DOI:
10.1039/D3NR06297J
(Paper)
Nanoscale, 2024,
16, 2974-2982
Ultralow-cost piezoelectric sensor constructed by thermal compression bonding for long-term biomechanical signal monitoring in chronic mental disorders†
Received
10th December 2023
, Accepted 15th January 2024
First published on 17th January 2024
Abstract
Wearable bioelectronic devices, which circumvent issues related to the large size and high cost of clinical equipment, have emerged as powerful tools for the auxiliary diagnosis and long-term monitoring of chronic psychiatric diseases. Current devices often integrate multiple intricate and expensive devices to ensure accurate diagnosis. However, their high cost and complexity hinder widespread clinical application and long-term user compliance. Herein, we developed an ultralow-cost poly(vinylidene fluoride)/zinc oxide nanofiber film-based piezoelectric sensor in a thermal compression bonding process. Our piezoelectric sensor exhibits remarkable sensitivity (13.4 mV N−1), rapid response (8 ms), and exceptional stability over 2000 compression/release cycles, all at a negligibly low fabrication cost. We demonstrate that pulse wave, blink, and speech signals can be acquired by the sensor, proposing a single biomechanical modality to monitor multiple physiological traits associated with bipolar disorder. This ultralow-cost and mass-producible piezoelectric sensor paves the way for extensive long-term monitoring and immediate feedback for bipolar disorder management.
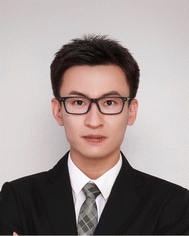 Benhui Hu | Benhui Hu is a Professor at Nanjing Medical University, China. He received his B.S. degree in science from Nanjing University (China), M.S. degree in polymer physics and chemistry from Chinese Academy of Science, and Ph.D. degree in materials science and engineering from Nanyang Technological University (Singapore). He has focused on biomechanical information perception and its related diagnosis and therapeutics. He also innovatively proposed an active-noise-reduction mechanism for decoding organ voiceprint. |
Introduction
Bipolar disorder is a multifaceted and severe chronic psychiatric disease, ranked 17th among all diseases globally,1 impacting over 1% of the global populace.2 Statistically, bipolar disorder patients face a twofold increased risk of mortality3 due to the elevated rates of suicide and physical ailments in this demographic,4,5 significantly impacting their mental and physical health, familial relationships, and societal development. Patients with bipolar disorder undergo intense emotional fluctuations, including recurrent episodes of mania and depression. During manic episodes, patients experience heightened emotional states characterized by intense euphoria and surges in blood pressure. Conversely, depressive episodes entail a contrasting experience marked by low moods, reduced energy levels, and heightened susceptibility to fatigue across various activities.6–9 Hence, long-term monitoring and intervention in this condition are imperative.10 Currently, diagnosing bipolar disorder relies heavily on verbal interviews and specific questionnaire assessments, lacking reliable and objective psychological indicators or biomarkers.11,12 Although auxiliary medical tools such as magnetic resonance imaging (MRI)13,14 and electroencephalographs15,16 are commonly used in psychiatric illness diagnosis, their high cost, bulkiness, and complexity restrict their use outside hospital settings.17,18 These limitations also prevent them from continuous, long-term monitoring of patients’ mental health conditions.
Recently, propelled by the urgent demand for advanced artificial intelligence and wearable medical devices, flexible wearable devices have attracted considerable attention due to their lightweight and portability.19–25 They enable continuous monitoring of physiological signals, thus supporting clinical decisions and enhancing the management of mental health disorders.26–29 For example, electroencephalogram (EEG) electrodes are developed for prolonged high-quality neurological state monitoring.30,31 Unfortunately, their reliance solely on electrophysiological signals is inaccurate due to the diversity of symptoms in psychiatric disorders. Since multiple physiological features can provide additional discriminative information,32 it is critical to integrate multiple devices into a single system. For instance, a wearable system integrating fabric electrodes and resistive sensors is designed to diagnose bipolar disorders, which poses a high accuracy of 95.81%.11 An adaptive method is also proposed to enhance classification accuracy by fusing multiple emotional modalities.33 Although these methods enhance accuracy by monitoring various physiological signals, their reliance on numerous devices, complex preparation, high cost, and intricate operation poses challenges for ensuring long-term user compliance.
Here, we demonstrate a thermal compression bonding piezoelectric sensor (TCBPS) based on poly(vinylidene fluoride) (PVDF)/zinc oxide (ZnO) nanofiber film, which is ultralow-cost and mass-producible. By analyzing the common symptoms of bipolar disorder, we identified three crucial physiological characteristics—blood pressure, fatigue level, and emotion—as key indicators for the long-term monitoring of bipolar disorder. By correlating and quantifying the above three physiological features with pulse wave, blink, and speech signals, we successfully proposed a single biomechanical modality for bipolar disorder monitoring. Specifically, we employed an electrospinning technique to prepare the PVDF/ZnO nanofiber film directly on a copper foil, thus forming a composite layer. Our TCBPS was constructed by a one-step integration method, i.e. combining two composite layers with fiber sides inwards and assembling them in a thermal compression bonding process. Straightforward fabrication processes and affordable and economical materials endow our TCBPS with negligible cost. Our TCBPS exhibits an excellent sensitivity of 13.4 mV N−1 and a rapid response time of 8 ms. It maintains stable voltage output over 2000 compression/release cycles without noticeable fatigue, allowing for sustainable monitoring of mechanical signals. As a whole, our TCBPS holds tremendous potential for accurate monitoring, long-term management, and timely intervention of bipolar disorder.
Experimental section
Materials
PVDF powder (Mw ≈ 534
000) was purchased from Sigma-Aldrich. ZnO nanoparticles (90 ± 10 nm) and N,N-dimethylformamide (DMF) were obtained from Aladdin Biochemical Technology Co., Ltd. Conductive copper foil was supplied by Kunshan Hanke's new material company. Acetone was purchased from Nanjing Chemical Reagent Co., Ltd.
