DOI:
10.1039/D3LC00714F
(Paper)
Lab Chip, 2024,
24, 2224-2236
Thermal segment microwell plate control for automated liquid handling setups†
Received
21st August 2023
, Accepted 14th February 2024
First published on 21st February 2024
Abstract
Automated high-throughput liquid handling operations in biolabs necessitate miniaturised and automatised equipment for effective space utilisation and system integration. This paper presents a thermal segment microwell plate control unit designed for enhanced microwell-based experimentation in liquid handling setups. The development of this device stems from the need to move towards geometry standardization and system integration of automated lab equipment. It incorporates features based on Smart Sensor and Sensor 4.0 concepts. An enzymatic activity assay is implemented with the developed device on a liquid handling station, allowing fast characterisation via a high-throughput approach. The device outperforms other comparable devices in certain metrics based on automated liquid handling requirements and addresses the needs of future biolabs in automation, especially in high-throughput screening.
1 Introduction
The evolution of automated liquid handling in biolabs has necessitated the development of miniaturized, integrated equipment. This paper introduces a thermal segment microwell plate control device, designed for high-throughput approaches in liquid handling setups. Central to this development is the drive towards standardization and integration in automated lab equipment, based on Smart Sensor and Sensor 4.0 concepts. These focus on enabling real-time monitoring, data visualization, condition monitoring, and adaptive control, significantly improving device reliability and efficiency.
Historically, after the introduction of liquid handling stations, additional equipment for those platforms has been introduced such as filtering stations, columns, shakers, pumps, transfer units, washers, cooling and heating devices, small footprint shakers, tip removal devices and specialized microscopes.1–6 This type of equipment is usually adapted to the size limitations inside of the liquid handlers' working area or are used adjacent to the liquid handlers. However, adjacent devices and physically large equipment have various negative side effects, such as pipettability problems, timing problems and parallelizability reduction. Thus, to reach the full potential of automated liquid handling experiments, small-scale devices are expedient for automated experiments in liquid handlers. Various small scale setups exist, relying on mini bioreactors, a variety of 96
384-well and 1536-well plates based screenings, organ on a chip setups and microfluidic applications which can all be used inside of a liquid handling setup.5,7–13
Automation-, system-, system component- and device developments in biolabs, such as specialized multi sensor based robotic biolab equipment has always been driven by the requirements of automated experiments to reach a high degree of automation and parallelization. Such equipment should be supported by the necessary hard- and software, which itself should also support multiple sensors working with well-established interface based communication technology.14–18 Academic literature has a few examples for the application of sensor networks and Smart Sensors in a biolab, which rely on a potpourri of systems, each equipped with sensors running in parallel forming a highly parallelized mesh of experiments.19–25
In modern biolabs, sensors and devices are subjected to a diverse array of requirements. Requirements for sensor-based devices, as identified by various authors, include a wide range of features. These features are error-free and highly accurate measurements, real-time data availability, maintenance-free sensorics, measurement without influencing the system, sensorics as retrofit, low costs, sterility, prevention of contamination spreading, robustness, inline capabilities, low cross-sensitivity, and the endurance in aqueous solutions.26,27
Experiments on automation platforms in biolabs that rely on microorganisms or enzymes are usually sensitive to temperature variations. Enzymes exhibit maximum activity and stability across a wide spectrum of temperatures from sub-zero temperatures to temperatures over 100 °C.28–34 Microbial growth and production, as well as stress responses, are also influenced by thermal conditions.35–38 This temperature dependency shows the importance of precise and consistent temperature control in automated biolab settings.
Section 2 explains the physical properties of the device and the used hard and software. Section 3 details the materials and methods for a case study in order to test the device's applicability. Section 4 presents the results of this case study, section 5 discusses the results, the device properties and performance and section 6 concludes this publication.
2 Thermal device: hard- and software components
The device described in this publication consists of three main parts: a thermal unit (Fig. 1 and S9†), a cooler (Fig. S10†) and a control unit (Fig. S11 and S12†). The thermal unit, detailed in section 2.1 and 2.2, provides the actual thermal heat transfer and measurements. The control unit's hardware and software, control algorithms, and digital interfaces, responsible for managing the thermal unit's operation, are described in sections 2.3, 2.4 and 2.5.
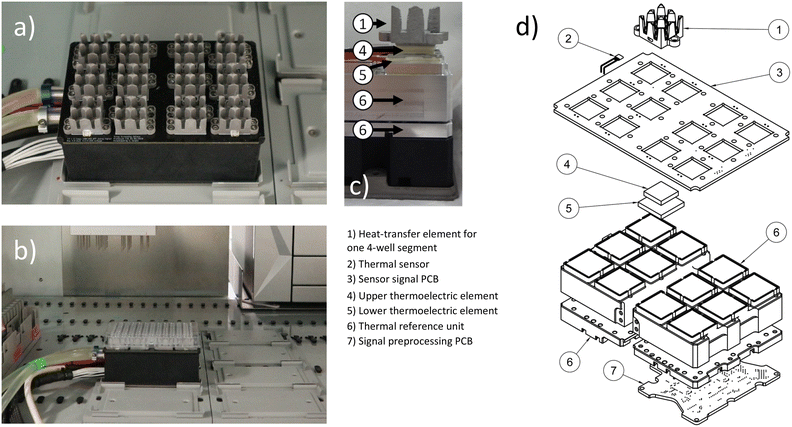 |
| Fig. 1 The thermal unit. a) Photograph of the thermal unit, showing the 12 independent heat transfer elements on top. The 12 aluminium elements connect to the indentations present in most 96-well plates b) photograph of the thermal unit carrying a 96-well plate. c) This photograph shows the layering of the functional elements. Labels 1) to 7) indicate components used in the assembly. For clarity, certain elements such as the top printed circuit board (PCB), sensors, and cables were omitted. d) The isometric exploded view drawing on the right shows the most relevant parts of the internal construction with one of the twelve measurement and actuator segments of one temperature control system. Each heat transfer element has its own thermal sensor. However, the drawing omits sensors, heat transfer elements, and TECs which do not belong to the one displayed measurement and actuator segment. Furthermore, the drawing does not include screws, cables, seals, protective caps, hull parts, spacers, structural components, and coolant connections. | |
2.1 Thermal device geometry
Automated liquid handling devices come with their own layouts or are usually centred around common biolab formats such as plate-based labware. Therefore, laboratory equipment developed for this type of environment should ideally conform to these dimensions. The thermal unit shown in Fig. 1 has the horizontal layout of a typical microwell plate or multiwell plate in its horizontal dimensions, and a height of 8 cm in its vertical dimension. Hence it fits into a liquid handler, such as the used Tecan EVO 200 platform for the liquid handling application. The thermal device part features 12 individual aluminium heat transfer elements. Once a 96-well plate is attached, each of the elements covers 4 wells from the bottom. Due to the nearly symmetrical design of each element, a consistent temperature is maintained across the wells covered by each element, ensuring no temperature shift occurs within one element. To minimize the influence of one four-well segment, covered by a single heat transfer element, on an adjacent four-well segment, an air gap equivalent to one row or column of wells is used as an insulation between the segments.
