DOI:
10.1039/D4GC02329C
(Tutorial Review)
Green Chem., 2024,
26, 9569-9598
High entropy materials: potential catalysts for electrochemical water splitting
Received
11th May 2024
, Accepted 8th July 2024
First published on 12th July 2024
Abstract
High entropy materials (HEM) have been attracting much attention as emerging catalysts for electrochemical water splitting. HEM catalysts have unique properties such as an interesting cocktail effect, broad design space, customizable electronic structure, and excellent entropy stabilization effect compared with single-digit catalysts. This paper provides a comprehensive overview of the use of HEM as a catalyst for hydrogen evolution reaction (HER), oxygen evolution reaction (OER), and water splitting. HEM design strategies including phase structure modulation, defect engineering, electrode configuration engineering, and computational aids are discussed to develop HEM catalysts with high performance and stability. In particular, the importance of density functional theory, high-throughput screening techniques, and machine learning for the discovery and design of HEM catalysts is emphasized. Finally, the challenges and imminent difficulties are prospected, and the corresponding strategies to deal with these challenges are put forward to promote the development of HEM catalysts in the field of electrochemical water splitting.
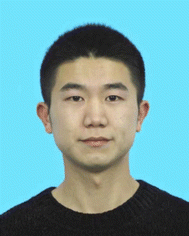 Zhong Wang | Zhong Wang received a bachelor's degree in 2019, and is currently studying for a doctorate in power engineering at Northeastern University. His tutors are Associate Professor Yuan Shuang, Professor Li Guojian and Professor Wang Qiang. His current research focuses on high entropy catalysts for global water decomposition, as well as density functional theory and machine learning. |
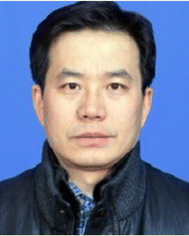 Qiang Wang | Qiang Wang joined Northeastern University as a professor in 2001. He obtained his Ph.D. Degree in Material processing engineering from Nagoya University (Japan) in 2001. His interests mainly focus on functional materials for energy conversion and magnetic material sciences. |
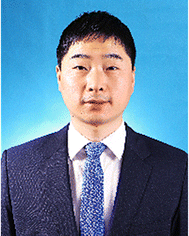 Shuang Yuan | Shuang Yuan received his PhD degree in Materials science from Jilin University and CIAC in 2015 (under the supervision of Prof. Xinbo Zhang). He joined Northeastern University as a Post-doctoral researcher in 2016, and was promoted to associate professor in 2018. His interests mainly focus on functional inorganic materials for batteries, supercapacitors and electrochemical catalysis. |
1. Introduction
In recent years, with the rapid development of modern industrial technology, the demand for fossil fuels in many new industries has been increasing year by year.1,2 As a non-renewable resource, the reserves of fossil fuels are decreasing dramatically, and their depletion rate has far exceeded the natural regeneration cycle. At the same time, fossil fuels commonly contain elements such as nitrogen and sulfur, which react with oxygen under certain conditions to produce acid gases such as NO2, SO2, and SO3, causing serious pollution to the environment.3,4 To solve these thorny problems, renewable energy sources such as solar, wind, and geothermal energy are utilized to generate electricity directly.5–7 These energy sources can effectively reduce the dependence on fossil energy. Unfortunately, this type of energy is affected by factors such as season, climate, temperature difference, and geographical location.8,9 In addition, the electric energy generated by them will produce a large amount of energy consumption in the transmission process through transmission and distribution lines, resulting in a waste of resources.10,11 It is exciting to convert water into clean energy carriers (H2) and high value-added products (O2) through electrocatalytic technology driven by renewable energy sources.12,13
Currently, precious metals such as Pt, Ru, and Ir are often used as electrocatalytic materials.14–16 However, their large-scale applications in the field of water electrolysis have been greatly restricted due to their high price and low storage capacity.17 Despite the progress made in non-precious metal electrocatalysts, it is still not sufficient for industrial applications as well as global commercialization.18,19 In recent years, the rapid development of density functional theory (DFT) has deepened the understanding of electrocatalytic reaction mechanism.20 Through calculation and simulation, researchers can predict the key parameters such as structure, electronic properties and reactivity of materials before the experiment, which provides powerful guidance for experimental design.21 This predictive ability not only greatly shortens the catalyst development cycle, but also reduces the experimental cost and improves the research and development efficiency. However, relying solely on theoretical calculation for catalyst design also has some limitations. On the one hand, theoretical calculations are usually based on a series of simplifications and assumptions, which may lead to inaccurate prediction results.22 On the other hand, the performance of catalysts is often affected by many complex factors, and the interaction and synergistic effects among these factors are difficult to be fully described by a single theoretical calculation.23 Therefore, in the process of catalyst design, theoretical calculation and experimental verification should complement each other.24 It has been shown that there is a strong link between the electrocatalytic activity and the adsorption energy of a series of intermediates (such as *OH, *OO, *CO, *OCO, etc.).25 Therefore, if the charge distribution density of the catalytic sites can be skillfully regulated to optimize the adsorption energy between the catalyst and surface molecules, the catalytic activity of the catalyst will be significantly enhanced.26,27 Numerous studies have demonstrated that the synergistic interaction between multiple components can not only modulate the electronic structure but also optimize the adsorption energy of intermediates.28,29
High entropy is a core concept in thermodynamics, which is used to describe the disorder degree of a system. The higher the disorder degree, the higher the entropy value, and the closer the system is to the “chaotic” state. In recent times, HEM has been recognized as strong candidates for electrocatalysts due to their high entropy effect, slow diffusion effect, lattice distortion, and cocktail effect.30 In terms of economy, precious metals (Pt, Ru, Ir) are still the main catalysts for water decomposition, but the high cost and scarcity limit their large-scale development.31,32 The multi-principal components of high entropy catalysts can not only ensure the catalytic performance, but also reduce the use of precious metals.33,34 In terms of stability, high entropy catalysts have the characteristics of multi-component “random” distribution and highly disordered structure because of their high configuration entropy.35 This highly disordered structure enables the high entropy catalyst to resist corrosion and oxidation during water electrolysis, thus maintaining its chemical and electrochemical stability. In terms of environmental impact, high entropy catalysts are usually based on non-noble metal elements, such as Fe, Co, Ni and other transition metals, which are relatively rich and environmentally friendly.36 In addition, HEM electrocatalysts are unique in their highly disordered structure and random distribution of multiple elements, a property that triggers more defects, vacancies, and ligand-unsaturated sites on the catalyst surface.37,38 This structural diversity allows HEM catalysts to expose more usable active centers than single-element catalysts, thus driving the hydrolysis reaction more efficiently.39,40 Therefore, the concept of high entropy provides a new and unique perspective to deepen the understanding of HER and OER reaction mechanisms.
In this paper, we comprehensively and systematically summarize the research progress of HEM in electrocatalysis in recent years, including HER, OER, and water splitting. First, an overview of HEM for water electrolysis is presented, including types, synthesis strategies, electrochemical reaction mechanisms, evaluation of catalyst indicators and characterization means. Next, the application of HEM for HER, OER, and water splitting is described. Subsequently, a rational design for improving HEM catalysts is proposed. Here, the importance of, in particular, DFT, high-throughput screening techniques, and machine learning for the discovery and design of HEM catalysts is emphasized. Finally, the opportunities and challenges facing HEM catalysts in this field are envisioned.
2. Overview of HEM for water electrolysis
2.1 Material types
2.1.1 High-entropy alloys.
HEA are special alloys consisting of a mixture of five or more metal elements in equal or approximately equal proportions with unique physical and chemical properties (Fig. 1a).41 There are two ways to define high entropy alloy. The first definition is that the atomic ratio of each element is 5 to 35 at%.42 Another definition uses a mixed configuration entropy (ΔSmix) greater than 1.5 R.43 HEA are used as catalysts in the electrolysis of water for the production of hydrogen and oxygen, which significantly improves the efficiency of electrolysis and reduces energy consumption. Their high conductivity enables faster electron transfer and higher reaction rates during water electrolysis.44,45 In addition, the corrosion resistance and stability of HEA enable them to maintain excellent catalytic performance in long-time operations and complex environments. SEM (Scanning Electron Microscope) diagram shows that the HEA with multi-stage pore structure provides an efficient mass transfer channel and reaction site for electrolysis of water due to its multi-scale pore structure and high specific surface area (Fig. 1b).46
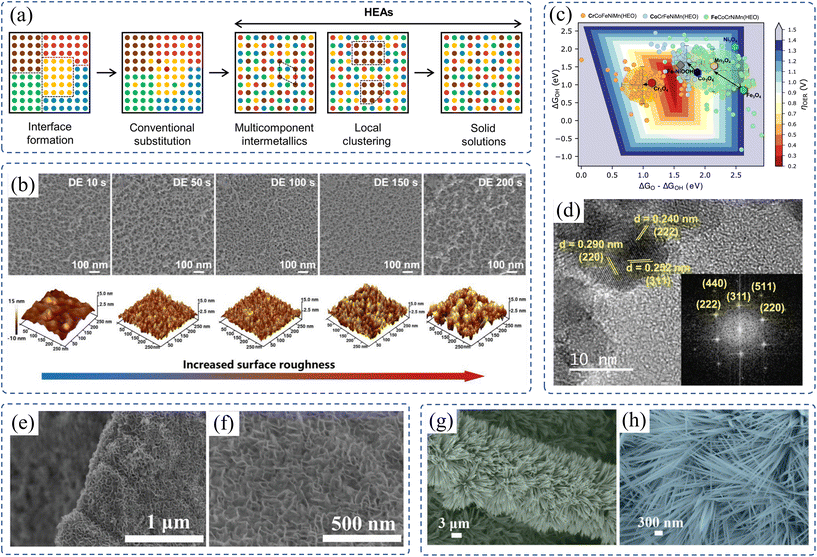 |
| Fig. 1 (a) Schematic diagram of high entropy alloy synthesis.41 (b) SEM and AFM Images of Porous High Entropy Alloy.46 (c and d) Volcanic maps and TEM images of HEO.50 (e and f) SEM images of Ag@CoCuFeAgMoOOH.52 (g and h) SEM images of CoZnCdCuMnS@CF.58 | |
2.1.2 High-entropy metal oxides.
HEO, as an emerging material system, expand the concept of HEA to the field of metal oxides, showing unique structural and performance characteristics, especially in electrolytic water catalysis shows great potential.47 HEO are characterized by their multi-element composition and complex crystal structures. Compared with the traditional single metal oxides, HEO form a more complex oxide system by introducing multiple metal elements.48 The synergistic effect between the elements of this system can effectively promote the catalytic reaction process.49 In addition, HEO also have high specific surface area and porosity, which is favorable for mass transfer and adsorption of reactants in the process of water electrolysis (Fig. 1c and d).50
2.1.3 High entropy metal hydroxides.
High-entropy metal hydroxides contain a variety of metal elements and exist as hydroxides, forming a complex chemical structure.51 This multifaceted mixture enables high-entropy metal hydroxides to provide abundant active sites in catalytic reactions, thus enhancing their catalytic performance (Fig. 1e and f).52 Meanwhile, during water electrolysis, its surface is rich in hydroxyl groups, which can further enhance its water adsorption and activation capacity.53 In addition, the diversity of metal elements brings rich electronic structure, which is favorable for electron transfer and reaction.54
2.1.4 Other types of high-entropy materials.
It should be noted that although some progress has been made in the research of HEA, HEO, and high-entropy hydroxides, the field of HEM is still full of unknowns and challenges. With further research and continuous technological advances, more novel high-entropy catalysts are being developed, including transition metal-based non-oxide inorganic compounds (carbides, fluorides, nitrides, phosphides, and sulfides) and MoF analogs (Fig. 1g and h).55–58
2.2 Synthesis strategies
2.2.1 Top-down.
In the synthesis of catalysts, the top-down synthesis strategy usually starts from existing materials or compounds that are physically or chemically treated to form high-entropy catalysts. Such typical methods include high-energy ball milling, dealloying, arc melting, and Pulsed laser ablation.59–62 In these methods, powders, pellets, or blocks are usually used as feedstocks, which are then converted into high-entropy catalysts by thermal treatment, synthetic reactions, or mechanical mixing.
High-energy ball milling is a preparation technique that utilizes mechanical force to strongly impact, grind, and agitate the HEM, and the raw material powder is refined to the nanoscale by the rotation or vibration of the ball mill.63,64 As shown in Fig. 2a, Mao et al.65 successfully synthesized high-entropy Cs(Pb1/3Mn1/3Ni1/3)Br3 perovskite nanocrystals using CsBr, PbBr2, MnBr2·4H2O and NiBr2·xH2O as raw materials. Zhang et al.66 prepared nanoporous NiCuMnCoFe HEA by the high-energy ball milling method, which exhibited excellent durability and better activity than most of the other reported HEA catalysts.
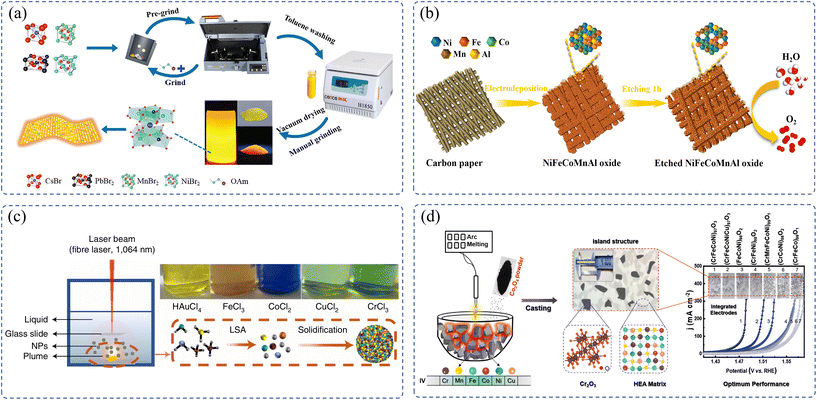 |
| Fig. 2 (a) Process flow for preparation of Cs(PbxMnyNi1−x−y)Br3 NCs by high energy ball milling method.65 (b) Schematic illustration of the synthetic procedures for nanoporous NiFeCoMnAl oxide grown on carbon paper.69 (c) The synthesis of AuFeCoCuCr NPs.72 (d) Atomic-level design and the preparation process of O-HEA electrode.77 | |
The dealloying method is mainly based on the differences in electrochemical properties between different elements in the alloys, and selectively removes the more active metal components of the alloys through chemical or electrochemical corrosion processes, leaving behind the more stable components.67 In addition, during the dealloying process, the original uniform alloy structure was transformed into a porous structure.68 As shown in Fig. 2b, Han et al.69 formed amorphous NiFeCoMnAl high-entropy oxides by a dealloying process, which allows the formation of nanoporous structures with abundant defects. The optimized NiFeCoMnAl high-entropy oxide exhibited an ultra-low oxygen precipitation overpotential of only 190 mV when the current density was 10 mA cm−2. Jin et al.70 used the dealloying method to homogeneously mix Ir (≈20 at%) with four other different metal elements into a single nanostructure.
Pulsed laser ablation of high entropy alloy is an advanced material synthesis and surface treatment technology. This method initiates rapid heating, evaporation and phase transformation of materials by focusing high-energy pulsed laser beam on the surface or target of high entropy alloy, thus generating nano-scale high entropy alloy particles or coatings.71 Wang et al.72 synthesized AuFeCoCuCr nanoparticles by laser ablation at room temperature and conventional atmospheric pressure in only 5 nanoseconds (Fig. 2c). The ultra-fast process can ensure the combination of different metals which are thermodynamically incompatible. When AuFeCoCuCr nanoparticles were prepared as electrocatalysts for water decomposition, the OER overpotential was only 185 mV at 10 mA cm−2, and its performance was better than that of noble metal catalysts. Waag et al.73 synthesized CoCrFeMnNi nanoparticles with ultra-small size (<5 nm) by ablating high entropy target with picosecond pulsed laser. Electrochemical performance tests show that CoCrFeMnNi nanoparticles exhibit excellent OER activity.
The arc melting method is applied to the preparation of HEM mainly to utilize the high-temperature environment of the method to fully mix and reach the molten state of multiple metal elements, thus realizing the precise preparation of HEA.74 The composition and structure of the alloy can be effectively controlled by the arc melting method to optimize the HEM properties.75 He et al.76 prepared CoCrxCuFeMnNiV HEA by the arc melting method and evaluated the effect of different Cr contents on their mechanical properties. As shown in Fig. 2d, Chen et al.77 successfully prepared a series of oxygen micro-alloyed high-entropy alloys (O-HEA) such as (CrMnFeCoNiO, CrFeCoNiCuO, CrFeCoNiO, FeCoNiO, CrFeNiO, CrCoNiO, and CrFeCoO). The results show that (CrFeCoNi)97O3 has the lowest precipitated oxygen overpotential in alkaline solutions.
2.2.2 Bottom-up.
Bottom-up synthesis usually starts from a single atom, molecule, or ion and forms high-entropy catalysts through stepwise assembly or chemical reactions.78,79 This approach usually involves techniques such as atomic layer deposition, sol–gel method, vapor phase deposition, and magnetron sputtering which allows for precise control of catalyst components and structure.80–82
According to the laws of thermodynamics, temperature is a key factor in promoting the homogeneous mixing of multiple elements. However, even if homogeneous mixing of elements is achieved at high temperatures, prolonged cooling can lead to phase separation. To avoid this situation, a combination of high-temperature synthesis technology and a rapid quenching process is needed, which ensures that the elements remain homogeneously mixed during the cooling process.83
The high-temperature carbon thermal shock (CTS) technique, with its transient heating characteristics, provides a controllable and efficient synthesis method.84 Yao et al.85 used this method to prepare high-entropy alloy nanoparticles of up to eight different metal elements. Since CTS requires conductive support, the Joule heating efficiency depends on the mass of carbon, making it difficult to heat uniformly along a large area. As shown in Fig. 3a, Cha et al.86 proposed a method compatible with ambient air, capable of large-area synthesis and remote control. The method successfully modified HEA nanoparticles of up to nine elements by inducing transient high-temperature annealing of carbon nanofibers through a single flash irradiation.
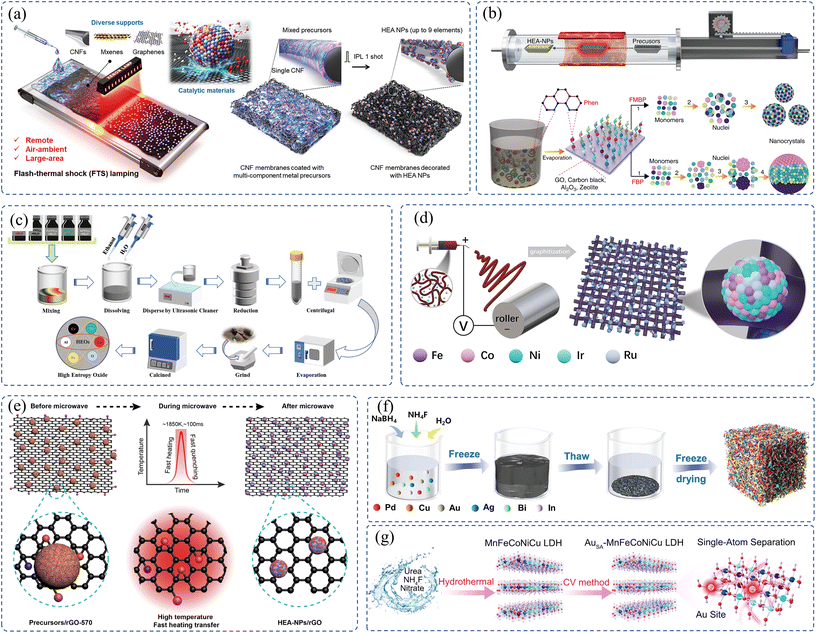 |
| Fig. 3 (a) Schematic illustration of the noncontact, mass-productive, and ambient-air synthetic method of HEA-NPs through the millisecond-scale (<20 ms) light pulse irradiations generated by a xenon lamp.86 (b) Schematic diagrams for synthesis of homogeneous and phase-separated HEA-NPs by FMBP and FBP strategies.89 (c) Synthesis process of the HEO catalysts.92 (d) Typical synthesis procedure for the FeCoNiIrRu/CNFs by combining the electrospinning and graphitization process.98 (e) Schematic illustration of the formation of HEA-NPs on rGO by microwave heating.104 (f) Schematic illustration of the preparation of PdCuAuAgBiIn HEAAs.105 (g) Synthesis schematic of AuSA-MnFeCoNiCu LDH.106 | |
Due to the high activity of high-entropy nanoparticles, it is often difficult for them to be stably anchored to the carrier surface during growth, which can lead to an inhomogeneous distribution of particle size.87 This instability not only affects the activity of the catalyst but may also hurt the selectivity of the catalytic reaction.88 Gao et al.89 innovatively proposed a fast-moving-bed pyrolysis (FMEP) based method to realize graphene oxide (GO) loaded MnCoNiCuRhPdSnIrPtAu HEA-NPs. This method was developed by controlling the rapid heating and cooling (rapidly reaching 923 K in 5 s with an advance rate of 20 cm s−1), which not only improved the homogeneity of the HEA-NPs but also effectively regulated the size of the nanoparticles (50 nm). The FMBP strategy can synthesize small-sized and uniformly distributed HEA-NPs efficiently, rapidly, stably, and scalably (Fig. 3b).