Preparation of PVDF/ZnO nanofibers and fabrication of the TCBPS
The fabrication process of our TCBPS is as follows. ZnO nanoparticles were added in a 1
:
1 (wt/wt) DMF/acetone mixture solvent and ultrasonically dispersed for 3 h to obtain a mixed solution. Subsequently, PVDF powder was introduced into the mixed solution and stirred at 50 °C for 12 h for the spinning solution. Once the solution was loaded into the syringe, a 21G metal needle was applied to connect the syringe outlet. The syringe was then mounted on a syringe pump to provide a constant flow rate of 0.5 ml h−1. Electrospinning was carried out at a positive voltage of 15 kV with a negative voltage of −2 kV under a fixed distance of 15 cm between the tip of the spinner and the collector. A rotating drum covering a piece of copper foil operating at 100 rpm collected the electrospun fibrous film. The process was carried out with an electrospinning machine (ET-1, Beijing Yongkang Leye Technology Development Co., LTD). Following the preparation of the composite layers consisting of the copper foils and PVDF/ZnO nanofibers, the TCBPS was fabricated by stacking two composite layers with their fiber sides inwards and integrating in thermal compression bonding (JXY-SD160B, Dongguan Jinxinyang Mold Technology Co., LTD) at 100 °C for 2 min. Finally, the device was made to be 20 mm × 20 mm for the following experiments.
Characterization and measurements
The morphology of the nanofiber films with 0%, 2%, 5%, 7%, and 10% ZnO and that with 7% ZnO after thermal compression was observed on a scanning electron microscope (SEM, thermo scientific Apreo 2C). Elemental composition was assessed through energy-dispersive X-ray spectroscopy (EDS, Oxford Ultim Max65). X-ray diffraction (XRD) was performed on an Ultima IV diffractometer (Rigaku Corporation, Japan) to elucidate the crystalline phase structure of nanofibers. Fourier Transform Infrared Spectroscopy (FT-IR) investigations were conducted using a spectrometer (Thermo Fisher Nicolet Is5), covering a wavelength range of 4000 to 400 cm−1 with a spectral resolution of 4 cm−1. The mechanical property test of devices was performed on a Criterion Electromechanical Test System (C42.503, MTS). The output voltage was measured by PowerLab (PL3516, AD Instruments), which was interfaced with a computer using the LabChart program. Before measuring, we pre-tighten the skin in case skin laxity affects the electrical signals of our TCBPS.
Machine learning methods of speech emotion recognition
For the training process, the sound emotion classifier was trained with a batch size of 64 under one NVIDIA GeForce RTX 4090 GPU. The model programming utilizes an environment of Anaconda 3, Python 3.8, and Pytorch1.13.1_gpu. We used Adam as the model optimizer and set the learning rate to be 1 × 10−3.
Results and discussion
Wearable bioelectronic sensors can acquire different physiological signals by sensing deformation or vibration in different locations of the human skin (Fig. 1a). Our TCBPS is capable of collecting pulse wave, blink, and speech signals to quantify multiple physiological traits including blood pressure, fatigue level, and emotion. Fig. 1b illustrates the fabrication process for the TCBPS which comprises three layers, i.e., bottom copper foil electrode, PVDF/ZnO nanofiber film, and top copper foil electrode (Fig. 1c and d). PVDF/ZnO nanofiber films were prepared by electrospinning directly onto the copper foil. To streamline the preparation process and minimize costs, a one-step integration method was proposed to assemble our sensor, whereby two composite layers consisting of copper foils and PVDF/ZnO nanofiber films were stacked inwards and incorporated by thermal compression bonding. This process eliminates complicated procedures such as magnetron sputtering34 or spin coating.35 A photograph of 7 wt% PVDF/ZnO nanofiber film after thermal compression taken by metallographic microscope is shown in Fig. S1,† which displays fiber fusion. Fig. 1e illustrates the working mechanism of our TCBPS for detecting dynamic forces during repeated compression and release. The mechanical stretching, high-voltage electric field polarization, and synergistic effect of ZnO during the electrospinning process result in the alignment of PVDF molecular chains along the fiber axis. This promotes the in situ polarization of PVDF and the formation of more β-phases in PVDF. Due to the in situ polarization of PVDF, the dipoles in PVDF align inside the fibers and arrange along the thickness direction, establishing the total polarization within the fiber film.36 When pressure is repeatedly applied and released, the total dipole moment within the fiber film changes, resulting in a change in the total polarization. This change induces a flow of charge on the surface of the film, eventually generating an electrical signal.
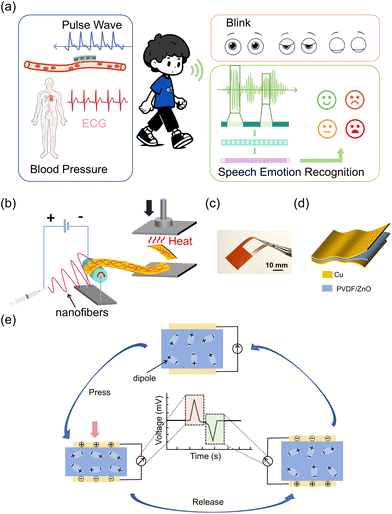 |
| Fig. 1 Applications and design of the TCBPS. (a) Diagram of the TCBPS on monitoring different physiological signals. (b) Schematic illustration of the fabrication process for the TCBPS. (c) A photograph of the TCBPS. (d) Schematic of the multilayer structure of the TCBPS. (e) The working mechanism of the TCBPS under compression and release. | |
The piezoelectric effect generated by pure PVDF is insufficient to monitor subtle pulse wave, blink, and speech signals. Consequently, we incorporated ZnO nanoparticles into PVDF to enhance its energy conversion efficiency.37 To confirm the presence of ZnO nanoparticles, SEM and EDS were utilized to observe the surface morphology of the electrospun PVDF/ZnO nanofiber films. As shown in Fig. 2a–f, we successfully fabricated nanofiber films with ZnO contents of 0%, 2%, 5%, 7%, and 10% (wt/wt), respectively. No nanoparticles are observed in the pure PVDF nanofiber film (Fig. 2a), whereas granular protrusions are observed in the films doped with ZnO nanoparticles, which indicates the encapsulation of ZnO nanoparticles within the fibers (Fig. 2e). This observation is also confirmed by the EDS images (Fig. 2g–j). Zinc (Fig. 2g) and oxygen (Fig. 2h) from ZnO are well-distributed throughout the PVDF matrix, without interfering with the existing dispersion of carbon (Fig. 2i) and fluorine (Fig. 2j) which are inherent in PVDF. The films with 7 wt% ZnO have a nearly 1
:
1 (wt%) ratio of zinc to oxygen (Table S1†), indicating the presence of ZnO nanoparticles in the films. To further verify the presence of ZnO, we characterized the crystalline phase structure of the PVDF/ZnO nanofiber films using the XRD technique. The XRD spectrum of pure PVDF consists of a characteristic peak of 18.3° for nonpolar α-phase and 20.6° for electroactive β-phase.38 As depicted in Fig. 2k, in the nanofiber films doped with ZnO nanoparticles, diffraction peaks at 2θ = 31.9°(100), 34.5°(002), 36.3°(101) correspond to the characteristic peaks of ZnO.39 The characteristic peaks increase as the ZnO doping ratio increases.
