DOI:
10.1039/D4RA03965C
(Paper)
RSC Adv., 2024,
14, 26897-26910
Artificial intelligence-aiding lab-on-a-chip workforce designed oral [3.1.0] bi and [4.2.0] tricyclic catalytic interceptors inhibiting multiple SARS-CoV-2 protomers assisted by double-shell deep learning†
Received
29th May 2024
, Accepted 20th August 2024
First published on 27th August 2024
Abstract
While each massive pandemic has claimed the lives of millions of vulnerable populations over the centuries, one limitation exists: that the Edisonian approach (human-directed with trial errors) relies on repurposing pharmaceuticals, designing drugs, and herbal remedies with the violation of Lipinski's rule of five druglikeness. It may lead to adverse health effects with long-term health multimorbidity. Nevertheless, declining birth rates and aging populations will likely cause a shift in society due to a shortage of a scientific workforce to defend against the next pandemic incursion. The challenge of combating the ongoing post-COVID-19 pandemic has been exacerbated by the lack of gold standard drugs to deactivate multiple SARS-CoV-2 protein targets. Meanwhile, there are three FDA-approved antivirals, Remdesivir, Molnupiravir, and Paxlovid, with moderate clinical efficacy and drug resistance. There is a pressing need for additional antivirals and prepared omics technology to combat the current and future devastating coronavirus pandemics. While there is a limitation of existing contemporary inhibitors to deactivate viral RNA replication with minimal rotational bonds, one strategy is to create Lipinski inhibitors with less than 10 rotational bonds and precise halogen bond placement to destabilize multiple viral protomers. This work describes the efforts to design gold-standard oral inhibitors of bi- and tri-cyclic catalytic interceptors with electrophilic heads using double-shell deep learning. Here, KS1 with and KS2 compounds designed by lab-on-a-chip technology attain 5-fold novel filtered-Lipinski, GHOSE, VEBER, EGAN, and MUEGGE druglikeness. The graph neural network (GNN) relies on module-initiation, expansion, relabeling atom index, and termination (METORITE) iterations, while the deep neural network (DNN) engages pinning, extraction, convolution, pooling, and flattening (PROOF) operations. The cyclic compound's specific halogen atom location enhances the nitrile catalytic head, which deactivates several viral protein targets. Initiating this lab-on-a-chip that is not susceptible to the aging process for creating clinical compounds can leverage a new path to many valuable drugs with speedy oral drug discovery, especially to defend the loss of vulnerable population and prevent multimorbidity that is susceptible to hidden viral persistence in the continuing aging times.
Introduction
History of pandemics has shown that humans and diseases have coevolved. Environmental changes speed up this process, and immunization is just one aspect of the effort. As aging times progress, slower workforce growth and aging populations may make it more challenges to defend against any pandemic catastrophes.1 In the beginning of the aging era, the severe acute respiratory syndrome coronavirus 2 (SARS-CoV-2) has caused the worst health crisis in the century, with about 7 million deaths and 776 cumulative infected million cases by the year 2024.2 Despite the recent development of mRNA vaccination, SARS-CoV-2 still remains a serious health concern, as the durability of the exploited vaccines is currently unknown. While a portion of the population chose not to get vaccinated due to religious reasons and concerns about long-term side effects, antivirals are significant alternatives to vaccines to combat both the current post-COVID-19 pandemic and future coronavirus outbreaks.3,4 The mutation occurrence that rules SARS-CoV-2's transmissibility and pathogenicity, the etiological agent of COVID-19 disease, makes the antiviral compounds that have been found so far susceptible to the strong viral response to treatment resistance.5 The post-COVID-19 condition Long COVID leads to permanent functional health impairment, and low productivity at work that can lead to economic burden.6 A persistent viral presence in the body following an infection is one assumption on the underlying etiology of Long COVID, as evidenced by traces of viral flotsam, RNA, and protein fragments found in the respiratory tract, blood, and extracellular vesicles of long-term COVID survivors. In living human beings, it is a difficult task to access deeper tissues to investigate viral particles with RNA replication.7 Particularly postulated peptidomimetic drugs have the obvious limitation of having larger molecular weight in translocation in a human host to degrade the viral RNA pockets.3 First tracked down in late 2023, the SARS-CoV-2 BA.2.86 (JN.1 variant) differs phylogenetically from the circulating SARS-CoV-2 omicron XBB lineages, such as EG.5.1 and HK.3. This SARS-CoV-2 JN.1 variant has a higher potential for immune evasion than XBB and BA.2 since it has more than 30 mutations in the spike protein with fastest-growing in the USA.8
The important cyclic structures have stood as inspiring targets for pharmaceutical drugs, such as anti-malarial mefloquine, cholesterol-lowering drug Lomitapide, heterocyclic carbenes, and epoxide derivatives.9–12 Despite their structural diversity and capability, it is unexpected that the bi-, tri-cyclic, medium-sized rings have been poorly represented in commercial drugs and rarely found in the library screening of drug discovery. Even the FDA-approved Paxlovid, which treats mild-to-moderate COVID-19, combines nirmatrelvir with the enhancer ritonavir. However, subsequent research revealed that nirmatrelvir's treatment resistance is caused by naturally occurring changes in the viral primary protease.13
Evidently, SAR-CoV-2 genome spans more than 30 kilobases, and open reading frame 1a (Orf1ab) encodes 16 predicted non-structural proteins (Nsps) and four primary coronavirus structural proteins. The 5′ Orf1ab gene-encoded polyproteins are auto-proteolytically processed to yield 16 Nsps, which come together to form a replicase–transcriptase complex (RTC). This RTC includes multiple enzymes: the papain-like protease (PLpro), adenosine diphosphate ribose monophosphatase (ADRP) (Nsp3), main protease (Mpro) (Nsp5), ribonucleic acid (RNA)-replicase (Nsp9), primary RNA-dependent RNA polymerase (RdRp) (Nsp12), helicase-triphosphatase (Nsp13), exoribonuclease (Nsp14), endoribonuclease (Nsp15) and 2′O-methyltransferases (Nsp10/Nsp16).14 SAR-CoV-2 infects cells via either direct plasma membrane fusion or endocytosis, depending on the degree of TMPRSS2 expression. The host ribosome translates the single-stranded positive-sense RNA to create the viral polyproteins pp1a and pp1ab, which are then cleaved by PLpro and Mpro to yield non-structural proteins (Nsps). The replication transcript complex, which is made of the Nsps, is responsible for mediating the replication of genomic and sub-genomic RNAs.15