The application of hydrothermal and solvothermal methods makes the preparation process of HEA more flexible and controllable. The composition and structure of the alloys can be precisely controlled by adjusting the parameters such as the type and concentration of metal salts and the reaction temperature to optimize the properties of the HEA.90,91 As shown in Fig. 3c, Wang et al.92 configured a mixture of Co
:
Cr
:
Fe
:
Ni
:
Al = 1
:
1
:
1
:
1
:
1
:
1
:
1 molar ratio, which was transferred to a 100 mL hydrothermal kettle and subjected to a water bath at 180 °C for 24 h. When the reaction was completed, washing, centrifugation, drying, as well as calcination resulted in the formation of a (CoCrFeNiAl)3O4 spinel-type high-entropy Oxide. The (CoCrFeNiAl)3O4 nanoparticles prepared by this method were well dispersed and small in size. Similarly, Wang et al.93 prepared (FeCoNi2CrMn)3O4 HEO with different ratios of components by the solvent-thermal method. The results of these studies show that the high-entropy catalysts prepared by the wet chemical method have the superior advantages of high homogeneity and small size.
The electrostatic spinning method uses a polymer solution in a strong electric field for jet spinning, in which the polymer fluid is electrostatically atomized into tiny jets through the action of electric field force, and finally solidified into fibers.94,95 The nanofiber structure prepared by this method not only increases the specific surface area of the material, which makes the HEM have more active sites in the catalytic reaction. At the same time, it also has excellent stability, which makes the HEM maintain good performance under extreme conditions.96 Tian et al.97 prepared (Mg0.2Mn0.2Ni0.2Co0.2Zn0.2)Fe2O4 with a high specific surface area by using this method. As shown in Fig. 3d, Zhu et al.98 dissolved Fe, Co, Ni, Ir, and Ru salts in polyvinylpyrrolidone (PVP) polymer solution to obtain a homogeneous precursor solution. The precursor solution was then converted into FeCoNiIrRu HEA nanoparticles by electrostatic spinning technique. The catalyst exhibited excellent OER stability in acidic media.
In addition to the above methods for the preparation of HEM, there are other novel methods such as iso-microwave-assisted synthesis, aerogel, electrodeposition, ultrasound, co-precipitation, and plasma vapor deposition.99–103 Conventional thermal synthesis techniques often require high temperatures and long reaction times, but microwave-assisted synthesis techniques can rapidly generate a large amount of energy for the sample and efficiently convert the energy required for the sample during compression into heat, thus greatly reducing the reaction time. Qiao et al.104 and others used this method to synthesize particle-size-consistent PtPdFeCoNi on reduced graphene oxide (RGO) HEA-NPs (Fig. 3e). The main reason for choosing RGO is that its large number of functional group defects can effectively absorb microwave energy and can reach high temperatures of 1850 K in a short time. The aerogel method is the condensation of gas molecules into solid materials by chemical or physical reaction. This material is characterized by high specific surface area, low density, and excellent adsorption properties. As shown in Fig. 3f, Liu et al.105 designed and developed PdCuAuAgBiIn with a unique three-dimensional structure using the aerogel method, and the test results showed that HEA had a high specific surface area (137.906 m2 g−1) and pore volume (0.677 cm3 g−1). Compared with other preparation methods, the electrodeposition preparation method has the advantages of simple synthesis and low cost, and this method can be utilized to prepare HEM on a large scale. As shown in Fig. 3g, Wang et al.106 used an electrodeposition strategy to prepare AuSA-MnFeCoNiCu LDH high-entropy layered hydroxides modified with Au single atoms and oxygen vacancies.
2.3 Electrochemical reaction mechanisms
2.3.1 Water splitting mechanism.
Currently, there are four main water splitting technologies, as shown in Fig. 4a. One is the alkaline water electrolyzers (AWE) with alkaline electrolyte KOH as the electrolyte, which uses Ni-based materials as electrodes, and it is the most widely used and most maturely developed electrolyzer in the industrialized and scaled hydrogen production.107 Proton exchange membrane water electrolyzers (PEMWE) technology transfers protons by utilizing a proton exchange membrane to obtain a large operating current density (2 A cm−2), a high-efficiency energy conversion rate (80–90%), and a purer product hydrogen (99.99%).108 In recent years, anion exchange membrane water electrolyzers (AEMWE) and solid oxide electrolysis cells (SOEC) are two new technologies for water electrolysis.109,110
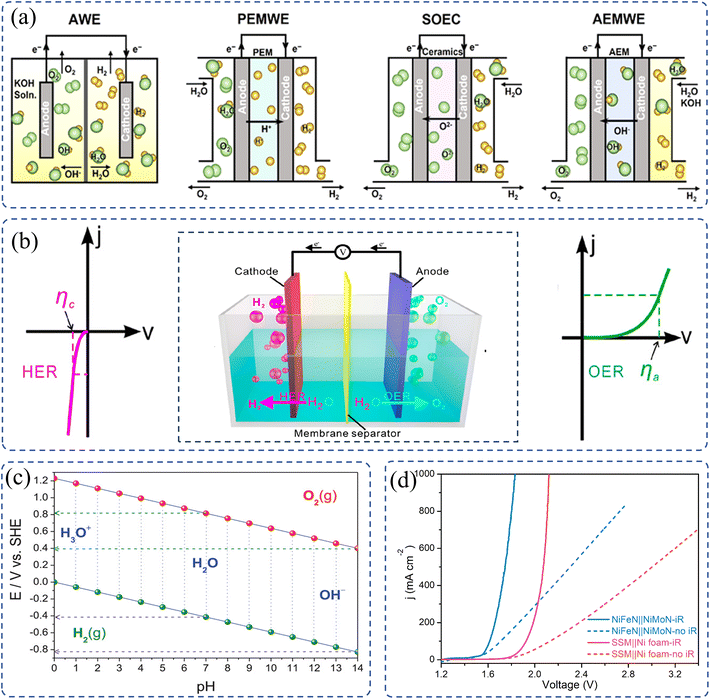 |
| Fig. 4 (a) Schematic illustrations of AWE, PEMWE, SOEC, and AEMWE.107 (b) Hydrogen evolution reaction and oxygen evolution reaction of electrocatalytic water splitting.111 (c) Pourbaix diagram of water showing the pH dependence of OER and HER.113 (d) Polarization curves of different catalysts for overall water splitting in 1 M KOH with and without iR compensation.114 | |
An electrolyzer consists of three main parts, including a cathode, an anode, and an electrolyte (acidic or alkaline). As shown in Fig. 4b, during water electrolysis, the cathode undergoes HER to yield high-purity hydrogen and the anode undergoes OER to yield high-purity oxygen.111 The total water electrolysis reaction is the same whether the electrolysis is carried out under acidic or alkaline electrolytes, producing hydrogen and oxygen.112
In alkaline electrolytes,
Cathodes:
| 2H2O + 2e− → H2 + 2OH− | (2) |
Anodic:
| 2OH− − 2e− → 1/2O2 + H2O | (3) |
In acidic electrolytes,
Cathodes:
Anodic:
| 2H2O − 2e− → 1/2O2 + 2H+ | (5) |
According to the above electrochemical reaction equation, the theoretical thermodynamic potential for water splitting in the standard state is 1.23 V. Unfortunately, in reality, water splitting is also affected by other factors. For example, the pH of the solution (Fig. 4c), solution resistance (Fig. 4d), electrochemical polarization, solution ion diffusion, catalyst contact resistance, and electrolyzer equipment loss, etc., so that the actual electrolytic water potential is higher than the theoretical thermodynamic potential of 1.23 V.113–116
2.3.2 HER mechanism.
As shown in Fig. 5a and b, the chemical reaction mechanisms of hydrogen precipitation catalysts in acidic or alkaline electrolytes are different because of the difference in electrolyte pH.117 The catalyst undergoes charge transfer on the cathode surface according to the Volmer–Heyrovsky or Volmer–Tafel reaction mechanism in either acidic or alkaline solutions.118,119
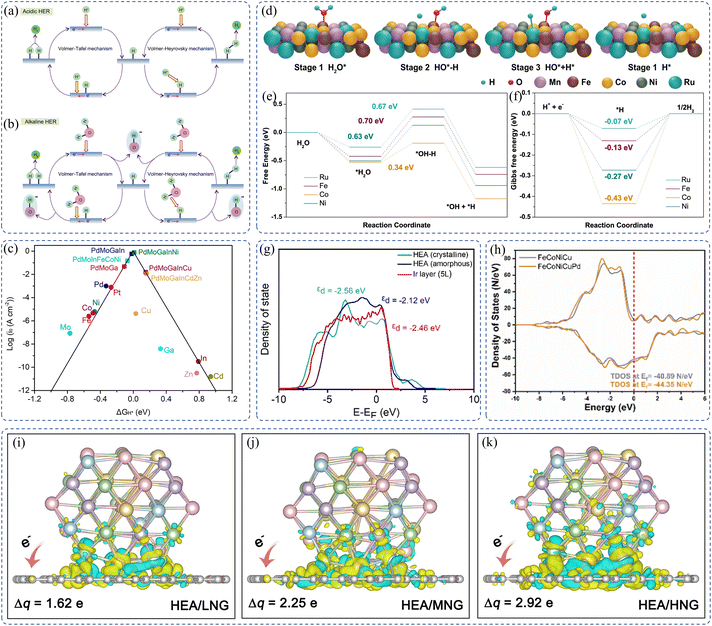 |
| Fig. 5 (a and b) HER pathways in the acidic and alkaline media.117 (c) Computational prediction of desirable composition for HER electrocatalyst.120 (d) The atomic configurations on catalytic sites of FeCoNiMnRu HEA at the four stages during H2O dissociation, (e) reaction energy profile for water dissociation on various catalytic sites of the FeCoNiMnRu HEA surface, (f) Gibbs free energy (ΔGH*) profiles on various catalytic sites of the FeCoNiMnRu HEA surface.126 (g) d-band of amorphous HEA, crystalline HEA, and Ir layer (5L).127 (h) TDOS of FeCoNiCu and FeCoNiCuPd.130 (i–k) Differential charge density distributions (the yellow and blue regions denote 0.003 e Å−3 electron accumulation and depletion, respectively).133 | |
HER in alkaline electrolyte,
| H2O + e− + M → M–Had + OH− | (6) |
| H2O + e− + M–Hads → H2 + OH− | (7) |
HER in acidic electrolyte,
| H3O+ + e− + M → M–Hads | (9) |
| H3O+ + e− + M–Hads → H2 + H2O | (10) |
In the reaction process of alkaline or acidic electrolytes, M denotes the active sites on the catalyst surface, and M–Hads denotes the H atoms adsorbed on the active sites. It can be clearly known from the above HER reaction steps that hydrogen atoms need to undergo a complex adsorption and desorption process on the surface of the catalyst, so the M–Hads between can neither be too weak nor too strong. When the binding energy is too weak, it is difficult to activate the hydrogen atoms on the surface of the catalyst to react with the metal active sites, so the Volmer step will limit the overall reaction rate. When the binding energy is too strong, the M–Hads formed on the catalyst surface are again difficult to dissociate, resulting in the blocking of the active site, and thus the Heyrovsky or Tafel step will limit reaction rate. Therefore, suitable binding energy is conducive to the enhancement of the hydrogen precipitation ability of the catalyst. As shown in Fig. 5c, the hydrogen precipitation performance of the catalyst can be evaluated by the Gibbs free energy of hydrogen (ΔGH*), and the closer the value of ΔGH* is to 0 eV, the closer the hydrogen precipitation activity of the catalyst is to the top of the volcano curve, which achieves comparable catalytic ability with that of the noble metals such as Pt, Ru and Rh.120–122
Based on the above discussion, the volcano curves demonstrate the relationship between the semi-quantitative relationship between the HER reaction rate and ΔGH*, which is closely related to the corresponding electronic structure of the catalyst surface. In addition, the free energy, total density of states (TDOS), d-band centers, and differential charge densities provide more insight into the synergistic interactions between the HEM multicomponents as well as the electronic structure.123–125 As shown in Fig. 5d–f, hydrolysis dissociation is the potential determining step (PDS) during the HER reaction of FeCoNiMnRu. The results show that the potential barrier (0.34 eV) needed to be overcome at the Co site is the lowest in the hydrolysis process, so the Co site is more favorable to accelerate hydrolysis to form intermediate (*HO–H).126 The d-band center of HEA (amorphous) (−2.12 eV) is further away from the Fermi energy level than the d-band centers of HEA (crystalline) and Ir layer (5L), as shown in Fig. 5g. d-Band center theory suggests that the adsorption strength decreases when the d-band center decreases. Conversely, when the d-band center rises, the adsorption strength increases.127 Therefore, the HER activity of HEM can be effectively controlled by adjusting the position of the d-band center.128,129 As shown in Fig. 5h, the calculated TDOS of FeCoNiCu and FeCoNiCuPd alloys are greater than 0 at the Femi energy level, which proves the metallic nature of the HEA samples. The TDOS of FeCoNiCuPd is greater than that of FeCoNiCu, which suggests that it has a higher electron transfer rate.130 Differential charge density maps are often used to reveal the movement and redistribution of charges during electrochemical reactions.131,132 As shown in Fig. 5i–k, the charge redistribution near the interface becomes more pronounced with increasing pyridine N content, and HEA and N-doped graphene exhibit increased electron loss and accumulation regions, respectively. The HEA/HNG system exhibits a maximum charge transfer of 2.29 e, which suggests that the strongly electronegative pyridine N induces the transfer of electrons from HEA to N-doped graphene via the M-pyridine N–C bonds.133
2.3.3 OER mechanism.
OER has a more complex four-electron transfer process, so the reaction kinetics is slower, and a higher overpotential is needed to overcome the reaction energy barrier.134–136 Therefore, OER is usually considered the main cause of lower catalyst efficiency and the decisive speed step for electrolytic hydrogen production in water.137,138 Due to the complexity and multiplicity of the OER, there is no clear conclusion about the reaction mechanism of OER. The adsorbate evolution mechanism (AEM) and lattice oxygen-mediated mechanism (LOM) are shown in Fig. 6a, of which the AEM is the OER mechanism generally recognized by academia at present.139 The corresponding reaction mechanisms are summarized below:
OER in alkaline electrolyte,
| H2O + * → OH* + (H+ + e−) | (12) |
| O* + H2O → OOH* + (H+ + e−) | (14) |
| OOH* → * + O2 + (H+ + e−) | (15) |
OER in acidic electrolyte,
| OH* + OH− → O* + H2O + e− | (17) |
| OOH* + OH− → * + O2 + H2O + e− | (19) |
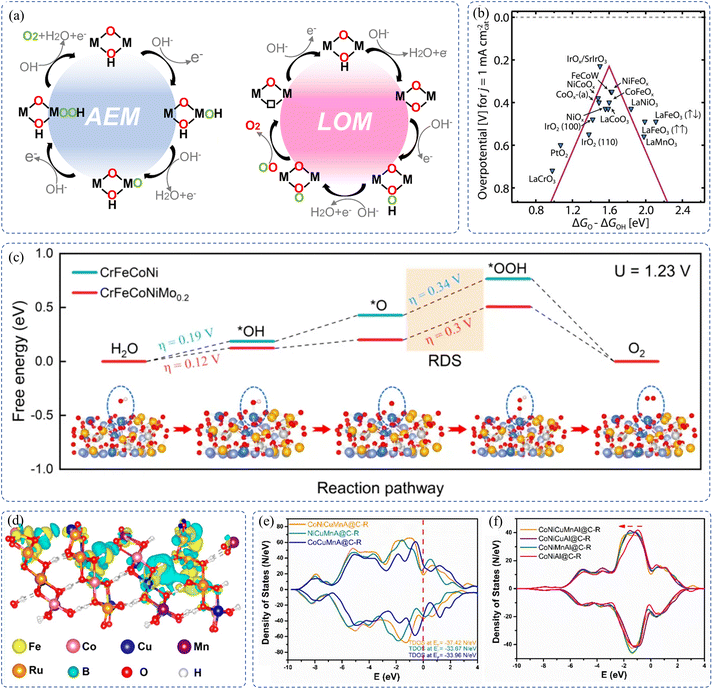 |
| Fig. 6 (a) Adsorbate evolution mechanism (AEM) and oxygen oxidation mechanism (LOM) on MnFeCoNiCuOOH.139 (b) Computational prediction of desirable composition for HER electrocatalyst.140 (c) Gibbs free energy diagram along the reaction pathway on the CrFeCoNi and CrFeCoNiMo0.2 models.141 (d) Differential charge density distributions of FeCoCuMnRuBOOH (yellow clouds, electron accumulation; cyan clouds, electron loss).142 (e and f) TDOS and d-band of CoNiCuMnAl@C-R, NiCuMnAl@C-R, and CoCuMnAl@C-R.143 | |
The active sites on the OER electrocatalyst are denoted by *, and the corresponding adsorbed intermediate species are OH*, O*, and OOH*. The OER process is complex and variable and involves the conversion of multiple intermediates. Combined with theoretical calculations, we obtained the difference between ΔG*O − ΔG*OH and constructed a volcano diagram (Fig. 6b) from this difference and the OER overpotential.140 This volcano diagram clearly shows that a moderate intermediate binding energy is essential to enhance the OER activity of HEM. Specifically, when the binding energy between the electrode surface and the intermediates is too strong, the intermediates will be firmly adsorbed on the electrode surface, thus preventing them from participating in the next reaction. On the contrary, if the binding energy is too weak, further oxidation of OH* will be limited, which will likewise hinder the reaction. The reaction path energy barrier diagrams for CrFeCoNi and CrFeCoNiMo are given in Fig. 6c.141 The results show that the reaction path from *O to *OOH is the rate-determining step. The potential barrier of CrFeCoNiMo decreases from 0.34 to 0.3 eV compared to CrFeCoNiMo, which suggests that the introduction of Mo optimizes the local coordination environment of the Ni site and reduces the reaction barrier of OER. In addition, the differential charge density map of FeCoCuMnRuB shows that electrons cluster around the B atom, which acts as an electron trap to receive off-domain electrons from neighboring metal sites (Fig. 6d).142 As shown in Fig. 6e and f, the TDOS of CoNiCuMnA at the Fermi energy level is larger than that of CoCuMnA and NiCuMnA, which implies that the electron rate of CoNiCuMnA is enhanced during the reaction process.143 Meanwhile, the PDOS can be used to better understand the localized electronic environment. The d-band center positions of the Cu and Mn-containing samples occupy deeper positions away from the Fermi energy levels, showing electron-rich properties. Therefore, the Cu and Mn sites can be used as electron reservoirs to accelerate electron transfer.
