DOI:
10.1039/D4NA00318G
(Paper)
Nanoscale Adv., 2024,
6, 3887-3894
Methylene blue removal using a nanomagnetic support: a response surface approach†
Received
17th April 2024
, Accepted 8th June 2024
First published on 18th June 2024
Abstract
Textile wastewater is commonly released into water bodies without appropriated treatment, resulting in environmental damages. Processes involving separation and adsorption using nanomagnetic supports have been considered a promising alternative to address this concern. However, challenges concerning the low stability of nanoparticles and the reproducibility of experiments make their large-scale application difficult. In this study, we evaluated the efficiency of methylene blue (MB) removal by Fe3O4 nanoparticles coated with sodium dodecyl sulfate (MNP-SDS). The nanomaterial was characterized by transmission electron microscopy (TEM), X-ray diffraction (XRD), Raman spectroscopy, and Fourier-transform infrared spectroscopy (FTIR). The adsorption process was optimized in two stages using factorial design. In the first stage the most influential variables (reaction time, temperature, agitation, pH, and dye concentration) were selected based on the existing literature and applied in a fractional factorial (2(5–1)). In the second stage, the main variables identified were used in a complete factorial (32). The highest removal percentage was obtained using 15 g L−1 of MNP-SDS, which led to a qexp of 1.5 mg g−1. Isothermal analysis parameters and a negative Gibbs free energy indicate that the process was spontaneous, favorable, and that the data were best fitted to the Langmuir model.
Introduction
Methylene blue (MB) is an organic chloride salt commonly applied in the wood, silk, and linen processing industries.1 Despite its importance, it can also be highly toxic, potentially leading to severe skin irritation, burns, respiratory failure, and even cancer.1,2 Because of its chemical structure, which consists of aromatic rings, MB exhibits high stability and resistance to both photo and thermal degradation. Thus, its removal from industrial wastewater has been the goal of several studies.3,4 Processes such as coagulation and flocculation,5 ozonation,6 electrochemical degradation,7 and filtration8 have been considered. However, issues such as high complexity and cost can render them unfeasible.9
Adsorption is another example of a pollutant removal technique characterized by high performance, even when applied to low concentrations of pollutants. In general, the process involves the transfer of the mass of molecules present in a liquid or gaseous medium (adsorbate) to the surface of a solid (adsorbent).10 Different materials can be used as adsorbents, varying both in composition polymers, metals, carbon materials and others11–13 and structure nanoparticles, nanotubes, gels, foams, membranes, films and others.14–17 The properties of the adsorbent such as surface area, porosity, active sites available on the surface of the adsorbent, surface charge and others are directly related to the performance of the adsorption process.18 Therefore, proper consideration of these characteristics and their relationship with the adsorbate can result in the separation of contaminants with a high degree of efficiency. A good example is the study carried out by El-Desouky et al.,19 where they obtained an adsorption capacity of tartrazine in aqueous solution of 710.75 mg g−1 using an organic framework alginate air-gel composite sponge as adsorbent, while Alsawat20 synthesized a CuSnO2TiO2 nanocomposite that showed a high adsorption capacity for Congo red dye, presenting a maximum adsorption capacity of 277 mg g−1.
In general, magnetic nanoparticles stand out as excellent adsorbents. They typically offer high adsorption performance, mainly due to their large surface area, in addition to low cost and potential for reuse.9 However, the agglomeration phenomenon can effectively reduce the magnetism of MNPs, creating a bottleneck in the application of this technique. A highly effective solution to circumvent this issue is the functionalization of nanoparticles with molecules such as inorganic acids, surfactants, and polymers.9,21 Surfactants are often described in the literature as barriers that not only prevent agglomeration but also modify the surface charge of MNPs.21,22 Sodium dodecyl sulfate (SDS), for instance, is an anionic surfactant23 capable of interacting with Fe3O4 nanoparticles, resulting in highly negative nanoparticles that can interact with the positive charges present on the surface of certain compounds, such as MB.24
Although the outcome is usually satisfactory, the large number of variables involved in the process can interfere with adsorption efficiency.25 In this scenario, factorial design experiments are an important asset, owing to their simplicity, reduced number of experiments, and the ability to simultaneously investigate multiple factors, allowing for the assessment of the relevance and statistical significance of the variables under study. Fractional factorial design is crucial in initial optimization steps, while the full factorial approach (second order; e.g., central composite design) can polish the results by combining levels of factors.26
In the present study, we synthesized iron oxide nanoparticles and functionalized them with SDS, aiming to address MB removal. The NPs were characterized using TEM, XRD, Raman spectroscopy, and FTIR spectroscopy. The efficiency of MB removal was first analyzed using a fractional factorial (2(5–1)), in which the range of variables used (time, temperature, pH, agitation, and concentration of reagents) was based on a thorough literature review. The most significant variables were optimized by a full factorial design experiment (32). In addition, the affinity between functionalized nanoparticles and MB and the maximum adsorption capacity were investigated through isothermal experiments, by fitting the data to the Langmuir and Freundlich models. Information on the spontaneity of the process was obtained through the calculation of the Gibbs free energy variation (ΔG°). Our results contribute to the comprehension of the adsorption process using nanomagnetic supports and experiment optimization, paving the way for future industrial-scale applications.
