DOI:
10.1039/D3LC00507K
(Paper)
Lab Chip, 2024,
24, 1887-1902
Comparison between dynamic versus static models and real-time monitoring of neuronal dysfunction in an amyloid-β induced neuronal toxic model on a chip platform†
Received
12th June 2023
, Accepted 18th February 2024
First published on 19th February 2024
Abstract
Microfluidics-based organs-on-a-chip offer a promising method for dynamic and 3-dimensional (3D) cell culture to evaluate the cell behaviors within the biomimetic environment. The purpose of this study was to establish neural network connections in a 3D neural stem cell (NSC)-based system with an interstitial level of flow for simulating the brain microenvironment toward a dynamic amyloid-β (Aβ) induced neuronal toxic model on a chip and to compare the biological effects and neurite dysfunction between static and dynamic systems. The brain-on-a-chip system consisted of an impedance analyzing layer, a structured well with a connected channel, and an interface coating with polypeptide films fabricated with modification based on our previous study. The cytotoxicity and percentage of neuron/astrocyte differentiation were all compared in both static and dynamic brain-on-a-chip systems. Reactive oxygen species production, neuron marker expression and neurotransmitter-acetylcholine release were all compared to evaluate functional neurite losses in both static and dynamic systems with/without Aβ addition. Moreover, real-time impedance recording was used to consecutively monitor the neurite connection/disconnection in both static and dynamic brain-on-a-chip systems. The NSC-based dynamic brain-on-a-chip may enable the application of different neurodegenerative disease in vitro models for pathogenesis studies, drug discovery and novel therapeutic method development.
1. Introduction
Neuronal differentiation and neurite outgrowth are very important in neural development. Neurons differentiate from progenitor cells and migrate to destination and outgrowth neurites to form neural networks through synaptic connections.2 Therefore, axonal outgrowth is directly related to the development of neurogenesis, and neurodegenerative disorders are caused by the disintegration of neural circuits.3–5In vitro cell-based assays supply simple and cost-effective tools to avoid the disadvantages of large-scale animal life losses, but conventional 2-dimensional (2D) cell-based assays cannot provide accurate information due to certain limitations.6 3D culture models can mimic real tissue in vivo, provide accurate information in comparison with conventional 2D cell-based assays, reduce animal usage, and complement the missing biological response of in vivo models while increasing the efficiency of drug screening.7,8
Alzheimer's disease (AD) is a kind of neurodegenerative disease and an enormous burden on society.9,10 A satisfactory in vitro brain model that can mimic the in vivo microenvironment for neurodegenerative disease studies may help develop effective novel treatments and facilitate drug discovery. Neural cells in a brain region are in 3D space and interact with a complex multicellular environment and 3D networks.11 Deposition of amyloid beta-peptide (Aβ) in plaques and neurofibrillary tangles have been shown to be the two dominant pathological characteristics of AD.12 Astrocytes are considered important for Aβ clearance and degradation, forming a protective barrier between Aβ deposits and neurons. However, a previous study also revealed that astrocytes were activated by Aβ assemblies and consequently released proinflammatory molecules, causing neuronal damage.13 Therefore, neural stem cell (NSC) spheroid-based models with 3D structures and microphysiological environments can be differentiated into multiple neural cells that have been considered the best candidates to better understand the progression of neurodegenerative disorders, including AD.14–17
Engineering techniques such as microfluidics can facilitate the demand for novel and biomimetic in vitro models for studying neurodegenerative diseases.18 Among such tools, organ-on-a-chip platforms have attracted attention due to their advantages in examining pathological variations and cell–protein interactions and in reducing drug development costs and time. Hence, the combination of microfluidic technology with 3D multicellular spheroid-based systems offers great potential for in vivo-like tissue-based applications. In comparison with traditional 2D and static culture systems, microfluidic-based organ-on-a-chip systems emulate the microphysiological environment by controlling spatiotemporal parameters to permit continuous nutrient exchange, cell–cell interactions, better oxygen perfusion, and physiological shear stress, which more closely reflect the conditions in living organisms. Microphysiological systems (MPSs) designed for the nervous system offer essential and physiologically relevant models for studying diseases and development. They are vital for advancing our understanding of cellular mechanisms, disease pathways, and screening potential therapeutic strategies for nervous system disorders. MPS platforms enable the recreation of intricate neural structures, overcoming the limitations of traditional 2D models. They also facilitate the incorporation of advanced instrumentation to directly measure outputs, including electrophysiology, with integrated electrodes being pivotal for direct probing of neuronal responses within MPS setups. Furthermore, microfluidic-based MPSs support media perfusion, ensuring a stable nutrient supply and efficient waste removal, enhancing the fidelity of these models in mimicking the neural microenvironment. Previous studies have introduced several commercial MPSs for application in the nervous system based on different designs.19 The Organoplate® is a 3D culture microfluidic device that harnesses gravity-driven flow for its operations. PhaseGuides™, a fundamental component of this technology, utilize surface tension to establish compartmentalization within the chip, all without the need for physical barriers. This innovative approach promotes meaningful cell–cell interactions. Xona Microfluidics provides disposable microfluidic chips for cell compartmentalization in larger channels connected by microchannels, guiding neurite growth and enabling synaptic connections. These chips prevent cell migration and have been instrumental in studying injury-induced Ca2+ release in peripheral nerve regeneration within the PNS.20 Nonetheless, models that can be utilized for central nervous system disease research while enabling real-time monitoring of synaptic growth remain relatively scarce. Therefore, developing an in vitro brain model that can adequately represent in vivo neurogenesis and the brain microenvironment is urgently needed for a better understanding of AD pathomechanisms and for the development of new drug leads. Several microfluidic platforms have been established in previous studies, including the AD model. A traditional model using neurons, astrocytes, and microglia in a 3D microfluidic platform was developed to investigate human microglial recruitment, neuroinflammatory responses and neuron/astrocyte damage.21 However, even in microfluid-based platforms, flow effects are often ignored. Generally, neurons are bathed by the interstitial fluid of the brain, which forms the microenvironment of the central nervous system. This dynamic system has the potential to improve many of the problems found in static cell culture systems. Previous studies have also developed livers and brains on chips that more closely mimic the in vivo microenvironment by providing a continuous flow of medium to the cells through osmotic pumping. This method also inspired the establishment of our dynamic system.22,23 These studies demonstrated that mimicking interstitial flow is closer to the physiology of the real tissue and the disease model, which may provide a better understanding of the pathophysiology. However, the development of a biomimetic AD-on-a-chip remains limited and lacks some elements due to its inherent complexity. Furthermore, while 3D neural spheroid models offer numerous advantages for investigating neural development and function, they also present challenges. 1) Spheroids represent complex 3D structures, rendering it challenging to access and monitor neural activity within the inner layers of these structures. 2) Spheroids frequently exhibit heterogeneity with regard to cell types, cell densities, and neural differentiation levels, posing difficulties in obtaining consistent and reproducible results when assessing neural activity. 3) Ensuring proper neural maturation and functionality within the spheroid can be a formidable task. 4) The technical complexity involved in interfacing spheroid models with external systems for monitoring and stimulation can be a notable challenge. These challenges underscore the need for ongoing research and innovation in the field of 3D neural spheroid modeling.
From our perspective, a cutting-edge and comprehensive neuronal toxicity model should encompass the following components: a multicellular composition that closely replicates the intricate microenvironment of the brain, a dynamic system capable of mimicking the interstitial flow conditions, a pattern specifically designed to regulate neurite outgrowth, and a real-time monitoring system for detecting neurite dysfunction. These are the fundamental criteria we used in our research endeavors. In this context, we employed the Aβ induced neuronal toxicity model as a demonstration model to compare and contrast static and dynamic systems. Additionally, we aimed to underscore the advantages of continuously monitoring neurite connection/disconnection via impedance determination. Consequently, continuous impedance monitoring can furnish valuable information concerning the time course of neurite dysfunction, which could serve as a novel approach for drug discovery.