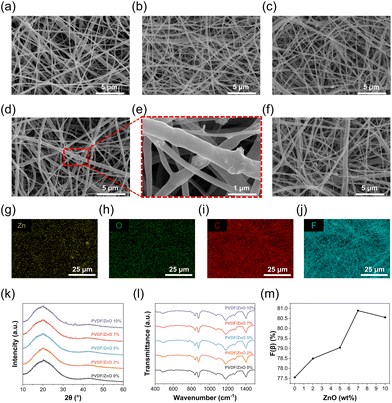 |
| Fig. 2 Characterization of PVDF/ZnO nanofiber films. The SEM images of PVDF/ZnO nanofibers with ZnO content of (a) 0 wt%, (b) 2 wt%, (c) 5 wt%, (d and e) 7 wt%, and (f) 10 wt%. The EDS images of PVDF/ZnO nanofibers: (g) Zn, (h) O, (i) C, and (j) F. (k) The XRD patterns of PVDF/ZnO nanofibers. (l) The FT-IR spectra of PVDF/ZnO nanofibers. (m) The calculated β-phase content of PVDF/ZnO nanofibers. | |
Moreover, the crystalline phase structure and chemical composition of PVDF/ZnO were analyzed by FT-IR spectroscopy to investigate the role of ZnO nanoparticles in forming the β-phase. Fig. 2l compares the FT-IR spectra of PVDF nanofiber films with different ZnO doping ratios. The vibrational peaks at 530 cm−1, 614 cm−1, 762 cm−1, 795 cm−1, and 975 cm−1 correspond to the α-phase, and the vibrational peaks at 510 cm−1, 840 cm−1 and 1279 cm−1 correspond to the β-phase (Fig. S2†).40,41 The fraction of the β-phase can be calculated by the following equation:42
where
Aα and
Aβ are the absorbance intensities at 762 cm
−1 and 840 cm
−1, respectively, which can be obtained by transmittance conversion of the FT-IR transmission curve.
Kα and
Kβ are absorption coefficients of 6.1 × 10
4 cm
2 mol
−1 and 7.7 × 10
4 cm
2 mol
−1 respectively. The resulting β-phase content was calculated to be 77.5%, 78.5%, 79.1%, 80.9%, and 80.5% at 0%, 2%, 5%, 7%, and 10% ZnO doping ratio, respectively (
Fig. 2m). The results show that the addition of ZnO nanoparticles can improve the content of β-phase in PVDF nanofibers. The role of ZnO in the formation of the β-phase in PVDF can be explained from two perspectives. On the one hand, there is an effective interaction between the CH
2 groups carrying positive charge density on the PVDF chain and the surface of ZnO nanoparticles with negative charge density. These negatively charged ZnO nanoparticles can act as β-phase nucleating agents.
41,43,44 On the other hand, during the electrospinning process, the addition of ZnO nanoparticles enhances the local electric field, which induces the formation of a greater quantity of β-phase crystals than pure PVDF.
45 Nevertheless, there is a decrease in the content of the β-phase when the ZnO addition is increased from 7 wt% to 10 wt%. The decrease in the content of the β-phase may be due to an increasing amount of ZnO detaching from the fibers during the electrospinning process and depositing on the fibers (Fig. S3
†) rather than being encapsulated by the fibers, leading to an ineffective induction of the β-phase formation.
46 In addition, it is reported that the nucleating effect of nanofillers is more pronounced at low levels since excessive nanofillers impede the movement of the polymer chains and the growth of crystals, thus hampering crystallization.
47 Consequently, excessive ZnO may affect the β-phase content by reducing the crystallinity of PVDF.
To investigate the electrical performance of our TCBPS, we used an electromechanical platform to simulate the external forces with different magnitudes (Fig. 3a). The electrical output of the TCBPS can be obtained by a multi-channel data acquisition system. To explore the effect of ZnO concentration on device sensitivity, we recorded the voltage response of our TCBPS under different forces at a frequency of 1 Hz. The output voltage of the TCBPS with different ratios of ZnO rises with the increase of force (Fig. 3b). Based on the linear slope of the curve in Fig. 3b, the sensitivity of the TCBPS can be calculated by the equation:
|  | (2) |
where Δ
F represents the relative change in applied force, and Δ
V represents the relative change in output voltage. The results show that the TCBPS with 7 wt% ZnO exhibits the highest sensitivity (13.4 mV N
−1), more than double that of a device made with pure PVDF (
S = 5.6 mV N
−1). It is also approximately 1.3 times higher than the sensor with 10 wt% ZnO (
S = 10.4 mV N
−1) due to the adverse effect of excess ZnO on the content of the β-phase. Therefore, TCBPS containing 7 wt% ZnO was chosen for further experimentation and application. The comparison of the sensitivity with sensors based on similar materials is shown in Table S2.