Nevertheless, process of finding a new drug candidate is long and expensive, with human-directed trial errors. The common timeline for novel drug discovery with traditional approaches usually takes years to decades, and the repurposing of existing non-Lipinski drugs could lead to health impairment for COVID-19 survivors. Hence, techniques that rapidly determine and expedite the high-throughput screening process for new prospective candidates have the potential to significantly expand the chemical space of pharmacological modulators, thereby allowing speedy therapeutic options with minimized harmful effects.16 At every stage of the drug discovery process, choosing new compounds for synthesis and testing poses a substantial challenge. Finding the best candidates from the large pool of possible compounds can assist to cut down on the time and expense associated with resource-intensive testing and eventually result in the development of more potent therapies.17 The development of computational omics technology has opened up new directions for exploring the undiscovered diversity of natural products and for exploring their potential as novel sources of drugs.18 Although the field of structure-based virtual screening is experiencing significant growth and presents new prospects, it is also facing limitations due to the continuous expansion of databases containing contemporary chemical medicines. In parallel, in-chip-with and without artificial intelligence (AI) approaches have created the development of lab-on-a-chip technology by evaluating flown digital data in real-time as well as storing digitized tools.19–27 For instance, AI was integrated to determine kidney diseases,20 operate immunodiagnostics,28 perform electronic nose,29 and more using non-invasive lab-on-a-chips.30 Remarkably, the AI-on-a-chip technology has established a potent anti-multimorbidity platform for assembling AI algorithms in a large-scale, cost-effective, high-throughput, and multiplexed approach, thereby posing as a candidate technology for overcoming the above challenges.20,31
A portion of the recently developed deep learning using multi-level DGCNN has been used to segment the coal mine point cloud against the backdrop of the carbon peak. This creates the groundwork for understanding the subterranean environment and achieving low-carbon development.32 Furthermore, the invention of deep learning with different generation model has made it possible to identify the intrusion of foreign items into transmission lines.33 While considerable deep learning efforts focus on the virtual screening of billions of existing drugs in the chemical database as the generative adversarial networks (GAN) of discriminators, very limited research has put an effort into using deep learning as the GAN of generators.34,35 In addition, most of lab-on-a-chip technologies have been used for disease diagnostics and are very limited for other directions.20,21 The important direction is to complement aging workforce to combat potential pandemics. Here, the article described a first use of dual-core lab-on-a-chip technology and the GAN approach to create SARS-CoV-2 inhibitors using double deep learning shells in the artificial intelligence environment, which can lead to an acceleration of drug discovery.
Materials and methodology
Electronics, chemicals, and materials
The STM8S003K3T6C microprocessors, LCD display, electronic capacitors, resistors were purchased from Mousser Electronics, USA. Parylene D was sourced from KISCO CO., LTD, USA. Pyralux copper-clad laminate sheet was acquired from Dupont Ltd, USA. The reagents used for wearable fabrication were of the analytical grade and were used directly without further purification.
Assembly of dual-core lab-on-a-chip for SENSE process
The Pyralux copper-clad laminate sheet was washed with isopropyl alcohol and dried using compressed air flow. Later, it was spin-coated (2000 rpm for 30 s) with 15 wt% parylene D in dimethylformamide (DMF) onto the top side of the Pyralux Kapton sheet and heated at 120 °C in an oven for 5 minutes. Then the as-prepared sheet was treated with 2 min of O2 plasma to enhance surface hydrophilicity and remove unexpected dirt. Subsequently, MCU modules were pasted to enable communication for the finding of SARS-CoV-2 inhibitors and regulate machine learning circuits. Integrated processors can run multiple instructions at the same time, which increases the speed of program execution and performance. Computing performance is generally defined as the amount of time required to complete the task of discovering an inhibitor. The system-level block diagram of the lab-on-a-chip to design an inhibitor in artificial intelligence-based chemical space using the shared memory model is further illustrated in Fig. S1.†
Inhibitors and protein target models
The three-dimensional structures of approved drugs were retrieved from the PubChem database, and the purposed drugs 13B,36 Jun9_62_2R,22 N3 inhibitor,37 S217622,38 PBI0451,39 PF07304814,40 as well as herbal Andrographolide,41 and Panduratin A42 were acquired from previous publications. All potential inhibitors and the above drugs were optimized by the MMFF94 force field parameters of the Avogadro software. Crystal structures of protein and non-structural protein targets were obtained from the protein data bank, including the SARS-CoV-2 spike receptor binding with ACE2 (PDB: 6M0J), main protease (Mpro) with nsp 8/9 substrate (PDB: 7MGR), the enzyme SARS-CoV-2 papain-like protease (PLpro) (PDB ID: 6WX4), RNA-dependent RNA polymerase Nsp12 (RdRP) (PDB ID: 6M71), 2′-O-methyltransferase (nsp16/nsp10 complex) (MTase) (PDB ID: 6W4H), RNA-replicase (RNArep) Nsp9 (PDB: 6WC1), ADP ribose phosphatase (ADRP) (PDB: 6W02), and endoribonuclease (Endo) Nsp15 (PDB: 6VWW). Human protein domains to determine the inhibitor selectivity include lung protein D (PDB: 3IKP), lung protein C (PDB: 2ESY), human activated protein C (PDB: 1AUT), human tetrameric LL-37 peptide (PDB: 7PDC), and human serum albumin (PDB: 6M5E).
