Theoretical insights into the compatibility of template-monomer-crosslinker-solvent for cortisol molecularly imprinted polymer pre-polymerization†
Received
9th May 2023
, Accepted 25th October 2023
First published on 25th October 2023
Abstract
Detection of cortisol (Cort), a stress hormone, is essential in monitoring chronic and mental health stress. As such, there is growing interest in the development of cortisol molecularly imprinted polymers (MIPs) as molecular receptors for sensor development. Of the cortisol MIPs described in the literature, the optimization of the functional monomers has been through trial-and-error experimentation. Through a computational approach, the number of optimization experiments can be reduced, which is time efficient and cost effective, while reducing chemical wastage. In addition to density functional theory (DFT) calculations, this study used an atomistic molecular dynamics simulation approach that resembles that of the real-life experimental methods to elucidate the compatibility of template-monomer-crosslinkers-solvent for cortisol MIP receptors that can efficiently recognize and capture cortisol from biological fluids. The functional monomer investigated were 4-vinylpyridine (4VP), acrylic acid (AA), acrylamide (AM), glycidyl methacrylate (GMA), 2-hydroxyethyl methacrylate (HEMA) and methylacrylic acid (MAA) with ethylene glycol dimethacrylate (EGDMA) as the crosslinker. The intermolecular hydrogen bonds and the template-monomer binding energies obtained through DFT suggested Cort-MAA as the most stable complex both in the gas phase and solution. Considering the calculated solvent energies, acetonitrile was recommended as a porogenic solvent. Through molecular dynamics simulation, various parameters were analyzed to explain the compatibility of the functional monomer with the cortisol template in the MIP development. From blend analysis of template-monomer, Cort-4VP was found to be the most miscible complex. For template-monomer-crosslinker (EGDMA), the mean square displacement (MSD) and diffusion coefficient analyses indicated 1
:
2 (cortisol/monomer) as the ratio in which the complexes are most stable. The highest peaks observed from the Radial distribution function were for Cort-MAA and Cort-AA at 1
:
4 indicating better interactions of the functional monomers with the Cort. Investigating the effect of solvents for template-monomer-crosslinker-solvent, the lowest MSD was at 1
:
4 with three complexes having the lowest values for solubility parameters at 1
:
4 confirming this ratio to be generally suitable for the composition of cortisol MIPs pre-polymerization.
Design, System, Application
There is growing interest in the development of cortisol (known as stress hormone biomarker) molecularly imprinted polymers (MIPs) as molecular receptors for sensor development. The study was designed to predict the best composition ratio of template-monomer for cortisol MIP pre-polymerization. The system contained six functional monomers, one crosslinker and a porogenic solvent. The density functional theory and atomistic molecular dynamics simulation approach were used to conclude the best functional monomers and the mixing ratios through a series of analyses which are quite difficult to analyze through an experimental approach and as such their investigation is very limited in the literature for cortisol MIP receptors. This kind of study will enhance the optimization of the functional monomers in the development of the cortisol MIP receptor that can efficiently recognize and isolate cortisol from biofluid samples.
|
Introduction
Designing the synthetic route and developing materials that can efficiently replicate the detection processes found in nature has become a significant field of research over the last few years. One of the vital synthetic methodologies to design and develop robust molecular recognition materials that can imitate natural recognition entities is molecular imprinting technology (MIT).1 MIT is an efficient emerging approach for preparing polymers that can specifically recognize biological and chemical compounds. Besides, MIT has dramatically attracted many researchers in the field of catalysis, drug delivery, amino acids and proteins, pollutants, food technology, chemical sensors, and separation and purification.2–8 The predictable specificity and selectivity of the biological receptors with favorable environmental stability and reduced cost can be achieved by exploring molecularly imprinted polymer (MIP).9 Besides their low cost, MIPs are easy to synthesize, have good storage stability, can be repeatedly reused without loss of activity, possess excellent mechanical strength, are resistant to heat and pressure, and can withstand chemical media which widens their applicability.10,11 The MIP synthesis follows these steps: (a) the template molecule and the monomer are blended with a rational ratio to form a pre-polymerization complex. The complex formation between the template molecule and the functional monomer is crucial for assembling MIP, and the excess cross-linking monomer envelops the complex. (b) Addition of the initiator and cross-link agent in the mixture (c) Polymerisation is achieved by thermal treatment or ultraviolet radiation. A network of three-dimensional polymers with trapped template molecules is formed following the completion of polymerization. The template molecule is withdrawn from the polymer host, leaving a cavity imprint with structural specificity to the target, which can be used for analyte rebinding.1 (d) The MIP is then subjected to the target-bearing sample, and the cavity selectively recognizes the target molecule and uptakes it from structurally similar compounds.12 The physical characteristics, morphology, and potential of the MIP are influenced by some polymerization factors such as selection in functional monomer, a cross-linking monomer, mixing ratios, reaction temperature, and time.13 Finding the optimal ratios for designing and synthesizing conventional MIP is carried out through trial and error experimentation, which is tedious, costly and not optimal. Computational studies such as molecular dynamics and quantum mechanics can be used to select functional monomers and investigate MIP selectivity by examining the mechanisms of imprinting.14 Computational studies for MIPs with a wide range of highly relevant biochemical species have not been reported.
Cortisol is a stress hormone biomarker critical in physiological processes such as metabolism, electrolyte balance, and blood pressure control that affect cognitive functions, including working memory, sleep habits, and mood.15 It has been reported that the reduction of cortisol in the body system can be associated with muscle weakness and abdominal pain. In contrast, high cortisol levels can lead to depression, obesity, and low immune response.16 Several studies on MIP-based cortisol sensors have been published.15,17–19 In the literature, though based on trial and error, cortisol MIPs were investigated with the functional monomers in the presence of different solvents at a ratio (cortisol/monomer) 1
:
3 (11 functional monomers investigated),20 1
:
4 (1 functional monomer investigated),21,22 1
:
6 (1 functional monomer investigated),23 1
:
10 (1 functional monomer investigated).24
Many experimental investigations have been performed on cortisol MIPs, but the optimization of the functional monomers has been through trial and error.19 Computer simulation has been effective in giving precise information for designing MIP receptors.25 Only a few studies used computational investigation for the optimization of cortisol MIPs pre-polymerization.23 Bearing in mind that the different combinations of functional monomers, crosslinkers and solvents with the template produce MIPs with different selectivity, this study aimed at predicting the best composition ratio for cortisol MIP pre-polymerization based on six functional monomer compositions. The density functional theory (DFT) calculations were used to investigate the mechanism of the intermolecular interaction of cortisol with the functional monomers. The best monomer and suitable solvent were determined through interaction and solvent energies respectively. The frontiers molecular orbitals (FMO) and molecular electrostatic potential (MEP) were used to predict the template and monomer reaction sites for the formation of template-monomer complexes. In addition to DFT calculations, this study used molecular simulation which resembles that of the experimental methods to elucidate the compatibility of template-monomer-crosslinker-solvent of cortisol MIPs which can enhance the optimization of this receptor in the experimental setup. Various parameters such as Flory-Huggins' parameters through blends module, mean square displacement (MSD), diffusion coefficient (D), thermodynamic equilibrium energies, radial distribution function (RDF), cohesive energy density (CED) and solubility parameter (δ), were analyzed to explain the compatibility of the functional monomer with the template in cortisol MIP receptor development. Some of these parameters are quite difficult to analyze through an experimental approach and as such their investigation is very limited in the literature for cortisol MIP receptors.
