DOI:
10.1039/D4LC00546E
(Paper)
Lab Chip, 2024,
24, 4974-4988
Engineered cortical microcircuits for investigations of neuroplasticity†
Received
26th June 2024
, Accepted 1st September 2024
First published on 3rd September 2024
Abstract
Recent advances in neural engineering have opened new ways to investigate the impact of topology on neural network function. Leveraging microfluidic technologies, it is possible to establish modular circuit motifs that promote both segregation and integration of information processing in the engineered neural networks, similar to those observed in vivo. However, the impact of the underlying topologies on network dynamics and response to pathological perturbation remains largely unresolved. In this work, we demonstrate the utilization of microfluidic platforms with 12 interconnected nodes to structure modular, cortical engineered neural networks. By implementing geometrical constraints inspired by a Tesla valve within the connecting microtunnels, we additionally exert control over the direction of axonal outgrowth between the nodes. Interfacing these platforms with nanoporous microelectrode arrays reveals that the resulting laminar cortical networks exhibit pronounced segregated and integrated functional dynamics across layers, mirroring key elements of the feedforward, hierarchical information processing observed in the neocortex. The multi-nodal configuration also facilitates selective perturbation of individual nodes within the networks. To illustrate this, we induced hypoxia, a key factor in the pathogenesis of various neurological disorders, in well-connected nodes within the networks. Our findings demonstrate that such perturbations induce ablation of information flow across the hypoxic node, while enabling the study of plasticity and information processing adaptations in neighboring nodes and neural communication pathways. In summary, our presented model system recapitulates fundamental attributes of the microcircuit organization of neocortical neural networks, rendering it highly pertinent for preclinical neuroscience research. This model system holds promise for yielding new insights into the development, topological organization, and neuroplasticity mechanisms of the neocortex across the micro- and mesoscale level, in both healthy and pathological conditions.
Introduction
The underlying microcircuit motifs and architectures of neural networks play a pivotal role in facilitating efficient information processing, transfer, and storage within the brain.1 However, our understanding of how these structural motifs that emerge during brain development contribute to efficient neural computations in physiological conditions, or influence the effect of pathological perturbations, remains incomplete. This knowledge gap is, in part, attributed to the inherent challenges of studying brain network dynamics at the micro- and mesoscale level in vivo. Engineered neural networks offer a complementary approach to in vivo models by enabling investigation of neuroplasticity in a controlled microenvironment in vitro. Engineered neural networks are based on the inherent property of dissociated neurons to self-organize over time into complex computational systems, recapitulating fundamental characteristics of brain networks.2,3 This process occurs under the influence of various chemically and physically regulated guidance cues from the microenvironment that operate in a spatiotemporal manner in tandem with inherent self-organizing properties of neurons and their spontaneous electrical activity.4–7 The presence of topological cues within the neurons' microenvironment is highly relevant for the emergence of microcircuit motifs and architectures that mimic the modular organisation of neural circuits of interest, thereby further improving the physiological relevance of the model. Current technologies, such as microfluidics, enable precise manipulation of network topology. Such platforms promote establishment of modular networks, where populations of neurons are segregated into distinct chambers connected by micrometer-sized tunnels accessible only to their neurites.8 Recent studies have shown the importance of modularity in inducing complex dynamics, balancing integrated and segregated activity akin to that seen across brain regions in vivo.9–12 By implementing geometrical constraints within the microtunnels, it is furthermore possible to precisely control the direction of axonal outgrowth between the distinct populations of neurons.13–17 This approach, in combination with microelectrode array (MEA) interfaces enabling electrophysiological recordings, can be utilised to recapitulate and study the feedforward microarchitectures observed in many brain areas. In this way, anatomically relevant microcircuits, such as the cortical–hippocampal projection, can be established, thereby providing a robust methodology for studying neural network function and dysfunction in a controlled setting.11,18
A better understanding of how the underlying structure of neural networks shapes their dynamics is critical for deciphering physiological behaviours, as well as network responses to pathological perturbations.19 Graph theory has emerged as a prominent mathematical approach for gleaning meaningful insights into neural network connectivity and function.20,21 Within this framework, several hallmarks of efficient neural network organization have been identified, including small-world properties with high local clustering and short path length between nodes, and a modular, hierarchical network architecture that promotes efficient information flow between distinct parts of the network.21,22 Furthermore, efficient neural circuits typically feature a small number of highly interconnected hubs, which are critical for integrating information across distinct network regions.23 These topological traits, believed to be genetically encoded in the neurons for instructing neural self-organization, are hypothesized to minimize the metabolic cost of the network while maintaining efficient information flow.24–26 Analyzing and recognizing such traits can provide insights into the computational complexity of neural networks in both in vivo and in vitro settings.27–30
Neocortical microcircuits, characterized by their well-defined, layered input/output structure, is a compelling system to engineer using modular, directional microfluidic platforms.31 The neocortex represents one of the most intricate structures in the human brain, being responsible for a broad spectrum of functions, ranging from storage of working memory and predictive coding to various cognitive and sensorimotor tasks.32–36 While exhibiting diverse functions across distinct cortical regions, the entire neocortex is theorized to comprise canonical elementary processing units known as minicolumns.37–40 The precise function of such minicolumns in cortical processing is still under debate, yet they are believed to represent fundamental units of cortical organization. Furthermore, cortical networks exhibit complex spatiotemporal activity patterns during development, even in the absence of external input, both in vivo and in vitro.2,3,13,41–44 Additionally, cortical circuits demonstrate a remarkable ability to undergo plasticity changes during development and in response to external stimuli or damage.45–47 Thus, the layered structural organization, intricate spontaneous functional dynamics and extensive plasticity of neocortical microcircuits render them compelling motifs for investigation under controlled conditions in vitro, offering valuable insights into the structure–function relationships of such circuits.
In this study, we present an advanced microfluidic MEA model for recapitulating the feedforward, hierarchical architecture of the neocortex in vitro. Furthermore, we demonstrate the utility of this model system for studying network plasticity in response to selective perturbation of hub nodes that are central for information flow between higher and lower nodes in the structured hierarchy. We evaluate changes in the networks' information processing by analyzing both spontaneously evoked and stimulation-induced activity and apply connectomics and graph theory to study alterations in information flow within the networks. We thus present an engineered cortical microcircuit model that facilitates the investigation of developmental network dynamics, as well as its behaviour under physiological and pathological conditions.
Materials and methods
Design & fabrication of microdevices
The design of the microfluidic MEA was created using Clewin 4 (WieWeb Software, Enschede), as depicted in Fig. S1.† The platform was organized hierarchically into four distinct layers with a feedforward architecture consisting of 12 interconnected chambers, referred to as nodes. Each node had a diameter of 3.5 mm. Two and two nodes were interconnected by 20 microtunnels, each 350 μm long, 10 μm wide and 5 μm high. These channels had geometries inspired by the Tesla valve to induce unidirectional axonal outgrowth between the nodes.13 Additionally, spine structures were integrated on the postsynaptic side to misguide any outgrowing axons trying to enter the tunnels in the unintended direction.17 The MEAs were engineered to be compatible with a MEA2100 workstation from Multichannel Systems. Electrodes, measuring 2 mm in length and 10 μm in width, were strategically positioned across each channel area, as well as at the entrances and exits of each tunnel area, to monitor all activity propagation between the nodes. Furthermore, two electrodes were placed in each of the nodes in layer 1 and layer 4, with an additional electrode positioned in node 4. All electrodes were functionalized with a thin layer of highly nanoporous platinum to enhance their signal-to-noise ratio. A reference electrode was also positioned in each of the 12 nodes, with all reference electrodes connected to the same channel on the recording system. The fabrication of all microelectrode arrays followed our recently reported protocol.13 Six separate MEAs were used for the cell experiments.
Coating, cell plating and maintenance
An overview of the experimental timeline is depicted in Fig. 1A. Prior to coating, all interfaces underwent sterilization in UV light for a minimum of 1 h. Subsequently, the samples were soaked in DMEM, low glucose (Gibco™, 11885084) for at least 48 h to remove any potentially toxic, uncured PDMS left in the microfluidic chips. The interfaces were then coated with 0.1 mg ml−1 poly-L-ornithine solution (PLO) (Sigma-Aldrich, A-004-C) overnight in a fridge at 4 °C. The following day, all chambers were washed three times with Milli-Q (MQ) water to remove unattached PLO, before coating the surfaces with a laminin solution consisting of 16 μg mL−1 natural mouse laminin (Gibco™, 23017015) diluted in phosphate-buffered saline (PBS, Sigma-Aldrich, D8537) at 37 °C, 5% CO2 for 2 h. To ensure flow of the coating solution through the microtunnels, a hydrostatic pressure gradient was established between the chambers by filling them with varying amounts of the solution.