2.2 Thermal device hardware and capabilities
The thermal device (Fig. 1) is built up from a coolant liquid circulated central thermal reference unit. This unit provides a base temperature on which heat can be sunk into or sourced from. There are twelve stacks of thermoelectric elements (TEC), each consisting of one smaller TEC of type CP60131H (CUI DEVICES, United States) on top of a larger TEC of type CP60231H (CUI DEVICES, United States) on top of the thermal reference unit. On top of each stack of thermoelectric elements are aluminium elements for heat transfer to a 96-well plate. This setup enables a temperature range from −30 °C to 115 °C with 3 °C s−1 when heating and 0.8 °C s−1 when cooling for each thermal heat transfer unit. Once a microwell plate is pushed into the aluminium heat transfer elements, the temperature range for an oil covered water filled well is −20 to 100 °C with approximately 0.5 °C s−1 when heating and 0.2 °C s−1 when cooling. The given data is valid under maintained standard laboratory room conditions. Each of the aluminium heat transfer elements is equipped with one digital thermal sensor LMT01ELPGQ1 (Texas Instruments, United States). Additional sensors are placed in the thermal reference unit. Each sensor provides a temperature measurement range of −50 to 150 °C. The guaranteed accuracy by the vendor is ±0.5 °C within the range of −20 to 90 °C with a resolution of 0.0625 °C.39 For the control operation and temperature estimation in the wells of a filled 96-well plate, the temperatures in the heat transfer elements and thermal reference unit are measured, while the temperatures in the 96 well plate wells are estimated. During testing, each block of the thermal unit achieves high accuracy under quasi-steady-state conditions, for example, when maintaining constant temperatures over extended periods. However, the assumption of uniform temperature distribution in the wells is no longer valid during phases of fast temperature change. In instances where mixing does not occur, local thermal differences of up to 30 degrees Celsius were observed via PT100 measurements within individual wells of the 96-well plate. This was particularly noticeable during thawing operations, when the temperature was shifted from −20 °C to plus 100 °C.
2.3 Control unit hard and software architecture
The operation of the thermal unit's thermoelectric elements and sensors is driven via a hardware, software, and communication infrastructure (Fig. 2) in the control unit (Fig. S11 and S12†). In order to solve the given control problem in real time and to provide the necessary data for the Sensor 4.0 services, each of the tasks is run by a specific hardware component. The main low-level program runs on an F28386D microprocessor unit (MCU) (Texas Instruments, United States). The central processing unit 2 (CPU2) and control law accelerator (CLA) are used for control functionalities such as running model predictive control (MPC), prediction algorithms and simulations. The adaptive MPC (section 2.4) is implemented to control all twelve stacks of TECs (section 2.2) in parallel. Functions which are difficult to solve via raw computational power, are approximated with the CLA. The control output from CPU2 is passed on to CPU1, which runs a state machine to switch between stand-by, control, shut down, intermediate and test modes. CPU1 generates the control signals via twelve signal generators, which are each used to generate two synchronized pulse signals, and one directional signal for each of the 24 power control elements on four power control cards. The power bus provides a maximum of 1000 watts to the control cards. Each power control element enables a smoothly regulated voltage supply to a TEC of the twelve stacks of thermoelectric elements, providing controlled operations in both heating and cooling operations.
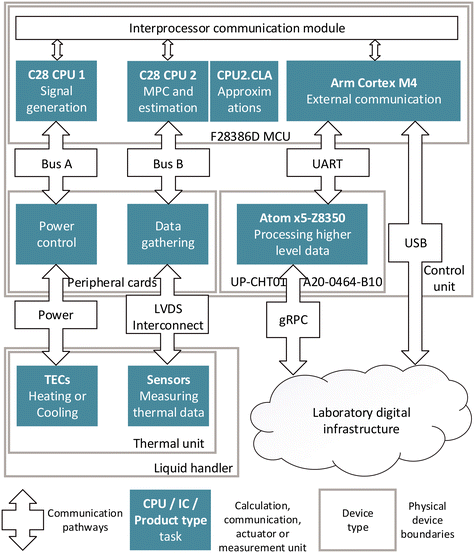 |
| Fig. 2 Physical lower level and higher-level communication infrastructure and protocols used by the control unit, the thermal unit in the liquid handler and the laboratory infrastructure. The schema shows the implemented structure used to execute the tasks, relayed via the laboratory infrastructure. The thermal unit as well as the control unit consists of various subsystems. The relayed tasks can either be processed via the F28386D MCU or the UP-CHT1 -A20-0464-B10 single board computer. Higher level communication tasks relayed to and from the laboratory infrastructure are processed by the Intel Atom processor while lower-level inputs are processed directly by the Arm Cortex M4. Data transfer to the laboratory digital infrastructure is established via ethernet cables or alternatively via USB. The main control operation is taken over by the F28386D MCU with its variety of cores and subcomponents. Data can be shared via the inter processor communication module and RAM between the cores. The necessary power conversion and sensor data acquisition is located on PCBs within the control unit. The components located in the liquid handling systems e.g. the thermal unit is connected via power- and sensor cables to the control unit. | |
CPU1 also initiates the signal measurement routines and passes the data to CPU2. The signal readout is performed using seven SN74LV8154MPWREP dual 16-bit counters (Texas Instruments, United States) which are accessed by the MCU.
For the external communication, the MCU utilizes an Arm Cortex M4. It enables bi-directional data transfer directly via universal asynchronous receiver transmitter (UART) protocol to an UP-CHT01-A20-0464-B10 single board computer (Aaeon, Taiwan), which is used for ethernet communication functionality. Alternatively, the Arm Cortex M4 allows communication directly via USB. Thus, the device offers a reliable and stable interface for data transfer for real time control, Sensor 4.0 and Smart Sensor features.
The DSP features, computational power and subcomponents of the F28386D MCU make it well-suited for the real-time control and signal processing application required for the given application.40
Low-voltage differential signalling (LVDS) is employed for communication between the control unit and the thermal unit. This choice is due to the interference-prone environment in an automated biolab, where data and power lines are in close proximity, and electric motors operate near the devices.
The described setup enables the fast execution of all measuring, control, and communication tasks, necessary for the operation of the device.
2.4 Computing capabilities close to the measurement
The capabilities of the MCU (section 2.3) used in the control unit, enables the use of computationally intensive control algorithms, such as the adaptive MPC, for the control of all TECs (section 2.2) in parallel. The adaptive MPC relies on a differential algebraic system of equations used to model the system behaviour and on temperature measurements above and below the stacked TECs, as both the heat transfer element and the thermal reference unit are equipped with sensors (Fig. 1). An MPC-based approach for controlling the thermal behaviour was chosen, due to the rather complex system behaviour, when stacking TECs of different size and performance. This approach enables operation while minimizing waste heat formation, maintaining similar temperature gradients in the stacked TECs and limiting the rate of voltage change as a side condition, and utilizing the whole allowable range of temperature overshoot and undershoot. Fig. 3c) shows the adaptive MPCs voltage output signal for one stack of TECs, using as much undershoot or overshoot temperature as the constraints allows for. However, as the related temperature plot Fig. 3b) shows, there is potential for further tuning of the used adaptive MPC, as the thermal measurement exceeds the overshoot limit for a few seconds during heat-up. The adaptive MPC approach allows for the adjustment of system variables during operation, including room temperature changes, changes in evaporation rate parameters, liquid addition, and removal of liquids from the wells during usage. This mechanism allows to compensate for the equivalent changes in laboratory conditions, to be considered in the devices control operation, allowing for the adjustment of the control output.