†Fig. 3c demonstrates the cyclic response of the 7 wt% ZnO TCBPS under different force magnitudes. By applying forces ranging from 5 N to 25 N repeatedly to the TCBPS, it maintains a high degree of consistency in its measurement signal at every magnitude, indicating its excellent reproducibility in cyclic compression measurements. Furthermore, conducting 2000 dynamic loading-unloading tests at 1 Hz and 5 N demonstrates minimal drift or hysteresis in the output voltage signal, verifying the mechanical durability of our TCBPS (
Fig. 3d). In addition to the sensitivity and durability, the response speed also plays an essential role in monitoring biomechanical signals. The response time of the sensor is defined as the time it takes for the voltage to rise from 10% to 90% (
Tr) or fall from 90% to 10% (
Tf) when pressure is applied.
48 Analysis of the voltage
versus time relationship reveals that both
Tr and
Tf are approximately 8 ms (
Fig. 3e). This fast response is attributed to the quick alignment or recovery of the dipoles in PVDF and the fast stress release of the nanofiber structure since there is an ample gap between the fibers to release the stress.
49 When the electrodes were connected in reverse, the overall polarization direction of the –CH
2/–CF
2 dipoles changed, causing a switch in the amplitude of the output voltage.
50 This phenomenon indicates that the measured output voltage is a genuine piezoelectric voltage, not generated by any instrumental artifacts (
Fig. 3f).
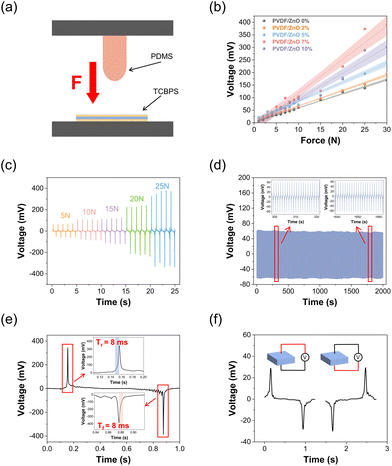 |
| Fig. 3 Electromechanical characterization of the TCBPS. (a) Diagram of the measurement platform. (b) Voltage versus force curves for the TCBPS with different ZnO contents when the force ranges from 1 to 30 N. (c) Robust response of voltage output of the TCBPS under different magnitudes of forces. (d) Cyclic stability test of the TCBPS over 2000 cycles under 1 Hz and 5 N. (e) Response time of the TCBPS at the frequency of 1 Hz. (f) Switching polarity test under forward and reverse connections. | |
Since manic episodes are accompanied by strong excitement and spikes in blood pressure, to assist in the auxiliary diagnosis and long-term management of bipolar disorder, our TCBPS is utilized to measure pulse wave signals and convert them into blood pressure. By attaching the TCBPS to the skin surface, the weak changes of arterial pulsations are captured. When the arteries pulse, the sensor generates corresponding voltage signals, which can be reconstructed into a pulse waveform through data collection and processing. Experiments were conducted in the head (temporal artery), neck (carotid artery), arm (brachial artery), wrist (radial artery), and ankle (dorsal pedal artery). The results show that our TCBPS can accurately measure pulse wave signals from various arteries, fully confirming its high sensitivity and broad applicability (Fig. 4a). By connecting two commercial Ag/AgCl electrodes to our TCBPS, it is feasible to calculate blood pressure from the time interval between the peak of the electrocardiogram (ECG) signal, R, and the peak of the pulse waveform, P1, during the same cardiac cycle, which is defined as the pulse transit time (PTT) (Fig. 4b). PTT signifies the speed of arterial pulse propagation between proximal and distal arteries, exhibiting a strong correlation with blood pressure. Diastolic blood pressure (DBP) and systolic blood pressure (SBP) are calculated according to the following equations:51
|  | (3) |
|  | (4) |
where SBP
0 and DBP
0 represent the measured values from commercial sphygmomanometers, used for calibration to initialize the monitoring sensor and convert the measured time delay into absolute blood pressure values. A stands for the subject-dependent coefficient, while PTT
0 denotes the initial PTT of the first recording cycle. The TCBPS was made to be 1 × 1 cm
2 in size to direct contact with the skin without being affected by the hair. For the monitoring of blood pressure, the TCBPS was affixed to the skin corresponding to the temporal artery (Fig. S4a
†), and the ECG electrodes were attached to both sides of the neck (Fig. S4b
†). Pulse wave signals (
Fig. 4c and f) and ECG signals (
Fig. 4d and g) were recorded before and after exercise. Calculated diastolic and systolic blood pressure values exhibited significant changes pre- and post-exercise and closely matched the readings from the commercial sphygmomanometer (
Fig. 4e and h). This non-invasively and continuous monitoring of blood pressure helps patients with bipolar disorder manage their cardiovascular health and provides personalized treatment for them.
