DOI:
10.1039/D3MA00825H
(Paper)
Mater. Adv., 2024,
5, 2347-2358
Controlled release of doxorubicin from gelatin-based nanoparticles: theoretical and experimental approach†
Received
9th October 2023
, Accepted 17th January 2024
First published on 18th January 2024
Abstract
Doxorubicin (DOX), a well-known chemotherapeutic agent, is extensively used for cancer therapy. However, cardiotoxicity and hypersensitivity are the major side effects of DOX. Other issues that need to be addressed include the short half-life, low stability, and high rate of drug release. An injectable drug delivery system (DDS) composed of pH-responsive crosslinked gelatin nanoparticles (Ge-NPs) is reported to lessen the side effects and address the aforementioned obstacles. Ge-NPs were made via a two-step desolvation procedure with two distinct concentrations of 5 wt% (Ge-NPs-5) and 10 wt% (Ge-NPs-10). The physicochemical properties of the Ge-NPs were characterized using Fourier transform infrared (FTIR) spectroscopy, scanning electron microscopy (SEM), and dynamic light scattering (DLS). The mean diameter of the Ge-NPs was measured at pH 7.4, 5.0, and 3.0. Doxorubicin was successfully loaded into the Ge-NPs, with encapsulation efficiencies of 65.12% (Ge-NPs-5) and 53.7% (Ge-NPs-10). Moreover, artificial neural networks (ANNs) were used to predict the cumulative release of DOX under different experimental conditions. ANNs exhibited considerably improved prediction ability and there was good agreement between the ANN's anticipated and experimentally observed drug release data. Overall, this study presents the synthesis of Ge-NPs for controlled drug release and the utilization of the ANN model as a tool to predict the release of DOX under different pH conditions.
1. Introduction
Cancer is one of the leading causes of mortality worldwide; one out of every six deaths is caused by this disease. According to an estimation, over 1.9 million new cases and more than half a million deaths are expected due to cancer in the United States in 2022.1 There are several flaws in existing chemotherapy, mainly related to poor bioavailability, low response, nonspecific distribution, drug resistance, and several side effects, which result in inconsistent clinical outcomes.2 However, the most challenging task in a chemotherapeutic formulation is controlling the drug concentration in the body in the desired range with an effective approach.3 The advancement of techniques with controlled release at the desired location without damaging the healthy surrounding areas should be an exciting challenge for controlled drug delivery carriers.4 Many researchers have been using polymeric nanoparticles in combination with chemotherapeutic agents like doxorubicin, paclitaxel, and 5-fluorouracil to improve cancer therapy in recent years.5 DOX is a well-known anticancer drug that is widely used in cancer therapy to treat various tumors such as bladder, stomach, breast, and lung cancer. A low dose of DOX has a significant disadvantage in terms of cancer cell internalization. On the other extreme, its high dosage not only compromises the cancer cell survival but also causes severe side effects in healthy cells and tissues, such as hypersensitivity and cardiotoxicity.6 Other challenges related to DOX include a short half-life, high rate of drug release, and lack of stability.7 To overcome these problems, a polymer-based controlled DDS is used to release the drug at the desired rate for a given duration of time.8 Polymeric hydrogels are 3D arrangements of polymers that absorb water due to their hydrophilic nature, making them suitable for interacting with human tissues.9–11
The development and design of stimuli-responsive hydrogels for DDSs have gained considerable attention in recent years. Stimuli-responsive DDSs are innovative platforms designed to release therapeutic agents in response to specific stimuli, ensuring precise control over drug release at targeted sites within the body. These systems offer significant advantages over conventional drug delivery methods by enhancing the therapeutic efficacy while minimizing side effects. There are two main categories of stimuli for triggering drug release: exogenous and endogenous stimuli. Exogenous stimuli originate externally and are applied or introduced into the body. Examples of exogenous stimuli include light, magnetic fields, ultrasound, and others. Among them, light-responsive DDSs have gained significant attention due to their precise control, non-invasiveness, and spatiotemporal specificity.12 On the other hand, endogenous stimuli arise from physiological conditions within the body, such as changes in pH, temperature, enzyme concentration, or the presence of specific biomolecules. pH-sensitive DDSs intelligently release therapeutic agents based on variations in acidity levels. pH-triggered DDSs utilize changes in pH within body compartments, such as tumor microenvironments, enabling selective drug release at specific sites. This precision allows for targeted treatment, minimizing side effects and enhancing therapeutic efficacy. pH-sensitive DDSs signify a groundbreaking strategy in drug delivery, offering tailored treatments that exploit physiological variations for optimized therapeutic outcomes.6,13
Hydrogels are available in a variety of sizes, ranging from nano-gels to macro-gels.14 Generally, small particles are recommended in drug treatment because they have a higher blood circulation period as well as the highest loading efficiency.15 Gelatin is a naturally occurring protein-polymer that is produced by heat denaturing collagen. It is divided into two types: type A and type B. Type-A gelatin is cationic and is made up of pigskin, whereas type-B gelatin is anionic and is formed from alkaline bovine collagen.14,16–18 It offers several advantages and can interact with both hydrophobic and hydrophilic medicines with good gelation characteristics.5,19,20 Gelatin-based nanoparticles have been extensively studied as drug carriers in controlled DDSs.21 These NPs are effective drug delivery vehicles because of their unique properties, such as biodegradability and biocompatibility, low cytotoxicity, and high binding capacity.22,23 Several techniques have been used to make Ge-NPs depending on the required results, such as emulsification, desolvation, coacervation-phase separation, water-in-oil emulsion, and so on.24,25 One of the most often used methods for making gelatin nanoparticles is desolvation. This technique depends on the aqueous polymer solution and poor solvents such as acetone, acetonitrile, or alcohol. Its challenging to make homogenous Ge-NPs in size with the large molecular weight distribution range of gelatin. The two-step desolvation method has solved this issue and is the most preferred technique for preparing Ge-NPs.26–28 Coester et al. first developed this approach and removed the low molecular weight gelatin followed by desolvation of the high molecular weight fraction.26 Gelatin nanoparticles provide various distinct benefits, including enhanced pharmacokinetics, release profile, and targeted drug delivery. The size distribution of Ge-NPs in drug carriers is an important factor because it influences how they interact with the cell membranes and their ability to cross physiological barriers. Therefore, controlling the size distribution, particle size, and surface morphology of NPs is essential.28
To enhance the characteristics of NP-based delivery systems, many factors in both biological and material aspects need to be optimized. However, prediction of the release kinetics from the hydrogel matrix and living organisms is very challenging. Each data point in NP-based delivery systems is labor-intensive and time-taking, and necessitates the purchase of expensive laboratory supplies.29 Mathematical models have recently sparked a surge of interest in the study of such systems. Many different models have been developed to examine the controlled release rates of therapeutic moieties from a 3D crosslinked polymeric network. Artificial neural networks (ANNs) are one of these methods. A neural network is a series of “neurons” that are layered one on top of the other (or “nodes”). The design of a neural network is made up of an ‘input’ layer, one or more ‘hidden’ layers, and an ‘output’ layer.30 It is a computational model that is based on the functioning mode of the human brain and can achieve, derive, and develop new information. ANN is an efficient modeling tool with well-established benefits across traditional mathematical and statistical techniques for identifying complicated correlations in input–output data. ANN has been studied as an effective technique to investigate and comprehend biological systems for the various variables.31 The main aim of this study was to develop and characterize pH-responsive Ge-NPs as a controlled drug delivery vehicle. Two different weight ratios (5 wt% and 10 wt%) of gelatin were used to synthesize Ge-NPs through a two-step desolvation method. The morphology and size of both Ge-NPs were analyzed by SEM and DLS, respectively. Next, the DOX release profile from the Ge-NPs was studied under different pH conditions (3.0, 5.0, and 7.4) for 48 hours. Moreover, an ANN-based model was developed to predict the drug release profile. The model was validated using the obtained experimental findings. Overall, this study finds that ANN might be a useful tool for modeling experimental datasets in the field of nanomedicine, allowing us to precisely predict the effects of critical factors on a system with less experimental effort and expenses.