First, an already-designed brain-on-a chip system with modification based on our previous study was used in this research.1 The chip consisted of an impedance-detected layer for real-time monitoring of neural dysfunction, a patterned culture well with a connected channel and interface modification with polypeptide films for NSC spheroid seeding and guidance in a preestablished direction for neurite outgrowth. Second, the interstitial level of the slow flow microfluidic system by an osmotic micropump was used for the dynamic model. The flow was simulated to the level of interstitial flow provided by the osmotic micropump system, and the flow rate was controlled in the range of 0.15–0.2 μL min−1. The cytotoxicity, cell viability, and relative percentage of neuron/astrocyte differentiation were compared in static and dynamic systems. Subsequently, Aβ and reactive oxygen species (ROS) accumulation, synapse dysfunction, and acetylcholine release were all compared in static and dynamic systems with/without Aβ addition. Finally, an impedance sensing system was used to monitor neural network formation and neurite dysfunction at different timepoints.
2. Materials and methods
2.1. Design and establishment of a dynamic amyloid-β induced neuronal toxic model-on-a-chip system
The design and fabrication of the brain-on-a-chip system with an interstitial level of slow flow was performed as described in our previous study with dynamic system modifications, as shown in Fig. 1(B) and (C).1,24–26Fig. 1(B) illustrates the chip design concept. The chip was constructed with a polydimethylsiloxane (PDMS; SylgardÒ 184, Dow Corning, USA) culture chamber layer, an SU-8 structure layer (SU-8 3050, MicroChem, USA), and an indium tin oxide (ITO)-glass detector layer. Each culture well featured an ITO electrode embedded in its bottom layer. The structure layer consisted of 3 × 3 arrays of culture wells (500 μm diameter) for NSC spheroid seeding, connected with 400 μm long channels for network formation, and 100–120 μm thick culture holes. Each sphere left in the hole was exposed to the culture medium with fluid flow. Approximately 200 μl of medium volume existed in each 3 × 3 array (one well). Then, the culture chamber was treated with oxygen plasma and modified with (PLL/PLGA)3.5 multilayer films to guide NSC differentiation and neural network formation.27 The step-by-step fabrication of the chip, (PLL/PLGA)3.5 multilayer film coating, and NSC spheroid seeding are also illustrated in Fig. 1(C).
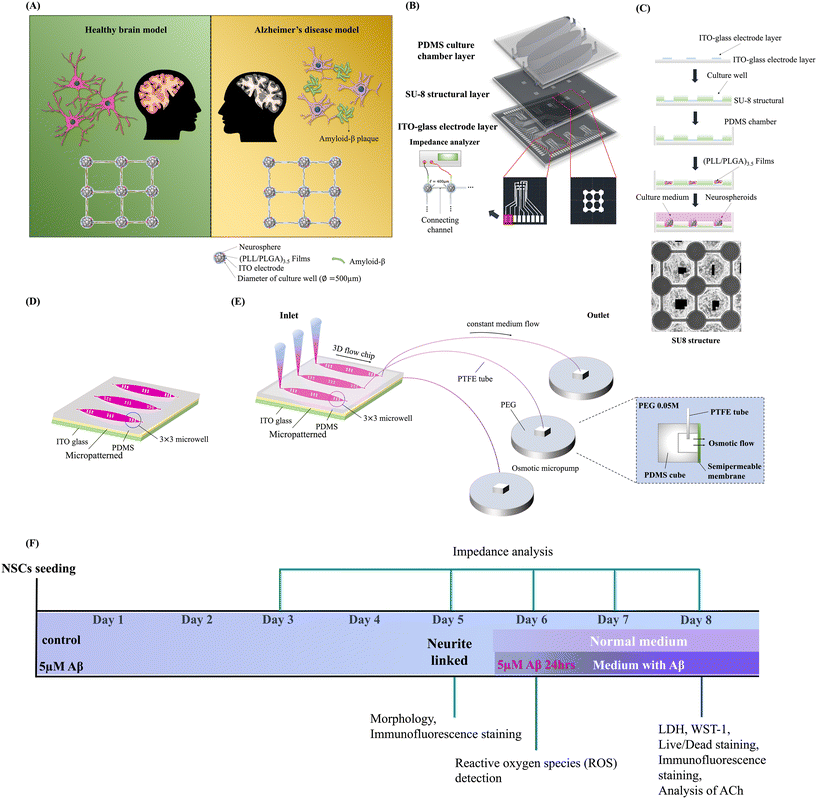 |
| Fig. 1 Design and fabrication of the biochip. (A) Schematic illustration (not to scale) of normal and amyloid-β induced neuronal toxic models on a brain-on-a-chip. (B) Illustration of the biochip design and impedance analysis. (C) Illustration of the step-by-step biochip fabrication, multilayer film coating, and NSC spheroid seeding. (D) Schematic illustration of the static system. (E) Schematic diagram of the in vitro dynamic system to simulate the microenvironment using a 3D cytoarchitecture and interstitial flow. The outlet is connected to the osmotic micropump system to achieve a steady flow of medium at the interstitial flow level. (F) Timeline of the experimental design. | |
Fig. 1(A) illustrates the concept of the NSC spheroid-based biochip with/without Aβ addition in static and dynamic systems. The static and dynamic devices are illustrated in Fig. 1(D) and (E), respectively. The timeline of the experimental procedure is shown in Fig. 1(F). This innovative system offers a 3D cytoarchitecture, interstitial flow, and brain microenvironment simulation. Our study was able to replicate a biomimetic brain microenvironment in both normal and neuronal toxic models. This facilitated the investigation of Aβ effects on static and dynamic models.
The interstitial level of the slow-flow microfluidic system initiated by the osmotic micropump was connected to the outlet to provide a continuous flow of the medium at a rate of 0.15–0.20 μL min−1, as indicated in Fig. 1(E). The osmotic micropump was composed of patterned PDMS chambers. The dimensions of the PDMS chambers (10 × 10 × 10 mm) with a cellulose membrane window (5 × 5 mm) were fabricated. Different concentrations of PEG, different materials of tubes connected to the outlet and different volumes of PEG were tested to optimize the parameters to provide a continuous flow of the medium at a rate of 0.15–0.20 μL min−1 as listed in Table 1. The osmotic micropump operated in the solution consisting of pure distilled water and different concentrations (0.01, 0.03 and 0.05 M) of poly(ethylene glycol) (PEG; Sigma, USA) solution was separated by a semipermeable cellophane film. Different materials of tubes connected to the outlet, including polytetrafluoroethylene (PTFE; inner diameter, 1.0 mm; outer diameter, 1.5 mm), PE and PE coil tubes of 15 cm, were connected at the outlet of the chip to sustain the flow over time. Each experiment was performed with more than three replicates, and more than two batches were used to optimize the parameters.
Table 1 Average flow rate of different parameters test for interstitial fluid model. (A) Different concentrations of PEG. (B) Different materials. (C) Different volumes of PEG used in the system
(A) |
PEG (M) |
24 h (μL min−1) |
48 h (μL min−1) |
72 h (μL min−1) |
0.05 |
0.151 ± 0.03
|
0.132 ± 0.03
|
0.133 ± 0.03
|
0.03 |
0.0606 |
0.0568 |
0.050 |
0.01 |
0.0227 |
0.0189 |
0.0252 |
(B) |
PEG (0.05 M) |
24 h (μL min−1) |
48 h (μL min−1) |
72 h (μL min−1) |
PTFE |
0.23 ± 0.03
|
0.20 ± 0.03
|
0.21 ± 0.03
|
PE coil tube |
0.288 |
0.42 |
— |
(C) |
PEG (ml) |
24 h |
12 |
0.23 |
10 |
0.18 ± 0.03
|
9 |
0.13 |
8 |
0.083 |
2.2. Isolation of cortical NSCs
Primary cortical NSCs were derived from the cerebral cortical regions of pregnant ED 16 Wistar rat embryos, and the detailed protocol followed our previous study with modification.28 Animal studies were performed in accordance with the recommendations of the Institutional Animal Care and Use Committee at National Tsing Hua University (IACUC approval no. 109042, August, 26, 2020 approved). Rat embryonic cerebral cortices were isolated, cut into small pieces and mechanically ground in cold Hank's balanced salt solution (HBSS) on ice. Then, the cells were collected by centrifugation and resuspended at a density of 50
000 cells per cm2 in DMEM/F12 culture medium with basic fibroblast growth factor (20 ng ml−1, Invitrogen, USA) under general culture conditions for 3 days. Subsequently, a single passage and purification process was carried out over an additional 4 days. The NSC spheroids were then seeded onto chips coated with (PLL/PLGA)3.5 and maintained in DMEM/F12 medium within a humidified incubator at 37 °C with 5% CO2. Then, after 5 days of incubation, NSC differentiation with network formation was observed on the micropatterned and material-regulated biochip.