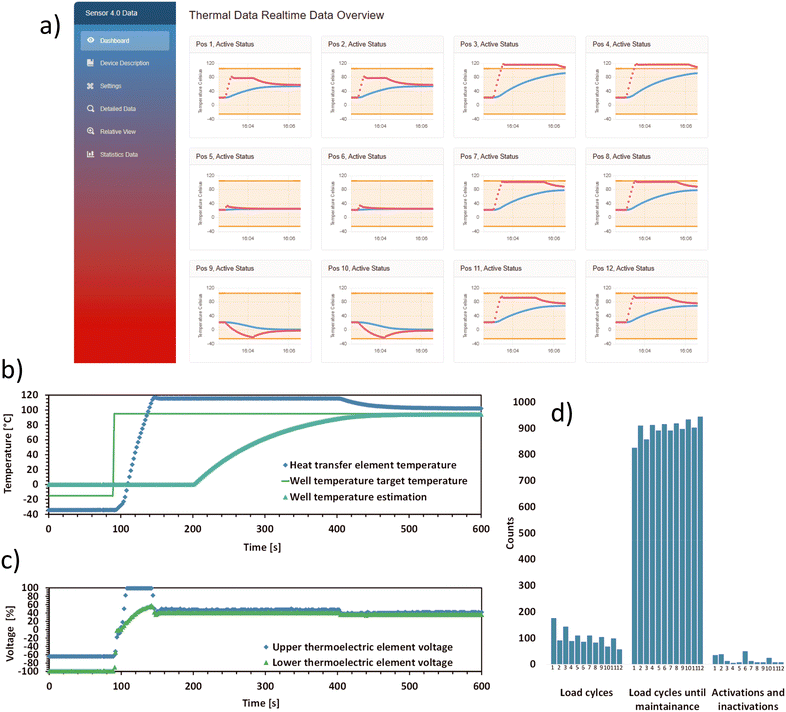 |
| Fig. 3 Real-time output of the thermal device such as data visualization, and condition monitoring as Sensor 4.0 features: a) Sensor 4.0 dashboard view from the graphical user interface (GUI), hosted via Blazor server. This view shows real-time thermal data over a 4 minute period, including the status (active/inactive) and position of heat transfer elements. Red dots represent measured heat transfer element temperatures, while blue dots represent estimated well temperatures. b) Heat transfer element and thermal reference unit data from a single heat transfer element (Fig. 1). The graph shows the measured temperature in the heat transfer element and the estimated well temperature. In the beginning, the graph shows a freezing or cooling down process. In the first 95 seconds, the wells' target temperature is −15 °C. The heat transfer element remains at −35 °C due to an over and undershoot temperature set at 20 °C. Water, set as the liquid in the well, is modelled to gradually transition to its solid phase. After 95 seconds the target temperature is set to 95 °C. The element rapidly heats up, causing the water–ice mixture to thaw and to eventually reach its target temperature. The shown estimated well temperature reflects this process. c) This graph shows the voltage controller output data used to generate the behaviour shown in b), used to power the stacked TEC elements via the control cards' control elements. The dots denote the applied percent of the maximum voltage for the smaller upper element and bigger lower element. It should be noted that the shown data was polled every three seconds, meanwhile the controller uses 150 ms timesteps between each measurement and control update. d) Estimated number of remaining load cycles for each TEC stack before the next maintenance is required. | |
The computational reserve of the MCU, after executing the MPC-related tasks, is sufficiently large to support Sensor 4.0 and Smart Sensor features, such as maintenance predictions (Fig. 3d)). This feature is important for planning the device's operation in an automated biolab.
2.5 Digital interfaces
The setup includes in total three communication interfaces. One for delivering the necessary Sensor 4.0 data via an ethernet-based interface, and two interfaces for bi-directional communication for automation, higher order control, scheduling, and orchestration via ethernet, or via USB (Fig. 2). The graphical user interface (GUI) as well as one of the direct control features are accessible via the local laboratory network, meaning that any personal computer or mobile device within the laboratory can view the data visualisation (Fig. 3). For efficient lightweight communication for the higher order control tasks, a standardized gRPC interface is utilized for data transfer, with Java Script Object Notation (JSON) nested inside of the message component. Using this setup has the advantage of being easily integrateable into other biolab systems and software and offers easy adjustability to the connecting server-side.
3 High-throughput enzyme assay study: materials and methods
The authors established a high-throughput experimentation setup to evaluate the developed thermal device. An enzymatic assay is selected as the test case to assess the performance of the thermal device in an automated liquid handling setup. Our study focuses on examining the temperature dependency of kinase activity based on the phosphorylation of nucleoside with ATP as phosphate donor. The aim is to acquire comprehensive data in a single experiment, utilizing a high-throughput approach. The experimental setup comprises two main components: an automated setup for assay execution, detailed in section 3.1, and a comprehensive system for data handling and communication, described in section 3.2. Section 3.3 describes the specifics of conducting an automated photometric enzyme assay. This assay is essential for validating the performance of the developed device, particularly in its application for temperature-sensitive enzymatic reactions. Lastly, section 3.4 offers a description of the underlying biochemical reactions involved in the study.
3.1 Automation device setup
The high-throughput screening setup, illustrated in Fig. 4, is utilized for the experiment. The central component of this setup is the Freedom EVO200 liquid handling robot (Tecan, Switzerland), which includes a MCA384 washstation extension (Tecan, Switzerland). Additional adjacent devices include a M1000 plate reader (Tecan, Switzerland), a Huber Minichiller 300 (Huber, Germany), as well as internally installed equipment such as the solvent washing station (in-house development) (Fig. S13†) and the previously described thermal device (in-house development). By strategically positioning all devices in close proximity within the main liquid handling station and moving the plates between the stations within the setup during the experiment, a sufficient experiment execution time for the high-throughput screening can be achieved.