2.4 Performance evaluation index
Electrolytic water performance evaluation parameters are important for assessing the catalytic activity of HEM. They mainly include overpotential (η), Tafel slope, electrochemically active area, impedance, turnover frequency (TOF), and stability.144–146
2.4.1 Overpotential.
Any electrochemical reaction is limited by thermodynamics and kinetics and will not reach a theoretical equilibrium state. In the standard state, the theoretical thermodynamic potential for water splitting is 1.23 V.147 Unfortunately, the actual electrolyzed water potential is higher than the theoretical thermodynamic potential of 1.23 V. To overcome the above reaction limitations, an applied potential is needed to facilitate the reaction, which is called an overpotential. In water splitting, there are three types of overpotentials: electrochemical overpotentials, concentration difference overpotentials, and system impedance overpotentials. The electrochemical overpotential is determined by the nature of the material itself. Concentration overpotentials, on the other hand, are caused by the concentration difference between the catalyst surface and the electrolyte due to slow ion transport. The system impedance overpotential is caused by the impedance present in the test system.148
2.4.2 Tafel slope.
Tafel slope reveals the relationship between potential and current density. Tafel slope is inversely proportional to the number of charge transfers, therefore, Tafel slope can be considered as an indicator of the charge transfer capacity.149 When the Tafel slope is large, the charge transfer capacity is poor. When the Tafel slope is small, the charge transfer capacity is good.150
2.4.3 Electrochemically active area.
Electrochemical active specific surface area (ECSA) is a concept widely used to estimate the effective catalytically active specific surface area of a working electrode and is based on the principle of electrochemical double-layer capacitance (Cdl).151 The larger the electrochemically active specific surface area, the more effective catalytic reaction sites are provided by the catalyst.
2.4.4 Impedance.
Electrochemical impedance spectroscopy (EIS) is a non-destructive test method used to explore the kinetics of electrochemical interfacial reactions and catalytic properties.152 The technique reveals characteristics of catalytic reactions and adsorption phenomena at the electrode surface by applying an AC potential wave and recording the impedance of the electrolytic substance as a function of frequency. During the test, by applying a constant potential, the diameter of the semicircle in the high-frequency region of the impedance spectrum is observed as the charge transfer resistance (Rct). In general, a smaller value of Rct indicates a faster catalytic reaction rate and a smaller overpotential.153
2.4.5 Turnover frequency.
The evaluation of the catalytic activity of catalysts is mainly based on the comparison of the magnitude of overpotential at the same current density. However, the mass and exposed surface area of catalytic loading may be different in different reactions, so it is not possible to accurately evaluate the catalytic activity of the catalyst. To solve this problem, research scholars proposed the concept of turnover frequency (TOF), which is the number of transformations of a single active site per unit of time, as a way to evaluate the reaction rate and intrinsic activity of a catalyst.154 In water electrolysis reaction, the higher the TOF value of the catalyst, the faster the reaction rate of the catalyst, so its catalytic activity is better.155
2.4.6 Stability.
Cyclic voltammetry (CV) is a periodic electrochemical test method, which is based on the principle of applying a series of potential waveforms over a certain potential range, and obtaining information on the catalytic reaction mechanism and electrocatalytic performance by observing the change of current with potential.156 By continuous CV cycling, the changes of LSV curves before and after CV cycling are compared, and the smaller the change, the better the stability of the catalytic material. The timed potential method or timed current method is used to obtain the electrochemical stability of catalysts by measuring the change of potential or current with time under fixed potential or current conditions.157
2.5 Characterization techniques
Improving the catalytic performance and lifetime of HEM requires a more comprehensive understanding of the intrinsic properties of HEM as well as the electrochemical reaction process. Several characterizations can be used to evaluate the intrinsic properties of HEM, such as electron microscopy (SEM), transmission electron microscopy (TEM), selected area electron diffraction (SAED), Energy Dispersive Spectroscopy (EDS), Energy Dispersive X-ray Spectroscopy (EDX), X-ray Diffraction (XRD), X-ray Photoemission Spectroscopy (XPS), Inductively Coupled Plasma Mass Spectrometry (ICP), Ultraviolet–Visible Absorption Spectrometry (UV-vis), Infrared Absorption Spectroscopy (FT-iR), Specific Surface Area Analyzer (BET), Vibrating Specimen Magnetometer (VSM), Near-Edge X-ray Absorption Fine Structure (XANES), and Extended X-ray Absorption Fine Structure (EXAFS). Unfortunately, HEM is prone to drastic compositional and structural changes when operated for long periods and at high currents density, which requires the use of in situ characterization techniques to study electrochemical reaction processes and observe compositional and structural changes of materials on an atomic scale. Commonly used in situ characterization techniques include in situ X-ray absorption spectroscopy (XAS), and in situ transmission electron microscopy (TEM).
2.5.1 Conventional characterization.
To adapt to the rapid development of materials science, researchers have developed many devices for exploring the morphology, structure, content, vacancy defects, coordination, specific surface area, and magnetic properties of nanomaterials.158–160 As shown in Fig. 7a–c, the morphology and structure of HEM catalysts can be realized by various means, including SEM, TEM, and HAADF-STEM.161,162 Meanwhile, XRD and SAED are used to determine the crystal structure of HEM catalysts.163,164 The compositional content of HEM catalysts is usually determined using ICP-MS and ICP-OES.165 Atom Probe Tomography (APT) not only provides the 3D structure of the internal material but also examines the elemental content down to the ppm level (Fig. 7d–f).166 BET can test the specific surface area, total pore volume, pore size distribution, and adsorption and desorption curves of HEM catalysts (Fig. 7g).105 VSM is used to characterize the saturation magnetization intensity, coercivity, and remanent magnetization of HEM catalysts (Fig. 7h).167 In addition, EDS and EDX are used to determine the distribution of elements contained in the HEM catalysts.168 The electronic structure of HEM catalysts is studied in detail with the help of XPS (Fig. 7i).169 It should be particularly emphasized that XANES analysis not only provides information about the valence states of the elements in the material but also offers a more in-depth view of its electronic structure (Fig. 7j–l).126 Meanwhile, EXAFS is commonly used to probe chemical bonding and local coordination environments.
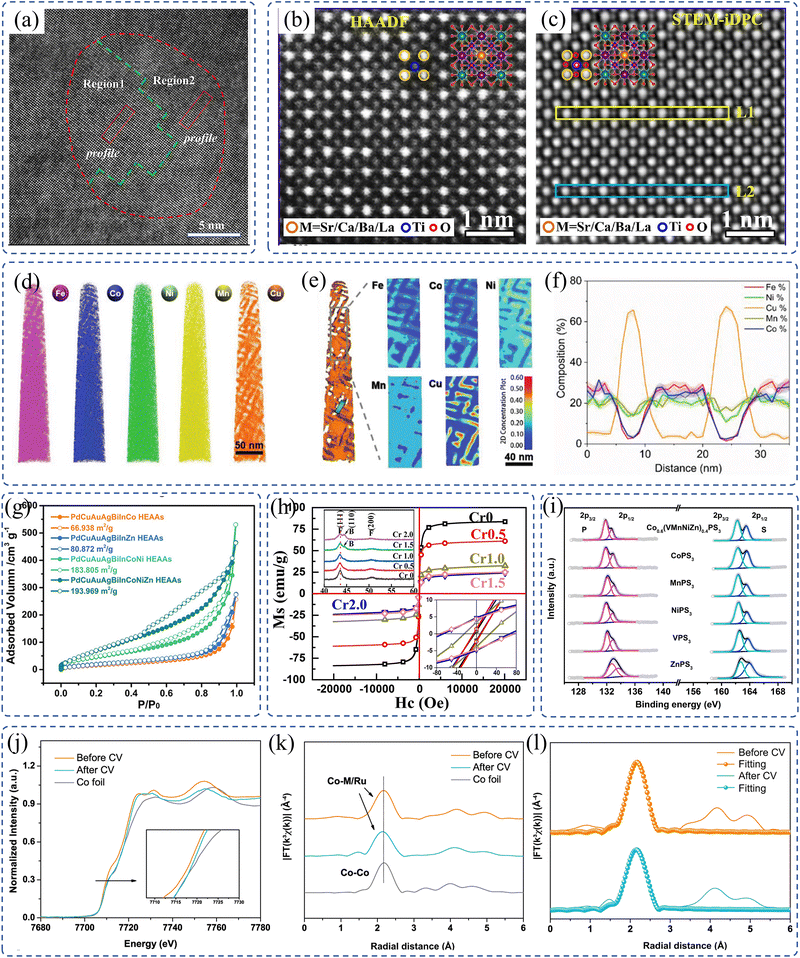 |
| Fig. 7 (a) HR-TEM results showing the structural evolution of the nanoparticles in the FCC phase.161 (b and c) HAADF and iDPC-STEM images and atomic resolution EDS results of (Sr0.25Ca0.25La0.25Ba0.25)0.9TiO3±δ ceramics.162 (d) APT tip reconstructions of Fe, Ni, Co, Mn, and Cu, (e) 50 at% iso-concentration surfaces of Cu and in-plane compositional analysis of the identical sample region marked on the iso-concentration surfaces, (f) 1D compositional profiles taken along the length direction of the cylinder presented in the 50 at% iso-composition surfaces of Cu.166 (g) Adsorption/desorption isotherms of sepetenary PdCuAuAgBiInCo HEAAs, sepetenary PdCuAuAgBiInZn HEAAs, octonary PdCuAuAgBiInCoNi HEAAs, and novenary PdCuAuAgBiInCoNiZn HEAAs.105 (h) Magnetic hysteresis loop of the HEAs.167 (i) P 2p and S 2p XPS spectra of Co0.6(VMnNiZn)0.4PS3, CoPS3, MnPS3, NiPS3, VPS3, and ZnPS3 NSs.169 (j) The Co K-edge XANES spectra and (k) FT-EXAFS spectra of FeCoNiMnRu/CNFs before and after stability test, (l) the corresponding FT-EXAFS fitting curves of FeCoNiMnRu/CNFs before and after stability test.126 | |
2.5.2 Advanced characterization.
In situ differential electrochemical mass spectrometry (DEMS) enables real-time monitoring and analysis of the gases or volatiles produced during electrochemical reactions by connecting an electrochemical device to a mass spectrometer.170 The high sensitivity of this technique allows for the detection of very small amounts of products, providing direct evidence for understanding electron transfer and material transformations during the reaction. When studying electrochemical reactions such as the electrolysis of water and reduction of carbon dioxide, DEMS can reveal exactly which gaseous products are generated under which conditions, thus helping to optimize reaction conditions and catalyst design (Fig. 8a).171In situ electrochemical transmission electron microscopy (EC-TEM) enables direct observation of the structural changes of catalysts during electrochemical reactions at the nanoscale, such as changes in particle morphology, and crystal phase transformations.172 This direct observation provides extremely valuable information for understanding the catalytic reaction mechanism and catalyst activity (Fig. 8b). In situ electrochemical nuclear magnetic resonance (EC-NMR) combines the advantages of electrochemical experiments with nuclear magnetic resonance (NMR) techniques to provide structural information about reactants, intermediates, and products at the molecular level.173 Particularly for liquid reaction systems, EC-NMR can reveal changes in species in the liquid phase and their interactions with solid-state electrode surfaces, thus providing insights into electrochemical reaction processes and catalytic mechanisms (Fig. 8c). Hard X-ray photoelectron spectroscopy (HAXPES) provides deeper information about the chemical state and electronic structure of a material at the surface as well as in the near-surface region.174 Compared to conventional XPS, HAXPES has a deeper depth of analysis because of the use of higher-energy X-rays, and can detect changes in chemical and electronic information beneath the surface of a catalyst or electrode material (Fig. 8d). This provides critical insight into understanding the behavior and stability of catalysts under reaction conditions. In situ XAS is a key in situ technique that enables real-time analysis, revealing the elemental type, chemical state, and structural changes of the material.175 In addition, by analyzing the absorption fringes and peak shapes of XAS, information about the structure of the material can be obtained, supporting the understanding of the structural evolution of the catalyst and interactions with reaction intermediates (Fig. 8e). The combined use of these techniques can provide a comprehensive view to understand and optimize electrochemical reactions and catalytic processes from the macroscopic to the microscopic, from the electronic to the atomic. Through this multidimensional analysis, researchers can more systematically reveal reaction mechanisms and optimize catalyst design, thereby advancing the development of energy conversion and storage technologies.
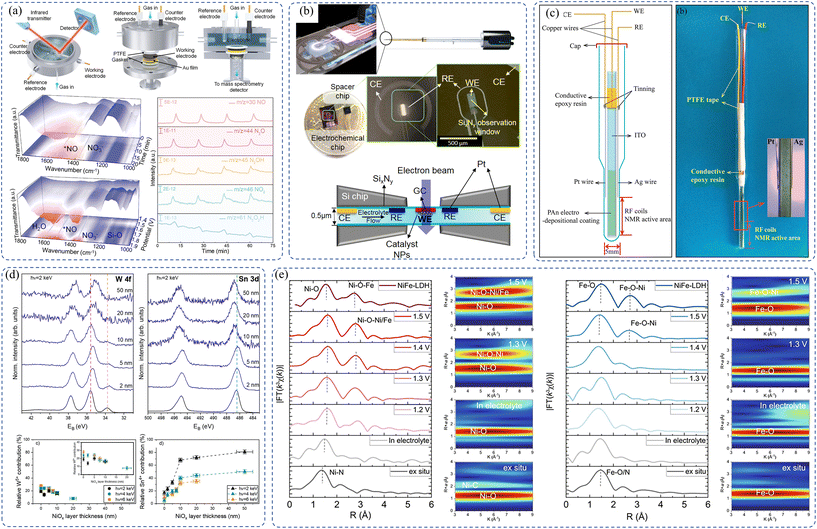 |
| Fig. 8 (a) Schematic diagrams for the devices of electrochemical operando FTIR and online DEMS measurements.171 (b) In situ liquid electrochemical Poseidon TEM holder from Protochips (the inset shows the tip of the holder where the miniaturized electrochemical cell is integrated).172 (c) Electrochemical cell designed for in situ EC-NMR.173 (d) W 4f and Sn 3d core level spectra measured with hν = 2 keV for a pristine α-SnWO4 film (black) and films coated with increasing NiOx layer thickness (blue).174 (e) Ni, Fe K-edge. XANES and FT-EXAFS spectra of NiFe-CNG under different applied potentials, and the corresponding XANES and FT-EXAFS spectra of their reference samples.175 | |
3. Application of HEM
The development of catalysts with high activity and stability is the key to breakthroughs in clean energy conversion technologies. Although this task is extremely challenging, novel HEM-based catalysts have received increasing attention in recent years due to the excellent performance they exhibit in catalytic reactions.176,177 These catalysts not only overcame the limitations of traditional catalysts but also achieved significant improvements in catalytic efficiency and stability.178 To deeply explore the potential of high-entropy catalysts in the field of clean energy conversion, several important catalytic conversion reactions are particularly selected for discussion in this paper, including HER, OER, and water splitting.