52
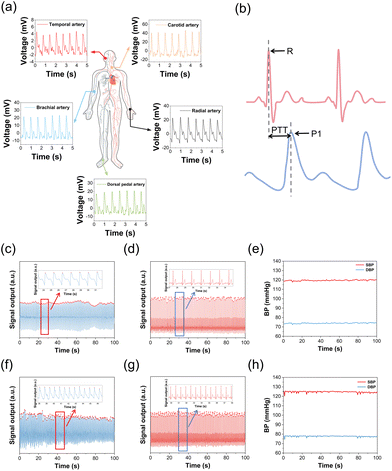 |
| Fig. 4 Arterial pulse wave detection and blood pressure measurement using our TCBPS. (a) Pulse wave signals detected from different arteries, including temporal, carotid, brachial, radial, and dorsal pedal arteries. (b) Definition of pulse transit time. (c) Temporal artery pulse waveforms recorded by the TCBPS, (d) ECG signals recorded by the commercial Ag/AgCl electrodes, and (e) Calculated DBP and SBP before exercise. (f) Temporal artery pulse waveforms recorded by the TCBPS, (g) ECG signals recorded by the commercial Ag/AgCl electrodes, and (h) Calculated DBP and SBP after exercise. | |
Given that the variance in blood pressure between depressive episodes and normal conditions is minimal,53,54 we detect blink frequency for fatigue assessment, thereby enhancing the accuracy of depressive episode recognition.55–57 The TCBPS is capable of capturing the deformation of eye muscles during intermittent blinks and continuous blinks. By affixing the TCBPS to three locations around the left eye (Fig. 5a), intermittent blinks (Fig. 5c, e and g) and continuous blinks (Fig. 5d, f and h) signals are captured. During blinking, contractions or relaxations of the orbicularis oculi and other eye muscles cause the sensor to bend, generating a voltage signal (Fig. 5b). During intermittent blinks, the average output voltage for eye closure and opening was 94.7 and −53.9 mV, respectively, when the TCBPS was affixed to the upper eyelid (Fig. 5c), 9.7 and −4.2 mV to the temporal skin (Fig. 5e), and 46.6 and −21.1 mV to the lower eyelid (Fig. 5g). As the upper eyelid exhibits the most movement during blinking, followed by the lower eyelid and then the temporal skin, the voltage changes follow this pattern,58 demonstrating that our TCBPS can capture blink signals with high accuracy. With increasing blink frequency (>3 Hz), it is observed that the TCBPS could record the voltage output at each blink without signal loss, which is due to the quick response of our sensor. By measuring eye blink signals, our TCBPS permits an effective assessment of the fatigue level, which is one of the most prominent symptoms in the depressive phase of bipolar disorder patients.59 Compared to measuring eye blinks through face recognition, the TCBPS affixed around the eye is free from factors such as ambient light and facial occlusion, ensuring the accuracy of the data.60
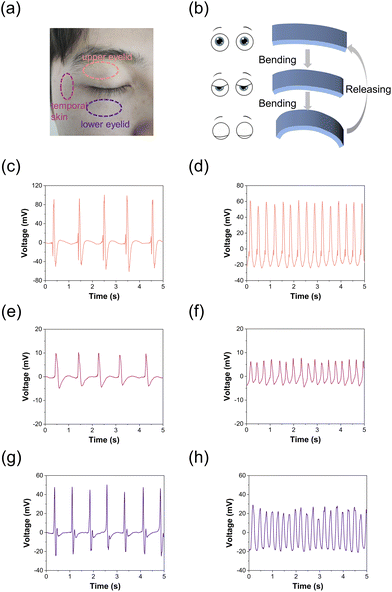 |
| Fig. 5 Detection of eye blink signals. (a) Photograph of a face showing 3 sensing locations (upper eyelid, temporal skin, and lower eyelid) for eye blink measurement. (b) Illustration of TCBPS bending and releasing motion. Voltage output of intermittent blinks and continuous blinks on (c and d) upper eyelid, (e and f) temporal skin, and (g and h) lower eyelid. | |
Emotion, a crucial indicator for swiftly assessing a patient's mental state,61,62 can be discerned through the detection and analysis of speech signals which has been widely used in mental health as a non-invasive and efficient method.63–65 Hence, it is a promising method for detecting bipolar disorder by speech signals. To evaluate the acoustic performance of our TCBPS, we affixed the sensor to a loudspeaker, as shown in Fig. 6a. This setup allows us to convert mechanical energy generated by sound vibration into an electrical signal. At a frequency of 300 Hz, as the sound pressure level (SPL) increased from 75 dB to 110 dB, the corresponding output voltage of our TCBPS escalated from 7 mV to 284 mV (Fig. 6b). Notably, within the range of 100 to 110 dB, the voltage output displayed a rapid increase proportional to the SPL. This substantial surge in voltage output resulted from amplified deformation and vibration. With increased SPL, the surface strain of the material expanded, leading to more energy conversion into resonant mechanical energy within the material. Moreover, the augmentation in resonance amplitude contributed significantly to the alteration in dipole moment.66Fig. 6c depicts the relationship between the voltage output and the sound frequency. The TCBPS exhibited heightened sensitivity to low-frequency sounds within the frequency range of 100 to 2000 Hz, with the maximum electrical output observed near 300 Hz. Additionally, when subjected to continuous 300 Hz and 95 dB sound stimuli, the output voltage experienced negligible decay within a span of 2 h (Fig. 6d). We placed the TCBPS against the larynx (Fig. S5†) to record the voltage signal when a “nanoscale” was read. Compared to the microphone, the sensor exhibits a higher frequency and temporal resolution (Fig. S6†).
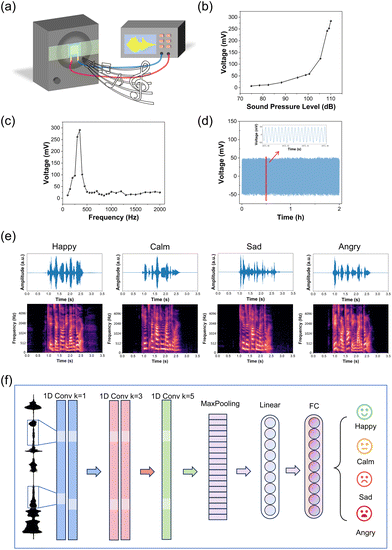 |
| Fig. 6 Acoustoelectric conversion characteristics of the TCBPS and speech emotion recognition. (a) Schematic diagram of the TCBPS placed on a loudspeaker to monitor the sound vibration. (b) Voltage output as a function of different SPL. (c) Responses of the TCBPS under different frequencies. (d) The voltage output of the TCBPS under long-term sound wave driving. (e) The speech signal and Mel-spectrograms of four emotions: happy, calm, sad, and angry. (f) The diagram of the emotion recognition model. | |
On this basis, we successfully recognized emotions using speech signals captured by our TCBPS. The speech data from the CASIA (the Chinese Academy of Sciences’ Institute of Automation) Chinese affective corpus was used as the sound source to preserve emotional accuracy and avoid introducing personal bias, and the sound was sent to the TCBPS through the loudspeaker. We have recorded 60 audio vibration signals using the TCBPS with a sampling frequency of 20 kHz. Each was manually classified and tagged with 4 emotion labels: happy, calm, sad, and angry, respectively. The ratio of the training set to the testing set was 8
:
