured its sensing properties toward H2S.91 The high chemical affinity of Ag to H2S enhanced the response and selectivity of Ag-doped Gr sensor compared to those of the pristine Gr sensor. Moreover, Wang et al. prepared a H2S sensor using Fe-based MIL-88B, synthesized by a solvothermal method (Fig. 7c).92 They controlled the pore size of MIL-88B by optimizing the solvent. An increase in the pore size of MIL-88B improved the H2S sensitivity (IgIa−1 − 1 = 10.56 to 100 ppm at RT) and selectivity compared to those of the MIL-88B with smaller pores. This enhancement is attributed to the increased surface area and improved gas accessibility to the inner Fe active sites, which was corroborated using Brunauer–Emmett–Teller (BET) analysis and DFT calculation. In addition, Lee et al. reported a thin-film-structured Ni3(HITP)2, a conducting MOF, which exhibited excellent H2S sensitivity (ΔRRa−1 = 20.85 to 5 ppm at RT).93 The author suggested that the 1D pore system achieved by the planar metal–ligand complex and the oriented nature of Ni3(HITP)2 promoted the binding and redox reaction to H2S.
3.6. Others
In addition to C2H4, TMA, NH3, and H2S, VOCs can also serve as food spoilage markers for real-time monitoring. For example, acetaldehyde (CH3CHO), a well-known byproduct of alcohol breakdowns, is produced during the spoilage of chicken, coffee, and dairy products, including milk.119–121 Hagedorn et al. proposed an Al-doped ZnO for the highly sensitive detection of acetaldehyde.98 By doping Al on ZnO, the amount of adsorbed oxygen species on the surface of ZnO increased significantly, resulting in an extremely high acetaldehyde response at low temperature (RaRg−1 = 356 to 5 ppm at 210 °C). Additionally, Li et al. fabricated the hierarchical butterfly-like SnO2 nanosheets via a hydrothermal method to detect acetaldehyde.99 The proposed SnO2 nanosheets possessed a highly porous structure with a high specific surface area and abundant active sites, attributable to a selective acetaldehyde sensing property (Sacetaldehyde/SI > 4.4).
Furthermore, acetic acid (CH3COOH) is produced during the alcoholic fermentation of wine, making it a useful indicator for assessing wine freshness.122,123 Jin et al. reported an acetic acid sensor using three-dimensional hierarchical flower-like SnO2 synthesized by a template-free hydrothermal method.100 The flower-like SnO2 exhibited a high response to acetic acid (RaRg−1 = 47.7 to 100 ppm at 260 °C) with a significantly rapid response and recovery time, owing to its high specific surface area. Similarly, Geng et al. enhanced the acetic acid sensing properties by increasing the surface area and porosity of CuO.101 The mesoporous CuO synthesized using a silica template showed a high response to acetic acid (RaRg−1 = 27.2 to 100 ppm at 200 °C) with reversible sensing behavior.
4. Challenges and future perspectives
Various advances in chemoresistive gas sensors for food freshness monitoring have been achieved, resulting in the developments of sensors with high response, selectivity, rapid response/recovery behavior, and relatively small LOD. Nevertheless, chemoresistive gas sensors still face challenges such as water poisoning, power consumption, and low data accuracy. In this section, we present the primary challenges in optimizing chemoresistive gas sensors and propose possible solutions.
4.1. Water poisoning
Water poisoning deteriorates the performance of chemoresistive gas sensors as H2O interacts with active sensing sites, resulting in the formation of hydroxyls on the surface of sensing materials. This hinders the formation of surface adsorbed oxygen species and/or adsorption of target molecules, leading to reduced gas sensing properties such as a decrease in response and change in resistance. Given that water vapor is a prevalent interferent in food freshness monitoring systems, achieving humidity-independent properties in gas sensors is important. Applying water-resistant layers on the sensing materials, inhibiting the penetrations of H2O while enabling O2 permeation, can be a viable solution to suppress water poisoning. For instance, Jeong et al. prepared a hydrophobic Tb4O7-coated In2O3 gas sensor to detect acetone without humidity dependence (Fig. 8a).124 The Tb4O7 overlayer blocked the water and prevented the formation of hydroxyl groups on the surface of sensing materials. This humidity resistance layer was also successfully applied to diverse materials including SnO2, ZnO, and Pd/SnO2, confirming the general validity of Tb4O7 as a water-blocking layer. This phenomenon is also observed in other hydrophobic materials such as PDMS125 and ZIF-8.126 In addition, additives such as Pd, Rh, Sb, NiO, CuO, CeO2, and Pr could be employed for suppressing humidity-dependent characteristics as they could capture or remove hydroxyls.127–132 For examples, Yoon et al. proposed the underlying mechanism of CeO2 to remove the humidity effect on sensing performance (Fig. 8b).133 Upon exposure to water vapor, Ce4+ ions reduces to Ce3+, and hydrogen ions (H+) are generated. These chemical species react with hydroxyl groups on the In2O3 surface, regenerating the Ce4+ species and water vapor molecule. This phenomenon, called a hydroxyl scavenging reaction, gives humidity-independence to the sensing materials. Accordingly, designing appropriate heterojunctions between sensing materials and water-resistant components can be further explored for achieving humidity-resistant properties.