3.1 Application of HEM in HER
HEM shows great promise for HER applications. The synergistic effect of alloying these metal elements provides a promising strategy for the development of inexpensive and efficient HER catalysts.179 During the HER process, high-entropy mixing effects and disordered atomic structure endow HEM with unique properties such as increased lattice distortion, slow diffusion rate, and phase stability.180 These properties enable HEM to exhibit ultra-high corrosion resistance in acidic and alkaline electrolytes, which further strengthens their application in HER.181 Liu et al.182 prepared noble metal high-entropy nanoparticles, PtAuPdRhRu, by using an ultrasound-assisted wet-chemical method. The results of electrochemical measurements show that at the current density of 30 mA cm−2 in 1 M KOH, the overpotential is 190 mV and the Tafel slope is 62 mV dec−1 (Fig. 9a and b). The excellent catalytic performance was mainly attributed to the synergistic effect between the noble metals. However, the expensive cost as well as the scarcity of noble metals have limited their scale-up applications.183 In a recent study, Fan et al.184 to reduce the content of precious metals in the catalyst, first synthesized high entropy oxides ((FeCoNiCrAl)3O4) by sol–gel method, and then loaded highly dispersed Pt nanoparticles onto HEO substrates using impregnation method. As shown in Fig. 9c, Pt-(FeCoNiCrAl)3O4 required only 22 mV in 1 M KOH at the current density of 10 mA cm−2. The high-entropy compound FeCoNiAlTi prepared by dealloying method by Jia et al.185 exhibited excellent electrocatalytic activity in alkaline solution (Fig. 9d). In addition, PtCoNiRuIr/C synthesized by Cui et al.186via high-temperature liquid impingement method showed excellent activity in acidic medium with an overpotential of only 18 mV at 10 mA cm−2. Impressively, it displayed smaller semicircles in the low-frequency range, indicating a faster electron transfer rate (Fig. 9e). Stability is the most critical index for the industrial application of catalysts.187,188 Zhou et al.189 prepared HEA with a porous structure by etching the microstructure. Subsequently, a surface improvement strategy for noble metal-doped layered hydroxides was proposed. Undoubtedly, Fig. 9f–h show that the LDH-PHEA catalysts can still maintain stable catalytic activity at high current density. Combining the above studies, Table 1 summarizes some typical HEM catalysts for HER.68,127,130,181,184–186,190–204,235,239
 |
| Fig. 9 (a) Polarization curves, and (b) Tafel plots of HEA-NPs/carbon, PtAuPdRh/carbon, PtAuPd/carbon, and commercial Pt/C (Pt loading = 20 wt%) catalysts in 1.0 M KOH.182 (c) Polarization curves of 0.6-Pt/HEO.184 (d) Comparison of overpotentials at 10 mA cm−2versus Tafel slopes for ≈60 different catalysts in 1.0 M KOH.185 (e) EIS curves of electrocatalysts at −0.02 V.186 (f) Stability testing via the amperometric i–t technique, (g) polarization curves of NiAlPt-LDH@PHEA for the durability test after 2000 CV cycles, (h) Cdl obtained by CV curves at specific scan rates.189 | |
Table 1 Electrocatalytic activity of several typical high entropy materials for HER
Catalysts |
Electrolyte |
J (mA cm−2) |
η (mV) |
Tafel plot (mV dec−1) |
Ref. |
PtAuPdRhRu |
1 M KOH |
30 |
190 |
62 |
181
|
Pt/(FeCoNiCrAl)3O4 |
1 M KOH |
10 |
22 |
25.9 |
184
|
FeCoNiAlTi |
1 M KOH |
10 |
88.2 |
40.1 |
185
|
FeAlCrMoV/MoS2/MoP |
1 M KOH |
10 |
148 |
71.98 |
190
|
NiCoFeMnCrP |
1 M KOH |
10 |
220 |
94.5 |
191
|
CoCrFeNiMo |
1 M KOH |
10 |
156.7 |
46.09 |
192
|
FeCoNiWMo |
1 M KOH |
10 |
35 |
31 |
193
|
H-FeCoNiCuMo |
1 M KOH |
10 |
7 |
19.4 |
194
|
HEA/JMPA |
1 M KOH |
10 |
85 |
59 |
195
|
FeCoNiCuPd |
1 M KOH |
10 |
29 |
47.2 |
130
|
FeCoNiCrMn@Pt |
1 M KOH |
10 |
13.7 |
30.6 |
196
|
NiCoFeMoMn |
1 M KOH |
10 |
14 |
29 |
197
|
CuCrFeNiCoP |
1 M KOH |
10 |
195 |
118 |
198
|
FeCoNiCuAl2Mn-400 |
1 M KOH |
10 |
9.7 |
57 |
68
|
PdPtCuNiP HEMG |
1 M KOH |
10 |
32 |
37.4 |
238
|
HEA-NPs-(14) |
1 M KOH |
10 |
18 |
30.7 |
199
|
PtCoNiRuIr |
0.5 M H2SO4 |
10 |
18 |
34.2 |
186
|
H-FeCoNiMnW |
0.5 M H2SO4 |
10 |
15 |
31 |
200
|
FeCoNiWMo |
0.5 M H2SO4 |
10 |
85 |
31 |
193
|
IrPdRhMoW/C |
0.5 M H2SO4 |
10 |
15 |
35 |
201
|
(W28Ni24Co24Mo17Ru7)POx/C |
0.5 M H2SO4 |
10 |
40 |
36 |
202
|
RhRuPtPdIr/GC |
0.5 M H2SO4 |
10 |
13 |
14 |
127
|
NiCoFePtRh |
0.5 M H2SO4 |
10 |
27 |
30.1 |
235
|
PdPtCuNiP |
0.5 M H2SO4 |
10 |
62 |
44 |
239
|
CoCrFeNiAl |
0.5 M H2SO4 |
10 |
125 |
39.7 |
203
|
Fe35Co25Ni25P7.5C7.5 |
0.5 M H2SO4 |
10 |
88 |
53 |
204
|
HEA-NPs-(14) |
0.5 M H2SO4 |
10 |
11 |
27.2 |
199
|
3.2 Application of HEM in OER
The OER plays a crucial role in the electrolysis of water.205,206 However, due to its inherent complexity, OER is often accompanied by large overpotentials and slower reaction kinetics, which undoubtedly poses a severe limitation on the efficiency of the energy conversion system.207 Given this situation, it is particularly crucial to develop efficient OER catalysts, which play an indispensable role in enhancing the efficiency of high-purity oxygen production during water electrolysis208 Recently, Hu et al.209 synthesized FeCoNiMnCr HEA-HEO with a heterogeneous structure via an in situ Cr-induced reconstruction strategy. The polarization curves showed an overpotential of 261 mV at a current density of 10 mA cm−2 (Fig. 10a). At the same time, its Tafel slope was 42.2 mV dec−1 (Fig. 10b) and Cdl was 2.83 mF cm−2 (Fig. 10c). To further investigate the electrode reaction kinetics in the catalytic OER process, EIS test was carried out. At an overpotential of 300 mV in 1 M KOH, FeCoNiMnCr HEA-HEO had the lowest Rct of ≈1.81 Ω (Fig. 10d). This may be attributed to the increase in exposed active sites formed on the heterogeneous structure of HEA-HEO and the unusual electronic configuration of the Cr ions. TOF indicates the number of transformations per unit time of a single active site as a means to evaluate the reaction rate and intrinsic activity of the catalyst.210Fig. 10e shows that the TOF of FeCoNiMnCr HEA-HEO is 4.08 times higher than IrO2 at a potential of 300 mV. To further highlight the excellent OER performance of FeCoNiMnCr HEA-HEO, recently reported high-entropy catalysts for OER applications are summarized and compared with overpotentials and Tafel slopes at 10 mA cm−2. The stability of electrocatalysts under high currents is crucial for practical applications (Fig. 10f).211 Tian et al.212 successfully prepared a series of 2D HEOs with abundant active sites by employing a self-sacrificial template approach in combination with a fast Joule heating technique. After up to 20
000 cycling tests on (FeCoNiMoRu)3O4 catalysts, the results showed that the overpotentials only changed by 16 mV and 17 mV at current densities of 10 and 100 mA cm−2, respectively (Fig. 10g). Further, under alkaline conditions, the (FeCoNiMoRu)3O4 catalyst exhibited excellent long-term OER stability at a current density of 500 mA cm−2 and was able to operate stably for up to 100 h with a performance decay of only 11.2% (Fig. 10h). After in-depth analysis, the slow diffusion effect and entropy stabilization effect of HEOs are considered to be the main reasons for significantly improving the durability of HEO materials in electrochemical processes. Combining the above studies, Table 2 summarizes some typical HEM catalysts for OER.130,190,191,194,196–198,200,201,209,212,213–216,241
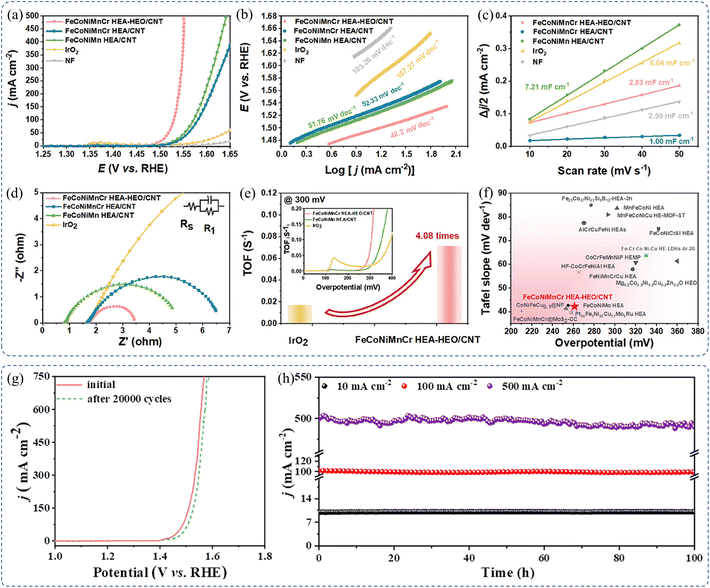 |
| Fig. 10 (a) polarization curves, (b) Tafel plots, (c) Cdl and (d) EIS of FeCoNiMnCr HEA-HEO/CNT, (e) comparison of TOF for the FeCoNiMnCr HEA-HEO/CNT and the commercial IrO2 catalysts at the overpotential of 300 mV, (f) comparison of overpotentials at 10 mA cm−2 and Tafel slopes for the FeCoNiMnCr HEA-HEO/CNT catalyst with the recently reported high-entropy OER electrocatalysts in 1 M KOH.209 (g) Polarization curves for (FeCoNiMoRu)3O4 before and after 20 000 CV cycles, (h) long-term durability test of (FeCoNiMoRu)3O4 at the current density of 10, 100 and 500 mA cm−2.212 | |
Table 2 Electrocatalytic activity of several typical high entropy materials for OER
Catalysts |
Electrolyte |
J (mA cm−2) |
η (mV) |
Tafel plot (mV dec−1) |
Ref. |
FeCoNiMnCr |
1 M KOH |
10 |
261 |
42.2 |
209
|
(FeCoNiMoRu)3O4 |
1 M KOH |
10 |
266 |
40 |
212
|
FeAlCrMoV/MoS2/MoP |
1 M KOH |
10 |
230 |
63.54 |
190
|
NiCoFeMnCrP |
1 M KOH |
10 |
270 |
52.5 |
191
|
H-FeCoNiCuMo |
1 M KOH |
10 |
194 |
43.9 |
194
|
FeCoNiCrMn750 |
1 M KOH |
10 |
294 |
48.6 |
213
|
CoCrFeNiMo-20 Mg |
1 M KOH |
10 |
220 |
59 |
241
|
FeCoNiCuPd |
1 M KOH |
10 |
194 |
39.8 |
130
|
FeCoNiCrMn@Pt |
1 M KOH |
10 |
180 |
64.4 |
196
|
CoFeGaNiZn |
1 M KOH |
10 |
370 |
71 |
214
|
NiCoFeMoMn |
1 M KOH |
10 |
243 |
37 |
197
|
CuCrFeNiCoP |
1 M KOH |
10 |
270 |
70.7 |
198
|
P-HF-CoCrFeNiMo |
1 M KOH |
10 |
220 |
30.3 |
215
|
H-FeCoNiMnW |
0.5 M H2SO4 |
10 |
512 |
31 |
200
|
RuNiMoCrFeOx/CNT |
0.5 M H2SO4 |
10 |
219 |
47 |
216
|
IrPdRhMoW/C |
0.5 M H2SO4 |
10 |
188 |
35 |
201
|
3.3 Application of HEM in water splitting
The overall efficiency of an electrochemical cell directly depends on the efficiency of the water splitting reaction, which includes two key steps, HER and OER.217 Therefore, to improve the overall efficiency of electrochemical cells, there is a need to design a catalyst that can efficiently catalyze both HER and OER processes. However, fabricating such a catalyst faces great challenges because it not only needs to have high activity, and durability, but also be able to drive both reactions at the same time with minimal overpotential.218 Typically, OER catalysts perform well in alkaline environments, while HER catalysts exhibit good stability and activity in acidic environments. This performance difference makes it particularly difficult to achieve both efficient HER and OER in a single catalyst.219 Therefore, there is an urgent need to synthesize a novel bifunctional electrocatalyst that can operate efficiently over a wide pH range. Zhang et al.220 proposed a unique de-alloying strategy to synthesize NiFeCoZn/NiZn-Ni/NF bifunctional electrocatalysts on nickel foam surfaces by electrodeposition, annealing, and etching. The NiFeCoZn/NiZn-Ni/NF constructed electrolyzer drove a current density of 100 mA cm−2 at 1.58 V (Fig. 11a). In addition, the NiFeCoZn/NiZn-Ni/NF||NiFeCoZn/NiZn-Ni/NF exhibited excellent stability compared to commercial electrolyzers, with no significant degradation of the performance when run at 100 mA cm−2 for 400 h (Fig. 11b). Huang et al.221 used a hydrothermal method to prepare the FeCoNiRu-450 catalyst. The required cell voltage for FeCoNiRu-450 was 1.565 V at a current density of 10 mA cm−2 (Fig. 11c). Industrialization is the top priority for the preparation of bifunctional catalysts. In recent years, Cui et al.222 prepared Fe20Co20Ni20Mo20Al20 HEA catalysts. As shown in Fig. 11d, the two-electrode electrolyzer exhibited remarkable stability with negligible potential decay of about 10 and about 40 mV for 120 h at 0.5 and 1 A cm−2. Based on the above studies, many reports have also simultaneously confirmed the great potential of HEM as a bifunctional catalyst for water electrolysis (Fig. 11e and f).223,224 Taken together, Table 3 summarizes some typical HEM catalysts for water splitting.130,190–192,194,196–198,200,201,220–224,225,226
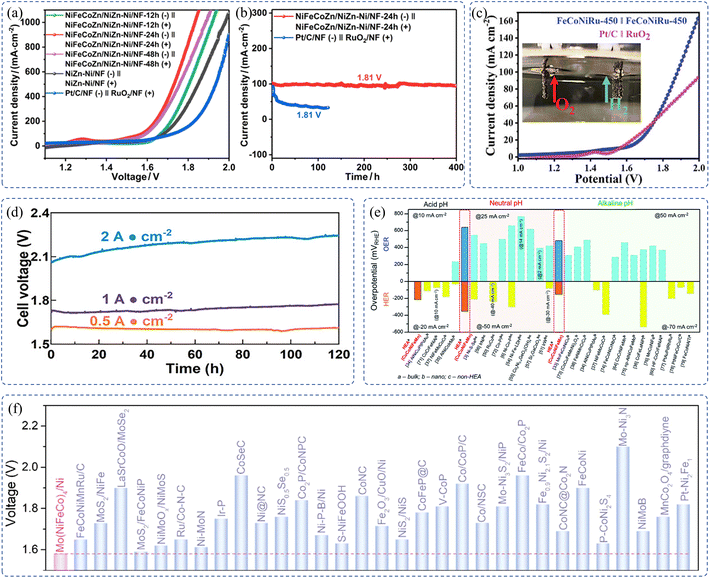 |
| Fig. 11 (a) LSV curves of different electrodes for overall water splitting, (b) chronoamperometric curve of the long-time stability for overall water splitting at a constant potential of 1.81 V.220 (c) Overall water splitting without iR compensation of FeCoNiRu-450||FeCoNiRu-450.221 (d) Long-term stability tests of Fe20Co20Ni20Mo20Al20 electrolyzer was carried out at 0.5, 1, and 2 A cm−2 for around 120 h.222 (e) Comparison of activity (overpotential) of bulk HEA with other reported HEA and non-HEA nanostructured electrocatalysts in acid (0.5 M H2SO4), neutral (1 M PBS) and alkaline (1 M KOH) electrolytes.223 (f) Comparison of working voltage of Mo(NiFeCo)4/Ni||EON-Mo(NiFeCo)4/Ni AWE to reach current density of 100 mA cm−2 with the values of electrolyzers reported previously.224 | |
Table 3 Electrocatalytic activity of several typical high entropy materials for water splitting
Catalysts |
Electrolyte |
J (mA cm−2) |
E (V) |
Ref. |
NiFeCoZn/NiZn||NiFeCoZn/NiZn |
1 M KOH |
100 |
1.58 |
220
|
FeCoNiRu-450||FeCoNiRu-450 |
1 M KOH |
10 |
1.563 |
221
|
Fe20Co20Ni20Mo20Al20||Fe20Co20Ni20Mo20Al20 |
1 M KOH |
1000 |
1.73 |
222
|
CuCoNiFeMn||CuCoNiFeMn |
1 M KOH |
40 |
1.80 |
223
|
Mo(NiFeCo)4/Ni||Mo(NiFeCo)4/Ni |
1 M KOH |
100 |
1.579 |
224
|
FeAlCrMoV/MoS2/MoP||FeAlCrMoV/MoS2/MoP |
1 M KOH |
10 |
1.6 |
190
|
NiCoFeMnCrP||NiCoFeMnCrP |
1 M KOH |
10 |
1.55 |
191
|
CoCrFeNiMo||CoCrFeNiMo |
1 M KOH |
10 |
1.86 |
192
|
PtIrCuNiCr||PtIrCuNiCr |
1 M KOH |
10 |
1.42 |
225
|
H-FeCoNiCuMo||H-FeCoNiCuMo |
1 M KOH |
10 |
1.416 |
194
|
FeCoNiCuPd||FeCoNiCuPd |
1 M KOH |
10 |
1.52 |
130
|
FeCoNiCrMn@Pt||FeCoNiCrMn@Ir |
1 M KOH |
10 |
1.56 |
196
|
NiCoFeMoMn||NiCoFeMoMn |
1 M KOH |
10 |
1.47 |
197
|
CuCrFeNiCoP||CuCrFeNiCoP |
1 M KOH |
10 |
1.69 |
198
|
CoCuFeNiMnMo1.5||CoCuFeNiMnMo0.5 |
1 M KOH |
10 |
1.76 |
226
|
H-FeCoNiMnW||H-FeCoNiMnW |
0.5 M H2SO4 |
10 |
1.76 |
200
|
IrPdRhMoW/C||IrPdRhMoW/C |
0.5 M H2SO4 |
10 |
1.48 |
201
|
4. Optimization strategy
HEM provides a broad platform for the development of high-performance electrocatalysts with its unique elemental composition and abundant active sites.227 Given its diverse elemental combinations, HEM exhibits a large number of potential active sites, which provides a great deal of opportunities for designing novel electrocatalysts.228 Usually, to enhance the electrocatalytic efficiency of HEM, two main focuses have been placed on increasing the number of active sites by optimizing their geometrical effects and enhancing the intrinsic activity of the material by tuning the electronic effects.229,230 In this section, strategies for designing HEM with high performance are outlined, including phase structure modulation, defect engineering, electrode conformation engineering, high-throughput screening, and machine learning.
In addition, high entropy materials are selected in composition. Selection of main elements: main elements are the basis of high entropy electrocatalytic materials, and metal elements with good conductivity and catalytic activity are usually selected. For example, noble metal elements such as platinum and palladium have excellent catalytic performance, but the cost is high; transition metal elements such as nickel and cobalt have relatively low cost and good performance, so they are commonly used as main elements.192,193 Selection of auxiliary elements: auxiliary elements are mainly used to adjust the properties of materials, such as improving catalytic activity and stability. When selecting auxiliary elements, the interaction and compatibility between auxiliary elements and main elements should be fully considered. For example, adding proper amount of rare earth elements can improve the catalytic activity and stability of materials; adding some nonmetallic elements can improve the conductivity and electrochemical properties of the materials.191,198 Optimization of composition ratio: composition ratio is one of the key factors affecting the performance of high entropy electrocatalytic materials. By adjusting the content and proportion of various elements, the properties of materials can be optimized.262
4.1 Phased structural modulation
The correlation between the catalytic performance of HEM and their nanoscale structure has been widely recognized.231 The crystal structure, especially the exposed crystal facets and crystal size, has a significant effect on its catalytic ability.232 Therefore, precise tuning of their nanoscale structures is considered a key strategy to enhance catalytic performance. However, existing synthesis techniques, although capable of preparing HEM, often yield bulk materials with limited surface area ratios.233 This structure limits the practical application of HEM in fields such as electrochemical catalysis. To overcome this limitation, it is important to focus on how to effectively reduce the particle size of HEM and thus significantly increase their specific surface area.234 Feng et al.235 prepared ultra-small NiCoFePtRh HEA nanoparticles of less than 2 nm by chemical co-reduction. Minamihara et al.236 used a continuous flow reactor to synthesize homogeneous 1 nm-sized particles of a very small high-entropy alloy, IrPdPtRhRu (Fig. 12a). The obtained HEA NPs showed excellent HER activity with a very small overpotential of 6 mV at 10 mA cm−2 in the acidic electrolyte (Fig. 12b). In addition, the polarization curves before and after the durability test remained almost identical (Fig. 12c). To increase the number of active sites and thus improve the electrocatalytic activity, the preparation of HEM with different morphologies has been proved to be an effective means, in addition to size reduction.237 Jia et al.238 prepared nanospongy PdPtCuNiP high-entropy metallic glasses using dealloyed (Fig. 12d and e), which provided abundant active sites. As shown in Fig. 12f–j, Zhan et al.239 synthesized PtRuNiCoFeMo HEA nanowires with a unique structure, which exhibited excellent electrocatalytic performance.
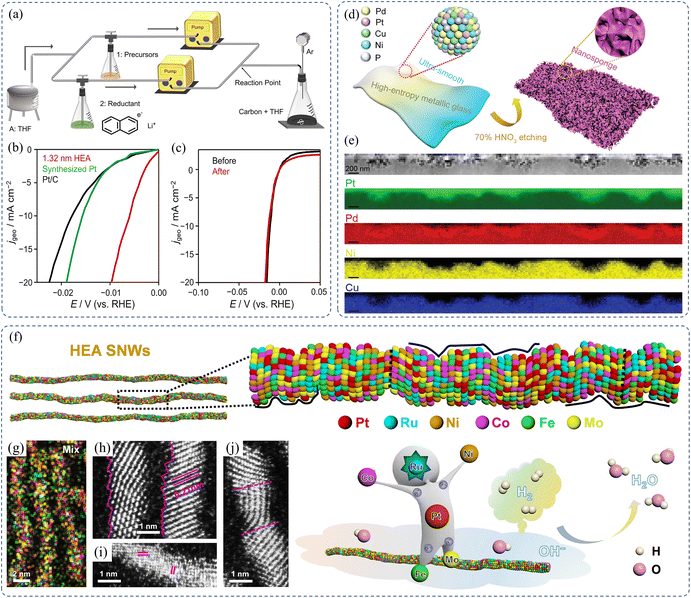 |
| Fig. 12 (a) Schematic of the developed continuous-flow reactor, (b) polarization curves of 1.32 nm HEA NPs, the synthesized Pt NPs, and commercial Pt/C, (c) comparison of the polarization curves before and after the durability test.236 (d) Schematic illustration of the preparation process of the nanosponge-like HEMG, (e) EELS elemental mapping of the nanocrystals and underlying matrix cross-section.237 (f) 3D models and enlarged atomic model of HEA SNWs, (g–i) HRSTEM images.239 | |
4.2 Defect engineering
Defects have a significant impact on improving the water electrolysis performance of HEM.240 These effects start with fine structural changes within the lattice, especially the presence of lattice defects such as dislocations and stacking faults.241 These defects lead to the deformation of the lattice, which changes the physical and chemical properties of the material.242 An important consequence of lattice deformation is the increase in free surface energy. The free surface energy is a key parameter that describes the surface properties of a material and it directly affects the reactivity of the material at the interface. When free surface energy increases, the interaction of the material surface with its surroundings becomes more active. The increase in surface energy further reduces the activation energy during water electrolysis. Activation energy is the minimum amount of energy required for a chemical reaction to occur, and it determines the rate and efficiency of the reaction. By reducing the activation energy, we can accelerate the kinetic process of electrochemical reactions and make the water electrolysis reaction more rapid and efficient.243 It was reported that Huang et al.244 prepared 3D dendritic FeCoNiMnCu HEA (Fig. 13a) by cathodic plasma-driven self-assembly. Surprisingly, a large number of lattice distortions existed on the surface of the structure (Fig. 13b), and the presence of these lattice distortions induced the enhancement of OER performance (Fig. 13c and d). Meanwhile, the team245 used one-step solvothermal and pyrolytic methods to grow nanorod-like MnFeCoNiCu HEA in situ on the carbon cloth surface (Fig. 13e). The TEM and HRTEM results showed (Fig. 13f and g) that many dislocations, including partial dislocations and stacking faults, appeared on the surface of MnFeCoNiCu. In addition to twins and dislocations, atomic displacements leading to the rearrangement of lattice planes were found. The mismatch of atomic sizes in HEA leads to lattice distortion and consequently to strain. This strain affects the arrangement and electronic structure of the surface atoms and enhances the selective adsorption of specific molecules by the alloy. Tensile strain, in particular, brings the d-band center close to the catalyst surface and enhances the reactant–catalyst interaction, thereby improving the catalytic performance.246 He et al.247 achieved the immobilization of a single atom of Mo on the tensile-strained PdPtNiCuZn using a simple one-pot liquid-phase synthesis method (Fig. 13h). EDS mapping of a single atom on the Mo1-PdPtNiCuZn SAHEA NSs with homogeneous distribution of the elements Pd, Pt, Ni, Cu and Zn as well as isolated Mo atoms (Fig. 13i).
