DOI:
10.1039/D4NH00010B
(Communication)
Nanoscale Horiz., 2024,
9, 1543-1556
Biomimetic laser-induced graphene fern leaf and enzymatic biosensor for pesticide spray collection and monitoring†
Received
7th January 2024
, Accepted 13th June 2024
First published on 19th June 2024
Abstract
Monitoring of pesticide concentration distribution across farm fields is crucial to ensure precise and efficient application while preventing overuse or untreated areas. Inspired by nature's wettability patterns, we developed a biomimetic fern leaf pesticide collection patch using laser-induced graphene (LIG) alongside an external electrochemical LIG biosensor. This “collect-and-sense” system allows for rapid pesticide spray monitoring in the farm field. The LIG is synthesized and patterned on polyimide through a high-throughput gantry-based CO2 laser process, making it amenable to scalable manufacturing. The resulting LIG-based leaf exhibits a remarkable water collection capacity, harvesting spray mist/fog at a rate approximately 11 times greater than a natural ostrich fern leaf when the collection is normalized to surface area. The developed three-electrode LIG pesticide biosensor, featuring a working electrode functionalized with electrodeposited platinum nanoparticles (PtNPs) and the enzyme glycine oxidase, displayed a linear range of 10–260 μM, a detection limit of 1.15 μM, and a sensitivity of 5.64 nA μM−1 for the widely used herbicide glyphosate. Also, a portable potentiostat with a user-friendly interface was developed for remote operation, achieving an accuracy of up to 97%, when compared to a standard commercial benchtop potentiostat. The LIG “collect-and-sense” system can consistently collect and monitor glyphosate spray after 24–48 hours of spraying, a time that corresponds to the restricted-entry interval required to enter most farm fields after pesticide spraying. Hence, this innovative “collect-and-sense” system not only advances precision agriculture by enabling monitoring and mapping of pesticide distribution but also holds the potential to significantly reduce environmental impact, enhance crop management practices, and contribute to the sustainable and efficient use of agrochemicals in modern agriculture.
New concepts
This manuscript addresses the pressing need for monitoring of pesticide spray in farm fields, crucial for enabling precise and efficient application while preventing overuse or untreated areas. We introduce the innovative concept of using a biomimetic “collect-and-sense” system to collect, channel, and quantify pesticide spray. The “collect-and-sense” system is constructed from hydrophilic and porous laser induced graphene (LIG) which is produced through a scalable, high-throughput gantry-based laser etching process that eliminates the need for ink formulation, printing, and post-print annealing typically associated with printed graphene systems. The collection patch of the system emulates the structure of an ostrich fern leaf, featuring eight symmetric LIG leaf pinnules that gradually broaden towards a central stem. This design facilitates the transport of pesticide residues to a singular point through the collection of spray mist and exhibits a remarkable 11-fold increase in fluid transport to a natural fern leaf. This improvement is primarily attributed to the distinctive porosity and wettability properties of LIG. Moreover, a LIG biosensor, enhanced with platinum nanoparticles and glycine oxidase, in conjunction with a developed portable potentiostat, accurately quantifies glyphosate pesticides collected from the LIG fern leaf at micromolar concentrations. Hence this LIG-based “collect-and-sense” system surpasses passive pesticide sampling and colorimetric test strip sensors that provide only average or qualitative measurements.
|
1. Introduction
The United Nations (UN) projects that a 60% increase in food production will be needed to meet the needs of an estimated world population of over 9 billion people by 2050.1 Pesticides are an integral solution to increase food production for our growing population, and without them, there would be an immediate 78% loss of fruit production, a 54% loss of vegetable production, and a 32% loss of cereal production.2 However, 64% of global agricultural land (approximately 24.5 million km2) is at risk of pesticide pollution by more than one active ingredient, and 31% is at high risk.3 Therefore, there is an urgent need for precision agriculture to help reduce the use of pesticides while maintaining crop yields. Such desperately needed pesticide reduction is challenging as reducing the number of spray applications during the growing season could reduce infestation control and reduce crop yield, while restrictive or excessive use can foster genetic resistance in the weeds, pathogens, or insects that are being treated.4 Therefore, the most viable path forward to reducing pesticide use on farmland is to apply them only when and where they are needed or, in other words, to use precision application or site-specific spraying.4 This can be accomplished by improved monitoring and decision-making through smart farming practices where, for example, the strategic placement of sensors throughout the farm field would allow farmers to gain a comprehensive understanding of the effectiveness of pesticide applications so that future applications can be tailored accordingly.5
Mapping pesticide concentrations from spray applications in a farm field presents a significant challenge due to the cost and current detection complexity. Typically, environmental pesticide residue from the air and water are monitored through passive techniques. Passive sampling devices use sorbent materials, such as activated carbon, polyurethane foam, polyethylene sheets, or polydimethylsiloxane to adsorb pesticides from the air or water over weeks or even months i.e., a time-integrated measurement.6–8 These sorbent materials are then collected and analyzed with traditional laboratory techniques (e.g., liquid chromatography–mass spectrometry (LC–MS)) to obtain an average pesticide concentration for that collection site. Hence, these passive sampling techniques do not provide real-time pesticide concentration measurements and require the user to ship samples to a laboratory for costly analysis. Consequently, such devices are not widely employed by farmers to map pesticide concentrations.
Electrochemical sensors could circumvent the drawbacks associated with passive sampling and testing by providing low-cost, real-time monitoring of pesticide concentration in solution, even in turbid fluid samples where optical sensors can fail.9 Indeed, electrochemical pesticide biosensors represent a rapidly growing field of research with recent reports demonstrating the ability to monitor a wide variety of insecticides, such as organophosphates and neonicotinoids, as well as herbicides, such as glyphosate and atrazine.10–17 The majority of these electrochemical pesticide sensors are based on test strips where a sample droplet is pipetted onto the sensor, or the sensor itself is briefly submerged in the sample to make quantifiable readings, much like the glucose test strips that are used to analyze blood glucose levels in diabetic patients.18–21 In other cases, these electrochemical sensors are integrated with a microfluidic device to monitor pesticides from a small, collected fluid sample.11,17,22–25 However, there are few reports of in-field “collect-and-sense” devices that are capable of both collecting and quantifying pesticide spray in the farm field.26 To create such a device, one can turn to nature for inspiration.
Nature has provided some of the most efficient patterned surfaces for collecting water droplets and harvesting fog or spray mist including the back of the Namib desert beetle, the wing of a butterfly, the spine of a cactus, and the leaf of a fern.27,28 These biological surfaces generally contain hydrophilic (water-attracting) regions that when properly arranged or surrounded by hydrophobic (water-repelling) regions, can collect and transport water to specific locations. The fern leaf with multiple individual petals or pinnules is one of the largest natural water droplet and fog harvesting surfaces as the size and shape of fern leaves, especially that of the ostrich fern leaf, provide a large surface area for water droplets to condense and collect.29 Such fern leaves provide a reticulate or net-like venation shape that gradually decreases in size as they approach the dripping point or apex of the leaf where water droplets collect and drip near the base of the plant for hydration.29–31 In essence, the fern leaf has a remarkable ability to harvest fog/spray mist and channel water rapidly and efficiently across its surface without the need for a pump, due to its integrated system of multiscale channels and surface microstructures.29
The ability to create large, high-surface area structures for pumpless fluid transport to a sensor can be challenging. Researchers have recently shown that open microfluidic devices can transport fluids across channels using Laplace pressure-driven flow, via spatial gradients of microchannel wettability, similar to how water is transported across natural surfaces like butterfly wings, cactus spines, beetle backs, and fern leaves.32–34 However, the patterned surfaces used to create the open microfluidics are often created with expensive cleanroom fabrication techniques (e.g., photolithography with UV masks, reactive ion etching).33,35,36 Moreover, these techniques are focused on solely developing the open microfluidics and hence a different set of potentially costly materials such as thin films of platinum, gold, or graphene grown via chemical vapor deposition is often needed to produce a connecting electrode that could be used to electrochemically sense target molecules within the transported fluid. Of course paper microfluidics, like open microfluidics, can channel and split fluid samples without a pump in a cost effective manner for myriad sensor applications.37–42 In fact, a recent study demonstrated that an origami paper microfluidic could channel pesticide spray through a pre-loaded enzyme, for subsequent sensing with a screen printed carbon electrode.26 Hence, paper microfluidics could be suitable for collecting pesticide spray, however, paper microfluidics are also sensitive to environmental conditions like humidity, temperature fluctuations, and exposure to sunlight, which could adversely effect their stability and performance during a long period of exposure on a farm field. Moreover, paper microfluidics need to be interfaced with a separate conductive electrode for electrochemical sensing. However, laser-induced graphene (LIG) has emerged as a promising material for both open microfluidic development through surface wettability patterning and conductive sensing components due to its porous nature, high surface area, high throughput manufacturing, and to the fact that graphene is more stable than paper.17,43 As such, LIG offers scalability for high-throughput manufacturing of sensor and open microfluidic systems, making it potentially conducive to wide-scale field deployment for mapping pesticide residues in crop fields.