Molecular dynamics simulation
All MD simulations were run using the GROMACS 5.1.4 software.43 The computation with the LINCS algorithm was exploited in a time step of 2.5 fs with a 100 ns duration. The algorithm was used for all bonding interactions involving the N and C polymerase termini as well as ligand hydrogen bonds. The simulated box was performed with isothermal–isobaric (NPT) settings using Parrinello–Rahman pressure coupling. The short-range interaction relied on the van der Waals interaction with the Lennard-Jones potential at a cutoff distance of 0.15 nm. The long-range electrostatic interactions employed the particle mesh Ewald (PME) approach with fast Fourier transform (FFT) grid spacing. The hybrid functional B3LYP/6-31G(d) was used to optimize the predicted organic drugs.44,45 The CHARMM force field parameters were implemented into the simulation as well as obtaining ligand topology.46 The search spaces of the encapsulated rectangular box for the eight target proteins (1) dimension 113 Å × 68 Å × 58 Å for spike protein, (2) dimension 69 Å × 40 Å × 33 Å for main protease, (3) dimension 90 Å × 47 Å × 34 Å for papain-like protease, (4) dimension 108 Å × 85 Å × 69 Å for RNA-dependent RNA polymerase, (5) dimension 65 Å × 48 Å × 45 Å for 2′-O-methyltransferase, (6) dimension 64 Å × 40 Å × 40 Å for RNA-replicase, (7) dimension 74 Å × 54 Å × 42 Å for Endoribonuclease, (8) dimension 81 Å × 35 Å × 30 Å for ADP ribose phosphatase. For the main protease (PDB ID: 7MGR), the underwent alanine mutation was replaced with the catalytic residue CYS145 to attain the atomistic structure similar to SARS-CoV-1 with the homology modeling using SWISSMODEL web server.47
Instrument and implementation for deep learning and lab-on-a-chip integration
Wolfram Mathematica was used to extract digital features of potential 6538 H-donor and H-acceptor docking pockets in protomer targets. The digitized data was trained with Latin hypercube sampling and Keras algorithm48 using Python 3.11 to generate possible KS compounds with atom type (e.g., C, H, N, O, and F). The 1024-node graph convolutional layer included the ReLU and Tanh activation functions. After obtaining the optimized hyperparameters (e.g., number of nodes in the graph convolutional layer, dropout rate, and learning rate) in the Keras model, Python scripts were translated into a C program49 to minimize memory operation and activate the STM8S003K3T6C microprocessor.
Graph neural network (GNN) for METEORITE process
The GNN relies with an artificial neural network acting as geometric deep learning to fit within the viral binding sub-pockets, relying on module initiation, expansion, relabeling atom index, and termination (METEORITE). It is represented as a tuple of G = (Vc, Ecc) in which a set of carbon nodes or vertices ν ∈ V and a set of carbon–carbon edges eij = (ci,cj) ∈ Ecc, defining as the linking nodes. Molecules can be visualized as graphs, with atoms and the bonds that connect them represented as nodes and edges.50 The probability of the inhibiting ligand group j bound to site i, the predicted probability, denotes as Pi,j′ = max Pi,j (j = 1,…n), in which n is the total ligand groups.51
Training and validation of outer-shell DNN for PROOF process
Vina scores obtained from Python 3.11 interfacing with AutoDock Vina 1.2.0 was used as a supervising data set for deep learning-based docking scores.52 Multiple inhibitor orientations can be specified with the batch option and improve efficiency when running different conformation inhibitor states on protein pockets through pinning, extraction, remapping ligand, convolution, pooling, flattening, and regression (PROOF). When multiple inhibitor orientations are involved in molecular docking with a single receptor, grid cache preparation is utilized. The docking box is divided into multiple grid boxes with quantized coordination. The trained DNN force field estimator assembles the summation of the 3N partial derivatives of all T training points.53
where F(x) is a force field vector containing the 3N forces predicted for molecular geometry x and α is the interpolating deep learning parameters. At the end of the model, a multilayer perceptron (MLP) and linear layer L(x) was employed to produce the inhibitor sensing output.54
MLP(x) = LN(LN−1…L2(L1(x))) |
ADMET assessment
Swiss ADME55 and pkCSM56 were utilized to evaluate the ADMET (adsorption, distribution, metabolism, excretion, and toxicity) profiles of the identified KS inhibitors and other repurposed, purposed and herbal inhibitors. The pharmacokinetics druglikeness includes Lipinski, Ghose, Veber, Egan, and Muegge filters.