2.3. Cell viability and cytotoxicity assay
The cytotoxicity assay and cell viability of NSCs on the chip in static and dynamic systems with/without Aβ incubation were determined by lactate dehydrogenase (LDH), WST1, and live/dead assays. The timelines of NSC network formation, Aβ addition, and assessment are shown in Fig. 1(F). A Cytotoxicity Detection Kit was purchased from ROCHE (Germany), and the cytotoxicity reagents were prepared according to the manufacturer's protocol. LDH activity can be quantified by using the NADH produced during the conversion of lactate to pyruvate to reduce a second compound in a coupled reaction into a product with properties that are easily quantitated. The culture media were directly collected from NSCs in static and dynamic systems and incubated with/without 5 μM Aβ. Then, 100 μl of the reagent was added to 100 μl of the supernatant in each well. After 15 min of incubation, the LDH content was assessed by enzyme-linked immunosorbent assay (ELISA) and was read at an absorbance of 490 nm in a multimode microplate reader (BioTek Instruments, USA) with a reference wavelength of 630 nm.
The WST-1 (ROCHE, Germany) measurement was performed according to the standard protocol of the manufacturer. Briefly, the NSC spheroids were seeded on a chip and incubated for 5 days. After neural network formation, 100 μl WST-1 was added to the media and incubated for another 4 h at 37 °C in the incubator. After the reaction was completed, the plate was read at 450 nm; a reference reading was performed at 630 nm.
Calculation of the survival rate of NSCs cultured in a static/dynamic system and with/without Aβ incubation followed the instructions of the manufacturer of the calcein-AM/PI-Live/Dead Cell Staining Kit (Life, USA). Specifically, 2 μl of 1 mol L−1 calcein-AM (staining live cells) and 2 μl of 1.5 mol L−1 PI (staining dead cells) were mixed into 1 mL of medium in a cell culture dish. After 5 days of incubation in the groups without 5 μM Aβ and the groups with more than 3 days of incubation with 5 μM Aβ, 500 μl of the dye reagent was mixed with 500 μl of the medium, added to the chip and incubated at 37 °C for 15 min. The cells were incubated with a cell-permeable green fluorescent dye to stain live cells and a red fluorescent dye with propidium iodide to stain dead cells, in which viable cells were actively pumped out of the cytoplasm. After incubation at 37 °C for 15 minutes in the dark, the medium was removed and the samples were washed 3 times with PBS. Stained cells were visualized by confocal microscopy (LSM 800 META, Zeiss, Germany). Live cells exhibited green fluorescence, while dead cells appeared red. The fluorescence intensity of nine punched holes was quantified and compared.
2.4. Scanning electron microscopy (SEM)
SEM (Scanning Electron Microscopy) using a Hitachi S-300N instrument from Japan was used to analyze the morphological changes in NSCs after incubation with varying Aβ concentrations for three days. The process involved cell fixation in ice-cold 2.5% glutaraldehyde in PBS for one hour, followed by three 10 minute PBS rinses. Subsequently, OsO4 was used for a one-hour fixation, followed by another round of three 10 minute PBS rinses. The cells were then dehydrated through graded ethanol washes, subjected to critical point drying, gold-coated in a vacuum, and examined by SEM.
2.5. Immunofluorescent staining
After 8 days of incubation, the cells were fixed in 70% methanol for 5 min and rehydrated in phosphate buffered saline (PBS). After fixing, primary monoclonal antibodies were diluted to an appropriate concentration in a solution containing blocking solution (10% bovine serum albumin) (Sigma, USA). Then, the cells were incubated with the following primary antibodies for 2 h at room temperature: an anti-microtubule-associated protein 2 (MAP-2) monoclonal antibody (1
:
500; Millipore, USA) to identify mature neurons, rabbit anti-glial fibrillary acidic protein polyclonal antibody (anti-GFAP; 1
:
1000; Millipore) for astrocyte detection, β-tubulin antibody (1
:
500; Merck, USA) to identify neurons, NEFH antibody (1
:
500; Abcam, USA) to determine filament expression, and anti-synapsin I (1
:
500; Millipore, Germany) to determine synapse formation. Then, the cells were washed in PBS 3 times, followed by incubation with diluted secondary antibodies for 2 h at room temperature: FITC-, rhodamine- and cy5-conjugated secondary antibodies (1
:
250, AP187F; AP181 R, Millipore, Germany) (1
:
250, Alexa Fluor 488; Alexa Fluor 647, Abcam, England). For nuclear staining, the cells were incubated with anti-Hoechst 33342 monoclonal antibody (1
:
2000; Invitrogen, USA) at room temperature. Amyloid plaque staining was also performed after fixation. After fixation, NSCs were washed with PBS three times and incubated in 1 μM thioflavin S (Sigma Aldrich, Germany) diluted in 50% ethanol for 10 min. Then, the samples were rinsed with 80% ethanol twice for 5 min and washed with PBS three times for 3 min. Cells were observed using a confocal microscope (LSM 800 META, Zeiss, Germany) and the fluorescence intensity was quantified by using ImageJ.
2.6. Measurement of intracellular levels of ROS
ROS levels were determined using the ROS Detection Assay Kit (BioVision Incorporated, USA) and were determined by cell imaging by fluorescence microscopy. NSC spheroids were seeded on a chip for 5 days of culture for network formation and were subsequently incubated in a static/dynamic system with/without 5 μM Aβ for another 24 h. After removing the medium, 500 μl of 2′,7′-dichlorofluorescin diacetate (DCFDA) probe solution was added to each well and incubated for 45 min at 37 °C in the dark. The immunostained cells were observed by fluorescence microscopy (Leica i8, Germany), and the fluorescence intensity was quantified by using ImageJ with a 3 × 3 array.
2.7. Acetylcholine chloride analysis
The acetylcholine chloride (ACh) concentration of NSCs after 8 days of culture in a static/dynamic system with/without Aβ incubation was determined by using a choline/ACh assay kit (Abcam, England) according to the manufacturer's instructions. First, lysis buffer was added to the chip and incubated for 15 min at room temperature. Then, 50 μl of sample was mixed with 50 μl of working reagent and incubated in the dark at room temperature for 30 min. After the reaction was complete, the plate was read at 570 nm. The color intensity at 570 nm was directly proportional to the ACh concentration in the sample and was calculated from the standard curve, as shown in ESI† S3.
2.8. FM1-43 assay
FM1-43 labeling of functional synapses followed established procedures.29 Saturation staining was conducted by utilizing a depolarizing extracellular solution to induce synaptic vesicle cycling while FM1-43 was present. This process resulted in the distinctive labeling of functional presynaptic terminals. After 8 days of culture, a solution containing 90 mM KCl and 2 μM FM1-43 (a fluorescent styryl membrane probe from Invitrogen) was applied for 60 seconds. Cells were then rinsed three times with normal saline for 5 minutes each to remove surface-bound FM1-43. To assess synaptic vesicle release and turnover, synapses were loaded with FM1-43 and subsequently de-stained by a 150 second stimulation with 90 mM KCl solution in the absence of FM1-43. The synaptic vesicles within these functional synapses were stained with the FM1-43 membrane dye, and subsequently, the fluorescence intensities of the synapses were examined before and after destaining. The changes in FM1-43 fluorescence were quantified.
2.9. Impedance measurement of the neural network connections
The network connection/disconnection of each pair of NSC spheroids was performed by measuring the impedance across each pair of electrodes based on our previous study.1 The impedance values of the NSC culture-on-a-chip in a static/dynamic system for 0 days, 3 days and 5 days of culture, with/without Aβ incubation after 24 h, 48 h, and 72 h, were determined by using an impedance analyzer (VersaSTAT 4; Princeton Applied Research, USA). A potential of 0.1 Vrms was applied across the electrodes. Based on our previous study, impedance at 1 kHz and a threshold of 40 kΩ were defined to determine the electrical connections of NSC spheroids.1 The impedance values between two electrodes below 40 kΩ indicate that the neural network connected to NSC spheroids, and the impedance values increased up to 40 kΩ, suggesting neural network dysfunction.