Herein, we introduce the concept of using a high surface area fern design, comprised of hydrophilic LIG, to not only collect sprayed pesticide residues, but transport the collected pesticides to a singular point for further subsequent quantification with an amperomtric LIG-based biosensor. The “collect-and-sense” device utilizes LIG to create both the fern leaf and corresponding biosensor. The LIG is synthesized and patterned all in one step using a gantry-based CO2 laser. This LIG manufacturing process is amenable to scalable manufacturing and circumvents the need for energy intensive and low-yield graphene synthesis techniques including chemical vapor deposition,44–47 microwave synthesis48,49 and nanotube unzipping.50,51 LIG also bypasses the need to create printed graphene devices that generally require mechanical exfoliation52,53 or chemical exfoliation54,55 of graphite to produce graphene flakes, energy intense sonication/mixing to breakdown the flakes into micro/nanoscale sizes, mixing of graphene with binders (e.g., ethyl or nitrocellulose) and often toxic solvents (e.g., cyclohexane), printing of inks through costly equipment (inkjet, aerosol, screen, or gravure printers), and performing energy intensive post-print annealing (high-temperature oven annealing, photonic annealing, or rapid pulse laser annealing) to carbonize ink binders and produce a printed graphene surface sufficiently conductive for sensing applications.56–60 The LIG developed herein is made hydrophilic and porous by tuning the settings of the CO2 laser to minimize micropatterning which can make the LIG hydrophobic or near superhydrophobic in some cases.17,61 The LIG electrochemical biosensor is functionalized with electrodeposited PtNPs and the enzyme glycine oxidase to selectively monitor the herbicide glyphosate collected from the LIG leaf. The sensors were calibrated with both a glyphosate salt from Sigma Aldrich (SA; glyphosate solution) and ready-to-spray glyphosate solution from Corteva Agricscience (CA; glyphosate solution) to understand the performance of the sensors to differently processed glyphosate solutions. Glyphosate is the most widely used herbicide in the United States and across the globe, making it a highly relevant pesticide for testing the developed “collect-and-sense” system.62 Finally, to create a completely stand-alone sensing platform, a portable potentiostat capable of running user defined amperometric measurements, that we call an amperometric measurement instrument (AMI), was developed to aquire signal responses from the LIG-based biosensors in the field. Glyphosate solutions were sprayed onto the LIG collection device to confirm its ability to transport glyphosate residues collected and to quantify them using the LIG enzymatic biosensor. Hence this low-cost, LIG-based “collect-and-sense” platform and developed AMI could be deployed on a large scale to monitor glyphosate and other pesticides deployed by agriculture workers.
2. Results and discussion
2.1 Fabrication of LIG fern leaf and biosensor
Both the biomimetic fern leaf and electrochemical biosensor were comprised of LIG (Fig. 1 and Experimental section). LIG was synthesized from polyimide by using a CO2 laser with laser settings of 15% speed, 7% power, and 1200 dots per inch (DPI) to create the hydrophilic LIG leaf and the 3 electrodes associated with the electrochemical biosensor. As we have demonstrated previously, such a high laser DPI setting leads to enhanced LIG homogeneity, decreased electrical resistivity, and lower water contact angles (i.e., higher hydrophilicity).17 The LIG leaf was geometrically inspired by the structure of the ostrich fern leaf which contains numerous hydrophilic microchannels that extend along each leaf segment or pinnule and converge toward an apex, facilitating the efficient collection and transport of fluid to a single point (as shown in Fig. 1(A) and (B)). This includes eight symmetric LIG leaf pinnules that converge into a central channel or stem to facilitate the delivery of fluid to a singular point to be collected (Fig. 1(E)). This completes the LIG leaf fabrication. PtNPs were then electrodeposited onto the working 3 mm electrode of the LIG sensor through a 30 second step function pulsed at −0.5 V versus a commercial Ag/AgCl electrode in a platinum plating solution to enhance the electroactive nature of the LIG towards hydrogen peroxide oxidation, the byproduct of the enzyme (glycine oxidase) used in this work (Fig. 1(C)). Silver (Ag) paste was applied to the reference electrode and heated/dried for 10 minutes at 100 °C to create a Ag reference electrode63 while the counter electrode was left as bare LIG.16 A mixture of the enzyme glycine oxidase (190 μM in 10× PBS pH 7.4), cofactor flavin adenine dinucleotide (FAD, 2 nM with pH of 8.4), and crosslinking agent glutaraldehyde (0.25%) was next drop coated onto the working electrode to create the glyphosate biosensor (Fig. 1(D)). Finally, an acrylic polish was applied across the stems of the three-electrodes (non-active area used for electrical connection to the potentiostat) to passivate this area so it would not electrochemically interact during experiments.
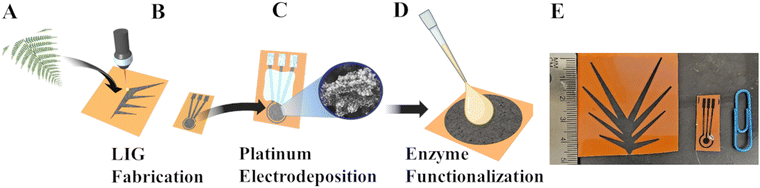 |
| Fig. 1 Fabrication and functionalization schematic of the LIG leaf and electrochemical biosensor. Schematic images demonstrate the (A) fern leaf inspiration, (B) polyimide conversion to LIG using a CO2 laser to create both the fern leaf pesticide collection patch and the 3-electrode electrochemical biosensor, (C) surface modifications including the electrodeposition of PtNPs onto the LIG working electrode, (D) functionalization of the LIG sensor with the enzyme glycine oxidase for selective glyphosate monitoring, (E) and an optical image of the completed LIG leaf and biosensor. Both a metric scale ruler and a standard no. 1-sized paperclip serve as measurement legends in (E). | |
2.2 Material and electrochemical characterization of the LIG fern leaf and biosensor
The material properties of the developed LIG were further elucidated with water contact angle, scanning electron microscopy (SEM), and Raman spectroscopy measurements (Fig. 2). Static water contact angle measurements revealed that the LIG was extremely hydrophilic (29.8°), in fact more hydrophilic than an actual ostrich fern leaf (63.7°) and much more hydrophilic than the surrounding hydrophobic non-lased polyimide (99.5°) (Fig. 2(A)). As will be explored in subsequent sections, these hydrophilic LIG tracks surrounded by hydrophobic polyimide (Fig. 2(B)) will enable Laplace pressure driven flow generally associated with open microfluidics. SEM micrographs of the bare LIG, non-lased polyimide and LIG interface, and LIG functionalized with PtNPs were also obtained (Fig. 2(B), (C), (E) and (F)). The bare LIG exhibited microscopic pores ranging from approximately 10–20 μm across in diameter (Fig. 2(B) and (C)), while the PtNPs electrodeposited onto the LIG exhibited a size range from 30–45 nm within larger Pt clusters approximately 5–6 μm in size (Fig. 2(E) and (F)).
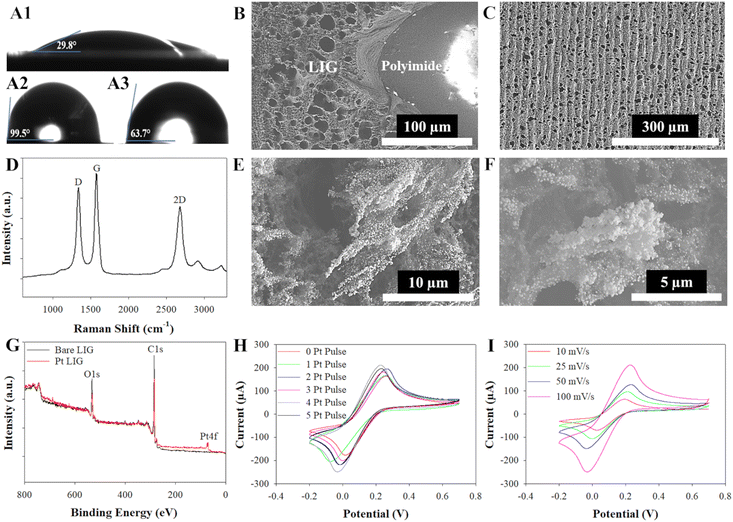 |
| Fig. 2 Material characterization of the fabricated LIG and LIG functionalized with PtNPs utilized in the leaf-shaped collection patch and connected biosensor. (A) Water contact angle images showing the static contact angles of water for the (A1) LIG, (A2) polyimide, and (A3) ostrich fern leaf. Scanning electron microscopy (SEM) micrographs displaying the (B) LIG and polyimide interface (C) overall LIG microstructure. (D) Raman spectrum of the developed LIG. Raman spectrum were obtained from 5 LIG electrodes. SEM micrographs of (E) platinum nanoparticles deposited on the LIG surface and (F) a close-up view of a platinum nanocluster on the LIG. (G) X-ray Photoelectron Spectroscopy (XPS) of the bare LIG and LIG with PtNPs and cyclic voltammetry (CV) in 5 mM ferri/ferrocyanide with 0.1 M KCl solution for (H) LIG subjected to varying platinum pulse electrodeposition treatments and for (I) LIG subjected to the 4-pulse platinum electrodeposition treatment and acquired at different scan rates. | |
Raman spectroscopy and X-ray photoelectron spectroscopy (XPS) were next employed to comprehensively characterize the LIG, allowing for the assessment of its structural properties, elemental composition, and chemical bonding configurations. The three characteristic bands of graphitic material-D band (∼1342 cm−1), G band (∼1578 cm−1), and 2D band (∼2686 cm−1) – were noted in the Raman spectra (Fig. 2(D)).64 The D band indicates defects in the graphitic layers,65 the G band suggests the presence of sp2-carbon atoms in graphene,66 and the 2D band indicates the presence of few-layer graphene.67 Then, the Lorentzian function68 was used to determine the band's intensity, location, and full width at half maximum. The average ID/IG band calculated from the data, 0.77 ± 0.07, suggests a high degree of disorder present in the graphene sample. We can infer from the average I2D/IG band calculated, 0.68 ± 0.04, that the sample contains multi-layer graphene.69 XPS analysis confirmed a strong composition of carbon (C1s), oxygen (O1s), and Pt in the samples (Fig. 2(G)). The C1s peaks were strong, around 284.4 eV, while the O1s peaks were strong, around 531.7 eV, in samples with and without PtNPs. In samples where PtNPs were present, a prominent peak at approximately 70.1 eV in the Pt 4f region was observed, providing confirmation of the successful electrodeposition of PtNPs onto the LIG surface, consistent with findings in prior studies.70