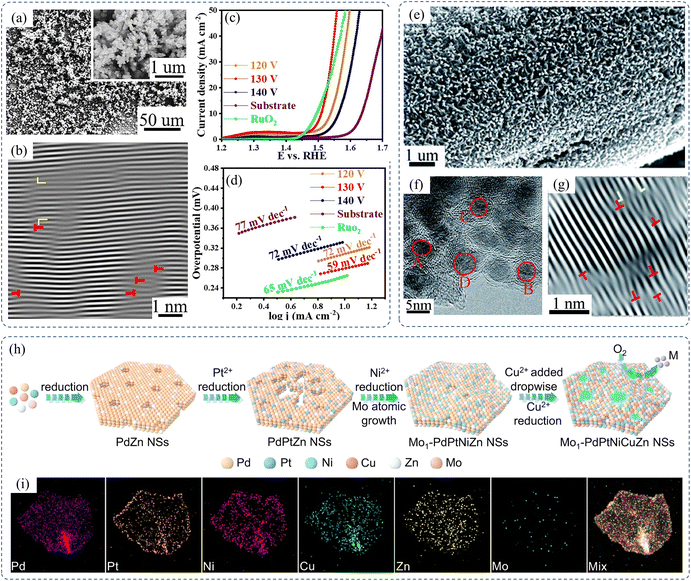 |
| Fig. 13 (a) SEM images of quinary FeCoNiMnCu HEA nanoparticles, (b) the IFFT images transformed along (1 1 1) planes, (c) LSV and (d) Tafel slope of FeCoNiMnCu HEA nanoparticles.244 (e) SEM, (f) TEM, and (g) HRTEM images of HEA nanoparticles.245 (h) Schematic illustration of the formation mechanism of Mo1-PdPtNiCuZn SAHEA NSs, (i) HAADF-STEM-EDS elemental mapping of a single Mo1-PdPtNiCuZn SAHEA NS.247 | |
4.3 Electrode configuration engineering
During electrocatalytic reactions, powder HEM tends to dislodge the catalyst from the electrodes due to their tendency to aggregate and undergo volume expansion, which can lead to poor electrode contact.248 These problems severely limit the catalytic activity and stability of powder HEM. Therefore, to overcome these limitations, HEM grown directly on conductive substrates are considered potential electrocatalyst candidates.249 This is because they not only have enhanced transport kinetics but also achieve low internal resistance due to the direct contact with the collector, thus promising to exhibit superior electrocatalytic performance. Metal-conducting substrates (nickel foam, copper foam, Ti foil, etc.) have been widely used due to their low cost, high catalyst loading capacity, and good electronic conductivity.250–252 Liu et al.253 first electrodeposited Cu(OH)2 nanowires on the surface of copper foam, followed by a hydrothermal reaction, and then finally the precursors were calcined in H2/Ar atmosphere to form CuNiMoWCo HEA (Fig. 14a). The SEM and TEM images show that the CuNiMoWCo HEA exhibits a petal morphology (Fig. 14b and c). A large number of these petals unfolded in an orderly manner with gaps between the layers, which facilitated the mass transfer during the catalytic process. As expected, CuNiMoWCo HEA required only 85.2 mV overpotential to realize a high current density of 100 mA cm−2. As shown in Fig. 14d, Feng et al.254 synthesized FeCoNiMnAl precursors on nickel foam surfaces by a hydrothermal method and formed (FeCoNiMnAl)3O4/NF with a flower-like structure after calcination in air for 2 h. In 1 M KOH test solution, when the current density was 50 mA cm−2, (FeCoNiMnAl)3O4/NF required an overpotential of 274 mV (Fig. 14e). In addition, the Cdl and EIS test results show that (FeCoNiMnAl)3O4/NF not only possesses a large electrochemical area (Fig. 14f), but also a fast electron transfer capability (Fig. 14g). Carbon substrate materials, such as carbon cloth and carbon paper, are known for their lightweight and flexibility, exhibiting different properties from metal substrate materials.255,256 Jo et al.257 prepared CoFeNiMoW HEA on the surface of carbon paper using an electrodeposition strategy, followed by tellurization at a low temperature of 300 °C to obtain CoFeNiMoWTe HEA (Fig. 14h). Surprisingly, as shown by SEM images (Fig. 14i and j), CoFeNiMoW presents nanospherical shape, while CoFeNiMoWTe presents porous nanosheet structure. Undoubtedly, CoFeNiMoWTe exhibited excellent acidic OER catalytic performance at a current density of 10 mA cm−2.
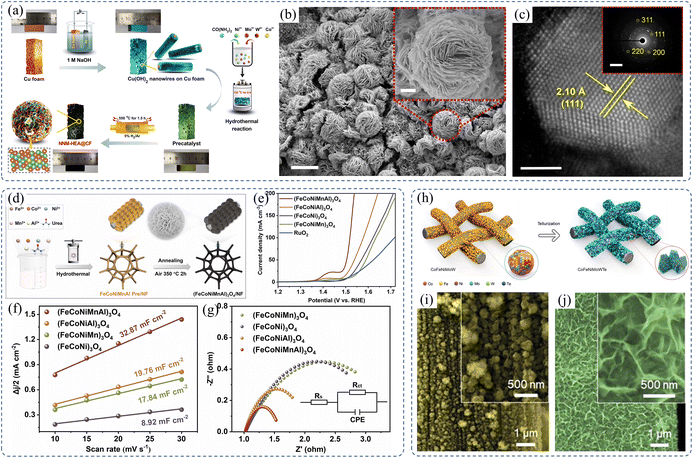 |
| Fig. 14 (a) Schematic illustration of the synthesis of the NNM-HEA@CF catalytic electrode, (b) SEM, and (c) HRTEM images of the NNM-HEA.253 (d) Schematic illustration of the synthesis process for 3D layered microflower-shape (FeCoNiMnAl)3O4 grown on the Ni foam, (e) LSV, (f) Cdl, and (g) EIS of (FeCoNiMnAl)3O4.254 (h) Schematic of the prepared CoFeNiMoW and CoFeNiMoWTe, (i) FE-SEM images of the CoFeNiMoW (inset shows a high-magnification SEM image of the CoFeNiMoW), (j) FE-SEM images of the CoFeNiMoWTe (inset shows a high-magnification SEM image of the CoFeNiMoWTe).257 | |
4.4 High-throughput screening and machine learning
The key to the optimization of the catalytic performance of HEM lies in the precise determination of its optimum components and ratios. Traditionally, experiments have been used as the main approach, and their effectiveness is self-evident. However, for complex systems such as HEM, the traditional “trial-and- error” method is not only inefficient but also time-consuming, which greatly hinders the development and research progress of HEM. To break through this bottleneck, it is necessary to utilize modern technology. Specifically, through in-depth analysis of a large amount of data obtained from experiments and computational simulations, a “high-throughput” approach can be applied, which will greatly facilitate and accelerate the screening and development process of HEM.258,259 At the same time, the compositional space of HEM is extremely vast, which means that the complexity of experimental or theoretical data is extremely high. Fortunately, the booming machine learning methods provide brand new opportunities to cope with such complex systems.260,261 Therefore, the combination of high-throughput screening methods and machine learning techniques is expected to be more effective in solving the problems of component and ratio determination in the optimization of catalytic performance of HEM and to provide a new level for advancing the development and research of HEM. As shown in Fig. 15a, Wu et al.262 proposed a topology-based machine learning algorithm to evaluate the stability and catalytic activity of diatomic catalysts (DACs). To evaluate the stability of the carbon surface-loaded DAC, the binding energy Eb of the DAC was defined, where the more negative the value of Eb, the more stable the DAC was on the carbon surface (Fig. 15b). Fig. 15c plots the overpotentials of the machine learning predicted OER for 16
767 possible DACs, as well as the DFT-calculated overpotentials for 164 random DACs. The results show that the larger the φ value of the DAC, the better the OER catalytic performance of the DAC, which is verified by both the DFT calculation results and the machine learning prediction results. Chun et al.263 decoupled the shape effect (morphology and size) and alloying effect (composition and conformation) to obtain PtFeCu nanoparticles efficiently, as shown in Fig. 15d. In addition, the thermodynamically most stable configurations with different alloy compositions were determined by Monte Carlo simulations (Fig. 15e–j).
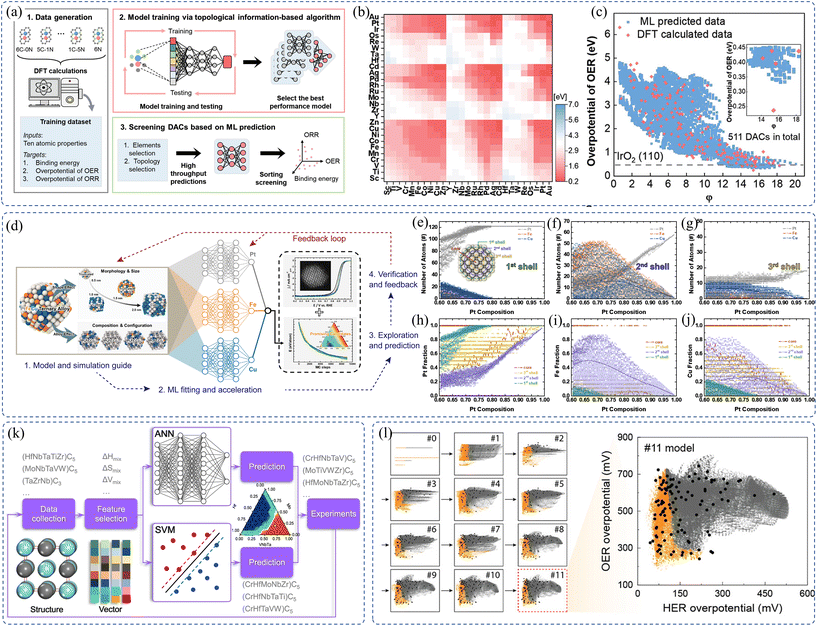 |
| Fig. 15 (a) Illustration of the workflow of the topological information-based ML model to screen DACs with optimal electrocatalytic performance from a vast design space, in three steps: (1) data generation, (2) training/test via a topological information-based algorithm, and (3) screening DACs based on ML prediction, (b) ML predicted binding energy of all DACs on a symmetric defect structure, (c) the overpotential of OER from both DFT calculations and ML predictions as a function of an intrinsic catalytic activity descriptor of φ.262 (d) Schematic diagram of ternary alloy configuration search and theoretical predictions and experimental validation for ORR, (e–j) Configurational spaces of PtFeCu ternary nanoparticles of 2.0 nm size in the range of 0.6 < Pt < 1.263 (k) ANN and SVM refer to artificial neural network and support vector machine.267 (l) Result of the Pareto active learning model for each step and the corresponding data points. The 11th model, which is the final model developed in this study, is plotted with HER and OER overpotential axes.268 | |
Despite these advances, current efforts can only cover, at best, <1% of the available compositions in HEM.264 Therefore, guided optimization and careful sampling are crucial to identify important data points to save exploration efforts. This can be achieved by using active learning methods (Bayesian optimization and reinforcement learning).265,266 These methods can also be combined with HEM surfaces and graph network descriptors for neural networks to accelerate the development of alternative computational models for surface and adsorption properties (Fig. 15k).267 Kim et al.268 explored the optimal water splitting catalyst Pt0.15Pd0.30Ru0.30Cu0.25 based on Pareto-based active learning. As shown in Fig. 15l, the optimal water splitting catalyst Pt0.15Pd0.30Ru0.30Cu0.25 is used for the surface and adsorption properties of the HEM surface and neural networks after the first 11 iterations from the initial database after 11 iterations, 110 experimental points were added from 77
946 predicted points and the Pareto active learning process was terminated. From the initial model to the 7th model, the shape of the model changed substantially as 10 experimental data points were added to the model. In particular, in the early stages of the model, only a few of the discarded points were located at the bottom. However, as the iterations progressed, some of the top-left and bottom-right portions were also categorized as discarded points, suggesting that Pareto active learning guided the categorization of discarded points among all data points well.
5. Summary and outlook
In recent years, significant progress has been made in the research and practical application of HEM in the preparation of stable and efficient electrocatalysts. To deepen the understanding of HEM in the direction of electrochemical water splitting, we comprehensively reviewed the basic concepts and characteristics of HEM, including definition, synthesis strategies, electrochemical reaction mechanisms, electrochemical performance evaluation indexes, and characterization means. In addition, contemporary research advances on HEM as an electrocatalyst for HER, OER, and water splitting are outlined. Finally, design strategies to improve the catalytic performance of HEM, such as phase structure modulation, defect engineering, electrode configuration engineering, high-throughput screening, and machine learning, are proposed. HEM catalysts despite some research advances, it is important to note that HEM catalysts for electrocatalysis are still in the early stages of research. Therefore, there are still some clear obstacles that must be addressed for the development of HEA electrocatalysts.
(1) Developing more emerging strategies to further synthesize HEM with unique morphologies and extremely rich active sites. Currently, most of the methods for preparing HEM catalysts involve high temperatures, rapid quenching, and high energies, whose harsh conditions greatly limit the large-scale production of HEM catalysts. In addition, most of such methods synthesize bulk HEM, which is characterized by limited surface area, small number of exposed surfaces, irregular morphology, and large particle size distribution. Therefore, it is imperative to design new synthesis methods or modify existing ones so that they can be used in mild environments and to produce nanostructured materials with unique morphologies that have considerable specific surface areas, large pore size distributions, and more exposed active sites.
(2) The development of electrocatalysts that can operate stably for long periods at high current densities and in harsh electrolyte environments is critical to advancing the industrial application of water electrolysis. In practical applications, especially under high currents and harsh environments, the stability of the electrocatalyst directly determines the operational efficiency of the water splitting system. However, most of the current durability tests of electrocatalysts are only conducted at current densities lower than 1000 mA cm−2, and the test time is short, making it difficult to meet the demand for long-term stable operation. Therefore, it is necessary to increase efforts to develop electrocatalysts that can operate stably for long periods under such harsh environments, to meet the urgent needs of industrialized applications of water electrolysis.
(3) More advanced characterization techniques are essential to fully analyze the complex structure of HEM catalysts, especially the synergistic interactions between different elements. Spectroscopically, XRD, XAS, and HAXPES are all important techniques for revealing the phase and electronic structures. However, how to effectively decouple them synergistically in these spectra remains unclear. This calls for more advanced characterization techniques such as in situ Fourier transform infrared, in situ Raman spectroscopy, in situ HAADF, in situ STEM, and in situ X-ray absorption spectroscopy (XANE and EXAFS), which provide real-time monitoring of atomic/electronic structural variations and changes in elemental distributions, and offer an important means of determining the relationship between the structure of HEM catalysts and catalytic performance.
(4) The construction of HEM databases through advanced theoretical computational tools, including DFT, molecular dynamics, machine learning, and artificial neural networks, has paved the way for infinite combinations of five or more elements, opening up a new era of unprecedented research. To completely abandon the inefficient “trial-and-error” approach, high-throughput screening and prediction must be resolutely implemented, which is a necessary way to push HEM research forward. Therefore, high-throughput screening techniques and machine learning technologies enable large-scale preparation of HEM specimens to rapidly and accurately reveal their potential properties.
(5) To cope with the increasing energy demand and the deep concern about the greenhouse effect, the applications of HEM in other cutting-edge fields can be extended, including supercapacitors, lithium batteries, sodium batteries, semiconductors, photocatalysis, nitrogen reduction, and carbon dioxide reduction.269–275 The core of these extended applications lies in deeply exploring and fully utilizing the unique geometries and electronic configurations possessed on the surface of HEM, which are capable of activating different functional units and thus exerting their unique efficacy in various fields.
HEM as a test for HER, OER, and water splitting catalysts is still in the exploratory stage for industrial basic applications. Although its full performance has not yet been fully grasped, a large number of preliminary studies have revealed the excellent electrocatalytic potential that HEM possesses. To fully realize this potential and enhance the overall electrocatalytic performance of HEM, it is necessary to continue to explore it in depth and carry out more systematic and comprehensive research work. Therefore, the paper aims to provide an in-depth overview of HEM in electrocatalytic applications, to help researchers better grasp their properties and advantages and provide valuable guidelines and insights for future studies.
Data availability
Data availability is not applicable to this article as no new data were created or analyzed in this study.
Conflicts of interest
The authors declared that they have no conflicts of interest to this work. We declare that we do not have any commercial or associative interest that represents a conflict of interest in connection with the work submitted.
Acknowledgements
This work was financially supported by the National Natural Science Foundation of China (Grant No. 52274294), the Liaoning Province Science and Technology Plan Joint Program (Key Research and Development Program Project) (2023JH2/101800058).
References
- S. Fakudze and J. Q. Chen, Chem. Eng. J., 2023, 457, 141004 CrossRef CAS
.
- K. Kohse-Hoeinghaus, Chem. Rev., 2023, 123, 5139–5219 CrossRef CAS PubMed
.
- Y. D. Zhou, J. Remón, Z. C. Jiang, A. S. Matharu and C. W. Hu, Green Energy Environ., 2023, 8, 722–743 CrossRef CAS
.
- W. Z. Zhang, M. H. Liu, X. Gu, Y. X. Shi, Z. F. Deng and N. S. Cai, Chem. Rev., 2023, 123, 7119–7192 CrossRef CAS
.
- L. T. Liu, R. R. Zhai and Y. D. Hu, Energy, 2023, 276, 127386 CrossRef CAS
.
- H. H. Cho, V. Strezov and T. J. Evans, Sustainable Mater. Technol., 2023, 35, e00567 CAS
.
- M. Farghali, A. I. Osman, I. M. A. Mohamed, Z. H. Chen, L. Chen, I. Ihara, P. S. Yap and D. W. Rooney, Environ. Chem. Lett., 2023, 21, 2003–2039 CrossRef CAS PubMed
.
- X. Ma, W. W. Yang, X. Y. Tang and Y. L. He, J. Cleaner Prod., 2024, 436, 140587 CrossRef CAS
.
- X. T. Xu, J. Feng, W. Y. Li, G. J. Wang, W. Feng and H. F. Yu, Prog. Polym. Sci., 2024, 149, 101782 CAS
.
- J. Hwang, K. Maharjan and H. J. Cho, Int. J. Hydrogen Energy, 2023, 48, 28629–28648 CrossRef CAS
.
- H. M. Teamah, J. Energy Storage, 2024, 81, 110483 CrossRef
.
- X. Xiao, L. J. Yang, W. P. Sun, Y. Chen, H. Yu, K. K. Li, B. H. Jia, L. Zhang and T. Y. Ma, Small, 2022, 18, 2105830 CrossRef CAS PubMed
.
- Y. Li, K. Wang, D. W. Huang, L. T. Li, J. H. Tao, N. A. A. Ghany and F. Jiang, Appl. Catal., B, 2021, 286, 119872 CrossRef CAS
.
- J. Wang, H. Yang, F. Li, L. G. Li, J. B. Wu, S. H. Liu, T. Cheng, Y. Xu, Q. Shao and X. Q. Huang, Sci. Adv., 2022, 8, eabl9271 CAS
.
- T. T. Li, J. Y. Cui, M. X. Xu, K. P. Song, Z. H. Yin, C. Meng, H. Liu and J. J. Wang, Nano Lett., 2024, 24, 958–965 CrossRef CAS PubMed
.
- Y. Qi, B. Y. Zhang, G. H. Zhang, Z. K. Zheng, T. F. Xie, S. S. Chen, G. J. Ma, C. Li, K. Domen and F. X. Zhang, Joule, 2024, 193–203 CrossRef CAS
.
- J. Yang, Y. Shen, Y. M. Sun, J. H. Xian, Y. J. Long and G. Q. Li, Angew. Chem., Int. Ed., 2023, 62, e202302220 CrossRef CAS
.
- S. Yuan, Z. Wang, Y. F. Hao, C. Qi, J. Q. Liu and Q. Wang, J. Solid State Chem., 2023, 317, 123705 CrossRef CAS
.
- S. Dutta, A. Indra, Y. Feng, H. Han and T. Song, Appl. Catal., B, 2019, 241, 521–527 CrossRef CAS
.