Results and discussion
METEORITE graph neural network for designing inhibitors
To initialize a SARS-CoV-2 inhibitor design with the inner-shell deep learning, the prediction of molecular structure was carried out by a graph neural network (GNN), as revealed in Fig. 1a. First, the sequence flow of the viral descriptors for multiple protomers, polymerases, and replicases was fed into the GNN model, which outputs lists of antiviral drugs with a simplified molecular-input line-entry system (SMILES) string. In addition, a probabilistic docking fitting percentage was obtained for protein pockets.
 |
| Fig. 1 (a) Information flow of H-bond donor and acceptor descriptors of SARS-CoV-2 targets for graph neural network (GNN) in determining RO5 antiviral inhibitors. (b) Overview of METEORITE in designing RO-5 antiviral drug. Generation starts with module initiation, followed by the expansion of designed molecules in protein pockets. The process sequentially relabels the atom and then ends with the termination method. | |
In this case, GNN output for a SARS-CoV-2 inhibiting candidate for fitting protein pockets with a probabilistic docking percentage is further given in Fig. S2.† As shown in Fig. 1b, METEORITE first selects module initiation to place carbon atoms in target pockets using trained neural networks. The initiated inhibiting moieties and the protein pocket include the three-dimensional coordinates. Later, fragments are bonded and are added to the inhibiting moieties in a stepwise manner by the expansion process to form the geometry of the attachment. Then the carbon feature could be replaced by another atom type (e.g., N, O, S, or F) using the relabeling atom procedure. Eventually, the selection process was ended through the termination method by adding or removing fragments as the designed inhibitors quantified rule-of-five Lipinski. After the inner-shell METEORITE GNN achieved in designing an inhibitor, the continuous process was carried out through outer-shell PROOF DNN for predicting inhibiting conformation and docking scores. As exhibited in Fig. 2a, the PROOF DNN framework includes information flow of seven sequential modules, including pinning, extraction, remapping, convolution, pooling, flattening, and regression. First, the input of the GNN-based KS inhibiting candidates was mapped onto a specific cleft of the viral SARS-CoV-2 polymerase fingerprints. The module of remapping KS compounds to a 2D-distance contact map includes the sequence of amino acids that encode the atomistic features and bonding interactions comparable to the 3D interpolated-charged SARS-CoV-2 polymerase structure given in Fig. S3.†
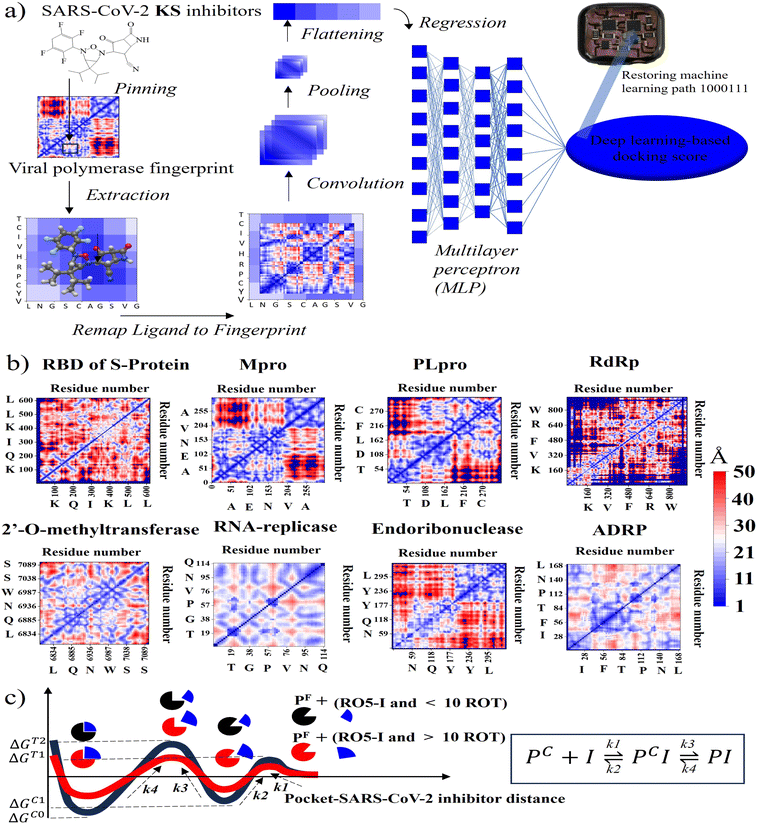 |
| Fig. 2 (a) Representative illustration of outer-shell DNN. The information flows from GNN-based KS inhibitors that are pinned onto 2D viral descriptors to predict ML docking scores with consecutive modules of pinning, extraction, remapping, convolution, pooling, flattening, and regression. (b) 2D SARS-CoV-2 fingerprints for RBD of S-protein, Mpro, PLpro, RdRp, MTase, RNA-replicase, Endo, and ADRP. (c) Simultaneous binding model with inducing locking. PF denotes the pocket conformation in the bound and unbound form and k1–k4 represents the forward and reverse rate constants for transitions between the locking states. | |
PROOF deep neural network for docking sensing scores