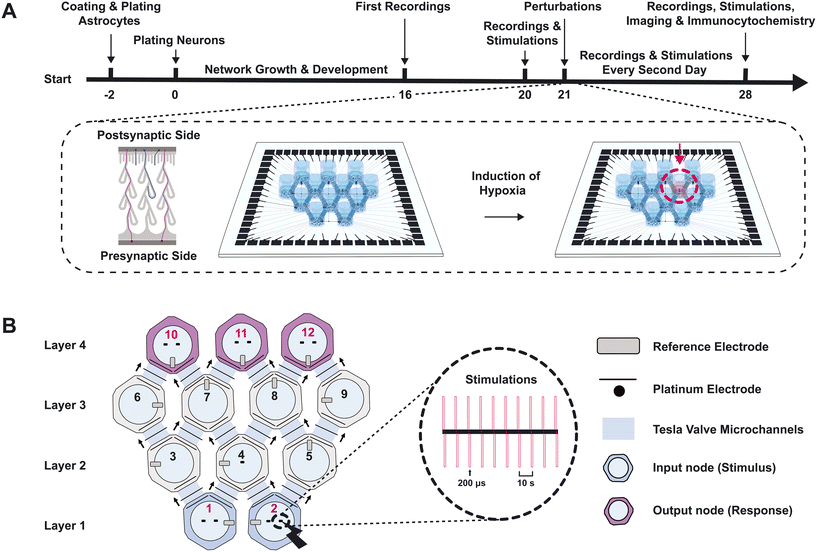 |
| Fig. 1 Experimental setup. (A.) Experimental timeline for cell experiments. An astrocytic feeder layer was plated two days prior to plating of neurons. Baseline recordings were conducted at 16 and 20 days in vitro (DIV). At 21 DIV, localized hypoxia was induced in one of the central nodes in layer 3 using CoCl2. Electrophysiological recordings were subsequently conducted every second day until 28 DIV. (B.) Schematic illustrating the organization of the 12 nodes, arranged into four distinct layers containing 2, 3, 4, and 3 nodes, respectively. Stimulations were administered following the recordings of spontaneously evoked activity from 20 to 28 DIV. Each stimulation session consisted of 10 consecutive spikes with a 10 s interspike interval and was applied to all input and output nodes (nodes 1, 2, 10, 11 & 12) in increasing order based on their node numbers. The illustrations are not drawn to scale. | |
An astrocytic feeder layer was plated two days prior to plating of neurons. Astrocyte medium consisted of DMEM, low glucose supplemented with 15% fetal bovine serum (Sigma-Aldrich, F9665) and 2% penicillin–streptomycin (Sigma-Aldrich, P4333). Rat astrocytes (Gibco™, N7745100) were plated at a density of 50 cells per mm2, equivalent to 750 cells per culturing chamber. The cells were allowed to expand for two days before the plating of neurons. The astrocyte medium was subsequently replaced by neuronal medium consisting of Neurobasal Plus Medium (Gibco™, A3582801) supplemented with 2% B27 Plus (Gibco™, A358201), 1% GlutaMax (Gibco™, 35050038) and 2% penicillin–streptomycin (Sigma-Aldrich, P4333). 0.1% Rock Inhibitor (Y-27632 dihydrochloride, Y0503, Sigma-Aldrich) was included in the medium during plating to enhance cell viability. Sprague Dawley rat cortical neurons (Gibco, A36511) were plated at a density of 500 cells per mm2, i.e., 7500 cells per chamber. Following this, half the cell medium was replaced with fresh neuronal medium at 4 h and 24 h after plating. Subsequently, the medium was replaced every second day throughout the experimental period. All electrophysiology experiments were conducted using cells from the same batches and cell vials.
Immunocytochemistry
For immunocytochemistry, cells were plated in microfluidic platforms bonded to glass coverslips (VWR International, 24 × 24 mm No. 1 Menzel-Gläser). Prior to fixation, cells were washed with PBS to remove debris. Fixation was performed for 15 min at room temperature using glyoxal solution comprising 20% absolute ethanol (Kemetyl, 100%), 8.0% glyoxal solution (Sigma-Aldrich, 128465), and 1% acetic acid (Sigma-Aldrich, 1.00063) in MQ-water.48 Following fixation, the cells underwent three consecutive PBS washes, each lasting 15 min. Subsequently, cells were permeabilized with 0.5% Triton-X (Sigma-Aldrich, 1086431000) diluted in PBS, followed by two additional PBS washes to remove excess Triton-X. The cells were then blocked with a solution consisting of 5% goat serum (Abcam, ab7481) diluted in PBS and incubated at room temperature on a shaking table at 30 rpm for 1 h. Primary antibody solutions, prepared in PBS with 5% goat serum as well as antibodies at concentrations listed in Table 1, were added to the cells. The cultures were then incubated overnight on a shaker table at 30 rpm at 4 °C. The following day, cells were rinsed three times with PBS for 15 min each, followed by incubation with 0.2% secondary antibodies diluted in PBS and 5% goat serum at room temperature for 3 h on a shaker table at 30 rpm. Prior to application, the secondary antibody solution was centrifuged at 6000 rpm for at least 15 min to remove precipitates. Subsequently, 0.1% Hoechst (Abcam, ab228550) diluted in PBS was added to the cultures, and the cultures were incubated for an additional 30 min on the shaker table. Before imaging, all cultures underwent three PBS washes followed by two rinses in MQ water.
Table 1 Antibodies and concentrations used for immunocytochemistry
Marker |
Catalogue number |
Concentration |
All antibodies were purchased from Abcam. |
GFAP |
Ab7260 |
1/1000 |
NeuN |
Ab279295 |
1/500 |
Neurofilament heavy |
Ab4680 |
1/5000 |
PSD95 |
Ab13552 |
1/250 |
Synaptophysin |
Ab32127 |
1/500 |
Viral transductions for structural characterization
To induce ubiquitous expression of GFP and mCherry within distinct nodes, viral transductions were performed using an AAV 2/1 serotype construct containing either pAAV-CMV-beta globin intron-EGFP-WPRE-PolyA or pAAV-CMV-beta globin intron-mCherry-WPRE-PolyA plasmids driven by a CMV promoter. The viral vectors were produced in-house by Rajeevkumar Raveendran Nair at the Viral Vector Core Facility, NTNU. Transductions were conducted at 9 DIV (n = 4). Initially, 3/4 of the neuronal media in the nodes located in layers 1 and 3 of the 12-nodal microfluidic chips were aspirated, and viruses for expression of GFP were introduced at a concentration of 5e2 viruses per cell. After a 3 h incubation period, the transduced nodes were replenished with fresh media. Subsequently, 3/4 of the media in the nodes situated in layers 2 and 4 were removed, and viruses for expression of mCherry were applied at a concentration of 5e2 viruses per cell. Following another 3 h of incubation, these node were also replenished with fresh media. Network imaging was conducted at 21 DIV (prior to induction of hypoxia) and at 28 DIV.
Quantitative analysis of structural unidirectionality was not conducted due to the inherent limitations of such methods. While this type of analysis can offer valuable insights into network structure, it may not capture all neuritic processes, as factors such as varying axon diameters, neurite growth across multiple layers, and multiple collaterals from a single axon can significantly bias fluorescence intensity. Consequently, we opted to focus on quantitative analysis of the functional network profile rather than the structural one.
Chemical perturbation to induce hypoxia
Cobalt chloride (CoCl2) (Merck, 15862) was used to induce hypoxia in either node 7 or 8 at 21 DIV.49 The node demonstrating the greatest functional connectivity among the electrodes within the incoming and outgoing microtunnels of the node at 21 DIV was selected for perturbation. Initially, 3/4 of the media in the targeted node were removed, followed by the addition of fresh cell media containing 1000 μM CoCl2. To keep the impact of the perturbation localized to a single node, the chamber was filled only up to half its volume, thereby establishing a hydrostatic pressure gradient between the targeted and neighboring nodes. This yielded an overall concentration of 500 μM CoCl2 in the targeted node.
Calcium imaging
To conduct calcium imaging, the cells in 6 microfluidic chips bonded to glass coverslips (VWR International, 24 × 24 mm No. 1 Menzel-Gläser) were transduced with in-house prepared viral vectors with an AAV8 serotype construct containing pAAV.CAG.GCaMP6s.WPRE.SV40 plasmids (Addgene, 100844). The cells were transduced at 17 DIV by replacing the cell medium with fresh neuronal medium containing a viral load of 5e2 viruses per cell. Imaging was conducted between 20–28 DIV.
Imaging
Fluorescence microscopy and calcium imaging were performed using either an EVOS M5000 microscope (Invitrogen) or an EVOS7000 microscope with an onstage incubator (Invitrogen). DAPI (AMEP4650), CY5 (AMEP4656), GFP (AMEP4651) and TxRed (AMEP4655) LED light cubes and Olympus UPLSAP0 4×/0.16 NA and 20×/0.75 NA objectives were utilized. Post-processing of images was conducted in ImageJ/Fiji or Adobe Photoshop 2020. For the calcium imaging, the microfluidic chips were covered by a custom-designed 3D-printed cap (UltiMaker Cura 3D printer) covered with a gas permeable membrane (MEA Membrane Cover, ALA scientific instruments). Recordings were conducted for 2 min at 3 frames per s.