Computational methods
Density functional theory calculations.
Cortisol (Cort) as a template, six functional monomers (4-vinylpyridine (4VP), acrylic acid (AA), acrylamide (AM), glycidyl methacrylate (GMA), 2-hydroxyethyl methacrylate (HEMA) and methylacrylic acid (MAA)), and one cross-linker (ethylene glycol dimethacrylate (EGDMA)) were retrieved from PubChem. The retrieved structures were optimized using the DFT method B3LYP and basis set 6-31G (d,p) performed with Gaussian 16 software26 to obtain the most stable configuration of the reacting species as well as to determine the binding site through the molecular electrostatic potential (MEP) for probable complexes. During the optimization processes, minimum geometries characterized by a zero-imaginary frequency were obtained for each of the systems. All calculations were performed for global reactivity indices in terms of the one-electron energies of the frontier molecular orbitals (HOMO and LUMO), as defined within the DFT27 according to eqn (1)–(3): |  | (1) |
| Chemical hardness, η = (ELUMO − EHOMO)/2 | (2) |
| 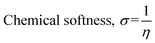 | (3) |
DFT using ORCA 4.2.0 with B3LYP and def2-TZVP28,29 as functional hybrid and basis sets respectively was used for the conformation optimization and energy calculation of the complexes formed between the template and functional monomers. The template-monomer binding energies of the optimized structures are calculated as
| ΔEbinding = Ecomplex − (Etemplate + Emonomer) | (4) |
The best complexes that resulted from template-monomer interactions were submitted for optimization in solvents such as acetonitrile, chloroform, toluene, methanol and water. The calculation in different solvents was performed using a conductor-like polarizable continuum model (CPCM).
30 The binding energies for the solvent effect are calculated as shown in
eqn (5).
| ΔEsolvation = ΔEgas − ΔEsolvent | (5) |
Molecular simulation
Atomistic molecular dynamics simulation can reveal the structures and behaviors of a molecule. To simulate the different types of cortisol MIPs as well as to gain specific intermolecular interactions within the systems, the molecular dynamic simulation was performed following the previous method31 using Material Studio 2020 software.32 Briefly, the simulation cells were constructed through an amorphous cell module with percentage compositions of template-functional monomer to make different ratios (cortisol
:
monomer = 1
:
1, 1
:
2, 1
:
3, 1
:
4, 1
:
5 and 1
:
6) in the presence of crosslinker (EGDMA) and solvent (acetonitrile). When creating the simulation box in the presence of EGDMA or acetonitrile, the weight percent was adjusted to give the desire ratio of cortisol/monomer in the system. The initial density was 1400 kg m−3, optimized using Smart algorithm for up to 5 × 102 steps, using convergence criteria of 8.37 × 10−5 kJ mol−1 for energy, 4.18 kJ mol−1 Å for forces and a displacement of 1.0 × 10−5 Å. The COMPASS III force field33 was used throughout the simulation studies with van der Waals cut off radius of 12 Å. The simulation cells were subjected to MD simulation using the Forcite module with Berendsen thermostat (with a decay constants of 0.1 ps) under the NVT (number of molecules, N, volume, V and temperature, T) canonical ensemble within the temperature range 298–500 K with 5 ramps per cycle and in NPT (pressure, P) isobaric-isothermal ensemble for 5.0 ns at 298 K, 100 kPa and Berendsen thermostat and Barostat (with a decay constants of 0.1 ps). After MD simulation, mean square displacement (MSD), diffusion coefficient (D), thermodynamic equilibrium energies, radial distribution function (RDF), cohesive energy density (CED) and solubility parameter (δ) were analyzed for the systems.
The mixing properties of the functional monomer with cortisol were determined by first optimizing the geometry of the monomers and cortisol using the Smart algorithm for up to 5 × 102 steps. The modified Flory–Huggins approach was employed as implemented in the Blends module of Materials Studio and as applied in the previous studies.31,34,35 Briefly, the mixing energy (Emix), coordination number, and free energy were determined as estimated by sampling from energetically favorable configurations using the volume constraint method, yielding average properties over an ensemble of molecular configurations.31 After the temperature-dependent interactions were determined, the binodal and spinodal curves were computed within the framework of the off-lattice Flory–Huggins theory, along with the critical point of the mixture, thereby yielding the binary phase diagram.31 To determine the coordination numbers, 105 cluster samples with 103 iterations per cluster were used, whereas, for the mixing energy computation, 107 samples were employed with a histogram bin width of 0.0837 kJ mol−1.31 The reference temperature for these calculations was set to 298.15 K.
Results and discussion
Chemical reactivity descriptors of the functional monomers
Studies employing HOMO–LUMO of organic compounds help in understanding the reactivity and stability of the compounds. Fig. 1 depicts the HOMO and LUMO densities with the energy band gap (LUMO–HOMO) of the optimized geometry structures of the cortisol and the functional monomers.
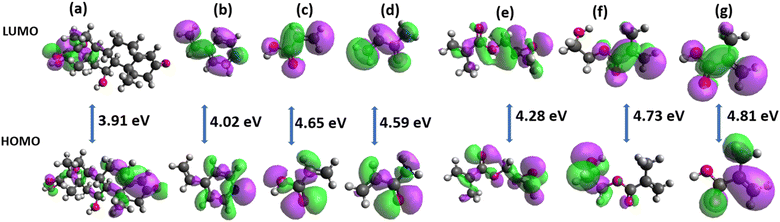 |
| Fig. 1 Ground state density surface plots showing frontier molecular orbitals (a) cortisol (b) 4VP (c) AA (d) AM (e) GMA (f) HEMA (g) MAA. The positive phases are purple, and the negative phases are green. | |
The chemical reactivity descriptors calculated for the functional monomers are presented in Table 1. The energy gap between the HOMO and the LUMO determines the chemical reactivity and the chemical hardness–softness of a molecule. A molecule with a small orbital energy gap is associated with a high chemical reactivity.36 The HOMO-LUMO gap (energy gap) calculated for GMA, HEMA and MAA are within 4.02 and 4.81 eV. The electrophilicity index (ω) measures the stabilization in terms of energy when a system acquires an additional electronic charge from the environment. The higher the value of ω, the greater the chemical reactivity.37 The electrophilicity index calculated for the functional monomers is generally high (ranging between 11.32–14.15 eV). By using the inverse relationship of chemical hardness to obtain global softness, σ, Pérez et al.38 demonstrated in a model that the maximum electrophilicity is found at the softest site of a molecule. As such, a molecule with a high electrophilicity index will be soft, reactive and less stable. As presented in Table 1, all the calculated quantum chemical properties showed that the investigated monomer molecules are highly reactive. In addition, the electrophilicity index measures the ability of a molecule to attract an electron. In other words, the higher the ω and μ, the more electron-accepting power of a molecule. Based on this, the order of electron acceptor for the functional monomers follows 4VP > AA > AM > GMA > MAA > HEMA.