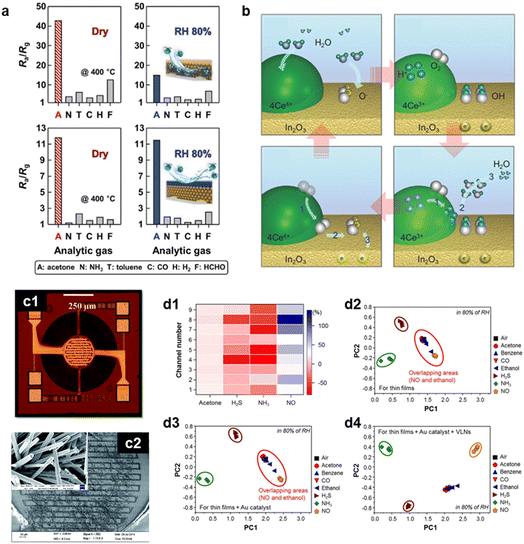 |
| Fig. 8 (a) Gas response (RaRg−1) of the pure In2O3 (upper) and the Tb4O7 coated In2O3 (lower) sensors exposed to 10 ppm analyte gases in dry and 80% RH conditions. Reproduced with permission.124 Copyright 2020, Wiley. (b) Illustrations showing the fundamental mechanisms behind the humidity-independent properties of a CeO2 coated In2O3 sensor. Reproduced with permission.133 Copyright 2016, Wiley. (c1) Optical microscope image of CMOS MEMS, (c2) SEM image of the MEMS based ZnO sensor. Reproduced with permission.137 Copyright 2015, The Royal Society of Chemistry. (d1) Color-mapped responses of sensor array to H2S, NH3, acetone and NO. PCA plots of (d2) thin films, (d3) Au functionalized thin films, (d4) Au functionalized villi-like structured thin films with PC1 and PC2 using responses of 8 different analyte gases in 80% RH conditions. Reproduced with permission.146 Copyright 2016, American Chemical Society. | |
4.2. Power consumption
In general, chemoresistive gas sensors require thermal activation to generate charge carriers and enhance the number of surface oxygen species. Therefore, an external heater is necessary to ensure a consistent temperature of sensors for an extended period, even in varying atmospheric conditions. Thus, the power consumption of the sensor system should be considered for its practical use in real-time applications. These limitations regarding high power consumption can be solved by fabricating MEMS based gas sensors. The chemoresistive gas sensors with MEMS technology are composed of sensing materials and a heater in a single chip, which have advantages in miniaturization and small operating power.134–136 For instance, Santra et al. utilized complementary metal oxide semiconductor (CMOS) MEMS to chemoresistive gas sensors to reduce power consumption of ZnO based ethanol sensor (Fig. 8c).137 The CMOS MEMS showed a high thermal efficiency of 8.2 °C mW−1, resulting in only 42.7 mW to heat up to 350 °C. Therefore, MEMS-based sensors can pave a new way for IoT application of food freshness monitoring. Furthermore, as an alternative to heaters, the utilizing of light sources such as light-emitting diodes (LEDs) can be suggested as another solution to enhance the material stability and lower operating power. A light source with a higher energy than the bandgap of the materials can generate the charge carriers and increase the conductivity at RT. This activation not only enhances the sensor response, but also improves the response and recovery kinetics.138,139 Various strategies were reported to optimize the light activation effect including nanostructure design, heterojunction formation, and localized surface plasmon resonance.140–142 Also, integration of the sensor platform with micro-LEDs can further enhance the sensor performance by reducing the distance between the light source and sensing materials.143–145 Likewise, a light-activated gas sensor platform can be suggested as a rational strategy for the use of chemoresistive gas sensors in food quality monitoring.
4.3. Sensor accuracy and reliability
The low reliability of chemoresistive sensor, accompanied by the difficulty in discriminating target gas over other interferents, should be improved for its practical use for real-time freshness monitoring applications. The integration of several sensors possessing different sensing properties and applying pattern recognition enables the precise discrimination of the mixture gases in real situations. For example, Moon et al. integrated nine different sensors by fabricating 3 × 3 sensor arrays and measured the responses of sensors toward eight different analytic gases (CH3COCH3, C6H6, CO, C2H5OH, H2S, NH3, and NO; Fig. 8d).146 They mapped the response amplitudes on a color scale and further investigated the data based on the principal component analysis (PCA). The sensor arrays showed the high selectivity toward H2S, NH3, and NO, implying that using multiple sensors is advantageous in precise discrimination of gases. Similarly, Jeong et al. discriminated between aromatic and nonaromatic gases by using a nine sensor array and PCA.147 Notably, this sensor array not only differentiated aromatic and nonaromatic gases, but also quantitatively identified aromatic gases.
Additionally, utilizing machine learning to chemoresistive gas sensors could increase the reliability of sensor arrays by analyzing the response signals with sensitivity, and response/recovery speed at different temperatures and concentrations. For instance, Schroeder et al. fabricated twenty CNT-based chemical sensor arrays to discriminate the odor of cheese, liquor, and edible oil.148 Through the investigation of gas sensing transient data using two different models followed by training and testing, the proposed model classified samples of several foods with up to 91% accuracy. Moreover, Oh et al. successfully discriminated five different indoor air pollutants (C6H6, C8H10, C7H8, HCHO, and C2H5OH) under varied temperature and humid conditions by adopting machine learning to In2O3 based sensor array.149 Overall, applying sensor array and machine learning to food quality monitoring applications is expected as a breakthrough in the reliability problem.
