DOI:
10.1039/D3FO04068B
(Paper)
Food Funct., 2024,
15, 338-354
Insoluble/soluble fraction ratio determines effects of dietary fiber on gut microbiota and serum metabolites in healthy mice†
Received
22nd September 2023
, Accepted 26th November 2023
First published on 28th November 2023
Abstract
Both soluble dietary fiber (SDF) and insoluble dietary fiber (IDF) play pivotal roles in maintaining gut microbiota homeostasis; whether the effects of the different ratios of IDF and SDF are consistent remains unclear. Consequently, we selected SDFs and IDFs from six representative foods (apple, celery, kale, black fungus, oats, and soybeans) and formulated nine dietary fiber recipes composed of IDF and SDF with a ratio from 1
:
9 to 9
:
1 (NDFR) to compare their impact on microbial effects with healthy mice. We discovered that NDFR treatment decreased the abundance of Proteobacteria and the ratio of Firmicutes/Bacteroidetes at the phylum level. The α diversity and relative richness of Parabacteroides and Prevotella at the genus level showed an upward trend along with the ratio of IDF increasing, while the relative abundance of Akkermansia at the genus level and the production of acetic acid and propionic acid exhibited an increased trend along with the ratio of SDF increasing. The relative abundance of Parabacteroides and Prevotella in the I9S1DF group (the ratio of IDF and SDF was 9
:
1) was 1.72 times and 5.92 times higher than that in the I1S9DF group (the ratio of IDF and SDF was 1
:
9), respectively. The relative abundance of Akkermansia in the I1S9DF group was 17.18 times higher than that in the I9S1DF group. Moreover, a high ratio of SDF (SDF reaches 60% or more) enriched the glycerophospholipid metabolism pathway; however, a high ratio of IDF (IDF reaches 80% or more) regulated the tricarboxylic acid cycle. These findings are helpful in the development of dietary fiber supplements based on gut microbiota and metabolites.
1. Introduction
Increasing evidence has indicated that the gut microbiota together with its metabolites are of vital importance in the genesis and progression of many metabolic-related diseases, such as metabolic liver disease, obesity, cardio-metabolic disease (CMD), and diabetes mellitus type 2 (T2DM).1 The typical microbial characteristics of obese individuals exhibit an increase in the rate of Firmicutes to Bacteroidetes and a decrease in the abundance of Akkermansia muciniphila (a typical mucin-degrader).2 Individuals with T2DM also display a decrease in the abundance of A. muciniphila, and an increase in the abundance of Bacteroides vulgatus, Clostridium clostridioforme, Clostridium hathewayi, and Prevotella copri.3,4 Individuals with metabolic liver disease have an enriched abundance of species assigned to Klebsiella pneumoniae, Streptococcus anginosus and Veillonella atypica, whereas Coprococcus comes and anti-inflammatory F. prausnitzii are less abundant.2,5,6 In addition, individuals with CMD had a reduced abundance of Enterobacter aerogenes and Escherichia coli with an increased abundance of F. prausnitzii, Klebsiella spp., R. gnavus, and Streptococcus spp.2
Dietary fiber (DF) is considered one of the main driving factors that regulate the structure, diversity, and richness of the intestinal microbiota.7–9 Most countries have proposed at least 25–35 g of DF daily intake for adults to maintain their overall health.10 Currently, most people choose processed foods that are often low in fiber content in their food plates (the global average daily intake of DF is about 10 g), and the lack of fiber intake has become an important threat to human health in this modern era.11 It is necessary to improve the status of insufficient DF intake globally. The development of dietary fiber supplement products is an important way to change this situation. DF includes resistant oligosaccharides (monomer units 3–9), non-starch polysaccharides (NSP) (monomer units ≥ 10), resistant starch (monomer units ≥ 10), lignin and other compounds related to polysaccharides or oligosaccharides in plant cell walls.12 Fruits, vegetables, grains, and legumes are the main sources of DF, i.e., mainly pectin, cellulose, β-glucans, xylans, and lignin.12 DF can be subtyped based on solubility, viscosity, and fermentation characteristics, which correlate with DF functional characteristics.13 Solubility is a particularly relevant characteristic for the efficient and rapid fermentation of DF by microorganisms. Soluble dietary fibers (SDF), such as β-glucan and pectin, are easily accessed by microbes in the intestinal lumen and are fermented to a great extent. However, insoluble dietary fiber (IDF) often exhibits low accessibility, but it can act as a nutritional niche and be colonized by microbes with relevant degrading functions.14,15
As mentioned above, human health is tied to gut microbiota, and both SDF and IDF have shown beneficial effects on gut bacterial ecology, diversity, and function. Therefore, understanding the impact of different ratios of IDF to SDF on the microbial effects (composition of gut microbiota and metabolites) of DF can provide strong evidence for the development of DF supplements. In this research, we designed nine ratios of IDF to SDF (from 1
:
9 to 9
:
1) and investigated whether the gut microbiota displayed a different response to the changes in such ratios in healthy mice fed DF extracted from the edible parts of six raw materials using 16S rDNA sequencing approaches combined with metabolomics to determine the optimal ratio of IDF and SDF regarding the effect of DF on gut microbiota.
2. Materials and methods
2.1 Materials and reagents
Apple (Malus pumila Mill.), celery (Apium graveolens Linn.), kale (Brassica oleraceae var. acephala DC.), oats (Avena sativa L.), black fungus (Auricularia heimuer (L.ex Hook.) Underw), and soybeans (Glycine max (L.) Merr.) cultivated in Luochuan City (Shaanxi Province), Weifang City (Shandong Province), Qingdao City (Shandong Province), Dongning City (Heilongjiang Province), Nei Monggol Autonomous Region, and Heihe City (Heilongjiang Province), respectively, were obtained. Papain (≥2000 IU mg−1), α-amylase (≥2100 IU g−1), and amyloglucosidase (100
000 IU mL−1) were purchased from Aladdin (Beijing, China). The TIANamp Stool DNA Kit and TIANgel Purification Kit were produced by TIANGEN Biotech Co., Ltd (Beijing, China). The primers (515F and 806R) of 16S rDNA PCR were supplied by Sangon Biotech (Shanghai, China). 2 × Phanta Max Master Mix (Dye Plus), Equalbit dsDNA HS Assay Kit and VAHTS Universal DNA Library Prep Kit for Illumina were purchased from Vazyme Biotech Co., Ltd (Nanjing, China). MiSeq Reagent Kit v2 (2 × 250 bp) was bought from Illumina (Illumina, San Diego, California, USA). Acetonitrile, ammonium acetate and formic acid (HPLC-grade) were supplied by Merck (Darmstadt, Germany).
2.2 Dietary fiber extraction
Dietary fiber was obtained using a gravimetric method based on enzymatic hydrolysis, which was modified based on the technique previously established by our lab.16,17 In brief, edible parts of six raw materials pre-treated with drying, crushing, sieving, and soaking in 85% ethanol, and residual ethanol removal were soaked in hot water for 2 h according to a specific solid–liquid ratio and temperature (the solid–liquid ratios of apple, celery, kale, black fungus, oats, and soybeans were 1
:
20, 1
:
30, 1
:
10, 1
:
60, 1
:
10, and 1
:
10, respectively; the extraction temperatures of apple, celery, kale, black fungus, oats, and soybeans were 95 °C, 95 °C, 95 °C, 95 °C, 52 °C and 95 °C, respectively). The liquid and residue fractions were separated using a 200-mesh double-layer sieve. The former is enzymatically hydrolyzed with 0.8% (w/v) heat-stable α-amylase (95 °C for 1 h), 0.8% (w/v) protease (60 °C for 1 h), and 0.8% (w/v) glucoamylase (pH 4.5) (60 °C for 1 h) in a sequential manner. After enzyme inactivation and centrifugation (5000 rpm 4 °C for 10 min), crude SDF was precipitated from the supernatant using 70% alcohol for 12 h at room temperature. The precipitate was dissolved with distilled water, and small molecules were removed through 8000–14
000 Da dialysis membranes for 48 h. Kale and soybeans require further treatment with JK008 cation exchange resin to remove impurities for 6 h. SDF was obtained after rotary evaporation concentration and vacuum freeze-drying. To obtain IDF, the residue fractions were soaked again in distilled water based on the extraction solid–liquid ratio, enzymatically hydrolyzed as the liquid fraction, centrifuged, and finally treated with four cycles of water and 95% ethanol (70 °C) washes. SDF from apple, celery, kale, black fungus, oats, and soybeans was named separately as ASDF, CSDF, KSDF, BSDF, OSDF, and SSDF, respectively. In the same way, IDF from apple, celery, kale, black fungus, oats, and soybeans were named correspondingly as AIDF, CIDF, KIDF, BIDF, OIDF, and SIDF, respectively.
2.3 Chemical composition and monosaccharide composition of DF
The quantitative determination of neutral sugar was analyzed using the phenol-sulfuric acid method.18 The assay of sulfuric acid-carbazole was used to determine the contents of the uronic acid.19 The detection of protein, ash, and IDF was performed based on AOAC Official Method 2001.11, AOAC Official Method 940.26, and AOAC Official Method 991.43, respectively. The monosaccharide composition was determined according to Tang et al. (2020).20 In fact, 5 mg of SDF was mixed with 0.5 mL of 12 mol L−1 H2SO4 in an ice cold thick-walled tube and stirred for 0.5 h. After the addition of 2.5 mL of ultrapure water, SDF was hydrolyzed using a 100 °C-oil bath for 2 h. 5 mg of IDF was reswelled with 0.17 mL of distilled water at 80 °C for 1 h before the same two steps of H2SO4 treatment, except that 0.33 mL and 1 h were used. After being diluted and filtered, the samples were analyzed using Dionex ICS-5000 ion chromatography (Dionex, USA) with a Dionex CarboPac™ PA20 column (3 mm × 150 mm) and guard column (3 mm × 30 mm). Rhamnose (Rha), fucose (Fuc), galactose (Gal), arabinose (Ara), xylose (Xyl), glucose (Glc), fructose (Fru), mannose (Man), galacturonic acid (GalA) and glucuronic acid (GlcA) were used as standards.
2.4 Molecular weight of SDF
The molecular weight (Mw) of SDFs was measured by high-performance gel permeation chromatography (HPGPC).20 The equipment includes a Waters e2695 system collocated with a Waters Ultrahy-drogel™ Linear column (7.8 mm × 300 mm) (Milford, USA), a Waters Ultrahydrogel™ Guard column (6 mm × 40 mm) and Agilent 2414 RI refractive index detector. 0.02% NaN3 was used as the mobile phase at a flow rate of 0.6 mL min−1. Dextran (T-10, T-40, T-70 and T-500), and glucose were used as standards.