Table 1 Calculated quantum chemical properties of the investigated monomers
Monomer |
E (eV) |
η (eV) |
σ (eV) |
μ (eV) |
ω (eV) |
Cortisol |
3.91 |
1.96 |
0.51 |
−7.16 |
13.12 |
4VP |
4.02 |
2.01 |
0.50 |
−7.54 |
14.15 |
AA |
4.65 |
2.33 |
0.43 |
−7.52 |
12.15 |
AM |
4.59 |
2.29 |
0.44 |
−7.41 |
11.96 |
GMA |
4.28 |
2.14 |
0.47 |
−7.09 |
11.75 |
HEMA |
4.73 |
2.37 |
0.42 |
−7.32 |
11.32 |
MAA |
4.81 |
2.41 |
0.42 |
−7.41 |
11.40 |
Determination of sites of action of the template and functional monomers
The selection of suitable functional monomers is an important task in the design and development of MIP that will have high specificity toward its target molecule. One of the means of achieving this is the computational approach which reduces the cost and time of the experimental investigation. The present study used molecular electrostatic potential (MEP) surfaces in the identification of different potential reactive sites of both the template and the functional monomers. MEP helps in locating the active sites of organic molecules involved in biological activities. The MEP map surfaces of the template and the functional monomers are presented in Fig. 2. The active sites are predicted according to the distribution of the electron cloud. The regions with more red are described as the negative electrostatic spots (atoms found within this region are mostly electron acceptors) while the regions with more blue are described as positive electrostatic spots (atoms found within this region are mostly electron donors). According to Fig. 2, the red regions with high electron densities are localized on oxygen atoms while the blue regions with low electron densities are localized on hydrogen atoms. The negative regions of cortisol are located around O4 and O5 making them susceptible to electrophilic attacks while positive regions are located on H49 and H52 with nucleophilic attacks. These four regions are identified as active sites for cortisol while the active sites for 4VP are N1, AA; O2 and H9, AM; O1, H7 and H8, GMA; O1 and O3, HEMA; O2, O3 and H19 and MAA; O2 and H12. Here, the hydrogen atoms within the blue regions are described as the proton donors and the oxygen atoms within the red regions as the proton acceptors. These reactive sites are responsible for the formation of hydrogen bonds in the non-covalent interaction necessary for the synthesis of the MIP receptor. Cortisol as a template in the study is both an electron donor and an electron acceptor. The functional monomers, 4VP and GMA are electron donors and acceptors respectively, AA, AM, HEMA, and MAA are both electron donors and acceptors. Possible binding sites were proposed by taking all the active sites of cortisol with that of the functional monomer. Different versions were proposed for the template-monomer complex containing a functional monomer having more than one red or blue active site. All versions were created according to the numbering of the element indicated on the MEP surfaces (Fig. 2) and the first numbering was from functional monomer followed by that of the template (Table 2). The probable products were then optimized to obtain the most stable configuration.
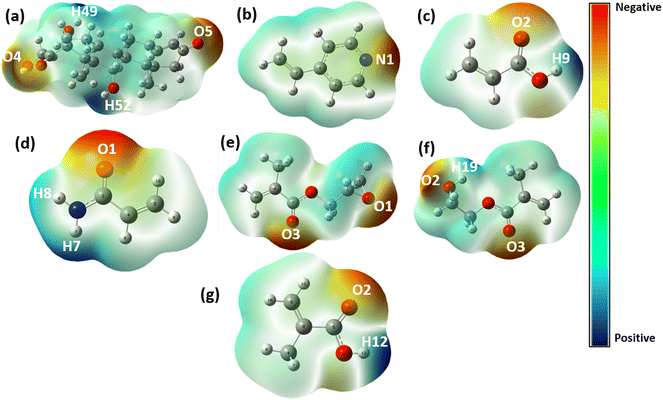 |
| Fig. 2 Molecular electrostatic potential map surfaces of the (a) cortisol, (b) 4VP, (c) AA, (d) AM, (e) GMA, (f) HEMA and (g) MAA with negative electrostatic (red) and positive electrostatic (blue) spots. The positive electrostatic spots are located on the hydrogen atoms while the negative electrostatic spots are located on the oxygen atoms (with higher electron density). | |
Table 2 Bond distances and change in binding energies of the monomer-template complexes in the gas phase
Complex |
Complex versions |
Bond type |
Bond distance (Å) |
ΔE (kcal mol−1) |
Cort-4VP |
Cort-4VP-v1 |
C–N1⋯H52–O |
1.93885 |
−7.871 |
Cort-4VP-v2 |
C–N1⋯H49–O |
1.90555 |
−9.147 |
Cort-AA |
Cort-AA-v1 |
C–O2⋯H49–O |
1.92809 |
−8.745 |
Cort-AA-v2 |
C–O2⋯H52–O |
1.97640 |
−6.562 |
Cort-AA-v3 |
O–H9⋯O4–C |
1.76052 |
−7.815 |
Cort-AA-v4 |
O–H9⋯O5–C |
1.73122 |
−10.838 |
Cort-AM |
Cort-AM-v1 |
C–O1⋯H49–O |
1.83180 |
−8.752 |
Cort-AM-v2 |
C–O1⋯H52–O |
1.91831 |
−7.474 |
Cort-AM-v3 |
O–H7⋯O4–C |
2.02478 |
−6.060 |
Cort-AM-v4 |
O–H7⋯O–C |
2.02688 |
−6.990 |
Cort-GMA |
Cort-GMA-v1 |
C–O3⋯H49–O |
1.89494 |
−6.553 |
Cort-GMA-v2 |
C–O1⋯H49–O |
1.91349 |
−6.582 |
Cort-GMA-v3 |
C–O1⋯H52–O |
2.03134 |
−5.495 |
Cort-GMA-v4 |
C–O3⋯H52–O |
1.96787 |
−6.678 |
Cort-HEMA |
Cort-HEMA-v1 |
O–H19⋯O4–C |
1.93830 |
−5.160 |
Cort-HEMA-v2 |
O–H19⋯O5–C |
1.89252 |
−7.832 |
Cort-HEMA-v3 |
C–O2⋯H49–O |
1.91004 |
−7.136 |
Cort-HEMA-v4 |
C–O2⋯H52–O |
1.94175 |
−5.978 |
Cort-HEMA-v5 |
C–O3⋯H52–O |
1.96556 |
−5.567 |
Cort-HEMA-v6 |
C–O3⋯H49–O |
1.87680 |
−7.476 |
Cort-MAA |
Cort-MAA-v1 |
O–H12⋯O5–C |
1.72548 |
−9.883 |
Cort-MAA-v2 |
O–H12⋯O4–C |
1.76599 |
−11.933 |
Cort-MAA-v3 |
C–O2⋯H49–O |
1.88996 |
−7.485 |
Cort-MAA-v4 |
C–O2⋯H52–O |
1.76633 |
−11.795 |
The binding energies are calculated for the various optimized complexes. The more negative the binding energy, the more stable the complex, and the better the MIP's specificity towards the target molecule. Table 2 presents the complexes resulting from monomer-template interactions derived from various active sites of both the template and the monomers. For 4VP, the most stable complex is Cort-4VP-v2 (ΔE = −9.147 kcal mol−1), for Cort-AA, Cort-AA-v4 (ΔE = −10.838 kcal mol−1), for Cort-AM, Cort-AM-v1 (ΔE = −8.752 kcal mol−1), for Cort-GMA, Cort-GMA-v4 (ΔE = −6.678 kcal mol−1), for Cort-HEMA, Cort-HEMA-v2 (ΔE = −7.832 kcal mol−1), and for Cort-MAA, Cort-MAA-v2 (ΔE = −11.933 kcal mol−1). Overall, the most stable complexes are identified with Cort-MAA with binding energies ranging between −7.485 and −11.933 kcal mol−1. Solvent plays an important role in the template-monomer interactions for the successful development of MIPs. Fig. 3 presents the solvent effect on the binding of the template-monomer complexes. The more negative the ΔEsolvation, the stronger the affinity of the solvent to the solute molecules thus reducing the adsorption selectivity of the MIP. A lower affinity of solvents to the template-monomer complex is essential for successful polymerization during MIP development. In the present study, the best solvent identified for the complexes is toluene with higher binding energies (ranging between −4.65 and −2.02 kcal mol−1) but fewer affinities. The decreasing order of binding affinities follows water > methanol > acetonitrile > chloroform > toluene. This trend observed supports the previous study on the solvent effects on the binding affinities.39,40 In this case, toluene could have been the best solvent for further investigations in the present study, but due to its high volatility which can lead to an increase in viscosity and in turn, can cause phase separation,41 it was not selected. Chloroform is generally used for MIPs due to its relatively apolar properties42 but it is also known as a toxic solvent.43 Methanol can cause hydrogen bonding interference to both the template and the functional monomer.40 Acetonitrile is chosen for further investigation due to its weak hydrogen bonding, water miscibility, and eco-friendly and is listed among the top green chemicals.44,45 The binding energy observed for the Cort-MAA is remarkable in all the solvents and therefore, MAA seems a more suitable monomer for cortisol MIPs. The decreasing order of stability of the complexes in acetonitrile follows Cort-MAA > Cort-GMA > Cort-AA > Cort-AM > Cort-HEMA > Cort-4VP.