2. Materials and methods
2.1. Materials
Gelatin type B from bovine skin (225 Bloom), acetone, glutaraldehyde (grade-I 25%), sodium hydroxide (NaOH), hydrochloric acid (HCl 37%), sodium chloride (NaCl), potassium chloride (KCl), disodium hydrogen phosphate dihydrate (Na2HPO4·2H2O), and monopotassium phosphate (KH2PO4) were purchased from Sigma-Aldrich (St. Louis, OM, USA). Doxorubicin was obtained from Carbosynth (Compton, UK). All the reagents and chemicals used in this study were of analytical grade and were used without further purification. Double distilled water was used in all the experiments.
2.2. Synthesis and characterization of gelatin nanoparticles
Gelatin nanoparticles were synthesized using a two-step desolvation technique as described previously.32 Two different concentrations (5 and 10 wt%) of gelatin solutions were prepared in distilled water at 50 °C. For this, 1.25 gm and 2.5 gm of gelatin was dissolved in 25 mL of distilled water to yield 5 and 10 wt% solutions, respectively. As a desolvating agent, 25 mL of acetone was added in each solution to separate the high molecular weight (HMW) gelatin. Sedimentation was allowed to happen by keeping the solutions at room temperature for 10 minutes. After that, the supernatant was discarded, and the precipitates of gelatin were collected and re-dissolved in 25 mL of distilled water at 50 °C. The pH of both gelatin solutions was adjusted to 3 with 6 N HCl. To form nanoparticles, 75 mL of acetone was added dropwise into the gelatin solutions under constant stirring at 50 °C. For the crosslinking of the nanoparticles, 300 μL of 25% glutaraldehyde was used to make Ge-NPs-5 and 600 μL was used to crosslink Ge-NPs-10. The resulting mixtures were stirred again for the next 2 hours at 50 °C. Finally, centrifugation was performed at 4400 rpm for 20 minutes, and the resultant nanoparticles were washed with an acetone/water mixture (3
:
7). The obtained nanoparticles were stored at 4 °C for further use. The structural information of gelatin, Ge-NPs-5, and Ge-NPs-10 was investigated through Fourier transform infrared (FTIR) spectroscopy. The surface morphology of the Ge-NPs-5 and Ge-NPs-10 was examined through scanning electron microscopy. For this, samples were collected on cleaned silicon wafers and dried overnight in a desiccator. Next, silicon wafers were attached to metal stubs with the aid of double-sided carbon tape and the surfaces of the NPs (Ge-NPs-5 and Ge-NPs-10) were coated with a thin layer of gold (∼15). The sample was observed under a microscope at 10.0 kV accelerated voltage with 500k magnification. The diameters of the Ge-NPs were measured with ImageJ®. Moreover, the particle size of the Ge-NPs-5 and Ge-NPs-10 was measured in three pH conditions (3.0, 5.0, and 7.4) by using a dynamic light scattering (DLS) instrument (Malvern Instruments, Ltd, Malvern, UK) with a Zetasizer 3000. Before measuring the size of the Ge-NPs, a suspension of Ge-NPs was sonicated for a short time using a sonicator and diluted with distilled water. The data was interpreted by examining the size distribution based on dispersed light intensity.
2.3. Drug loading and release studies
DOX was used as a model drug for drug loading and release studies. To measure the drug loading efficiency, the drug content in the DOX-loaded Ge-NPs was determined by the difference between the total quantity added in the Ge-NP dispersion and the amount of free drug present in the solvent system. DOX was dissolved in distilled water to prepare stock solutions (1 mg mL−1). Both Ge-NPs-5 and Ge-NPs-10 nanoparticles (15 mg) were dispersed in 3 mL of PBS separately, which contained 300 μg of DOX. For maximum drug loading, the resulting solution was shaken continuously at around 75 rpm for 2 hours at room temperature and then incubated in the dark for 24 hours. Next, centrifugation was carried out at 4000 rpm for 10 minutes to remove the unbound drug and the pellet was then resuspended in ultrapure water. The absorbance of the supernatant was measured with a UV-VIS Spectrophotometer (UV-2800 Biotechnology Medical Services, USA) at 480 nm. To determine a standard calibration curve, different concentrations of DOX solutions were prepared. Eqn (1) was used to calculate the drug encapsulation efficiency: | Encapsulation efficiency (%) = Wdrug/Wo × 100 | (1) |
where Wo is the initial weight of the drug and Wdrug is the weight of the loaded drug. Drug release studies were carried out at three different pHs (7.4, 5.0, and 3.0) in PBS at physiological temperature (37 °C). 1N HCl solution was used to alter the pH of the PBS buffer. Drug-loaded nanoparticles were immediately re-dispersed in 3 mL of PBS and kept on shaking at 100 rpm. To measure the release of DOX from both types of nanoparticles (Ge-NPs-5 and Ge-NPs-10), each sample in triplicate was taken and centrifuged for 20 minutes. Next, 0.2 mL from each sample was withdrawn at different time points (1, 2, 3, 4, 6, 10, 12, 24, and 48 h) to check the released drug. After each withdrawal, the solution volume was adjusted by adding the same quantity of fresh PBS. The release of DOX was calculated using eqn (2) at various time intervals. |  | (2) |
where Mt represents the mass released during time t, Ct denotes the concentration of drug at time t, V represents the total volume of released solution (3 mL), and Vs is the volume of sample withdrawn (0.2 mL). The percentage of cumulative drug release, Q, was determined by using eqn (3).where M∞ represents the total weight of DOX-loaded into Ge-NPs.