2.5 Fourier transform infrared spectrum of DF
All dried SDFs and IDFs were mixed with KBr powder to make a transparent sheet with a tablet press.21 Then, a Nicolet 5700 Fourier transform infrared spectrophotometer (FT-IR) (Thermo Electron, USA) was applied to harvest the signal (wavenumbers from 4000 to 400 cm−1). The number of scans was set to 32.
2.6
13C NMR analysis of DF
The 13C NMR spectra of SDFs and IDFs were determined using a Bruker Avance 400 M spectrometer (Bruker, Germany). Samples were ground, smashed, piped, and sampled with a CP/MAS mode. The contact time, acquisition time, and cycling delay were set at 2.0 ms, 0.0127 s and 4 s, respectively. The number of scans was 1500.16
2.7 Scanning electron microscope
All dried SDFs and IDFs were prepared into a solution with a mass fraction of 0.5% and were quickly frozen with liquid nitrogen to form a uniform state before freeze-drying. Freeze-dried samples were spread on a conductive adhesive tape and then treated with platinum sputter spraying. A JSM6701F scanning electron microscope (JEOL Ltd, Japan) was used to obtain scanning electron microscope (SEM) images with 200, 1000 and 2000 magnification under a high vacuum condition.21
2.8 Nine dietary fiber recipes preparation
Nine dietary fiber recipes composed of IDF and SDF with a ratio from 1
:
9 to 9
:
1 (NDFR) were formulated. The Dietary Guidelines for Chinese Residents recommend a maximum daily intake of 500 g, 350 g, and 150 g for vegetables, fruits, whole cereals and legumes, respectively. The ratio of the intake of vegetables, fruits, whole cereals and legumes is 5
:
3.5
:
1.5. According to the above result, both IDF and SDF were prepared from the vegetables (with celery, kale, and black fungus of one third), fruits (apple), cereals and legumes (the ratio of oats and soybeans was half and half) fiber in a ratio of 5
:
3.5
:
1.5. Nine custom feeds (CFs) for mice were obtained by replacing cellulose with NDFR in the AIN93G diet (FBSH Biotechnology Co., Ltd, Shanghai, China) (Table S1†). The nine CFs were named I1S9DF_CF (IDF
:
SDF, 1
:
9), I2S8DF_CF (IDF
:
SDF, 2
:
8), I3S7DF_CF (IDF
:
SDF, 3
:
7), I4S6DF_CF (IDF
:
SDF, 4
:
6), I5S5DF_CF (IDF
:
SDF, 5
:
5), I6S4DF_CF (IDF
:
SDF,6
:
4), I7S3DF_CF (IDF
:
SDF, 7
:
3), I8S2DF_CF (IDF
:
SDF, 8
:
2), and I9S1DF_CF (IDF
:
SDF, 9
:
1) (Table S1†).
2.9 Ethics statement and animal experiment design
Male C57BL/6J mice (16–22 g, 5-week-old) were provided by the SLAC Jingda Laboratory Animal Company (Hunan, China). All animals were reared under fit conditions of temperature of 23 ± 2 °C, relative moisture of 50 ± 10% and 12 h/12 h light/dark cycle. All animal use procedures were approved by the Animal Care and Use Committee of Nanchang University [SYXK (Gan) 2015-0002] in accordance with the National Research Council's Guide for the Care and Use of Laboratory Animals.
The detailed experimental design is shown in Fig. S1.† After seven days of acclimatization, mice were divided into 10 groups randomly (twelve mice per group): control group (AIN93G) and nine CF groups (I1S9DF, I2S8DF, I3S7DF, I4S6DF, I5S5DF, I6S4DF, I7S3DF, I8S2DF and I9S1DF groups). During the 14 days of diet intervention, the mice were housed individually with free access to feed and sterile distilled water. Body weight and feed intake were monitored daily. At the end of the experiment, feces were sterilely collected and stored at −80 °C. Serum, liver, spleen, and cecal contents were obtained and stored at −80 °C for the experiment after dissection of the mice.
2.10 Fecal microbiota 16S rDNA sequencing
A TIANamp Stool DNA Kit was used to isolate microbial genomic DNA from feces according to the specification. The V4 region of microbial 16S rDNA was amplified with the polymerase 2 × Phanta Max Master Mix (Dye Plus). The concentration of purified V4 amplicons was quantified using Qubit 3.0 (Thermo Scientific, Wilmington, USA) with an Equalbit dsDNA HS Assay Kit. Subsequently, DNA libraries were constructed with the VAHTS Universal DNA Library Prep Kit and quantified via Qubit 3.0 before mixing. The mixed DNA library was sequenced on MiSeq (Illumina, San Diego, California, USA) based on the protocols supplied by the MiSeq Reagent Kits v2.
2.11 Serum metabolome profiling
100 μL of serum sample was mixed with 400 μL of pre-cooled acetonitrile–water (4
:
1, v/v) and then placed on ice for 20 min to precipitate protein. Afterwards, the serum samples were centrifuged at 4 °C and 14
000 rpm for 15 min, and the supernatant was filtered using a 0.22 μm organic filter. 10 μL of each sample was mixed as a quality control (QC).
Metabolome analysis of serum samples was carried out using a UPLC-QTOF/MS. A Shim-pack GIST C18 column (2.1 mm I.D. × 75 mm L., 2 μm) on a Shimadzu Nexera X2 UHPLC system (Kyoto, Japan) was used to perform liquid chromatographic separation at 40 °C at a flow of 0.45 mL min−1 with an injection volume of 5.0 μL. The mobile phase was composed of solvent A (0.1% formic acid-water) and solvent B (0.1% formic acid-acetonitrile) under the positive mode, while the negative mode consisted of solvent A (10 mmol L−1 ammonium acetate solution) and solvent B (0.1% acetonitrile). The elution gradient of solvent B was set as 5–30% (0–8 min), 30–65% (8–16 min), 65% (16–20 min), 65–90% (20–24 min), 90% (24–26 min) and 90–5% (26–30 min). The mass system (AB SCIEX Triple TOF 5600) worked in both positive and negative modes, and the mass parameter settings were referred to in a previous study.22
2.12 Short chain fatty acid analysis of cecal contents
A certain mass of the cecal contents was acidified and further extracted with anhydrous ethers (400 μL). Quantification analysis of short chain fatty acids (SCFAs) was performed by gas chromatography according to a previously described method.23
2.13 Data analysis
Statistical analysis was performed using GraphPad Prism 9.0 (GraphPad, American). Data were expressed as the mean ± SD. One-way ANOVA compares multiple groups to identify the significance of differences, where p < 0.05 represents a significant difference.
Microbiome data analysis was as follows: the raw FASTQ data were screened according to the conditions that the average sequencing quality of each base must be ≥Q20 and the ineligible sequences were spliced. QIIME software (v1.8.0, https://qiime.org/) was used to check and eliminate chimera sequences by calling USEARCH (v5.2.236, https://www.drive5.com/usearch/). The sequence similarity >97% is used as the operational taxonomic unit (OTU) division threshold using the UCLUST blast tool.24 OTU taxonomy table was obtained after comparing it with the Greengene database (Release 13.8, https://greengenes.secondgenome.com/). According to the OTU abundance matrix, we further analyzed α diversity, β diversity, and the species community structure at each classification level (Phylum, Class, Order, Family, Genus) with QIIME. The taxonomic cladogram was generated using LEfSe via the online analysis platform Galaxy (https://huttenhower.sph.harvard.edu/galaxy/) with a logarithmic LDA score threshold of 3.
Metabolome data processing was carried out using Progenesis QI (Waters, America).22 Identification of metabolites was based on the METLIN MS and MS/MS databases with an accuracy error of 10 ppm. Statistical analysis, principal component analysis (PCA) and pathway analysis were performed using online website MetaboAnalyst 5.0 (https://www.metaboanalyst.ca/home.xhtml). Pareto scaling was used for data normalization. Potential metabolite markers changing among NDFR-treatment groups were selected according to the variable importance in the projection (VIP > 1) from partial least-squares-discrimination analysis (PLS-DA) and Student's t-test (p < 0.05, FDR < 0.05). ChemRICH (https://chemrich.fiehnlab.ucdavis.edu/) analysis was devoted to analyzing chemical similarity enrichment for differential metabolites. The correlation analysis was performed using genescloud tools, a free online platform for data analysis (https://www.genescloud.cn).
3. Results
3.1 Chemical composition and monosaccharide composition of six SDFs and six IDFs
Six SDFs (ASDF, CSDF, KSDF, BSDF, OSDF, and SSDF) and six IDFs (AIDF, CIDF, KIDF, BIDF, OIDF, and SIDF) were obtained. We investigated the chemical and monosaccharide compositions of six SDFs (Table 1). Six SDFs were low in protein (0.43%–9.18%) and ash (1.96%–8.76%). The neutral sugar contents of ASDF, CSDF, KSDF, BSDF, OSDF, and SSDF are 44.54%, 48.80%, 70.30%, 68.08%, 86.03%, and 67.09%, respectively. The uronic acid contents of ASDF, CSDF, KSDF, BSDF, OSDF, and SSDF are 59.44%, 40.00%, 9.58%, 25.03%, 1.66%, and 23.15%, respectively. These data showed that the main component of the six SDFs was carbohydrates.