Finally, cyclic voltammetry in 5 mM ferri/ferrocyanide prepared in 0.1 M KCl was used to compare the electroactive nature of the bare LIG and LIG electrodes created with increasing amounts of Pt nanoparticles (1–5 pulses, see Experimental section) (Fig. 2(H)). The peak currents increased as the number of platinum pulses increased at 100 mV s−1, which correlated to an increase in the surface area available to engage with the redox probe. An increase in electroactive surface area (ESA) is confirmed from calculations in Table S1 (ESI†). However, during the fifth pulse, the peak current was observed to remain consistent with that of the 4-pulse platinum electrode, as confirmed through averaging data from n = 3 sensors. This phenomenon can be attributed to the oversaturation of PtNPs on the LIG, leading to a change from nanostructured to microstructured platinum, which, in turn, diminishes the electroactive nature of the platinum as previously demonstrated with PtNP-graphene electrochemical glucose biosensors.71 The 4-pulse platinum process was selected for further analysis of its electrochemical properties and used for the characterization of the LIG sensor. It is important to note that the peak-to-peak separation (ΔEp) increased as shown by the drift in peak currents at each scan rate as the number of electrodeposition pulses increased. This behavior is typically seen with an electrochemically quasi-reversible system.72,73 The Pt-coated LIG and electrochemical properties were analyzed using the Randle's-Sevcik equation for a quasi-reversible system as well as performing X-ray photoelectron spectroscopy to validate the deposition of PtNPs onto the LIG surface. The Randles–Sevcik equation describes the current intensity (i) in an electrochemical cell at the peak location (Ep) for a redox species, particularly in the context of cyclic voltammetry (eqn (1)). The ESA of the LIG working electrode or (A) in the Randles–Sevcik equation can therefore be calculated by monitoring the peak current (ip) of a redox equation during cyclic voltammetry and noting the scan rate (ν), redox probe diffusion coefficient (D), number of electrons transferred in the redox probe (n), the bulk concentration of the redox species (C), Faraday constant (F), universal gas constant (R), and temperature in Kelvin (T).74
| 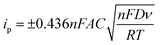 | (1) |
In this study, the ESA was calculated using a scan rate of 100 mV s−1 for a 4-pulsed platinum electrode. The ESA for bare LIG and 4-pulse Pt-LIG was calculated to be 0.136 cm2 and 0.176 cm2, respectively; see ESI† for values, calculations, and Randles–Sevick plot (Table S1 and Fig. S1). The planar geometric surface area for an electrode with a diameter of 3 mm is only 0.071 cm2; therefore, the creation of LIG and Pt-LIG electrodes boosts this surface area by approximately 192% and 248%, respectively. When the geometric surface area is smaller than that of the ESA, the redox probe solution has significant accessibility to the edge sites of the material, in this case the porous, 3D nature of LIG and PtNP structure.75
2.3 Fluid transport properties of LIG fern leaf
2.3.1 Geometric refinement of LIG fern leaf pinnules – wedge angle comparison.
The geometry of the LIG fern leaf pinnules that flow into a central track (stem) was first analyzed and refined to improve the transport of fog/mist to the connected biosensor (Fig. 1(E)). The LIG fern leaf pinnules are made of wedge tracks that increase in size as they reach the central track. This wedge design of the hydrophilic LIG track surrounded by hydrophobic non-lased polyimide was inspired by recent research that develops open microfluidics for high rate, pumpless fluid transport via wettability patterning.33,34 A variety of 30 mm wedge tracks created with varying angles (i.e., 3°, 5°, 7°, and 10°) were tested to verify the optimal wedge angle for fluid transport (Fig. S2, ESI†). Fig. 3 and Movie 1 (ESI†) demonstrate how a singular water droplet of 5 μL, mixed with fluorescent dye for visualization, deposited at the narrow end of the 7° wedge track flows across the surface. Once the droplet encountered the LIG surface, the fluid quickly traversed from the narrow end of the wedge track (0.5 mm width) to the wide portion (2 mm width). Interestingly, the fluid moved rapidly across the first half of the wedge channel within 0.3 s while substantially slowing in the 2nd half, reaching the end of the track by 6.97 s (Table 1). Similar results were obtained with the other wedge angle tracks (with wedge angles of 3°, 5°, and 10°) where fluid flow was substantially more rapid in the first half of the track than the second half. During the first half of the wedge, we assume the fluid follows rapid transport associated with Laplace Pressure driven flow which is also associated with wedge channel open microfluidics, while in the latter half of the LIG, the fluid follows slower capillary wicking driven flow, often associated with paper microfluidics, as the initial water droplet bulge and fluid velocity is completely diminished.39,65,75,76 Such a dual flow regime is most likely caused by the unique high porous structure of LIG which can clearly permit wicking flow in addition to Laplace driven flow depending upon the geometry of the channels. The wedge angle of 7° was chosen for the pinnule wedge angles of the LIG fern leaf as it provided the most consistent fluid flow velocities (lowest standard deviation), although narrower wedge tracks appeared to transport fluid more rapidly (Table 1).
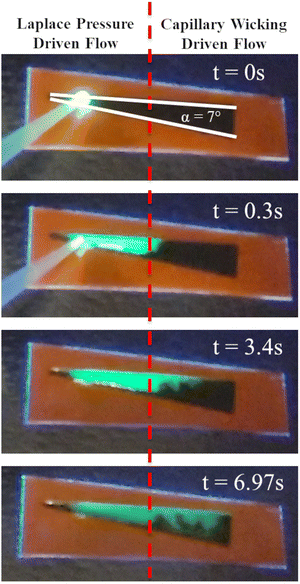 |
| Fig. 3 Time stamp images of fluid flow experiment to compare Laplace pressure driven flow (first half of the wedge track to the left of the red dotted line) and capillary wicking driven flow (second half of the wedge track to the right of the red dotted line) on hydrophilic LIG using a 7° wedge track. The length of each track is 30 mm. A green, fluorescent dye was added to the water to visualize the fluid flow. | |
Table 1 Time and velocity comparison of fluid flow within the LIG wedge tracks created with varying wedge angles
Flow regime |
Laplace pressure driven flow (first half of wedge track) |
Capillary wicking driven flow (second half of wedge track) |
Wedge angle |
t
half [s] |
V
half [mm s−1] |
t
full [s] |
V
full [mm s−1] |
Note: t – time, V – velocity. |
3° |
0.23 ± 0.06 |
77.00 ± 7.92 |
2.76 ± 0.86 |
5.97 ± 1.60 |
5° |
0.28 ± 0.06 |
52.43 ± 4.13 |
2.84 ± 0.73 |
5.43 ± 0.91 |
7° |
0.30 ± 0.05 |
44.62 ± 1.33 |
6.97 ± 0.87 |
2.19 ± 0.13 |
10° |
0.38 ± 0.08 |
35.00 ± 2.33 |
9.97 ± 0.20 |
1.50 ± 0.26 |
2.3.2 Fluid flow along an incline.
Next, the impact of gravitational forces on fluid flow across the LIG leaf fern was analyzed to better provide insights relevant to applications such as fog harvesting and surface flow dynamics. For these experiments, the LIG wedge track created with a 7° wedge angle was chosen due to its consistent flow results as shown in Table 1. Fig. S3–S5 (ESI†) shows images from an aerial view of fluid flowing up the 7° wedge track at an incline of 3°, 5°, and 7° where a 10 mm by 10 mm square hydrophilic reservoir would collect any water that reached the top of the incline. As the droplets increased from 1 to 30, each 5 μL in volume, the fluid tended to congregate at the bottom of the incline at the narrow end of the wedge track. Approximately 10 μL of the total volume (150 μL) was propelled to the reservoir at the top of the inclined track. Angles above and below the final 5° incline (3° and 7°) were also tested with the 7° LIG wedge. Both the 3° and 7° incline showed fluid reaching the square reservoir like the 5° wedge after the first 5 μL droplet touched the LIG surface. However, the reservoir only became wetted (less than 3 μL fluid), and the majority of the fluid congregated at the bottom of the wedge track whereas about 10 μL was able to be propelled to the reservoir with the 5° incline. Previously in our group, we conducted similar studies creating hydrophilic wedge tracks surrounded by hydrophobic walls using spin coated graphene ink.34 This spin coated graphene was treated with oxygen plasma to create the hydrophilic tracks and further laser patterned to create the hydrophobic walls. For this material with an identical wedge design, it was found that the maximum incline that water fills the top patterned reservoir was 7°. Another similar example study was conducted with a hydrophobic substrate made from polyethylene terephthalate (PET), titanium dioxide NPs, and ethanol. Super hydrophilic tracks were created onto the hydrophobic surface by using a photomask under UV treatment.33 It was found that the maximum incline angle for water transport on this incline was approximately 13°. In summary, these experiments shed light on the influence of gravitational forces on fluid flow across the LIG fern leaf, offering valuable insights applicable to spray mist harvesting and surface flow dynamics.