Electrophysiological recordings
Electrophysiological recordings were performed using a MEA2100 workstation (Multichannel Systems) with the sampling rate set to 25
000 Hz. This rate significantly exceeds the Nyquist frequency needed for neural spikes, which typically range from 300 to 3000 Hz, enabling detailed characterization of spike shapes. With neural spikes generally lasting 1–2 ms, this sampling rate provides at least 25 to 50 data points per spike, improving the accuracy of both spike and burst detection. A constant temperature of 37 °C was maintained using a temperature controller (TC01, Multichannel Systems). Sterility of the cultures during recordings was ensured by employing a 3D-printed plastic cap with a gas permeable membrane (MEA Membrane Cover, ALA scientific instruments). Prior to recordings, the neural networks were allowed to equilibrate for 5 min on the recording stage, followed by a 15 min recording period. This recording time was chosen to ensure the detection of a sufficient number of network-wide events for robust statistical analysis, while minimizing the duration that the neural networks needed to be outside the incubator. All recordings were conducted 24 h after media changes.
Electrical stimulations
Stimulations were applied subsequent to the recordings of spontaneous network activity on 20, 22, 24, 26 and 28 DIV. The stimulation protocol applied a series of 10 consecutive pulses at ±800 mV amplitude (positive phase first), each lasting 200 μs, with an interspike interval of 10 s (Fig. 1B). These stimulation parameters were selected to elicit a sufficient response from nearby neurons while staying within the “water window” to prevent potentially harmful electrochemical reactions, such as oxidation and reduction, that could compromise neuronal viability.50 Nodes 1, 2, 10, 11 and 12 in layers 1 and 4 of the 12-nodal networks were selected for stimulation to verify the establishment of functional feedforward microcircuits. Stimulations were applied to the nodes in increasing order. Each spike train was delivered to the most active electrode positioned at the center of the targeted node, determined by the firing rate of the electrodes.
Data analysis calcium imaging
For analysis of the calcium imaging recordings, the open-source software CALIMA was utilized.51,52 Downscaling was set to 2× to shorten execution time prior to analysis. The standard deviation was set to 2.5, 3.5 and 0.003 for the three Gaussian filters employed by the software to detect regions of interest (ROI), respectively. Spike detection was based on the mean values (ΔF/F0) per ROI, and parameters were set to 10 s windows, a Z-score of 3.0 and an m value of 0.6. Matlab R2021b was used for further analysis. The SpikeRasterPlot function developed by Kraus53 was adapted and used for creating raster plots.
Data analysis electrophysiology
Data analysis was performed using Matlab R2021b, with graphs plotted using the linspecer function, based on the colorBrewer palette.54,55 To preprocess the data, a 4th-order Butterworth bandpass filter was applied to remove frequencies below 300 Hz and above 3000 Hz. Additionally, noise from the power supply mains at 50 Hz was eliminated using a notch filter. Zero-phase digital filtering was employed to avoid group delay in the output signal. Spike detection was carried out using the Precise Timing Spike Detection (PTSD) algorithm developed by Maccione et al.56 The data was thresholded at 8 times the standard deviation of the noise, with maximum peak duration and refractory time set to 1 ms and 1.6 ms, respectively. This threshold was selected based on the method established in the original paper and validated through visual inspection.56 The SpikeRasterPlot function developed by Kraus53 was adapted to create raster plots.
After binning the data into 50 ms time bins, functional connectivity was analyzed using Pearson correlation. To visualize key network features, graphs were plotted with electrodes as nodes and Pearson correlation as edges. Edges with connectivity less than 0.05 were removed to highlight only the strongest connections. Node color was used to represent firing rate (Hz), while node size corresponded to PageRank centrality. Community detection was performed using the Louvain algorithm, which delineated nodes into distinct communities based on the strength of their interconnections.57 Node edge colors in the graphs indicated community membership. Graph theoretical measures represented in the graphs were calculated using the Brain Connectivity Toolbox developed by Rubinov & Sporns.58
To calculate the path length between nodes in layers 1 and 4 of the networks, the inverse of the summed correlation between nodes was utilized. Specifically, the correlation for distinct paths was determined by summing the correlations between nodes/tunnels along the path. For example, for path 1 going from node 1 to 10, a sum of the correlation between the electrodes in node 1 and tunnel 1, tunnel 1 and tunnel 2, tunnel 2 and tunnel 3 and tunnel 3 and node 10 was used. If one or more correlation values were zero, the path length was considered infinite and the data point not included in the graph. The total path length between two nodes was calculated by summing the path lengths of all available paths connecting the nodes.
To remove stimulation artifacts, the stimulation data was processed using the SALPA filter developed by Wagenaar et al.59 Additionally, 15 ms of filtered data was blanked following each stimulation time point. The data was then binned into 30 ms time intervals, and peristimulus time histograms (PSTHs) of the average response of stimulations in each tunnel were plotted. Tunnels were considered active if they exhibited an integrated average response of at least 10 spikes within the first 300 ms following the stimulations, which served as the threshold for evaluating stimulation-evoked propagation of activity across the layers. All PSTHs were additionally manually inspected to identify false negatives and false positives. This decision was based on the presence of clearly distinguishable peaks and whether these peaks followed the correct order according to the sequence of the channels between the nodes.
Results
The 12-nodal microfluidic devices facilitate establishment of feedforward, hierarchical cortical microcircuits
To assess the efficacy of the 12-nodal microfluidic platform in fostering the development of mature feedforward, hierarchical neural microcircuits, structural characterization was conducted using immunocytochemistry, optical, and fluorescence microscopy. By 16 DIV, neurons had organized into densely connected networks within the individual nodes (Fig. 2A) and established long-range connections across the microtunnels interconnecting the nodes (Fig. 2B). Geometric features inspired by the Tesla valve design were integrated into the microtunnels to promote unidirectional axonal outgrowth between the nodes, complemented by saw-tooth structures on the postsynaptic side to prevent axons from finding the inlets.13 The application of viral tools facilitated the expression of different fluorescent probes in distinct nodes of the multi-layer cortical networks. This confirmed the effectiveness of the Tesla valves in redirecting axons from the postsynaptic node back to their node of origin while guiding axons from the presynaptic node to the opposite node (Fig. 2C). Furthermore, immunocytochemistry validated the structural maturation of the networks within the nodes by 21 DIV, with the markers neural nuclear protein (NeuN) and neurofilament heavy (NFH) (Fig. 2D).60,61 Additionally, GFAP staining was employed to identify the astrocytic feeder layer.62 Colocalization of the pre- and postsynaptic markers synaptophysin and PSD95 confirmed the presence of mature synaptic connections in the networks (Fig. 2E).63,64 Overall, this characterization confirmed structural and functional maturity, with networks exhibiting a feedforward, hierarchical architecture across the distinct layers and nodes.
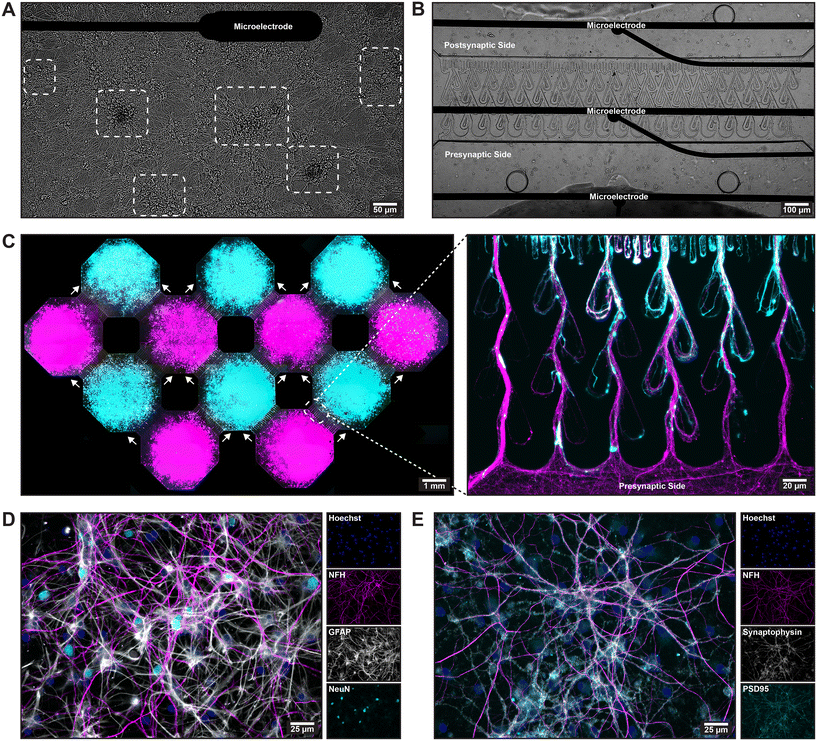 |
| Fig. 2 Establishment of mature feedforward, hierarchical neural microcircuits within the 12-nodal platforms. (A.) Micrograph showing a neural network growing on top of a microelectrode array (MEA) at 21 days in vitro (DIV). The white boxes outline aggregated clusters of neurons. (B.) Micrograph illustrating the outgrowth of axons through the Tesla valve microtunnels at 21 DIV. Three electrodes can be seen spanning the width of the tunnels to capture neural activity propagating across the two displayed nodes. (C.) Micrographs of a 12-nodal network with neurons expressing either GFP (layers 1 and 3, magenta) or mCherry (layers 2 and 4, cyan) at 28 DIV. The images show how the Tesla valve microfluidic tunnels promote feedforward axonal outgrowth, by rerouting axons growing in the unintended direction back to their node of origin. (D.) Immunocytochemistry micrograph showing that the neural networks reached a mature state by 27 DIV, indicated by the presence of the mature structural and nuclei markers neurofilament heavy (NFH) and NeuN, respectively. (E.) Immunocytochemistry micrograph showing the colocalization of the pre- and postsynaptic markers synaptophysin and PSD95, indicating the establishment of mature synapses. | |
At 16 DIV, electrophysiological recordings unveiled a complex functional interplay of segregated and integrated activity across all network layers and nodes (Fig. S2†). Network-wide bursts were observed propagating from the bottom layer (layer 1) to the uppermost layer (layer 4) of nodes across the three layers of microtunnels (Fig. 3A). Additionally, externally applied electrical stimulations to the nodes in layer 1 of the networks induced activity that propagated across all three layers of microtunnels in more than 50% of the available pathways. In contrast, stimulations to the nodes in layer 4 did not evoke detectable responses beyond the first layer of microtunnels in over 99% of available pathways (Fig. 3B–D). 60% of stimulation-induced activity in layer 4 was detected in the first layer of microtunnels, i.e., between layer 3 and 4. However, it is worth noting that the electrodes detecting this activity were positioned halfway through the tunnels, and is not representative of activity propagating across the full length of the tunnels. Nevertheless, these findings illustrate a defined hierarchical structural and functional organization within the networks, with potent propagation of activity from layer 1 to layer 4, but limited propagation of activity in the opposite direction from layer 4 to the lower layers.