Table 1 Chemical composition and monosaccharide composition of six SDFs
|
ASDF (%) |
CSDF (%) |
KSDF (%) |
BSDF (%) |
OSDF (%) |
SSDF (%) |
Data are presented as means ± SD (n = 3). n.d., not detected or lower than the limit of quantification. |
Neutral sugar |
44.54 ± 1.42 |
48.80 ± 3.26 |
70.30 ± 3.00 |
68.08 ± 0.33 |
86.03 ± 2.08 |
67.09 ± 1.57 |
Uronic acid |
59.44 ± 0.49 |
40.00 ± 0.06 |
9.58 ± 0.56 |
25.03 ± 0.23 |
1.66 ± 0.85 |
23.15 ± 0.98 |
Protein |
0.43 ± 0.07 |
3.92 ± 0.17 |
9.18 ± 0.56 |
3.76 ± 0.06 |
1.31 ± 0.11 |
8.04 ± 0.43 |
Ash |
2.23 ± 0.20 |
6.76 ± 0.25 |
8.76 ± 0.30 |
3.73 ± 0.15 |
2.16 ± 0.30 |
1.96 ± 0.15 |
Fucose |
n.d. |
n.d. |
n.d. |
n.d. |
n.d. |
0.78 ± 0.06 |
Rhamnose |
5.56 ± 0.21 |
12.11 ± 0.11 |
3.67 ± 0.49 |
n.d. |
n.d. |
2.50 ± 0.51 |
Arabinose |
13.87 ± 0.27 |
19.57 ± 0.04 |
18.50 ± 0.96 |
n.d. |
1.01 ± 0.10 |
6.33 ± 0.61 |
Galactose |
8.29 ± 0.05 |
24.43 ± 0.10 |
26.80 ± 2.55 |
10.02 ± 0.81 |
n.d. |
20.98 ± 1.15 |
Glucose |
10.32 ± 0.44 |
5.71 ± 0.08 |
9.04 ± 5.02 |
38.20 ± 1.78 |
96.61 ± 0.36 |
28.62 ± 1.45 |
Xylose |
4.45 ± 0.15 |
1.78 ± 0.05 |
1.08 ± 1.15 |
5.91 ± 0.39 |
2.38 ± 0.29 |
25.01 ± 1.68 |
Mannose |
n.d. |
n.d. |
36.40 ± 6.4 |
18.63 ± 1.10 |
n.d. |
n.d. |
Galacturonic acid |
57.50 ± 0.39 |
36.40 ± 0.40 |
4.52 ± 0.11 |
n.d. |
n.d. |
15.79 ± 1.60 |
Glucuronic acid |
n.d. |
n.d. |
n.d. |
27.24 ± 0.66 |
n.d. |
n.d. |
ASDF mainly consisted of GalA (57.50%), and the others were Ara (13.87%), Glc (10.32%), Gal (8.29%), Rha (5.56%), and Xyl (4.45%). CSDF was also high in GalA (36.40%), followed by Gal (24.43%), Ara (19.57%), Rha (12.11%), Glc (5.71%), and Xyl (1.78%). These data indicated that ASDF and CSDF were pectin-type DF.16,25 Meanwhile, OSDF was almost made up of Glc (96.61%) with a few Ara (1.01%) and Xyl (2.38%), suggesting a kind of glucan.26 Compared with the three SDFs, the rest had a more balanced monosaccharide composition, where no sugar had a proportion of more than 40%. KSDF was characterized by a high proportion of Man (36.4%) and also contained 26.80% Gal, 18.50% Ara, 9.04% Glc, 4.52% GalA, 3.67% Rha, and 1.08% Xyl. SSDF was composed of seven monosaccharides (28.62% Glc, 25.01% Xyl, 20.98% Gal, 15.79% GalA, 6.33% Ara, 2.50% Rha, and 0.78% Fuc), and BSDF consisted of 5 (38.20% Glc, 27.24% GlcA, 18.63% Man, 10.02% Gal, 5.91% Xyl). The molecular weight of the six SDFs was further analyzed, as shown in Fig. S2.† Six SDFs belong to heteropolysaccharides with more than one main peak.
The chemical and the monosaccharide compositions of the six IDFs are shown in Table 2. The purity of the six IDFs was more than 87%, accompanied by a small amount of protein (3.61%–5.36%) and ash (2.16%–7.33%). The shared main monosaccharide in the six IDFs was glucose, which had a proportion exceeding 30%. Man (29.89%), Xyl (38.28%), and Xyl (13.26%) were the major monosaccharides in BIDF, OIDF, and SIDF, respectively. The proportion of Rha in the OIDF was 25.39%. In addition, GalA had a larger proportion in AIDF (17.51%), CIDF (20.87%) and KIDF (20.99%). IDF mainly contains cellulose, lignin and insoluble hemicellulose.27 Cellulose is a polysaccharide composed of glucose. The main glycosyl groups constituting hemicellulose are D-xylosyl, D-mannosyl, D-glucosyl, D-galactosyl, L-arabinosyl, 4-O-methyl-D-glucuronic acid-based, D-galacturonic acid and D-glucuronic acid groups.28 Our results are consistent with reported data in the literature.
Table 2 Chemical composition and monosaccharide composition of six IDFs
|
AIDF (%) |
CIDF (%) |
KIDF (%) |
BIDF (%) |
OIDF (%) |
SIDF (%) |
Data are presented as means ± SD (n = 3). n.d., not detected or lower than the limit of quantification. |
IDF content |
92.84 ± 0.30 |
89.63 ± 0.26 |
87.30 ± 0.35 |
91.55 ± 0.42 |
93.72 ± 0.28 |
89.66 ± 0.15 |
Protein |
4.99 ± 0.02 |
5.00 ± 0.03 |
5.36 ± 0.02 |
3.68 ± 0.10 |
3.61 ± 0.05 |
5.60 ± 0.04 |
Ash |
2.16 ± 0.30 |
5.36 ± 0.25 |
7.33 ± 0.35 |
4.76 ± 0.40 |
2.66 ± 0.25 |
4.73 ± 0.15 |
Fucose |
2.5 ± 0.09 |
0.82 ± 0.04 |
1.28 ± 0.1 |
n.d. |
n.d. |
1.02 ± 0.1 |
Rhamnose |
8.65 ± 0.02 |
5.81 ± 0.29 |
4.75 ± 1.92 |
n.d. |
25.39 ± 1.11 |
5.68 ± 0.006 |
Arabinose |
3.36 ± 0.57 |
4.06 ± 0.91 |
6.13 ± 0.44 |
n.d. |
n.d. |
n.d. |
Galactose |
8.73 ± 0.15 |
7.80 ± 0.06 |
7.62 ± 0.2 |
n.d. |
2.22 ± 0.11 |
8.02 ± 0.18 |
Glucose |
41.46 ± 0.55 |
43.68 ± 1.85 |
41.45 ± 0.35 |
68.64 ± 3.13 |
30.26 ± 3.16 |
59.05 ± 0.25 |
Xylose |
13.93 ± 0.38 |
10.16 ± 0.54 |
11.31 ± 0.74 |
7.87 ± 1.78 |
38.28 ± 2.57 |
13.26 ± 0.22 |
Mannose |
3.45 ± 0.01 |
6.52 ± 0.32 |
5.3 ± 0.68 |
29.89 ± 2.21 |
1.94 ± 0.071 |
5.17 ± 0.17 |
Galacturonic acid |
17.51 ± 0.42 |
20.87 ± 0.41 |
20.99 ± 2.5 |
n.d. |
0.77 ± 0.72 |
7.43 ± 0.33 |
Glucuronic acid |
0.42 ± 0.3 |
0.28 ± 0.17 |
1.16 ± 0.09 |
6.29 ± 1.95 |
1.14 ± 0.55 |
0.37 ± 0.11 |
3.2 Structure characterization of six SDFs and six IDFs
The characteristic functional groups of six SDFs and six IDFs were measured by FT-IR spectroscopy (Fig. 1A and B). In the FT-IR spectra of six SDFs and six IDFs, absorption peaks close to 3400 cm−1, 2900 cm−1, 1630 cm−1, and 1000–1100 cm−1 were the shared bands. The absorption close to 3400 cm−1 corresponds to O–H stretching vibrations together with the intra- and inter-molecular hydrogen bonds of hydroxyl groups.16,29 Bands of almost 2900 cm−1 were related to the C–H tension and bending vibration of the methyl group.16,29 Peaks around 1630 cm−1 and 1000–1100 cm−1 were arranged for –OH flexural vibrations and the skeletal modes of pyranose rings, respectively.20,29 ASDF, BSDF, OSDF, SSDF, and six IDFs also shared the band close to 1745 cm−1. Peaks at 1745 cm−1 were linked to the atomic vibration of C
O, which may be related to uronic acid residue.20,29 In addition, both BSDF and OSDF exhibited absorption bands close to 1370 cm−1, and the former had characteristic absorption bands at 1247 cm−1. Peaks around 1370 cm−1 and 1240 cm−1 were arranged for the symmetric C–H flexural vibration of the methyl group and the C–O vibration of the O-acetyl group (OAc), respectively.29 The signal broad absorption of OSDF and BSDF close to 890 cm−1 indicated the β-configuration of glucose.29
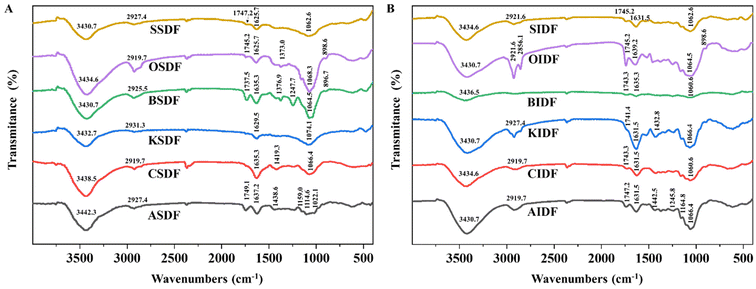 |
| Fig. 1 FTIR spectra of DF. (A) Six SDFs and (B) six IDFs. | |
A 13C solid-state NMR was applied to further clarify the structural difference between six SDFs (Fig. 2A–F) and six IDFs (Fig. 3A–F). The anomeric sugar peak close to 105 ppm matched the chemical shift of the anomeric C1. The peak in the range of 70–80 ppm belonged to chemical shifts of C2–C5. The characteristic peaks close to 60–70 ppm and 80–90 ppm sequentially come under the jurisdiction of the chemical shift of C6 and C4 in amorphous cellulose. The signal peak around 175 ppm belonged to C6 (–COOH) of GalA or/and GlcA, which were consistent with the monosaccharide composition (Tables 1 and 2). In turn, a signal peak near 50 ppm was attributed to the chemical shift of the methoxy group (OMe) and that close to 20 ppm belonged to the chemical shift of OAc.16 Compared to the NMR signals of six SDFs (Fig. 2A–F), characteristic signals of amorphous cellulose (60–70 ppm and 80–90 ppm were sequentially assigned to the chemical shift of C-6 and C-4 in amorphous cellulose) by 13C NMR qualitative analysis exist in six IDFs (Fig. 3A–F). The existence of lignin in KIDF and OIDF was evident from the signal peak close to 128 ppm in the aromatic region.30 These data combined with the results of FT-IR spectra (1060.6 cm−1–1066.4 cm−1 of cellulose characteristic peak) (Fig. 1B) and the monosaccharide composition (glucose and xylose accounted for a large proportion for all six IDFs) (Table 2) further proved that six IDFs mainly consisted of cellulose, hemicellulose and lignin.