2.10. Statistical analysis
All data were obtained from at least three independent biological samples. All of the quantification results in the text and in the figures are presented as the mean values ± the standard deviation (SD). 3–6 images were randomly taken for image quantification in each analysis. Student's t test was used for two-sample comparisons. The statistical significance level was set as follows: *, p < 0.05, **, p < 0.01, ***, p < 0.005, and ****, p < 0.001.
3. Results and discussion
3.1. Gradient flow generation and analysis
To provide an in vitro strategy for investigating neural network dysfunction with Aβ addition and modeling the flow rate of the brain interstitium, a microfluidic system using an osmotic micropump that can simulate slow interstitial flow (0.1 to 0.3 μL min−1) in brain tissue was integrated into the platform.30 The image of the osmotic micropump setup is shown in ESI† S1. The driving force of the flow was generated by the osmotic micropump, which was affected by the different concentrations of PEG (Table 1(A)), different materials of the tubes connected to the outlet (Table 1(B)) and different volumes of PEG (Table 1(C)). The flow rate increased as the molar concentration of PEG increased, and it was considered that a higher concentration would provide a larger driving force, which affected the flow rate. In addition, different materials of tubes, including PTFE, PE, and PE coil, were compared, and it was demonstrated that the stability of the flow rate by using PTFE materials was better than those of other materials. In addition, the volume of PEG may affect the active area of the semipermeable cellophane film, which also affected the osmotic pressure and flow rate. Finally, the optimized parameters, 0.05 M PEG, PTFE tube (inner diameter, 1.0 mm; outer diameter, 1.5 mm) and 10 ml PEG, were chosen to control the flow rate within 0.15–0.20 μL min−1.
3.2. Comparison of neural network formation and differentiation percentage in static and dynamic systems
The NSC neurospheroids were cultured in the microwell of the chip for 5 days in both static and dynamic systems. Differentiated cells derived from NSC neurospheroid and neurite outgrowth were investigated in both systems. The NSC neurospheres grew in the space-limited channels inside the microwell. In addition, neurites grew out and connected to another microwell in the two neurospheroids after 5 days of culture, as shown in Fig. 2(A).
 |
| Fig. 2 Neural network formation and NSC spheroid differentiation in static and dynamic systems. (A) Microscopy images of the neural network-on-a-chip in (a) static and (b) dynamic systems. Red arrows indicate neurite outgrowth. (B) Immunofluorescence images of NSC spheroid differentiation in the (a) static system and (b) dynamic system (purple staining corresponds to MAP2 expression, green staining corresponds to the GFAP expression and blue staining corresponds to Hoechst). (C) Quantification of the percentages of astrocyte (GFAP) and neural (MAP-2) cells. Asterisks denote significant differences where indicated (****p < 0.001) as determined by Student's t test. (D) Immunofluorescence images of NSC spheroid differentiation in the (a) static system and (b) dynamic system (purple staining corresponds to β-tubulin expression and blue staining corresponds to Hoechst). | |
Furthermore, the immunostaining results of MAP2 (neurons) and GFAP (astrocytes) are shown in Fig. 2(B). The quantification results of MAP2 (red) and GFAP (green) are shown in Fig. 2(C). Fig. 2(B) shows that MAP2 was highly expressed in the dynamic group in comparison with the static group. In contrast, GFAP was highly expressed in the static group, which demonstrated that more astrocyte differentiation was observed in the static system. Further quantification of the relative percentages of MAP2 and GFAP showed that the neuron percentage was upregulated over 80% in the dynamic system. Continuous medium flow enhanced neural-related cell differentiation derived from NSCs, especially neurons. Previous research has also revealed that continuous medium flow dynamically facilitates the delivery of oxygen and nutrients, which may promote differentiation and enhance neurite extension.31 This result is consistent with the previous literature.
In addition to MAP2, the β-tubulin isotype is also widely recognized as a neuronal hallmark within the fields of developmental neurobiology and stem cell research. The immunostaining findings for β-tubulin are presented in Fig. 2(D). Fig. 2(D) illustrates that β-tubulin exhibited significantly heightened expression in the dynamic group when compared to the static group, a consistent outcome with the MAP2 immunostaining results. Besides, Fig. 2(D) also illustrates that within the dynamic system, NSCs differentiate into a larger number of denser and more intricate neural networks, with connections between channels becoming notably more pronounced.
3.3. Effect of the Aβ concentration on NSCs
The Aβ concentration's impact on neurospheroids within a biochip was pretested. Results from a cell viability assay and SEM images of NSCs exposed to 1 μM, 3 μM, and 5 μM Aβ for three days are presented in Fig. S5.† Fig. S5(A)† indicates a significant decrease in cell viability after NSCs were exposed to these Aβ concentrations. Notably, higher Aβ concentrations corresponded to lower cell viability. In addition, SEM images in Fig. S5(B)† further show NSC morphologies post-incubation with varying Aβ concentrations. In Fig. S5(B)-(a),† cell attachment, migration from spheroids, and process outgrowth were seen in the group without Aβ. Conversely, varying degrees of cell death and neurite degeneration were observed in groups incubated with 1 μM, 3 μM, and 5 μM Aβ, with notable shriveled cells and neurite damage in the 5 μM Aβ group (Fig. S5(B)-(d)†). Consequently, a 5 μM Aβ concentration was considered suitable for modeling neuronal damage and network degeneration in Alzheimer's disease (AD).
3.4. Comparison of the cytotoxicity and viability of NSC-based chips in static and dynamic systems with/without Aβ addition
Fig. 3(A) and (B) show the cytotoxicity and cell viability of NSCs on a chip incubated with static or dynamic systems within 5 days of network formation with/without 5 μM Aβ incubation for 3 days, respectively. The cytotoxicities and cell viabilities of the four groups, NSCs in the static system, NSCs in the dynamic system, NSCs in the static system with Aβ incubation and NSCs in the dynamic system with Aβ incubation, were compared. As shown in Fig. 3(A), the LDH assay showed that the cytotoxicities in static systems without Aβ incubation were significantly higher than that in the dynamic system (p < 0.05). Also, the cytotoxicities in static systems with Aβ incubation also showed significant difference in comparison with that in the dynamic system (p < 0.01). In addition, the cytotoxicities of the groups incubated with Aβ were higher than those of the groups without Aβ, as predicted. In addition, the WST-1 assay, as shown in Fig. 3(B), demonstrated the cell viabilities of the 4 groups. It was also shown that NSCs cultured in a dynamic system exhibited better cell activity than those cultured in a static system, and the groups incubated with Aβ exhibited lower cell viability, which revealed the damage caused by Aβ. As shown in Fig. 3(B), to compare the groups with Aβ addition, the cell viability of the group in the dynamic system is significantly higher than that in the static system (p < 0.05). Therefore, both the LDH and WST-1 assays showed significant differences among the static and dynamic systems and among the groups with/without 5 μM Aβ. Furthermore, the results of the live/dead staining in the static and dynamic systems and the groups with/without 5 μM Aβ are shown in Fig. 3(C). The quantification result of the relative percentages of live/dead staining is shown in Fig. 3(D). As shown in Fig. 3(D), the percentage of live cells is about 70% in the static system and about 85% in the dynamic system, respectively. The results also showed a significant difference (p < 0.05) and provide the evidence that continuous medium flow dynamically facilitates the delivery of oxygen and nutrients for a better culture environment. In addition, after 8 days of culture, in the groups without Aβ incubation, most of the NSCs were alive, and only a few dead cells were observed. In contrast, few live cells and a large number of dead cells were seen in the groups with 5 μM Aβ treatment. The statistical analysis shows a significant difference between the groups with 5 μM Aβ treatment in dynamic and static systems which also revealed that the dynamic system may provide a better culture environment. Therefore, it is considered that the dynamic system may provide a more biomimetic environment that is closer to real tissue than the static system, which affects cell viability not only in the brain chip system but also in the AD-on-a-chip system.