Methods
Materials
FeCl2·4H2O (Sigma-Aldrich 44939), FeCl3·6H2O (Sigma-Aldrich F2877), ammonium hydroxide (Proquimios), sodium hydroxide (Neon 1824), hydrochloric acid (Neon 1789), sodium dodecyl sulfate (Sigma-Aldrich, L5750), methylene blue (Neon, L44107), Argon (99.99%, White Martins, Brazil), and ultrapure water (Millipore, Synergy UV).
Synthesis, functionalization, and characterization of magnetic nanoparticles
MNPs were synthesized by the chemical coprecipitation method. In a volumetric flask, 40 mL of 0.09 M FeCl2·4H2O and 40 mL of 0.18 M FeCl3·6H2O were combined. After complete salt dissolution, 7.5 mL of NH4OH (28%) was added at a flow rate of 5 mL min−1, under constant stirring, for 10 min at 65 °C, resulting in a black precipitate (Fe3O4). At this stage, the reaction was placed in an ice bath for immediate interruption. The resulting nanoparticles were washed three times with ultrapure water and functionalized using 2% SDS (CH3(CH2)11SO4Na), by stirring them together for 15 min at 25 °C under constant agitation (150 rpm). The functionalized nanoparticles (MNP-SDS) were washed three times for removing unreacted SDS, resuspended in ultrapure water, and stored at 8 °C. The nanomaterial was characterized by transmission electron microscopy (TEM), X-ray diffraction (XRD), Raman spectroscopy, and Fourier-transform infrared spectroscopy (FTIR).
Factorial design
On account of the wide range of variables involved in the adsorption process, we carried out a preliminary screening of the most influent variables by applying a fractional factorial design 2(5–1). The variables were selected based on a comprehensive literature review (Tables S1 and S2 ESI†). Following this screening, a full factorial design (32) was conducted, in order to optimize the process and determine the best experimental conditions for MB removal. Tables 1 and 2 present the simplified matrix of the fractional factorial design 2(5–1) and that of the complete factorial design (32), respectively. Detailed matrices for the fractional and complete factorial designs can be found in the ESI (Tables S3 and S4).†
Table 1 Simplified matrix of the fractional factor design 2(5–1)
Variable |
Min. level (−) |
Max. level (+) |
Time (min) |
1 |
20 |
Temperature (°C) |
25 |
45 |
pH |
5 |
9 |
Adsorbent mass (mg) |
5 |
25 |
Agitation (rpm) |
100 |
300 |
Table 2 Simplified matrix of the complete factorial design (32)
Variable |
Min. level (−) |
(0) |
Max. level (+) |
Time (min) |
1 |
31 |
61 |
Temperature (°C) |
20 |
30 |
40 |
Experiments were carried out in an orbital shaker, using 10 mL of MB dye (20 mg L−1) and 1 mL of MNP-SDS. At the end of the reaction time, the solution was exposed to a magnetic field for 15 min and the concentration of MB in the supernatant determined by UV-vis spectrophotometry (685 nm).
Adsorption study
Following the optimization step, the adsorption curve experiments were conducted with the variables and levels fixed as such: reaction time, 32.2 min; temperature, 22.6 °C; agitation, 150 rpm; pH 5; and dye concentration, 25 mg L−1. The assays were performed in an orbital shaker, MNP-SDS concentrations varying from 0 to 50 mg L−1. After defining the optimal concentration of MNP-SDS (15 mg L−1), adsorption isothermal experiments were carried out to determine the maximum adsorptive capacity.