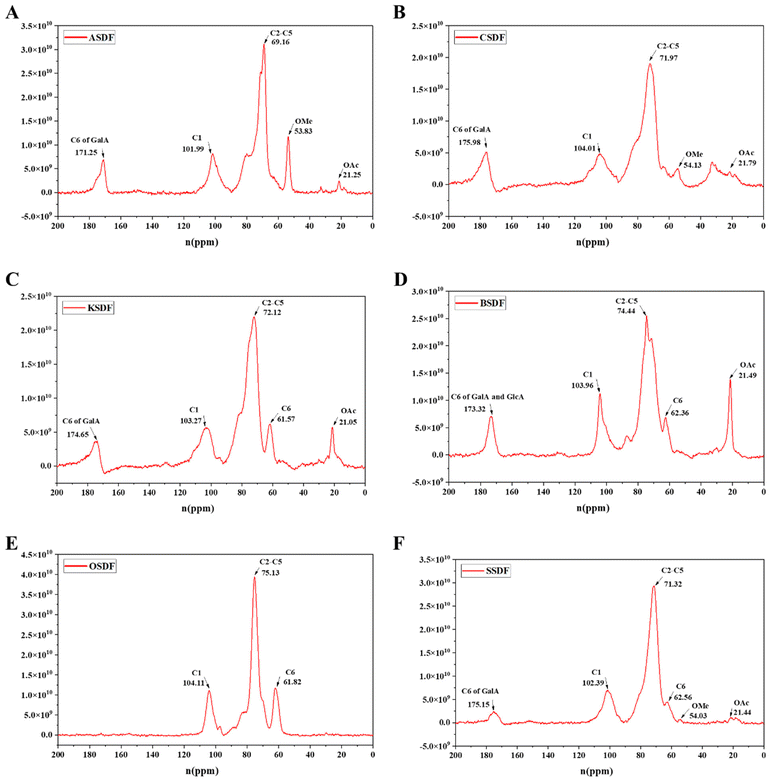 |
| Fig. 2
13C NMR spectra of six SDFs. (A) ASDF, (B) CSDF, (C) KSDF, (D) BSDF, (E) OSDF, and (F) SSDF. | |
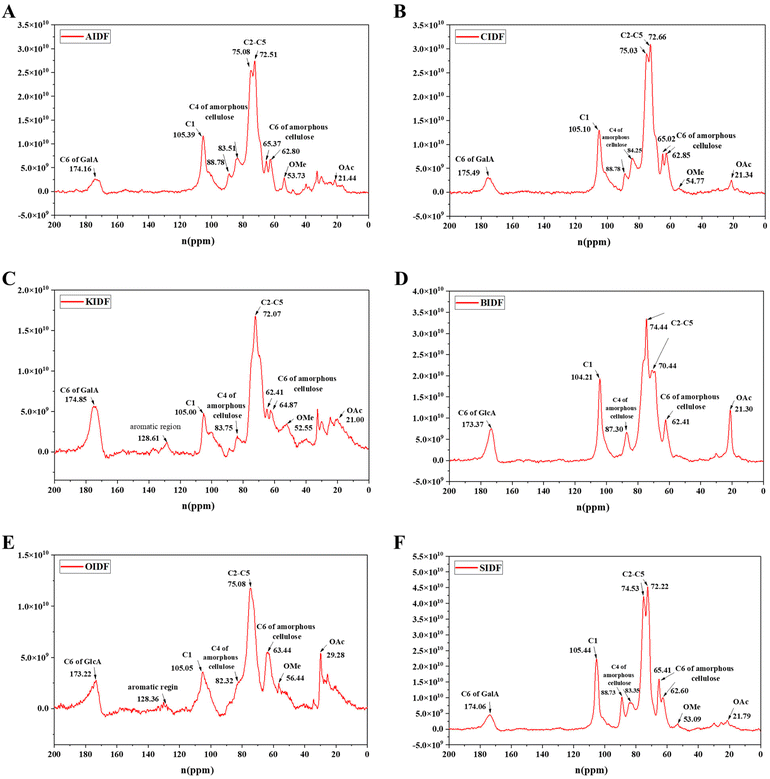 |
| Fig. 3
13C NMR spectra of six IDFs. (A) AIDF, (B) CIDF, (C) KIDF, (D) BIDF, (E) OIDF, and (F) SIDF. | |
The SEM images of the six SDFs and six IDFs at enlargement of 200-, 1000-, and 2000-fold are shown in Fig. 4. ASDF, CSDF, KSDF, BSDF, and SSDF showed haphazardly scattered sheet-like and filamentous emergence, while OSDF exhibited some inconstant micro-globular shapes along the filaments. AIDF, CIDF, KIDF, OIDF, and SIDF mainly presented sheet-like structures; then, a filamentous shape was found in the BIDF. The surface morphology results may be related to the different structures.
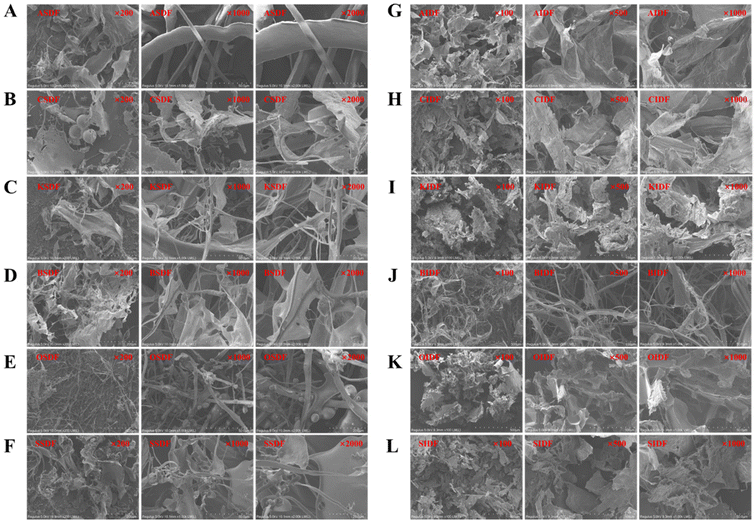 |
| Fig. 4 SEM images of six SDFs and six IDFs under the magnifications 200, 1000 and 2000. (A) ASDF, (B) CSDF, (C) KSDF, (D) BSDF, (E) OSDF, (F) SSDF, (G) AIDF, (H) CIDF, (I) KIDF, (J) BIDF, (K) OIDF, and (L) SIDF. | |
3.3 Nine ratios of insoluble to soluble dietary fibers with similar effects on the appetite and basic growth characteristics of healthy mice
To evaluate the effect of different proportions of IDF and SDF on healthy mice, nine groups of mice were fed nine proportions of IDF and SDF CFs based on AIN93G for 14 days, with mice fed with AIN93G as the Control group (Fig. S1†). Food intake, water consumption, and body weight were monitored every day. We found that there was no meaningful metamorphose in diet and water intake and body weight after the intervention of different proportions of IDF and SDF (Fig. 5A–C). The average daily feed intake of mice with an average body weight of 22 g (Fig. 5C) was 2.5 g (Fig. 5A). A guide for human and animal dose conversion indicates that drug consumption for mice is 9.01 times that for humans.31 Thus, the total DF intake for an adult weighing 60 kg was 37.83 g. After dissection, the main organ index of the liver and spleen of different diet intervention mice was analyzed. In the same way, there was no significant distinctness in the organ index of the liver (Fig. 5D) and spleen (Fig. 5E) among all the treated groups. These results indicate that intervention with NDFR does not affect the appetite and basic growth characteristics of mice.
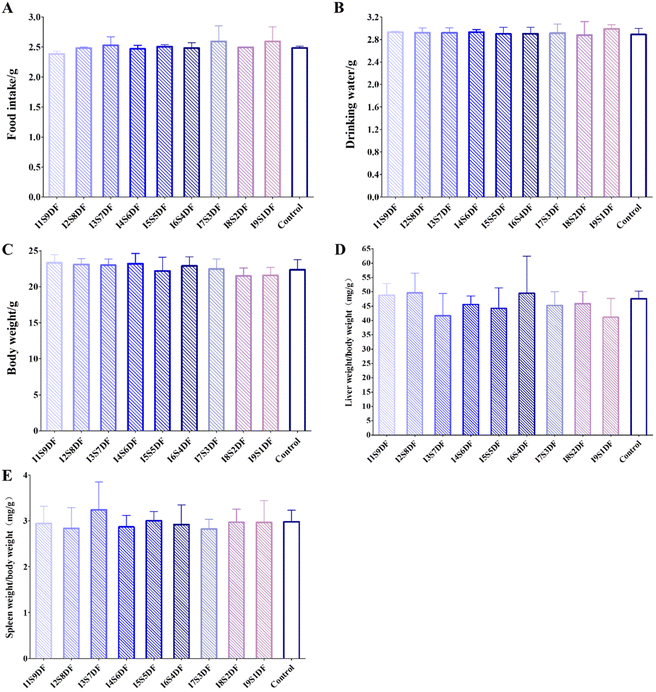 |
| Fig. 5 Effects of nine ratios of insoluble to soluble dietary fibers on appetite and basic growth characteristics of healthy mice. (A) Food intake, (B) drinking water, (C) body weight, (D) organ index of liver, and (E) organ index of spleen in mice from different groups. Data were expressed as the mean ± SD (n = 12). | |
3.4 Nine ratios of insoluble to soluble dietary fibers differently impact the gut microbiota of healthy mice
To clarify the effects of NDFR on intestinal microbiota, we performed a 16S rDNA sequencing analysis of fecal microbial DNA. The Chao1 index, Shannon index, and Observed OTUs were used to assess the α diversity (Fig. 6A–C). The α diversity exhibited an increased trend along with the proportion of IDF increased. We found that when the proportion of IDF was greater than or equal to 50%, the observed OTUs (except for the I7S3DF group) (Fig. 6C) and the Chao1 index (Fig. 6A) of all the NDFR-treated groups were remarkably higher than those of the Control group (p < 0.05). However, the Shannon index (Fig. 6B) showed only significant differences in the I8S2DF group (p < 0.01) compared with the Control group. In addition, the β diversity was displayed using NMDS analysis based on the Bray–Curtis distance at the genus level (Fig. 6D and E). Results indicated that gut microbiota had a significantly different β diversity among all experimental groups ([ANOSIM]R = 0.34041; p < 0.001; [NMDS]Stress = 0.18185).