5. Conclusions
Chemoresistive gas sensors have emerged as a promising tool for real-time freshness monitoring, addressing the growing demands of the food industry. In this review, we have summarized the recent progress and future perspectives regarding chemoresistive sensors for monitoring food freshness. The detection of representative food quality marker gases, including C2H4, NH3, C3H9N, and H2S, has been achieved using metal oxides, carbon-based materials, TMDs, and other emerging materials. The selective detection of target gases, even in the presence of interferences, was achieved through the design of sensing materials with distinct chemical affinities or catalytic effects for specific gases. Therefore, designing sensing materials with desired chemical properties appears to be a promising strategy for real-time food freshness assessments. Nevertheless, challenges and limitations remain in the field of chemoresistive gas sensors, such as humidity-dependency, power consumption, and a lack of reliability. Thus, we have also described recent attempts to develop high-performance sensors by designing sensing materials and utilizing new technologies. Moreover, employing sensor arrays and machine learning based signal processing in gas analysis enables the selective discrimination between food quality marker gases. The development of diverse chemoresistive gas sensors with the adoption of innovative technologies will open up a new avenue in a wide range of agricultural and food packaging applications.
Author contributions
Seon Ju Park: investigation, visualization, original draft preparation. Soo Min Lee: investigation, visualization, original draft preparation. Mi-Hwa Oh: writing – reviewing and editing, funding acquisition. Yoon Suk Huh: writing – reviewing and editing, funding acquisition. Ho Won Jang: supervision, writing – reviewing and editing, supervision.
Conflicts of interest
There are no conflicts to declare.
Acknowledgements
This research was financially supported by Cooperative Research Program for Agriculture Science and Technology Development (Project No. PJ017067022022) Rural Development Administration, Republic of Korea. This work also supported by Nano Material Technology Development Program (2022M3H4A1A01011993) through NRF (National Research Foundation of Korea), funded by the Ministry of Science and ICT, Republic of Korea.
Notes and references
- M. Alizadeh-Sani, E. Mohammadian, J.-W. Rhim and S. M. Jafari, Trends Food Sci. Technol., 2020, 105, 93–144 CrossRef CAS.
- B. Kuswandi, N. P. Asih, D. K. Pratoko, N. Kristiningrum and M. Moradi, Packag. Technol. Sci., 2020, 33, 321–332 CrossRef CAS.
- E. Balbinot-Alfaro, D. V. Craveiro, K. O. Lima, H. L. G. Costa, D. R. Lopes and C. Prentice, Food Eng. Rev., 2019, 11, 235–244 CrossRef.
- C. Medina-Jaramillo, O. Ochoa-Yepes, C. Bernal and L. Famá, Carbohydr. Polym., 2017, 176, 187–194 CrossRef CAS PubMed.
- Y. Yano, M. Numata, H. Hachiya, S. Ito, T. Masadome, S. Ohkubo, Y. Asano and T. Imato, Talanta, 2001, 54, 255–262 CrossRef CAS PubMed.
- G. Pampoukis, A. E. Lytou, A. A. Argyri, E. Z. Panagou and G.-J. E. Nychas, Sensors, 2022, 22, 2800 CrossRef CAS PubMed.
- R. S. Andre, L. A. Mercante, M. H. Facure, R. C. Sanfelice, L. Fugikawa-Santos, T. M. Swager and D. S. Correa, ACS Sens., 2022, 7, 2104–2131 CrossRef CAS PubMed.
- S. P. Burg and E. A. Burg, Science, 1965, 148, 1190–1196 CrossRef CAS PubMed.
- S. Y. Jeong, J. S. Kim and J. H. Lee, Adv. Mater., 2020, 32, 2002075 CrossRef CAS PubMed.
- S. Y. Park, Y. Kim, T. Kim, T. H. Eom, S. Y. Kim and H. W. Jang, InfoMat, 2019, 1, 289–316 CrossRef CAS.
- S. Lee, S. Kim, G. B. Nam, T. H. Eom and H. W. Jang, J. Semicond. Technol. Sci., 2022, 22, 244–258 CrossRef.
- N. Yamazoe, G. Sakai and K. Shimanoe, Catal. Surv. Asia, 2003, 7, 63–75 CrossRef CAS.
- S.-J. Choi and I.-D. Kim, Electron. Mater. Lett., 2018, 14, 221–260 CrossRef CAS.
- Y. K. Jo, S.-Y. Jeong, Y. K. Moon, Y.-M. Jo, J.-W. Yoon and J.-H. Lee, Nat. Commun., 2021, 12, 1–9 CrossRef PubMed.
- S. M. Lee, Y. K. Moon, K. Lim, S.-W. Park, S. J. Park, T.-H. Kim, S. Y. Kim, J.-H. Lee and Y.-M. Jo, Sens. Actuators, B, 2023, 382, 133494 CrossRef CAS.
- Y. G. Song, J. Y. Park, J. M. Suh, Y.-S. Shim, S. Y. Yi, H. W. Jang, S. Kim, J. M. Yuk, B.-K. Ju and C.-Y. Kang, Chem. Mater., 2018, 31, 207–215 CrossRef.
- S. J. Park, Y. K. Moon, S.-W. Park, S. M. Lee, T.-H. Kim, S. Y. Kim, J.-H. Lee and Y.-M. Jo, ACS Appl. Mater. Interfaces, 2023, 15, 7102–7111 CrossRef CAS PubMed.
- J.-M. Jeon, Y.-S. Shim, S. D. Han, Y. H. Kim, C.-Y. Kang, J.-S. Kim, M. Kim and H. W. Jang, J. Mater. Chem. A, 2015, 3, 17939–17945 RSC.