For instance, all fragments of artificial intelligence-designed KS compound analogues to deactivate the CYS145 and HIS41 catalytic dyads of the SARS-CoV-2 main protease are illustrated in Fig. S4.† Apparently, the designed fragments are conformed to fit in the pockets with different distinct chemical terminals. In a later section, the inhibitory chemical terminals that neutralize the viral protein target are discussed in more detail. The output units from the remapping process then served as convoluted parameters for the pooling module for feature extraction. The flattening process arranges the pooled layers into a vector that extracts the ligated protein complex interaction. Afterward, the regression procedure handles the iteration of the multilayer perceptron (MLP) with k hidden layers that output the DNN-based docking scores. The information from ML neuron training was later stored on the artificial intelligence-based lab-on-a-chip, or DNN-based microprocessor. The 2D-descriptor inputs of viral SARS-CoV-2 targets embedding toward the digitized flow for PROOF deep learning are revealed in Fig. 2b. To determine the conserved pocket regions, the viral cavities are pinned as the neighbouring amino acids clustering together with the 2D viral descriptor with shaped-related and physicochemical properties. Distinctively, the binding pocket space (light blue area) of RNA-replicase is confined with smaller sizes than that of major protease, papain-like protease, and the RBD of S-protein. Compared to moderate-sized peptidomimetic inhibitors, Lipinski (RO5) inhibitors of a molecular weight of less than 500 Da may be able to pass through the small dynamic pockets. While there has been significant progress in the development of antivirals like Paxlovid (nirmatrelvir-ritonavir), which target the Mpro and the S-protein, or Molnupiravir, which can degrade the viral RdRp and have an emergency use authorization, little work has been done in conjunction with the other various SARS-CoV-2 targets.3 Nevertheless, the Mpro mutations inferred drug resistance to Nirmatrelvir.13 In addition, as seen in Table 1, Nirmatrelvir does not meet Veber's filtration and has more than 10 rotational bonds.57 To overcome the hurdle of drug resistance, the insight work was demonstrated in Fig. 2c as the RO5 inhibitor with less than and more than 10 rotational bonds locking into RNA-replicase pockets.
Table 1 METEORITE-PROOF sensing (in bold) and Vina docking scores (in parenthesis) of contemporary repurposed, purposed, herbal, and artificial-intelligence designing inhibitors for deactivating SARS-CoV-2 protein targets and validation of Lipinski, Ghose, Veber, Egan, and Muegge druglikenessa
Compound |
S-protein |
Mpro |
PLpro |
RdRp |
MTase |
RNArep |
Endo |
ADRP |
Lip. Vio |
Gho. Vio. |
V./E./M |
S-protein: spike glycoprotein, Mpro: main protease, PLpro: papain-like protease, RdRp: RNA-dependent RNA polymerase, MTase: 2′-O-methyltransferase, RNArep: RNA-replicase, Endo: endoribonuclease, ADRP: ADP ribose phosphatase, Lip. Vio.: numbers of Lipinski violation, Gho. Vio.: numbers of Ghose violation, V./E./M.: whether a drug passes all Veber, Egan, and Muegge druglikeness. |
Remdesivir |
−8.0(−8.1) |
−8.1(−8.2) |
−6.5(−6.3) |
−8.5(−8.5) |
−7.2(−7.3) |
−7.0(−6.8) |
−8.4(−8.3) |
−8.2(−8.4) |
2 |
3 |
No |
Saquinavir |
−8.8(−8.9) |
−8.7(−8.8) |
−7.3(−7.3) |
−8.7(−8.9) |
−9.7(−9.5) |
−8.3(−8.2) |
−10.2(−10.1) |
−10.3(−10.4) |
2 |
3 |
No |
Indinavir |
−10.2(−10.1) |
−8.3(−8.4) |
−7.2(−7.3) |
−8.7(−8.7) |
−8.7(−8.9) |
−6.6(−6.7) |
−8.6(−8.7) |
−9.4(−9.6) |
1 |
3 |
No |
Zafirlukast |
−8.9(−9.0) |
−7.8(−7.9) |
−8.3(−8.3) |
−8.9(−8.9) |
−9.4(−9.5) |
−7.7(−7.5) |
−9.1(−9.2) |
−9.3(−9.4) |
1 |
4 |
No |
Molnupira−vir |
−7.2(−7.3) |
−7.7(−7.5) |
−6.4(−6.4) |
−7.3(−7.3) |
−7.1(−7.0) |
−5.9(−5.8) |
−7.7(−7.6) |
−7.4(−7.5) |
0 |
1 |
No |
N3 inhibitor |
−8.5(−8.6) |
−7.6(−7.8) |
−6.5(−6.8) |
−8.8(−8.7) |
−7.9(−7.9) |
−7.0(−6.9) |
−8.5(−8.6) |
−8.3(−8.3) |
2 |
3 |
No |
Perampanel |
−8.2(−8.2) |
−8.1(−8.3) |
−7.3(−7.2) |
−8.1(−8.0) |
−8.2(−8.2) |
−7.0(−7.1) |
−8.9(−8.8) |