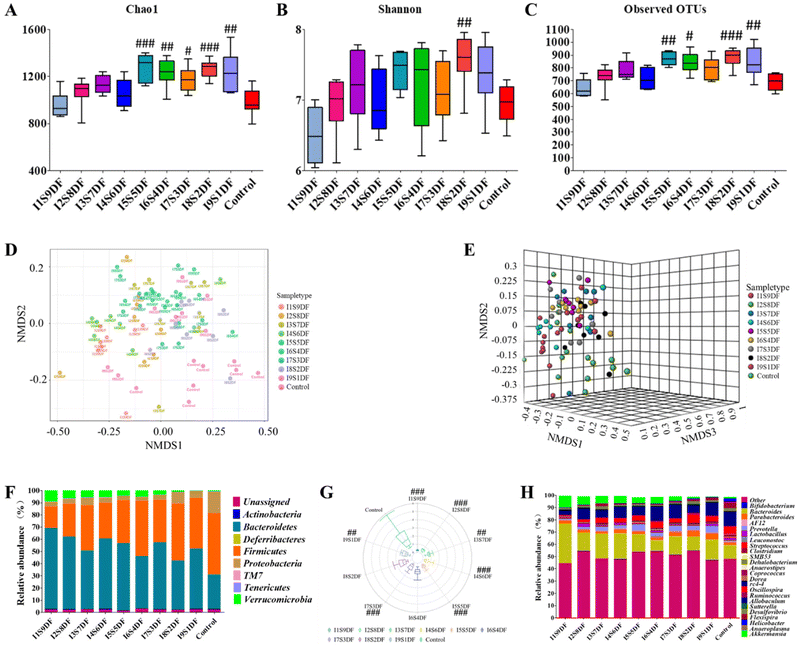 |
| Fig. 6 Effects of nine ratios of insoluble to soluble dietary fibers on gut microbiota. The α diversity index of (A) Chao1; (B) Shannon; (C) observed OTUs at genus level; (D) nonmetric multidimensional scaling (NMDS) of Bray–Curtis index distance displayed in 2D picture; (E) NMDS of Bray–Curtis index distance displayed in 3D picture ([ANOSIM]R = 0.34041; p < 0.001; [NMDS]Stress = 0.18185) at the genus level; (F) relative abundance of operational taxonomic units (OTUs) at phylum levels; (G) Firmicutes/Bacteroidetes (F/B) ratio; and (H) relative abundance of OTUs at genus levels detected by 16s rDNA sequencing in feces of mice fed NDFR for 14 days (n = 8 per group). Significant differences between groups were evaluated using one-way ANOVA, p < 0.05 was considered significant. #p < 0.05, ##p < 0.01, and ###p < 0.001 were compared with the Control group. | |
The compositions of gut microbial at the phylum levels are displayed in Fig. 6F. Results showed that Actinobacteria, Bacteroidetes, Deferribacteres, Firmicutes, Proteobacteria, TM7, Tenericutes, and Verrucomicrobia were mainly the top 8 microbes at the phylum level. In contrast with the Control group, the abundance of Proteobacteria in NDFR-treated groups decreased to different degrees. The relative abundance of Verrucomicrobia exhibited an increased trend along with the proportion of SDF increase (from 0.55% to 9.45%). For the Control group, 28.32% and 50.03% were attributed to Bacteroidetes and Firmicutes, respectively. The relative abundance of Bacteroidetes in NDFR-treated groups was higher than that in the Control group, whereas the relative abundance of Firmicutes was lower than that of the Control group; hence, the F/B rate was decreased after NDFR treatment (Except for I6S4DF and I8S2DF groups p > 0.05, p < 0.01 in other groups) (Fig. 6G). To further investigate the differences in gut microbiota between NDFR-treated groups and the Control group, the abundance of the top 25 bacteria at the genus level was surveyed (Fig. 6H). The 7 highest abundant bacteria at the genus level in the NDFR-treated groups were Bacteroides, Akkermansia, Allobaculum, Parabacteroides, Oscillospira, Desulfovibrio, and Prevotella.
To determine key characteristic bacteria in response to the ratio alteration of SDF or IDF with statistical significance in NDFR-treated groups, the dominant bacteria between the I1S9DF group and the Control group, and the I9S1DF group and the Control group in the current study were further revealed by LEfSe analysis according to α < 0.1 and LDA > 3 (Fig. 7A and B). Then, the relative abundance of characteristic bacteria in the I1S9DF and I9S1DF groups was analysed. The results indicated that the characteristic bacteria in the I1S9DF group were Bacteroides, Clostridium, Sutterella, and Akkermansia (Fig. 7A), and the dominant bacteria in the I9S1DF group were Parabacteroides, Prevotella, Clostridium, SMB53, rc4_4, Sutterella, and Helicobacter (Fig. 7B). Interestingly, the relative abundance of Akkermansia showed an increasing trend as the ratio of SDF increased (the I1S9DF group was 17.18 times that of the I9S1DF group) (Fig. 7C). However, the relative abundance of Parabacteroides and Prevotella (the I9S1DF group was 1.72, and 5.92 times that of the I1S9DF group, respectively) showed an increasing trend as the ratio of IDF increased (Fig. 7D and E). We preliminarily inferred that Akkermansia was the characteristic bacteria that responded to SDF, and Parabacteroides and Prevotella were the ones for IDF.
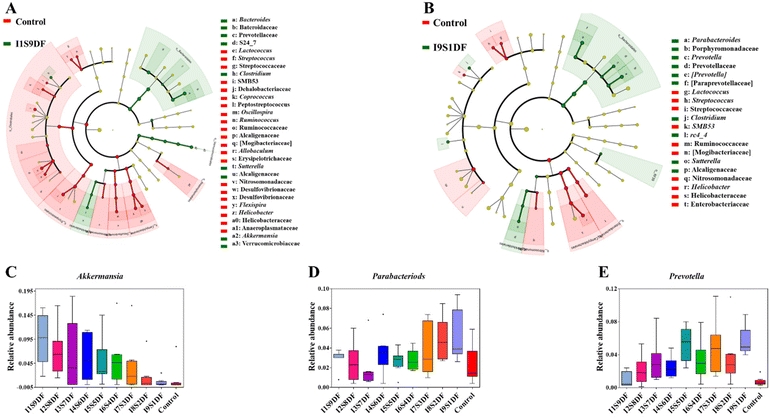 |
| Fig. 7 Key characteristic bacteria respond to the ratio alteration of SDF or IDF with statistical significance. (A) Taxonomic cladogram generated from default LEfSe analysis between the I1S9DF group and the Control group (with an LDA score of >3) (n = 8); (B) taxonomic cladogram generated from default LEfSe analysis between the I9S1DF group and the Control group (with an LDA score of >3) (n = 8); (C) relative abundances of Akkermansia; (D) relative abundances of Parabacteriods; and (E) relative abundances of Prevotella. | |
3.5 Nine ratios of insoluble to soluble dietary fibers differently impact the serum metabolites and SCFAs of healthy mice
The differences in serum metabolite profiles between different treated groups and the Control group were characterized by PCA. The results showed that the serum metabolite profiles of the NDFR-treated groups were remarkably different from those of the Control group (Fig. S3†). The higher the value in the ratio of SDF to IDF, the higher the score in PC1 (from 17.2% of the I9S1DF group to 31.4% of the I1S9DF group), indicating that this parameter was mainly projecting to PC1. Compared with the Control group, a total of 64, 62, 49, 65, 60, 72, 54, 44, and 44 endogenous metabolites (VIP > 1, FDR < 0.05, p < 0.05) were considered biomarkers in the I9S1DF group to the I1S9DF group (Fig. 8A). In addition, all the treatments share a change in 9 potential biomarkers, i.e., Sphingomyelin (d18:0/14:0), 3-hydroxyhexadecadienoylcarnitine, 9,12-hexadecadienoylcarnitine, 1,3-dihydroxypropan-2-yl (8Z,11Z,14Z,17Z)-icosa-8,11,14,17-tetraenoate (MG(0:0/20:4(8Z,11Z,14Z,17Z)/0:0)), O-(2-tetradecenoyl)-carnitine, trans-hexadec-2-enoyl carnitine, MG(0:0/20:5(5Z,8Z,11Z,14Z,17Z)/0:0), 1β-hydroxycholic acid, and 6-hydroxypentadecanedioic acid (Fig. 8B). The numbers of metabolites significantly altered by the I1S9DF and I9S1DF-treated groups were 17 and 16 (p < 0.05), respectively, which were higher than that of the other-treated groups.
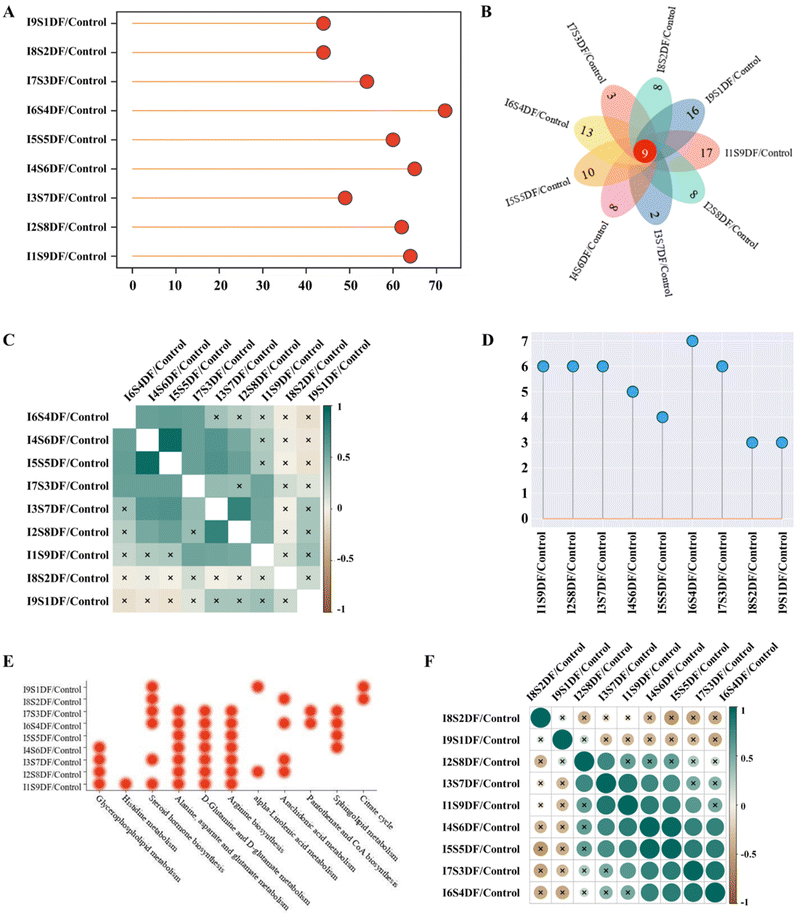 |
| Fig. 8 Effects of NDFR intervention on serum metabolites of healthy mice (n = 8). (A) The number of significantly changed metabolites; (B) the Venn diagram of significantly altered metabolites; (C) correlation analysis of ChemRICH enrichment results with significance identification using Pearson correlation algorithm; (D) Lollipop chart of metabolic pathway numbers; (E) bubble chart of metabolic pathways enriched (based on impact factor > 0.1); and (F) correlation analysis of metabolic pathways with significance identification using Pearson correlation algorithm between the NDFR-treated groups and the Control group (based VIP > 1, p < 0.05, FDR < 0.05). × represent significant differences (p < 0.05). | |
The chemRICH enrichment analysis of biomarkers was executed between the NDFR-treated groups and the Control group. 24 metabolite clusters (carnitine, saturated_lysophospholipids, phosphatidylinositols, ethanolamines, diglycerides, glycerides, cholic acids, pregnenes, alkenes, lysophosphatidylcholines, polyunsaturated alkamides, saturated_fatty acids, oxysterols, saturated_ceramides, lysophospholipids, oxo-ETE, cholesterol, glycerophospholipids, tricarboxylic acids, pregnenediones, O=FA_22_6, OH-FA_27_2_2, O=FA_18_0, and OH-FA_20_0_2) were significantly altered by the administration of NDFR (Fig. S4†). Pearson's correlation heatmap was created and presented the differences in chemRICH enrichment analysis between the NDFR-treated groups and the Control group, as shown in Fig. 8C. It was found that the I8S2DF and I9S1DF groups were significantly different in chemRICH enrichment analysis from other treated groups (these groups with SDF accounting for 30% or more) (p < 0.05). The I1S9DF group showed significant differences in chemRICH enrichment analysis from the I4S6DF, I5S5DF, I6S4DF, I8S2DF, and I9S1DF groups (these five groups with IDF accounting for over 40%) (p < 0.05). The I2S8DF group showed significant differences in chemRICH enrichment analysis from the I6S4DF, I7S3DF, I8S2DF, and I9S1DF groups (these four groups with IDF account for over 60%) (p < 0.05). This result also indicated that the changes in some metabolites were related to the dosage of SDF and IDF. Metabolic pathways of the biomarkers between the NDFR-treated groups and the Control group are displayed in Fig. S5† (based on impact factor >0.1). The I6S4DF group possessed the highest number of metabolic pathways (based on impact factor >0.1) (Fig. 8D), including steroid hormone biosynthesis, alanine, aspartate and glutamate metabolism, sphingolipid metabolism, arachidonic acid metabolism, D-glutamine and D-glutamate metabolism, arginine biosynthesis, and pantothenate and CoA biosynthesis (Fig. 8E). When the ratio of SDF reaches 60% or more (the I4S6DF, I3S7DF, I2S8DF, and I1S9DF groups), the glycerophospholipid metabolism pathway was significantly enriched (p < 0.05) (Fig. 8E). Moreover, when the ratio of IDF reaches 80% or more (the I8S2DF and I9S1DF groups), the citrate cycle (TCA cycle) pathway was significantly regulated (p < 0.05) (Fig. 8E). To further investigate the correlation of metabolic pathways between the different groups, Pearson's correlation analysis was performed (Fig. 8F). The results showed that the I8S2DF and I9S1DF groups separately exhibited significant differences from all other treated groups (p < 0.05) (Fig. 8F).