- Y. Wang, J. Liu, X. Cui, Y. Gao, J. Ma, Y. Sun, P. Sun, F. Liu, X. Liang and T. Zhang, Sens. Actuators, B, 2017, 238, 473–481 CrossRef CAS.
- J. Xu, X. Wang and J. Shen, Sens. Actuators, B, 2006, 115, 642–646 CrossRef CAS.
- L. Wang, Z. Lou, R. Wang, T. Fei and T. Zhang, Sens. Actuators, B, 2012, 171, 1180–1185 CrossRef.
- E. Llobet, Sens. Actuators, B, 2013, 179, 32–45 CrossRef CAS.
- Y. H. Kim, S. J. Kim, Y.-J. Kim, Y.-S. Shim, S. Y. Kim, B. H. Hong and H. W. Jang, ACS Nano, 2015, 9, 10453–10460 CrossRef CAS PubMed.
- D. R. Kauffman and A. Star, Angew. Chem., Int. Ed., 2008, 47, 6550–6570 CrossRef CAS.
- Y. H. Kim, J. S. Park, Y.-R. Choi, S. Y. Park, S. Y. Lee, W. Sohn, Y.-S. Shim, J.-H. Lee, C. R. Park and Y. S. Choi, J. Mater. Chem. A, 2017, 5, 19116–19125 RSC.
- E. Singh, M. Meyyappan and H. S. Nalwa, ACS Appl. Mater. Interfaces, 2017, 9, 34544–34586 CrossRef CAS PubMed.
- C. W. Lee, S. E. Jun, S. J. Kim, T. H. Lee, S. A. Lee, J. W. Yang, J. H. Cho, S. Choi, C. j. Kim and S. Y. Kim, InfoMat, 2023, e12427 CrossRef CAS.
- J. M. Suh, T. H. Lee, K. Hong, Y. G. Song, S. H. Cho, C.-Y. Kang, Y.-S. Shim, D. Lee, K. C. Kwon and H. W. Jang, Sens. Actuators, B, 2022, 369, 132319 CrossRef CAS.
- E. Lee, Y. S. Yoon and D.-J. Kim, ACS Sens., 2018, 3, 2045–2060 CrossRef CAS PubMed.
- Y. Hu, W. Zheng, S. Fan, J. Zhang and X. Liu, Appl. Phys. Rev., 2023, 10 CAS.
- R. Kumar, W. Zheng, X. Liu, J. Zhang and M. Kumar, Adv. Mater. Technol., 2020, 5, 1901062 CrossRef CAS.
- C. W. Lee, T. H. Eom, S. H. Cho and H. W. Jang, Adv. Sens. Res., 2023, 2200057 CrossRef.
- X. Liu, T. Ma, N. Pinna and J. Zhang, Adv. Funct. Mater., 2017, 27, 1702168 CrossRef.
- H. Furukawa, K. E. Cordova, M. O'Keeffe and O. M. Yaghi, Science, 2013, 341, 1230444 CrossRef PubMed.
- H.-C. Zhou, J. R. Long and O. M. Yaghi, Chem. Rev., 2012, 112, 673–674 CrossRef CAS PubMed.
- M. K. Lee, M. Shokouhimehr, S. Y. Kim and H. W. Jang, Adv. Energy Mater., 2022, 12, 2003990 CrossRef CAS.
- Y. M. Jo, Y. K. Jo, J. H. Lee, H. W. Jang, I. S. Hwang and D. J. Yoo, Adv. Mater., 2022, 2206842 Search PubMed.
- W.-T. Koo, J.-S. Jang and I.-D. Kim, Chem, 2019, 5, 1938–1963 CAS.
- Y. Sun, B. Wang, Y. Hou, K. Suematsu, Z. Zhao, W. Zhang, K. Shimanoe and J. Hu, J. Chem. Eng., 2023, 465, 142818 CrossRef CAS.
- M. G. Campbell, S. F. Liu, T. M. Swager and M. Dinca, J. Am. Chem. Soc., 2015, 137, 13780–13783 CrossRef CAS PubMed.
- Z. Meng, R. M. Stolz and K. A. Mirica, J. Am. Chem. Soc., 2019, 141, 11929–11937 CrossRef CAS.
- W. C. Ko, M.-S. Kim, Y. J. Kwon, J. Jeong, W. R. Kim, H. Choi, J. K. Park and Y. K. Jeong, J. Mater. Chem. A, 2020, 8, 19246–19253 RSC.
- E. Lee, A. VahidMohammadi, Y. S. Yoon, M. Beidaghi and D.-J. Kim, ACS Sens., 2019, 4, 1603–1611 CrossRef CAS PubMed.
- S. J. Kim, H.-J. Koh, C. E. Ren, O. Kwon, K. Maleski, S.-Y. Cho, B. Anasori, C.-K. Kim, Y.-K. Choi and J. Kim, ACS Nano, 2018, 12, 986–993 CrossRef CAS.
- W.-N. Zhao, N. Yun, Z.-H. Dai and Y.-F. Li, RSC Adv., 2020, 10, 1261–1270 RSC.
- S. Sun, M. Wang, X. Chang, Y. Jiang, D. Zhang, D. Wang, Y. Zhang and Y. Lei, Sens. Actuators, B, 2020, 304, 127274 CrossRef CAS.
- M. Liu, Z. Wang, P. Song, Z. Yang and Q. Wang, Sens. Actuators, B, 2021, 340, 129946 CrossRef CAS.