 |
| Fig. 3 Cell viability assessments were conducted in both dynamic and static systems, with and without 5 μM Aβ incubation for incubation of 3 days. (A) LDH assay of NSC spheroids-on-a-chip after 5 days of network formation in a static/dynamic system with/without 5 μM Aβ incubation for 3 days. (B) Cell viability assay of NSC spheroids-on-a-chip after 5 days of network formation in a static/dynamic system and incubated with/without 5 μM Aβ for 3 days. (C) Live/dead staining of NSCs-on-a-chip after 5 days of network formation in (a) a static system without Aβ addition, (b) a static system with Aβ addition, (c) a dynamic system without Aβ addition and (d) a dynamic system with Aβ addition for 3 days. (D) Quantification of the relative percentages of fluorescent intensity of the live/dead cell staining. Asterisks denote significant differences where indicated (****p < 0.001) as determined by Student's t test. | |
3.5. Comparison of ROS production in NSC-based chips in static and dynamic systems with/without Aβ addition
Oxidative stress and ROS are toxic agents and ubiquitous in biological systems. Any imbalance between ROS production and antioxidant defense creates oxidative stress that advances various pathophysiological conditions of degenerative nerve diseases, including Alzheimer's and Parkinson's diseases. Previous studies have indicated that the toxicity of Aβ peptide aggregation to neurons is related to the production of ROS.32 Therefore, the accumulation levels of ROS in the four groups, NSCs in the static system, NSCs in the dynamic system, NSCs in the static system with Aβ incubation and NSCs in the dynamic system with Aβ incubation, were determined and compared, as shown in Fig. 4(A). In addition, the fluorescence intensities of the 3 × 3 biochip in the static and dynamic systems and the groups with/without 5 μM Aβ were quantified and normalized to the neurosphere area, as shown in Fig. 4(B). Fig. 4(A)-a and c show that there was little ROS release in the static and dynamic systems without Aβ addition. In contrast, fluorescence images and quantification data all demonstrated that both the static and dynamic systems with Aβ addition showed significant differences in higher ROS production in comparison with the groups without Aβ addition. Furthermore, both dynamic systems also showed significant differences in lower ROS production in comparison with static systems. These results are consistent with the cytotoxicity results, which revealed that the dynamic system may contribute to toxic metabolism, resulting in the downregulation of ROS production.
 |
| Fig. 4 ROS production was conducted in both dynamic and static systems, with and without 5 μM Aβ incubation for 1 day. (A) ROS detection in NSC spheroids-on-a-chip after 5 days of network formation in (a) a static system without Aβ, (b) a static system incubated with Aβ, (c) a dynamic system without Aβ addition and (d) a dynamic system incubated with Aβ for 1 day. (B) Quantification of the fluorescence intensity of ROS production. Asterisks denote significant differences where indicated (**p < 0.01 and ****p < 0.001) as determined by Student's t test. | |
3.6. Immunostaining of NSC-based chips in static and dynamic systems with/without Aβ addition
Fig. 5 shows the double staining of fluorescence images and the quantification results of MAP2 and thioflavin S expression. MAP2 (purple) and thioflavin S (green) staining levels in the static and dynamic systems with/without 5 μM Aβ addition are shown in Fig. 5(A) and (B), respectively. Thioflavin S is a mixture of ammonium salts and has been shown to bind fibrillar Aβ aggregates that are generally used for staining and visualizing Aβ plaques in Alzheimer's disease. MAP2 is a neuron-specific cytoskeletal protein and is widely used to identify mature neurons. As shown in Fig. 5(A) and (C), thioflavin S accumulation was significantly higher in the NSC-based chips with Aβ addition than in the groups without Aβ incubation. However, the quantification results of thioflavin S expression between the static and dynamic systems did not show a significant difference. In addition, Fig. 5(B)-a and c demonstrate that neuron cell differentiation and neurite growth were clearly observed in the systems without Aβ incubation; moreover, neurites even grew along the channels connecting adjacent neurospheres. However, as shown in Fig. 5(B)-b and d, neuronal cells incubated with Aβ exhibited a large number of damaged neurons and neurites, and the connections between neurites in the channel were clearly disrupted. The neurites were highly degenerated in both static and dynamic neuronal toxic model-on-a-chip systems with Aβ addition. Among the four groups, MAP2 demonstrated the lowest expression in the static system with Aβ addition after 5 days of neural network formation and 3 days of Aβ incubation. In contrast, the highest MAP2 expression was observed in the groups treated with the dynamic system without Aβ addition. Quantification of the MAP2 results also showed that the percentage of neurites in the dynamic condition was higher than that in the static condition with/without 5 μM Aβ.
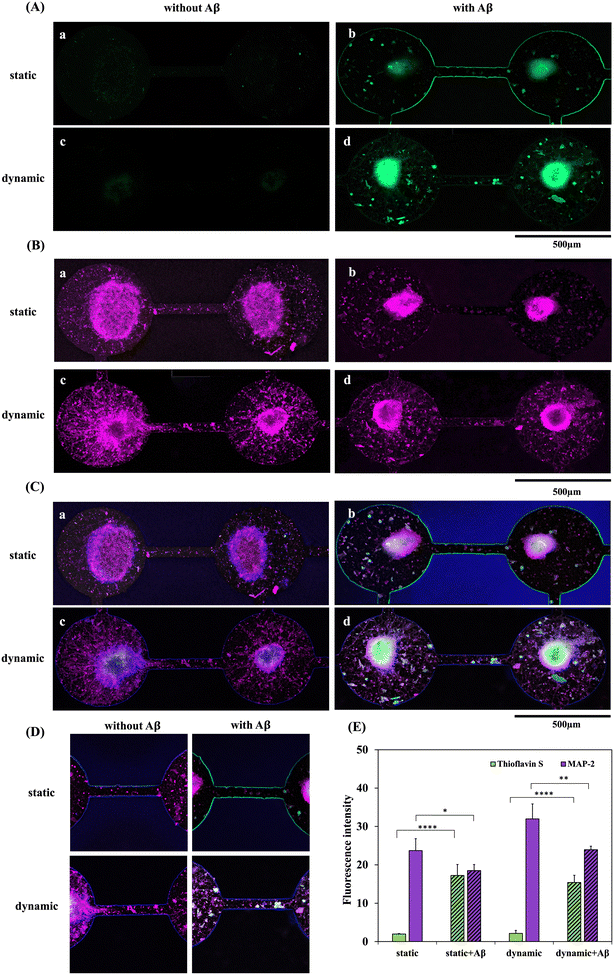 |
| Fig. 5 Immunostaining for thioflavin S and MAP2 expression was performed in both dynamic and static systems, with and without 5 μM Aβ incubation for a 3 day duration. (A) Immunofluorescence staining of thioflavin S (green) and (B) MAP2 (purple) expression in NSC spheroids-on-a-chip after 5 days of network formation in (a) a static system without Aβ addition, (b) a static system incubated with Aβ, (c) a dynamic system without Aβ addition and (d) a dynamic system incubated with Aβ for 3 days. (C) Merged image of thioflavin S (green), MAP2, and Hoechst. (D) Images of immunofluorescence staining in the channel. (E) Quantification of the fluorescence intensity of thioflavin S and MAP-2 expression. Asterisks denote significant differences where indicated (**p < 0.01, ***p < 0.005, and ****p < 0.001) as determined by Student's t test. | |
3.7. Comparison of synapse formation, synaptic functionality and ACh secretion in static and dynamic systems with/without Aβ addition
In addition to cytotoxicity and immunostaining, the functionality of neurites and the influence of the important neurotransmitter ACh affected by Aβ were also compared in static and dynamic systems. Fig. 6 shows the synapse immunostaining results and the ACh concentration after 5 days of culturing in the static/dynamic system and with/without 5 μM Aβ for another 3 days. Neuronal loss and neuronal function impairment are the main events that result in the irreversible progression of neurodegenerative diseases, including AD.33 Synaptic proteins are important for the normal functioning of synapses. The expression of the presynaptic protein synapsin-1 in differentiated neurons derived from NSCs was observed by immunohistochemical staining. Fig. 6(A)-a and c show the fluorescence of synapsin I in the static/dynamic system without Aβ incubation, demonstrating that the expression of synapsin I in the dynamic system is significantly higher than that in the static system. Additionally, the neurites around the neurospheroid and in the channel were solid and dense. Since the percentage of neuron differentiation in the dynamic system was significantly higher than that in the static system, the result of synaptic functionality was also consistent with the immunostaining. It is well established that the oligomeric forms of Aβ are neurotoxic as well as synapse-toxic.34 In the present study, we found that after incubation with Aβ, many cells demonstrated obvious apoptotic morphology. In contrast, as shown in Fig. 6(A)-b and d, after NSCs were incubated with 5 μM Aβ, synaptic expression showed a