We further investigated the effect of NDFR treatment on SCFAs (Fig. 9A–C). Both the levels of cecal acetic acid and propionic acid showed a decreasing trend as the ratio of SDF decreased. When the ratio of SDF reaches 20% or less, the levels of acetic acid and propionic acid are significantly lower compared with those of the I1S9DF group (p < 0.05) (Fig. 9A and B). However, the production of butyric acid was not dose-dependent on SDF (Fig. 9C).
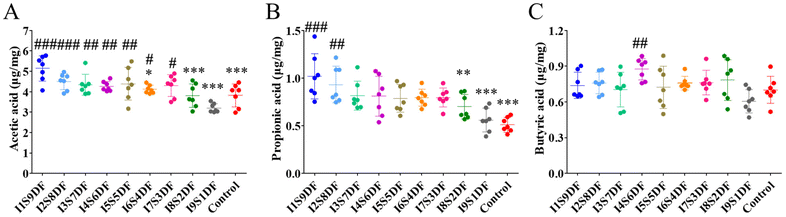 |
| Fig. 9 Effects of nine ratios of insoluble to soluble dietary fibers on SCFAs of healthy mice. Significant differences between groups were evaluated using one-way ANOVA; p < 0.05 was considered significant. (A) Acetic acid, (B) propionic acid, and (C) butyric acid. *p < 0.05, **p < 0.01, and ***p < 0.001 were compared with I1S9DF group; #p < 0.05, ##p < 0.01, and ###p < 0.001 were compared with I9S1DF group (n = 7). | |
4. Discussion
An increasing number of studies have shown that a low intake of DF is responsible for the risk of developing metabolic diseases, highlighting the need to enhance human DF intake globally.11,32–34 The development of DF supplements is an important way to improve DF deficiency. Solubility, as an important physical property, affects the function of DF. However, there is no scientific basis for the ratio of IDF to SDF in the process of developing DF supplements. Fruits, vegetables, grains, and legumes are the main sources of DF.35,36 Consequently, we isolated six SDFs and six IDFs from apple, celery, kale, black fungus, oats, and soybeans to design NDFR with nine ratios of IDF to SDF (from 1
:
9 to 9
:
1). DF mainly plays a role in the large intestine either as a substrate for microorganisms to ferment or as functional niches for microbial colonization. Positive regulation of gut microbiota is a key manifestation of DF's health effects. In this study, we assessed the effect of DF with nine ratios of IDF to SDF on gut microbiota and metabolism regulation in healthy mice using amplicon sequencing and metabolomics. We found that the characteristic responsive bacteria for SDF and IDF were distinctive. This study provides a scientific basis for the ratio of IDF and SDF in the process of developing DF supplements based on gut microbiota and metabolites.
Six SDFs and six IDFs were separately obtained with little protein and ash remaining. SDF was mainly composed of pectin (ASDF and CSDF), β-glucan (OSDF), and other neutral NSP (KSDF, BSDF, and SSDF), whereas IDF mainly consisted of cellulose, hemicellulose and lignin. Pectin, β-glucan, cellulose, and hemicellulose are representative DF sources in our natural food,12,37 indicating that our research has certain practical guiding significance.
Both SDF and IDF intervention can alter the landscape of gut microbiota and intestinal microbiota diversity.14,15,38–41 It was observed that the α diversity increased as the ratio of IDF increased, indicating that a high ratio of IDF is more advantageous to improve the species richness in communities than a high ratio of SDF. A lot of research evidence suggests that the expansion of Proteobacteria is a microbial characteristic of gut dysbiosis.42 Unhealthy diets, such as high-sugar or/and high-fat, are also important factors driving the expansion of Proteobacteria.43 The alteration of the relative abundance of Proteobacteria is one of the indicators to evaluate dietary health effects. It was interesting to find that the relative abundance of Proteobacteria decreased in the NDFR-treated groups, but there was no dose-dependent relationship with the ratios of IDF and SDF. Changes in the relative abundance of the two prominent bacterial divisions, the Bacteroidetes, and the Firmicutes were the typical characteristics of the gut microbiota in obese individuals.44 Obesity is often accompanied by a decrease in Bacteroidetes and an increase in Firmicutes, that is, the ratio of F/B increases. Nine ratios of IDF and SDF treatment decreased the F/B ratio, and the decrease rate increased as the ratio of SDF increased. The LEfSe analysis demonstrated that the characteristic bacteria in the I1S9DF group were Bacteroides and Akkermansia and those in the I9S1DF group were Parabacteroides and Prevotella. The genomics of Bacteroides species were rich in the gene responsible for the uptake and breakdown of complex polysaccharides-based DF.45 Our previous investigation demonstrated that A. muciniphila does not directly utilize dietary fiber as a substrate, and the intervention of dietary fiber greatly affects its abundance.14 Dietary fiber improves host health, which is usually accompanied by the increase in A. muciniphila.14A. muciniphila exerts beneficial effects on alleviating glucose tolerance, insulin resistance, abnormal cholesterol levels and related disorders.25Parabacteroides distasonis, the ex-holotype of Parabacteroides, plays an important role in human health, including diabetes, colorectal cancer and inflammatory bowel disease.46P. distasonis was a typical example of pectinolytic intestinal commensals.25 Studies have shown that the abundance of both Prevotella and Bacteroides enterotypes is particularly described as features indicating fiber intake.47Prevotella copri types with enhanced potential for carbohydrate catabolism were associated with fiber-rich diets.48 SDF enters the intestine and can be fermented partly or entirely by large intestine probiotics, resulting in a large number of SCFAs, which are closely linked to human health. A. muciniphila is an acetic acid and propionic acid producer.14 Interestingly, the relative abundance of Akkermansia and the level of acetic acid and propionic acid showed increasing trends as the ratio of SDF increased. In addition, the relative abundance of Parabacteroides and Prevotella showed an increasing trend as the ratio of IDF increased. Thus, we must consider whether Akkermansia is the characteristic responsive bacteria of the SDF, and whether Parabacteroides and Prevotella are the characteristic responsive bacteria of the IDF. Further research is urgently needed to confirm this inference.
The serum metabolome analysis indicated that a high ratio of SDF (≥60%) significantly enriched the glycerophospholipid metabolism pathway. Glycerophospholipid is the most abundant phospholipid in the organism and is an important component of the membrane; in addition, glycerophospholipids are involved in protein recognition and signal transduction of cell membranes.49,50 Studies have shown that the fermentation of SDF produces SCFAs, which may have positive effects on lipid metabolism.36 However, a high ratio of IDF (≥80%) may tend to regulate the TCA cycle. The TCA cycle is distributed throughout mitochondria and is the final metabolic pathway and connection hub of sugars, lipids, and amino acids. Furthermore, the TCA cycle is closely associated with energy metabolism.51
5. Conclusion
In summary, our study suggests that DF with distinct ratios of IDF to SDF differentially affects gut microbiota and serum metabolism in healthy mice. A high ratio of SDF tends to improve the abundance of Akkermansia, production of acetic acid and propionic acid and regulation of the glycerophospholipid metabolism pathway. However, a high ratio of IDF may tend to enrich the relative abundance of Parabacteroides and Prevotella and together regulate the TCA cycle. We preliminarily infer that both a high-SDF diet and a high-IDF diet could prevent metabolic disease with a significant disturbance of glucose and lipid metabolism and gut microbiota in healthy individuals. These findings are helpful in the development of dietary fiber supplements based on gut microbiota and metabolites. However, further experimental verification is needed to validate our predictions. It is also necessary to explore action mechanisms in future research that will have more guiding significance for product development. We selected SDFs and IDFs from only six representative foods. It remains to be verified whether the regulation rule commonly exists in all DF.
Author contributions
Yanli Zhang: Data curation, investigation, methodology, software, visualization, writing – original draft. Jielun Hu: Conceptualization, methodology, data curation, writing – review & editing. Yadong Zhong: Conceptualization, methodology, data curation, writing – review & editing. Shuai Liu: Methodology, investigation. Liandi Liu: Methodology, investigation. Xinyi Mu: Methodology, investigation. Chunhua Chen: Data curation, investigation. Shenji Yang: Methodology, investigation. Guohao Li: Methodology, investigation. Duoduo Zhang: Data curation, investigation. Xinru Huang: Methodology, investigation. Jinrui Yang: Data curation, investigation. Xiaojun Huang: Data curation, investigation. Shuigen Bian: Methodology, investigation. Shaoping Nie: Conceptualization, funding acquisition, supervision, project administration, writing – review & editing.