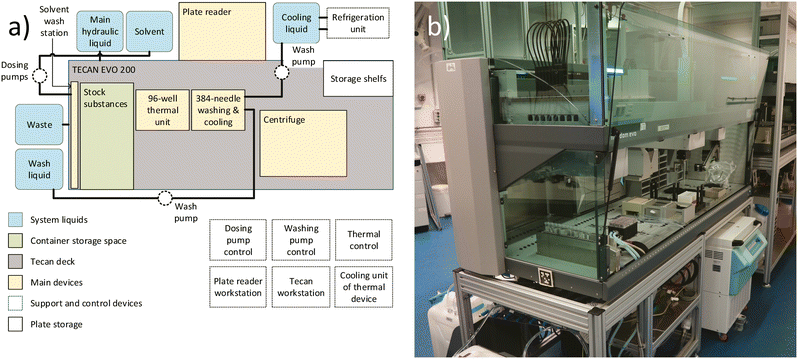 |
| Fig. 4 High-throughput screening setup. a) Schematic view of the setup used in the screening procedure. Central is the thermal unit of the thermal device described in this paper. The liquid handling and plate moving arms are not visible in the schematic b) photo of the setup, which is displayed schematically in the drawing a). Some parts, such as the refrigeration unit and tanks are not visible, as they are placed behind the liquid handling station. The three robotic arms are responsible for pipetting liquids and transporting plates. | |
3.2 Data handling and communication setup
Like the described communication setup for the thermal device (section 2.5), all other devices need the capability to communicate via the given laboratory digital infrastructure. All devices communicate using their native ethernet-based interfaces or, when not available, they use a middleware using gRPC for communication. All communication passes through a common extract, transform, load (ETL) layer in Apache NiFi (Apache Software Foundation, United States). The scheduling is executed by Cylc v. 8.0 software (CYLC). Orchestration for assigning values and temporary data storage is executed by an orchestrator (Inhouse development). Many different devices are used in parallel for this setup.
Since all devices have differing reliabilities, the orchestrator must coordinate failed steps and their repetition, which however may lead to slight variations in the time-length between certain steps.
3.3 High-throughput enzyme assay procedure
The high-throughput assay is based on measuring the concentration of the cosubstrate ATP during a nucleoside kinase catalyzed reaction.41–44 Regular samples are taken to determine the ATP concentration using a luminescence-based photometric assay.45 These measurements are used to gain information about the enzyme kinetics. An overview of the process schedule is given in Fig. 5.
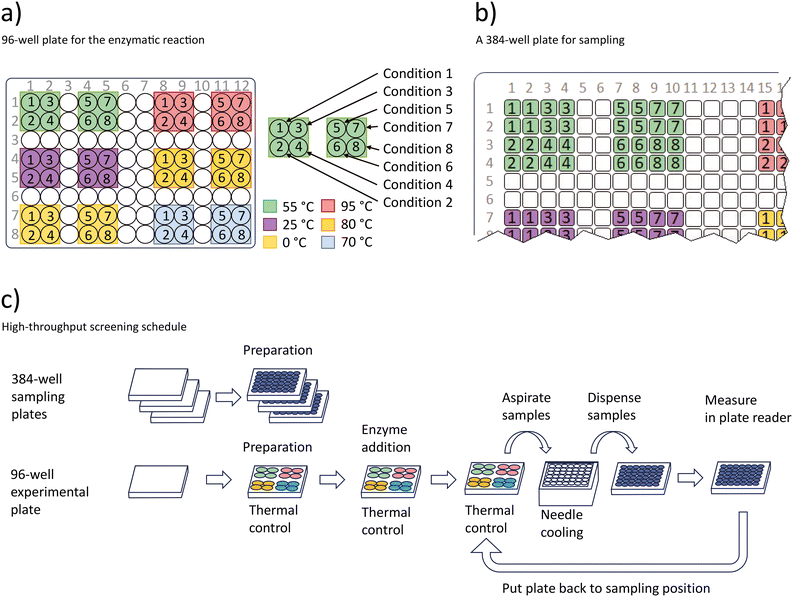 |
| Fig. 5 Procedure for the high-throughput enzymatic screening experiment. a) Conditions used in the temperature controlled 96-well plate. In total 12 temperature zones are used to set six temperatures and 8 different conditions specified in Table 1 b) three 384-well plates are prepared with the luminescence liquid from the assay kit to receive samples. The schema marks the positions of the 384 well plate that receive samples from the 96-well plate shown in a) as indicated by the numbers 1 to 8 and colours in a) and b). c) Workflow of the sampling procedure. | |
Table 1 Different conditions used for each temperature. Each condition lists the final concentration in each of the used wells of the used 96-well plate
|
Condition 1 |
Condition 2 |
Condition 3 |
Condition 4 |
Condition 5 |
Condition 6 |
Condition 7 |
Condition 8 |
pH value adjusted to 7.6 for each target temperature.
|
Puropose |
Control |
Control |
Control |
Activity test |
Activity test |
Activity test |
Activity test |
Activity test |
Tris-HCla |
70 mM |
70 mM |
70 mM |
70 mM |
70 mM |
70 mM |
70 mM |
70 mM |
MgCl2 |
2 mM |
2 mM |
2 mM |
2 mM |
2 mM |
2 mM |
2 mM |
2 mM |
ATP |
0.4 mM |
0 mM |
0.4 mM |
0.4 mM |
0.4 mM |
0.4 mM |
0.4 mM |
0.4 mM |
Thymidine kinase |
0 μg |
1.6 μg |
1.6 μg |
1.6 μg |
0.53 μg |
0.17 μg |
0.059 μg |
0.018 μg |
Thymidine |
0.33 mM |
0.33 mM |
0 mM |
0.33 mM |
0.33 mM |
0.33 mM |
0.33 mM |
0.33 mM |
To evaluate the thermal stability of the thymidine kinase, the kinase reaction and the luminescence-based photometric assay were prepared in parallel. The Kinase-Glo® luminescent kinase assay kit liquid (Promega, United States) is prepared by thawing and mixing the kit component. The buffer for the kinase reaction, prepared from TRIS, is 700 mM Tris-HCl (Carl Roth). The pH was adjusted to a value of 7.6 at 0 °C, 25 °C, 55 °C, 70 °C, 80 °C and 95 °C based on the buffer–temperature–pH relationship ΔpKa/°C = −0.031. For each of the six buffers, two variations are prepared: One variant without any ATP and one with 4 mM ATP from adenosine-5′-triphosphate disodium salt (Carl Roth, Germany) resulting in 12 stock buffers. Additionally, the buffers contain 20 mM MgCl2 (Carl Roth, Germany). 10 mM thymidine (TCI, Japan) is used as nucleoside. A thermostable thymidine kinase46 was produced and provided by BioNukleo and is prepared in dilutions of 1
:
3, 1
:
9, 1
:
27, 1
:
81 and 1
:
243. Other required substances are deionized water and silicone oil (IKA, Germany) with a viscosity of 50 mPas at 25 °C. These prepared substances are stored at −20 °C until the start of the experiment. To initiate the high-throughput screening procedure, all substances are organized in the stock substance section of the liquid handling setup, as illustrated in Fig. 4. This includes all buffer variants in 15 ml tubes, deionized water and luminescence kinase assay kit liquids in 100 ml trays, silicone oil in 25 ml high retention trays, and enzymes and thymidine in 1.5 ml Eppendorf tubes.
Three black 384-well plates (Thermo Fisher Scientific, United States) for luminescence measurement are stored in the storage shelfs. One 96-well F plate (Greiner, Austria) is placed onto the thermal unit.