dotted and discontinuous pattern, meaning that the neurites were disconnected and fractured. Furthermore, Fig. 6(B) shows the quantification of the relative fluorescence intensity, which also demonstrated that the level of synapsin-1 was markedly reduced in the Aβ-treated groups, and the level in the dynamic system was also higher than that in the static system. Moreover, membrane dynamics of NSC in both static and dynamic systems with/ without amyloid-β addition were investigated for imaging vesicle recycling by using the membrane-impermeant dye FM1-43. The uptake of fluorescent molecules dependent on activity allows for the monitoring of presynaptic vesicle dynamics. This approach capitalizes on the endocytotic phase of activity-dependent rapid vesicular recycling, which is a unique characteristic of synapses, to load synaptic vesicles with fluorescent probes.35 Synaptic vesicle membranes were labeled using the FM1-43 lipid dye. Subsequent stimulation with a high potassium solution resulted in a reduction of the fluorescent intensity associated with the lipid dye. Remarkably, even after a second round of stimulation with a high potassium solution, conducted without the presence of the lipid dye, the decrease in fluorescence persisted. This phenomenon, depicted in Fig. 6(D) and the quantification result in (E), underscores the functionality and reusability of synaptic vesicles in both dynamic and static systems in the absence of Aβ addition. As demonstrated in Fig. 6(D), NSCs exhibited enhanced synaptic functionality in the dynamic system compared to the static system. This finding also supports our hypothesis that the dynamic system possesses the potential to induce NSCs to differentiate into neurons and enhance synaptic function when compared to the static system. Furthermore, the introduction of Aβ protein into both dynamic and static systems resulted in a diminished synaptic functionality. This observation is consistent with the results of Fig. 6(B). Furthermore, neurofilament heavy polypeptide (NEFH), an intermediate filament component, was used to investigate the neurofilament expression in both dynamic and static systems with/without Aβ addition. As depicted in Fig. 6(F)-a and c, the fluorescence labeling of MAP2 (purple) and NEFH (green) in the static and dynamic systems without Aβ incubation reveals a notably higher NEFH expression in the dynamic system compared to the static system. Moreover, in comparison to the static system, NEFH displays a more intricate and densely patterned expression in the dynamic system. This suggests that the dynamic system plays a role in fostering the development of a more resilient neural network. Conversely, as illustrated in Fig. 6(F)-b and d, following the incubation of NSCs with 5 μM Aβ, NEFH exhibits a punctate, fragmented, and discontinuous pattern.
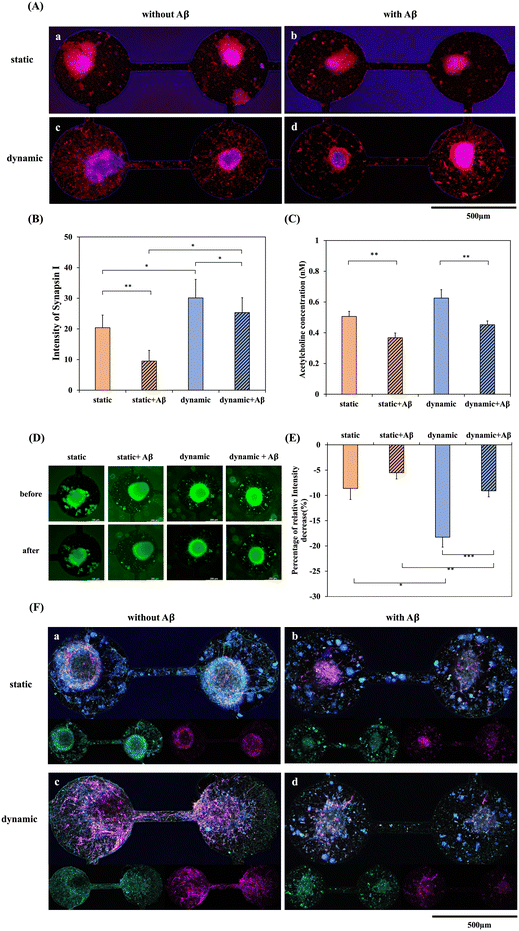 |
| Fig. 6 Immunostaining for synapsin I, NEFH expression and detection of synaptic functionality were conducted in both dynamic and static systems, both with and without 5 μM Aβ incubation, over a 3 day duration. (A) Immunofluorescence staining of synapsin I (red) expression in NSCs-on-a-biochip after 5 days of network formation in (a) a static system without Aβ addition, (b) a static system incubated with Aβ, (c) a dynamic system without Aβ addition and (d) a dynamic system incubated with Aβ for 3 days. The blue color corresponds to staining with DAPI for the nuclei. (B) Quantification of the fluorescence intensity of synapsin I expression. (C) Analysis of ACh release from NSC spheroids-on-a-chip in a static or dynamic system and incubated with/without 5 μM Aβ for 3 days. (D) Fluorescence photomicrographs depicting FM1-43 labeling of recycling synaptic vesicles within NSC spheroids-on-a-chip, under static and dynamic conditions, and incubated with or without 5 μM Aβ for a 3 day duration. (E) Quantification of the percentage of the relative fluorescence intensity decrease before and after stimulation. (F) Immunofluorescence staining of MAP2 (purple) and NEFH (green) expression in NSCs-on-a-biochip after 5 days of network formation in (a) a static system without Aβ addition, (b) a static system incubated with Aβ, (c) a dynamic system without Aβ addition and (d) a dynamic system incubated with Aβ for 3 days. Asterisks denote significant differences where indicated (*p < 0.05, **p < 0.01, and ***p < 0.005) as determined by Student's t test. | |
ACh is a neurotransmitter of importance in Alzheimer's disease. Dysfunctional ACh regulation in the brain causes neuropsychiatric disorders, including AD. It has been shown that the level of ACh receptors is reduced in AD and that cellular dysfunction and imbalance in neurotransmitter signaling could be responsible for the symptoms of AD.36Fig. 6(C) shows the quantification of ACh concentration after 5 days of culture with neural network formation in the static/dynamic system and with/without 5 μM Aβ addition for 3 days. The ACh concentration was downregulated in the Aβ-treated groups in both the static and dynamic systems, which represented a significant decrease compared with the groups without Aβ incubation. Therefore, this system developed a dynamic NSC-based chip for the brain environment. After Aβ addition, amyloid accumulation, cell apoptosis, ROS production, neurite dysfunction, and ACh neurotransmitter downregulation were all observed. These results demonstrated that the dynamic system may provide a better microenvironment that is close to in vivo conditions, which could supply reliable data for disease evaluation and drug discovery.
3.8. Real-time monitoring of impedance variation to track network connection and disconnection in static and dynamic systems
Investigation of neural network formation is an important issue in in vitro brain models. The tracking of neural damage and neurite disconnection in a degenerative nerve disease model will benefit drug discovery and the simulation of disease progression. Our previous study observed network formation by inverted microscopy, compared the impedance data from each pair of neurospheroids in a 3 × 3 chip, and defined a threshold of 40 kΩ for impedance analysis of neurite connections.1 Herein, we tracked the neural network connection of 3 × 3 NSC-based chips in both dynamic and static systems after 5 days of culture in real time. In addition, 3 consecutive days (24 h, 48 h, and 72 h) of impedance recording were used to track neurite dysfunction in both dynamic and static systems with/without 5 μM Aβ incubation. Before NSC spheroid seeding, we further validate the impact of Aβ accumulation on impedance measurements. As observed in Fig. S6,† the impedance variation caused by Aβ accumulation after 1 day, 2 days, and 3 days of incubation was minimal and did not significantly differ from the value observed in the medium only group. Then, the impedance of each pair of neurospheroids in the 3 × 3 chip was recorded. Fig. 7 illustrates the timeline of impedance recording, including the background (medium only), 0 h after NSC seeding, 3 days after NSC seeding in both dynamic and static systems, neural network formation after 5 days of culture in both dynamic and static systems, and neurite disconnection after 24, 48, and 72 h of Aβ incubation in both dynamic and static systems. It has been proven that the impedance is higher than 40 kΩ when NSCs are just seeded without network formation, and the values are reduced and lower than 40 kΩ when neural network formation occurs as the electrical connection is generated. Herein, impedance was monitored for 3 consecutive days after 5 μM Aβ incubation in both static and dynamic systems, and Aβ damage may affect the electrical connection and upregulate the impedance values over the threshold.