2.3.3 Water collection experiments.
Fog or spray mist is a utilized source of water supply for many plants as it can help reduce water loss where the plants are able to directly absorb the condensed water droplets on their leaves, stems, or nearby roots.76 Numerous references in the literature utilize various fern species, including the ostrich fern leaf, as collection substrates for condensed water droplets from fog or mist.29,76,77 This choice is attributed to their extensive surface area and wettability patterns, which facilitate the channeling of collected water from fog or mist to a central point on the plant, enabling subsequent plant hydration. Hence, the LIG-based pesticide spray collection patch was geometrically patterned after an ostrich fern leaf to assist in collection of pesticide spray that would later desorb and transport to a single biosensor after a fog or mist event (Fig. 1). This allows for the collection patch to be readily rinsed with a buffered solution sprayed onto it, enabling rapid quantification of the adsorbed pesticide concentration at the edge of the farm field. To better understand the fluid transport properties of the patch, the water collection capability of the replica LIG fern leaf was subsequently compared to that of an actual ostrich fern leaf. This comparison was conducted by using a cold mist humidifier (Fig. 4) with an air flow of 5.55 mL min−1 to understand how condensed water droplets from simulated fog/spray mist would flow on different substrates. The samples were exposed to the deionized water mist at 10 cm, which allowed complete coverage of the fern leaf substrates by mist exhausting from the humidifier. This distance seemed to minimize mist evaporating to the ambient air, though some mist invariably did not reach the leaves. Water collected from the samples followed the wedge tracks or fern leaf pinnules and flowed to the apex of the leaf where the fluid fell into a 100 mL beaker. The water collection experiments were conducted on three different samples (an actual ostrich fern leaf, the LIG replica of the fern leaf, and a control polyimide substrate cut out in the shape of the fern leaf) to demonstrate the effectiveness of LIG fern leaf in collecting water by mist. These three substrates were exposed to the cold mist at three orientations (0°, 45°, 90°) (Fig. 4). The humidifier used 500 mL of water, and the water collected from the fern and replica leaves was calculated as a percentage of total water used. The 0° orientation (apex pointing down) gave the largest water collection efficiency of 2%, 9%, and 12% for the control, replica, and actual leaves, respectively. Once the optimal orientation of the samples was determined (i.e., apex pointing down), the samples were subjected to cold mist for 90 minutes with 300 mL of mist projected from the humidifier during that time. During the 90 minutes under mist conditions, the ostrich fern leaf collected 43.3 ± 1.2 mL of water mist. Almost identically, the LIG replica leaf collected 33.0 ± 2.2 mL of water mist. The control leaf collected 7.8 ± 0.2 mL of water mist. The LIG replica leaf was similar to the ostrich fern leaf in terms of collecting water mist (collecting nearly 77% of the cold mist to that of the real fern leaf as opposed to only 18% for the polyimide control) towards the apex of the device to a functionalized sensor.
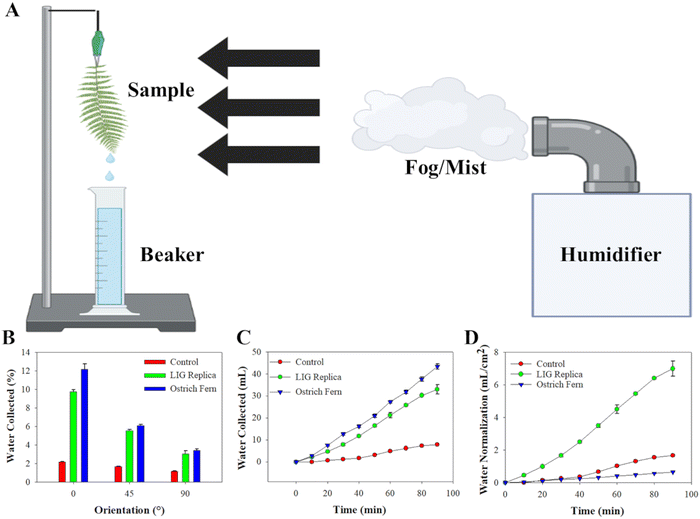 |
| Fig. 4 (A) Schematic showing the fog harvesting experimental set-up and resultant fog harvesting tests for the LIG replica fern, actual ostrich fern, and unpatterned polyimide showing (B) water mist collection capability at different orientation angles, (C) total water mist collected at the vertical position (0° angle), and (D) total water mist collected at the vertical position (0° angle) normalized to the geometric area of the collection material. | |
The data from the collected water mist over the 90-minute period illustrated that the transport mechanism of the capillary system is consistent. Initially, the water collected was slow as the water droplets from the cold mist condensed onto the surface of the three test materials. After approximately 10–15 minutes, the water collected started to show a linear trend on all three materials. It is important to note that with the control polyimide, the water droplets tend to fall off the wedge tracks unlike the ostrich fern and LIG replica. This can be accounted for by microchannels within the ostrich fern and porosity of the LIG which, due to its hydrophilic behavior, causes water to absorb onto the surface and follow the tracks towards the apex(s).29 In contrast, droplets on the control polyimide tended to fall off the tracks instead of traversing along them, which can be attributed to its inherent hydrophobic nature. Finally, we normalized the water collection data from Fig. 4(C) to the surface area of each material. Even though the total water collected was higher for the ostrich fern leaf compared to the LIG replica or polyimide control, the projected surface area of the ostrich fern leaf was much larger (66.67 cm2vs. 4.72 cm2 for LIG replica and polyimide control). Hence, the replica LIG leaf demonstrated an approximately 11-fold increase in efficiency in collecting and transporting water compared to the natural ostrich fern leaf when the data was normalized to geometric area. This underscores the superior water collection capability of LIG fern leaf patch compared to a natural ostrich fern leaf, confirming the effectiveness of the fern leaf design for collecting and transporting pesticide spray.
2.4 Electrochemical sensing of glyphosate with the LIG-based biosensor
2.4.1 Glyphosate calibration with SA glyphosate solution.
The Sigma Aldrich (SA) glyphosate (as described in the Experimental Section) was detected using amperometry at a fixed potential of +0.6 V versus Ag in PBS pH 7.4 with the developed LIG-based biosensor. Glyphosate is catalyzed by the enzyme glycine oxidase to produce H2O2, glyoxylate, and ammonia. The generated H2O2 is oxidized on the biosensor surface by the PtNPs deposited on the biosensor surface and the +0.6 V potential bias applied to the electrode versus the Ag reference electrode.78–80 Through this oxidation, two electrons are released and read as an amperometric signal, which allows the concentration of glyphosate to be directly correlated to a change in current. Consequently, the resultant glyphosate sensor demonstrated a linear sensing range of 10–260 nM (pmodel = 0.001; plack-of-fit = 0.549; R2 = 0.9942), sensitivity of 5.64 nA μM−1, and LOD of 1.15 μM (Fig. 5(A) and (B)). Previously, we developed a test strip working glyphosate biosensor using LIG functionalized with PtNPs and the enzyme glycine oxidase as well.16 Besides presenting a similar linear sensing range (10–260 μM of glyphosate), this previous sensor exhibited reduced sensitivity (0.991 nA μM−1) and higher LOD (3.03 μM). It is worth noting that the earlier work only focused on developing the LIG-based working electrode, necessitating the use of an external Ag/AgCl reference electrode and a platinum counter electrode for its operation. Therefore, this current study illustrates the enhancement in performance achieved by utilizing an all-LIG-based working, reference, and counter-electrode configuration. Furthermore, the sensor implemented in this study was previously tested against major herbicides and insecticides, displaying specificity to glyphosate.16
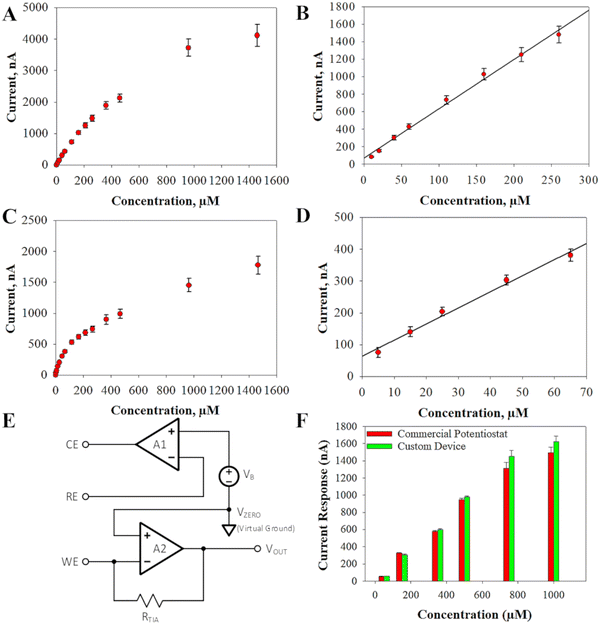 |
| Fig. 5 Calibration of the developed LIG biosensor for the selective detection of the herbicide glyphosate. (A) Electrochemical calibration plot in (A) analytical grade glyphosate and resultant (B) linear sensing range from 10 to 260 μM (n = 8; pmodel = 0.001; plack-of-fit = 0.549; R2 = 0.9942) with an LOD of 1.15 μM. Electrochemical calibration plot in (C) commercial grade glyphosate and resultant (D) linear sensing range from 5 to 65 μM (n = 7; pmodel = 0.001; plack-of-fit = 0.631; R2 = 0.9892) with an LOD of 1.57 μM. (E) Electrical block diagram of the developed portable potentiostat. (F) The current response of the LIG-based biosensor with increasing concentrations of glyphosate as measured from the developed portable potentiostat and a commercial potentiostat. | |
2.4.2 Glyphosate calibration with CA glyphosate solution.
Next, the Corteva Agriscience (CA) glyphosate solution (Abundit Edge herbicide, see Experimental section) was used to better understand the complexity of detecting glyphosate with the developed LIG-based biosensor in a standard ready-to-spray pesticide solution that is applied to farm fields. The LIG-based biosensor presented a linear sensing range from 5 to 65 μM (pmodel = 0.001; plack-of-fit = 0.631; R2 = 0.9892) for the CA glyphosate solution (Fig. 5(C) and (D)), exhibiting a slight decrease in sensitivity (5.07 nA μM−1) and an increase in LOD (1.57 μM) compared to the SA glyphosate solution. Furthermore, a slight improvement at the lower end (10 to 5 μM) but a considerable reduction, four times lower, in the upper range (260 to 65 μM) was also observed. These results are similar to other carbon-based glyphosate biosensors reported in the literature. For example, a graphite-based material sensor with an LOD of 0.148 μM, utilizing horseradish peroxidase as a biorecognition element for testing in river/tap water,81 and a graphene-based material sensor employing zinc oxide NPs to detect glyphosate in green tea, corn juice, and mango juice.82 It is worth noting that while we anticipate our sensors being selective for glyphosate in commercially available solutions, there is some uncertainty regarding their accuracy. Companies in the United States manufacturing commercial pesticide solutions are required to register their ingredients, including the active ingredient, with the Environmental Protection Agency (EPA). However, only the active ingredient is publicly disclosed, making it challenging to identify other chemical species that might inhibit the glycine oxidase enzyme or oxidize the PtNPs electrodeposited onto the LIG surface. Future research will be necessary to address this concern.