The automated enzymatic assay starts by fetching plates from the storage shelfs and pipetting 10 μL luminescence liquid and 20 μL deionized water into each of the wells to be used of the 384-well sampling plate. Fig. 5 visualizes these wells in the 384-well plates with colors. A total of three plates require 579 wells for the sampling steps to be prepared. Next, the experiment plate is prepared. The substances which are to be pipetted into the experiment plate are detailed in Table 1. These are pipetted into the wells in the following order: deionised water, silicone oil, buffer, nucleoside, and finally, enzyme. Deionised water is used to fill up the aqueous phase in the wells to a volume of 200 μL. After pipetting silicone oil into the wells, the thermal control is started with an allowed over/undershoot temperature of 10 °C from the heat transfer element to well target temperature. Between the start of the temperature control and the enzyme addition, a minimum of 15 minutes has passed, due to intermediate pipetting steps and thus, the wells have reached the desired temperatures. Finally, once the enzyme is pipetted into the wells, the sampling procedure is executed twelve times consecutively. For each sampling step the modified 384 needle head takes 6 μl of sample from each of the 48 filled wells of the 96-well plate. The needles with the sampling liquid aspirated inside of the needles are placed into the 384 needle washing station. Coolant at 4 °C is pumped through the washing station for 30 seconds to bring all samples to the same temperature and to stop the enzyme-based conversion of the ATP to be measured. The samples are then transferred into one of the prepared 384-well sampling plates, containing the luminescent assay liquid and are mixed with the liquids in the wells. The 384-well sampling plate is then measured in the plate reader. The plate reader measures luminescence signals for 500 ms and uses an attenuation time of 250 ms per well. Subsequently the plate is returned to the deck. In total the sampling step is repeated 12 times for the used 48 wells of the 96-well experiment plate resulting in 12 data points for each of the 48 wells in the experiment plate and a total of 579 sampling data points within the complete experiment.
3.4 Enzyme catalyzed kinase and luminescent assay reaction description
The high-throughput assay described in section 3.3 is based on two reactions: One enzymatic nucleoside kinase reaction, which is to be characterized and modeled to obtain information about the enzyme behaviour and one luminescence reaction used in the assay, which is a well established method to measure the amount of ATP left in a kinase catalyzed reaction.
In the enzyme catalyzed kinase reaction, the cosubstrate ATP serves as phosphate donor for the phosphorylation of thymidine. The terminal phosphate group of ATP is transferred to thymidine, resulting in the formation of thymidine 5′-monophosphate (TMP) and adenosine 5′-diphosphate (ADP).44–47 Mg2+ is a required at very low concentrations as a cofactor for ATP.48–50 This reaction is dependent on various variables such as the enzyme concentration and the temperature, which are the variables varied in the experiment (Fig. 5).47
The luminescent assay is used to track ATP levels in the reaction. This is realized by regularly taking samples from the experiment plate and measuring ATP via the luminescent assay procedure (section 3.3). In the presence of the catalyst luciferase and Mg2+, luciferin reacts with ATP and oxygen.44–46 This reaction produces AMP, oxyluciferin, CO2, diphosphate, and light. The intensity of light is directly proportional to the amount of ATP in a the range 0–0.4 mM.45 This means that when samples from the experiment plate containing ATP are mixed with the luminescent assay liquids, a light signal is emitted. This signal can be detected using a plate reader, allowing for the straightforward calculation of the ATP concentration. The reaction is dependent on temperature and therefore the sampling process requires all samples from the experiment to be brought to a uniform temperature in order to ensure comparable measurement results.
Time series measurements of cosubstrate ATP can be used to determine the characteristics of the enzyme kinetics. Thus, the described high-throughput photometric enzyme assay procedure (section 3.3) can be used to determine the dependency of the reaction kinetics on the temperature and the enzyme concentration.
4 High-throughput enzyme assay study: results
In order to test the usability of the thermal device in an automated biolab setup, the described automated assay (section 3.3) was executed for a thermostable thymidine kinase. An overview of the significant results, the time-series data for various enzyme concentrations and temperatures is shown in Fig. 6. Higher conversion rates were observed at higher temperatures, with a maximum suspected at around 80 °C. Additionally, increased enzyme concentrations resulted in proportionally higher conversion rates.
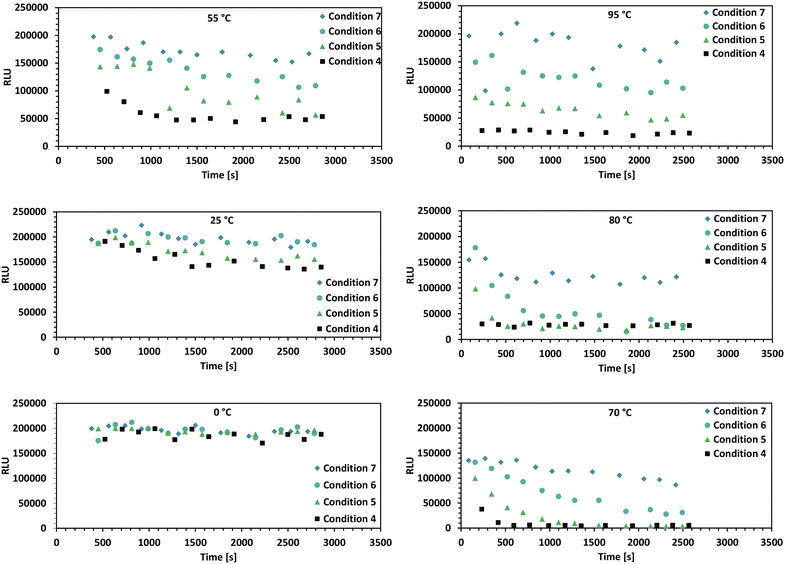 |
| Fig. 6 Plate reader luminescence measurements from the high-throughput enzyme assay described in section 3.3. This figure displays the most relevant time-series data for different reaction temperatures, ranging from 0 °C to 95 °C. The luminescence signal is directly proportional to the given ATP concentration. The shown conditions 4 to 7 in each plot are detailed in Table 1. The timepoints at which the enzyme is added to the wells of the 96-well plate denote the reaction starting time (t = 0). Since the enzyme can only be added one row at a time to the 96-well plate by the 8-needle head, the timespans between the start of the reaction and the first measurement are different for all datapoints. | |
A model-based approach was used for the evaluation of the data. The reaction kinetics can be described by a common multi substrate reaction equation dcATP/dt = −k1(T) cEnzymecATPcNucleoside (Table 2).51–53 This model or differential equation is slightly modified to include an ATP degradation term for higher temperatures.54,55 The results of a parameter estimation for the reaction rates k1(T) based on a regression using interior point algorithm in Matlab are displayed in Table 2 (Fig. S2 to S7†). According to the parameter estimation, the highest reaction rate k1(T) in the given data occurs at 80 °C. Furthermore, the model describes the data with the lowest residuum in the area 55–80 °C and with the highest residuum at 95 °C.