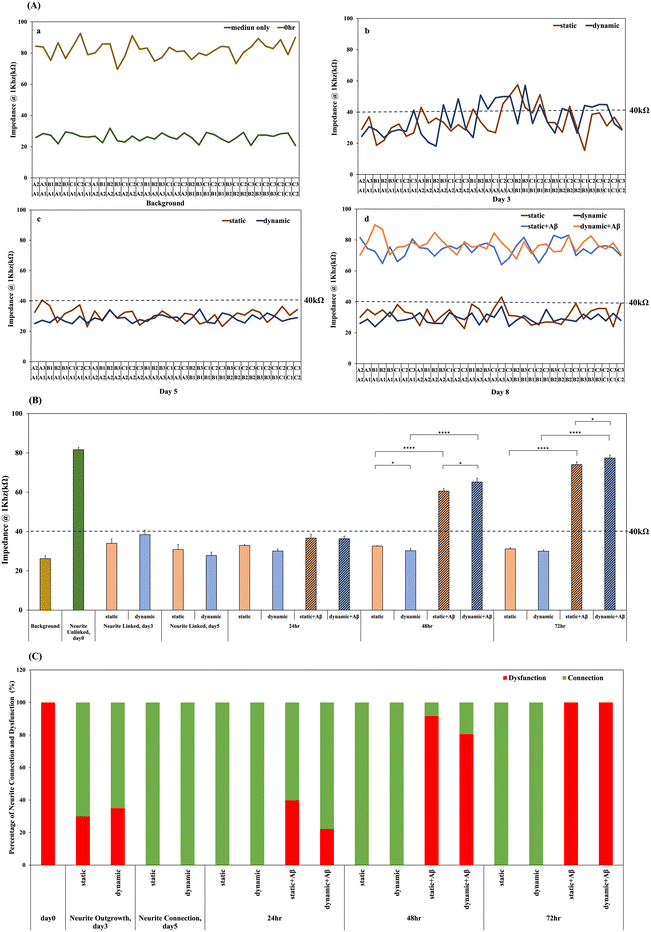 |
| Fig. 7 Continuous impedance analyses were carried out in both dynamic and static systems, both in the presence and absence of 5 μM Aβ incubation, spanning a 8 day duration. (A) Impedance analysis of neural network formation and dysfunction. (a) Analysis background impedance values between each pair of electrodes (green line means medium only and yellow line means NSC spheroid seeding at 0 h), (b) analysis impedance values between each pair of electrodes from NSC spheroids-on-a-chip in a static or dynamic system for 3 days of culture, (c) analysis impedance values between each pair of electrodes from NSC spheroids-on-a-chip in a static or dynamic system for 5 days of culture, and (d) analysis impedance values between each pair of electrodes from NSC spheroids-on-a-chip in a static or dynamic system for 5 days of culture and then incubated with/without 5 μM Aβ for another 3 days. (B) The impedance values determined for each pair of electrodes in a 3 × 3 array for the background (medium only), unconnected neurites, connected neurites, static or dynamic system and with/without 5 μM Aβ incubation for 24, 48, and 72 h. (C) The percentages of connected and disconnected neurites in the static system/dynamic system for 5 days of culture and then incubated with/without 5 μM Aβ for 24, 48, and 72 h. Asterisks denote significant differences where indicated (*p < 0.05 and ****p < 0.001) as determined by Student's t test. | |
As shown in Fig. 7(A), the impedance of each pair of NSC spheroids in the 3 × 3 NSC-based chip was recorded. As shown in Fig. 7(A)-a, the average impedance value of the medium only group was approximately 26.21 ± 1.5kΩ, and all of the values were lower than 40 kΩ. In addition, after seeding NSCs on the chip for 0 h, as shown in Fig. 7(A)-a, the average impedance value was approximately 81.62 ± 1.19 kΩ, and all of the values were obviously higher than 40 kΩ, which revealed that the network was connected. Fig. 7(A)-b shows the impedance records after 3 days of culture. Some of the impedance records were higher than 40 kΩ, and others were not, meaning that not all of the networks were connected in the 3 × 3 chip, which is consistent with our previous observation. In addition, Fig. 7(A)-b indicates that after 3 days of culturing, 70% of the recorded impedance values in the 3 × 3 neural network in the static system and 65% of those in the dynamic system were above 40 kΩ. In our previous studies, we know that the neurospheroid cultured in the SU-8 microwell within 3 days was differentiating and neurite outgrowing. It is suggested that there is no significant difference in the network formation rate in dynamic and static systems. Moreover, the neurites were growing and became attached to other adjacent neurospheroids within 5 days as shown in Fig. 7(A)-c, and complete neural network formation was observed in the 3 × 3 chip. Therefore, it was also demonstrated that after 5 days of culturing, 100% of the recorded impedance values in the 3 × 3 neural network in the static/dynamic system were below 40 kΩ, as shown in Fig. 7(A)-c, meaning that all of the neurites in each pair of neurospheroids were linked in the 3 × 3 chip in both the static and dynamic systems. In the static system, the average value was approximately 30.83 ± 2.65 kΩ, and in the dynamic system, the average value was approximately 27.82 ± 1.68 kΩ. Furthermore, after 5 days of culture and network formation to model the brain environment, 5 μM Aβ was added to both the static and dynamic systems for amyloid-β induced neuronal toxic model establishment, and the impedance variations for 24, 48, and 72 h were recorded. As shown in Fig. 7(A)-d, all of the impedance records in the 3 × 3 chip in both systems were 100% higher than 40 kΩ, indicating that 100% of neurites in the static/dynamic system were unlinked; in the static system, the average value was approximately 74.04 kΩ, and in the dynamic system, the average value was approximately 74.40 kΩ. Fig. 7(B) shows the average means of impedance before and after 5 μM Aβ addition. In the amyloid-β induced model, it was demonstrated that after incubation with 5 μM Aβ, the average impedance values increased as the incubation time increased. In addition, all of the impedance values in the 3 × 3 chip were transferred to the linked neurites (values below 40 kΩ) and the unlinked neurites (values over 40 kΩ), and the relative percentages are shown in Fig. 7(C). After incubation with 5 μM Aβ, the increasing percentage of neurite disconnection was related to the incubation time. After 24 h, the percentage of unlinked neurites in the static system was 39.82%, and that in the dynamic system was 22.22%; the percentages had increased to 91.66% in the static system and 80.55% dynamic system after 48 h, and had increased to 100% after 72 h of incubation. The impedance analysis performed in this study was consistent with biochemical measurements, which demonstrated a method to monitor neural network connections/disconnections in real time. The design of the microfluidic device used in this study provides a dynamic brain microenvironment model and real-time monitoring of neurite connections/disconnections, which could provide an alternative to drug discovery for different types of degenerative neural diseases.
4. Conclusion
This study successfully developed a dynamic neural disease model that consisted of a biomimetic brain environment, a neural network formation control, interstitial-level flow, and a real-time network disconnection monitoring system. The impedance analysis performed in this study was consistent with biochemical measurements. The comparison data between static and dynamic systems provide evidence that biomimetic systems with interstitial-level flow are more suitable for drug discovery and treatment evaluation.
Ethics approval and consent to participate
The animal experiments performed in this study were conducted in accordance with the recommendations of the Institutional Animal Care and Use Committee.
Author contributions
Professor I-Chi Lee spearheaded the conceptualization, experimental design, acquisition of funding, and played a substantial role in crafting the initial draft of the article. Furthermore, Professor Lee meticulously reviewed and edited the manuscript. Chu-Chun Liang executed the majority of the experiments, conducted investigations, carried out data visualization, and performed data analysis. Professor Po-Yen Chen reviewed and editing the article. Nien-Che Liu assisted in designing the dynamic system. All authors have read and approved the final manuscript.