2.5 Development of portable amperometric measuring instrument and testing of integrated LIG fern leaf and biosensor system
2.5.1 Construction and programming of the portable amperometric measuring instrument.
The typical choice of test equipment for interfacing with an electrochemical cell, as described in this manuscript, is a commercial potentiostat. While potentiostats come in various forms, a laboratory-grade benchtop potentiostat is the most common. These devices offer several advantages such as flexibility, precision, and accuracy. However, they are often large, expensive, and consume relatively large amounts of power, making them ill-suited for use in the field.83 To move the development of sensor technology into practical application spaces, a portable, inexpensive, and low-power sensor interface is required using custom-designed hardware, several examples of which, have been also reported in the research literature.84–87 To make the developed biosensor system portable and ready for use in a farm field, we created a portable potentiostat or amperometric measurement instrument (AMI) for the LIG-based biosensor system. A block diagram of the developed AMI is shown in Fig. S6 (ESI†). The analog front end is used to properly bias the electrochemical cell as well as to convert the cell current into an analog output voltage. The microcontroller (MCU) is used to digitize the output voltage from the analog front end and transmit the data to the host computer via USB. Additionally, the microcontroller allows the user to control the configuration of the analog front end. More details about these components are given below.
The analog front end is implemented using the LMP91000 analog front end potentiostat from Texas Instrument. A behavioral block diagram of the LMP91000 is shown in Fig. 5(E). The LMP91000 consists of three elements: the bias voltage generator, the transimpedance amplifier, A2, and the control amplifier, A1. The bias voltage generator allows the user to control the voltage potential between the working and reference electrodes. The bias voltage, VB, is set by the user, and can be varied from −600 mV to +600 mV in 50 mV steps. The control amplifier compares the potential between the working and reference electrodes to the desired bias voltage set by the bias generator, VB. The error signal is amplified and applied to the counter electrode, thereby maintaining a constant working-to-reference voltage potential. According to the datasheet of the LMP91000, the nominal gain of the control amplifier is 120 dB. The control amplifier can supply up to 750 μA into the counter electrode. The current flowing into the reference terminal is less than 90 pA.
The negative feedback of the transimpedance amplifier holds the working electrode at a constant DC voltage, referred to as the zero voltage, VZERO. The zero voltage is the nominal output voltage of the transimpedance amplifier when the cell current, ICELL, is zero. This allows for the output voltage of the analog front end to indicate both positive and negative cell currents. Because VZERO is constant, it serves as a virtual ground internally to the LMP91000. However, the absolute value of VZERO, with respect to ground, must be accounted for by the analog-to-digital converter. The smallest cell current that can be detected by the analog front is determined by the transimpedance amplifier as well as the analog-to-digital converter, and can be expressed as:
| ICELL_min = VREF/(RTIA × 2N) | (2) |
where
VREF and
N are the reference voltage and resolution of the analog-to-digital converter, respectively.
RTIA is the gain of the transimpedance amplifier. The largest variation in the cell current that can be detected, before the output voltage saturates, can be expressed as:
| ICELL_fs = (VREF − VZERO)/RTIA | (3) |
Typically, the reference voltage is fixed, so the values of ICELL_min and ICELL_fs are primarily determined by the gain of the transimpedance amplifier, RTIA. The gain of the transimpedance amplifier is set by the user and can be varied from 2.75 kΩ to 350 kΩ in six discrete steps.
The developed portable AMI also uses a MSP430 microcontroller from Texas Instruments which has an integrated 12-bit analog-to-digital converter with a reference voltage of VREF = 2.5 V. The zero voltage is set internally to be VZERO = 1.25. By setting RTIA = 350 kΩ, the smallest change in the cell current that can be detected is approximately 1.7 nA. This excellent resolution comes at the cost of a relatively small full-scale range for the cell current. When RTIA = 350 kΩ, the full-scale current range is approximately 3.57 μA. If a larger full-scale current range is desired, then the gain of the transimpedance amplifier can be reduced. For example, if RTIA is set to its minimum value of 2.75 kΩ then the full-scale current range becomes ± 455 μA. The smallest change in ICELL that can be detected with RTIA = 2.75 kΩ is 222 nA. A complete listing of the minimum detectable currents and the full-scale range that can be achieved for the different values of RTIA is shown in Table 2. Moreover, a snapshot of the graphical user interface that was developed to run the AMI from a computer is displayed in Fig. S7 (ESI†).
Table 2 Performance metrics for the portable AMI under varying transimpedance amplifier (RTIA) gain values
R
TIA (kΩ) |
Minimum detectable current (nA) |
Full-scale current (μA) |
2.75 |
222 |
455 |
3.5 |
174 |
357 |
7 |
82 |
179 |
14 |
44 |
89 |
35 |
17 |
36 |
120 |
5.1 |
10 |
350 |
1.7 |
3.6 |
Verification tests were conducted between the developed AMI and a CH Instruments tabletop potentiostat for the accuracy of current responses generated from the LIG-based glyphosate biosensors. Single volumetric additions were added to a beaker containing PBS and the glyphosate sensor. These additions had equivalent concentrations as displayed in Fig. 5(F). Our developed AMI showed up to 97% accuracy to the commercial potentiostat at concentrations within the linear range of our sensor. As the glyphosate concentration went beyond that range, our device still showed high accuracy of up to 92%.
2.5.2 Quantification of glyphosate pesticide spray with integrated LIG fern leaf and biosensor.
To demonstrate the capability of the LIG collection patch, glyphosate collection tests were performed intermittently over a 2-week period. A solution was collected from each device and tested with the calibrated LIG biosensor and developed AMI to sense glyphosate (from four different sensors, n = 4) from multiple patches collected from an outdoor field within 24–48 hours of pesticide spray as well as one week and two weeks after spraying (from 5 different patches for each time period, n = 5). The LIG collection patches were initially gathered after 24 hours to replicate the restricted-entry interval (REI) commonly observed in agricultural fields. This period allows the pesticide to dry before farmers and agricultural workers enter, minimizing health risks associated with exposure to pesticide inhalation, skin contact, or ingestion. The pesticide from the LIG patches were solubilized via a PBS buffer rinse and three solutions were tested with the LIG sensors yielding a biosensor recovery signal of 73.64 ± 4.94%, 14.02 ± 5.18%, and no response for the collection patches gathered 24–48 hours, 1 week, and 2 weeks after pesticide spraying respectively. In these experiments, it is assumed that a portion of the sprayed pesticide becomes trapped within the pores and high surface area of the LIG and thus is unable to desorb after rinsing with water mist, resulting in a low recovery percentage. However, the consistent biosensor recovery signals mean that these trapped pesticide residues could be accounted for, and the biosensor signal was normalized accordingly. For example, by using a correction factor of 1.36 we could accurately predict the true concentration of pesticide sprayed and monitored through the LIG-based “collect-and-sense” system for the pesticide acquired from the LIG patches collected 24 to 48 hours after spraying. However, this data also underscores the significance of promptly collecting the LIG patches following a pesticide spray event. The short half-life of glyphosate, ranging from 10.4 to 26.6 days when sprayed on foliage and litter88 and potentially reduced to 33.7 hours with water and sunlight due to photodegradation and biodegradation,89 leads to a nearly 60% decline in the sensor response signal after one week and renders it unreadable after two weeks. The temperature, moisture, and overall weather conditions of the LIG patches placed in an outdoor field to acquire this time-dependent data are detailed in the Experimental Section.
3. Conclusions
In conclusion, this work demonstrates the use of a hydrophilic LIG device to collect sprayed glyphosate and transport glyphosate residue to a singular point to create a testable solution. This solution was then used alongside a developed enzymatic biosensor and portable AMI for the selective monitoring of glyphosate from pesticide sprays—demonstrating a field deployable “collect-and-sense” system which circumvents the need to send samples back to a laboratory for testing. Adjusting laser parameters during LIG fabrication enabled the creation of a highly porous and hydrophilic fern pinnule-shaped collection patch for efficient water and pesticide transport to a connected LIG biosensor. The LIG biosensor, functionalized with PtNPs and glycine oxidase, was able to accurately monitor the SA glyphosate solution with a sensitivity of 5.64 nA μM−1, LOD of 1.15 μM, and linear sensing range of 10–260 μM. Moreover, the biosensor displayed a sensitivity of 5.07 nA μM−1, LOD of 1.57 μM, and linear sensing range of 5–65 μM for the CA glyphosate solution. The developed AMI exhibited a high level of accuracy, with sensor signals monitored closely matching those obtained from a standard commercial benchtop potentiostat, achieving up to 97% similarity. This capability allows for the use of the developed biosensor systems in remote locations, including farm fields. The “collect-and-sense” system could consistently collect and monitor glyphosate spray after 24–48 hours of spraying in a farm field, but sensor signal rapidly decayed after one week of spraying and yielded negligible signal after two weeks due to the rapid decay of the pesticide. Therefore, it is important to collect the sensors from the farm field soon after the restricted-entry interval (REI) to ensure accurate detection of pesticide residues and maximize the effectiveness of the LIG-based “collect-and-sense” system in pesticide monitoring.