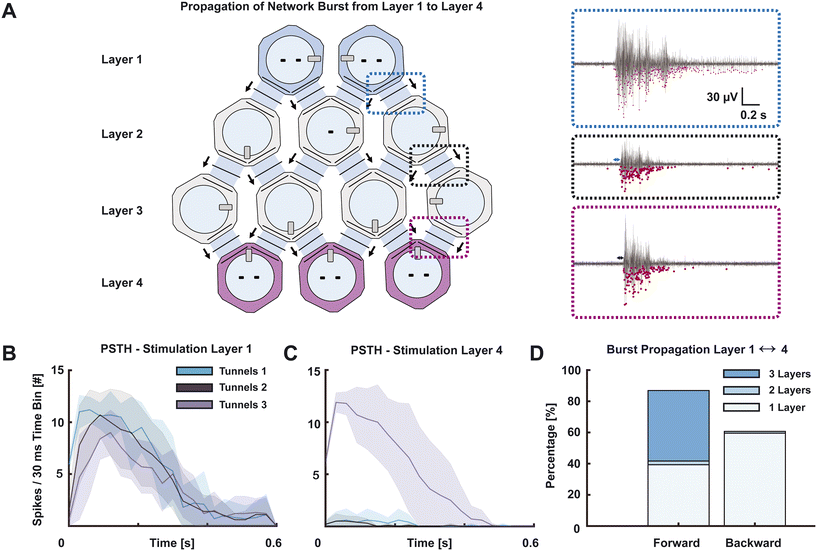 |
| Fig. 3 Electrophysiological characterization of information flow in the laminar cortical microcircuits. (A.) Schematic of the 12 nodes organized into 4 layers. Boxes indicate where the voltage traces, shown in the insets to the right, were recorded. The insets display a representative burst propagating from layer 1 to layer 4 through the connecting tunnels. (B.) and (C.) Peristimulus time histograms (PSTHs) displaying the average response of 10 consecutive stimulations in each of the three tunnels connecting layer 1 to layer 4 in the hierarchy, when stimulating nodes in layer 1 and 4, respectively. Stimulations in one of the nodes of layer 1 induced clear responses in the consecutive layers, while no response was observed beyond the first tunnels upon stimulation of the nodes in layer 4. (D.) Histogram showing percentage of stimulation induced activity spanning 1, 2 or 3 layers when stimulating nodes in layer 1 (forward) or 4 (backward), respectively. While more than 50% of available pathways exhibited activity propagating across all three layers of microtunnels in the feedforward direction, stimulations to the nodes in layer 4 did not evoke detectable responses beyond the first layer of microtunnels in more than 99% of available pathways in the backward direction. | |
In addition to MEA-based electrophysiology, calcium imaging was utilized to examine activity within distinct nodes in greater spatial detail (Fig. S3A†). This technique was employed to investigate whether the multi-nodal design, with multiple inputs to each node, influenced the synchronization of activity within the individual nodes. The calcium imaging revealed high degrees of intranodal synchrony across all layers, including the top layer receiving inputs along multiple pathways (Fig. S3B and C†). This finding not only demonstrated the efficacy of the design in promoting coordinated neural activity but also highlighted the potential for combining electrophysiology and calcium imaging on these platforms to study structure–function dynamics with high spatiotemporal precision. Additionally, the observed synchrony both across and within the nodes underscored the functional maturation of the networks, setting a reliable baseline for the perturbations performed at 21 DIV.
Localized perturbations induce node ablation and alters the network connectome
To demonstrate the applicability of the model system in studying neuroplasticity in response to localized perturbations, chemical hypoxia was induced in a central node of layer 3 at 21 DIV. Neural networks rely critically on a continuous supply of oxygen for healthy functioning, and hypoxia has been implicated in the pathogenesis of several neurodegenerative diseases.29,65,66 In the days following the perturbation, all networks exhibited rapid and extensive fragmentation within the targeted node (Fig. 4A and B). This fragmentation was particularly noticeable towards the center of the targeted nodes, but could also be observed extending towards the microtunnels (Fig. 4C). Moreover, axons entering the targeted node were observed to retract from the tunnels over time (Fig. 4D). However, the perturbation did not significantly impact the viability of the neurons within the neighboring nodes (Fig. 4E). Analysis of electrophysiological activity detected by electrodes in the microtunnels leading to the targeted nodes revealed a rapid functional disconnection of the nodes from the remaining parts of the networks (Fig. 4F and G). These results clearly demonstrate that induction of hypoxia had a detrimental impact on the structural and functional connectivity of the targeted nodes within the first 24 h following perturbation, as intended.
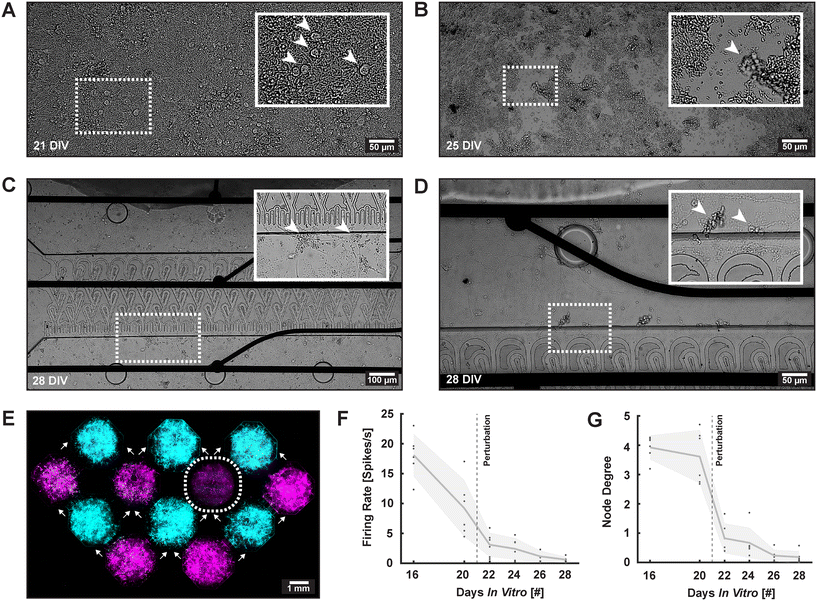 |
| Fig. 4 Induction of localized perturbation to a hub node. (A.) Micrograph depicting a healthy neural network prior to perturbation at 21 days in vitro (DIV). The enlarged inset illustrates the healthy attachment of the neural network, with white arrows highlighting the neural somata. (B.) Micrograph illustrating the fragmentation of the same neural network 4 days after perturbation. The enlarged inset shows the neural network detachment, with a white arrow pointing to a cluster of detached neurons. (C.) Micrograph displaying the microtunnels connecting a healthy presynaptic node and a perturbed postsynaptic node 7 days after perturbation. The enlarged inset highlights neurite fragmentation as they enter the node from the microtunnels, with white arrows pointing to detached neurons in the perturbed node. (D.) Micrograph showing the presynaptic side of microtunnels leading to a perturbed node, depicting the retraction of neurites growing towards the perturbed node. The enlarged inset highlights neurite fragmentation at the presynaptic node as they enter the microtunnels, with white arrows pointing to clusters of detached neurons. (E.) Micrograph of a 12-nodal network with neurons expressing either GFP (layer 1 and 3, magenta) or mCherry (layer 2 and 4, cyan). The image demonstrates the clear difference in fluorescence intensity within the perturbed node (white box) compared to the surrounding, unperturbed nodes. (F.) Firing rate detected by electrodes within the microtunnels connected to the perturbed node, exhibiting a steep decline following perturbation at 21 DIV. (G.) Correlation between the activity detected by the electrodes in the microtunnels directly adjacent the perturbed node, indicating the extent to which activity entering the node elicits an outgoing response. A steep decline can be seen following perturbations at 21 DIV. In figures F and G, each point represents a recording from an individual neural network at a specific developmental time point (DIV), with a total of n = 6 networks. The thick dark gray line shows the mean value, and the shaded area represents the standard deviation. | |
To investigate dynamic changes in the functional connectome of the networks before and after perturbation, graph theoretical analysis was applied. Initially, at 16 DIV, robust interconnectivity was evident throughout the networks (Fig. 5A). By 20 DIV, a subtle decrease in functional connectivity was observed, potentially reflecting network pruning and refinement as the networks matured (Fig. 5B). Based on the recordings at 20 DIV, Pearson correlation was used to identify the most central node in layer 3 for functional integration between layer 1 and layer 4 of each network (outlined with a pink arrow in Fig. 5B). This node was subsequently selected for perturbation at 21 DIV. By 24 DIV, 3 days following the perturbation, functional connectivity across the targeted node was markedly disrupted, while functional connections in unperturbed regions of the networks appeared strengthened (Fig. 5C). By 28 DIV, further refinement and alterations in the connectome were apparent, as evidenced by the differential delineation of the networks into distinct communities using the Louvain algorithm (Fig. 5D). In summary, the application of graph theory revealed dynamic alterations in the functional connectome of the 12-nodal neural networks following localized perturbation, highlighting the intricate adaptive responses and network reorganization processes in response to perturbation. Furthermore, these graph representations demonstrate the strength of integrating multiple metrics—such as community structure, centrality, and firing characteristics—to reveal distinct changes in network parameters over time, both individually and in combination. This approach can be used to study how different parts of the network participate in adaptive and maladaptive responses to induced perturbations.