Table 2 Estimated reaction rate constant k1 modelled by a common multi-substrate reaction equation
(ref. 48–50) with an ATP degradation term for higher temperatures, based on a parameter estimation of the dataset represented in Fig. 6
Temperature |
0 °C |
25 °C |
55 °C |
70 °C |
80 °C |
95 °C |
Estimated reaction rate parameter k1(T) 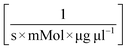 |
1.67 × 10−7 |
0.032 |
0.77 |
6.04 |
7.59 |
1.88 |
Squared 2-norm of the residual |
0.6088 |
0.6137 |
0.5927 |
0.5867 |
0.5909 |
0.6683 |
The different target temperatures in the 96-well plate were reached within the expected timeframe (Fig. S8†) and could also be maintained during the measurement timeframe, enabling the described high-throughput enzymatic assay.
5 Discussion
The following section discusses the thermal device development (section 5.1) as well as the results from the used case study (section 5.2).
5.1 Thermal device development
The motivation behind the development of the thermal device for temperature control of a 96-well plate was to create a test vehicle for Sensor 4.0 and Smart Sensor ideas,14,26,56–58 miniaturization and compartmentalization, integration into automation platforms, and standardization based on common platforms. Therefore, one of the objectives was to prioritize the use of a common 96-well plate, instead of specialized device-specific plates for temperature control. This approach enables researchers to use a common and widely available format.
In terms of design and application, the thermal device differs significantly from modern PCR cyclers. The purpose of the developed device in this paper is not PCR, but independent temperature control in individual well segments for experimentation on an automated liquid handling station. Thus, the development was based on a different design philosophy. The temperature range is higher, the elements are working more independent, the number of concurrent samples is lower, the plate format is not common for PCR, the size and shape of the device, as well as the weight is adjusted to automated liquid handling dimensions, and the temperature accuracy is worse.
Splitting up the device into three distinct subunits (thermal unit, control unit, cooling unit) reduces the size and complexity of each subunit, making it more accessible and modifiable for researchers, particularly in academic and small-scale automated high-throughput lab settings. The miniaturization and compartmentalization of these subunits facilitate efficient system integration. For example, the thermal unit is designed to be the same size as a standard microwell plate, allowing it to be integrated into a liquid handling system without excessive space requirements or strain on the platform. This improves the efficiency due to more efficient space use within the liquid handling setup. This approach not only optimizes space utilization within the liquid handling setup but also minimizes the manual labour involved in system integration, unlike the integration of larger, modern benchtop PCR cyclers which might necessitate physical cutouts in the liquid handler's deck.
Taking into account the described factors, it might be possible to view the presented device as potentially more useful in some specific automated liquid handling applications when compared to PCR cyclers available on the market today.
5.2 Discussion on high-throughput enzyme assay results
A maximal enzyme activity close to 80 °C may be assumed based on the given data and parameter estimation. However, the data for 95 °C may not be properly described by the used equation, as indicated by its larger residual deviation from the estimation (Table 2). The behaviour may be explained by enzyme denaturation at increased temperatures, which is a well described phenomenon for enzymes.32,59,60 If this phenomenon is taking place, then the addition of terms such as temperature dependent enzyme degradation rate constant multiplied with the enzyme concentration into the reaction kinetics model could result in a lower residuum in a parameter estimation for the given high temperature data. Using the enzyme degradation hypothesis, it is possible, that close to 100 °C, the reaction rate k1(T) is still proportional to the reaction temperature, however the enzyme is inactivated very fast by these harsh thermal conditions. Furthermore the slow decrease of the ATP signal over time in all plots with high temperatures can be attributed to ATP degradation by temperature.54,55
High temperature sampling was observed to be problematic, as the sampling data reveals existing outliers and increased noise (95 °C plot at Fig. 6). These phenomena are a result of volumetric errors during sampling, becoming more significant and frequent with temperature increase. Temperature related pipetting accuracy problems are known in literature.61,62 In the presented setup these volumetric errors may be due to a mixture of physical reasons, such as gas bubbles forming at the needle-tips and technical reasons or problems with the automation setup, such as leakage from the needle seals, which was observed prior to this specific experiment. It is believed by the authors that this problem can be partially mitigated with further modifications of the 384 needle pipetting head.
Oil (section 3.3) was used in the 96-well plate to prevent evaporation and condensate formation.63,64 Past tests with plastic, silicone, and aluminium covers at high temperatures proved to be less effective. Oil's insulating properties are advantageous in automated platforms using electrical conductivity and capacitance for liquid detection such as the used Tecan platform because the needles will detect aqueous liquids under the oil. However, oil can adhere to the used pipetting needles and is not easily removed in standard washing stations. Therefore, a specialized solvent washing station was developed and integrated for this screening experiment, ensuring standardized cleaning, and overcoming issues with oil residue accumulation observed in initial solvent tray tests.
The experiment preparation necessitated several manual procedures, as described in section 3.3. This included preparing 12 individually adjusted buffers, followed by their storage at −20 °C and subsequent thawing before the experiment. Such labour-intensive processes and large time frames may have introduced factors that have led to unforeseen outcomes. Notably, the low ATP concentrations observed in the 70 °C plot of Fig. 6 suggest a possible lack of ATP in the prepared buffer used for the experimentation at 70 °C. Simplifying the preparation steps may be essential to ensure the quality and consistency of further experiment results.
Although this experiment revealed some weaknesses in the process and experiment setup, it showed that the device is capable of running in a fully automated setup, which enabled a sampling, processing, and a measurement rate of approximately 700 samples per hour of 48 different conditions including six different temperatures for a single standard 96-well plate in a single liquid handling station.
6 Conclusion
In summary, the creation of a thermal device for microwell plate temperature control in automated liquid handling systems is progress in standardizing and automating lab equipment. This development emphasizes using Smart Sensor and Sensor 4.0 features, standard 96-well flat bottom multiwell plates over specialized ones and focuses on miniaturization and compartmentalization to fit standard well plate formats, simplifying system integration like incorporation into liquid handling robots. The thermal unit is lightweight to suit the liquid handling platform and offers a broad temperature range for various operations.
Considering the results of the enzyme characterisation or screening experiment with the implemented device inside of the automation platform, we conclude that the thermal device showed potential in high-throughput experimentation and especially in screening applications.
The device capabilities enable further developments including the integration of machine learning algorithms into the device's lower-level control system and by server-based applications via the higher-level communication layer, allowing for various control strategies and experiment setups.
The used data handling and communication setup and the data-handling capabilities of the thermal device used in this study enabled real-time gathering, assignment, and reorganization of all collected data. The setup could be further improved using online estimation and calculation tools. Thus, a possible future application could be the application of Bayesian optimization and optimal experimental design. These methods could be used in developing well-fitting models, locating maxima in reaction rates, and quantifying dependencies on factors like pH and ion concentration, as well as other variables affecting the enzymatic conversion of substrates.
Other potential future applications of the thermal device include small scale fermentation and investigation of heat shock responses.
Additionally, further integration of the devices with other developments could be explored. Since the device is only using the underside of a microwell plate, it enables easy access via the top side by typical biolab automation platforms. However, the current measurement strategies for a plate in the shown experiment is still at- or offline. Thus, further developments should allow for integration of future online measurement solutions which can be placed on top of the microwell plate but still allow pipetting.