Hence, this work presents a novel approach for monitoring and quantifying pesticide spray/residue using a portable, cost-effective, and energy-efficient “collect-and-sense” system. This user-friendly technology empowers researchers and agricultural professionals to precisely track and map pesticide applications, enabling more accurate spatial distribution of pesticide applications in farm fields and addressing concerns about pesticide pollution on unintended surfaces due to drift. Furthermore, this study establishes a foundation for potential future applications involving a combination of an LIG collection device, LIG sensor, and portable electrochemical unit. For instance, this sensor system could be adapted for collecting human urine samples in smart toilets, enabling point-of-care detection of key markers like creatinine and albumin,90 uric acid,91 or even glucose.92 These biomarkers play pivotal roles as indicators of various organ diseases, enhancing early disease detection and healthcare monitoring. Another application lies in deploying the collection device to potential contamination sources such as field runoffs (e.g., tile drainage lines or streams adjacent to farm fields) where glyphosate,62,93 dicamba,94 or other pesticides accumulate post-application on crops. Similarly, the “collect-and-sense” system could be employed to monitor heavy metals95,96 or plant nutrients,97,98 offering real-time testing capabilities for solutions of soil or water.
4. Experimental section
4.1 Materials and reagents
Phosphate buffer saline tablets, glutaraldehyde (25%), flavin adenine dinucleotide, sodium chloride, potassium chloride, potassium hexacyanoferrate(II) trihydrate, potassium ferricyanide, perchloric acid (70%), chloroplatinic acid (8%), and analytical grade glyphosate were purchased from Sigma Aldrich (SA). Glycine oxidase was prepared by collaborators at the U.S. Naval Research Laboratory and shipped to our lab at Iowa State University. Glyphosate and glutaraldehyde solutions were prepared in PBS pH 7.4. Kapton polyimide sheets (0.125 μm thick) were purchased from McMaster-Carr. Abundit Edge herbicide from Corteva Agriscience (CA) was mixed with water (32 ounce/acre herbicide per 15 GPA water).
4.2 Fabrication and characterization of LIG replica leaf and enzymatic sensors
The LIG replica leaf and glyphosate sensor were created on Kapton polyimide film that was taped to an aluminum plate, cleaned with isopropyl alcohol, and placed on the bed of a 75 W CO2 (Epilog Fusion M2) laser. A defocus of 2 mm was used alongside laser settings of 15% speed, 7% power, 50% frequency, and 1200 dots per inch (DPI) was used to create the LIG collection patch. The 3-electrode LIG used similar laser settings, except for the laser speed which decreased to 7%. The laser converted the polyimide into a 3-electrode LIG design with a 3 mm diameter working electrode as shown in Fig. 1. The LIG fern leaf used 7° wedge tracks or pinnules that converged to a central stem and was designed in CorelDRAW (Fig. 1). Acrylic polish was used across the stern of the electrodes to maintain a constant working area. Ag paste was applied to the right-most LIG electrode to create the reference electrode (Fig. 1). The 3-electrode sensor was placed on a hot plate and cured at 100 °C for 10 minutes. A 3-electrode cell, with an Ag/AgCl (0.1 M KCl), platinum counter wire, and connection to the LIG working electrode, was applied with a step function in perchloric acid (0.1 M) and chloroplatinic acid (5 mM) at −0.5 V for 30 seconds to deposit PtNPs on the surface of the LIG working and counter electrodes. This platinum deposition process was repeated 4 times for the LIG biosensor.
4.3 Water collection experiments
Water collection experiments were performed by using a commercial humidifier (Honeywell Ultrasonic) to expose the LIG replica leaf, ostrich fern leaf, and polyimide control leaf to deionized (DI) water in the form of cool mist at an air flow rate of 5.55 mL min−1 and at a distance of 10 cm from the samples. Water collected fell into a 100 mL beaker to observe and calculate water collection rates.
4.4 Functionalization of enzymatic sensors
Glycine oxidase (190 μM in 10× PBS pH 7.4), glutaraldehyde (0.25%), and flavin adenine dinucleotide (FAD) (2 nM in 10× PBS pH 8.4) were mixed in equal volume ratios. The solution was drop-coated onto a Petri dish where it was mixed via pipette mixing for 20 cycles. An aliquot of 3 μL of this solution was then pipetted onto the working electrode and allowed to dry in air for approximately 30 minutes. Sensors were stored at 8 °C overnight and were not removed until tested.
4.5 Development of portable amperometry device
An analog front-end board (LMP91000) and microcontroller (MCU) were purchased from Texas Instruments. Firmware and the graphical user interface (GUI) of AMI were developed by Dr Nathan Neihart, professor of electrical engineering at Iowa State University.
4.6 Electrochemical analysis
A CH Instruments potentiostat was used for all sensor calibration electrochemical measurements. The developed AMI, which utilized the programmable analog front-end board and MCU as previously mentioned, was used in parallel to a CH Instruments potentiostat for all other measurements. For PtNPs deposited onto the working electrode, a commercial counter wire and Ag/AgCl reference (0.1 M KCl) purchased from CH Instruments was utilized to assist in this process. All other electrochemical measurements used the 3-electrode LIG design that was described previously. Electrochemical sensing with the completed LIG biosensor was performed in 5 mL of the PBS pH 7.4 solution with an applied potential of +0.6 V at a stir rate of 150 rpm. Amperometry was used to measure the current from the glyphosate oxidation. Results were reported as the change in current between each plateau of glyphosate addition and the recorded baseline before the additions.
4.7 Glyphosate collection and detection
LIG collection patches were created and sprayed with solutions prepared from the SA glyphosate salt. We first tested 5 LIG devices by spraying 4.7 mL of 5 mM glyphosate onto the LIG and letting them dry for 24–48 hours. The reasoning behind this was to imitate what happens when a pesticide is sprayed in a field, where farmers and agricultural workers must wait 24–48 hours before entering a pesticide sprayed field, i.e., the REI, so the pesticide can dry. After 24–48 hours we collected a solution from each patch to test with our calibrated sensors. We placed 10 collection devices outside to observe how environmental conditions impact the glyphosate collection process. We collected 5 devices after a 1-week period. During this time, the average high temperature was 60.1°F, average low temperature of 34.9°F and total precipitation of 0.01 inches of precipitation. We collected the remaining 5 devices after another week, making a total of 2 weeks for this experiment. During the 2-weeks, the average high temperature was 62.3°F, average low temperature of 38.2°F and total precipitation of 0.04 inches of precipitation. The LIG collection patch was rinsed with 10 mL of PBS to solubilize the pesticide for subsequent testing. This rinsing of pesticide from the LIG collection patch is visualized in Movie 2 (ESI†) where fluorescent dye is added to the solution for visualization (ESI†). The glyphosate sensors (n = 4) were placed in a beaker containing 10 mL of fresh PBS and 100 μL of the collected solutions were pipetted into the beaker to measure the electrical current response in the cell using the developed AMI. After obtaining the detectable current from each solution, the linear regression equations from the previous calibrations were used to determine the concentration of the solutions in each beaker to obtain the recovery data.
4.8 Data/statistical analysis
The study was carried out in a completely randomized design with at least four replicates, and the results were reported as mean ± standard deviation. Data was collected with both the CH Instruments potentiostat and the custom built potentiostat (i.e., the AMI). Data was saved either directly as a.csv file or a text file and converted to a.csv file. For sensor calibration studies, experiments were performed with n = 8 sensors. For glyphosate collection studies, n = 5 collection devices with n = 4 sensors per device were performed. Regression analysis was performed to determine the linear sensing range and the functional correspondence between the concentration of glyphosate and the current signal at a level of significance of 5% (α = 0.05). JMP Pro v.17 statistical software (SAS Institute, Cary, NC, USA) was used to perform the regression analysis. The limit of detection was calculated according to the 3-Sigma method.99
Author contributions
This study was conceptualized by N. Jared, Z. T. Johnson, N. M. Neihart, and J.C. Claussen. N. Jared created the biomimetic collection patch, conducted sample wettability experiments, developed the glyphosate LIG sensor with Z. T. Johnson, and conducted field experiments with K. K. Bez. N. Jared and J. C. Claussen analyzed the collection patch and sensor data results. K. Bez and E. A. Smith conducted Raman measurements and corresponding analysis. N. M. Neihart developed the AMI and N. Jared validated the accuracy of the device. S. L. Hooe, J. C. Breger, I. L. Medintz provided knowledge and materials for glycine oxidase creation and use. C. Pola conducted a formal analysis for the sensor calibration study. N. Jared, Z. T. Johnson, N. M. Neihart, and J. C. Claussen wrote the manuscript and all authors provided manuscript review and edits.
Data availability
The data supporting this article have been included as part of the ESI.†
Conflicts of interest
There are no conflicts to declare.