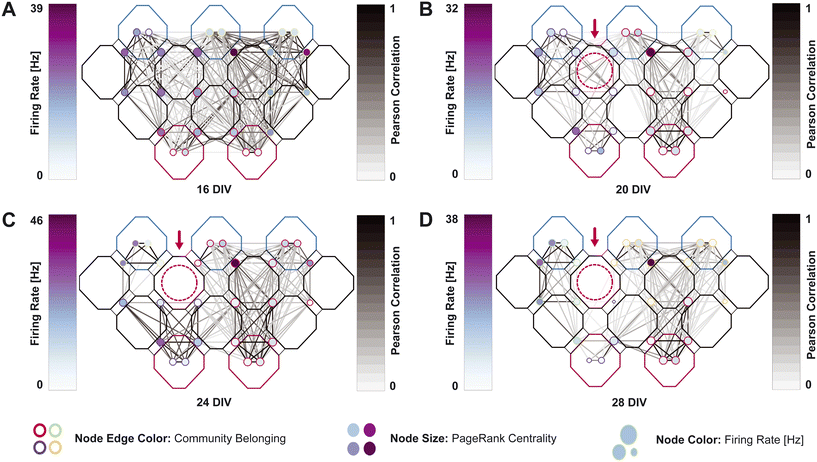 |
| Fig. 5 Dynamic changes in the network connectome before and after perturbation. (A.)–(D.) Representative graphs of a single 12-nodal network at 16, 20, 24 and 28 days in vitro (DIV). The graphs depict individual electrodes as nodes and their correlation as edges. Node color represents firing rate, node size represents PageRank centrality, and the color of edges around the nodes represents community belonging based on the Louvain algorithm. Following perturbation at 21 DIV, it is evident how functional connectivity across the hypoxic node is disrupted. Additionally, a strengthening of functional connections in unperturbed parts of the networks is observed at 24 DIV. | |
The hierarchical microfluidic layout enables investigations of plasticity in information transmission pathways following localized perturbation
To evaluate plasticity changes in the networks following localized perturbation, its impact on information processing pathways going between layer 1 and 4 was evaluated (Fig. 6A and S4†). The path length between nodes was calculated as the inverse of the correlation, summing over all paths connecting two nodes. Path lengths increased over time, with the degree of increase contingent on the centrality of the hypoxic node in information transmission (Fig. 6B and S5†). Additionally, individual networks exhibited differential susceptibility to the perturbation, with some maintaining more robust and consistent paths between nodes in layer 1 and 4 post-perturbation. For instance, node 1 had a total of three pathways connecting it to node 10 (paths 1, 2 and 4), with two of these pathways passing directly through the targeted node (paths 2 and 4) (Fig. 6A). The lateral pathway to the hypoxic node (path 1) maintained a consistent path length over time, both before and after perturbation (Fig. 6C). Conversely, the paths through the hypoxic node (paths 2 and 4) exhibited increased path length over time, with an increasing number of networks showing functionally disrupted pathways as time progressed post-perturbation (Fig. 6D and S6H†). Similar results were found for the pathways connecting the other nodes (Fig. S6†). These results demonstrate the robustness of this model system for studying plasticity changes in the functional connectome following localized perturbations.
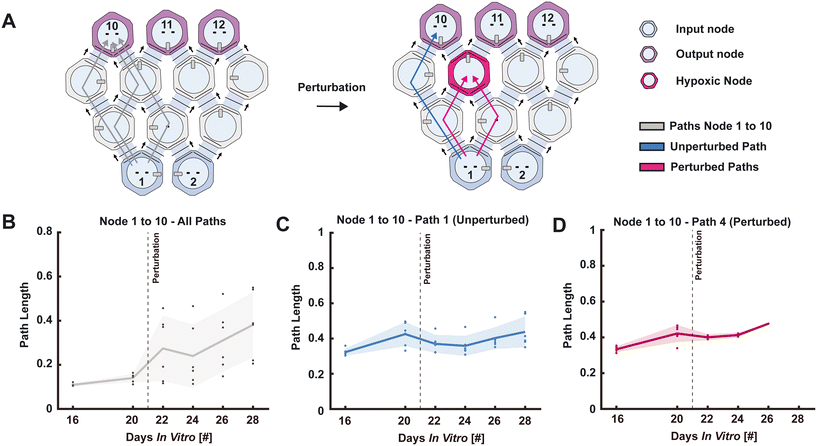 |
| Fig. 6 Alterations in information processing pathways following localized perturbation. (A.) Schematic illustrating changes in the three information processing pathways between node 1 in layer 1 and node 10 in layer 4 following perturbation of a central node in layer 3. (B.) Graph showing changes in path length between node 1 and node 10 before and after localized perturbation. The path length increases gradually over time, with varying degrees across the individual networks. (C.) Graph depicting the path length of the only pathway not passing through the hypoxic node connecting node 1 and 10. A slight decline in path length is observed the day after perturbation, followed by a gradual increase over time. (D.) Graph displaying the path length of one of the other two pathways connecting node 1 and node 10, going directly through the hypoxic node. A decreasing number of networks maintain functional connections through the hypoxic node over time, as reflected by the increased path length and diminishing number of data points. For figures B–D, each point represents a recording from an individual neural network at a specific developmental time point (DIV), with a total of n = 6 networks. The thick darker line shows the mean value, and the shaded area represents the standard deviation. | |
Perturbation alters stimulation responsiveness across pathways
To assess the effects of localized perturbation on signal transmission within the networks, changes in the forward propagation of stimulation-induced activity from layer 1 to layer 4 were analysed between 20 and 28 DIV. The majority of pathways not passing through the hypoxic node maintained their sensitivity to stimulations, displaying a consistent response in the days following the localized perturbation (Fig. 7A). In contrast, pathways that passed directly through the perturbed node showed a gradual decline in responsiveness over time (Fig. 7B and C). Prior to perturbations, stimulations induced activity that propagated across all three layers of microtunnels in more than 40% of all available pathways. By 22 DIV, one day after the perturbation, there was a 10% increase in activated pathways going around the hypoxic node, reaching 56.2%, while less than 3% of pathways passing through the hypoxic node exhibited activity propagating through all three layers of microtunnels (Fig. 7D). Although pathways going around the hypoxic nodes also showed a moderate decline in responsiveness to stimulations past 22 DIV, the extent of this decline was less pronounced compared to the pathways passing through the hypoxic node. External stimulations were also applied to all nodes in level 4 between 20 to 28 DIV, confirming maintenance of a feedforward microcircuit configuration by the absence of activity propagating beyond the first layer of microtunnels (results not shown). This analysis reveals that localized perturbations disrupted signal transmission within the networks, with pathways directly passing through the perturbed node showing a gradual decline in responsiveness over time, while pathways going around the targeted node maintained a more stable response to stimulations.