Author contributions
Simon Seidel: conceptualization, design, acquisition, engineering: electronics and mechanical, methodology, assay implementation, control and modelling, testing, literature research, data analysis, visualization, writing. Katja F. Winkler: experiment design, assay conceptualization, result evaluation. Anke Kurreck: experiment design, result evaluation. Mariano Nicolas Cruz-Bournazou: supervision, resources, conceptualization, management. Sebastain Groß: supervision, design requirements. Katharina Paulick: supervision, spelling. Peter Neubauer: supervision, resources.
Conflicts of interest
Anke Kurreck is CEO of the biotech company BioNukleo GmbH.
Acknowledgements
We want to thank all current and former members of the HTBD and KIWI-Biolab group for their input and supervision. Special thanks to student Kevin R. R. Stegemann who helped with digitalisation and modelling procedures and laboratory work. We thank the Bundesministerium für Bildung und Forschung for providing funding through the funded research topic 'UfIB: Systemintegration einer standardisierten IT-Infrastruktur über den Produkt-Lebenszyklus' for development and engineering work.
References
- W. Ouyang, R. W. Bowman, B. Wang, K. E. Bumke, J. T. Collins, S. Ola, C.-P. Jordi and D. Benedict, Adv. Biol., 2022, 6, 2101063 CrossRef CAS PubMed
.
- J. Courtemanche, S. King and D. Bouck, SLAS Technol., 2018, 23, 448–455 CrossRef PubMed
.
- S. Rudnicki and S. Johnston, Curr. Protoc. Chem. Biol., 2009, 1, 43–54 CrossRef PubMed
.
- M. A. Torres-Acosta, G. J. Lye and D. Dikicioglu, Biochem. Eng. J., 2022, 188, 108713 CrossRef
.
- B. Haby, S. Hans, E. Anane, A. Sawatzki, N. Krausch, P. Neubauer and M. N. Cruz Bournazou, SLAS Technol., 2019, 24, 569–582 CrossRef CAS PubMed
.
- M. Bailly, C. Mieczkowski, V. Juan, E. Metwally, D. Tomazela, J. Baker, M. Uchida, E. Kofman, F. Raoufi, S. Motlagh, Y. Yu, J. Park, S. Raghava, J. Welsh, M. Rauscher, G. Raghunathan, M. Hsieh, Y.-L. Chen, H. T. Nguyen, N. Nguyen, D. Cipriano and L. Fayadat-Dilman, mAbs, 2020, 12, 1743053 CrossRef PubMed
.
- R. P. Hertzberg and A. J. Pope, Curr. Opin. Chem. Biol., 2000, 4, 445–451 CrossRef CAS PubMed
.
- D. A. Pereira and J. A. Williams, Br. J. Pharmacol., 2007, 152, 53–61 CrossRef CAS PubMed
.
- F. Kong, L. Yuan, Y. F. Zheng and W. Chen, SLAS Technol., 2012, 17, 169–185 CrossRef CAS PubMed
.
- Y. Zhu, Y.-X. Zhang, L.-F. Cai and Q. Fang, Anal. Chem., 2013, 85, 6723–6731 CrossRef CAS PubMed
.
- J. Hemmerich, S. Noack, W. Wiechert and M. Oldiges, Biotechnol. J., 2018, 13, 1700141 CrossRef CAS PubMed
.
- K. Anantanawat, N. Pitsch, C. Fromont and C. Janitz, BioTechniques, 2019, 66, 290–294 CrossRef CAS PubMed
.
- C. M. Leung, P. de Haan, K. Ronaldson-Bouchard, G.-A. Kim, J. Ko, H. S. Rho, Z. Chen, P. Habibovic, N. L. Jeon, S. Takayama, M. L. Shuler, G. Vunjak-Novakovic, O. Frey, E. Verpoorte and Y.-C. Toh, Nat. Rev. Methods Primers, 2022, 2, 33 CrossRef CAS
.
- A. Schütze, N. Helwig and T. Schneider, Sens. Sens. Syst., 2018, 1(7), 359–371 CrossRef
.
-
D. Juchli, in Smart Biolabs of the Future, Springer, 2022, pp. 147–174 Search PubMed
.
-
A. Ajith, A. Kaklauskas, T.-P. Hong and U. Singh, Smart Sensor Networks, 2021 Search PubMed
.
- B. Wang, Z. Wang, T. Chen and X. Zhao, Front. Bioeng. Biotechnol., 2020, 8, 7 CrossRef PubMed
.
-
S. Seidel, in Smart Biolabs of the Future, 2022, pp. 61–82 Search PubMed
.
- B. Wang, Z. Wang, T. Chen and X. Zhao, Front. Bioeng. Biotechnol., 2020, 8, 7 CrossRef PubMed
.
- N. Krausch, J. W. Kim, T. Barz, S. Lucia, S. Groß, M. C. Huber, S. M. Schiller, P. Neubauer and M. N. Cruz Bournazou, Biotechnol. Bioeng., 2022, 119, 3584–3595 CrossRef CAS PubMed
.
- S. Hans, B. Haby, N. Krausch, T. Barz, P. Neubauer and M. N. Cruz-Bournazou, Bioengineering, 2020, 7, 145 CrossRef CAS PubMed
.
- A. Kusterer, C. Krause, K. Kaufmann, M. Arnold and D. Weuster-Botz, Bioprocess Biosyst. Eng., 2008, 31, 207–215 CrossRef CAS PubMed
.
- L. Bromig, N. von den Eichen and D. Weuster-Botz, Bioprocess Biosyst. Eng., 2022, 45, 1927–1937 CrossRef CAS PubMed
.
- N. Von Den Eichen, M. Osthege, M. Dölle, L. Bromig, W. Wiechert, M. Oldiges and D. Weuster-Botz, Bioprocess Biosyst. Eng., 2022, 45, 1939–1954 CrossRef PubMed
.
- L. Dong, P. S. Ravaynia, Q.-A. Huang, A. Hierlemann and M. M. Modena, ACS Sens., 2020, 5(7), 2036–2043 CrossRef CAS PubMed
.
-
M. Bassler, M. Deilmann, W. Ens, F. Frenzel, M. Gerlach, J. Großmann, F. Grümbel, M. Heisterkamp, U. Kaiser, A. Lambrecht, M. Maiwald, R. Ohlenkamp, T. Pötter, P. Pyka, E. Roos, A. Schmidt, U. Schünemann, M. Theuer, A. Tulke and N. Weber, Technologie-Roadmap „Prozess-Sensoren 2027+“, Namur, 2021, pp. 1–63 Search PubMed.
- G. W. Hunter, J. R. Stetter, P. J. Hesketh and C.-C. Liu, Electrochem. Soc. Interface, 2021, 1–63 CAS
.
- J. Belehradek, Nature, 1954, 173, 70–71 CrossRef CAS PubMed
.
- A. L. Fink and A. I. Ahmed, Nature, 1976, 263, 294–297 CrossRef CAS PubMed
.