Isothermal and thermodynamic parameters
Adsorption isothermal experiments were performed using different MB concentrations (5–400 mg L−1), whereas the other variables were set at their optimal values. NP-SDS and MB were incubated for 32.2 min under constant stirring at 22.6 °C. At the end of the reaction time, the solution was exposed to a magnetic field for 15 min and the concentration of MB in the supernatant determined by UV-vis spectrophotometry (685 nm). The results were fitted with two different isothermal models, Langmuir and Freundlich (eqn (1) and (2), respectively), in order to identify adsorption behavior.In which q is the amount of solute adsorbed per gram of adsorbent at equilibrium (mg g−1), qmax is the maximum adsorption capacity (mg g−1), KL and KF are the Langmuir and Freundlich adsorption capacity constants (mg L−1), which reflect the interaction between adsorbate and adsorbent, Ce is the concentration of adsorbate at equilibrium (mg L−1), and 1/n is related to surface heterogeneity. Regarding thermodynamic parameters, the Gibbs free energy variation ΔG° was calculated as follows (eqn (3)).27 | ΔG° = −RT ln K | (3) |
In which R is the ideal gas constant (8.3144 J K−1 mol−1), T is the temperature in Kelvin, and K is the equilibrium constant.27
Results and discussion
Synthesis and characterization of nanoparticles
The magnetic iron oxide nanoparticles were synthesized through the chemical precipitation of Fe2+/Fe3+ ions in an alkaline medium and inert argon atmosphere. A black precipitate was observed at the end of the reaction, with magnetic properties becoming apparent upon the application of an external magnetic field. The UV/visible absorption spectrum for the MNPs is shown in Fig. 1A. A broader band can be observed in the ultraviolet region (300–400 nm), reflecting the surface Plasmon resonance of iron oxide NPs.28 Isolated particles had an absorption peak at 210 nm, which is typical of magnetite (Fe3O4).29
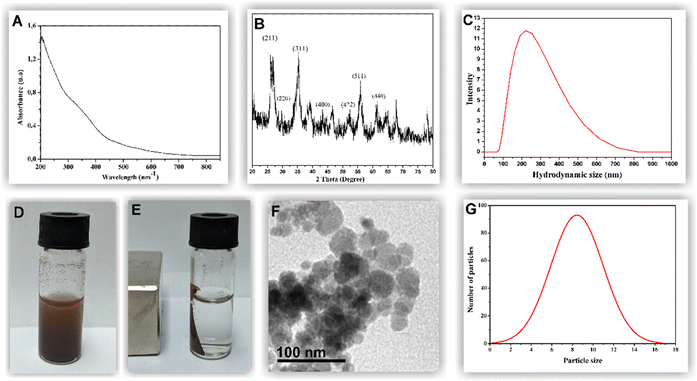 |
| Fig. 1 UV-vis spectrum (A) and XRD of Fe3O4 particles (B). Histogram obtained by DLS (C). Nanomagnetic supports suspended in the solution (D). Nanomagnetic supports attracted by a magnetic field (E). Image of Fe3O4 MNPs by TEM (F). Histogram of the size distribution of MNPs obtained by TEM. (G). | |
The analysis performed by DLS revealed an average hydrodynamic particle size of 200 nm, possibly due to the hydration layer adhered to the metallic surface (Fig. 1C). The zeta potential of −40 mV indicates high chemical stability, which is attributed to the OH groups adhered to the metallic surface. X-ray diffraction analysis determined the crystallite structure of the MNP-SDS (Fig. 1B). Five characteristic diffraction peaks were observed, (220), (311), (400), (440), (511), which correspond to the inverse spinel structure of the magnetite.30 Peaks at (113) and (210), corresponding to goethite and maghemite, were not detected, thus confirming the purity of the sample.31 The nanomagnetic showed excellent magnetic property (Fig. 1D and E). TEM images of the MNPs revealed the predominance of spherical nanoparticles, which was confirmed by an aspect ratio of 0.96 ± 0.30. On average, the nanoparticles analyzed measured 8.44 ± 2.54 nm in size (Fig. 1F and G).