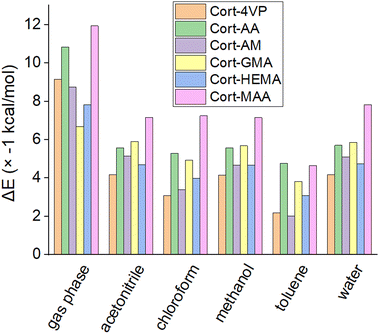 |
| Fig. 3 The binding energies of template-monomer complexes in the gas phase and different solvents. | |
Molecular dynamics simulation
A molecular dynamics simulation is an essential tool when it comes to deeper insights into the mechanisms occurring during MIP developments. The parameters such as Flory–Huggins' parameters through blends, MSD, diffusion coefficient, thermodynamic equilibrium of state, RDF, CED and solubility parameter were investigated for the suitability of the functional monomers to determine their usability in cortisol MIP development.
Template-monomer blends
The Blends module was used to estimate the miscibility behavior of cortisol and the functional monomers. The well-known thermodynamics model of mixing and the most straightforward one is the Flory–Huggins. A parameter to describe the miscibility behavior is Flory–Huggins' interaction, χ (Chi) which is related to the energy of mixing (Emix) according to eqn (6): |  | (6) |
The nearer to zero or negative the χ value is, the better the miscibility. Fig. 4(a) and (b) presented the results for χ and Emix. Equation A shows that χ is directly proportional to Emix and this is what is observed in Fig. 4(a) and (b). According to χ and Emix, the miscibility of the functional monomer with the cortisol can be arranged in the decreasing order as Cort-4VP > Cort-HEMA > Cort-GMA > Cort-AA > Cort-AM > Cort-MAA. The analysis of the phase diagram generated the binodal and spinodal curves as well as the critical points (Fig. S1–S6†). From the critical points of view, 4VP and HEMA exhibited both low and upper critical points (363 and 1270 K) and (83.7 and 9006 K) respectively, AA, AM and MAA did not exhibit both upper and critical points and GMA exhibits only upper critical points (2009 K). The 4VP and HEMA that exhibited low critical points can cause degradation of cortisol on account of miscibility in sufficiently large concentrations at low temperatures. The AA, AM and MAA that did not show any critical points indicate they can be miscible with the cortisol at any temperature.46 The visible and sharp peaks derived from the energy distribution of the potential energy (P(E)) (Fig. 4(c)) also support the miscibility behavior of the complexes. Also, the positive χ values observed for most of the complexes are an indication that functional monomers they contained would have relatively poor miscibility with the cortisol or not follow the ideal mixing pattern.
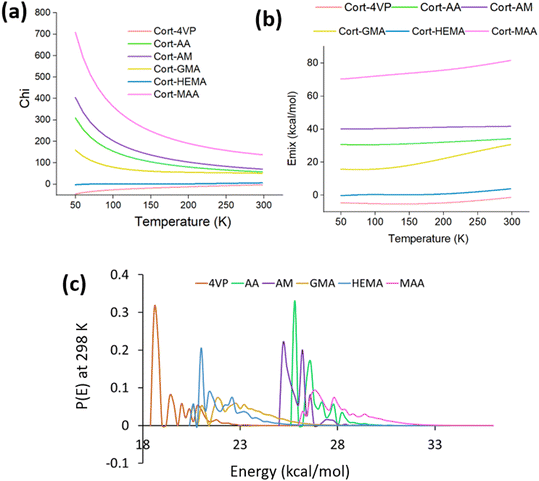 |
| Fig. 4 Flory–Huggins' parameters (a) Chi, (b) mixing energy and (c) binding energy. | |
The dynamic behavior of template-monomer-crosslinker complexes
Thermodynamic equilibrium energies.
The equilibrium of the simulation of template-monomer-crosslinker-solvent was evaluated through the thermodynamic equilibrium energies (potential, kinetic, non-bond and total energy) during the MD simulation. These energies are represented in Fig. S7(a–f)† extracted from the ratio 1
:
2 (cortisol/monomer) systems after 5 ns. The kinetic energy was stable throughout the simulation time for all the systems. The potential, non-bond and total energy decreases until a simulation time of 2.0 ns after which they become fixed with little fluctuation. It can be concluded from the equilibrium achieved for all the energies that the simulation time was sufficient for all the systems.
Radial distribution function.
Radial distribution function (RDF) is the probability of another atom at a particular distance centering around a specific atom49 which reflects the orderliness of a matter along the appearance of peaks at the speculated distances. It means the higher peaks denote how ordered the matter is, leading to more interactions between the substances. The interactions between cortisol, functional monomers and EGDMA at various compositions were further investigated using RDF. RDF analysis of the modeled systems is presented in Fig. 6(a) at r = 1.11 Å which is the maximum of g(r) indicating the internal distance in a molecule. RDF can be used to analyze the covalent bond and secondary interactions between the molecules involved. The sharp peaks (Fig. 6(b)) recorded for the systems according to the composition of the functional monomers may be the covalent molecular interactions through hydrogen bonding with either the H atom of hydroxyl groups or O atom of ketone groups of cortisol and sometimes with both groups of cortisol. For instance, 4VP as a functional monomer is inhibited by the N atom, AA by O and H atoms from the carboxylic group, AM by the two H and O atoms from the amide group, GMA by the O atoms of methacrylate and epoxy group, HEMA by the O atoms from hydroxyl and methacrylate groups and MAA by the H and O atoms of the carboxylic group. All these atoms mentioned for the functional monomers are the atoms that can form imprinting sites for the cortisol MIP receptor. Since the investigation was in the presence of EGDMA as a crosslinker, there may be contributions to the sharp peaks observed for all the systems from its two methacrylate groups. The highest peaks attained are within 328.09 and 415.21 which belongs to Cort-AM and Cort-AA at ratio 1
:
4 and 1
:
1 (cortisol/monomer) respectively. The higher peaks depict stronger interaction50 which were generally observed in the systems with a 1
:
4 (cortisol/monomer) ratio except for Cort-HEMA and Cort-AM which were low. The order of RDF in 1
:
4 based on the composition of functional monomer follows Cort-MAA > Cort-AA > Cort-GMA > Cort-AM > Cort-HEMA > Cort-4VP. High RDF was generally observed for all the systems containing functional monomers with carboxylic and methacrylate groups. This effect is obvious with the complexes of Cort-MAA and Cort-AA containing functional monomers with a carboxylic group. This is because the oxygen atoms in the carboxylic group of the functional monomer can inhibit strong hydrogen bond formation with the cortisol's hydrogen atoms.51 Other peaks between 1.3 and 5.0 could have corresponded to non-hydrogen atoms.