- R. K. SAiK, D. H. Gelfand, S. Stoffel, S. J. Scharf, R. Higuchi, G. T. HoRN, K. B. Mullis and H. A. Erlich, Science, 1988, 239, 487–491 CrossRef PubMed
.
- D. Georlette, V. Blaise, T. Collins, S. D'Amico, E. Gratia, A. Hoyoux, J.-C. Marx, G. Sonan, G. Feller and C. Gerday, FEMS Microbiol. Rev., 2004, 28, 25–42 CrossRef CAS PubMed
.
- L. D. Unsworth, J. van der Oost and S. Koutsopoulos, FEBS J., 2007, 274, 4044–4056 CrossRef CAS PubMed
.
- Y. Cao and Y. Wang, ChemCatChem, 2016, 8(17), 2740–2747 CrossRef CAS
.
- Z. T. Baumer and T. A. Whitehead, Science, 2021, 373, 391–392 CrossRef CAS PubMed
.
- A. W. Bartlett, New Phytol., 1909, 8, 310–316 CrossRef
.
- J. Běhrádek, Biol. Rev., 1930, 5, 30–58 CrossRef
.
- K. Isett, G. Hugh, H. Wayne and A. Ashraf, Biotechnol. Bioeng., 2007, 98, 1017–1028 CrossRef CAS PubMed
.
- V. A. Lyubetsky, O. A. Zverkov, L. I. Rubanov and A. V. Seliverstov, BioMed Res. Int., 2020, 2020, 1–10 CrossRef PubMed
.
-
Texas Instruments, LMT01-Q1 Device Description, 2016, vol. 1, p. 34 Search PubMed.
-
Texas Instruments, TMS320F2838x Device Description, 2019, vol. 1, p. 309 Search PubMed.
- P. Schelling, G. Folkers and L. Scapozza, Anal. Biochem., 2001, 295, 82–87 CrossRef CAS PubMed
.
- S. Lutz, J. Lichter and L. Liu, J. Am. Chem. Soc., 2007, 129, 8714–8715 CrossRef CAS PubMed
.
- B. Munch-Petersen, W. Knecht, C. Lenz, L. Søndergaard and J. Piškur, J. Biol. Chem., 2000, 275, 6673–6679 CrossRef CAS PubMed
.
- K. F. Hellendahl, M. Fehlau, S. Hans, P. Neubauer and A. Kurreck, Int. J. Mol. Sci., 2021, 22, 11558 CrossRef CAS PubMed
.
-
Promega, Kinase-Glo Luminescent Kinase Assay Plattform TB 372, Promega Corporation, USA, 2015, vol. 2 Search PubMed.
- K. F. Winkler, L. Panse, C. Maiwald, J. Hayeß, P. Fischer, M. Fehlau, P. Neubauer and A. Kurreck, J. Biol. Chem., 2023, 299, 104746 CrossRef CAS PubMed
.
- S. Kamel, I. Thiele, P. Neubauer and A. Wagner, Biochim. Biophys. Acta, Proteins Proteomics, 2020, 1868, 140304 CrossRef CAS PubMed
.
- M. Umekawa, A. Nishikawa, N. Isono and S. Karita, Sci. Rep., 2022, 12, 16991 CrossRef CAS PubMed
.
- C. Kanellopoulou, A. B. George, E. Masutani, J. L. Cannons, J. C. Ravell, T. N. Yamamoto, M. G. Smelkinson, P. D. Jiang, M. Matsuda-Lennikov, J. Reilley, R. Handon, P.-H. Lee, J. R. Miller, N. P. Restifo, L. Zheng, P. L. Schwartzberg, M. Young and M. J. Lenardo, J. Exp. Med., 2019, 216, 1828–1842 CrossRef CAS PubMed
.
- R. M. Biondi, B. Schneider, E. Passeron and S. Passeron, Arch. Biochem. Biophys., 1998, 353, 85–92 CrossRef CAS PubMed
.
-
S. Liu, in Bioprocess Engineering, Elsevier, 2017, pp. 297–373 Search PubMed
.
- D. Vasic-Racki, U. Kragl and A. Liese, Chem. Biochem. Eng. Q., 2003, 17(1), 3–14 Search PubMed
.
- A. A. Saei, C. M. Beusch, P. Sabatier, J. A. Wells, H. Gharibi, Z. Meng, A. Chernobrovkin, S. Rodin, K. Näreoja, A.-G. Thorsell, T. Karlberg, Q. Cheng, S. L. Lundström, M. Gaetani, Á. Végvári, E. S. J. Arnér, H. Schüler and R. A. Zubarev, Nat. Commun., 2021, 12, 1296 CrossRef CAS PubMed
.
- E. Leibrock, P. Bayer and H.-D. Lüdemann, Biophys. Chem., 1995, 54, 175–180 CrossRef CAS PubMed
.
- Y. Matsuura, M. Takehira, Y. Joti, K. Ogasahara, T. Tanaka, N. Ono, N. Kunishima and K. Yutani, Sci. Rep., 2015, 5, 15545 CrossRef CAS PubMed
.
-
R. Werthschützky, B. Bock, P.-G. Paul-Gerald, K. Ettrich, T. Fröhlich, V. Graf, V. Große, F. Hänschke, H.-D. Hartmann, D. Hoffmann, K.-P. Hofmann, T. Ortlepp, F. Schmidt, A. Schütze, T. Simmons, W. Sinn, R. Slatter, T. Hannes, G. Tschulena, J. Wilde and G. Zieger, Sensor Technologien 2022, AMA Verband für Sensorik und Messtechnik e.V., 2018 Search PubMed.
- T. Kalsoom, N. Ramzan, S. Ahmed and M. Ur-Rehman, Sensors, 2020, 20, 6783 CrossRef CAS PubMed
.
-
V. Großer, D. Heydenbluth, R. Moos, D. Rein, J. Suerer, T. Simmons, W. Sinn, R. Werthschützky and J. Wilde, Sensor Trends 2014, AMA Verband für Sensorik und Messtechnik e.V., 2010 Search PubMed.
- N. A. Turner and E. N. Vulfson, Enzyme Microb. Technol., 2000, 27, 108–113 CrossRef CAS PubMed
.
- R. M. Daniel, Enzyme Microb. Technol., 1996, 19, 74–79 CrossRef CAS
.
- F. Millet and T. Barthlen, Nat. Methods, 2007, 4, iii–iv CrossRef CAS
.
- Z. Shang, X. Zhou, C. Li and S.-B. Tsai, Sci. Rep., 2018, 8, 5757 CrossRef PubMed
.
- I. Aranberri, B. P. Binks, J. H. Clint and P. D. I. Fletcher, Langmuir, 2004, 20, 2069–2074 CrossRef CAS PubMed
.
- J. H. Clint, P. D. I. Fletcher and I. T. Todorov, Phys. Chem. Chem. Phys., 1999, 1, 5005–5009 RSC
.
|
This journal is © The Royal Society of Chemistry 2024 |
Click here to see how this site uses Cookies. View our privacy policy here.