- Y.-J. Chen, Y.-Y. Wen, W.-H. Li, Z.-H. Fu, G.-E. Wang and G. Xu, Nano Lett., 2023, 23, 3614–3622 CrossRef CAS PubMed.
- L. Zhou, Y. Hu, S. Li, X. Liu and J. Zhang, Appl. Phys. Lett., 2023, 123 Search PubMed.
- D. H. Kim, Y.-S. Shim, J.-M. Jeon, H. Y. Jeong, S. S. Park, Y.-W. Kim, J.-S. Kim, J.-H. Lee and H. W. Jang, ACS Appl. Mater. Interfaces, 2014, 6, 14779–14784 CrossRef CAS PubMed.
- Y.-M. Jo, T.-H. Kim, C.-S. Lee, K. Lim, C. W. Na, F. Abdel-Hady, A. A. Wazzan and J.-H. Lee, ACS Appl. Mater. Interfaces, 2018, 10, 8860–8868 CrossRef CAS PubMed.
- K. J. Choi and H. W. Jang, Sensors, 2010, 10, 4083–4099 CrossRef CAS PubMed.
- J. M. Suh, K. C. Kwon, T. H. Lee, C. Kim, C. W. Lee, Y. G. Song, M.-J. Choi, S. Choi, S. H. Cho and S. Kim, Sens. Actuators, B, 2021, 333, 129566 CrossRef CAS.
- H. G. Moon, Y.-S. Shim, H. W. Jang, J.-S. Kim, K. J. Choi, C.-Y. Kang, J.-W. Choi, H.-H. Park and S.-J. Yoon, Sens. Actuators, B, 2010, 149, 116–121 CrossRef CAS.
- J.-H. Lee, Sens. Actuators, B, 2009, 140, 319–336 CrossRef CAS.
- L. Zhou, Z. Li, X. Chang, X. Liu, Y. Hu, M. Li, P. Xu, N. Pinna and J. Zhang, Small, 2023, 2301485 CrossRef CAS PubMed.
- G. Lei, H. Pan, H. Mei, X. Liu, G. Lu, C. Lou, Z. Li and J. Zhang, Chem. Soc. Rev., 2022, 7260–7280 RSC.
- Y.-S. Shim, H. G. Moon, L. Zhang, S.-J. Yoon, Y. S. Yoon, C.-Y. Kang and H. W. Jang, RSC Adv., 2013, 3, 10452–10459 RSC.
- J. M. Suh, Y. S. Shim, D. H. Kim, W. Sohn, Y. Jung, S. Y. Lee, S. Choi, Y. H. Kim, J. M. Jeon and K. Hong, Adv. Mater. Technol., 2017, 2, 1600259 CrossRef.
- X. Kou, N. Xie, F. Chen, T. Wang, L. Guo, C. Wang, Q. Wang, J. Ma, Y. Sun and H. Zhang, Sens. Actuators, B, 2018, 256, 861–869 CrossRef CAS.
- C.-S. Lee, Z. Dai, H.-Y. Li, Y.-M. Jo, B.-Y. Kim, H.-G. Byun, I. Hwang and J.-H. Lee, Sens. Actuators, B, 2018, 273, 1–8 CrossRef CAS.
- K.-I. Choi, H.-J. Kim, Y. C. Kang and J.-H. Lee, Sens. Actuators, B, 2014, 194, 371–376 CrossRef CAS.
- K. Lim, Y.-M. Jo, S. Kim, J.-W. Yoon, S.-Y. Jeong, J.-S. Kim, H. J. Choi, Y. Cho, J. Park and Y. W. Jeong, Sens. Actuators, B, 2021, 348, 130665 CrossRef CAS.
- R. Zhou, X. Lin, D. Xue, F. Zong, J. Zhang, X. Duan, Q. Li and T. Wang, Sens. Actuators, B, 2018, 260, 900–907 CrossRef CAS.
- S. H. Kim, Y. K. Moon, J.-H. Lee, Y. C. Kang and S.-Y. Jeong, J. Mater. Chem. A, 2023, 11, 1159–1169 RSC.
- Y. K. Moon, S. Y. Jeong, Y. M. Jo, Y. K. Jo, Y. C. Kang and J. H. Lee, Adv. Sci., 2021, 8, 2004078 CrossRef CAS PubMed.
- Y. Y. Broza, X. Zhou, M. Yuan, D. Qu, Y. Zheng, R. Vishinkin, M. Khatib, W. Wu and H. Haick, Chem. Rev., 2019, 119, 11761–11817 CrossRef CAS.
- L. Capelli, S. Sironi and R. D. Rosso, Sensors, 2014, 14, 19979–20007 CrossRef PubMed.
- S. Y. Jeong, Y. K. Moon, T. H. Kim, S. W. Park, K. B. Kim, Y. C. Kang and J. H. Lee, Adv. Sci., 2020, 7, 1903093 CrossRef CAS PubMed.
- Y. K. Moon, J. H. Kim, S.-Y. Jeong, S. M. Lee, S. J. Park, T. H. Kim, J.-H. Lee and Y. C. Kang, J. Mater. Chem. A, 2023, 11, 666–675 RSC.
- B. Esser, J. M. Schnorr and T. M. Swager, Angew. Chem., Int. Ed., 2012, 51, 5752–5756 CrossRef CAS PubMed.