The MNPs were functionalized with SDS according to the protocol described in the methods section. To evaluate the interaction of this surfactant with the metallic surface, functionalized MNPs were evaluated by FTIR and Raman spectroscopy. A wide band in the 3000–3500 cm−1 range was observed in the FTIR spectrum (Fig. 2A). This band is characteristic of O–H bonds, with resonance at 1952 cm−1, possibly due to the vibration of the oxygen atom.32 The bands observed in the 1000–1400 cm−1 range of the MNP-SDS spectrum are related to the SO2 and SO groups of sulfur compounds, which usually produce strong infrared bands in this region due to S
O stretching, thus indicating the success of the functionalization process.32–34 The bands around 2916 and 2847 cm−1, observed in the MNP-SDS spectrum, are assigned to C–H stretching vibrations, also confirming presence of SDS.35 The Raman scattering spectra (Fig. 2B) showed vibrations at 480 cm−1 that are related to Fe–O interactions.36 The 830 cm−1 band reflects CO–SO3 stretching vibrations. The peak at 896 cm−1 is attributed to the C–C single bond.37,38 The 995 cm−1 band is attributed to the stretching vibration of S–OC.39 The 1060 cm−1 and 1,081 cm−1 bands are assigned to the full-reflex vibration and a twist mode of the C–C bond, respectively, and both may be mixed with SO3 vibrations. The aforementioned results strongly suggest that SDS has adhered to the metallic surface of the nanoparticles.
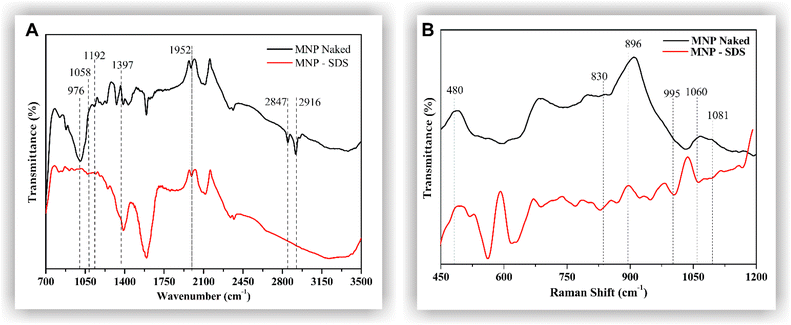 |
| Fig. 2 FTIR (A) and Raman (B) spectra of naked Fe3O4 NPs and nanoparticles functionalized with SDS (MNP-SDS). | |
Factorial design results
A thorough literature review was carried out to determine which variables and levels are most important for the removal of methylene blue with MNP-SDS (Tables S1 and S2 of the ESI†). As many variables can affect the removal process, we used the 2(5–1) fractional factorial design to select the most significant ones (p value < 0.05), considering removal percentage (R%) as response variable. As can be observed in the Pareto charts (Fig. 3), time and temperature were the most influent variables in the MB removal process, although time did not reach significancy. Therefore, these variables were selected for the optimization process in a full factorial design. We observed an increase in R% as a function of the decrease in temperature and increase in time.
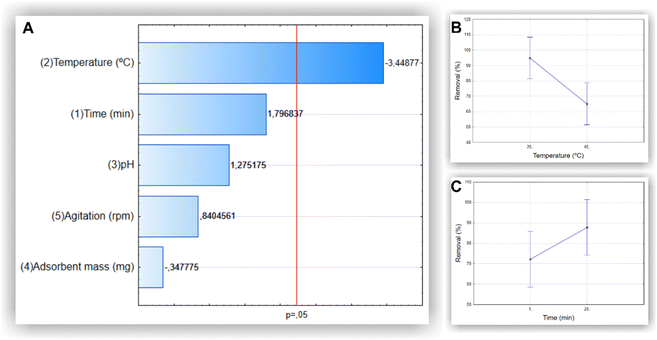 |
| Fig. 3 Pareto charts indicate the effect of variables in the MB removal process (%) (A). Bars that surpass the vertical line indicate that the parameters are significant (p < 0.05). Individual influences of the variables temperature (B) and time (C) on MB removal (%). | |
A complete experimental factorial design (32) with three levels and two factors (time and temperature) was used to optimize the experimental conditions for methylene blue removal. The analyses of the individual and combined effects of the variables evaluated are presented in the Pareto chart and surface response plot shown in Fig. 4A and B, respectively. The Pareto chart confirms that temperature was the most influential variable in the process. The surface response plot reveals the optimal interaction point between temperature and time, enabling the attainment of the optimal reaction conditions.