2 in this study. Before training, the raw vibration data defined by 4 emotion expressions, 15 for each and lasting for approximately 1 second, were pre-processed by a band-pass filter to eliminate the extremely low or high-frequency signals. The speech signal was transferred into the frequency-domine Mel-spectrogram for better feature capture and emotion classification. The filtered signals were first cut into small frames by a Hann window with a window size of 1024 milliseconds and a hop length of 320 milliseconds. Then, the acoustic frames went through a Fast Fourier Transform (FFT) and an 80-band Mel-filter to transform from time-domain waveform into frequency-domain Mel-spectrograms, as seen in Fig. 6e. The 80 channels Mel-spectrogram were directly sent into our convolutional neural network (CNN) classifier for emotion discrimination. The classifier is a CNN-based structure and consists mainly of a 5-layer CNN, each with a kernel size of [1, 1, 3, 3, 5]. The different kernel sizes for a set of CNNs help the model deeply and widely capture the latent features from the input speech signal. Then, the hidden states went through a MaxPooling layer that helps reduce the computational cost and variance. Following the MaxPooling layer are the linear and fully connected layers, which are responsible for an emotion projection as a final classification result. The detailed schematic diagram of the model can be found in Fig. 6f. The accuracy for 4 emotion classification achieves 75% in total based on the input vibration signal collected from the proposed sensor (Table S3†). For patients with bipolar disorder, the manic or depressive phase lasts for several days. For instance, most of the time patients in the manic phase have their moods discriminated as happy or angry, and only a few times are they discriminated as sad or calm. Statistics and analysis of characteristics such as the frequency of mood swings, the occurrence frequency and duration of different moods can be beneficial in the diagnosis of bipolar disorder.67
Conclusions
In summary, we present an ultralow-cost, mass-productive piezoelectric sensor by integrating PVDF/ZnO nanofiber film and two copper foils in a thermal compression bonding process. Incorporating ZnO into PVDF nanofibers significantly enhances the energy conversion efficiency of the TCBPS, showcasing a superior sensitivity of 13.4 mV N−1. Furthermore, our TCBPS exhibits a quick response time of ∼8 ms and sustained stability over 2000 cycles. Best of all, it harvests the benefits in terms of ultralow cost and ease of manufacture. We collected pulse wave, blink, and speech signals, and applied them for blood pressure calculation, fatigue assessment, and emotion recognition, respectively. Accordingly, we proposed a unified biomechanical model proficient in monitoring physiological traits associated with bipolar disorder. This cost-effective and mass-producible piezoelectric sensor holds tremendous potential for long-term monitoring and real-time feedback in bipolar disorder. Its affordability, accuracy, and reliability make it ideal for large-scale application among patient populations, thereby shedding new light on the possibilities for refined monitoring and diagnosis.
Author contributions
X.D.S. and Z.N.C. conceived the idea, fabricated and characterized the device, prepared the figures, wrote the manuscript, and revised the manuscript. J.X.Y. wrote the manuscript. F.Z.L. and S.S.C. revised the manuscript. J.F.X., Y.H.Y., and B.L. assisted with part of the measurements. Q.J. and P.Y. analyzed and discussed the results. B.H.H. proposed the concept and supervised this study. All authors discussed the results and commented on the manuscript.
Conflicts of interest
There are no conflicts of interest to declare.
Acknowledgements
This work was supported by the National Natural Science Foundation of China (No. 81971701), the National Key Research and Development Program of China (No. 2019YFA0210104 and 2023YFC2410500), and the Fourteenth Research Institute of China Electronics Technology Group Corporation (2022KF0195).
References
- D. Vigo, G. Thornicroft and R. Atun, Lancet Psychiatry, 2016, 3, 171–178 CrossRef PubMed.
- I. Grande, M. Berk, B. Birmaher and E. Vieta, Lancet, 2016, 387, 1561–1572 CrossRef PubMed.
- E. R. Walker, R. E. McGee and B. G. Druss, JAMA Psychiatry, 2015, 72, 334–341 CrossRef PubMed.
- J. F. Hayes, J. Miles, K. Walters, M. King and D. P. J. Osborn, Acta Psychiatr. Scand., 2015, 131, 417–425 CrossRef CAS PubMed.
- L. V. Kessing, E. Vradi, R. S. McIntyre and P. K. Andersen, J. Affective Disord., 2015, 180, 142–147 CrossRef PubMed.
- A. F. Carvalho, J. Firth and E. Vieta, N. Engl. J. Med., 2020, 383, 58–66 CrossRef CAS PubMed.
- R. S. McIntyre, M. Berk, E. Brietzke, B. I. Goldstein, C. López-Jaramillo, L. V. Kessing, G. S. Malhi, A. A. Nierenberg, J. D. Rosenblat, A. Majeed, E. Vieta, M. Vinberg, A. H. Young and R. B. Mansur, Lancet, 2020, 396, 1841–1856 CrossRef CAS PubMed.
- D. V. Skjelstad, U. F. Malt and A. Holte, J. Affective Disord., 2010, 126, 1–13 CrossRef PubMed.
- R. S. McIntyre, M. Alda, R. J. Baldessarini, M. Bauer, M. Berk, C. U. Correll, A. Fagiolini, K. Fountoulakis, M. A. Frye, H. Grunze, L. V. Kessing, D. J. Miklowitz, G. Parker, R. M. Post, A. C. Swann, T. Suppes, E. Vieta, A. Young and M. Maj, World Psychiatry, 2022, 21, 364–387 CrossRef PubMed.
- J. Firth, N. Siddiqi, A. Koyanagi, D. Siskind, S. Rosenbaum, C. Galletly, S. Allan, C. Caneo, R. Carney, A. F. Carvalho, M. L. Chatterton, C. U. Correll, J. Curtis, F. Gaughran, A. Heald, E. Hoare, S. E. Jackson, S. Kisely, K. Lovell, M. Maj, P. D. McGorry, C. Mihalopoulos, H. Myles, B. O'Donoghue, T. Pillinger, J. Sarris, F. B. Schuch, D. Shiers, L. Smith, M. Solmi, S. Suetani, J. Taylor, S. B. Teasdale, G. Thornicroft, J. Torous, T. Usherwood, D. Vancampfort, N. Veronese, P. B. Ward, A. R. Yung, E. Killackey and B. Stubbs, Lancet Psychiatry, 2019, 6, 675–712 CrossRef PubMed.