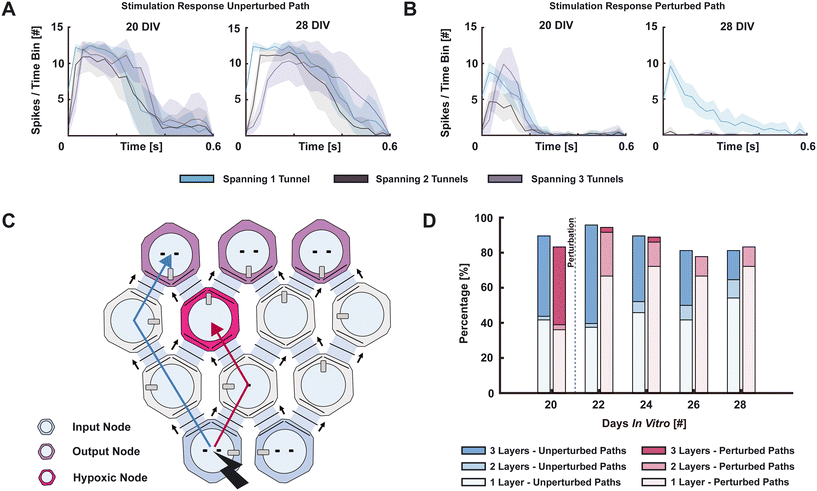 |
| Fig. 7 Alterations in the propagation of stimulation-evoked activity following localized perturbation. (A.) Peristimulus time histograms (PSTHs) displaying the average response of 10 consecutive stimulations going through an unperturbed pathway between node 1 and 10 at 20 and 28 days in vitro (DIV). The response to stimulation remained consistent over time. (B.) PSTHs displaying the average response of 10 consecutive stimulations across a pathway going through the hypoxic node between node 1 and 10 at 20 and 28 DIV. A progressive decrease in response is observed across tunnels 2 and 3 between 20 and 28 DIV. (C.) Schematic illustrating the pathway going around the hypoxic node, and the pathway passing directly through the hypoxic node in figures A and B. (D.) Histogram showing the percentage of stimulation-induced activity spanning 1, 2, or 3 layers of microtunnels when stimulating nodes in layer 1 over time for n = 6 networks. Blue boxes represent pathways that do not pass through the perturbed node, while pink boxes represent pathways that pass directly through the perturbed node. The propagation of stimulation-evoked activity is markedly reduced in the pathways going through the hypoxic node, in contrast to the more moderate reduction seen in pathways going around the hypoxic node. | |
Discussion
In this study, we have demonstrated an advanced model system for structuring and studying hierarchical multi-nodal cortical neural networks with controlled connectivity in vitro. Neocortical microcircuits have a well-defined, layered structure with controlled, feedforward axonal projections connecting the distinct layers. The model system presented here recapitulates the fundamental attributes of this structure through the implementation of controlled topological constraints. We show that selective perturbation of an identified hub node within the network abolished information flow across the targeted node and significantly influenced neighboring pathways for information transmission in the multilayer networks. These results illustrate the powerful potential of neuroengineering approaches to model physiologically relevant neural microcircuits with predefined hierarchies.
Such engineered neocortical circuits are highly relevant for disease modelling. A range of neurological and psychiatric disorders, among others Alzheimer's disease, schizophrenia, stroke and Huntington's disease, are linked to abnormalities in the neocortex.67–70 Numerous studies have shown that brain networks from patients with these conditions exhibit clear deviations from those of healthy subjects.71,72 One such deviation is the increased vulnerability of hub areas to pathological perturbations.73–75 Over time, pathological damage to these hubs leads to overload and node failure as the node cannot handle the massive amount of incoming information, likely due to the high energetic demand required to maintain them.76 Due to their central role in network integration, failure of these hubs can thus have widespread effects on network function.25 Microfluidic models harbor substantial, albeit underutilized, potential for elucidating pathological mechanisms and changes in network dynamics within controlled microenvironments.77,78 For example, several studies have illustrated how the dysfunction of one node affects the function of connected healthy nodes in various diseases, including how misfolded protein aggregates can spread in such networks.11,79–82 A key advantage of the presented model system is that it enables the application of localized perturbations to individual nodes within a complex network hierarchy, while allowing precise monitoring of spatiotemporal changes in neighboring areas, as demonstrated. Various types of perturbations can be applied to study their impact on network functionality, ranging from electrical,83 chemical,84 chemogenetic,85 to pharmacological.86–88 Additionally, by selectively perturbing distinct nodes within the 12-nodal interface, it is also possible to analyze how the centrality of different nodes influences network functionality and adaptation to damage. The inherent plasticity of cortical networks renders them particularly suitable for such investigations.89,90
Several models have been proposed to explain the changes in brain network dynamics over time in the presence of disease or trauma, yet a unified understanding remains elusive.91 Furthermore, a persistent challenge lies in the significant variability of both structural and functional alterations observed across studies.74 For instance, in Alzheimer's disease, some studies report increased path length and decreased global efficiency with time, while others have observed the opposite.72,92–96 This underscores the complexity of brain network alterations in response to trauma and disease, and the importance of improved model systems to study dynamic changes at early stages to understand and predict their progression and impact at multiple spatiotemporal scales. Engineering neural networks with multiple layers and pathways connecting the different nodes across these layers, as shown here, can facilitate the examination of a wide spectrum of adaptive and maladaptive responses to induced or inherent pathological perturbations. This includes the study of compensatory mechanisms such as neural reserves or degeneracy, where in the presence of an evolving perturbation, other network components can compensate for the damage.25,97,98 The emergence of new functional connections and the strengthening of existing ones in non-perturbed pathways three days after perturbation demonstrate that the presented model system can effectively recapitulate such compensatory mechanisms in vitro. While most studies to date have employed a two-nodal device featuring one healthy and one diseased population, the larger number of nodes adopted in this study offers a distinct advantage. Overall, the intricate design of the presented hierarchical platform facilitates the study of a wide range of connectomic adaptations to localized perturbations.
Engineered neural networks can exhibit variations in developmental timelines and established connectivity, similar to their in vivo counterparts. Factors such as the number of axons passing through microtunnels connecting nodes can influence activity spread, leading to variations in self-organization and functional profiles across different networks. In this study, while the networks displayed consistent developmental characteristics, such as firing rates, path lengths between layers, and average node degrees, distinct variability was observed in connection strength between nodes along different pathways before perturbation. Perturbations were applied to either node 7 or 8, based on which node had higher functional centrality for information transfer between layer 1 and layer 4. This ensured a significant impact on overall network connectivity, allowing for both adaptive and maladaptive changes in surrounding pathways. Understanding these variations is crucial for interpreting network behavior and ensuring consistency in results across experimental setups. Therefore, developmental differences should be considered during statistical analysis to ensure reproducibility of results.
Microfluidic MEAs can readily be used to co-culture distinct neuronal subtypes, enhancing the physiological relevance of emerging network behaviours.11,99–102 For this study, we used cortical embryonic rat neurons and demonstrated the fundamental attributes of this model system for engineering complex networks, identifying central hubs in the system, selectively perturbing them, and investigating the effects of the perturbation across the hierarchical network. Despite the canonical structure of cortical microcircuits, there is significant heterogeneity across the cortex, with different layers containing distinct neuronal subtypes that contribute to diverse connectivity patterns and functions.40 The engineered microcircuit shown in the present study is an excellent model system for studying physiological and pathophysiological changes in multi-layer, hierarchical neocortical networks in vitro. Future work may thus incorporate region-specific cortical cells into the model, for example, for specific investigations of cortical microcircuit formation and encoding.
Conclusion
Engineered neural networks represent a versatile tool for investigating the dynamics of neural circuits in controlled microenvironments. This study has introduced a novel microfluidic platform featuring 12 hierarchically interconnected nodes, designed to mimic the laminar organization of neocortical neural networks in vitro. A significant advantage with the large number of interconnected nodes is the facilitation of precise, targeted perturbations, enabling the study of adaptive compensatory mechanisms such as neural reserves and degeneracy. By inducing hypoxia in a central hub node, we have demonstrated the utility of this platform for probing plasticity changes, revealing adaptations in both directly impacted and surroundings network regions. This makes the present model highly relevant for advanced disease modelling.
Data availability
The design used for the microfabrication is included in the ESI.† The raw data produced and analyzed in this study are available from the corresponding author upon reasonable request. All data supporting the results of this study can be accessed in accordance with the journal's data-sharing policies.
Author contributions
The author contributions follow the CRediT (https://credit.niso.org/) system. NWH: conceptualization, methodology, software, investigation (chip design & manufacturing, cell experiments, ICC, electrophysiology, formal analysis), writing – original draft, visualization. PS, AS, IS: conceptualization, methodology, writing – review & editing, resources, funding acquisition.
Conflicts of interest
The authors declare that the research was conducted in the absence of any commercial or financial relationships that could be construed as a potential conflict of interest.
Acknowledgements
This work was supported by NTNU Enabling Technologies and the Central Norway Regional Health Authority. The Research Council of Norway is acknowledged for the support to the Norwegian Micro- and Nano-Fabrication Facility, NorFab, project number 295864. We would like to thank Dr. Rajeevkumar Nair Raveendran at the Viral Vector Core Facility, Kavli Institute for Systems Neuroscience, for designing and preparing the AAV viruses used for structural analysis and calcium imaging. Prof. Michela Chiappalone and Prof. Sergio Martinoia, University of Genova are acknowledged for generously providing the scripts for the Precise Timing Spike Detection algorithm. Polina Malahov is acknowledged for optimizing the tools used for calcium imaging.
References
- L. Luo, Science, 2021, 373, eabg7285 CrossRef CAS PubMed
.
- V. Pasquale, P. Massobrio, L. L. Bologna, M. Chiappalone and S. Martinoia, Neuroscience, 2008, 153, 1354–1369 CrossRef CAS PubMed
.