−8.6(−8.4) |
0 |
0 |
Yes |
Penicillin |
−8.3(−8.2) |
−7.9(−7.7) |
−7.4(−7.4) |
−7.1(−7.1) |
−7.1(−7.2) |
−6.4(−6.4) |
−8.3(−8.2) |
−8.5(−8.4) |
0 |
0 |
Yes |
Jun9_62_2R |
−8.9(−8.8) |
−6.6(−6.8) |
−7.0(−7.0) |
−7.6(−7.7) |
−7.9(−7.9) |
−7.5(−7.4) |
−8.5(8.5) |
−10.5(−10.4) |
1 |
3 |
No |
Nirmatrelvir |
−8.9(−8.9) |
−8.2(−8.2) |
−7.0(−7.1) |
−8.4(−8.2) |
−8.0(−8.1) |
−7.1(−7.0) |
−7.8(−7.9) |
−9.2(−9.3) |
0 |
1 |
No |
Ritonavir |
−8.4(−8.2) |
−7.9(−8.0) |
−6.4(−6.2) |
−8.6(−8.6) |
−8.0(−8.0) |
−7.3(−7.4) |
−7.6(−7.5) |
−9.0(−9.1) |
2 |
4 |
No |
Amoxicillin |
−8.1(−8.1) |
−7.7(−7.7) |
−7.0(−7.0) |
−7.4(−7.5) |
−7.9(−7.8) |
−6.6(−6.6) |
−7.5(−7.4) |
−8.7(−8.6) |
0 |
0 |
No |
Clavulanic acid |
−5.3(−5.5) |
−6.3(−6.0) |
−5.8(−5.9) |
−5.7(−5.7) |
−6.3(−6.3) |
−4.8(−4.8) |
−5.8(−5.8) |
−6.7(−6.8) |
0 |
1 |
No |
S217622 |
−9.6(−9.7) |
−9.2(−9.2) |
−8.7(−8.7) |
−8.9(−8.9) |
−9.3(−9.1) |
−8.1(−8.0) |
−9.0(−9.1) |
−10.1(−10.0) |
2 |
1 |
No |
PF07304814 |
−7.7(−7.7) |
−8.3(−8.5) |
−8.0(−7.9) |
−8.4(−8.5) |
−8.1(−8.2) |
−7.1(−7.1) |
−8.4(−8.5) |
−7.9(−7.9) |
3 |
3 |
No |
PBI0451 |
−8.7(−8.7) |
−8.0(−8.0) |
−7.4(−7.5) |
−7.9(−7.9) |
−8.7(−8.5) |
−7.9(−7.6) |
−8.4(−8.2) |
−10.0(−10.1) |
0 |
0 |
Yes |
13B |
−8.4(−8.4) |
−6.4(−6.5) |
−6.7(6.9) |
−8.1(−8.3) |
−7.2(−7.2) |
−7.3(−7.1) |
−8.6(−8.7) |
−9.1(−9.2) |
1 |
3 |
No |
Andro-grapholine |
−7.5(−7.4) |
−7.3(−7.4) |
−7.1(−7.1) |
−7.4(−7.4) |
−7.3(−7.2) |
−6.2(−6.2) |
−8.0(−8.1) |
−7.6(−7.6) |
0 |
0 |
Yes |
Panduratin-A |
−7.9(−7.8) |
−7.0(−7.1) |
−6.1(−6.2) |
−7.0(−7.0) |
−7.2(−7.2) |
−7.0(−7.0) |
−8.2(−8.2) |
−8.7(−8.6) |
0 |
1 |
No |
KS 1 |
−9.4(−9.4) |
−9.4(−9.4) |
−8.3(−8.2) |
−8.7(8.8) |
−8.9(−8.8) |
−8.1(−8.0) |
−9.5(−9.4) |
−9.4(−9.4) |
0 |
0 |
Yes |
KS 2 |
−10.2(−10.3) |
−9.3(−9.3) |
−8.4(−8.4) |
−9.9(−9.8) |
−9.7(−9.7) |
−8.8(−8.8) |
−10.2(−10.2) |
−9.4(−9.5) |
0 |
0 |
Yes |
KS 3 |
−9.0(−9.0) |
−9.3(−9.3) |
−8.3(−8.3) |
−9.6(−9.6) |
−9.5(−9.6) |
−8.3(−8.3) |
−10.1(−10.0) |
−9.9(−10.0) |
0 |
0 |
Yes |
KS 4 |
−8.5(−8.4) |
−8.8(−8.9) |
−7.6(−7.7) |
−9.7(−9.6) |
−8.7(−8.7) |
−8.0(−8.0) |
−9.7(−9.7) |
−9.1(−9.0) |
0 |
0 |
No |
KS 5 |
−8.3(−8.3) |
−8.6(−8.6) |
−7.5(−7.5) |
−9.0(−9.0) |
−8.1(−8.1) |
−7.5(−7.5) |
−9.1(−9.0) |
−9.4(−9.4) |
0 |
1 |
Yes |
KS 6 |
−8.2(−8.2) |
−8.5(−8.4) |
−8.3(−8.3) |
−8.6(−8.6) |
−8.6(−8.8) |
−7.3(−7.1) |
−9.1(−9.2) |
−9.9(−9.9) |
0 |
1 |
Yes |
KS 7 |
−7.6(−7.6) |
−8.4(−8.4) |
−7.6(−7.6) |
−7.4(−7.2) |
−8.4(−8.4) |
−6.8(−6.8) |
−8.0(−8.0) |
−7.1(−7.1) |
0 |
1 |
No |
KS 8 |
−8.4(−8.5) |
−8.5(−8.4) |
−7.3(−7.5) |
−8.8(−8.9) |
−8.8(−8.7) |
−7.3(−7.1) |
−8.8(−8.9) |
−7.8(−7.9) |
0 |
3 |
No |
KS 9 |
−9.0(−9.0) |
−8.2(−8.2) |
−7.9(−7.9) |
−8.9(−8.8) |
−9.0(−9.0) |
−6.8(−6.8) |
−8.5(−8.5) |
−8.1(−8.0) |
0 |
0 |
Yes |
KS10 |
−8.1(−8.0) |
−7.9(−8.0) |
−7.2(−7.2) |
−7.1(−7.1) |
−7.9(−7.8) |
−6.5(−6.5) |
−7.3(−7.3) |
−8.1(−8.2) |
0 |
1 |
No |
KS11 |
−7.9(−7.9) |
−8.0(−8.0) |
−7.5(−7.6) |
−7.3(−7.5) |
−8.2(−8.1) |
−6.6(−6.6) |
−7.6(−7.6) |
−7.7(−7.7) |
0 |
0 |
Yes |
KS12 |
−8.5(−8.4) |
−7.6(−7.6) |
−7.4(−7.4) |
−8.1(−8.0) |
−8.1(−8.1) |
−7.0(−7.0) |
−8.5(−8.6) |
−8.6(−8.7) |
0 |
1 |
Yes |
Two-dimensional inhibitor descriptors
The forward and reverse rate constant are governed in the consecutive steps dominating binding and unlocking process. With faster forward constant rates, the RO5 inhibitor less than 10 rotational bonds (KS1 and KS2 compounds in Table 1) were able to overcome the large uphill or transitional binding free energy (ΔGT) and achieve the lowest docking free energy (ΔGC) in the final conformational states. On other hand, RO5 inhibitors with more than 10 rotational bonds need more free energy to get past the greater barrier inducing by the RNA-replicase motion that causes pocket gates to open and close.