2.4. Artificial neural network (ANN) model development
The neural network toolbox and MATLAB® 2019a were used to develop the ANN model. Three input variables (Ge-NPs concentration, pH, and time) and one output variable (drug release) were used to develop the ANN model. The training and test groups were selected at random using the dataset given in Table 3. The designed ANN model was used to validate with test data. The data was obtained from 66 different in vitro experiments to investigate the DOX release rate from the Ge-NPs at different pH levels and time intervals. The effects of time, pH, and concentration of Ge-NPs on the cumulative release of DOX were predicted using an ANN model and compared with experimental data. 70% of the dataset was used for training purposes, while the remaining 30% was employed to validate the model. 48 samples were used as training data, whereas 18 samples were employed as test data. These values correspond to the system's experimental boundaries. The neural networks must be trained to modify the weights and biases.33 A network can be trained by using multiple methods such as genetic algorithm (GA), quasi-Newton (Levenberg–Marquardt), gradient descent, and other techniques may be used. The Levenberg–Marquardt optimization technique (‘trainlm’) was used in this study to train the model and modify the weights because it is a fast-response and highly preferred methodology for drug release profiles with the lowest mean square error (MSE). A varying number of epochs were tried to generate a well-trained network (100, 300, 1000). In this study, the network is made up of an input layer with 3 neurons, a hidden layer containing 10 neurons, and an output layer of a single neuron. This output is referred to as the target. To evaluate the validity of ANN and to predict the drug release rate from the Ge-NPs, the feedforward backpropagation methodology was chosen, and the number of hidden layers was modified until the testing results had the lowest mean squared error (MSE) and the highest correlation determination (R2).31 The following eqn (4) was used to calculate R2: | 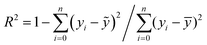 | (4) |
where yi, ỹ, and ȳ are the dependent variables being known as observed, predicted, and mean, respectively. Root-mean-squared error (RMSE) is calculated by using eqn (5): | 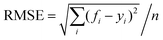 | (5) |
3. Results and discussion
The Ge-NPs have multiple physicochemical properties, such as surface charge influence, size, shape, drug delivery efficiency, therapeutic release kinetics, in vivo circulation time, and metabolic activity.34 We evaluated the particle size, entrapment efficiency, and surface structure of the synthesized gelatin nanoparticles. The main steps involved in the present work are represented in Fig. 1. In this study, we first synthesized two types of gelatin nanoparticles, Ge-NPs-5 and Ge-NPs-10, using a two-step desolvation method illustrated in Fig. 1A. Following that, we loaded DOX into the Ge-NPs and tested the drug release in three distinct pH mediums 7.4, 5, and 3. The drug release profile from the pH responsive Ge-NPs was studied at various time intervals (Fig. 1B). Finally, we developed an ANN model to predict the drug release rates at different time intervals using different pH media and varied Ge-NP concentrations. Three inputs (Ge-NPs concentration, pH, and time), as well as one output variable (drug release rate) were used to develop the ANN model. The schematic of the ANN model is illustrated in Fig. 1C, and the parameters that we used to train the ANN model are provided in Table 1.
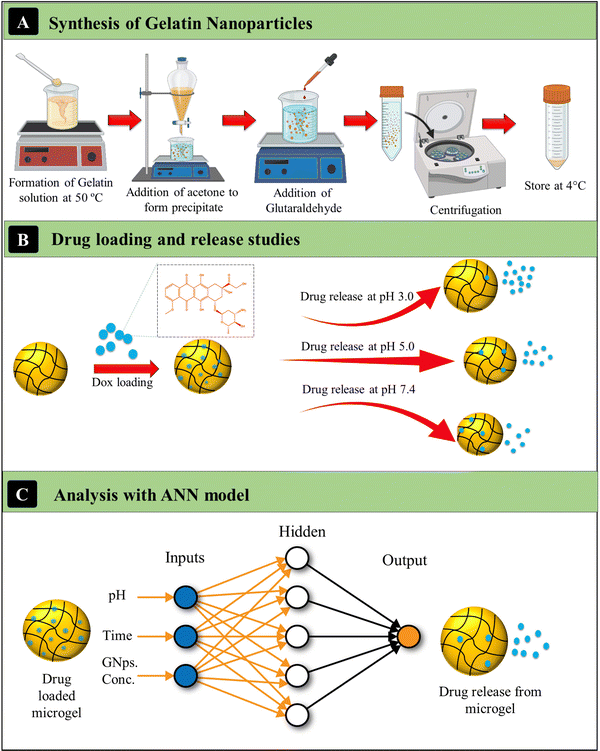 |
| Fig. 1 Schematics of the synthesis of gelatin nanoparticles (A), investigating the loading and release kinetics of DOX in various pH media (B), and the ANN model to predict the release kinetics of DOX (C). | |
Table 1 Summary of the ANN parameters
Parameters |
The release rate of DOX from the Ge-NPs |
Backpropagation type |
Levenberg–Marquardt |
Number of layers |
2 |
Maximum number of epochs |
1000 |
Minimum number of epochs |
1 × 10−7 |
3.1. FTIR analysis
FTIR spectroscopy was used to determine the crosslinking of the Ge-NPs. Fig. 2A shows the FTIR spectrum of gelatin, Ge-NPs-5, and Ge-NPs-10. The main characteristic peaks of pure gelatin at 1627 cm−1 and 1539 cm−1 correspond to C
O stretching for amide I, and N–H bending of amide II. Other sharp peaks at 3348 cm−1 and 2927 cm−1 of gelatin are related to the N–H stretching of the amide group and asymmetric stretching of the C–H group, respectively.35,36