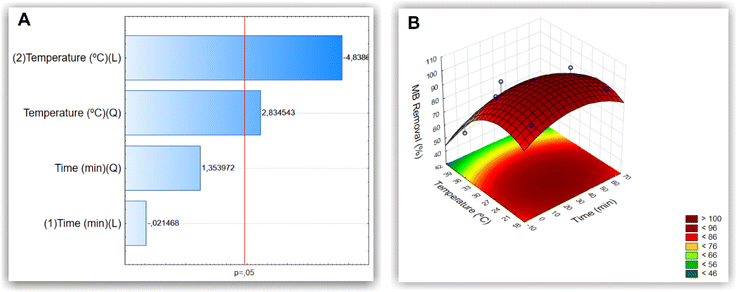 |
| Fig. 4 Pareto chart indicates the effect of the variables analyzed in the MB removal process (%) (A). Bars that surpass the vertical line indicate that the parameters are significant (p < 0.05). Surface response plot of MB removal (%) (B). | |
Characterization of MNP-SDS–methylene blue
The adsorption of methylene blue on the surface of the MNP-SDS was assessed through FTIR (Fig. 5A) and Raman (Fig. 5B) spectroscopy. The FTIR analysis shows the out-of-plane bending vibrations of the ring's C–H at 861 cm−1. A C–S–C vibration was detected at 1064 cm−1 and the –C–N stretching absorption peak at 1252 cm−1. Stretching vibrations of the –CH2 or –CH3 groups can be found from 1400 cm−1 to 1300 cm−1. A band that extends from 1400 to 1500 cm−1 corresponds to C
C side ring stretching. A vibration of the CH
N bond was identified at 1600 cm−1.40 The Raman spectrum (Fig. 5B) indicates that the characteristic peaks related to the MB C–H groups are observed at 770 cm−1 and 1154 cm−1.41 The band at 1625 cm−1 reflects the stretching of the C–C bonds of the MB's aromatic rings. The bands at 1398 cm−1 and 1470 cm−1 represent vibrations of N–C bonds and CH3 groups, respectively.42
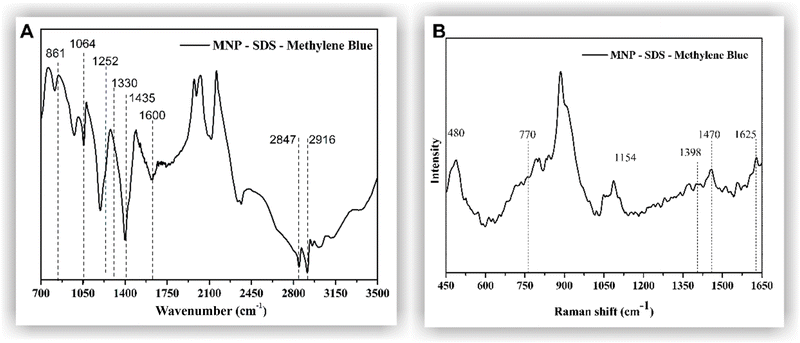 |
| Fig. 5 FTIR (A) and Raman (B) spectra of MNP-SDS–methylene blue. | |
Influence of adsorbent mass, isothermal, and thermodynamic parameters
Adsorbent mass is a crucial factor in MB removal. A judicious determination of the adsorbent quantity can prevent wastage by avoiding excessive usage without compromising its performance. The highest R% = 90% (qexp = 1.5 mg g−1) was obtained using 15 g L−1 of MNP-SDS (Fig. 6A). Higher adsorbent concentrations (≥30 g L−1) decreased removal efficiency, probably due to the agglomeration process, which can reduce available sites.43 Regarding removal capacity, the highest q value was obtained by applying 1 g L−1 of adsorbent (q = 19.75 mg g−1; R% = 79%). From then onwards, the q value dropped sharply, attributed to the increase in adsorbent mass; however, the R% parameter did not increase proportionally. Therefore, we opted to use the adsorbent mass that resulted in the highest R% value for both isothermal and thermodynamic experiments, that is, 15 g L−1 of MNP-SDS. The results obtained are deemed satisfactory when compared to existing literature. For instance, Yimin et al. utilized HA-Fe3O4 for MB removal and achieved a removal capacity of 0.291 mmol g−1.44 On the other hand, Zhang et al. achieved a higher value (qexp = 28 mg g−1) using humic acid-coated Fe3O4 nanoparticles, surpassing the removal capacity of the previously mentioned adsorbent.45 Nevertheless, it is important to consider other factors, such as cost and system structure, to ascertain the applicability of the adsorbent.