- G. Valenza, M. Nardelli, A. Lanatà, C. Gentili, G. Bertschy, R. Paradiso and E. P. Scilingo, IEEE J. Biomed. Health Inf., 2014, 18, 1625–1635 Search PubMed.
- Z. Jan, N. Ai-Ansari, O. Mousa, A. Abd-alrazaq, A. Ahmed, T. Alam and M. Househ, J. Med. Internet Res., 2021, 23, e29749 CrossRef PubMed.
- W. Yan, M. Zhao, Z. Fu, G. D. Pearlson, J. Sui and V. D. Calhoun, Schizophr. Res., 2022, 245, 141–150 CrossRef PubMed.
- J. A. Frazier, S. Chiu, J. L. Breeze, N. Makris, N. Lange, D. N. Kennedy, M. R. Herbert, E. K. Bent, V. K. Koneru, M. E. Dieterich, S. M. Hodge, S. L. Rauch, P. E. Grant, B. M. Cohen, L. J. Seidman, V. S. Caviness and J. Biederman, Am. J. Psychiatry, 2005, 162, 1256–1265 CrossRef PubMed.
- F. M. Howells, H. S. Temmingh, J. H. Hsieh, A. V. Van Dijen, D. S. Baldwin and D. J. Stein, Transl. Psychiatry, 2018, 8, 1–11 CrossRef PubMed.
- Y. Lei, A. N. Belkacem, X. Wang, S. Sha, C. Wang and C. Chen, Biomed. Signal Process. Control, 2022, 72, 103370 CrossRef.
- M. S. Bhamla, B. Benson, C. Chai, G. Katsikis, A. Johri and M. Prakash, Nat. Biomed. Eng., 2017, 1, 1–7 CrossRef.
- K. Nan, S. Babaee, W. W. Chan, J. L. P. Kuosmanen, V. R. Feig, Y. Luo, S. S. Srinivasan, C. M. Patterson, A. M. Jebran and G. Traverso, Nat. Biomed. Eng., 2022, 6, 1092–1104 CrossRef CAS PubMed.
- Z. Ma, Q. Huang, Q. Xu, Q. Zhuang, X. Zhao, Y. Yang, H. Qiu, Z. Yang, C. Wang, Y. Chai and Z. Zheng, Nat. Mater., 2021, 20, 859–868 CrossRef CAS PubMed.
- T. Zhao, Y. Fu, C. Sun, X. Zhao, C. Jiao, A. Du, Q. Wang, Y. Mao and B. Liu, Biosens. Bioelectron., 2022, 205, 114115 CrossRef CAS PubMed.
- H. Cho, I. Lee, J. Jang, J. H. Kim, H. Lee, S. Park and G. Wang, Nat. Electron., 2023, 6, 619–629 CrossRef.
- X. Ren, Y. Zhou, F. Lu, L. Zhai, H. Wu, Z. Chen, C. Wang, X. Zhu, Y. Xie, P. Cai, J. Xu, X. Tang, J. Li, J. Yao, Q. Jiang and B. Hu, ACS Sens., 2023, 8, 2691–2701 CrossRef CAS PubMed.
- W. Tang, Y. Zhou, S. Chen, S. Yu, Y. Yang, J. Lin, S. Yin, Y. Ma and B. Hu, ACS Mater. Lett., 2021, 3, 1385–1393 CrossRef CAS.
- S. Chen, X. Ren, J. Xu, Y. Yuan, J. Shi, H. Ling, Y. Yang, W. Tang, F. Lu, X. Kong and B. Hu, ACS Nano, 2023, 17, 2134–2147 CrossRef CAS PubMed.
- Y. Yang, W. Tang, J. Wang, R. Liu, P. Yang, S. Chen, Y. Yuan, J. Xu, X. Ren, S. Yu, H. Wu, Y. Zhou, L. Zhai, X. Shao, Z. Chen and B. Hu, Sci. China Mater., 2022, 65, 2289–2297 CrossRef CAS.
- A. H. Anwer, N. Khan, M. Z. Ansari, S.-S. Baek, H. Yi, S. Kim, S. M. Noh and C. Jeong, Sensors, 2022, 22, 4460 CrossRef CAS PubMed.
- N. Long, Y. Lei, L. Peng, P. Xu and P. Mao, Math. Biosci. Eng., 2022, 19, 7899–7919 Search PubMed.
- S. Gedam and S. Paul, IEEE Access, 2021, 9, 84045–84066 Search PubMed.
- S. Yasin, S. A. Hussain, S. Aslan, I. Raza, M. Muzammel and A. Othmani, Comput. Methods Programs Biomed., 2021, 202, 106007 CrossRef PubMed.
- S. Lin, J. Liu, W. Li, D. Wang, Y. Huang, C. Jia, Z. Li, M. Murtaza, H. Wang, J. Song, Z. Liu, K. Huang, D. Zu, M. Lei, B. Hong and H. Wu, Nano Lett., 2019, 19, 6853–6861 CrossRef CAS PubMed.
- C. Wang, H. Wang, B. Wang, H. Miyata, Y. Wang, M. O. G. Nayeem, J. J. Kim, S. Lee, T. Yokota, H. Onodera and T. Someya, Sci. Adv., 2022, 8, eabo1396 CrossRef CAS PubMed.
- W. Lin and C. Li, Appl. Sci., 2023, 13, 2573 CrossRef CAS.
- M. Khezri, M. Firoozabadi and A. R. Sharafat, Comput. Methods Programs Biomed., 2015, 122, 149–164 CrossRef PubMed.
- Z. Liu, S. Zhang, Y. M. Jin, H. Ouyang, Y. Zou, X. X. Wang, L. X. Xie and Z. Li, Semicond. Sci. Technol., 2017, 32, 064004 CrossRef.
- T. Yang, H. Pan, G. Tian, B. Zhang, D. Xiong, Y. Gao, C. Yan, X. Chu, N. Chen, S. Zhong, L. Zhang, W. Deng and W. Yang, Nano Energy, 2020, 72, 104706 CrossRef.
- S. Mirjalali, A. M. Varposhti, S. Abrishami, R. Bagherzadeh, M. Asadnia, S. Huang, S. Peng, C. H. Wang and S. Wu, Macromol. Mater. Eng., 2023, 308, 2200442 CrossRef CAS.