Conflicts of interest
There are no conflicts to declare.
Acknowledgements
The authors acknowledge National Tsing Hua University and Chang Gung University for the financial support and summit project grants of the Ministry of Science and Technology, Taiwan (Project no.: MOST 110-2124-M-005-001-MY3, MOST 110-2314-B-007-001-MY3 and MOST 107-2314-B-182-017-MY3). This research project is supported by the summit project grants of the Ministry of Science and Technology, Taiwan (Project no.: MOST 110-2124-M-005-001-MY3, MOST 110-2314-B-007-001-MY3, and MOST 107-2314-B-182-017-MY3) and National Tsing Hua University. Additionally, the authors would like to acknowledge the equipment support from the lab of Dr. Kin Fong Lei and the Instrumentation Center, National Tsing Hua University, Taiwan. The authors are grateful for the support from the confocal imaging core in National Tsing Hua University, Taiwan, which is sponsored by the National Science and Technology Council (NSTC 112-2740-M-007-001-). We thank the technical support from Ms. Ya-Hsien Chou at the confocal imaging core in National Tsing Hua University. P.-Y. C. acknowledges the financial supports from the Start-Up Fund of the University of Maryland, College Park (KFS No.: 2957431), the MOST-AFOSR Taiwan Topological and Nanostructured Materials Grant under Grant No. FA2386-21-1-4065 (KFS No.: 5284212), and the UMD Grand Challenges Team Project Grant (KFS No.: 2957821).
References
- Y.-C. Liu, I. C. Lee and K. F. Lei, ACS Appl. Mater. Interfaces, 2018, 10, 5269–5277 CrossRef CAS PubMed.
- J. D. Lathia, M. P. Mattson and A. Cheng, J. Neurochem., 2008, 107, 1471–1481 CrossRef CAS PubMed.
- M. Götz and W. B. Huttner, Nat. Rev. Mol. Cell Biol., 2005, 6, 777–788 CrossRef PubMed.
- F. H. Gage, J. Neurosci., 2002, 22, 612–613 CrossRef CAS PubMed.
- E. T. Stoeckli, Development, 2018, 145, dev151415 CrossRef PubMed.
- K. Duval, H. Grover, L.-H. Han, Y. Mou, A. F. Pegoraro, J. Fredberg and Z. Chen, Physiology, 2017, 32, 266–277 CrossRef CAS PubMed.
- R. Prantil-Baun, R. Novak, D. Das, M. R. Somayaji, A. Przekwas and D. E. Ingber, Annu. Rev. Pharmacol. Toxicol., 2018, 58, 37–64 CrossRef CAS PubMed.
- Y. Li and K. A. Kilian, Adv. Healthcare Mater., 2015, 4, 2780–2796 CrossRef CAS PubMed.
- Alzheimer's Association, Alzheimer's Dementia, 2018, 14, 367–429 CrossRef.
- J. Cummings, G. Lee, T. Mortsdorf, A. Ritter and K. Zhong, Alzheimer's Dement.: Transl. Res. Clin. Interv., 2017, 3, 367–384 CrossRef PubMed.
- M. Frega, M. Tedesco, P. Massobrio, M. Pesce and S. Martinoia, Sci. Rep., 2014, 4, 5489 CrossRef CAS PubMed.
- J. Hardy and D. J. Selkoe, Science, 2002, 297, 353–356 CrossRef CAS PubMed.
- J. M. Rubio-Perez and J. M. Morillas-Ruiz, Sci. World J., 2012, 2012, 756357 Search PubMed.
- R. Sacco, E. Cacci and G. Novarino, Curr. Opin. Neurobiol., 2018, 48, 131–138 CrossRef CAS PubMed.
- C. Papadimitriou, H. Celikkaya, M. I. Cosacak, V. Mashkaryan, L. Bray, P. Bhattarai, K. Brandt, H. Hollak, X. Chen, S. He, C. L. Antos, W. Lin, A. K. Thomas, A. Dahl, T. Kurth, J. Friedrichs, Y. Zhang, U. Freudenberg, C. Werner and C. Kizil, Dev. Cell, 2018, 46, 85–101.e108 CrossRef CAS PubMed.
- Q. Gu, E. Tomaskovic-Crook, R. Lozano, Y. Chen, R. M. Kapsa, Q. Zhou, G. G. Wallace and J. M. Crook, Adv. Healthcare Mater., 2016, 5, 1429–1438 CrossRef CAS PubMed.
- Y. Zhang, H. Chen, X. Long and T. Xu, Bioact. Mater., 2022, 11, 192–205 CAS.
- T. Osaki, Y. Shin, V. Sivathanu, M. Campisi and R. D. Kamm, Adv. Healthcare Mater., 2018, 7, 1700489 CrossRef PubMed.
- K. Nichols, R. Koppes and A. Koppes, Curr. Opin. Biomed. Eng., 2020, 14, 42–51 CrossRef.
- M. Oñate, A. Catenaccio, G. Martínez, D. Armentano, G. Parsons, B. Kerr, C. Hetz and F. A. Court, Sci. Rep., 2016, 6, 21709 CrossRef PubMed.
- J. Park, I. Wetzel, I. Marriott, D. Dréau, C. D'Avanzo, D. Y. Kim, R. E. Tanzi and H. Cho, Nat. Neurosci., 2018, 21, 941–951 CrossRef CAS PubMed.
- J. Park, B. K. Lee, G. S. Jeong, J. K. Hyun, C. J. Lee and S.-H. Lee, Lab Chip, 2015, 15, 141–150 RSC.
- S.-A. Lee, D. Y. No, E. Kang, J. Ju, D.-S. Kim and S.-H. Lee, Lab Chip, 2013, 13, 3529–3537 RSC.
- J. Park, B. K. Lee, G. S. Jeong, J. K. Hyun, C. J. Lee and S. H. Lee, Lab Chip, 2015, 15, 141–150 RSC.
- D. Yoon No, K. H. Lee, J. Lee and S. H. Lee, Lab Chip, 2015, 15, 3822–3837 RSC.
- Y. J. Choi, S. Chae, J. H. Kim, K. F. Barald, J. Y. Park and S.-H. Lee, Sci. Rep., 2013, 3, 1921 CrossRef PubMed.
- I. C. Lee, Y. C. Wu, E. M. Cheng and W. T. Yang, J. Biomater. Appl., 2015, 29, 1418–1427 CrossRef CAS PubMed.
- I. C. Lee and Y. C. Wu, Colloids Surf., B, 2014, 121, 54–65 CrossRef CAS PubMed.
- K. F. Lei, I. C. Lee, Y. C. Liu and Y. C. Wu, Langmuir, 2014, 30, 14241–14249 CrossRef CAS PubMed.
- N. J. Abbott, Neurochem. Int., 2004, 45, 545–552 CrossRef CAS PubMed.
- Y. J. Choi, S. Chae, J. H. Kim, K. F. Barald, J. Y. Park and S. H. Lee, Sci. Rep., 2013, 3, 1921 CrossRef PubMed.
- J. Park, B. K. Lee, G. S. Jeong, J. K. Hyun, C. J. Lee and S.-H. Lee, Lab Chip, 2015, 15, 141–150 RSC.
- H.-L. Song, S. Shim, D.-H. Kim, S.-H. Won, S. Joo, S. Kim, N. L. Jeon and S.-Y. Yoon, Ann. Neurol., 2014, 75, 88–97 CrossRef CAS PubMed.
- N. L. Malinin, S. Wright, P. Seubert, D. Schenk and I. Griswold-Prenner, Proc. Natl. Acad. Sci. U. S. A., 2005, 102, 3058–3063 CrossRef CAS PubMed.
- W. J. Betz and G. S. Bewick, Science, 1992, 255, 200–203 CrossRef CAS PubMed.
- P. Sorrentino, A. Iuliano, A. Polverino, F. Jacini and G. Sorrentino, FEBS Lett., 2014, 588, 641–652 CrossRef CAS PubMed.
|
This journal is © The Royal Society of Chemistry 2024 |
Click here to see how this site uses Cookies. View our privacy policy here.