- Q. Zhao, Z. Duan, Z. Yuan, X. Li, W. Si, B. Liu, Y. Zhang, Y. Jiang and H. Tai, Chin. Chem. Lett., 2020, 31, 2045–2049 CrossRef CAS.
- L.-P. Wang, Z. Jin, T. Luo, Y. Ding, J.-H. Liu, X.-F. Wang and M.-Q. Li, New J. Chem., 2019, 43, 3619–3624 RSC.
- T. Järvinen, G. S. Lorite, J. Peräntie, G. Toth, S. Saarakkala, V. K. Virtanen and K. Kordas, Nanotechnol, 2019, 30, 405501 CrossRef PubMed.
- K. Lee, R. Gatensby, N. McEvoy, T. Hallam and G. S. Duesberg, Adv. Mater., 2013, 25, 6699–6702 CrossRef CAS PubMed.
- T. Kim, T. H. Lee, S. Y. Park, T. H. Eom, I. Cho, Y. Kim, C. Kim, S. A. Lee, M.-J. Choi and J. M. Suh, ACS Nano, 2023, 17, 4404–4413 CrossRef CAS PubMed.
- Z. Ma, P. Chen, W. Cheng, K. Yan, L. Pan, Y. Shi and G. Yu, Nano Lett., 2018, 18, 4570–4575 CrossRef CAS PubMed.
- A. T. Güntner, M. Wied, N. J. Pineau and S. E. Pratsinis, Adv. Sci., 2020, 7, 1903390 CrossRef PubMed.
- D. Zhang, S. Yu, X. Wang, J. Huang, W. Pan, J. Zhang, B. E. Meteku and J. Zeng, J. Harzard. Mater., 2022, 423, 127160 CrossRef CAS.
- S. F. Liu, A. R. Petty, G. T. Sazama and T. M. Swager, Angew. Chem., Int. Ed., 2015, 54, 6554–6557 CrossRef CAS PubMed.
- Y. H. Cho, Y. C. Kang and J.-H. Lee, Sens. Actuators, B, 2013, 176, 971–977 CrossRef CAS.
- P. Srinivasan and J. B. B. Rayappan, Sens. Actuators, B, 2021, 349, 130759 CrossRef CAS.
- J.-S. Kim, K. B. Kim, H.-Y. Li, C. W. Na, K. Lim, Y. K. Moon, J. W. Yoon and J.-H. Lee, J. Mater. Chem., 2021, 9, 16359–16369 RSC.
- Y. H. Cho, Y. N. Ko, Y. C. Kang, I.-D. Kim and J.-H. Lee, Sens. Actuators, B, 2014, 195, 189–196 CrossRef CAS.
- W. Yan, H. Xu, M. Ling, S. Zhou, T. Qiu, Y. Deng, Z. Zhao and E. Zhang, ACS Sens., 2021, 6, 2613–2621 CrossRef CAS PubMed.
- T.-H. Kim, J.-W. Yoon, Y. C. Kang, F. Abdel-Hady, A. Wazzan and J.-H. Lee, Sens. Actuators, B, 2017, 240, 1049–1057 CrossRef CAS.
- Q. Liu, S. Mukherjee, R. Huang, K. Liu, T. Liu, K. Liu, R. Miao, H. Peng and Y. Fang, Chem.–Asian J., 2019, 14, 2751–2758 CrossRef CAS PubMed.
- Y. Li, W. Luo, N. Qin, J. Dong, J. Wei, W. Li, S. Feng, J. Chen, J. Xu and A. A. Elzatahry, Angew. Chem., Int. Ed., 2014, 53, 9035–9040 CrossRef CAS PubMed.
- T. Wang, J. Liu, Y. Zhang, Q. Liang, R. Wu, H.-S. Tsai, Y. Wang and J. Hao, J. Mater. Chem. A, 2022, 10, 4306–4315 RSC.
- Y.-X. Jin, J. Chen, Y.-J. Chen, W.-H. Deng, X.-L. Ye, G.-E. Wang and G. Xu, J. Mater. Chem. A, 2023, 11, 7179–7183 RSC.
- O. Ovsianytskyi, Y.-S. Nam, O. Tsymbalenko, P.-T. Lan, M.-W. Moon and K.-B. Lee, Sens. Actuators, B, 2018, 257, 278–285 CrossRef CAS.
- C. Z. Wang, J. Chen, Q. H. Li, G. E. Wang, X. L. Ye, J. Lv and G. Xu, Angew. Chem., Int. Ed., 2023, 62, e202302996 CrossRef CAS PubMed.
- T. Lee, J. O. Kim, C. Park, H. Kim, M. Kim, H. Park, I. Kim, J. Ko, K. Pak and S. Q. Choi, Adv. Mater., 2022, 34, 2107696 CrossRef CAS PubMed.
- X. Guo, Y. Ding, C. Liang, B. Du, C. Zhao, Y. Tan, Y. Shi, P. Zhang, X. Yang and Y. He, Sens. Actuators, B, 2022, 357, 131424 CrossRef CAS.
- H. Fang, S. Li, W. Jiang, H. Zhao, C. Han, J. Li, G. Wang, Y. Zhang, S. Wang and J. Deng, Sens. Actuators, B, 2022, 368, 132225 CrossRef CAS.
- Q. Zhou, L. Xu, Z. Kan, L. Yang, Z. Chang, B. Dong, X. Bai, G. Lu and H. Song, J. Harzard. Mater., 2022, 426, 128075 CrossRef CAS PubMed.