Acknowledgements
J. C. Claussen gratefully acknowledges funding support for this work from the National Science Foundation under award number CMMI-2037026 as well as from the National Institute of Food and Agriculture, U.S. Department of Agriculture under award numbers 2021-67021-34457 and 2021-67011-35130. I. L. Medintz acknowledges the Office of Naval Research, the U.S. Naval Research Laboratory (NRL), the NRL Nanoscience Institute, and the National Institute of Food and Agriculture, U.S. Department of Agriculture, under award number 2020-67021-31254 for funding support. The authors wish to acknowledge Sensitive Instrument Facility (SIF) and Materials Analysis and Research Laboratory (MARL) staff at Iowa State University for the support and help with the SEM and XPS experiments. Fig. 1 and 4 were partially created with BioRender.com.
References
- J. G. da Silva, UN Chron, 2012, 49, 15–17 CrossRef.
- M. Tudi, H. D. Ruan, L. Wang, J. Lyu, R. Sadler, D. Connell, C. Chu and D. T. Phung, Int. J. Environ. Res. Public Health, 2021, 18, 1–24 CrossRef PubMed.
- F. H. M. Tang, M. Lenzen, A. McBratney and F. Maggi, Nat. Geosci., 2021, 14, 206–210 CrossRef CAS.
- A. R. A. Zanin, D. C. Neves, L. P. R. Teodoro, C. A. da Silva Júnior, S. P. da Silva, P. E. Teodoro and F. H. R. Baio, Sci. Rep., 2022, 12, 5638 CrossRef CAS PubMed.
- A. Walter, R. Finger, R. Huber and N. Buchmann, Proc. Natl. Acad. Sci. U. S. A., 2017, 114, 6148–6150 CrossRef CAS PubMed.
- A. Martin, C. Margoum, M. Coquery and J. Randon, J. Sep. Sci., 2016, 39, 3990–3997 CrossRef CAS.
- S. E. Hale, T. J. Martin, K. U. Goss, H. P. H. Arp and D. Werner, Environ. Pollut., 2010, 158, 2511–2517 CrossRef CAS PubMed.
- T. Gouin, F. Wania, C. Ruepert and L. E. Castillo, Environ. Sci. Technol., 2008, 42, 6625–6630 CrossRef CAS PubMed.
- T. O. Hara and B. Singh, ACS ES&T Water, 2021, 1, 462–478 Search PubMed.
- Z. T. Johnson, K. Williams, B. Chen, R. Sheets, N. Jared, J. Li, E. A. Smith and J. C. Claussen, ACS Sens., 2021, 6, 3063–3071 CrossRef CAS.
- J. A. Hondred, Z. T. Johnson and J. C. Claussen, J. Mater. Chem. C, 2020, 8, 11376–11388 RSC.
- J. A. Hondred, J. C. Breger, N. J. Alves, S. A. Trammell, S. A. Walper, I. L. Medintz and J. C. Claussen, ACS Appl. Mater. Interfaces, 2018, 10, 11125–11134 CrossRef CAS.
- J. A. Hondred, J. C. Breger, N. T. Garland, E. Oh, K. Susumu, S. A. Walper, I. L. Medintz and J. C. Claussen, Analyst, 2017, 142, 3261–3271 RSC.
- A. Cioffi, M. Mancini, V. Gioia and S. Cinti, Environ. Sci. Technol., 2021, 55, 8859–8865 CrossRef CAS.
- I. S. Kucherenko, B. Chen, Z. Johnson, A. Wilkins, D. Sanborn, N. Figueroa-Felix, D. Mendivelso-Perez, E. A. Smith, C. Gomes and J. C. Claussen, Anal. Bioanal. Chem., 2021, 413, 6201–6212 CrossRef CAS.
- Z. T. Johnson, N. Jared, J. K. Peterson, J. Li, E. A. Smith, S. A. Walper, S. L. Hooe, J. C. Breger, I. L. Medintz, C. Gomes and J. C. Claussen, Glob. Chall., 2022, 6, 2200057 CrossRef.
- B. Chen, Z. T. Johnson, D. Sanborn, R. G. Hjort, N. T. Garland, R. R. A. Soares, B. Van Belle, N. Jared, J. Li, D. Jing, E. A. Smith, C. L. Gomes and J. C. Claussen, ACS Nano, 2022, 16, 15–28 CrossRef CAS.
- Q. Dong, H. Ryu and Y. Lei, Electrochim. Acta, 2021, 370, 137744 CrossRef CAS.
- D. Du, J. Wang, L. Wang, D. Lu and Y. Lin, Anal. Chem., 2012, 84, 1380–1385 CrossRef CAS PubMed.
- J. Guo and X. Ma, Biosens. Bioelectron., 2017, 94, 415–419 CrossRef CAS PubMed.
- L. Wang, D. Lu, J. Wang, D. Du, Z. Zou, H. Wang, J. N. Smith, C. Timchalk, F. Liu and Y. Lin, Biosens. Bioelectron., 2011, 26, 2835–2840 CrossRef CAS PubMed.
- E. L. Fava, T. A. Silva, T. M. do Prado, F. C. de Moraes, R. C. Faria and O. Fatibello-Filho, Talanta, 2019, 203, 280–286 CrossRef CAS.
- C. Te Kung, C. Y. Hou, Y. N. Wang and L. M. Fu, Sens. Actuators, B, 2019, 301, 126855 CrossRef.
- T. O. Hara and B. Singh, ACS ES&T Water, 2021, 1, 462–478 Search PubMed.
- M. D. Fernández-Ramos, A. L. Ogunneye, N. A. A. Barbarinde, M. M. Erenas and L. F. Capitán-Vallvey, Talanta, 2020, 218, 121108 CrossRef PubMed.
- V. Caratelli, G. Fegatelli, D. Moscone and F. Arduini, Biosens. Bioelectron., 2022, 205, 114119 CrossRef CAS.
- S. Zhang, J. Huang, Z. Chen and Y. Lai, Small, 2017, 13 Search PubMed.
- F. Bai, J. Wu, G. Gong and L. Guo, Adv. Sci., 2015, 2, 150047 Search PubMed.
- V. Sharma, R. Balaji and V. Krishnan, Biomimetics, 2018, 3, 7 CrossRef.
- D. W. M. Puente and P. Baur, Pest Manage. Sci., 2011, 67, 798–806 CrossRef CAS PubMed.
- É. Smedbol, M. Lucotte, S. Maccario, M. P. Gomes, S. Paquet, M. Moingt, L. L. C. Mercier, M. R. P. Sobarzo and M. A. Blouin, J. Agric. Food Chem., 2019, 67, 6133–6142 CrossRef.
- Y. Temiz, R. D. Lovchik, G. V. Kaigala and E. Delamarche, Microelectron. Eng., 2015, 132, 156–175 CrossRef CAS.
- A. Ghosh, R. Ganguly, T. M. Schutzius and C. M. Megaridis, Lab Chip, 2014, 14, 1538–1550 RSC.
- L. S. Hall, D. Hwang, B. Chen, B. Van Belle, Z. T. Johnson, J. A. Hondred, C. L. Gomes, M. D. Bartlett and J. C. Claussen, Nanoscale Horiz., 2021, 6, 24–32 RSC.
- V. Jokinen, L. Sainiemi and S. Franssila, Adv. Mater., 2008, 20, 3453–3456 CrossRef CAS.
- A. Nakajima, Y. Nakagawa, T. Furuta, M. Sakai, T. Isobe and S. Matsushita, Langmuir, 2013, 29, 9269–9275 CrossRef CAS PubMed.
- A. W. Martinez, S. T. Phillips, M. J. Butte and G. M. Whitesides, Angew. Chem., 2007, 119, 1340–1342 CrossRef.
- E. M. Fenton, M. R. Mascarenas, G. P. López and S. S. Sibbett, ACS Appl. Mater. Interfaces, 2009, 1, 124–129 CrossRef CAS.
- E. Fu, T. Liang, J. Houghtaling, S. Ramachandran, S. A. Ramsey, B. Lutz and P. Yager, Anal. Chem., 2011, 83, 7941–7946 CrossRef CAS.
- K. Yang, Q. Wang, K. S. Novoselov and D. V. Andreeva, Nanoscale Horiz., 2023, 8, 1243–1252 RSC.
- G. Moro, F. Fama, N. Colozza, A. Gambaro, M. Bassanello, F. Arduini and C. Zanardi, Green, Anal. Chem., 2023, 7, 100076 Search PubMed.
- P. Sawetwong, S. Chairam, P. Jarujamrus and M. Amatatongchai, Talanta, 2021, 225, 122077 CrossRef CAS.
- K. S. Novoselov, Nature, 2012, 490, 192–200 CrossRef CAS.
- M. R. Habib, T. Liang, X. Yu, X. Pi, Y. Liu and M. Xu, Rep. Prog. Phys., 2018, 81 Search PubMed.
- Y. Zhang, L. Zhang and C. Zhou, Acc. Chem. Res., 2013, 46, 2329–2339 CrossRef CAS.
- H. Shu, X. Chen, X. Tao and F. Ding, ACS Nano, 2012, 6, 3243–3250 CrossRef CAS.
- H. Shu, X. M. Tao and F. Ding, Nanoscale, 2015, 7, 1627–1634 RSC.
- J. Lin, Y. Huang, S. Wang and G. Chen, Ind. Eng. Chem. Res., 2017, 56, 9341–9346 CrossRef CAS.
- Z. Xu, H. Li, W. Li, G. Cao, Q. Zhang, K. Li, Q. Fu and J. Wang, Chem. Commun., 2011, 47, 1166–1168 RSC.
- N. L. Rangel, J. C. Sotelo and J. M. Seminario, J. Chem. Phys., 2009, 131, 031105 CrossRef PubMed.
- T. Sun and S. Fabris, Nano Lett., 2012, 12, 17–21 CrossRef CAS.