To assure that the RO5-compound with less than 10 rotational bonds could dock into multiple RNA-replicase pocket gaits with enhanced potent binding, the simulation of promising KS1 inhibiting candidate bound onto the replicase was demonstrated in Video S1† and simulation parameters given in Fig. S5.† In general, the architecture of 3D-neural networks imposes limitations, especially in computational efficiency, where costs rise with the third power. To overcome it, precise coordinates for every constituent atom in 3D molecules were transferred into 2D molecular descriptors. As shown in Fig. 3, selected primary GNN-based SARS-CoV-2 inhibitors are exhibited with embedded distinct cyclic structures. As a result, the designed bicyclic KS1 compound or 3-(6,6-diisopropyl-4-(2,3,5,6-tetrafluorocyclohexa-2,5-dien-1-yl)-3-oxa-2,4-diazabicyclo sp2)-F probe of 2,4-difluorobicyclo[4.2.0]octa-1,4-diene could act as carbonyl moiety with bond polarization, given that its dipole is quite comparable to C–O. The pocket distance phase of 0.1 nm interspace from KS1 to KS5 and KS12 shows the similarity as these retain bi and tricyclic structures. In contrast, the (1s,5s)-2,4-dimethyl-3-oxa-2, 4-diazatricyclo[3.2.2.01,5] nonane chain of KS9 provide distinct inner pocket phase boundary of 0.1 nm distance apart.
 |
| Fig. 3 Selected primary GNN-based SARS-CoV-2 inhibitors with corresponding 2D molecular descriptors. | |
METEORITE-PROOF process in lab-on-a-chip
The lab-on-a-chip with the close-loop of SARS-CoV-2 RO5-inhibitor discovery and sensing operations includes all the essential components needed for automating covalent and non-covalent docking of designed warheads to multiple viral receptors, as shown in Fig. 4a. The discovery modes combined a graph neural network to design covalent inhibitors and a deep neural network to predict the binding interaction with docking scores. The sense mode would output the docking scores using the lab-on-a-chip readout. The docking poses of KS1 compound inhibiting allosteric sites of protein targets was shown in Fig. 4b, indicating the inhibitor can arrest on multiple pocket gates. To facilitate the conformational placement of KS inhibitors, dual processor core combines the dual deep learning process as exhibited in system-level block diagram of Fig. 4c. As given in Fig. 4d with the correlation matrix and Table 1, KS2 inhibitor possess the distinct capability to deactivate multiple protein targets as compared with other contemporary inhibitors.