- Q. Zhou, L. Yang, Z. Kan, J. Lyu, M. X. Wang, B. Dong, X. Bai, Z. Chang, H. Song and L. Xu, J. Chem. Eng., 2022, 450, 138014 CrossRef CAS.
- K. Hagedorn, W. Li, Q. Liang, S. Dilger, M. Noebels, M. R. Wagner, J. S. Reparaz, A. Dollinger, J. Schmedt auf der Günne and T. Dekorsy, Adv. Funct. Mater., 2016, 26, 3424–3437 CrossRef CAS.
- C. Li, Y. Liu, W. Wan, Y. Li, Y. Ma, J. Zhang, X. Ren and H. Zhao, Sens. Actuators, B, 2020, 318, 128209 CrossRef CAS.
- W. Jin, S. Ma, Z. Tie, W. Li, J. Luo, L. Cheng, X. Xu, T. Wang, X. Jiang and Y. Mao, Appl. Surf. Sci., 2015, 353, 71–78 CrossRef CAS.
- W. Geng, Z. Ma, J. Yang, L. Duan, F. Li and Q. Zhang, Sens. Actuators, B, 2021, 334, 129639 CrossRef CAS.
- V. Shumeiko, E. Malach, Y. Helman, Y. Paltiel, G. Bisker, Z. Hayouka and O. Shoseyov, Sens. Actuators, B, 2021, 327, 128832 CrossRef CAS.
- N. Keller, M.-N. Ducamp, D. Robert and V. Keller, Chem. Rev., 2013, 113, 5029–5070 CrossRef CAS PubMed.
- M. E. Saltveit, Postharvest Biol. Technol., 1999, 15, 279–292 CrossRef CAS.
- K.-H. Kim, R. Pal, J.-W. Ahn and Y.-H. Kim, Waste Manage., 2009, 29, 1265–1273 CrossRef CAS PubMed.
- C.-C. Zhao and J.-B. Eun, J. Food Sci. Technol., 2018, 55, 5082–5090 CrossRef CAS PubMed.
- X. Liu, W. Zheng, R. Kumar, M. Kumar and J. Zhang, Coord. Chem. Rev., 2022, 462, 214517 CrossRef CAS.
- L. Gram and H. H. Huss, Int. J. Food Microbiol., 1996, 33, 121–137 CrossRef CAS PubMed.
- G. Moula, M. Bose and S. Sarkar, Inorg. Chem., 2013, 52, 5316–5327 CrossRef CAS PubMed.
- J. Sujiwo, D. Kim and A. Jang, Poult. Sci., 2018, 97, 2887–2894 CrossRef CAS PubMed.
- A. E.-D. A. Bekhit, B. W. Holman, S. G. Giteru and D. L. Hopkins, Trends Food Sci. Technol., 2021, 109, 280–302 CrossRef CAS.
- G. Esposito, S. Sciuto and P. L. Acutis, Food Control, 2018, 94, 162–166 CrossRef CAS.
- C. C. Balamatsia, A. Patsias, M. G. Kontominas and I. N. Savvaidis, Food Chem., 2007, 104, 1622–1628 CrossRef CAS.
- K. Mitsubayashi, Y. Kubotera, K. Yano, Y. Hashimoto, T. Kon, S. Nakakura, Y. Nishi and H. Endo, Sens. Actuators, B, 2004, 103, 463–467 CrossRef CAS.
- N. C. Jeong, J. S. Lee, E. L. Tae, Y. J. Lee and K. B. Yoon, Angew. Chem., Int. Ed., 2008, 47, 10128–10132 CrossRef CAS PubMed.
- D. Dave and A. E. Ghaly, Am. J. Agric. Biol. Sci., 2011, 6, 486–510 CrossRef CAS.
- P. Dalgaard, L. Gram and H. H. Huss, Int. J. Food Microbiol., 1993, 19, 283–294 CrossRef CAS PubMed.
- H. Li, W. Geng, X. Sun, W. Wei, X. Mu, W. Ahmad, M. M. Hassan, Q. Ouyang and Q. Chen, Meat Sci., 2021, 177, 108507 CrossRef CAS PubMed.
- D. B. Rodriguez, H. A. Frank and H. Y. Yamamoto, J. Sci. Food Agric., 1969, 20, 15–17 CrossRef CAS PubMed.
- G. Kim, B.-K. Cho, S. H. Oh and K.-B. Kim, J. Biosyst. Eng., 2020, 45, 261–271 CrossRef.
- R. Pierami and K. Stevenson, J. Dairy Sci., 1976, 59, 1010–1015 CrossRef CAS.
- E. J. Bartowsky, Lett. Appl. Microbiol., 2009, 48, 149–156 CrossRef CAS PubMed.
- E. J. Bartowsky and P. A. Henschke, Int. J. Food Microbiol., 2008, 125, 60–70 CrossRef CAS PubMed.
- S. Y. Jeong, Y. K. Moon, J. K. Kim, S. W. Park, Y. K. Jo, Y. C. Kang and J. H. Lee, Adv. Funct. Mater., 2021, 31, 2007895 CrossRef CAS.
- F. Qu, S. Zhang, C. Huang, X. Guo, Y. Zhu, T. Thomas, H. Guo, J. P. Attfield and M. Yang, Angew. Chem., Int. Ed., 2021, 60, 6561–6566 CrossRef CAS PubMed.