- D. A. Wagenaar, J. Pine and S. M. Potter, BMC Neurosci., 2006, 7, 11 CrossRef PubMed
.
- L. N. Borodinsky, Y. H. Belgacem, I. Swapna, O. Visina, O. A. Balashova, E. B. Sequerra, M. K. Tu, J. B. Levin, K. A. Spencer, P. A. Castro, A. M. Hamilton and S. Shim, Dev. Neurobiol., 2015, 75, 349–359 CrossRef PubMed
.
- G. S. Withers, C. D. James, C. E. Kingman, H. G. Craighead and G. A. Banker, J. Neurobiol., 2006, 66, 1183–1194 CrossRef
.
- E. W. Dent, S. L. Gupton and F. B. Gertler, Cold Spring Harbor Perspect. Biol., 2011, 3, 1–39 Search PubMed
.
- G. Gangatharan, S. Schneider-Maunoury and M. A. Breau, Biol. Cell, 2018, 110, 125–136 CrossRef PubMed
.
- A. M. Taylor, S. W. Rhee, C. H. Tu, D. H. Cribbs, C. W. Cotman and N. L. Jeon, Langmuir, 2003, 19, 1551–1556 CrossRef CAS PubMed
.
- H. Yamamoto, S. Moriya, K. Ide, T. Hayakawa, H. Akima, S. Sato, S. Kubota, T. Tanii, M. Niwano, S. Teller, J. Soriano and A. Hirano-Iwata, Sci. Adv., 2018, 4, eaau4914 CrossRef PubMed
.
- S. B. Lassers, Y. S. Vakilna, W. C. Tang and G. J. Brewer, Front. Neural Circuits, 2023, 17, 1272925 CrossRef PubMed
.
-
K. S. Hanssen, N. Winter-Hjelm, S. N. Niethammer, A. Kobro-Flatmoen, M. P. Witter, A. Sandvig and I. Sandvig, bioRxiv, 2024, preprint, DOI:10.1101/2023.06.26.546556.
- M. U. Park, Y. Bae, K.-S. Lee, J. H. Song, S.-M. Lee and K.-H. Yoo, Lab Chip, 2021, 21, 951–961 RSC
.
- N. Winter-Hjelm, Å. B. Tomren, P. Sikorski, A. Sandvig and I. Sandvig, J. Neural Eng., 2023, 20, 046024 CrossRef PubMed
.
- J.-M. Peyrin, B. Deleglise, L. Saias, M. Vignes, P. Gougis, S. Magnifico, S. Betuing, M. Pietri, J. Caboche, P. Vanhoutte, J.-L. Viovy and B. Brugg, Lab Chip, 2011, 11, 3663–3673 RSC
.
- A. Gladkov, Y. Pigareva, D. Kutyina, V. Kolpakov, A. Bukatin, I. Mukhina, V. Kazantsev and A. Pimashkin, Sci. Rep., 2017, 7, 15625 CrossRef PubMed
.
- P. M. Holloway, G. I. Hallinan, M. Hegde, S. I. R. Lane, K. Deinhardt and J. West, Lab Chip, 2019, 19, 1484–1489 RSC
.
- R. Renault, J.-B. Durand, J.-L. Viovy and C. Villard, Lab Chip, 2016, 16, 2188–2191 RSC
.
-
P. Sterling and S. Laughlin, Principles of neural design, The MIT Press, 2015 Search PubMed
.
- O. Sporns, NeuroImage, 2012, 62, 881–886 CrossRef
.
- D. J. Watts and S. H. Strogatz, Nature, 1998, 393, 440–442 CrossRef CAS
.
- E. Bullmore and O. Sporns, Nat. Rev. Neurosci., 2009, 10, 186–198 CrossRef CAS
.
- C. J. Stam and E. C. W. van Straaten, Clin. Neurophysiol., 2012, 123, 1067–1087 CrossRef CAS PubMed
.
- S. Oldham and A. Fornito, Dev. Cognit. Neurosci., 2019, 36, 100607 CrossRef
.
- E. Bullmore and O. Sporns, Nat. Rev. Neurosci., 2012, 13, 336–349 CrossRef CAS PubMed
.
- A. Fornito, A. Zalesky, D. S. Bassett, D. Meunier, I. Ellison-Wright, M. Yücel, S. J. Wood, K. Shaw, J. O'Connor, D. Nertney, B. J. Mowry, C. Pantelis and E. T. Bullmore, J. Neurosci., 2011, 31, 3261–3270 CrossRef CAS PubMed
.
- M. P. van den Heuvel and O. Sporns, Trends Cognit. Sci., 2013, 17, 683–696 CrossRef PubMed
.
- F. V. Farahani, W. Karwowski and N. R. Lighthall, Front. Neurosci., 2019, 13, 585 CrossRef PubMed
.
- P. Massobrio, V. Pasquale and S. Martinoia, Sci. Rep., 2015, 5, 10578 CrossRef CAS PubMed
.
-
V. Fiskum, N. Winter-Hjelm, N. Christiansen, A. Sandvig and I. Sandvig, bioRxiv, 2024, preprint, DOI:10.1101/2024.01.04.574167.
- K. Heiney, O. H. Ramstad, V. Fiskum, A. Sandvig, I. Sandvig and S. Nichele, Front. Neural Circuits, 2022, 16, 980631 CrossRef CAS PubMed
.
- P. Rakic, Nat. Rev. Neurosci., 2009, 10, 724–735 CrossRef CAS PubMed
.
- P. S. Goldman-Rakic, Neuron, 1995, 14, 477–485 CrossRef CAS PubMed
.
- A. M. Bastos, W. M. Usrey, R. A. Adams, G. R. Mangun, P. Fries and K. J. Friston, Neuron, 2012, 76, 695–711 CrossRef CAS PubMed
.
- C. Koch, M. Massimini, M. Boly and G. Tononi, Nat. Rev. Neurosci., 2016, 17, 307–321 CrossRef CAS PubMed
.
- E. K. Miller, Nat. Rev. Neurosci., 2000, 1, 59–65 CrossRef CAS PubMed
.
- A. E. Papale and B. M. Hooks, Neuroscience, 2018, 368, 283–297 CrossRef CAS PubMed
.
- R. Lorente De Nó, J. Neurophysiol., 1938, 1, 207–244 CrossRef
.
- V. B. Mountcastle, J. Neurophysiol., 1957, 20, 408–434 CrossRef CAS PubMed
.
- D. P. Buxhoeveden and M. F. Casanova, Brain, 2002, 125, 935–951 CrossRef PubMed
.
- V. B. Mountcastle, Brain, 1997, 120, 701–722 CrossRef PubMed
.
- I. Thompson, Curr. Biol., 1997, 7, R324–R326 CrossRef CAS PubMed
.
- A. H. Leighton and C. Lohmann, Front. Neural Circuits, 2016, 10, 71 Search PubMed
.
- D. Poli, V. P. Pastore and P. Massobrio, Front. Neural Circuits, 2015, 9, 57 Search PubMed
.
- M. Chiappalone, M. Bove, A. Vato, M. Tedesco and S. Martinoia, Brain Res., 2006, 1093, 41–53 CrossRef CAS PubMed
.
- T. K. Hensch, Nat. Rev. Neurosci., 2005, 6, 877–888 CrossRef CAS PubMed
.
- T. Wieloch and K. Nikolich, Curr. Opin. Neurobiol., 2006, 16, 258–264 CrossRef CAS
.
- M. Sur and C. A. Leamey, Nat. Rev. Neurosci., 2001, 2, 251–262 CrossRef CAS PubMed
.
- K. N. Richter, N. H. Revelo, K. J. Seitz, M. S. Helm, D. Sarkar, R. S. Saleeb, E. D'Este, J. Eberle, E. Wagner, C. Vogl, D. F. Lazaro, F. Richter, J. Coy-Vergara, G. Coceano, E. S. Boyden, R. R. Duncan, S. W. Hell, M. A. Lauterbach, S. E. Lehnart, T. Moser, T. F. Outeiro, P. Rehling, B. Schwappach, I. Testa, B. Zapiec and S. O. Rizzoli, EMBO J., 2018, 37, 139–159 CrossRef CAS PubMed
.
- W. Zou, M. Yan, W. Xu, H. Huo, L. Sun, Z. Zheng and X. Liu, J. Neurosci. Res., 2001, 64, 646–653 CrossRef CAS PubMed
.
- C. Boehler, T. Stieglitz and M. Asplund, Biomaterials, 2015, 67, 346–353 CrossRef CAS PubMed
.
- E. A. L. Raaijmakers, N. Wanders, R. M. C. Mestrom and R. Luttge, Micromachines, 2021, 12, 412 CrossRef PubMed
.
- F. D. W. Radstake, E. A. L. Raaijmakers, R. Luttge, S. Zinger and J. P. Frimat, Comput. Methods Programs Biomed., 2019, 179, 104991 CrossRef CAS PubMed
.
-
B. Kraus, Spike Raster Plot, 2022, https://github.com/MATLAB-Graphics-and-App-Building/spike-raster-plot/releases/tag/v1.0.1.