- P. Zhang, S. Y. Xu, Z. Y. Li, Z. F. An, H. S. Park and P. J. Yoo, Chem. Eng. J., 2024, 485, 149486 CrossRef CAS
.
- W. B. Xu, E. Diesen, T. W. He, K. Reuter and J. T. Margaf, J. Am. Chem. Soc., 2024, 146, 7698–7707 CrossRef CAS
.
- Y. Wang, J. X. Mi and Z. S. Wu, Chem Catal., 2022, 2, 1624–1656 CrossRef CAS
.
- Y. G. Yao, Q. Dong, A. Brozena, J. Luo, J. W. Miao, M. F. Chi, C. Wang, G. F. Wang, A. Anapolsky and L. B. Hu, Science, 2022, 376, 6589 CrossRef PubMed
.
- W. H. Jing, G. W. He, S. J. Bai, F. Wang, Y. Liu and L. J. Guo, ACS Mater. Lett., 2024, 6, 1347–1355 CrossRef CAS
.
- B. Fang, J. T. Jin, Y. Q. Li, H. F. Dang, M. M. Shao, L. Y. Zhao, N. L. Yin and W. L. Wang, Small, 2024, 2310825 CrossRef CAS
.
- D. L. Wu, B. Liu, R. D. Li, D. Chen, W. H. Zeng, H. Y. Zhao, Y. T. Yao, R. Qin, J. Yu, L. Chen, J. A. Zhang, B. Li and S. C. Mu, Small, 2023, 19, 2300030 CrossRef CAS
.
- J. W. Zhu, J. Q. Chi, T. Cui, L. L. Guo, S. Q. Wu, B. Li, J. P. Lai and L. Wang, Appl. Catal., B, 2023, 328, 122487 CrossRef CAS
.
- Y. A. Yu, G. D. Liu, S. H. Jiang, R. Y. Zhang, H. Q. Deng, E. A. Stach, S. J. Bao, Z. H. Zeng and Y. J. Kang, Nano Res., 2023, 16, 10742–10747 CrossRef CAS
.
- Q. J. Che, Q. Li, X. H. Chen, Y. Tan and X. Xu, Appl. Catal., B, 2020, 263, 118388 CrossRef
.
- D. S. Wu, K. Kusada, Y. Nanba, M. Koyama, T. Yamamoto, T. Toriyama, S. Matsumura, O. Seo, I. Gueye, J. Kim, L. S. R. Kumara, O. Sakata, S. Kawaguchi, Y. Kubota and H. Kitagawa, J. Am. Chem. Soc., 2022, 144, 3365–3369 CrossRef CAS PubMed
.
- L. L. Zhu, C. D. Li, R. B. Zheng, W. P. Cheng, Y. D. He, C. Z. Gong, M. Liu, Y. A. Huang, X. B. Zhu and Y. P. Sun, Int. J. Hydrogen Energy, 2024, 51, 638–647 CrossRef CAS
.
- J. Y. Du, S. Liu, Y. Liu, G. Wu, X. C. Liu, W. J. Zhang, Y. Zhang, X. Hong, Q. W. Li and L. X. Kang, J. Am. Chem. Soc., 2024, 146, 8464–8471 CrossRef CAS PubMed
.
- A. Chatterjee, A. Ghosh, D. Ganguly, R. Sundara and S. S. Bhattacharya, Energy Technol., 2023, 11, 2300490 CrossRef CAS
.
- J. H. Shi, H. S. Jiang, X. L. Hong and J. Tang, Appl. Surf. Sci., 2024, 642, 158598 CAS
.
- H. Wu, J. F. Zhang, Q. Lu, Y. J. Li, R. Jiang, Y. Liu, X. R. Zheng, N. Q. Zhao, J. J. Li, Y. D. Deng and W. B. Hu, ACS Appl. Mater. Interfaces, 2023, 15, 38423–38432 CrossRef CAS
.
- L. Y. Yi, S. M. Xiao, Y. P. Wei, D. Z. Li, R. F. Wang, S. F. Guo and W. H. Hu, Chem. Eng. J., 2024, 469, 144015 CrossRef
.
- S. S. Aamlid, M. Oudah, J. Rottler and A. M. Hallas, J. Am. Chem. Soc., 2023, 145, 5991–6006 CrossRef CAS PubMed
.
- Y. Tang, R. X. Wang, B. Xiao, Z. R. Zhang, S. Li, J. W. Qiao, S. X. Bai, Y. Zhang and P. K. Liaw, Prog. Mater. Sci., 2023, 135, 101090 CrossRef CAS
.
- S. Li, L. K. Tong, Z. J. Peng, B. Zhang and X. L. Fu, Green Chem., 2024, 26, 384–395 RSC
.
- Z. Y. Ding, J. J. Bian, S. Shuang, X. D. Liu, Y. C. Hu, C. W. Sun and Y. Yang, Adv. Sustainable Syst., 2020, 4, 1900105 CrossRef CAS
.
- Y. F. Sun and S. Dai, Sci. Adv., 2021, 7, eabg1600 CrossRef CAS PubMed
.
- Y. G. Yao, Q. Dong, A. Brozena, J. Luo, J. W. Mao, M. F. Chi, C. Wang, I. G. Keverekidis, Z. J. Ren, J. Greeley, G. F. Wang, A. A. Anapolsky and L. G. Hu, Science, 2022, 376, eabn3103 CrossRef CAS PubMed
.
- Y. Y. Zhai, X. G. Ren, B. L. Wang and S. Z. Liu, Adv. Funct. Mater., 2022, 32, 2207536 CrossRef CAS
.
- J. X. Yang, B. H. Dai, C. Y. Chiang, I. C. Chiu, C. W. Pao, S. Y. Lu, I. Y. Tsao, S. T. Lin, C. T. Chiu, J. W. Yeh, P. C. Chang and W. H. Hung, ACS Nano, 2021, 15, 12324–12333 CrossRef CAS PubMed
.
- C. Liu, H. Zhu, S. L. Lu, F. Duan and M. L. Du, New J. Chem., 2021, 45, 22255–22260 RSC
.
- X. Y. Zhang, Y. Y. Yang, Y. J. Liu, Z. Jia, Q. Q. Wang, L. G. Sun, L. C. Zhang, J. J. Kruzic, J. Lu and B. L. Shen, Adv. Mater., 2023, 35, 2303439 CrossRef CAS
.
- S. Q. Zhao, H. Y. Wu, R. Yin, X. N. Wang, H. Z. Zhong, Q. Fu, W. J. Wan, T. Cheng, Y. Shi, G. X. Cai, C. Z. Jiang and F. Ren, J. Alloys Compd., 2021, 868, 159108 CrossRef CAS
.
- P. Edalati, Q. Wang, H. Razavi-Khosroshahi, M. Fuji, T. Ishihara and K. Edalati, J. Mater. Chem. A, 2020, 8, 3814–3821 RSC
.
- Z. Y. Liu, Y. Liu, Y. J. Xu, H. L. Zhang, Z. P. Shao, Z. B. Wang and H. S. Chen, Green Energy Environ., 2023, 8, 1341–1357 CrossRef CAS
.
- J. Baek, M. D. Hossain, P. Mukherjee, J. H. Lee, K. T. Winther, J. Leem, Y. Jiang, W. C. Chueh, M. Bajdich and X. L. Zheng, Nat. Commun., 2023, 14, 5839 CrossRef
.
- H. T. Xu, Y. X. Hu, X. R. Lin, D. Liu, J. F. Liang, X. F. Bian, K. M. Reddy and H. J. Qiu, Appl. Surf. Sci., 2023, 633, 157624 CrossRef CAS
.
- L. J. Zhang, W. W. Cai, N. Z. Bao and H. Yang, Adv. Mater., 2022, 34, 2110511 CrossRef CAS
.
- L. J. Zhang, W. W. Cai and N. Z. Bao, Adv. Mater., 2021, 33, 2100745 CrossRef CAS
.
- T. G. Ritter, A. H. Phakatkar, M. G. Rasul, M. T. Saray, L. V. Sorokina, T. Shokuhfar, J. M. Goncalves and R. Shahbazian-Yassar, Cell Rep. Phys. Sci., 2022, 3, 100847 CrossRef CAS
.
- T. Z. Xiong, X. C. Yao, Z. X. Zhu, R. Xiao, Y. W. Hu, Y. C. Huang, S. Q. Zhang and M. S. Balogun, Small, 2022, 18, 2105331 CrossRef CAS
.
- X. H. Zhao, Z. M. Xue, W. J. Chen, Y. Q. Wang and T. C. Mu, ChemSusChem, 2020, 13, 2038–2042 CrossRef CAS
.
- R. Mohili, N. R. Hemanth, H. E. Jin, K. Lee and N. Chaudhari, J. Mater. Chem. A, 2023, 11, 10463–10472 RSC
.
- Y. T. Lei, L. L. Zhang, W. J. Xu, C. L. Xiong, W. X. Chen, X. Xiang, B. Zhang and H. S. Shang, Nano Res., 2022, 15, 6054–6061 CrossRef CAS
.
- S. W. Xue, H. B. Mao, J. H. Li, X. E. Sun, Z. Y. Gong, B. B. Fan, G. Shao, H. L. Wang, H. L. Xu, R. Zhang and H. X. Lu, Ceram. Int., 2023, 49, 35202–35212 CrossRef CAS
.
- K. S. Ming, L. L. Li, Z. M. Li, X. F. Bi and J. Wang, Sci. Adv., 2019, 5, eaay0639 CrossRef CAS PubMed
.
- Y. L. He, M. Q. Cong, W. N. Lei, Y. H. Ding, T. Xv and Z. L. Han, Mater. Today Adv., 2023, 20, 100443 CrossRef CAS
.
- H. Schwarz, J. Apell, H. K. Wong, P. Henning, R. Wonneberger, N. Rösch, T. Uhlig, F. Ospald, G. Wagner, A. Undisz and T. Seyller, Adv. Mater., 2023, 35, 2301526 CrossRef CAS
.
- R. B. Strozi, K. Sakaki, H. Kim, A. Machida, Y. Nakahira, J. Cizek, O. Melikhova, G. Zepon, W. J. Botta and C. Zlotea, J. Alloys Compd., 2023, 969, 172415 CrossRef CAS
.
- A. Martinez-Garcia, I. Estrada-Guel, E. Reguera, R. Amaro-Hernandez, S. González, C. G. Garay-Reyes and R. Martínez-Sánchez, Int. J. Hydrogen Energy, 2024, 50, 670–684 CrossRef CAS
.
- H. B. Mao, L. Wang, J. H. Li, X. Jiang, S. W. Xue, M. L. Li, J. P. Zhu, B. B. Fan, T. T. Xu, G. Shao, H. L. Xu, H. L. Wang, R. Zhang and H. X. Lu, Part. Part. Syst. Charact., 2022, 39, 2200073 CrossRef CAS
.
- Z. J. Zhang, J. P. Guo, S. H. Sun, Q. Sun, Y. W. Zhao, Y. F. Zhang, Z. Y. Yu, C. S. Li, Y. Sun, M. M. Zhang and Y. Jiang, Rare Met., 2023, 42, 3607–3613 CrossRef CAS
.
- J. W. Ma, X. Wang, B. W. Xing, H. S. Yu, X. J. Zuo and N. N. Zhang, J. Therm. Spray Technol., 2024, 33, 951–963 CrossRef
.
- C. Y. Wang, S. Zhao, G. Q. Han, H. W. Bian, X. R. Zhao, L. N. Wang and G. W. Xie, Small Methods, 2024, 2301691 CrossRef
.
- M. Han, C. H. Wang, J. Zhong, J. R. Han, N. Wang, A. Seifitokaldani, Y. F. Yu, Y. C. Liu, X. H. Sun, A. Vomiero and H. Y. Liang, Appl. Catal., B, 2022, 301, 120764 CrossRef CAS
.
- Z. Y. Jin, J. Lv, H. L. Jia, W. H. Liu, H. L. Li, Z. H. Chen, X. Lin, G. Q. Xie, X. J. Liu, S. H. Sun and H. J. Qiu, Small, 2019, 15, 1904180 CrossRef CAS
.
- R. Ninomiya, D. Kim, N. Takata, S. M. Lyth, K. Ishikawa and Y. Miyajima, Appl. Phys.Lett., 2024, 124, 201903 CrossRef CAS
.
- B. Wang, C. Wang, X. W. Yu, Y. Gao, L. F. Gao, C. P. Wu, Y. F. Yao, Z. Q. Lin and Z. G. Zou, Nat. Synth., 2022, 1, 138–146 CrossRef
.
- F. Waag, Y. Li, A. R. Ziefu, E. Bertin, M. Kamp, V. Duppel, G. Marzun, L. Kienle, S. Barcikowski and B. Gokce, RSC Adv., 2019, 9, 18547–18558 RSC
.
- W. Muftah and V. Vishnyakov, Vacuum, 2021, 188, 110181 CrossRef CAS
.
- W. Tillmann, L. Wojarski, D. Stangier, M. Manka and C. Timmer, Weld. World, 2020, 64, 1597–1604 CrossRef CAS
.
- W. He, C. Zeng, W. Yang, W. H. Chen and Y. L. Ai, J. Mater. Res. Technol., 2022, 21, 4577–4590 CrossRef CAS
.
- Z. J. Chen, T. Zhang, X. Y. Gao, Y. J. Huang, X. H. Qin, Y. F. Wang, K. Zhao, X. Peng, C. Zhang, L. Liu, M. H. Zeng and H. B. Yu, Adv. Mater., 2021, 33, 2101845 CrossRef CAS
.
- Y. Q. Kang, O. Cretu, J. Kikkawa, K. Kimoto, H. Nara, A. S. Nugraha, H. Kawamoto, M. Eguchi, T. Liao, Z. Q. Sun, T. Asahi and Y. Yamauchi, Nat. Commun., 2023, 14, 4182 CAS
.
- X. J. He, Y. Zhang, X. L. Gu, J. W. Wang, J. L. Qi, J. Hao, L. P. Wang, H. Huang, M. Wen, K. Zhang and W. T. Zheng, Nat. Commun., 2023, 14, 775 CrossRef CAS
.
- L. Jing, Y. M. Zou, R. Goei, L. Y. Wang, J. A. Ong, A. Kurkin, Y. Li, K. W. Tan and A. I. Y. Tok, Langmuir, 2023, 39, 3142–3150 CrossRef CAS
.
- B. Niu, F. Zhang, H. Ping, N. Li, J. Y. Zhou, L. W. Lei, J. J. Xie, J. Y. Zhang, W. M. Wang and Z. Y. Fu, Sci. Rep., 2017, 7, 3421 CrossRef
.
- J. Yi, J. X. Zhao, C. H. Liu, J. P. Long, N. Chen, L. J. Fan, H. Q. Liu, L. X. He, J. Wang and H. Chang, Adv. Eng. Mater., 2023, 25, 2300051 CrossRef CAS
.
- A. Abdelhafiz, B. M. Wang, A. R. Harutyunyan and J. Li, Adv. Energy Mater., 2022, 12, 2200742 CrossRef CAS
.
- X. X. Zou, J. Y. Xie, Z. Y. Mei, Q. Jing, X. L. Sheng, C. H. Zhang, Y. X. Yang, M. J. Sun, F. T. Ren, L. L. Wang, T. W. He, Y. C. Kong and H. Guo, Proc. Natl. Acad. Sci. U. S. A., 2024, 121, E2313239121 CrossRef CAS PubMed
.
- Y. G. Yao, Z. N. Huang, P. F. Xie, S. D. Lacey, R. J. Jacob, H. Xie, F. J. Chen, A. M. Nie, T. C. Pu, M. Rehwoldt, D. W. Yu, M. R. Zachariah, C. Wang, R. Shahbazian-Yassar, J. Li and L. B. Hu, Science, 2018, 359, 1489–1494 CrossRef CAS PubMed
.
- J. H. Cha, S. H. Cho, D. H. Kim, D. Jeon, S. Park, J. W. Jung, I. Kim and S. Y. Choi, Adv. Mater., 2023, 35, 2305222 CrossRef CAS PubMed
.
- Y. T. Wang and Y. H. Wang, Nano Energy, 2022, 104, 107958 CAS
.
- W. Q. Wan, K. M. Liang, P. Y. Zhu, P. He and S. Y. Zhang, J. Mater. Sci. Technol., 2024, 178, 226–246 CrossRef CAS
.
- S. J. Gao, S. Y. Hao, Z. N. Huang, Y. F. Yuan, S. Han, L. C. Lei, X. W. Zhang, R. Shahbazian-Yassar and J. Lu, Nat. Commun., 2020, 11, 2016 CrossRef CAS PubMed
.
- T. X. Nguyen, C. C. Tsai, V. Nguyen, Y. J. Huang, Y. H. Su, S. Y. Li, R. K. Xie, Y. J. Lin, J. F. Lee and J. M. Ting, Chem. Eng. J., 2023, 466, 143352 CrossRef CAS
.
- Y. H. Bai, L. Wang, S. Lu, A. X. Zhang, W. Y. Qu, K. He and N. Hu, J. Environ. Chem. Eng., 2023, 11, 110703 CrossRef CAS
.
- C. Wang, W. Liu, M. Z. Liao, J. H. Weng, J. Shen, Y. Chen and Y. P. Du, Nanoscale, 2023, 15, 8619–8632 RSC
.
- D. Wang, C. Q. Duan, H. He, Z. Y. Wang, R. G. Zheng, H. Y. Sun, Y. G. Liu and C. L. Liu, J. Colloid Interface Sci., 2023, 646, 89–97 CrossRef CAS PubMed
.
- S. P. Wang, Q. C. Liu, S. K. Li, F. Z. Huang and H. Zhang, ACS Nano, 2024, 18, 5040–5050 CrossRef CAS
.
- P. C. Zhang, C. H. Zheng, M. X. Li and H. P. Wang, J. Mater. Sci., 2023, 58, 8189–8209 CrossRef CAS
.
- Y. Xing, W. Q. Dan, Y. C. Fan and X. A. Li, J. Mater. Sci. Technol., 2022, 103, 215–220 CrossRef CAS
.
- L. Y. Tian, Z. Zhang, S. Liu, G. R. Li and X. P. Gao, Energy Environ. Mater., 2022, 5, 645–654 CrossRef CAS
.
- H. Zhu, Z. F. Zhu, J. C. Hao, S. H. Sun, S. L. Lu, C. Wang, P. M. Ma, W. F. Dong and M. L. Du, Chem. Eng. J., 2022, 431, 133251 CrossRef CAS
.
- W. W. Cao, X. Y. Yang, W. J. Dai, B. Wu, Y. D. Zhang, C. J. Zhao, Y. W. Sui and S. F. Huang, New J. Chem., 2023, 47, 12670–12677 RSC
.
- X. W. Yu, B. Wang, C. Wang, C. Zhuang, Y. F. Yao, Z. S. Li, C. P. Wu, J. Y. Feng and Z. G. Zou, Small, 2021, 17, 2103412 CrossRef CAS
.
- H. D. Li, Y. Han, H. Zhao, W. J. Qi, D. Zhang, Y. D. Yu, W. W. Cai, S. X. Li, J. P. Lai, B. L. Huang and L. Wang, Nat. Commun., 2020, 11, 5437 CrossRef CAS
.
- J. Wang, W. Wang, X. N. Liu, S. S. Shang, Y. T. Chen, L. Y. Shi and S. Cui, Ceram. Int., 2024, 50, 1795–1806 CrossRef CAS
.
- Y. R. Ding, Z. Y. Wang, Z. J. Liang, X. P. Sun, Z. H. Sun, Y. X. Zhao, J. L. Liu, C. Y. Wang, Z. Y. Zeng, L. Fu, M. Q. Zeng and L. Tang, Adv. Mater., 2023, 2302860 CrossRef PubMed
.
- H. Y. Qiao, M. T. Saray, X. Z. Wang, S. M. Xu, G. Chen, Z. N. Huang, C. J. Chen, G. Zhong, Q. Dong, M. Hong, H. Xie, R. Shahbazian-Yassar and L. B. Hu, ACS Nano, 2021, 15, 14928–14937 CrossRef CAS
.
- H. J. Li, H. G. Huang, Y. Chen, F. L. Lai, H. Fu, L. S. Zhang, N. Zhang, S. X. Bai and T. X. Liu, Adv. Mater., 2023, 35, 2209242 CrossRef CAS PubMed
.