- G. S. Kim, Y. Lim, J. Shin, J. Yim, S. Hur, H. C. Song, S. H. Baek, S. K. Kim, J. Kim and C. Y. Kang, Adv. Sci., 2023, 2301002 CrossRef CAS PubMed.
- N. Ma, K. Suematsu, M. Yuasa, T. Kida and K. Shimanoe, ACS Appl. Mater. Interfaces, 2015, 7, 5863–5869 CrossRef CAS PubMed.
- K.-I. Choi, S.-J. Hwang, Z. Dai, Y. C. Kang and J.-H. Lee, RSC Adv., 2014, 4, 53130–53136 RSC.
- K. Suematsu, M. Sasaki, N. Ma, M. Yuasa and K. Shimanoe, ACS Sens., 2016, 1, 913–920 CrossRef CAS.
- H. R. Kim, A. Haensch, I. D. Kim, N. Barsan, U. Weimar and J. H. Lee, Adv. Funct. Mater., 2011, 21, 4456–4463 CrossRef CAS.
- Y. Sun, Z. Zhao, K. Suematsu, P. Li, Z. Yu, W. Zhang, J. Hu and K. Shimanoe, ACS Sens., 2020, 5, 1040–1049 CrossRef CAS PubMed.
- J.-S. Kim, C. W. Na, C.-H. Kwak, H.-Y. Li, J. W. Yoon, J.-H. Kim, S.-Y. Jeong and J.-H. Lee, ACS Appl. Mater. Interfaces, 2019, 11, 25322–25329 CrossRef CAS PubMed.
- J. W. Yoon, J. S. Kim, T. H. Kim, Y. J. Hong, Y. C. Kang and J. H. Lee, Small, 2016, 12, 4229–4240 CrossRef CAS PubMed.
- Q. Zhou, A. Sussman, J. Chang, J. Dong, A. Zettl and W. Mickelson, Sens. Actuators, A, 2015, 223, 67–75 CrossRef CAS.
- L. Xiao, S. Xu, G. Yu and S. Liu, Sens. Actuators, B, 2018, 255, 2002–2010 CrossRef CAS.
- N. Luo, C. Wang, D. Zhang, M. Guo, X. Wang, Z. Cheng and J. Xu, Sens. Actuators, B, 2022, 354, 130982 CrossRef CAS.
- S. Santra, A. De Luca, S. Bhaumik, S. Ali, F. Udrea, J. Gardner, S. Ray and P. Guha, RSC Adv., 2015, 5, 47609–47616 RSC.
- T. H. Eom, S. H. Cho, J. M. Suh, T. Kim, T. H. Lee, S. E. Jun, J. W. Yang, J. Lee, S.-H. Hong and H. W. Jang, J. Mater. Chem. A, 2021, 9, 11168–11178 RSC.
- T. H. Eom, S. H. Cho, J. M. Suh, T. Kim, J. W. Yang, T. H. Lee, S. E. Jun, S. J. Kim, J. Lee and S. H. Hong, Small, 2022, 18, 2106613 CrossRef CAS PubMed.
- D. Cho, J. M. Suh, S. H. Nam, S. Y. Park, M. Park, T. H. Lee, K. S. Choi, J. Lee, C. Ahn and H. W. Jang, Adv. Sci., 2021, 8, 2001883 CrossRef CAS PubMed.
- Y.-M. Jo, K. Lim, J. W. Yoon, Y. K. Jo, Y. K. Moon, H. W. Jang and J.-H. Lee, ACS Cent. Sci., 2021, 7, 1176–1182 CrossRef CAS PubMed.
- K. Lim, Y. M. Jo, J. W. Yoon, J. S. Kim, D. J. Lee, Y. K. Moon, J. W. Yoon, J. H. Kim, H. J. Choi and J. H. Lee, Small, 2021, 17, 2100438 CrossRef CAS PubMed.
- J. M. Suh, T. H. Eom, S. H. Cho, T. Kim and H. W. Jang, Mater. Adv., 2021, 2, 827–844 RSC.
- O. Casals, N. Markiewicz, C. Fabrega, I. Gràcia, C. Cané, H. S. Wasisto, A. Waag and J. D. Prades, ACS Sens., 2019, 4, 822–826 CrossRef CAS PubMed.
- J. E. Ryu, S. Park, Y. Park, S. W. Ryu, K. Hwang and H. W. Jang, Adv. Mater., 2022, 2204947 Search PubMed.
- H. G. Moon, Y. Jung, S. D. Han, Y.-S. Shim, B. Shin, T. Lee, J.-S. Kim, S. Lee, S. C. Jun and H.-H. Park, ACS Appl. Mater. Interfaces, 2016, 8, 20969–20976 CrossRef CAS PubMed.
- S.-Y. Jeong, Y. K. Moon, J. Wang and J.-H. Lee, Nat. Commun., 2023, 14, 233 CrossRef CAS PubMed.
- V. Schroeder, E. D. Evans, Y.-C. M. Wu, C.-C. A. Voll, B. R. McDonald, S. Savagatrup and T. M. Swager, ACS Sens., 2019, 4, 2101–2108 CrossRef CAS PubMed.
- J. Oh, S. H. Kim, M.-J. Lee, H. Hwang, W. Ku, J. Lim, I.-S. Hwang, J.-H. Lee and J.-H. Hwang, Sens. Actuators, B, 2022, 364, 131894 CrossRef CAS.
Footnote |
† These authors contributed equally to this work. |
|
This journal is © The Royal Society of Chemistry 2024 |
Click here to see how this site uses Cookies. View our privacy policy here.