- M. Yi and Z. Shen, J. Mater. Chem. A, 2015, 3, 11700–11715 RSC.
- A. Martinez, K. Fuse and S. Yamashita, Appl. Phys. Lett., 2011, 99, 121107 CrossRef.
- W. Wu, M. Liu, Y. Gu, B. Guo, H. X. Ma, P. Wang, X. Wang and R. Zhang, Chem. Eng. J., 2020, 381, 122592 CrossRef CAS.
- L. Zhang, X. Li, Y. Huang, Y. Ma, X. Wan and Y. Chen, Carbon N. Y., 2010, 48, 2367–2371 CrossRef CAS.
- Q. He, S. R. Das, N. T. Garland, D. Jing, J. A. Hondred, A. A. Cargill, S. Ding, C. Karunakaran and J. C. Claussen, ACS Appl. Mater. Interfaces, 2017, 9, 12719–12727 CrossRef CAS.
- S. R. Das, S. Srinivasan, L. R. Stromberg, Q. He, N. Garland, W. E. Straszheim, P. M. Ajayan, G. Balasubramanian and J. C. Claussen, Nanoscale, 2017, 9, 19058–19065 RSC.
- P. He, J. Cao, H. Ding, C. Liu, J. Neilson, Z. Li, I. A. Kinloch and B. Derby, ACS Appl. Mater. Interfaces, 2019, 11, 32225–32234 CrossRef CAS PubMed.
- L. Gamba, Z. T. Johnson, J. Atterberg, S. Diaz-Arauzo, J. R. Downing, J. C. Claussen, M. C. Hersam and E. B. Secor, ACS Appl. Mater. Interfaces, 2023, 15, 3325–3335 CrossRef CAS PubMed.
- E. B. Secor, S. Lim, H. Zhang, C. D. Frisbie, L. F. Francis and M. C. Hersam, Adv. Mater., 2014, 26, 4533–4538 CrossRef CAS.
- R. G. Hjort, R. R. A. Soares, J. Li, D. Jing, L. Hartfiel, B. Chen, B. Van Belle, M. Soupir, E. Smith, E. McLamore, J. C. Claussen and C. L. Gomes, Microchim. Acta, 2022, 189, 122 CrossRef CAS PubMed.
- M. Helander, I. Saloniemi and K. Saikkonen, Trends Plant Sci., 2012, 17, 569–574 CrossRef CAS PubMed.
- J. S. Noori, M. Dimaki, J. Mortensen and W. E. Svendsen, Sensors, 2018, 18, 2961 CrossRef PubMed.
- Z. Ni, Y. Wang, T. Yu and Z. Shen, Nano Res., 2008, 1, 273–291 CrossRef CAS.
- Q. H. Wang, Z. Jin, K. K. Kim, A. J. Hilmer, G. L. C. Paulus, C. J. Shih, M. H. Ham, J. D. Sanchez-Yamagishi, K. Watanabe, T. Taniguchi, J. Kong, P. Jarillo-Herrero and M. S. Strano, Nat. Chem., 2012, 4, 724–732 CrossRef CAS PubMed.
- J. S. Park, A. Reina, R. Saito, J. Kong, G. Dresselhaus and M. S. Dresselhaus, Carbon N. Y., 2009, 47, 1303–1310 CrossRef CAS.
- A. C. Ferrari, J. C. Meyer, V. Scardaci, C. Casiraghi, M. Lazzeri, F. Mauri, S. Piscanec, D. Jiang, K. S. Novoselov, S. Roth and A. K. Geim, Phys. Rev. Lett., 2006, 97, 7401 Search PubMed.
-
W. Gough, The graphical analysis of a Lorentzian function and a differentiated Lorentzian function, 1968 Search PubMed.
- V. T. Nguyen, H. D. Le, V. C. Nguyen, T. T. T. Ngo, D. Q. Le, X. N. Nguyen and N. M. Phan, Adv. Nat. Sci.: Nanosci. Nanotechnol., 2013, 4, 5012 Search PubMed.
- M. Y. Smirnov, A. V. Kalinkin, E. I. Vovk, P. A. Simonov, E. Y. Gerasimov, A. M. Sorokin and V. I. Bukhtiyarov, Appl. Surf. Sci., 2018, 428, 972–976 CrossRef CAS.
- J. C. Claussen, A. Kumar, D. B. Jaroch, M. H. Khawaja, A. B. Hibbard, D. M. Porterfield and T. S. Fisher, Adv. Funct. Mater., 2012, 22, 3399–3405 CrossRef CAS.
- N. Aristov and A. Habekost, World J. Chem. Educ., 2015, 3, 82–86 CrossRef CAS.
- Y. Uchida, E. Kätelhön and R. G. Compton, J. Electroanal. Chem., 2018, 810, 135–144 CrossRef CAS.
- A. G. M. Ferrari, C. W. Foster, P. J. Kelly, D. A. C. Brownson and C. E. Banks, Biosensors, 2018, 8, 53 CrossRef CAS.
- P. Nayak, N. Kurra, C. Xia and H. N. Alshareef, Adv. Electron. Mater., 2016, 2, 1600185 CrossRef.
- M. Ebner, T. Miranda and A. Roth-Nebelsick, J. Arid Environ., 2011, 75, 524–531 CrossRef.
- Z. Ali, S. Merrium, M. Habib-ur-Rahman, S. Hakeem, M. A. B. Saddique and M. A. Sher, Environ. Sci. Pollut. Res., 2022, 29, 30967–30985 CrossRef.
- I. Katsounaros, W. B. Schneider, J. C. Meier, U. Benedikt, P. U. Biedermann, A. A. Auer and K. J. J. Mayrhofer, Phys. Chem. Chem. Phys., 2012, 14, 7384–7391 RSC.
-
V. G. Prabhu, L. R. Zarapkar and R. G. Dhaneshwar, Electrochemical studies of hydrogen peroxide at a platinum disc electrode, 1981 Search PubMed.
- S. B. Hall, E. A. Khudaish and A. L. Hart, Electrochim. Acta, 1998, 43, 579–588 CrossRef CAS.
- L. A. Zambrano-Intriago, C. G. Amorim, A. N. Araújo, D. Gritsok, J. M. Rodríguez-Díaz and M. C. B. S. M. Montenegro, Sci. Total Environ., 2023, 855, 158865 CrossRef CAS.
- P. Traiwatcharanon, S. Velmurugan, M. Zacharias and C. Wongchoosuk, Nanotechnology, 2023, 34, 5501 CrossRef PubMed.
- A. A. Rowe, A. J. Bonham, R. J. White, M. P. Zimmer, R. J. Yadgar, T. M. Hobza, J. W. Honea, I. Ben-Yaacov and K. W. Plaxco, PLoS One, 2011, 6, 23783 CrossRef.
- T. Dobbelaere, P. M. Vereecken and C. Detavernier, HardwareX, 2017, 2, 34–49 CrossRef.
- S. D. Adams, E. H. Doeven, K. Quayle and A. Z. Kouzani, IEEE Access, 2019, 7, 31903–31912 Search PubMed.
- C. Mercer, R. Bennett, P. Conghaile, J. F. Rusling and D. Leech, Sens. Actuators, B, 2019, 290, 616–624 CrossRef CAS.
- V. Bianchi, A. Boni, S. Fortunati, M. Giannetto, M. Careri and I. De Munari, IEEE Trans. Instrum. Meas., 2020, 69, 3232–3240 Search PubMed.
- M. Newton, K. M. Howard, B. R. Kelpsas, R. Danhaus, C. Marlene Lottman and S. Dubelman, J. Agric. Food Chem., 1984, 32, 1144–1151 CrossRef CAS.
- J. An, Y. Jiang, H. Cao, C. Yi, S. Li, M. Qu and G. Liu, Ecotoxicol. Environ. Saf., 2023, 263, 115211 CrossRef CAS PubMed.
- Z. Shi, C. Dai, P. Deng, Y. Wu, G. Liu, Z. An, H. Liang, F. Zhang, Y. Lu and Q. Liu, Lab Chip, 2023, 23, 3424–3432 RSC.
- E. Akyilmaz, M. K. Sezgintürk and E. Dinçkaya, Talanta, 2003, 61, 73–79 CrossRef CAS.
- M. Miyashita, N. Ito, S. Ikeda, T. Murayama, K. Oguma and J. Kimura, Biosens. Bioelectron., 2009, 24, 1336–1340 CrossRef CAS PubMed.
- A. L. Valle, F. C. C. Mello, R. P. Alves-Balvedi, L. P. Rodrigues and L. R. Goulart, Environ. Chem. Lett., 2019, 17, 291–317 CrossRef CAS.
- E. Oseland, M. Bish, L. Steckel and K. Bradley, Pest Manage. Sci., 2020, 76, 3282–3291 CrossRef CAS.
- M. F. Siddiqui, S. Kim, H. Jeon, T. Kim, C. Joo and S. Park, Sensors, 2018, 18, 777 CrossRef PubMed.
- V. H. C. Liao, M. Te Chien, Y. Y. Tseng and K. L. Ou, Environ. Pollut., 2006, 142, 17–23 CrossRef CAS.
- W. K. Abdelbasset, S. V. Savina, D. Mavaluru, R. A. Shichiyakh, D. O. Bokov and Y. F. Mustafa, Talanta, 2023, 252 Search PubMed.
- B. Kashyap and R. Kumar, IEEE Access, 2021, 9, 14095–14121 Search PubMed.
-
A. D. W. A. McNaught, IUPAC Compendium of Chemical Terminology, 1997, vol. 1669 Search PubMed.
|
This journal is © The Royal Society of Chemistry 2024 |
Click here to see how this site uses Cookies. View our privacy policy here.