Abbreviations
DF | Dietary fiber |
SDF | Soluble dietary fiber |
IDF | Insoluble dietary fiber |
TCA | Tricarboxylic acid |
PCR | Polymerase chain reaction |
T2DM | Diabetes mellitus type 2 |
F/B | Firmicutes/Bacteroidetes |
CMD | Cardio-metabolic disease |
AOAC | Association of official analytical chemists |
HPGPC | High-performance gel permeation chromatography |
FT-IR | Fourier transform infrared spectrum |
NDFR | Nine dietary fiber recipes composed of IDF and SDF with a ratio from 1 : 9 to 9 : 1 |
SEM | Scanning electron microscope |
CFs | Custom feeds |
QC | Quality control |
PLS-DA | Partial least-squares-discrimination analysis |
PCA | Principal component analysis |
Data availability
Data will be made available on request.
Conflicts of interest
There are no conflicts of interest to declare.
Acknowledgements
This work was supported by the National Natural Science Foundation of China for Distinguished Young Scholars (31825020); the Technological Innovation Guidance Science and Technology Project of Jiangxi Province (20203AEI007); the Key Technological Project of Jiangxi Province (20212AAF01005); and Key Laboratory of Bioactive Polysaccharides of Jiangxi Province (20212BCD42016).
References
- J. Wu, K. Wang, X. Wang, Y. Pang and C. Jiang, The role of the gut microbiome and its metabolites in metabolic diseases, Protein Cell, 2021, 12, 360–373, DOI:10.1007/s13238-020-00814-7.
- Y. Fan and O. Pedersen, Gut microbiota in human metabolic health and disease, Nat. Rev. Microbiol., 2021, 19, 55–71, DOI:10.1038/s41579-020-0433-9.
- K. H. Allin, V. Tremaroli, R. Caesar, B. A. H. Jensen, M. T. F. Damgaard, M. I. Bahl, T. R. Licht, T. H. Hansen, T. Nielsen, T. M. Dantoft, A. Linneberg, T. Jorgensen, H. Vestergaard, K. Kristiansen, P. W. Franks, T. Hansen, F. Backhed, O. Pedersen and I. D. Consortium, Aberrant intestinal microbiota in individuals with prediabetes, Diabetologia, 2018, 61, 810–820, DOI:10.1007/s00125-018-4550-1.
- H. Zhong, H. Ren, Y. Lu, C. Fang, G. Hou, Z. Yang, B. Chen, F. Yang, Y. Zhao, Z. Shi, B. Zhou, J. Wu, H. Zou, J. Zi, J. Chen, X. Bao, Y. Hu, Y. Gao, J. Zhang, X. Xu, Y. Hou, H. Yang, J. Wang, S. Liu, H. Jia, L. Madsen, S. Brix, K. Kristiansen, F. Liu and J. Li, Distinct gut metagenomics and metaproteomics signatures in prediabetics and treatment-naive type 2 diabetics, Ebiomedicine, 2019, 47, 373–383, DOI:10.1016/j.ebiom.2019.08.048.
- T. Le Roy, M. Llopis, P. Lepage, A. Bruneau, S. Rabot, C. Bevilacqua, P. Martin, C. Philippe, F. Walker, A. Bado, G. Perlemuter, A.-M. Cassard-Doulcier and P. Gerard, Intestinal microbiota determines development of non-alcoholic fatty liver disease in mice, Gut, 2013, 62, 1787–1794, DOI:10.1136/gutjnl-2012-303816.
- J. Yuan, C. Chen, J. Cui, J. Lu, C. Yan, X. Wei, X. Zhao, N. Li, S. Li, G. Xue, W. Cheng, B. Li, H. Li, W. Lin, C. Tian, J. Zhao, J. Han, D. An, Q. Zhang, H. Wei, M. Zheng, X. Ma, W. Li, X. Chen, Z. Zhang, H. Zeng, S. Ying, J. Wu, R. Yang and D. Liu, Fatty liver disease caused by high-alcohol-producing Klebsiella pneumoniae, Cell Metab., 2019, 30, 675–688, DOI:10.1016/j.cmet.2019.11.006.
- D. Rothschild, O. Weissbrod, E. Barkan, A. Kurilshikov, T. Korem, D. Zeevi, P. I. Costea, A. Godneva, I. N. Kalka, N. Bar, S. Shilo, D. Lador, A. V. Vila, N. Zmora, M. Pevsner-Fischer, D. Israeli, N. Kosower, G. Malka, B. C. Wolf, T. Avnit-Sagi, M. Lotan-Pompan, A. Weinberger, Z. Halpern, S. Carmi, J. Fu, C. Wijmenga, A. Zhernakova, E. Elinav and E. Segal, Environment dominates over host genetics in shaping human gut microbiota, Nature, 2018, 555, 210–215, DOI:10.1038/nature25973.
- S. Subramanian, L. V. Blanton, S. A. Frese, M. Charbonneau, D. A. Mills and J. I. Gordon, Cultivating healthy growth and nutrition through the gut microbiota, Cell, 2015, 161, 36–48, DOI:10.1016/j.cell.2015.03.013.
- N. Zhang, Z. Ju and T. Zuo, Time for food: The impact of diet on gut microbiota and human health, Nutrition, 2018, 51–52, 80–85, DOI:10.1016/j.nut.2017.12.005.
- S. K. Gill, M. Rossi, B. Bajka and K. Whelan, Dietary fibre in gastrointestinal health and disease, Nat. Rev. Gastroenterol. Hepatol., 2021, 18, 101–116, DOI:10.1038/s41575-020-00375-4.
- A. Afshin, P. J. Sur, K. A. Fay, L. Cornaby, G. Ferrara, J. S. Salama, E. C. Mullany, K. H. Abate, C. Abbafati, Z. Abebe, M. Afarideh, A. Aggarwal, S. Agrawal, T. Akinyemiju, F. Alahdab, U. Bacha, V. F. Bachman, H. Badali, A. Badawi, I. M. Bensenor, E. Bernabe, S. H. Biryukov, S. K. K. Biadgilign, L. E. Cahill, J. J. Carrero, K. M. Cercy, L. Dandona, R. Dandona, A. K. Dang, M. G. Degefa, M. E. S. Zaki, A. Esteghamati, S. Esteghamati, J. Fanzo, C. S. E. S. Farinha, M. S. Farvid, F. Farzadfar, V. L. Feigin, J. C. Fernandes, L. S. Flor, N. A. Foigt, M. H. Forouzanfar, M. Ganji, J. M. Geleijnse, R. F. Gillum, A. C. Goulart, G. Grosso, I. Guessous, S. Hamidi, G. J. Hankey, S. Harikrishnan, H. Y. Hassen, S. I. Hay, C. L. Hoang, M. Horino, F. Islami, M. D. Jackson, S. L. James, L. Johansson, J. B. Jonas, A. Kasaeian, Y. S. Khader, I. A. Khalil, Y.-H. Khang, R. W. Kimokoti, Y. Kokubo, G. A. Kumar, T. Lallukka, A. D. Lopez, S. Lorkowski, P. A. Lotufo, R. Lozano, R. Malekzadeh, W. Marz, T. Meier, Y. A. Melaku, W. Mendoza, G. B. M. Mensink, R. Micha, T. R. Miller, M. Mirarefin, V. Mohan, A. H. Mokdad, D. Mozaffarian, G. Nagel, M. Naghavi, C. T. Nguyen, M. R. Nixon, K. L. Ong, D. M. Pereira, H. Poustchi, M. Qorbani, R. K. Rai, C. Razo-Garcia, C. D. Rehm, J. A. Rivera, S. Rodriguez-Ramirez, G. Roshandel, G. A. Roth, J. Sanabria, T. G. Sanchez-Pimienta, B. Sartorius, J. Schmidhuber, A. E. Schutte, S. G. Sepanlou, M.-J. Shin, R. J. D. Sorensen, M. Springmann, L. Szponar, A. L. Thorne-Lyman, A. G. Thrift, M. Touvier, B. X. Tran, S. Tyrovolas, K. N. Ukwaja, I. Ullah, O. A. Uthman, M. Vaezghasemi, T. J. Vasankari, S. E. Vollset, T. Vos, G. T. Vu, V. Linh Gia, E. Weiderpass, A. Werdecker, T. Wijeratne, W. C. Willett, J. H. Wu, G. Xu, N. Yonemoto, C. Yu, C. J. L. Murray and G. B. D. D. Collaborators, Health effects of dietary risks in 195 countries, 1990-2017: a systematic analysis for the Global Burden of Disease Study 2017, Lancet, 2019, 393, 1958–1972, DOI:10.1016/S0140-6736(21)01342-8.
- A. M. Stephen, M. M. J. Champ, S. J. Cloran, M. Fleith, L. van Lieshout, H. Mejborn and V. J. Burley, Dietary fibre in Europe: current state of knowledge on definitions, sources, recommendations, intakes and relationships to health, Nutr. Res. Rev., 2017, 30, 149–190, DOI:10.1017/S095442241700004X.
- J. O'Grady, E. M. O'Connor and F. Shanahan, Review article: dietary fibre in the era of microbiome science, Aliment. Pharmacol. Ther., 2019, 49, 506–515, DOI:10.1111/apt.15129.
- Y. Zhang, J. Hu, H. Tan, Y. Zhong and S. Nie,
Akkermansia muciniphila, an important link between dietary fiber and host health, Curr. Opin. Food Sci., 2022, 47, 100905, DOI:10.1016/j.cofs.2022.100905.
- T. Sauvaitre, L. Etienne-Mesmin, A. Sivignon, P. Mosoni, C. M. Courtin, T. Van de Wiele and S. Blanquet-Diot, Tripartite relationship between gut microbiota, intestinal mucus and dietary fibers: towards preventive strategies against enteric infections, FEMS Microbiol. Rev., 2021, 45, fuaa052, DOI:10.1093/femsre/fuaa052.
- H. Wang, X. Huang, S. Xia, C. Chen, X. Chen, Y. Zhang, M. A. Farag, J. Xiao and S. Nie, Celery soluble dietary fiber antagonizes flavonoids ameliorative effect on dextran-sodium-sulfate-induced colitis in mice, J. Adv. Res., 2023, 52, 73–88, DOI:10.1016/j.jare.2023.01.013.
- S. Liu, M. Jia, J. Chen, H. Wan, R. Dong, S. Nie, M. Xie and Q. Yu, Removal of bound polyphenols and its effect on antioxidant and prebiotics properties of carrot dietary fiber, Food Hydrocolloids, 2019, 93, 284–292, DOI:10.1016/j.foodhyd.2019.02.047.
- M. Dubois, K. A. Gilles, J. K. Hamilton, P. A. Rebers and F. Smith, Colorimetric method for determination of sugars and related substances, Anal. Chem., 1956, 28, 350–356, DOI:10.1021/ac60111a017.
- T. Bitter and H. M. Muir, A modified uronic acid carbazole reaction, Anal. Biochem., 1962, 4, 330–334, DOI:10.1016/0003-2697(62)90095-7.