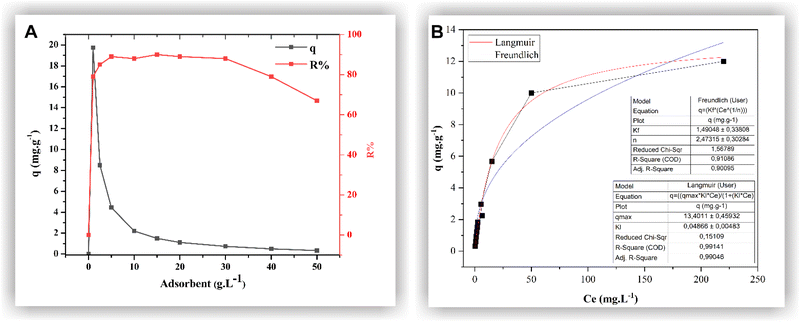 |
| Fig. 6 Influence of MNP-SDS mass in adsorption process (A); isothermal parameters of the adsorption (B). | |
The isothermal parameters of the adsorption are important not only to identify the affinity level between adsorbent/adsorbate but also to investigate adsorption equilibrium, i.e., when adsorption and desorption occur at the same rate. In Fig. 6B, a favorable isothermal shape is evident, indicating a high adsorbate mass retained per unit mass for a low equilibrium concentration of adsorbate in the liquid phase.46 The model that best fit the data was the Langmuir model, with R2 = 0.9914. This model assumes the existence of a defined range of available sites, equivalent energy, and monolayer adsorption, with dye molecules not interacting with each other. Thus, each available site admits only one molecule, unlike the Freundlich model, in which adsorption would take place in a heterogeneous surface and multilayers.46
The Gibbs free energy, calculated based on eqn (3), resulted in −5.45 kJ mol−1. The negative ΔG indicates that energy is being released in the process, which translates into a spontaneous, favorable reaction.46,47 Based on the results obtained here, we propose a possible adsorption mechanism between MNP-SDS and the methylene blue dye (Fig. 7).
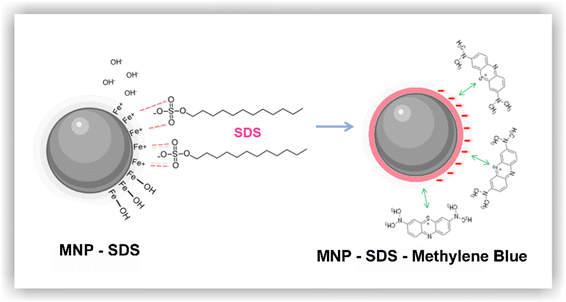 |
| Fig. 7 Adsorption mechanism between MNP-SDS and MB dye. | |
During the functionalization process, it is possible that the iron present in the nanoparticles released OH− into the medium due to a pH elevation induced by the addition of SDS. Subsequently, the positively charged iron present on the surface of the nanoparticles attracted sulphonate groups from SDS, leading to the formation of MNP-SDS nanoparticles. Although the nanoparticles retain a negative charge, functionalization usually reduces the agglomeration phenomenon, rendering binding sites available. Finally, the cationic dye may be attracted by the negative surface charge of MNP-SDS through a monolayer mechanism.
Conclusions
The presence of aquatic contaminants on a molecular scale has become increasingly common in recent years. Among them, dyes stand out by being of difficult retention within wastewater treatment plants. In this study, we investigated the adsorption efficiency of methylene blue in an aqueous solution using Fe3O4 nanoparticles functionalized with SDS. Promising results were obtained, as the adsorbent achieved an MB removal percentage of 90% (qexp = 1.5 mg g−1). Through factorial experiments we optimized the adsorption process and identified that temperature and time were the most influent variables in this system. Determining the ideal concentration of adsorbent was fundamental to avoid nanoparticle wastage, thus reducing the application costs. The adsorption reaction was spontaneous, favorable, and the data were best fitted to the Langmuir model, indicating adsorption occurs in monolayer. Therefore, we believe that the adsorbent developed here might play a satisfactory role in controlling aquatic pollution by MB.
Data availability
All data generated or analyzed during this study are included in this published article and its ESI.†
Author contributions
JNR and JPO conceived the project. TC and TAS performed the experiments, characterizations, and analysis. TC, ACB, JPO and JNR wrote the paper. STAC, AVFNR, MGP and MCCG revised the writing of the manuscript and data curation. JNR and JPO guided the research.
Conflicts of interest
The authors declare that they have no competing interests.
Acknowledgements
This study used the equipment facilities at the Laboratory of Cellular Ultrastructure Carlos Alberto Redins and LabPetro (UFES, Brazil). Our thanks to both Labs for providing the equipment and technical support for experiments.
References
- A. Badeenezhad, A. Azhdarpoor, S. Bahrami and S. Yousefinejad, Mol. Simul., 2019, 45, 564–571 CrossRef CAS.