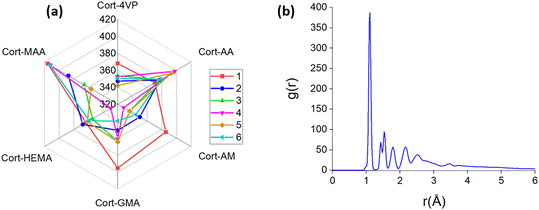 |
| Fig. 6 Radial distribution function, g(r) (a) extracted for all the complexes from ratio 1–6 (monomer/cortisol) at r = 1.11 Å and (b) Cort-MAA demonstrating the sharp peak at r = 1.11 Å (resulted from hydrogen bonding with either the H atom of hydroxyl groups or O atom of ketone groups of cortisol) as observed for all the complexes. | |
The stability and solubility of template-monomer-crosslinker-solvent complexes
Stability.
The stability of the complexes was investigated in the presence of acetonitrile as the solvent. Considering the template-monomer-crosslinker-solvent, MSD as the characterization of the diffusion coefficient in a different system, it was generally low at a ratio 1
:
4 (cortisol/monomer) of cortisol to functional monomer Fig. S8.† In the presence of acetonitrile, the result of the analysis of MSD is much lower (ranged between 1.87 and 2.72 Å2) compared to the previous report for only the template-monomer-crosslinker (ranged between 17.23 and 59.68 Å2). The stronger the hydrophobic interaction of a system, the lower the MSD/diffusion coefficient of the system and therefore promoting the hydrogen bonding interaction between the functional monomer and the template molecule.52 This could have happened in the presence of acetonitrile through the hydrophobic interactions of the organic mobile phase are very weak.
The equilibrium of the simulation of template-monomer-crosslinker-solvent was evaluated through the potential, kinetic, non-bond and total energy during the MD simulation. These energies are represented in Fig. 7 extracted from the ratio 1
:
4 (cortisol/monomer) system after 5.0 ns. The kinetic energy was stable throughout the simulation time for the systems. The system containing 4VP as the functional monomer achieved equilibrium for the potential and total energy after 1.8 while after 4.0 ns for non-bond, AA after 2.4 ns for potential and total energy but 4.0 ns for non-bond, AM after 2.0 ns for potential and total energy but 3.0 ns for non-bond, GMA after 0.9 ns for potential and total energy but 2.0 ns for non-bond, HEMA after 1.5 ns for potential and total energy but 4.0 ns for non-bond and MAA after 2.4 ns for potential and total energy but 4.0 ns for non-bond. This is an indication that the simulation time was sufficient for all the systems based on the thermodynamic equilibrium achieved for all the energies. According to the composition of the functional group, the final potential energy follows the order of Cort-AM = Cort-GMA = Cort-HEMA < Cort-4VP < Cort-MAA < Cort-AA, non-bond energy follows Cort-AM < Cort-GMA < Cort-HEMA < Cort-4VP < Cort-MAA < Cort-AA and total energy follow Cort-GMA < Cort-AM = Cort-HEMA < Cort-4VP < Cort-MAA < Cort-AA. This shows that the complexes containing neutral (AM, GMA & HEMA) and the basic (4VP) functional monomers were more thermodynamically stable than the acidic ones (MAA & AA).
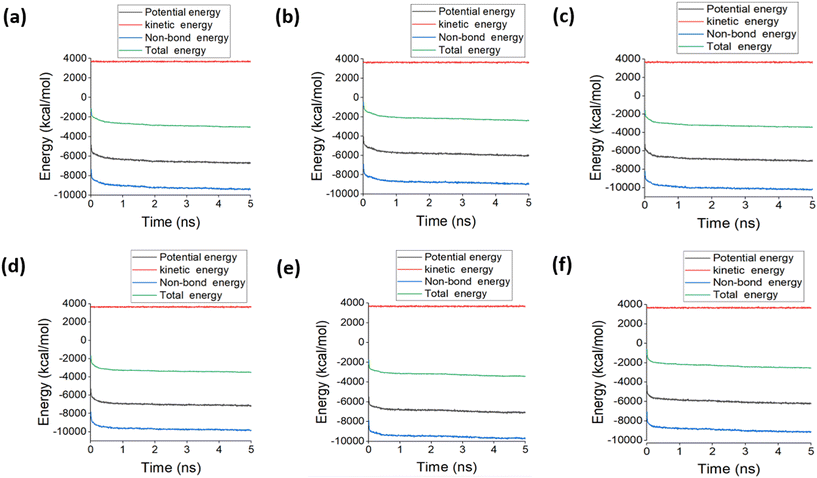 |
| Fig. 7 Thermodynamic equilibrium energies observed for the systems at ratio 1 : 4 (monomer/cortisol) during 5.0 ns simulation time. (a) Cort-4VP, (b) Cort-AA, (c) Cort-AM, (d) Cort-GMA, (e) Cort-HEMA and (f) Cort-MAA. | |
Solubility of the complexes in acetonitrile.