-
J. C. Lansey, Beautiful and distinguishable line colors + colormap, 2021, https://se.mathworks.com/matlabcentral/fileexchange/42673-beautiful-and-distinguishable-line-colors-colormap.
- C. A. Brewer, G. W. Hatchard and M. A. Harrower, Cartogr. Geogr. Inf. Sci., 2003, 30, 5–32 CrossRef
.
- A. Maccione, M. Gandolfo, P. Massobrio, A. Novellino, S. Martinoia and M. Chiappalone, J. Neurosci. Methods, 2009, 177, 241–249 CrossRef PubMed
.
- V. D. Blondel, J.-L. Guillaume, R. Lambiotte and E. Lefebvre, J. Stat. Mech.: Theory Exp., 2008, 2008, P10008 CrossRef
.
- M. Rubinov and O. Sporns, NeuroImage, 2010, 52, 1059–1069 CrossRef PubMed
.
- D. A. Wagenaar and S. M. Potter, J. Neurosci. Methods, 2002, 120, 113–120 CrossRef PubMed
.
- R. J. Mullen, C. R. Buck and A. M. Smith, Development, 1992, 116, 201–211 CrossRef CAS
.
- W. W. Schlaepfer and J. Bruce, J. Neurosci. Res., 1990, 25, 39–49 CrossRef CAS PubMed
.
- L. F. Eng, R. S. Ghirnikar and Y. L. Lee, Neurochem. Res., 2000, 25, 1439–1451 CrossRef CAS PubMed
.
- B. Wiedenmann and W. W. Franke, Cell, 1985, 41, 1017–1028 CrossRef CAS PubMed
.
- K.-O. Cho, C. A. Hunt and M. B. Kennedy, Neuron, 1992, 9, 929–942 CrossRef CAS PubMed
.
- J. Burtscher, R. T. Mallet, M. Burtscher and G. P. Millet, Ageing Res. Rev., 2021, 68, 101343 CrossRef CAS PubMed
.
- V. Fiskum, A. Sandvig and I. Sandvig, Front. Integr. Neurosci., 2021, 15, 792863 CrossRef CAS PubMed
.
- H. Braak and E. Braak, Acta Neuropathol., 1991, 82, 239–259 CrossRef CAS PubMed
.
- L. D. Selemon and P. S. Goldman-Rakic, Biol. Psychiatry, 1999, 45, 17–25 CrossRef CAS PubMed
.
- J. S. Paulsen, V. A. Magnotta, A. E. Mikos, H. L. Paulson, E. Penziner, N. C. Andreasen and P. C. Nopoulos, Biol. Psychiatry, 2006, 59, 57–63 CrossRef CAS PubMed
.
- R. J. Nudo, Stroke, 2007, 38, 840–845 CrossRef PubMed
.
- D. J. Sharp, G. Scott and R. Leech, Nat. Rev. Neurol., 2014, 10, 156–166 CrossRef PubMed
.
- Y. He, Z. Chen and A. Evans, J. Neurosci., 2008, 28, 4756–4766 CrossRef CAS PubMed
.
- N. A. Crossley, A. Mechelli, J. Scott, F. Carletti, P. T. Fox, P. Mcguire and E. T. Bullmore, Brain, 2014, 137, 2382–2395 CrossRef PubMed
.
- B. M. Tijms, A. M. Wink, W. de Haan, W. M. van der Flier, C. J. Stam, P. Scheltens and F. Barkhof, Neurobiol. Aging, 2013, 34, 2023–2036 CrossRef PubMed
.
- M. P. van den Heuvel, I. L. C. van Soelen, C. J. Stam, R. S. Kahn, D. I. Boomsma and H. E. H. Pol, Eur. Neuropsychopharmacol., 2013, 23, 19–23 CrossRef CAS PubMed
.
- C. J. Stam, Nat. Rev. Neurosci., 2014, 15, 683–695 CrossRef CAS PubMed
.
- J. A. del Rio and I. Ferrer, Front. Bioeng. Biotechnol., 2020, 8, 570692 CrossRef PubMed
.
- T. Osaki, Y. Shin, V. Sivathanu, M. Campisi and R. D. Kamm, Adv. Healthcare Mater., 2018, 7, 1700489 CrossRef PubMed
.
- A. Virlogeux, E. Moutaux, W. Christaller, A. Genoux, J. Bruyère, E. Fino, B. Charlot, M. Cazorla and F. Saudou, Cell Rep., 2018, 22, 110–122 CrossRef CAS PubMed
.
- V. D. Valderhaug, O. H. Ramstad, R. van de Wijdeven, K. Heiney, S. Nichele, A. Sandvig and I. Sandvig, Front. Cell. Neurosci., 2024, 18, 1366098 CrossRef CAS PubMed
.
- E. C. Freundt, N. Maynard, E. K. Clancy, S. Roy, L. Bousset, Y. Sourigues, M. Covert, R. Melki, K. Kirkegaard and M. Brahic, Ann. Neurol., 2012, 72, 517–524 CrossRef CAS PubMed
.
- G. I. Hallinan, M. Vargas-Caballero, J. West and K. Deinhardt, J. Neurosci., 2019, 39, 9623–9632 CrossRef CAS PubMed
.
- D. A. Wagenaar, R. Madhavan, J. Pine and S. M. Potter, J. Neurosci., 2005, 25, 680–688 CrossRef CAS PubMed
.
- P. Massobrio, C. N. G. Giachello, M. Ghirardi and S. Martinoia, BMC Neurosci., 2013, 14, 22 CrossRef CAS PubMed
.
- J. S. Weir, N. Christiansen, A. Sandvig and I. Sandvig, Front. Neural Circuits, 2023, 17, 1020487 CrossRef CAS PubMed
.
- M. Frega, V. Pasquale, M. Tedesco, M. Marcoli, A. Contestabile, M. Nanni, L. Bonzano, G. Maura and M. Chiappalone, Neurotoxicol. Teratol., 2012, 34, 116–127 CrossRef CAS PubMed
.
- A. Odawara, H. Katoh, N. Matsuda and I. Suzuki, Sci. Rep., 2016, 6, 26181 CrossRef CAS PubMed
.
- I. Colombi, S. Mahajani, M. Frega, L. Gasparini and M. Chiappalone, Front. Neuroeng., 2013, 6, 10 CAS
.
- J. van Pelt, I. Vajda, P. S. Wolters, M. A. Corner and G. J. A. Ramakers, Prog. Brain Res., 2005, 147, 171–188 Search PubMed
.
- P. Massobrio, J. Tessadori, M. Chiappalone and M. Ghirardi, Neural Plast., 2015, 2015, 196195 Search PubMed
.
- R. Ashish and P. Fon, Biol. Psychiatry Cogn. Neurosci. Neuroimaging, 2018, 3, 788–797 Search PubMed
.
- C.-Y. Lo, P.-N. Wang, K.-H. Chou, J. Wang, Y. He and C.-P. Lin, J. Neurosci., 2010, 30, 16876–16885 CrossRef CAS PubMed
.
- C. J. Stam, B. F. Jones, G. Nolte, M. Breakspear and P. Scheltens, Cereb. Cortex, 2007, 17, 92–99 CrossRef CAS PubMed
.
- E. J. Sanz-Arigita, M. M. Schoonheim, J. S. Damoiseaux, S. A. R. B. Rombouts, E. Maris, F. Barkhof, P. Scheltens and C. J. Stam, PLoS One, 2010, 5, e13788 CrossRef PubMed
.
- C. J. Stam, W. de Haan, A. Daffertshofer, B. F. Jones, I. Manshanden, A. M. van Cappellen van Walsum, T. Montez, J. P. A. Verbunt, J. C. de Munck, B. W. van Dijk, H. W. Berendse and P. Scheltens, Brain, 2009, 132, 213–224 CrossRef CAS PubMed
.
- W. de Haan, Y. A. L. Pijnenburg, R. L. M. Strijers, Y. van der Made, W. M. van der Flier, P. Scheltens and C. J. Stam, BMC Neurosci., 2009, 10, 101 CrossRef PubMed
.
- A. Avena-Koenigsberger, B. Misic and O. Sporns, Nat. Rev. Neurosci., 2018, 19, 17–33 CrossRef CAS PubMed
.
- U. Noppeney, K. J. Friston and C. J. Price, J. Anat., 2004, 205, 433–442 CrossRef PubMed
.
- C. H. Chang, T. Furukawa, T. Asahina, K. Shimba, K. Kotani and Y. Jimbo, Front. Neurosci., 2022, 16, 873664 CrossRef PubMed
.
- S. Han, S. Bang, H. N. Kim, N. Choi and S. H. Kim, Mol. Brain, 2023, 16, 13 CrossRef CAS PubMed
.
- S. Dauth, B. M. Maoz, S. P. Sheehy, M. A. Hemphill, T. Murty, M. K. Macedonia, A. M. Greer, B. Budnik and K. K. Parker, J. Neurophysiol., 2017, 117, 1320–1341 CrossRef CAS PubMed
.
- Y. S. Vakilna, W. C. Tang, B. C. Wheeler and G. J. Brewer, Front. Neural Circuits, 2021, 15, 660837 CrossRef PubMed
.
|
This journal is © The Royal Society of Chemistry 2024 |
Click here to see how this site uses Cookies. View our privacy policy here.