- D. Yimin, Z. Jiaqi, L. Danyang, N. Lanli, Z. Liling, Z. Yi and Z. Xiaohong, Colloids Surf., A, 2018, 550, 90–98 CrossRef.
- M. A. Farghali, M. M. Abo-Aly and T. A. Salaheldin, Inorg. Chem. Commun., 2021, 126, 108487 CrossRef CAS.
- H. Hosseini, P. Pirahmadi, S. E. Shakeri, E. Khoshbakhti, S. Sharafkhani, V. Fakhri, A. Saeidi, D. J. McClements, W. H. Chen, C.-H. Su and V. Goodarzi, Int. J. Biol. Macromol., 2022, 201, 133–142 CrossRef CAS PubMed.
- S. Ihaddaden, D. Aberkane, A. Boukerroui and D. Robert, J. Water Proc. Eng., 2022, 49, 102952 CrossRef.
- A. K. H. al jibouri, J. Wu and S. R. Upreti, J. Water Proc. Eng., 2015, 8, 142–150 CrossRef.
- Z. Hu, C. Guo, P. Wang, R. Guo, X. Liu and Y. Tian, Chemosphere, 2022, 305, 135447 CrossRef CAS PubMed.
- G. Shi, C. Ruan, S. He, H. Pan, G. Chen, Y. Ma, H. Dai, X. Chen and X. Yang, Colloids Surf., A, 2021, 613, 126053 CrossRef CAS.
- Z. Lou, Z. Zhou, W. Zhang, X. Zhang, X. Hu, P. Liu and H. Zhang, J. Taiwan Inst. Chem. Eng., 2015, 49, 199–205 CrossRef CAS.
-
Y. Artioli, in Encyclopedia of Ecology, Five-Volume Set, Academic Press, 2008, vol. 1–5, pp. 60–65 Search PubMed.
- X. Peng, Sep. Purif. Technol., 2024, 350, 127943 CrossRef CAS.
-
L. A. Camacho-Cruz, M. A. Velazco-Medel, A. Cruz-Gómez, G. Cedillo-Valverde and E. Bucio, in Magnetic Nanoparticles and Polymer Nanocomposites, ed. I. Khan, M. M. A. Khan and A. Khan, Woodhead Publishing, 2024, pp. 441–458 Search PubMed.
- M. Wang and X. You, Proc. Combust. Inst., 2023, 39, 1157–1164 CrossRef.
- A. S. Alsaman, H. Maher, M. Ghazy, E. S. Ali, A. A. Askalany and B. Baran Saha, Therm. Sci. Eng. Prog., 2024, 49, 102455 CrossRef CAS.
- S. Lavanya, T. R. Kumar, A. V. Juliet, J. Hakami, I. M. Ashraf and M. Shkir, Solid State Sci., 2022, 128, 106889 CrossRef CAS.
- G. Jia, J. Guo, Y. Guo, F. Yang and Z. Ma, Case Stud. Constr. Mater., 2024, 20, e03171 Search PubMed.
- A. C. De Lima Barizão, M. F. Silva, M. Andrade, F. C. Brito, R. G. Gomes and R. Bergamasco, J. Environ. Chem. Eng., 2020, 8, 103618 CrossRef.
- L. Yan, X. Song, J. Miao, Y. Ma, T. Zhao and M. Yin, J. Water Proc. Eng., 2024, 60, 105215 CrossRef.
- M. G. El-Desouky, A. A. A. Alayyafi, G. A. A. M. Al-Hazmi and A. A. El-Bindary, J. Mol. Liq., 2024, 399, 124392 CrossRef CAS.
- M. Alsawat, Int. J. Electrochem. Sci., 2024, 19, 100611 CrossRef CAS.
- P. Zhang, I. Lo, D. O'Connor, S. Pehkonen, H. Cheng and D. Hou, J. Colloid Interface Sci., 2017, 508, 39–48 CrossRef CAS PubMed.
- Y. Y. Xu, M. Zhou, H. J. Geng, J. J. Hao, Q. Q. Ou, S. da Qi, H. L. Chen and X. G. Chen, Appl. Surf. Sci., 2012, 258, 3897–3902 CrossRef CAS.
- P. Damberg, J. Jarvet and A. J. Gräslund, Methods Enzymol., 2001, 339, 271–285 CAS.
- S. Shariati, M. Faraji, Y. Yamini and A. A. Rajabi, Desalination, 2011, 270, 160–165 CrossRef CAS.
- S. A. Kordkandi and M. Forouzesh, J. Taiwan Inst. Chem. Eng., 2014, 45, 2597–2604 CrossRef CAS.