- L. Lu, W. Ding, J. Liu and B. Yang, Nano Energy, 2020, 78, 105251 CrossRef CAS.
- H. Shao, J. Fang, H. Wang, C. Lang and T. Lin, ACS Appl. Mater. Interfaces, 2015, 7, 22551–22557 CrossRef CAS PubMed.
- P. Thakur, A. Kool, N. A. Hoque, B. Bagchi, F. Khatun, P. Biswas, D. Brahma, S. Roy, S. Banerjee and S. Das, Nano Energy, 2018, 44, 456–467 CrossRef CAS.
- A. Salimi and A. A. Yousefi, Polym. Test., 2003, 22, 699–704 CrossRef CAS.
- Md. M. Alam, A. Sultana and D. Mandal, ACS Appl. Energy Mater., 2018, 1, 3103–3112 CrossRef CAS.
- B. Mahanty, S. K. Ghosh, S. Jana, K. Roy, S. Sarkar and D. Mandal, Sustainable Energy Fuels, 2021, 5, 1003–1013 RSC.
- D. Mandal, K. Henkel and D. Schmeißer, Phys. Chem. Chem. Phys., 2014, 16, 10403–10407 RSC.
- C. M. Wu and M. H. Chou, eXPRESS Polym. Lett., 2019, 14, 103–114 CrossRef.
- Y. Wu, S. L. Hsu, C. Honeker, D. J. Bravet and D. S. Williams, J. Phys. Chem. B, 2012, 116, 7379–7388 CrossRef CAS PubMed.
- M. Kim and J. Fan, Adv. Fiber Mater., 2021, 3, 160–171 CrossRef CAS.
- S. C. Tjong, Mater. Sci. Eng., R, 2006, 53, 73–197 CrossRef.
- W. Deng, T. Yang, L. Jin, C. Yan, H. Huang, X. Chu, Z. Wang, D. Xiong, G. Tian, Y. Gao, H. Zhang and W. Yang, Nano Energy, 2019, 55, 516–525 CrossRef CAS.
- G. Tian, W. Deng, Y. Gao, D. Xiong, C. Yan, X. He, T. Yang, L. Jin, X. Chu, H. Zhang, W. Yan and W. Yang, Nano Energy, 2019, 59, 574–581 CrossRef CAS.
- K. Maity, S. Garain, K. Henkel, D. Schmeißer and D. Mandal, ACS Appl. Polym. Mater., 2020, 2, 862–878 CrossRef.
- Y. Xu, B. Sun, Y. Ling, Q. Fei, Z. Chen, X. Li, P. Guo, N. Jeon, S. Goswami, Y. Liao, S. Ding, Q. Yu, J. Lin, G. Huang and Z. Yan, Proc. Natl. Acad. Sci. U. S. A., 2020, 117, 205–213 CrossRef CAS PubMed.
- N. M. McGowan, M. Nichols, A. C. Bilderbeck, G. M. Goodwin and K. E. A. Saunders, Int. J. Bipolar Disord., 2021, 9, 5 CrossRef CAS PubMed.
- S. Paterniti, A. Alperovitch, P. Ducimetiere, M.-J. Dealberto, J.-P. Lepine and J. C. Bisserbe, Psychosom. Med., 1999, 61, 77 CrossRef CAS PubMed.
- M. Wiehe, S. C. Fuchs, L. B. Moreira, R. S. Moraes, G. M. Pereira, M. Gus and F. D. Fuchs, J. Hum. Hypertens., 2006, 20, 434–439 CAS.
- P. Ferentinos, V. Kontaxakis, B. Havaki-Kontaxaki, D. Dikeos and G. Papadimitriou, Psychiatry Res., 2010, 177, 114–119 CrossRef PubMed.
- M. Fava, S. Ball, J. C. Nelson, J. Sparks, T. Konechnik, P. Classi, S. Dube and M. E. Thase, Depression Anxiety, 2014, 31, 250–257 CrossRef PubMed.
- K.-F. Chung, B. Y.-M. Yu, K.-P. Yung, W.-F. Yeung, T. H. Ng and F. Y.-Y. Ho, Compr. Psychiatry, 2014, 55, 1671–1678 CrossRef PubMed.
- N. I. Kim, J. Chen, W. Wang, M. Moradnia, S. Pouladi, M.-K. Kwon, J.-Y. Kim, X. Li and J.-H. Ryou, Adv. Funct. Mater., 2021, 31, 2008242 CrossRef CAS.
- M. J. Weintraub, C. D. Schneck and D. J. Miklowitz, Bipolar Disord., 2020, 22, 128–138 CrossRef PubMed.
- J. W. Bang, H. Heo, J. S. Choi and K. R. Park, Sensors, 2014, 14, 16467–16485 CrossRef PubMed.
- J. Townsend and L. L. Altshuler, Bipolar Disord., 2012, 14, 326–339 CrossRef PubMed.
- C. Henry, M. Phillips, E. Leibenluft, K. M'Bailara, J. Houenou and M. Leboyer, Front. Biosci., Elite Ed., 2012, 4, 2722–2730 Search PubMed.
- M. El Ayadi, M. S. Kamel and F. Karray, Pattern Recognit., 2011, 44, 572–587 CrossRef.
- B. W. Schuller, Commun. ACM, 2018, 61, 90–99 CrossRef.
- D. Li, Y. Zhou, Z. Wang and D. Gao, Inf. Sci., 2021, 548, 328–343 CrossRef.
- Z. Yu, Y. Zhang, Y. Wang, J. Zheng, Y. Fu, D. Chen, G. Wang, J. Cui, S. Yu, L. Zheng, H. Zhou and D. Li, Nano Energy, 2022, 97, 107205 CrossRef CAS.
- C. Henry, D. Van den Bulke, F. Bellivier, I. Roy, J. Swendsen, K. M'Baïlara, L. J. Siever and M. Leboyer, Psychiatry Res., 2008, 159, 1–6 CrossRef PubMed.
|
This journal is © The Royal Society of Chemistry 2024 |
Click here to see how this site uses Cookies. View our privacy policy here.