The aim of investigating the solubility of the complexes is to predict at what ratio is the best fit for the systems containing a different composition of the functional monomers. The Flory–Huggins approach was used to estimate the solubility of template-monomer-crosslinker in acetonitrile towards the development of cortisol MIP receptors. The solubility parameters (δ) which is a square root of cohesive energy density (CED) of the different complexes were investigated focusing on the ratio of cortisol to the functional monomer in the composition. The smaller the δ between two components, the higher the miscibility of the two53 resulting in a stronger interaction. According to the plots presented in Fig. 8, the δ ranged between 8.84 and 30.79 (J cm−3)1/2. The lowest δ reported for different ratios (cortisol/monomer) Cort-GMA is at 1
:
3 (23.14 (J cm−3)1/2), Cort-MAA, Cort-4VP and Cort-HEMA are at 1
:
4 (9.97, 17.92 and 22.49 (J cm−3)1/2 respectively), Cort-AA and Cort-AM at 1
:
6 (17.22 and 19.14 (J cm−3)1/2 respectively). This indicated a possible ratio to achieve the best cortisol MIPs using the functional monomers. The Cort-MAA showed the lowest δ in ratio 1
:
4 which is equivalent to the ratio observed in the previous studies for the successful synthesis of magnetic and nano-based cortisol MIPs21,22 using MAA and HEMA respectively. The rationale behind the selected ratio was not reported, but the present study showed that this would be the suitable ratio for the development of cortisol MIP with MAA as a functional monomer. In an experimental setup, the effects of functional monomer, crosslinker and solvents on the binding analysis of corticosteroids using cortisol as the template for the MIPs with the ratio of 1
:
3 (template/monomer) was investigated.20 The importance of investigating cortisol MIPs in a different ratio is confirmed. For instance, MAA and AA which look more promising in the present research showed fewer binding affinities while AM and 4VP proved more efficient than others in the previous report because it was limited to a ratio of 1
:
3.20 If the ratio had been varied, a better result could have been obtained. Notwithstanding, comparing the trends of the result presented in a ratio 1
:
3 of the present study to the previous reports,20 there is an agreement for the activities of 4VP and AM. The δ reported for AM at a ratio of 1
:
3 in Fig. 9 was lower (better) than that of 4VP. Also, looking at the result of MSD and diffusion coefficient presented in Fig. 5(a) and (d) respectively at the same ratio of 1
:
3, AM results were still better than those of 4VP. And generally, the results presented in Fig. 6 for RDF and Fig. 7 for thermodynamic energies showed that AM would be more suitable as a functional monomer for cortisol MIPs than 4VP. The 3D-isosurface density field at the individual best solubility ratio is shown in Fig. 9.
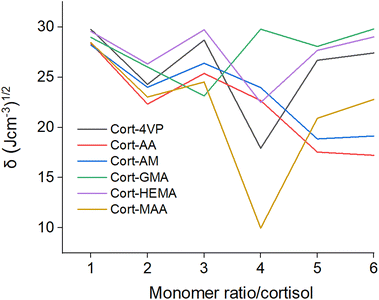 |
| Fig. 8 Solubility parameter (δ) for all the systems at ratio 1–6 (monomer/cortisol). | |
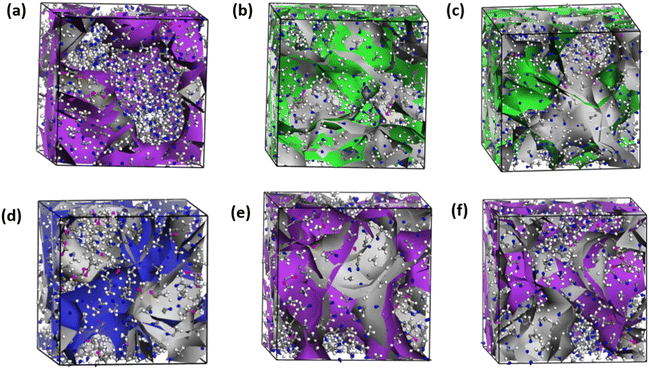 |
| Fig. 9 3D-isosurfaces of the complexes at the lowest solubility parameter for (a) Cort-4VP, (b) Cort-AA, (c) Cort-AM, (d) Cort-GMA, (e) Cort-HEMA and (f) Cort-MAA with color indicating monomer/cortisol ratio (purple = 1 : 4, green = 1 : 6 and blue = 1 : 3). | |
Cortisol imprinted polymer selectivity prediction
One of the methods to evaluate the selectivity of MIP against the target molecule is adsorption.21,22 The adsorption locator module of Material Studio 2020 was used to investigate the selectivity of the predicted cortisol-MIP against cortisol and other steroids and non-steroid molecules (Fig. 10). Firstly, cortisol was removed from the simulated system containing 1
:
4 ratio of cortisol/MAA and thereafter the imprinted polymer left was used for the adsorption of cortisol and other tested structures. The results are presented in Table 3. Adsorption locator computations investigated the selectivity of cortisol-MIP as compared to cortisol and other steroids and non-steroid molecules. The results of these calculations demonstrated a higher affinity for cortisol and other similar compounds, with dexamethasone having the adsorption energy (−261.65 kcal mol−1) closest to that of cortisol (−269.67 kcal mol−1). A reason for this may be its structural similarity, which is supported by experimental evidence from the literature (including a comparison with cortisone).20 The energy of stigmasterol was found to be very similar to that of cholesterol, an observation which is also supported by experiments found in the literature.54 The affinity of progesterone for cortisol-MIP is lower than that of testosterone, even though they both possess a similar structure. This observation was also supported by previous studies published in the literature.20 The adsorption energy for caffeine (118.86 kcal mol−1) is too poor which is expected as the structure is different (non-steroid) from the steroid structures investigated. Caffeine was included to test the lock and key of the predicted MIP for cortisol detection.
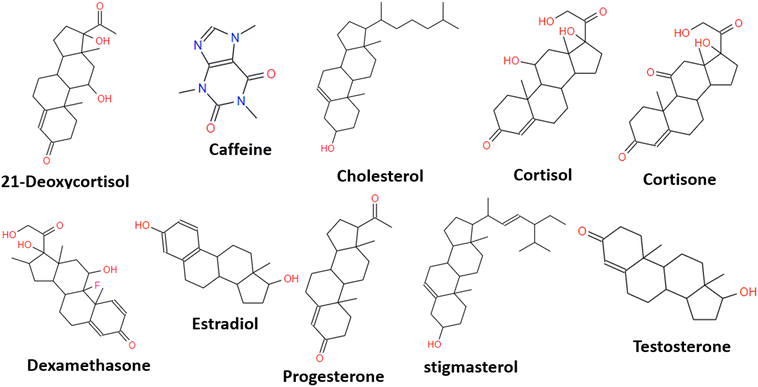 |
| Fig. 10 Steroids and non-steroids (caffeine) used for selectivity testing. | |
Table 3 The adsorption energies of steroids and non-steroid structures investigated
|
Adsorption energy kcal mol−1 |
Cortisol |
−269.67 |
Dexamethasone |
−261.65 |
Estradiol |
−227.07 |
Cortisone |
−209.42 |
21-Deoxycortisol |
−185.90 |
Stigmasterol |
−168.62 |
Cholesterol |
−168.16 |
Testosterone |
−129.60 |
Progesterone |
−54.33 |
Caffeine |
118.86 |
Conclusion
In this article, we examined the binding of six different functional monomers including glycidyl methacrylate (GMA), methylacrylic acid (MAA), 2-hydroxyethyl methacrylate (HEMA), 4-vinylpyridine (4VP), acrylamide (AM) and acrylic-acid (AA) to stress hormone biomarker cortisol selective MIPs. The aim was to investigate the best composition ratio of different functional monomers to elucidate precise information for designing MIP receptors that can efficiently recognize and isolate cortisol from biofluid samples. This type of report is lacking in the literature for cortisol MIPs. In the study, for the first time, a computational approach was used to investigate the selection techniques of the six common experimental proven functional monomers for the cortisol MIPs in different compositions. The simulated results were compared with the available reported experimental results to derive a reasonable conclusion. The crosslinker involved was EGDMA and five porogenic solvents (acetonitrile, chloroform, toluene, methanol and water). The intermolecular hydrogen bonds and the template-monomer binding energies obtained through DFT calculations suggested Cort-MAA as the most stable complex both in the gas phase and solvents followed by Cort-AA and then Cort-GMA. The solvent selected for MIPs development should have less interference with non-covalent template-monomer interactions. Among the five porogenic solvents investigated, acetonitrile proved to be more suitable for cortisol MIPs preparation.