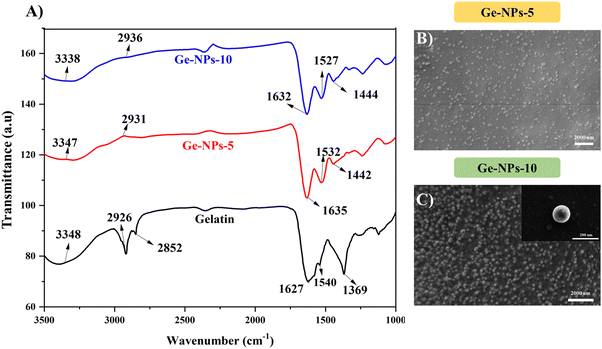 |
| Fig. 2 FTIR spectra of gelatin (black color), Ge-NPs-5 (red color) and Ge-NPs-10 (blue color) (A). Surface morphology analysis of Ge-NPs-5 (B) and Ge-NPs-10 (C). | |
All these peaks can be seen in the Ge-NPs-5 and Ge-NPs-10 spectra. In the amide-I and amide-II regions, there was a minor shift. The increased intensity of the amide-I absorption peaks at 1635 cm−1 and 1632 cm−1, of Ge-NPs-5 and Ge-NPs-10, respectively, as compared to pure gelatin, which is at 1627 cm−1, confirmed that the gelatin chains were successfully crosslinked with glutaraldehyde.10 The amide-II absorption peaks of the Ge-NPs-10, Ge-NPs-5, and gelatin samples are at 1528 cm−1, 1532 cm−1, and 1540 cm−1 respectively. Moreover, strong peaks were observed at 1442 cm−1 and 1444 cm−1 in the crosslinked Ge-NPs-5 and Ge-NPs-10 due to aldimine linkage.37 The aldehyde group of glutaraldehyde, which behaves like a cross-linking agent, reacts with the amino groups of the gelatin protein. As a result, after crosslinking, the overall intensity of this peak increased. As a result, the gelatin crosslinking was satisfactorily validated by the FTIR spectra.
3.2. Surface morphology and particle size analysis
The surface morphology of the Ge-NPs was investigated through a scanning electron microscope (SEM), as shown in Fig. 2B and C. As demonstrated, the average size of the Ge-NPs as examined by SEM microscopy is smaller than that of DLS analysis because the samples are dried before SEM imaging compared to the fully hydrated particles in aqueous dispersion.38 In our study, Image J® was used to measure the particle size (n = 50), and we found the sizes of the Ge-NPs-5 and Ge-NPs-10 in SEM to be 94 ± 48 nm and 118 ± 24 nm respectively, as opposed to the DLS analysis, where the size range was found to be between 190 ± 91 nm and 459 ± 92 nm. The observed images further reveal that the particle shape in the Ge-NPs-10 is nearly spherical and more homogeneous compared to the Ge-NPs-5. Moreover, the hydrodynamic size distribution measurements and polydispersity index (PDI) of the synthesized Ge-NP samples were carried out via DLS. The particle size data shows that the produced nanoparticles were quite small with a low polydispersity index indicating a relatively narrow distribution of particle sizes.39 We examined the hydrodynamic size of Ge-NPs in three distinct pH environments: pH 7.4, pH 5.0, and pH 3.0. Our observations show that the swelling of the particles is caused by the ionization of the carboxyl groups in an acidic medium. The diameters of the Ge-NPs-5 were measured to be 255 ± 80 nm and 190 ± 91 nm at pH 3.0, and 5.0, respectively (Fig. 3C and B). In the case of Ge-NPs-10, we discovered a similar trend. The size profiles of the Ge-NPs-10 were 459 ± 92 nm and 295 ± 84 nm at pH 3.0, and 5.0, respectively, as shown in Fig. 3F and E. Furthermore, the zeta sizes of the Ge-NPs-5 and Ge-NPs-10 at physiological pH were observed to be 220 ± 74 nm and 396 ± 122 nm, respectively (Fig. 3A and D). These findings revealed how Ge-NPs react to different pH, as shown in Table 2.
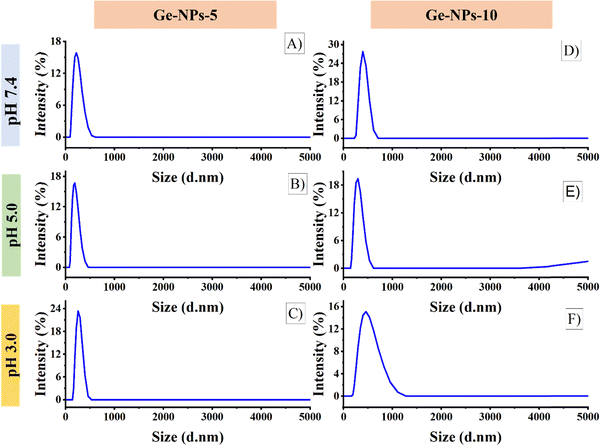 |
| Fig. 3 The size profiles of the Ge-NPs-5 at pH levels 7.4 (A), 5.0 (B), and 3.0 (C), and for Ge-NPs-10 at pH levels 7.4 (D), 5.0 (E), and 3.0 (F). | |
Table 2 The particle sizes and polydispersity index of Ge-NPs-5 and Ge-NPs-10 were measured under varying pH conditions in a PBS buffer
|
Ge-NPs-5 |
Ge-NPs-10 |
Medium |
Zeta size (nm) |
PDI |
Zeta size (nm) |
PDI |
pH 3.0 |
255 ± 80 |
0.258 |
459 ± 92 |
0.247 |
pH 5.0 |
190 ± 91 |
0.223 |
295 ± 84 |
0.297 |
pH 7.4 |
220 ± 74 |
0.232 |
396 ± 122 |
0.173 |
Gelatin is a polyamphoteric macromolecule because its side chains include many carboxylic (–COOH) and amino groups (–NH2). The swelling behavior and size distribution range of gelatin are fully dependent on whether it is acting as an acid or a base at particular pH levels.40 Shifting the pH levels from a physiological range to an acidic medium triggers a significant swelling response in gelatin nanoparticles, primarily due to the increased degree of ionization. On the other hand, due to their isoelectric point at pH 5.0, the Ge-NPs-5 and Ge-NPs-10 hardly swell.41 The medium has a low ionic strength, and also at this pH, gelatin achieves its isoelectric point, where the amount of positive and negative charges is equal and the electrical interaction between these opposed charges will cause network collapse.42 Therefore, the Ge-NPs-5 and Ge-NPs-10 exhibited the smallest size at pH 5 (Fig. 3B and E).