- F. Q. Wang, P. C. Zou, Y. Y. Zhang, W. L. Pan, Y. Li, L. M. Liang, C. Chen, H. Liu and S. J. Zheng, Nat. Commun., 2023, 14, 6019 CrossRef CAS
.
- R. Abbasi, B. P. Setzler, S. S. Lin, J. H. Wang, Y. Zhao, H. Xu, B. Pivovar, B. Y. Tian, X. Chen, G. Wu and Y. S. Yan, Adv. Mater., 2019, 31, 1805876 CrossRef
.
- A. Villagra and P. Millet, Int. J. Hydrogen Energy, 2019, 44, 9708–9717 CrossRef CAS
.
- I. Vincent, A. Kruger and D. Bessarabov, Int. J. Electrochem. Sci., 2018, 13, 11347–11358 CrossRef CAS
.
- M. Ebrahimi, B. Naghali and M. Aryanfar, Energy Convers. Manage., 2022, 258, 115499 CrossRef
.
- J. Zhu, L. S. Hu, P. X. Zhao, L. Y. S. Lee and K. Y. Wong, Chem. Rev., 2020, 120, 851–918 CrossRef CAS
.
- L. Quan, H. Jiang, G. L. Mei, Y. J. Sun and B. You, Chem. Rev., 2024, 124, 3694–3812 CrossRef CAS PubMed
.
- S. Anantharaj and S. Noda, Small, 2020, 16, 1905779 CrossRef CAS
.
- L. Yu and Z. Ren, Mater. Today Phys., 2020, 14, 100253 CrossRef
.
- G. L. Gao, X. L. Chen, L. Han, G. Zhu, J. Jia, A. Cabot and Z. X. Sun, Coord. Chem. Rev., 2024, 503, 215639 CrossRef CAS
.
- S. Safari, F. Esmaeilion, A. Rabanian, D. H. Jamali, S. Negi, S. Hoseinzadeh, F. Sayedin, S. S. Bhoglla, M. El. Haj Assad, B. Das, M. A. Ehyaei, A. Ahmadi, M. Soltani and H. Afshari, Environ. Dev. Sustain., 2024 DOI:10.1007/s10668-024-04699-y
.
- S. Zhang, X. Zhang, Y. Rui, R. H. Wang and X. J. Li, Green Energy Environ., 2021, 6, 458–478 CrossRef CAS
.
- Y. Yan, B. Y. Xia, B. Zhao and X. Wang, J. Mater. Chem. A, 2016, 4, 17587–17603 RSC
.
- Y. J. Li, Y. J. Sun, Y. N. Qin, W. Y. Zhang, L. Wang, M. C. Luo, H. Yang and S. J. Guo, Adv. Energy Mater., 2020, 13, 12088–12101 Search PubMed
.
- X. B. Fu, J. H. Zhang, S. Q. Zhan, F. J. Xia, C. J. Wang, D. S. Ma, Q. Yue, J. S. Wu and Y. J. Kang, ACS Catal., 2022, 12, 11955–11959 CrossRef CAS
.
- J. Greeley, T. F. Jaramillo, J. Bonde, I. B. Chorkendorff and J. K. Norskov, Nat. Mater., 2006, 5, 909–913 CrossRef CAS PubMed
.
- Z. P. Ifkovits, J. M. Evans, M. C. Meier, K. M. Papadantonakis and N. S. Lewis, Energy Environ. Sci., 2021, 14, 4740–4759 RSC
.
- T. W. Wu, M. M. Melander and K. Honkala, ACS Catal., 2022, 12, 2505–2512 CrossRef CAS
.
- K. Shah, R. Y. Dai, M. Mateen, Z. Hassan, Z. W. Zhuang, C. H. Liu, M. Israr, W. C. Cheong, B. T. Hu, R. Y. Tu, C. Zhang, X. Chen, Q. Peng, C. Chen and Y. D. Li, Angew. Chem., Int. Ed., 2022, 61, e202114951 CrossRef CAS
.
- A. Radwan, H. H. Jin, D. P. He and S. C. Mu, Nano-Micro Lett., 2021, 13, 132 CrossRef CAS PubMed
.
- J. C. Hao, Z. C. Zhuang, K. C. Cao, G. H. Gao, C. Wang, F. L. Lai, S. L. Lu, P. M. Ma, W. F. Dong, T. X. Liu, M. L. Du and H. Zhu, Nat. Commun., 2022, 13, 2662 CrossRef CAS PubMed
.
- Y. M. Zou, L. Jing, J. H. Zhang, S. Z. Luo, L. Y. Wang, Y. Li, R. Goei, K. W. Tan and A. L. Y. Tok, J. Mater. Chem. A, 2024, 12, 5668–5678 RSC
.
- J. S. Wang, S. S. Xin, Y. Xiao, Z. F. Zhang, Z. M. Li, W. Zhang, C. J. Li, R. Bao, J. Peng, J. H. Yi and S. L. Chou, Angew. Chem., Int. Ed., 2022, 61, e202202518 CrossRef CAS
.
- C. Q. Huang, L. Yu, W. Zhang, Q. Xiao, J. Q. Zhou, Y. L. Zhang, P. F. An, J. Zhang and Y. Yu, Appl. Catal., B, 2020, 276, 120367 CrossRef
.
- S. Q. Wang, B. L. Xu, W. Y. Huo, H. C. Feng, X. F. Zhou, F. Fang, Z. H. Xie, J. K. Shang and J. Q. Jiang, Appl. Catal., B, 2022, 313, 121472 CrossRef CAS
.
- P. Sharma, S. Kainth, O. P. Pandey and R. Mahajan, ACS Appl. Nano Mater., 2023, 6, 21788–21802 CrossRef CAS
.
- J. L. Wang, Y. C. Huang, Y. Q. Wang, H. Deng, Y. C. Shi, D. X. Wei, M. T. Li, C. L. Dong, H. Jin, S. S. Mao and S. H. Shen, ACS Catal., 2023, 13, 2374–2385 CrossRef CAS
.
- J. Y. Wang, J. H. Zhang, Y. J. Hu, H. Jiang and C. Z. Li, Sci. Bull., 2022, 67, 1890–1897 CrossRef CAS PubMed
.
- H. H. You, D. S. Wu, D. H. Si, M. N. Cao, F. F. Sun, H. Zhang, H. M. Wang, T. F. Liu and R. Cao, J. Am. Chem. Soc., 2022, 144, 9254–9263 CrossRef CAS PubMed
.
- C. L. Chen, M. Z. Sun, F. Zhang, H. J. Li, M. R. Sun, P. Fang, T. L. Song, W. X. Chen, J. C. Dong, B. Rosen, P. W. Chen, B. L. Huang and Y. J. Li, Energy Environ. Sci., 2023, 16, 1685–1696 RSC
.
- F. Nie, Z. Li, X. P. Dai, X. L. Yin, Y. H. Gan, Z. H. Yang, B. Q. Wu, Z. T. Ren, Y. H. Cao and W. Y. Song, Chem. Eng. J., 2022, 431, 134080 CrossRef CAS
.
- W. Q. Li, H. Zhang, M. Z. Hong, L. L. Zhang, X. Feng, M. F. Shi, W. X. Hu and S. C. Mu, Chem. Eng. J., 2022, 431, 134072 CrossRef CAS
.
- D. H. Park, M. H. Kim, M. Kim, J. H. Byeon, J. S. Jang, J. H. Kim, D. Lim, S. H. Park, Y. H. Gu, J. Kim and K. W. Park, Appl. Catal., B, 2023, 327, 122444 CrossRef CAS
.
- J. J. Song, C. Wei, Z. F. Huang, C. T. Liu, L. Zeng, X. Wang and Z. C. J. Xu, Chem. Soc. Rev., 2020, 49, 2196–2214 RSC
.
- N. T. Suen, S. F. Hung, Q. Quan, N. Zhang, Y. J. Xu and H. M. Chen, Chem. Soc. Rev., 2017, 46, 337–365 RSC
.
- T. Zhang, H. F. Zhao, K. Y. Wang, Z. J. Chen, L. Li, J. Peng, X. Peng, Y. J. Huang and H. B. Yu, Mater. Futures, 2023, 2, 045101 CrossRef CAS
.
- L. Liu, T. H. Liu, C. Xu, W. Y. Zhao, J. P. Fan, J. Liu, X. G. Ma and W. S. Fu, Nano Lett., 2024, 24, 2831–2838 CrossRef CAS PubMed
.
- S. Q. Wang, W. Y. Huo, F. Fang, Z. H. Xie, J. K. Shang and J. Q. Jiang, Chem. Eng. J., 2022, 429, 132410 CrossRef CAS
.
- A. Raveendran, M. Chandran and R. Dhanusuraman, RSC Adv., 2023, 13, 3843–3876 RSC
.
- G. J. Dong, L. L. Yan and Y. P. Bi, J. Mater. Chem. A, 2023, 11, 3888–3903 RSC
.
- X. D. He, X. Han, X. Y. Zhou, J. D. Chen, J. Wang, Y. Chen, L. H. Yu, N. Zhang, J. Li, S. Wang and H. L. Jin, Appl. Catal., B, 2023, 331, 122683 CrossRef CAS
.
- G. Huang, M. Hu, X. T. Xu, A. A. Alothman, M. S. S. Mushab, S. J. Ma, P. K. Shen, J. L. Zhu and Y. Yamauchi, Small Struct., 2023, 4, 2200235 CrossRef CAS
.
- X. Xu, G. W. Fu, Y. X. Wang, Q. H. Cao, Y. R. Xun, C. Li, C. Guan and W. Huang, Nano Lett., 2023, 23, 629–636 CrossRef CAS
.
- Q. Yang, H. X. Liu, P. Yuan, Y. Jia, L. Z. Zhuang, H. W. Zhang, X. C. Yan, G. H. Liu, Y. F. Zhao, J. Z. Liu, S. Q. Wei, L. Song, Q. L. Wu, B. Q. Ge, L. Z. Zhang, K. Wang, X. Wang, C. R. Chang and X. D. Yao, J. Am. Chem. Soc., 2022, 144, 2171–2178 CrossRef CAS
.
- Q. Liu, S. J. Sun, L. C. Zhang, Y. S. Luo, Q. Yang, K. Dong, X. D. Fang, D. D. Zheng, A. A. Alshehri and X. P. Sun, Nano Res., 2022, 15, 8922–8927 CrossRef CAS
.
- J. Y. Zhang, J. S. Lian, Q. Jiang and G. Y. Wang, Chem. Eng. J., 2022, 439, 135634 CrossRef CAS
.
- H. Nady, M. M. El-Rabiei, G. M. A. El-Hafez and A. M. Fekry, Int. J. Hydrogen Energy, 2021, 46, 21785–21795 CrossRef CAS
.
- R. V. Digraskar, V. S. Sapner, A. V. Ghule and B. R. Sathe, J. Electroanal. Chem., 2021, 882, 114983 CrossRef CAS
.
- X. Xiao, Z. Li, Y. Xiong and Y. W. Yang, J. Am. Chem. Soc., 2023, 145, 16548–16556 CrossRef CAS PubMed
.
- X. Song, Z. H. Li, L. Sheng and N. Xiao, Appl. Surf. Sci., 2023, 639, 158225 CrossRef CAS
.
- Y. Teng, L. Zhou, Y. Z. Chen, J. Z. Gan, Y. Xi and H. L. Jia, Dalton Trans., 2023, 52, 15839–15847 RSC
.
- J. L. Chen, S. Y. Feng, C. J. Lu and J. F. Huang, Chem. Eng. J., 2023, 468, 143761 CrossRef CAS
.
- H. M. Zhang, K. Li, X. Y. Guo, L. P. Zhang, D. Cao and D. J. Cheng, Small, 2024, 20, 2306694 CrossRef CAS
.
- Y. C. Dang, G. Q. Wang, X. Li, X. Ma, F. Yue, C. T. Wang, L. J. Gao and F. Fu, Int. J. Hydrogen Energy, 2023, 48, 17035–17044 CrossRef CAS
.
- P. F. Liu, Y. Shi, X. Zhang, J. Yin, D. Zhang, T. N. Wang, J. W. Fei, T. R. Zhan, G. J. Li, J. P. Lai and L. Wang, Appl. Catal., B, 2024, 341, 123332 CrossRef CAS
.
- W. Li, W. Wang, M. C. Niuc, K. Yang, J. H. Luane, H. Zhang and Z. Jiao, Acta Mater., 2024, 262, 119426 CrossRef CAS
.
- P. Zhang, L. Y. Gong, X. Xu, Z. H. Lou, Z. Y. Wei, P. H. Chen, Z. Z. Wu, J. Xu and F. Gao, Chem. Eng. J., 2023, 472, 144974 CrossRef CAS
.
- H. L. Huang, Y. C. Chang, Y. C. Huang, L. L. Li, A. C. Komarek, L. H. Tjeng, Y. Orikasa, C. W. Pao, T. S. Chan, J. M. Chen, S. C. Haw, J. Zhou, Y. F. Wang, H. J. Lin, C. T. Chen, C. L. Dong, C. Y. Kuo, J. Q. Wang, Z. W. Hu and L. J. Zhang, Nat. Commun., 2023, 14, 2112 CrossRef CAS PubMed
.
- Y. Dang, P. Han, Y. Li, Y. Zhang and Y. Z. Zhou, J. Mater. Sci., 2020, 55, 13951–13963 CrossRef CAS
.
- V. Gatard, D. De Masi, R. Chattot, I. M. Marin, J. M. A. Revert, P. F. Fazzini, T. Encinas, V. Martin, S. Faure, J. Deseure, J. Carrey, B. Chaudret and M. Chatenet, Electrocatalysis, 2021, 11, 567–577 CrossRef
.
- Z. Y. Rao, B. Dutta, F. Körmann, W. J. Lu, X. Y. Zhou, C. Liu, A. K. da Silva, U. Wiedwald, M. Spasova, M. Farle, D. Ponge, B. Gault, J. Neugebauer, D. Raabe and Z. M. Li, Adv. Funct. Mater., 2021, 31, 2007668 CrossRef CAS
.
- R. F. Zhao, B. Ren, G. P. Zhang, Z. X. Liu, B. Cai and J. J. Zhang, J. Magn. Magn. Mater., 2019, 491, 165574 CAS
.
- M. Akbar, A. Shah, F. J. Iftikhar, G. Ali, H. Han and G. Rahman, Int. J. Energy Res., 2021, 45, 2931–2944 CAS
.
- R. Wang, J. Z. Huang, X. H. Zhang, J. C. Han, Z. H. Zhang, T. L. Gao, L. L. Xu, S. W. Liu, P. Xu and B. Song, ACS Nano, 2022, 16, 3593–3603 CrossRef CAS
.
- A. Bazan-Aguilar, G. García, E. Pastor and A. M. Baena-Moncada, Electrochim. Acta, 2024, 479, 143881 CrossRef CAS
.
- H. L. Guo, Z. Y. Guo, K. B. Chu, W. Zong, H. Zhu, L. Q. Zhang, C. W. Liu, T. X. Liu, J. Hofkens and F. L. Lai, Adv. Funct. Mater., 2023, 33, 2308229 CrossRef CAS
.
- N. O. Peña, D. Ihiawakrim, M. Han, B. Lassalle-Kaiser, S. Carenco, C. Sanchez, C. Laberty-Robert, D. Portehault and O. Ersen, ACS Nano, 2019, 13, 11372–11381 CrossRef
.
- X. P. Zhang, W. L. Jiang, S. H. Cao, H. J. Sun, X. Q. You, S. H. Cai, J. L. Wang, C. S. Zhao, X. Wang, Z. Chen and S. G. Sun, Electrochim. Acta, 2018, 273, 300–306 CrossRef CAS
.
- P. Schnell, M. Kolbach, M. Schleuning, K. Obata, R. Irani, I. Y. Ahmet, M. Harb, D. E. Starr, R. van de Krol and F. F. Abdi, Adv. Energy Mater., 2021, 11, 2003183 CrossRef CAS
.
- W. C. Wan, Y. G. Zhao, S. Q. Wei, C. A. Triana, J. G. Li, A. Arcifa, C. S. Allen, R. Cao and G. R. Patzke, Nat. Commun., 2021, 12, 5589 CAS
.
- S. L. Qi, K. X. Zhu, T. Xu, H. F. Zhang, X. Y. Guo, J. H. Wang, F. X. Zhang and X. Zong, Adv. Mater., 2024, 2403328 CAS
.
- X. M. Li, Y. F. Zhou, C. R. Feng, R. Wei, X. G. Hao, K. Y. Tang and G. Q. Guan, Nano Res., 2023, 16, 4411–4437 CrossRef
.
- H. M. Zhang, S. F. Zhang, L. H. Zuo, J. K. Li, J. X. Guo, P. Wang, J. F. Sun and L. Dai, Rare Met., 2024, 43, 2371–2390 CrossRef CAS
.
- A. Ahmad, A. Nairan, Z. Feng, R. M. Zheng, Y. L. Bai, U. Khan and J. K. Gao, Small, 2024, 2311929 CrossRef CAS
.
- Z. X. Cai, H. Goou, Y. Ito, T. Tokunaga, M. Miyauchi, H. Abe and T. Fujita, Chem. Sci., 2021, 12, 11306–11315 RSC
.
- X. M. Wang, Q. Q. Peng, X. L. Zhang, X. C. Lv, X. Wang and Y. Z. Fu, J. Colloid Interface Sci., 2022, 607, 1580–1588 CrossRef CAS
.
- M. M. Liu, Z. H. Zhang, F. Okejiri, S. Z. Yang, S. H. Zhou and S. Dai, Adv. Mater. Interfaces, 2019, 6, 1900015 CrossRef CAS
.
- D. Zhang, Y. Shi, H. Zhao, W. J. Qi, X. L. Chen, T. R. Zhan, S. X. Li, B. Yang, M. Z. Sun, J. P. Lai, B. L. Huang and L. Wang, J. Mater. Chem. A, 2021, 9, 889–893 RSC
.
- M. Y. Fan, J. J. Wang, J. Zhao, H. Zhang, T. Y. Ma, X. P. Han and W. B. Hu, Rare Met., 2024, 43, 1537–1546 CrossRef CAS
.
- Z. Jia, T. Yang, L. G. Sun, Y. L. Zhao, W. P. Li, J. H. Luan, F. C. Lyu, L. C. Zhang, J. J. Kruzic, J. J. Kai, J. C. Huang, J. Lu and C. T. Liu, Adv. Mater., 2020, 32, 2000385 CrossRef CAS
.
- X. Y. Cui, Y. C. Liu, X. Y. Wang, X. L. Tian, Y. X. Wang, G. Zhang, T. Liu, J. Ding, W. B. Hu and Y. N. Chen, ACS Nano, 2024, 18, 2948–2957 CrossRef CAS
.
- Y. S. Cho and J. Kang, Nanoscale, 2024, 16, 3936–3950 RSC
.
- H. Kamaruddin, J. H. Zhang, Y. Liang, Y. F. Wei and Y. Z. Huang, J. Mater. Chem. A, 2024, 12, 9933–9961 RSC
.
- R. T. Zhou, X. Han, Q. Chen, L. W. Peng, X. Y. Qiu, P. Wang, C. Guo, J. C. Wang, Z. J. Wang and J. H. Hao, J. Mater. Chem. A, 2024, 12, 5719–5730 RSC
.
- W. Q. Chen, X. Yan, Z. L. Liu, X. C. Zhang and C. F. Du, Int. J. Hydrogen Energy, 2023, 48, 29969–29981 CrossRef CAS
.
- D. W. Lai, Q. L. Kang, F. Gao and Q. Y. Lu, J. Mater. Chem. A, 2021, 9, 17913–17922 RSC
.
- X. R. Huo, X. J. Zuo, X. Wang, B. W. Xing and N. N. Zhang, Chem. – Asian J., 2023, 18, e202300456 CrossRef CAS PubMed
.
- Y. T. He, X. H. Zhu, C. X. Zhang, Z. Q. Liu, B. B. Cao, L. Y. Sheng, Y. Yang, Q. G. Feng, N. Wang, J. Z. Ou and Y. Xu, ACS Sustainable Chem. Eng., 2023, 11, 5055–5064 CrossRef CAS
.