- C. R. T. Tarley, G. Silveira, W. N. L. dos Santos, G. D. Matos, E. G. P. da Silva, M. A. Bezerra, M. Miró and S. L. C. Ferreira, Microchem. J., 2009, 92, 58–67 CrossRef CAS.
- C. Silveira, Q. L. Shimabuku, M. Fernandes Silva and R. Bergamasco, Environ. Technol., 2018, 39, 2926–2936 CrossRef CAS PubMed.
- T. T. Nguyen, F. Mammeri and S. Ammar, Nanomaterials, 2018, 8, 149 CrossRef PubMed.
- A. Bouafia, S. E. Laouini, A. Khelef, M. L. Tedjani and F. Guemari, J. Cluster Sci., 2021, 32, 1033–1041 CrossRef CAS.
- L. A. Cobos Cruz, C. A. Martínez Perez, A. M. Villafañe, J. A. Matutes Aquino, J. R. Farias Macilla and P. E. G. Casillas, Influence of Cyclodextrin in the Synthesis of Magnetite, MRS Online Proc. Libr., , 2009, 1243, 11 Search PubMed.
- M. Masuku, L. Ouma and A. Pholosi, Environ. Nanotechnol., Monit. Manage., 2021, 15, 100429 CAS.
-
B. Stuart, Infrared Spectroscopy Fundamentals and Applications, John Wiley & Sons, 2004, vol. 1 Search PubMed.
- J. Lin, F. Meng, Y. Zhong, J. Liu, G. Chen, Y. Wan, K. Qian and M. T. Sitaramanjaneya, J. Mater. Res., 2010, 25, 1992–2000 CrossRef.
- D. Sarahia Guzmán-Hernández, M. Teresa Ramirez-Silva, M. Alberto Romero-Romo, M. A. E Hafizah, A. F. Riyadi and A. Manaf, Mater. Sci. Eng., 2019, 515, 012080 Search PubMed.
- V. A. J. Silva, P. L. Andrade, M. P. C. Silva, A. D. Bustamante, L. de Los Santos Valladares and J. Albino Aguiar, J. Magn. Magn. Mater., 2013, 343, 138–143 CrossRef CAS.
- L. Slavov, M. V. Abrashev, T. Merodiiska, C. Gelev, R. E. Vandenberghe, I. Markova-Deneva and I. Nedkov, J. Magn. Magn. Mater., 2010, 322, 1904–1911 CrossRef CAS.
- D. Han, B. Li, Y. Chen, T. Wu, Y. Kou, X. Xue, L. Chen, Y. Liu and Q. Duan, Nanotechnology, 2019, 30, 465703 CrossRef CAS PubMed.
- E. Onoja, S. Chandren, F. I. A. Razak and R. A. Wahab, J. Biotechnol., 2018, 283, 81–96 CrossRef CAS PubMed.
- B. Hao, K. Wang, Y. Zhou, C. Sui, L. Wang, R. Bai and Z. Yang, ACS Omega, 2020, 5, 1109–1119 CrossRef CAS PubMed.
- A. A. Alshehri and M. A. Malik, J. Mater. Sci.: Mater. Electron., 2019, 30, 16156–16173 CrossRef CAS.
- R. R. Naujok, R. V. Duevel and R. M. Corn, Langmuir, 1993, 9, 1771–1774 CrossRef CAS.
- M. R. Shattique and M. Stepanova, Plasmonics, 2020, 15, 427–434 CrossRef CAS.
- T. Wu, G. Yang, J. Cao, Z. Xu and X. Jiang, Chem. Eng. Res. Des., 2022, 188, 330–341 CrossRef CAS.
- D. Yimin, Z. Jiaqi, L. Danyang, N. Lanli, Z. Liling, Z. Yi and Z. Xiaohong, Colloids Surf., A, 2018, 550, 90–98 CrossRef.
- X. Zhang, P. Zhang, Z. Wu, L. Zhang, G. Zeng and C. Zhou, Colloids Surf., A, 2013, 435, 85–90 CrossRef CAS.
-
R. F. Nascimento, A. C. A. Lima, C. B. Vidal, D. Q. Melo and G. S. C. Raulino, Adsorption: Theoric aspects and environmental applications, UFC Imprensa Universitária, 2014, vol. 1, p. 256 Search PubMed.
-
C. J. Cleveland and C. Morris, Dictionary of Energy, 2014, pp. 1–680 Search PubMed.
|
This journal is © The Royal Society of Chemistry 2024 |