The gelatin network formed a cationic gel at low pH and an anionic gel at high pH. The swelling degree of the cationic gel was greater than that of the anionic gel. Similar findings have been observed in prior research.40,43Eqn (6) and (7) illustrate the anionic and cationic gel formation of gelatin, respectively.
| Gelatin − NH2 + H+ → Gelatin − NH3+ | (6) |
| Gelatin − COOH + OH− → Gelatin − COO− + H2O | (7) |
3.3. Drug loading and release studies
Stimuli-responsive biomaterials have shown the ability to improve the delivery of drug moieties to cancerous cells in a controlled and sustained manner.14 As previously reported, pH-responsive Ge-NPs were used to selectively target an acidic tumor microenvironment. In this study, DOX was loaded into Ge-NPs-5 and Ge-NPs-10. The calibration curve of DOX was plotted by using the mean absorbance value versus the drug concentration (Fig. 4A). The drug loading efficiency of the Ge-NPs-5 and Ge-NPs-10 was found to be 65 ± 12% and 53 ± 07%, respectively. Higher encapsulation efficiency was noted in the Ge-NPs-5. Drug loading into the Ge-NPs may occur through various mechanisms, including electrostatic attraction, physical entrapment, or chemical bonding. In our study DOX is loaded into Ge-NPs through electrostatic interactions44 on the surface and inside the particles as well. Fig. 4B and C illustrate the release kinetics of DOX from Ge-NPs-5 and Ge-NPs-10 at 37 °C in three distinct pH media (3.0, 5.0, and 7.4). The release mechanism of DOX-loaded Ge-NPs was significantly influenced by the pH environment. After 24 hours, the percentage of DOX release rate from the Ge-NPs-5 and Ge-NPs-10 was evaluated as 23% and 17%, respectively at physiological pH (Fig. 4B and C). When these Ge-NPs were incubated at a slightly acidic pH 5, the percent drug release increased to 68% in Ge-NPs-5 and 55% in Ge-NPs-10. To confirm the impact of pH on drug release, we conducted a cumulative drug release assessment in a more acidic solution with a pH of 3.0. In this environment, the release rates significantly increased, reaching 95% for Ge-NPs-5 and 81% for Ge-NPs-10. These findings reveal that the drug was released quickly in an acidic (pH 3.0) medium as compared to a neutral condition (pH 7.4). Moreover, these results show that the pH of the medium has a direct impact on the overall amount of DOX released. The drug release mechanisms from Ge-NPs include desorption, diffusion, and the biodegradation of the Ge-NPs. Moreover, the release pattern is dependent on the drug's solubility in various pH buffers. Specifically, DOX demonstrates high solubility at lower pH levels, potentially accelerating its release from the Ge-NPs due to the protonation of amine groups present in DOX.45 Furthermore, gelatin has a chain conformation that is affected by temperature and pH levels. Additionally, gelatin can relax at acidic pH levels due to the electrostatic repulsion among the charged gelatin molecules. This leads to a reduced tendency for the molecules to form a helix or a three-dimensional network, as reported by Zandi et al.46 As a result, the relaxed Ge-NPs and reduced electrostatic interactions enable a higher degree of DOX release from DOX-loaded Ge-NPs in an acidic environment. The release patterns of DOX from the Ge-NPs are also influenced by the crosslinker concentrations. The rate of drug release from the Ge-NPs was reduced by increasing the glutaraldehyde concentration.47 In the present study, we used two different concentrations of glutaraldehyde such as 300 μL and 600 μL to synthesize Ge-NPs-5 and Ge-NPs-10, respectively. The reduced doxorubicin release rates observed in the Ge-NPs-10 in comparison to Ge-NPs-5 could be due to the higher degree of crosslinking.37 In the Ge-NPs, drug loading may not affect a highly crosslinked network produced by significant crosslinking. However, it's important to consider various factors that may affect the behavior and properties of Ge-NPs, such as pH level, concentration of crosslinking agent and isoelectric point. That's why the encapsulation effectiveness of DOX in Ge-NPS-10 was slightly poor as compared to Ge-NPs-5.
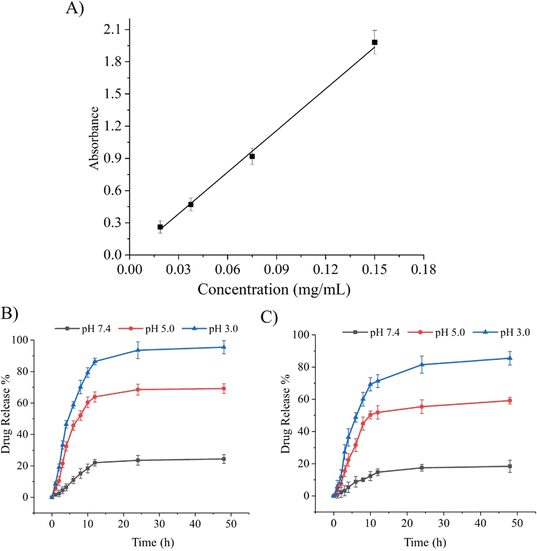 |
| Fig. 4 Calibration curve of DOX (A). The percentage release profiles of DOX from Ge-NPs-5 (B) and Ge-NPs-10 (C) across three different pH environments (3.0, 5.0, and 7.4). | |
3.4. Predicting the release kinetics of DOX loaded Ge-NPs
Recently, ANNs have been used to optimize complex data. In this work, we used an ANN model to predict the drug release profile from a Ge-NP system. We developed an experimental dataset that included 66 data points. Moreover, we used three input variables, including gelatin concentration, pH, and time, and one output variable, which was the drug release profile. We used different layers and nodes to form the neural network and randomly divided it into training and test groups. The Levenberg–Marquardt model predicted a release rate with a strong correlation to train the data.48 Our primary objective in employing this approach was to identify the optimal neural network configuration that would enable effective generalization. R2 values around “1” for the given dataset (train and test) indicate a better model prediction.31 We aimed to discover the best setup for accurate predictions while avoiding overfitting. This involved systematically testing various numbers of hidden layers and comparing the performance metrics. Furthermore, Fig. 5 indicates a reasonably good correlation between the experimental and predicted DOX release profile from Ge-NPs. For training data (Fig. 5A) and testing data (Fig. 5B), the R2 values were computed as 0.97 and 0.98, respectively.