- C. L. Huang, Y. G. Lin, C. L. Chiang, C. K. Peng, D. S. Raja, C. T. Hsieh, Y. A. Chen, S. Q. Chang, Y. X. Yeh and S. Y. Lu, Appl. Catal., B, 2023, 320, 122016 CrossRef CAS
.
- W. H. Luo, Y. Wang, L. X. Luo, S. Gong, Y. X. Li and X. P. Gan, Appl. Surf. Sci., 2022, 606, 154808 CrossRef CAS
.
- W. H. Shi, H. W. Liu, Z. Z. Li, C. H. Li, J. H. Zhou, Y. F. Yuan, F. Jiang, K. Fu and Y. G. Yao, SusMat, 2022, 2, 186–196 CrossRef CAS
.
- H. Liu, H. Y. Qin, J. L. Kang, L. Y. Ma, G. X. Chen, Q. Huang, Z. J. Zhang, E. Z. Liu, H. M. Lu, J. X. Li and N. Q. Zhao, Chem. Eng. J., 2022, 435, 134898 CrossRef CAS
.
- T. T. Zhang, J. Q. Li, B. Y. Zhang, G. Q. Wang, K. Jiang, Z. L. Zheng and J. H. Shen, J. Alloys Compd., 2023, 969, 172439 CrossRef CAS
.
- Y. Wang, W. H. Luo, S. Gong, L. X. Luo, Y. X. Li, Y. Y. Zhao and Z. Li, Adv. Mater., 2023, 35, 2302499 CrossRef CAS
.
- S. Q. Chang, C. C. Cheng, P. Y. Cheng, C. L. Huang and S. Y. Lu, Chem. Eng. J., 2022, 446, 137452 CrossRef CAS
.
- D. Zhang, Y. Shi, X. L. Chen, J. P. Lai, B. L. Huang and L. Wang, Chin. J. Catal., 2023, 45, 174–183 CrossRef CAS
.
- Z. C. Wang, X. Y. Zhang, X. K. Wu, Y. Pan, H. D. Li, Y. Han, G. R. Xu, J. Q. Chi, J. P. Lai and L. Wang, Chem. Eng. J., 2022, 437, 135375 CrossRef CAS
.
- P. Y. Ma, M. M. Zhao, L. Zhang, H. Wang, J. F. Gu, Y. C. Sun, W. Ji and Z. Y. Fu, J. Materiomics, 2020, 6, 736–742 CrossRef
.
- Y. Lu, S. Xu, L. M. Zhang, Q. Z. Hu, J. J. Song, F. B. Zhang and B. Zhang, Sustainable Mater. Technol., 2022, 33, e00455 CrossRef CAS
.
- Y. H. Sun, T. M. Tang, L. Y. Xiao, J. Y. Han, X. Bai, M. Y. Shi, S. Y. Chen, J. R. Sun, Y. Y. Ma and J. Q. Guan, Chem. – Eur. J., 2024, 30, e202303779 CrossRef CAS
.
- Y. H. Sun, T. M. Tang, L. Y. Xiao, J. Y. Han, X. Bai, M. Y. Shi, S. Y. Chen, J. R. Sun, Y. Y. Ma and J. Q. Guan, ACS Appl. Nano Mater., 2024, 7, 4008–4013 CrossRef CAS
.
- Z. J. Zhang, N. Yu, Z. Y. Jin, Y. S. Zhang, Y. C. Li, Y. L. Zhou, Y. M. Chai and B. Dong, Colloids Surf., A, 2024, 684, 133073 CrossRef CAS
.
- Z. X. Cai, S. Bolar, Y. Ito and T. Fujita, Nanoscale, 2024, 16, 4803–4810 RSC
.
- J. Hu, T. Q. Guo, X. Y. Zhong, J. Li, Y. J. Mei, C. X. Zhang, Y. B. Feng, M. Z. Sun, L. J. Meng, Z. Y. Wang, B. L. Huang, L. B. Zhang and Z. C. Wang, Adv. Mater., 2024, 36, 2310918 CrossRef CAS
.
- K. Bera, S. S. Roy, R. Madhu, A. D. T. De, P. Gudlur and S. Kundu, Catal. Sci. Technol., 2024, 14, 2858–2867 RSC
.
- Z. Li, C. S. Xu, Z. Y. Zhang, S. Xia, D. S. Li, L. R. Liu, P. Chen and X. C. Dong, Adv. Sci., 2024, 2308477 CrossRef CAS
.
- X. F. Tian, H. D. Li, R. Chang, Y. Yang, Z. H. Wang, T. Dong, J. P. Lai, S. H. Feng and L. Wang, J. Mater. Chem. A, 2024, 12, 3276–3282 RSC
.
- H. H. Wei, Q. Wang, Y. Zhang, J. Li, P. Liu, N. N. Wang and X. Q. Gong, Fuel, 2024, 358, 130011 CrossRef CAS
.
- L. Sharma, N. K. Katiyar, A. Parui, R. Das, R. Kumar, C. S. Tiwary, A. K. Singh, A. Halder and K. Biswas, Nano Res., 2022, 15, 4799–4806 CrossRef CAS
.
- J. Tang, J. L. Xu, Z. G. Ye, Y. C. Ma, X. B. Li, J. M. Luo and Y. Z. Huang, J. Alloys Compd., 2021, 885, 160995 CrossRef CAS
.
- Y. D. Yu, H. D. Li, J. Liu, W. X. Xu, D. Zhang, J. Xiong, B. Li, A. O. Omelchuk, J. P. Lai and L. Wang, J. Mater. Chem. A, 2022, 10, 21260–21265 RSC
.
- Y. J. He, W. Liu and J. Q. Liu, J. Colloid Interface Sci., 2024, 661, 409–435 CrossRef CAS PubMed
.
- V. Yadav, Megha, P. Sen and M. M. Shaijumon, J. Mater. Chem. A, 2024, 12, 5319–5330 RSC
.
- H. J. Qiu, G. Fang, J. J. Gao, Y. R. Wen, J. Lv, H. L. Li, G. Q. Xie, X. J. Liu and S. H. Sun, ACS Mater. Lett., 2019, 1, 526–533 CrossRef CAS
.
- Q. P. Zhang, X. Wang, T. Z. Jian, W. Q. Ma, C. X. Xu, Q. X. Zhou and H. Liu, Chin. J. Chem., 2024, 42, 1465–1473 CrossRef CAS
.
- K. Huang, J. Y. Xia, Y. Lu, B. W. Zhang, W. C. Shi, X. Cao, X. Y. Zhang, L. M. Woods, C. C. Han, C. J. Chen, T. Wang, J. S. Wu and Y. Z. Huang, Adv. Sci., 2023, 10, 2300094 CrossRef CAS PubMed
.
- Y. F. Cui, S. D. Jiang, Q. Fu, R. Wang, P. Xu, Y. Sui, X. J. Wang, Z. L. Ning, J. F. Sun, X. Sun, A. Nikiforov and B. Song, Adv. Funct. Mater., 2023, 33, 2306889 CrossRef CAS
.
- A. Sivanantham, H. Lee, S. W. Hwang, B. Ahn and I. S. Cho, J. Mater. Chem. A, 2021, 9, 16841–16851 RSC
.
- H. Shi, X. Y. Sun, Y. Liu, S. P. Zeng, Q. H. Zhang, L. Gu, T. H. Wang, G. F. Han, Z. Wen, Q. R. Fang, X. Y. Lang and Q. Jiang, Adv. Funct. Mater., 2023, 33, 2214412 CrossRef CAS
.
- B. Wang, W. G. Liu, Y. C. Leng, X. W. Yu, C. Wang, L. H. Hu, X. Zhu, C. P. Wu, Y. F. Yao and Z. G. Zou, iScience, 2023, 26, 106326 CrossRef CAS
.
- A. A. Alamdari, H. Jahangiri, M. B. Yagci, K. Igarashi, H. Matsumoto, A. Motallebzadeh and U. Unal, ACS Appl. Energy Mater., 2024, 7, 2423–2435 CrossRef
.
- T. A. A. Batchelor, J. K. Pedersen, S. H. Winther, I. E. Castelli, K. W. Jacobsen and J. Rossmeisl, Joule, 2019, 3, 834–845 CrossRef CAS
.
- H. Wu, Z. X. Li, Z. C. Wang, Y. J. Ma, S. R. Huang, F. Ding, F. Q. Li, Q. X. Zhai, Y. L. Ren, X. W. Zheng, Y. R. Yang, S. C. Tang, Y. Deng and X. K. Meng, Appl. Catal., B, 2023, 325, 122356 CrossRef CAS
.
- Z. Wang, C. Zhu, H. Tan, J. Liu, L. L. Xu, Y. Q. Zhang, Y. P. Liu, X. X. Zou, Z. Liu and X. H. Lu, Adv. Funct. Mater., 2021, 31, 2104735 CrossRef CAS
.
- Q. Q. Wang, J. Q. Li, Y. J. Li, G. M. Shao, Z. Jia and B. L. Shen, Nano Res., 2022, 15, 8751–8759 CrossRef CAS
.
- S. D. Jiang, Y. H. Yu, H. He, Z. Y. Wang, R. G. Zheng, H. Y. Sun, Y. G. Liu and D. Wang, Small, 2024, 2310786 CrossRef CAS
.
- B. Wang, X. Zhu, X. D. Pei, W. G. Liu, Y. C. Leng, X. W. Yu, C. Wang, L. H. Hu, Q. M. Su, C. P. Wu, Y. F. Yao, Z. Q. Lin and Z. G. Zou, J. Am. Chem. Soc., 2023, 145, 13788–13795 CrossRef CAS PubMed
.
- F. McKay, Y. X. Fang, O. Kizilkaya, P. Singh, D. D. Johnson, A. Roy, D. P. Young, P. T. Sprunger, J. C. Flake, W. A. Shelton and Y. Xu, J. Phys. Chem. C, 2021, 125, 17008–17018 CrossRef CAS PubMed
.
- X. Yang, R. K. Guo, R. Cai, Y. J. Ouyang, P. P. Yang and J. F. Xiao, Int. J. Hydrogen Energy, 2022, 47, 13561–13578 CrossRef CAS
.
- G. Feng, F. H. Ning, J. Song, H. F. Shang, K. Zhang, Z. P. Ding, P. Gao, W. S. Chu and D. G. Xia, J. Am. Chem. Soc., 2021, 143, 17117–17127 CrossRef CAS
.
- H. Minamihara, K. Kusada, D. S. Wu, T. Yamamoto, T. Toriyama, S. Matsumura, L. S. R. Kumara, K. Ohara, O. Sakata, S. Kawaguchi, Y. Kubota and H. Kitagawa, J. Am. Chem. Soc., 2022, 144, 11525–11529 CrossRef CAS PubMed
.
- A. Hota, J. K. Das, P. K. Panda, A. A. Mohammed, A. Biswal, B. Rakesh and B. C. Tripathy, Dalton Trans., 2024, 53, 4544–4550 RSC
.
- Z. Jia, K. Nomoto, Q. Wang, C. Kong, L. G. Sun, L. C. Zhang, S. X. Liang, J. Lu and J. J. Kruzic, Adv. Funct. Mater., 2021, 31, 2101586 CrossRef CAS
.
- C. H. Zhan, Y. Xu, L. Z. Bu, H. Z. Zhu, Y. G. Feng, T. Yang, Y. Zhang, Z. Q. Yang, B. L. Huang, Q. Shao and X. Q. Huang, Nat. Commun., 2021, 12, 6261 CrossRef CAS
.
- Y. Q. Zhang, D. D. Wang and S. Y. Wang, Small, 2022, 18, 2104339 CrossRef CAS
.
- J. Tang, J. L. Xu, Z. G. Ye, X. B. Li and J. M. Luo, J. Mater. Sci. Technol., 2021, 79, 171–177 CrossRef CAS
.
- R. Z. Wan, C. Y. Wang, R. Chen, M. Liu and F. Yang, Int. J. Hydrogen Energy, 2022, 47, 32039–32049 CrossRef CAS
.
- X. M. Li, Q. Y. Hu, H. Y. Wang, M. Chen, X. G. Hao, Y. F. Ma, J. Liu, K. Y. Tang, A. Abudula and G. Q. Guan, Appl. Catal., B, 2021, 292, 120172 CrossRef CAS
.
- K. Huang, D. D. Peng, Z. X. Yao, J. Y. Xia, B. W. Zhang, H. Liu, Z. B. Chen, F. Wu, J. S. Wu and Y. Z. Huang, Chem. Eng. J., 2021, 425, 131533 CAS
.
- K. Huang, B. W. Zhang, J. S. Wu, T. Y. Zhang, D. D. Peng, X. Cao, Z. Zhang, Z. Li and Y. Z. Huang, J. Mater. Chem. A, 2020, 8, 11938–11947 RSC
.
- F. He, Y. J. Zhao, X. X. Yang, S. X. Zheng, B. Yang, Z. J. Li, Y. B. Kuang, Q. H. Zhang, L. C. Lei, M. Qiu, L. M. Dai and Y. Hou, ACS Nano, 2022, 16, 9523–9534 CrossRef CAS PubMed
.
- L. He, M. G. Li, L. Y. Qiu, S. Geng, Y. Q. Liu, F. Y. Tian, M. C. Luo, H. Liu, Y. S. Yu, W. W. Yang and S. J. Guo, Nat. Commun., 2024, 15, 2290 CrossRef CAS PubMed
.
- X. Du, L. L. Yin, W. J. Zhang, M. L. Zhang, K. M. Su and Z. H. Li, J. Mater. Sci. Technol., 2023, 135, 26–33 CrossRef CAS
.
- M. Y. Shi, T. M. Tang, L. Y. Xiao, J. Y. Han, X. Bai, Y. H. Sun, S. Y. Chen, J. R. Sun, Y. Y. Ma and J. Q. Guan, Chem. Commun., 2023, 59, 11971–11974 RSC
.
- S. Li, L. K. Tong, Z. J. Peng, B. Zhang and X. L. Fu, J. Mater. Chem. A, 2023, 11, 13697–13707 RSC
.
- T. Gu, Z. Y. Jing, F. Miao, W. Wu, Y. H. Zhao, H. Hou and X. B. Liang, Int. J. Hydrogen Energy, 2024, 56, 1464–1474 CrossRef CAS
.
- Y. L. Wang, Y. L. Ai, S. W. Yang, B. L. Liang, Z. H. Zheng, S. Ouyang, W. He, W. H. Chen, C. H. Liu, J. J. Zhang and Z. Y. Liu, J. Inorg. Mater., 2021, 36, 425–430 CrossRef
.
- S. Liu, Y. Wang, T. L. Jiang, S. Jin, M. Sajid, Z. D. Zhang, J. W. Xu, Y. P. Fan, X. Y. Wang, J. H. Chen, Z. C. Liu, X. H. Zheng, K. Zhang, Q. S. Nian, Z. X. Zhu, Q. Peng, T. Ahmad, K. Li and W. Chen, ACS Nano, 2024, 18, 4229–4240 CrossRef CAS
.
- C. R. Feng, Y. F. Zhou, M. Chen, L. N. Zou, X. M. Li, X. W. An, Q. Zhao, P. Xiaokaiti, A. Abudula, K. Yan and G. Q. Guan, Appl. Catal., B, 2024, 349, 123875 CrossRef CAS
.
- Y. T. Liao, R. T. Zhu, W. J. Zhang, H. Y. Zhu, Y. Sun, J. L. Chen, Z. H. Dong and R. H. Lv, Nano Res., 2024, 17, 3379–3389 CrossRef CAS
.
- A. L. Maulana, P. C. Chen, Z. X. Shi, Y. Yang, C. Lizandara-Pueyo, F. Seeler, H. D. Abruña, D. Muller, K. Schierle-Arndt and P. D. Yang, Nano Lett., 2023, 23, 6637–6644 CrossRef CAS PubMed
.
- S. Jo, M. C. Kim, K. B. Lee, H. Choi, L. Zhang and J. I. Sohn, Adv. Energy Mater., 2023, 13, 2301420 CrossRef CAS
.
- R. Feng, C. A. Zhang, M. C. Gao, Z. R. Pei, F. Zhang, Y. Chen, D. Ma, K. An, J. D. Poplawsky, L. Z. Ouyang, Y. Ren, J. A. Hawk, M. Widom and P. K. Liaw, Nat. Commun., 2021, 12, 4329 CrossRef CAS PubMed
.
- J. C. Ren, J. J. Zhou, C. J. Butch, Z. G. Ding, S. Li, Y. H. Zhao and W. Liu, J. Mater. Sci. Technol., 2023, 138, 70–79 CrossRef CAS
.
- J. Zhang, X. P. Xiang, B. Xu, S. S. Huang, Y. X. Xiong, S. H. Ma, H. J. Fu, Y. Ma, H. Y. Chen, Z. G. Wu and S. J. Zhao, Curr. Opin. Solid State Mater. Sci., 2023, 27, 101057 CrossRef CAS
.
- T. Fujita, Mater. Trans., 2023, 64, 2386–2393 CrossRef CAS
.
- L. P. Wu, T. Guo and T. Li, Adv. Funct. Mater., 2022, 32, 2203439 CrossRef CAS
.
- H. Chun, E. Lee, K. Nam, J. H. Jang, W. Kyoung, S. H. Noh and B. Han, Chem. Catal., 2021, 1, 855–869 CrossRef CAS
.
- Y. G. Yao, Q. Dong, A. Brozena, J. Luo, J. W. Miao, M. F. Chi, C. Wang, I. G. Kevrekidis, Z. J. Ren, J. Greeley, G. F. Wang, A. Anapolsky and L. B. Hu, Science, 2022, 376, 151–162 CrossRef
.
- V. A. Mints, J. K. Pedersen, A. Bagger, J. Quinson, A. S. Anker, K. M. O. Jensen, J. Rossmeisl and M. Arenz, ACS Catal., 2022, 12, 11263–11271 CrossRef CAS
.
- W. B. Xu, E. Diesen, T. W. He, K. Reuter and J. T. Margraf, J. Am. Chem. Soc., 2024, 146, 7698–7707 CrossRef CAS PubMed
.
- J. Zhang, B. A. Xu, Y. X. Xiong, S. H. Ma, Z. Wang, Z. G. Wu and S. J. Zhao, npj Comput. Mater., 2022, 8, 5 CrossRef
.
- M. Kim, Y. Kim, M. Y. Ha, E. Shin, S. J. Kwak, M. Park, I. Kim, W. B. Jung, W. B. Lee, Y. Kim and H. T. Jung, Adv. Mater., 2023, 35, 211497 Search PubMed
.
- X. Wang, W. Guo and Y. Z. Fu, J. Mater. Chem. A, 2021, 9, 663–701 RSC
.
- Q. D. Wang, C. L. Zhao, J. L. Wang, Z. P. Yao, S. W. Wang, S. G. H. Kumar, S. Ganapathy, S. Eustace, X. D. Bai, B. H. Li and M. Wagemaker, Nat. Commun., 2023, 14, 440 CAS
.
- J. Peng, B. Zhang, W. B. Hua, Y. R. Liang, W. Zhang, Y. M. Du, G. Peleckis, S. Indris, Q. F. Gu, Z. X. Cheng, J. Z. Wang, H. K. Liu, S. X. Dou and S. L. Chou, Angew. Chem., Int. Ed., 2023, 62, e202215865 CrossRef CAS PubMed
.
- R. R. Katzbaer, F. M. D. Vieira, I. Dabo, Z. Q. Mao and R. E. Schaak, J. Am. Chem. Soc., 2023, 145, 6753–6761 CrossRef CAS PubMed
.
- Y. Xin, S. H. Li, Y. Y. Qian, W. K. Zhu, H. B. Yuan, P. Y. Jiang, R. H. Guo and L. B. Wang, ACS Catal., 2020, 10, 11280–11306 CrossRef CAS
.
- K. B. Chu, J. J. Qin, H. Y. Zhu, M. De Ras, C. Wang, L. Xiong, L. S. Zhang, N. Zhang, J. A. Martens, J. Hofkens, F. L. Lai and T. X. Liu, Sci. China Mater., 2022, 65, 2711–2720 CrossRef CAS
.
- Z. W. Chen, Z. Gariepy, L. X. Chen, X. Yao, A. Anand, S. J. Liu, C. G. T. Feugmo, I. Tamblyn and C. V. Singh, ACS Catal., 2022, 12, 14864–14871 CrossRef CAS
.
|
This journal is © The Royal Society of Chemistry 2024 |