For MD simulations, a series of analyses were performed including Flory–Huggins' parameters such as χ and mixing energy, mean square displacement (MSD), diffusion coefficient (D), thermodynamic equilibrium energies, radial distribution function (RDF), cohesive energy density (CED) and extended Flory–Huggins' parameters such as solubility parameter (δ). Considering the χ values generated along with the critical points during the blend analysis, it is concluded that the mixing behavior of the functional monomer with the cortisol may not follow an ideal mixing pattern in the development of cortisol MIP receptors. For template-monomer-crosslinker (EGDMA), all complexes through MSD and diffusion coefficient analyses, were generally stable at a ratio of 1
:
2 (cortisol/monomer) except Cort-AM at 1
:
5. The equilibrium of the systems was established through the potential, kinetic, non-bond and total energy during the MD simulation. The RDF analysis revealed the high contributions of carboxylic and methacrylate groups to the sharp peaks observed as a result of strong hydrogen bonding most especially the systems containing AA and MAA as functional monomers and mostly at a ratio of 1
:
4. Considering the template-monomer-crosslinker-solvent, low MSD was observed at ratio 1
:
4 (cortisol/monomer). A solvent effect was observed on the stability of the complexes through MSD as the reported values were much lower with the systems with acetonitrile (ranged between 1.87 and 2.72 Å2) than for the systems in the absence of the solvent (ranged between 17.23 and 59.68 Å2). This is an indication of a high level of stability in the presence of acetonitrile. To ascertain that the systems were well equilibrated, the potential, kinetic, non-bond and total energy were analyzed. The thermodynamic energies were much lower in the systems containing neutral and basic functional monomers than in those that contained acidic ones. The results for solubility parameters showed that the complexes had their least solubility values in a different ratio. Notwithstanding, three of the complexes had the lowest solubility parameters at a ratio of 1
:
4.
Generally, the results presented for AM and 4VP in the present study agreed with the previously reported experimental investigation that AM would be a more suitable functional monomer than 4VP for cortisol MIP receptors. With the computational results and the previously reported experimental results, MAA, AA, and GMA may be considered the most suitable functional monomers in acetonitrile at a ratio of 1
:
4 (cortisol/monomer). All other functional monomers considered in the present study look promising as potential monomers in the development of cortisol MIP receptors as their results are not too far from MAA.
A key benefit of the approach presented in this study, as opposed to other methodologies described in the literature, is that it does not hinder the assessment of the functional monomer at the level of DFT, as commonly occurs. Instead, the analysis is extended to include all functional monomers under investigation, subjecting each of them to MD simulations for detailed scrutiny based on insights yielded by DFT calculations. The insufficiency of quantum chemical calculations in this regard occurs because of complexities associated with attempting to model the behavior and features of molecules within a large system. There are limitations in the inability to continue onward with all investigated solvents in terms of DFT to MD simulation and vary parameters such as crosslinkers different from EGDMA. While such constraints may apply, it is important to note that addressing all of them with absolute certainty within a single article is not feasible and hence such considerations can suggest directions for future research efforts. It is worth noting that the findings presented in the present article provide further support and additional insights into the previously reported experimental results for cortisol-MIPs.
Author contributions
V. T. Adeleke: conceptualization, data curation, formal analysis, methodology, software, validation, visualization, writing – original draft, writing – review & editing, O. Ebenezer: conceptualization, methodology, writing – original draft, writing – review & editing, M. Lasich: conceptualization, data curation, formal analysis, methodology, project administration, resources, software, supervision, validation, visualization, writing – review & editing and S. M. Mugo: conceptualization, methodology, project administration, validation, writing – review & editing.
Conflicts of interest
The authors declare no conflicts of interest.
Acknowledgements
The Mangosuthu University of Technology is appreciated for the Postdoctoral Fellowship award and Centre for High Performance Computing (CHPC) South Africa for resources used in the simulation studies.
References
- G. Vasapollo, R. Del Sole, L. Mergola, M. R. Lazzoi, A. Scardino, S. Scorrano and G. Mele, Int. J. Mol. Sci., 2011, 12, 5908–5945 CrossRef CAS PubMed.
- G. Guan, J. H. Pan and Z. Li, Chemosphere, 2021, 265, 129077 CrossRef CAS PubMed.
- D. Juric, N. A. Rohner and H. A. von Recum, Macromol. Biosci., 2019, 19, 1800246 CrossRef PubMed.
- W. Zhang, Y. Zhang, R. Wang, P. Zhang, Y. Zhang, E. Randell, M. Zhang and Q. Jia, Anal. Chim. Acta, 2022, 1234, 340319 CrossRef CAS PubMed.
- Y. Liu, Z. Lian, F. Li, A. Majid and J. Wang, Mar. Pollut. Bull., 2021, 169, 112541 CrossRef CAS PubMed.
- C. Huang, H. Wang, S. Ma, C. Bo, J. Ou and B. Gong, J. Chromatogr. A, 2021, 1657, 462579 CrossRef CAS PubMed.
- O. Cakir, M. Bakhshpour, F. Yilmaz and Z. Baysal, Mater. Sci. Eng., C, 2019, 102, 483–491 CrossRef CAS PubMed.
- M. I. Malik, H. Shaikh, G. Mustafa and M. I. Bhanger, Sep. Purif. Rev., 2019, 48, 179–219 CrossRef CAS.
- A. G. Ayankojo, J. Reut, A. Öpik, A. Furchner and V. Syritski, Biosens. Bioelectron., 2018, 118, 102–107 CrossRef CAS PubMed.
- H. Yan and K. H. Row, Int. J. Mol. Sci., 2006, 7, 155–178 CrossRef CAS.
- G. Vlatakis, L. I. Andersson, R. Müller and K. Mosbach, Nature, 1993, 361, 645–647 CrossRef CAS PubMed.
- E. N. Ndunda, J. Mol. Recognit., 2020, 33, e2855 CrossRef CAS PubMed.
- W. J. Cheong, F. Ali, J. H. Choi, J. O. Lee and K. Yune Sung, Talanta, 2013, 106, 45–59 CrossRef CAS PubMed.
- S. Suryana, Mutakin, Y. Rosandi and A. N. Hasanah, Molecules, 2021, 26 Search PubMed.
- S. M. Mugo, W. Lu and S. Robertson, Biosensors, 2022, 12 Search PubMed.
- W. Tang, L. Yin, J. R. Sempionatto, J.-M. Moon, H. Teymourian and J. Wang, Adv. Mater., 2021, 33, 2008465 CrossRef CAS PubMed.
- S. M. Mugo and J. Alberkant, Anal. Bioanal. Chem., 2020, 412, 1825–1833 CrossRef CAS PubMed.
- S. M. Mugo, J. Alberkant, N. Bernstein and O. V. Zenkina, Anal. Methods, 2021, 13, 4169–4173 RSC.
- K. Sreenivasan, Angew. Makromol. Chem., 1997, 246, 65–69 CrossRef CAS.
- C. Baggiani, P. Baravalle, C. Giovannoli, L. Anfossi and G. Giraudi, Biosens. Bioelectron., 2010, 26, 590–595 CrossRef CAS PubMed.