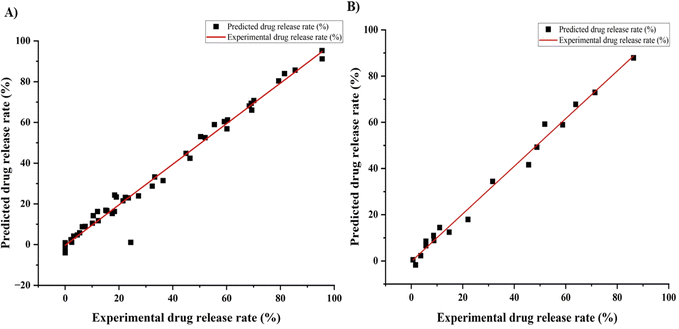 |
| Fig. 5 Correlation between the ANN predicted and experimental release profile of DOX from gelatin nanoparticles for two experimental data sets: (A) training data and (B) testing data. | |
The ANN model was trained using 48 datasets as shown in ESI† Table S1. Following the training of the model, the testing and validation steps were carried out. For testing the ANN model, 18 datasets (Table 3) were used.
Table 3 Observed and predicted release profile for the test data set
Sample No. |
GNPs conc. (%) |
pH |
Time (h) |
Experimental DOX release rate (%) |
Predicted release rate (%) |
Release rate error (%) |
1 |
5 |
7.4 |
1 |
1.655 |
−1.71 |
−3.365 |
2 |
5 |
7.4 |
6 |
11 |
14.44 |
3.44 |
3 |
5 |
7.4 |
12 |
22 |
17.96 |
−4.04 |
4 |
5 |
5.0 |
1 |
5.655 |
6.629 |
0.974 |
5 |
5 |
5.0 |
6 |
45.569 |
41.626 |
−3.943 |
6 |
5 |
5.0 |
12 |
63.832 |
67.771 |
3.939 |
7 |
5 |
3.0 |
1 |
8.655 |
11.014 |
2.359 |
8 |
5 |
3.0 |
6 |
58.76 |
58.94 |
0.18 |
9 |
5 |
3.0 |
12 |
86.32 |
87.89 |
1.57 |
10 |
10 |
7.4 |
1 |
0.655 |
0.49 |
−0.165 |
11 |
10 |
7.4 |
6 |
8.787 |
8.86 |
0.073 |
12 |
10 |
7.4 |
12 |
14.72 |
12.45 |
−2.27 |
13 |
10 |
5.0 |
1 |
3.655 |
2.30 |
−1.35 |
14 |
10 |
5.0 |
6 |
31.569 |
34.438 |
2.869 |
15 |
10 |
5.0 |
12 |
51.832 |
59.223 |
7.391 |
16 |
10 |
3.0 |
1 |
5.655 |
8.514 |
2.859 |
17 |
10 |
3.0 |
6 |
48.76 |
49.246 |
0.486 |
18 |
10 |
3.0 |
12 |
71.32 |
72.93 |
1.61 |
Fig. 6 shows 3D surface plots illustrating the actual release pattern of DOX from Ge-NPs. In the acidic medium, the amount of DOX released increases with decreasing pH of the medium and the particles swell more due to the ionization of the carboxyl groups.49 The total amount of constant charge increases depending on the level of ionization, which increases the electrostatic repulsion force across the chains. In general, when the pH becomes more acidic, the release rate increases dramatically. Initially, a significant amount of loaded DOX is released, but with the passage of time, the release rate slows down as equilibrium states are achieved. As shown in Fig. 6, in Ge-NPs-5, the release of DOX after 48 hours varied with the pH level: it was 95% in an acidic environment, 69% at pH 5, and 24% at pH 7.4. In the context of Ge-NPs-10, after 48 hours, the release of DOX was 85% in an acidic environment, 59% at pH 5, and 18% at pH 7.4. This information showcases how the drug release varies under different pH conditions for this particular nanoparticle formulation. Moreover, Fig. 7 illustrates the expected outcome of the DOX release rate by using the developed ANN model. According to the test results, the ANN model could predict the drug release rate effectively when parameters like Ge-NP concentration (5 wt% and 10 wt%), time interval (1, 6 and 12 h), and pH of the medium (7.4, 5.0, and 3.0) were changed. In our study, at an acidic pH, we investigated a high release rate of DOX from Ge-NPs whereas at physiological pH, the drug release rate was sustained and much lower. After 12 hours, Ge-NPs-5 released around 17.9% of the drug at normal body pH, while Ge-NPs-10 released about 12.4%. Whereas at pH 3, the predicted drug release rates from Ge-NPs-5 and Ge-NPs-10 drastically increased to 86.3% and 71.3%, respectively. The release rate of DOX from Ge-NPs-10 was slower than that of Ge-NPs-5 because of increased gelatin crosslinking. The actual drug release profile and the expected one had minor differences. Fig. 6 and 7, along with Tables S1 and Table 3 (ESI†), clearly demonstrate the high accuracy of the developed model.