- W. Tang, C. Liu, J. Liu, L. Hu, Y. Huang, L. Yuan, F. Liu, S. Pan, S. Chen, S. Bian, X. Huang, J. Yin and S. Nie, Purification of polysaccharide from Lentinus edodes water extract by membrane separation and its chemical composition and structure characterization, Food Hydrocolloids, 2020, 105, 105851, DOI:10.1016/j.foodhyd.2020.105851.
- Y. Wang, J. Yin, X. Huang and S. Nie, Structural characteristics and rheological properties of high viscous glucan from fruit body of Dictyophora rubrovolvata, Food Hydrocolloids, 2020, 101, 105514, DOI:10.1016/j.foodhyd.2019.105514.
- Q. Nie, H. Chen, J. Hu, H. Gao, L. Fan, Z. Long and S. Nie, Arabinoxylan attenuates type 2 diabetes by improvement of carbohydrate, lipid, and amino acid metabolism, Mol. Nutr. Food Res., 2018, 62, e1800222, DOI:10.1002/mnfr.201800222.
- J. Hu, S. Nie, F. Min and M. Xie, Polysaccharide from seeds of Plantago asiatica L. increases short-chain fatty acid production and fecal moisture along with lowering pH in mouse colon, J. Agric. Food Chem., 2012, 60, 11525–11532, DOI:10.1021/jf302169u.
- J. Wen, M. Li, H. Gao, J. Hu, Q. Nie, H. Chen, Y. Zhang, M. Xie and S. Nie, Polysaccharides from fermented Momordica charantia L. with Lactobacillus plantarum NCU116 ameliorate metabolic
disorders and gut microbiota change in obese rats, Food Funct., 2021, 12, 2617–2630, 10.1039/d0fo02600j.
- H. Tan and S. Nie, Deciphering diet-gut microbiota-host interplay: Investigations of pectin, Trends Food Sci. Technol., 2020, 106, 171–181, DOI:10.1016/j.tifs.2020.10.010.
- S. Sammalisto, M. Laitinen and T. Sontag-Strohm, Baking quality assessment of twenty whole grain oat cultivar samples, Foods, 2021, 10, 2461, DOI:10.3390/foods10102461.
- F. J. Dai and C. F. Chau, Classification and regulatory perspectives of dietary fiber, J. Food Drug Anal., 2017, 25, 37–42, DOI:10.1016/j.jfda.2016.09.006.
- W. Fan, G. Ge, Y. Liu, W. Wang, L. Liu and Y. Jia, Proteomics integrated with metabolomics: analysis of the internal causes of nutrient changes in alfalfa at different growth stages, BMC Plant Biol., 2018, 18, 78, DOI:10.1186/s12870-018-1291-8.
- X. Shi, J. Yin, L. Zhang, X. Huang and S. Nie, Studies on O-acetyl-glucomannans from Amorphophallus species: Comparison of physicochemical properties and primary structures, Food Hydrocolloids, 2019, 89, 503–511, DOI:10.1016/j.foodhyd.2018.11.013.
- J. Mao, K. M. Holtman, J. T. Scott, J. F. Kadla and K. Schmidt-Rohr, Differences between lignin in unprocessed wood, milled wood, mutant wood, and extracted lignin detected by 13C solid-state NMR, J. Agric. Food Chem., 2006, 54, 9677–9686, DOI:10.1021/jf062199q.
- H. Han, Y. Ma, X. Wang, R. Yun, S. Lu, M. Xia, Y. Wang, Q. Shi, W. Zhai, Q. Liang and H. Xu, Fang-Ji-Huang-Qi-Tang attenuates degeneration of early-stage KOA mice related to promoting joint lymphatic drainage function, J. Evidence-Based Complementary Altern. Med., 2020, 2020, 3471681, DOI:10.1155/2020/3471681.
- A. Reynolds, J. Mann, J. H. Cummings, N. Winter, E. Mete and L. Te Morenga, Carbohydrate quality and human health: a series of systematic reviews and meta-analyses, Lancet, 2019, 393, 434–445, DOI:10.1016/S0140-6736(18)31809-9.
- X. Liu, W. Yang, J. L. Petrick, L. M. Liao, W. Wang, N. He, P. T. Campbell, Z. Zhang, E. Gioyannucci, K. A. McGlynn and X. Zhang, Higher intake of whole grains and dietary fiber are associated with lower risk of liver cancer and chronic liver disease mortality, Nat. Commun., 2021, 12, 6388, DOI:10.1038/s41467-021-26448-9.
- H. C. Wastyk, G. K. Fragiadakis, D. Perelman, D. Dahan, B. D. Merrill, F. B. Yu, M. Topf, C. G. Gonzalez, W. Van Treuren, S. Han, J. L. Robinson, J. E. Elias, E. D. Sonnenburg, C. D. Gardner and J. L. Sonnenburg, Gut-microbiota-targeted diets modulate human immune status, Cell, 2021, 184, 4137–4153, DOI:10.1016/j.cell.2021.06.019.
- J. A. Marlett and T. F. Cheung, Database and quick methods of assessing typical dietary fiber intakes using data for 228 commonly consumed foods, J. Am. Diet. Assoc., 1997, 97, 1139–1151, DOI:10.1016/S0002-8223(97)00275-7.
- V. Tejada-Ortigoza, L. E. Garcia-Amezquita, S. O. Serna-Saldivar and J. Welti-Chanes, Advances in the functional characterization and extraction processes of dietary fiber, Food Eng. Rev., 2016, 8, 251–271, DOI:10.1007/s12393-015-9134-y.
- A. Papathanasopoulos and M. Camilleri, Dietary fiber supplements: Effects in obesity and metabolic syndrome and relationship to gastrointestinal functions, Gastroenterology, 2010, 138, 65–72, DOI:10.1053/j.gastro.2009.11.045.
- M. Qiu, J. Hu, H. Peng, B. Li, J. Xu, X. Song, C. Yu, Z. Zhang, X. Du, G. Bu, A. Huang, X. Han, X. Zeng, C. Yang and F. Kong, Research Note: The gut microbiota varies with dietary fiber levels in broilers, Poult. Sci., 2022, 101, 101922, DOI:10.1016/j.psj.2022.101922.
- J. M. Lattimer and M. D. Haub, Effects of dietary fiber and its components on metabolic health, Nutrients, 2010, 2, 1266–1289, DOI:10.3390/nu2121266.
- M. Serino, E. Luche, S. Gres, A. Baylac, M. Berge, C. Cenac, A. Waget, P. Klopp, J. Iacovoni, C. Klopp, J. Mariette, O. Bouchez, J. Lluch, F. Ouarne, P. Monsan, P. Valet, C. Roques, J. Amar, A. Bouloumie, V. Theodorou and R. Burcelin, Metabolic adaptation to a high-fat diet is associated with a change in the gut microbiota, Gut, 2012, 61, 543–553, DOI:10.1136/gutjnl-2011-301012.
- X. Zhai, D. Lin, Y. Zhao, W. Li and X. Yang, Effects of dietary fiber supplementation on fatty acid metabolism and intestinal microbiota diversity in C57BL/6J mice fed with a high-fat diet, J. Agric. Food Chem., 2018, 66, 12706–12718, DOI:10.1021/acs.jafc.8b05036.
- Y. Litvak, M. X. Byndloss, R. M. Tsolis and A. J. Baumler, Dysbiotic Proteobacteria expansion: a microbial signature of epithelial dysfunction, Curr. Opin. Microbiol., 2017, 39, 1–6, DOI:10.1016/j.mib.2017.07.003.
- V. G. de Oliveira Neves, D. T. de Oliveira, D. C. Oliveira, L. O. Perucci, T. A. P. dos Santos, I. da Costa Fernandes, G. G. de Sousa, N. R. Barboza and R. Guerra-Sa, High-sugar diet intake, physical activity, and gut microbiota crosstalk: Implications for obesity in rats, Nutr. Food Sci., 2020, 8, 5683–5695, DOI:10.1002/fsn3.1842.
- P. J. Turnbaugh, R. E. Ley, M. A. Mahowald, V. Magrini, E. R. Mardis and J. I. Gordon, An obesity-associated gut microbiome with increased capacity for energy harvest, Nature, 2006, 444, 1027–1031, DOI:10.1038/nature05414.
- N. D. Schwalm II and E. A. Groisman, Navigating the gut buffet: Control of polysaccharide utilization in Bacteroides spp, Trends Microbiol., 2017, 25, 1005–1015, DOI:10.1016/j.tim.2017.06.009.
- Q. Zhao, M.-Y. Dai, R.-Y. Huang, J.-Y. Duan, T. Zhang, W.-M. Bao, J.-Y. Zhang, S.-Q. Gui, S.-M. Xia, C.-T. Dai, Y.-M. Tang, F. J. J. Gonzalez and F. Li,
Parabacteroides distasonis ameliorates hepatic fibrosis potentially via modulating intestinal bile acid metabolism and hepatocyte pyroptosis in male mice, Nat. Commun., 2023, 14, 1829, DOI:10.1038/s41467-023-37459-z.
- R. L. Hughes, M. E. Kable, M. Marco and N. L. Keim, The role of the gut microbiome in predicting response to diet and the development of precision nutrition models. Part II: Results, Adv. Nutr., 2019, 10, 979–998, DOI:10.1093/advances/nmz049.
- F. De Filippis, E. Pasolli, A. Tett, S. Tarallo, A. Naccarati, M. De Angelis, E. Neviani, L. Cocolin, M. Gobbetti, N. Segata and D. Ercolini, Distinct genetic and functional traits of human intestinal Prevotella copri strains are associated with different habitual diets, Cell Host Microbe, 2019, 25, 444–453, DOI:10.1016/j.chom.2019.01.004.
- S. Wang, K. Tang, Y. Lu, Z. Tian, Z. Huang, M. Wang, J. Zhao and J. Xie, Revealing the role of glycerophospholipid metabolism in asthma through plasma lipidomics, Clin. Chim. Acta, 2021, 513, 34–42, DOI:10.1016/j.cca.2020.11.026.
- J. Li, L. Li, R. Liu, L. Zhu, B. Zhou, Y. Xiao, G. Hou, L. Lin, X. Chen and C. Peng, Integrative lipidomic features identify plasma lipid signatures in chronic urticaria, Front. Immunol., 2022, 13, 933312, DOI:10.3389/fimmu.2022.933312.
- L. J. Sweetlove, K. F. M. Beard, A. Nunes-Nesi, A. R. Fernie and R. G. Ratcliffe, Not just a circle: flux modes in the plant TCA cycle, Trends Plant Sci., 2010, 15, 462–470, DOI:10.1016/j.tplants.2010.05.006.
|
This journal is © The Royal Society of Chemistry 2024 |
Click here to see how this site uses Cookies. View our privacy policy here.