- J. E. L. Villa, S. Khan, L. C. S. Neres and M. D. P. T. Sotomayor, J. Polym. Res., 2021, 28, 298 CrossRef CAS.
- G. E. Yılmaz, Y. Saylan, I. Göktürk, F. Yılmaz and A. Denizli, Biosensors, 2022, 12 Search PubMed.
- E. Daniels, Y. L. Mustafa, C. Herdes and H. S. Leese, ACS Appl. Bio Mater., 2021, 4, 7243–7253 CrossRef CAS PubMed.
- C. Baggiani, G. Giraudi, F. Trotta, C. Giovannoli and A. Vanni, Talanta, 2000, 51, 71–75 CrossRef CAS PubMed.
- T. Cowen, K. Karim and S. Piletsky, Anal. Chim. Acta, 2016, 936, 62–74 CrossRef CAS PubMed.
-
M. J. Frisch, G. W. Trucks, H. B. Schlegel, G. E. Scuseria, M. A. Robb, J. R. Cheeseman, G. Scalmani, V. Barone, G. A. Petersson, X. Nakatsuji, H. Li, M. Caricato, A. V. Marenich, J. Bloino, B. G. Janesko, R. Gomperts, B. Mennucci, H. P. Hratchian, J. V. Ortiz, A. F. Izmaylov, J. L. Sonnenberg, D. Williams-Young, F. Ding, F. Lipparini, F. Egidi, J. Goings, B. Peng, A. Petrone, T. Henderson, D. Ranasinghe, V. G. Zakrzewski, J. Gao, N. Rega, G. Zheng, W. Liang, M. Hada, M. Ehara, K. Toyota, R. Fukuda, J. Hasegawa, M. Ishida, T. Nakajima, Y. Honda, O. Kitao, H. Nakai, T. Vreven, K. Throssell, J. A. Montgomery Jr, J. E. Peralta, F. Ogliaro, M. J. Bearpark, J. J. Heyd, E. N. Brothers, K. N. Kudin, V. N. Staroverov, T. A. Keith, R. Kobayashi, J. Normand, K. Raghavachari, A. P. Rendell, J. C. Burant, S. S. Iyengar, J. Tomasi, M. Cossi, J. M. Millam, M. Klene, C. Adamo, R. Cammi, J. W. Ochterski, R. L. Martin, K. Morokuma, O. Farkas, J. B. Foresman and D. J. Fox, Gaussian 16, Revision B.01, Gaussian, Inc., Wallingford CT, 2016 Search PubMed.
- R. G. Parr and W. Yang, J. Am. Chem. Soc., 1984, 106, 4049–4050 CrossRef CAS.
- F. Neese, WIREs Comput. Mol. Sci., 2012, 2, 73–78 CrossRef CAS.
- F. Weigend and R. Ahlrichs, Phys. Chem. Chem. Phys., 2005, 7, 3297–3305 RSC.
- V. Barone and M. Cossi, J. Phys. Chem. A, 1998, 102, 1995–2001 CrossRef CAS.
- M. Lasich and V. T. Adeleke, J. Mol. Liq., 2023, 377, 121577 CrossRef CAS.
-
D. Frenkel, Monte Carlo Simulations, in Computer Modelling of Fluids Polymers and Solids, ed. C. R. A. Catlow, S. C. Parker and M. P. Allen, Nato Science Series C, Springer, Dordrecht, 1990, pp. 83–123 Search PubMed.
- H. Sun, J. Phys. Chem. B, 1998, 102, 7338–7364 CrossRef CAS.
- R. Hafizi, R. A. Taheri and H. Moghimi, J. Mol. Liq., 2018, 266, 535–539 CrossRef CAS.
- S. M. Khumalo, M. Lasich, B. F. Bakare and S. Rathilal, Water, 2022, 14 Search PubMed.
- B. J. Powell, T. Baruah, N. Bernstein, K. Brake, R. H. McKenzie, P. Meredith and M. R. Pederson, J. Chem. Phys., 2004, 120, 8608–8615 CrossRef CAS PubMed.
- S. J. Enoch, M. T. D. Cronin, T. W. Schultz and J. C. Madden, Chem. Res. Toxicol., 2008, 21, 513–520 Search PubMed.
- P. Pérez, L. R. Domingo, M. José Aurell and R. Contreras, Tetrahedron, 2003, 59, 3117–3125 CrossRef.
- L. Xie, N. Xiao, L. Li, X. Xie and Y. Li, Int. J. Mol. Sci., 2020, 21 Search PubMed.
- L. Wu, K. Zhu, M. Zhao and Y. Li, Anal. Chim. Acta, 2005, 549, 39–44 CrossRef CAS.
- R. H. Schmidt, A.-S. Belmont and K. Haupt, Anal. Chim. Acta, 2005, 542, 118–124 CrossRef CAS.
- T. Z. Mordasini Denti, W. F. van Gunsteren and F. Diederich, J. Am. Chem. Soc., 1996, 118, 6044–6051 CrossRef.
- L. Vajrabhaya, S. K. Suwannawong, R. Kamolroongwarakul and L. Pewklieng, Oral Surg. Oral Med. Oral Pathol. Oral Radiol. Endod., 2004, 98, 756–759 CrossRef PubMed.
- A. Singh, D. Gangopadhyay, R. Nandi, P. Sharma and R. K. Singh, J. Raman Spectrosc., 2016, 47, 712–719 CrossRef CAS.
-
E. Yilmaz and M. Soylak, Type of green solvents used in separation and preconcentration methods, in New Generation Green Solvents for Separation and Preconcentration of Organic and Inorganic Species, ed. M. Soylak and E. Yilmaz, Elsevier, 2020, ch. 5, pp. 207–266 Search PubMed.
- I. Salahshoori, M. Namayandeh Jorabchi, K. Valizadeh, A. Yazdanbakhsh, A. Bateni and S. Wohlrab, J. Mol. Liq., 2022, 363, 119793 CrossRef CAS.
- F. Pan, F. Peng and Z. Jiang, Chem. Eng. Sci., 2007, 62, 703–710 CrossRef CAS.
- K. Wang and W. Zhu, Comput. Mater. Sci., 2020, 178, 109643 CrossRef CAS.
- E. Lowry, M. Sedghi and L. Goual, J. Mol. Liq., 2017, 230, 589–599 CrossRef CAS.
- A. Hatami, I. Salahshoori, N. Rashidi and D. Nasirian, Chin. J. Chem. Eng., 2020, 28, 2267–2284 CrossRef CAS.
- Y. Du, Y. Zhu, Y. Ji, H. Xu, H. Zhang and S. Yuan, J. Mol. Liq., 2021, 325, 115161 CrossRef CAS.
- C. Yu, O. Ramström and K. Mosbach, Anal. Lett., 1997, 30, 2123–2140 CrossRef CAS.
- H. Abou-Rachid, L.-S. Lussier, S. Ringuette, X. Lafleur-Lambert, M. Jaidann and J. Brisson, Propellants, Explos., Pyrotech., 2008, 33, 301–310 CrossRef CAS.
- M. P. Pešić, M. D. Todorov, G. Becskereki, G. Horvai, T. Ž. Verbić and B. Tóth, Talanta, 2020, 217, 121075 CrossRef PubMed.
|
This journal is © The Royal Society of Chemistry 2024 |