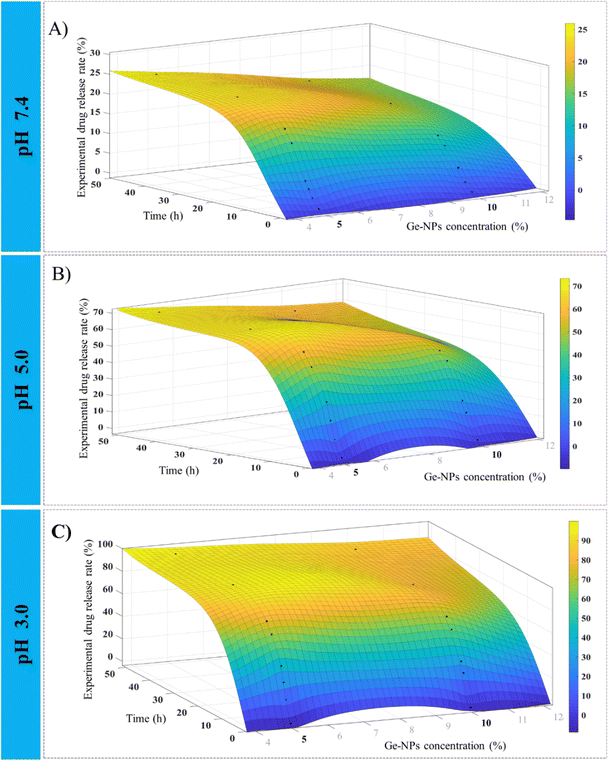 |
| Fig. 6 Experimental observations for the release profile of DOX from Ge-NPs at various pH mediums 7.4 (A), 5.0 (B), and 3.0 (C) with intervals of time. Plots are constructed by using the data from Table S1 (ESI†). | |
 |
| Fig. 7 For comparative analysis, the experimental and predicted release profiles for DOX were compared using an ANN model varying by time intervals and pH level. | |
The results of the predicted release revealed that the developed model was able to properly estimate the drug release rate; however, certain observations slightly changed from the expected values. The information gained from parameter fitting might be beneficial for analyzing and predicting the release rate of DOX.
4. Conclusions
In the present study, we developed pH-responsive gelatin nanoparticles designed for the controlled release of doxorubicin. These nanoparticles were synthesized using a two-step desolvation method, employing two distinct weight ratios, specifically 5% and 10%. We used glutaraldehyde as a crosslinking agent in the process. Various techniques were used to investigate detailed physicochemical characterizations such as FTIR, SEM, and DLS. It has been demonstrated that particle size is a significant property of a nanoencapsulation product used for drug delivery because the drug release rate depends on size and size distribution. The experimental results showed that when the pH level is changed from normal to acidic, the gelatin nanoparticles undergo a remarkable swelling effect due to the increase in degree of ionization. On the other hand, due to their isoelectric point at pH 5.0, Ge-NPs-5 and Ge-NPs-10 have the smallest size. The experiment demonstrated that the amount of DOX released from the Ge-NPs varied with pH, concentration, and time. Moreover, we developed an artificial neural network model and validated it using an experimental data set. This model was used to predict the cumulative release of DOX under different experimental conditions. The ANN model results accurately showed how the release rate of doxorubicin varied at various time points and pH conditions. In this way, we were able to estimate the release of doxorubicin from different kinds of Ge-NPs. Overall, this study demonstrates that the artificial neural networks might be a valuable tool for modeling experimental data sets in nanomedicine, allowing us to correctly predict the impacts of critical factors on a system with minimal experimental effort and expenditure. Conclusively, this study might be able to take a step forward towards the goal of enhancing therapeutic effects while minimizing side effects.
Conflicts of interest
There are no conflicts of interest to declare.
Acknowledgements
The authors would like to acknowledge the financial support from the Office of Research, Innovation and Commercialization (ORIC), National Textile University, Faisalabad, under the short research support program with funding support no. SRSP-3/2021-11/83. The schematics were created by using a paid version of BioRender®.
References
- R. L. Siegel, K. D. Miller, H. E. Fuchs and A. Jemal, Ca-Cancer J. Clin., 2022, 72, 7–33 CrossRef PubMed.
- K. H. Wong, A. Lu, X. Chen and Z. Yang, Molecules, 2020, 25, 3620 CrossRef PubMed.
- S. Gavas, S. Quazi and T. M. Karpiński, Nanoscale Res. Lett., 2021, 16, 173 CrossRef CAS PubMed.
- R. H. Prabhu, V. B. Patravale and M. D. Joshi, Int. J. Nanomed., 2015, 10, 1001 CAS.
- R. P. Das, B. G. Singh and A. Kunwar, Biomater. Sci., 2020, 8, 4251–4265 RSC.
- S. R. Batool, M. A. Nazeer, D. Ekinci, A. Sahin and S. Kizilel, Int. J. Biol. Macromol., 2020, 150, 315–325 CrossRef CAS PubMed.
- S. D. Steichen, M. Caldorera-Moore and N. A. Peppas, Eur. J. Pharm. Sci., 2013, 48, 416–427 CrossRef CAS PubMed.
- V. Sivasankarapillai, S. Das, F. Sabir, M. Sundaramahalingam, J. Colmenares, S. Prasannakumar, M. Rajan, A. Rahdar and G. Kyzas, Mater. Today Chem., 2021, 19, 100382 CrossRef CAS.
- S. R. Batool, M. A. Nazeer, E. Yildiz, A. Sahin and S. Kizilel, Int. J. Biol. Macromol., 2021, 185, 165–175 CrossRef CAS PubMed.
- M. A. Nazeer, E. Yilgor and I. Yilgor, Polymer, 2019, 168, 86–94 CrossRef CAS.
- O. C. Onder, S. R. Batool and M. A. Nazeer, Mater. Adv., 2022, 3, 6920–6949 RSC.
- M. A. Haghighat Bayan, Y. J. Dias, C. Rinoldi, P. Nakielski, D. Rybak, Y. B. Truong, A. L. Yarin and F. Pierini, J. Polym. Sci., 2023, 61, 521–533 CrossRef CAS.
- T. Sarwar, Z. A. Raza, M. A. Nazeer and A. Khan, Int. J. Biol. Macromol., 2024, 256, 128525 CrossRef CAS PubMed.
-
M. Nazeer, S. Batool and S. Kizilel, Soft Matter for Biomedical Applications, 2021, pp. 542–565 Search PubMed.
- S. Ko and S. Gunasekaran, J. Microencapsulation, 2006, 23, 887–898 CrossRef CAS PubMed.
- P. Erkoc, I. Uvak, M. A. Nazeer, S. R. Batool, Y. N. Odeh, O. Akdogan and S. Kizilel, Macromol. Biosci., 2020, 20, 2000106 CrossRef CAS PubMed.
- F. Mushtaq, Z. A. Raza, S. R. Batool, M. Zahid, O. C. Onder, A. Rafique and M. A. Nazeer, Int. J. Biol. Macromol., 2022, 218, 601–633 CrossRef CAS PubMed.
- T. Sarwar, Z. A. Raza, M. A. Nazeer and A. Khan, Int. J. Biol. Macromol., 2023, 253, 126588 CrossRef CAS PubMed.
- A. E. Koletti, E. Tsarouchi, A. Kapourani, K. N. Kontogiannopoulos, A. N. Assimopoulou and P. Barmpalexis, Int. J. Pharm., 2020, 578, 119118 CrossRef CAS PubMed.
- A. Kulshrestha, S. Sharma, K. Singh and A. Kumar, Mater. Adv., 2022, 3, 484–492 RSC.
- H. Khan, R. Shukla and A. Bajpai, Mater. Sci. Eng., C, 2016, 61, 457–465 CrossRef CAS PubMed.
- K. S. Shamarekh, H. A. Gad, M. E. Soliman and O. A. Sammour, J. Pharm. Invest., 2020, 50, 189–200 CrossRef CAS.
- S. Patra, P. Basak and D. Tibarewala, Mater. Sci. Eng., C, 2016, 59, 310–318 CrossRef CAS PubMed.
- C. Park, C. L.-N. Vo, T. Kang, E. Oh and B.-J. Lee, Eur. J. Pharm. Biopharm., 2015, 89, 365–373 CrossRef CAS PubMed.
- A. O. Elzoghby, J. Controlled Release, 2013, 172, 1075–1091 CrossRef CAS PubMed.
- C. Coester, K. Langer, H. Von Briesen and J. Kreuter, J. Microencapsulation, 2000, 17, 187–193 CrossRef CAS PubMed.
- C.-L. Tseng, K.-H. Chen, W.-Y. Su, Y.-H. Lee, C.-C. Wu and F.-H. Lin, J. Nanomater., 2013, 2013 Search PubMed.
- W. Lohcharoenkal, L. Wang, Y. C. Chen and Y. Rojanasakul, BioMed Res. Int., 2014, 2014, 180549 Search PubMed.
- N. O. Dogan, U. Bozuyuk, P. Erkoc, A. C. Karacakol, A. Cingoz, F. Seker-Polat, M. A. Nazeer, M. Sitti, T. Bagci-Onder and S. Kizilel, Adv. NanoBiomed Res., 2022, 2, 2100033 CrossRef CAS.
- F. Amato, A. López, E. M. Peña-Méndez, P. Vaňhara, A. Hampl and J. Havel, J. Appl. Biomed., 2013, 11, 47–58 CrossRef CAS.
- U. Bozuyuk, N. O. Dogan and S. Kizilel, ACS Appl. Mater. Interfaces, 2018, 10, 33945–33955 CrossRef CAS PubMed.
- J. A. Carvalho, A. S. Abreu, V. T. P. Ferreira, E. P. Gonçalves, A. C. Tedesco, J. G. Pinto, J. Ferreira-Strixino, M. Beltrame Junior and A. R. Simioni, J. Biomater. Sci., Polym. Ed., 2018, 29, 1287–1301 CrossRef CAS PubMed.
-
T. Dumitriu, R. P. Dumitriu and V. Manta, 2016.
- F. Danışman-Kalındemirtaş, İ. A. Kariper, G. Erdemir, E. Sert and S. Erdem-Kuruca, Sci. Rep., 2022, 12, 10686 CrossRef PubMed.
- S. Y. Ooi, I. Ahmad and M. C. I. M. Amin, Ind. Crops Prod., 2016, 93, 227–234 CrossRef CAS.
- N. Nieto González, G. Cerri, J. Molpeceres, M. Cossu, G. Rassu, P. Giunchedi and E. Gavini, Pharmaceuticals, 2022, 15, 662 CrossRef PubMed.
- A. Pal, A. Kumar Bajpai and J. Bajpai, J. Macromol. Sci., Part A: Pure Appl.Chem., 2019, 56, 206–214 CrossRef CAS.
- C. de Souza, J. A. Carvalho, A. S. Abreu, L. P. de Paiva, J. A. Ambrosio, M. B. Junior, M. A. de Oliveira, J. Mittmann and A. R. Simioni, J. Biomater. Sci., Polym. Ed., 2021, 32, 1–21 CrossRef CAS PubMed.
- S. K. Bhattacharyya, M. Dule, R. Paul, J. Dash, M. Anas, T. K. Mandal, P. Das, N. C. Das and S. Banerjee, ACS Biomater. Sci. Eng., 2020, 6, 5662–5674 CrossRef CAS PubMed.
- W. H. Wan Ishak, N. A. Rosli, I. Ahmad, S. Ramli and M. C. I. Mohd Amin, J. Mater. Res. Technol., 2021, 15, 7145–7157 CrossRef CAS.
-
O. S. Yin, I. Ahmad and M. C. I. M. Amin, 2014.
- R. Leu Alexa, H. Iovu, J. Ghitman, A. Serafim, C. Stavarache, M.-M. Marin and R. Ianchis, Polymers, 2021, 13, 727 CrossRef PubMed.
- S. Boral, A. N. Gupta and H. Bohidar, Int. J. Biol. Macromol., 2006, 39, 240–249 CrossRef CAS PubMed.
- S. Suarasan, M. Focsan, M. Potara, O. Soritau, A. Florea, D. Maniu and S. Astilean, ACS Appl. Mater. Interfaces, 2016, 8, 22900–22913 CrossRef CAS PubMed.
-
A.-V. Weiß, 2018.
-
M. Zandi, H. Mirzadeh and C. Mayer, 2007.
- H. Fan and A. K. Dash, Int. J. Pharm., 2001, 213, 103–116 CrossRef CAS PubMed.
- H. S. Ali, N. Blagden, P. York, A. Amani and T. Brook, Eur. J. Pharm. Sci., 2009, 37, 514–522 CrossRef CAS PubMed.
- C. Boztepe, A. Künkül and M. Yüceer, J. Drug Delivery Sci. Technol., 2020, 57, 101603 CrossRef CAS.
|
This journal is © The Royal Society of Chemistry 2024 |
Click here to see how this site uses Cookies. View our privacy policy here.