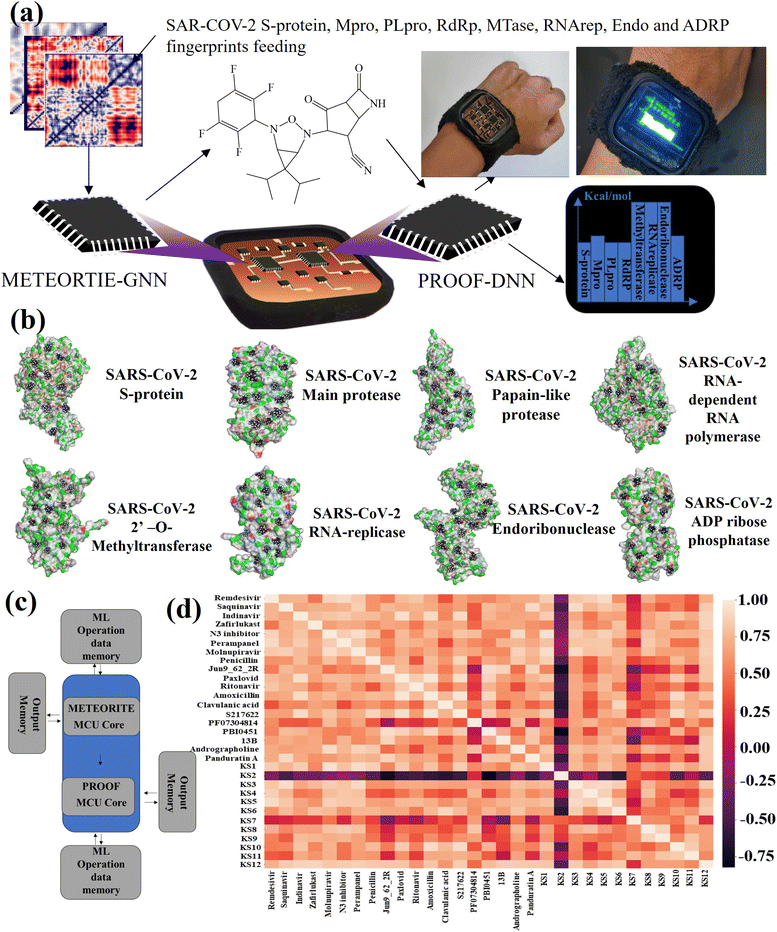 |
| Fig. 4 (a) Illustration of information flows through the lab-on-a-chip with RO5-drug discovery and sense modes. (b) Snapshots of the selected KS1 inhibitors binding on multiple allosteric sites of SARS-CoV-2 protein targets. (c) System-level block diagram of the lab-on-a-chip for deep learning-based discovery mode. (d) Correlation matrix describes the docking performance comparison among the chosen existing SARS-CoV-2 drugs, herbal remedies, and artificial intelligence-designed KS compounds. | |
With the established pharmacokinetic principles, Lipinski's rule of 5 requires inhibiting H-bonds < 5, H-acceptors < 10, molecular weight < 500 Da, and octanol–water partition coefficient (log
P) < 5.58 The GHOSE's filter denotes that the inhibiting log
P is between −0.4 and 5.6, molecular weight is between 160 and 480, molar refractivity is between 40 and 130, and the total number of atoms is between 20 and 70.59 Meanwhile, Veber's filter encloses all rotational bonds less than 10 and topological polar surface area (TPSA) less than 140.57 Specifically, Egan's filter includes WLogP (lipophilicity) less than 5.99 and the total polar surface area less than 131.60 Muegge's filter accepts the molecular weight between 200 and 600 Da, XLOGP3 (lipophilicity) between −2 and 5, the total polar surface area less than 150, the number of rings less than 7, the number of carbons greater than 4, the number of heteroatoms larger than 1, the number of rotatable bonds less than 15, the hydrogen bond acceptors less than 10, and the hydrogen bond donors less than 5.61 Table 1 shows that the AI-based sensing scores correlate well with the Vina docking scores. As listed with repurposed, purposed, and herbal drugs (inhibitor nomenclatures given in Fig. S6†) in the table, [3.1.0] bicyclic KS1 and [4.2.0] tricyclic KS2 inhibitors were developed in the METEORITE-PROOF process to deactivate multiple protein targets, despite the fact that Paxlovid, which combines the inhibitors nirmatrelvir and ritonavir, resists to the viral main protease as discussed in the previous section.13 In addition, an example to obtain AI-based sensing using 2D molecular inhibiting and SARS-CoV-2 descriptors through the METEORITE-PROOF process is given in Fig. S7.†
Binding interactions of KS2 inhibitors bound on SARS-CoV-2
To validate the binding interaction of the artificial intelligence-designing compounds, KS2 triple cyclic inhibitor was selected to search for the most probable binding postures at the optimum binding affinity. Fig. 5a exhibited the best KS2 compound docked configuration on the SARS-CoV-2 spike receptor-binding domain (RBD) with a vina docking score of −10.3 kcal mol−1. The obtained highest docking scores compared to other reported inhibitors are attributed to Lipinski drug transport within pocket gates of 5 Å radius, as given by the docking snapshot in Fig. S8.† The nitrile head was intacted with PHE72 benzene ring, while TYR385 residue could either bind hydrogen bonding with the oxygen tail of azetidine-2-one or halogen fluorine bonding with difluorocyclopentane. The aligned PHE390 residue forms pi–pi interactions with the cyclobutene tails of bicyclo[3.2.0]heptane and bicyclo[4.2.0]octa-1,4-diene segments and allows weakly fluorine bonding with difluorocyclohexa-1,4-diene.
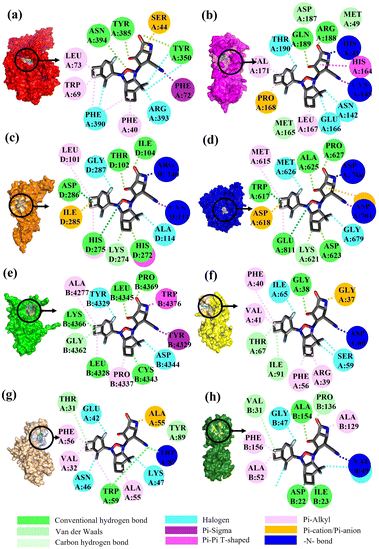 |
| Fig. 5 Molecular interactions between the KS2 inhibitor and the SARS-CoV-2 protein targets (a) Receptor-binding domain (RBD) of spike glycoprotein (S-protein). (b) Main protease (Mpro). (c) Papain-like protease (PLpro). (d) RNA-dependent RNA polymerase (RdRp). (e) 2′-O-Methyltransferase (MTase). (f) RNA-replicase (RNArep). (g) Endoribonuclease (Endo). (h) ADP ribose phosphatase (ADRP). | |
Previous studies demonstrated that the active dyad site of the main protease has CYS145 and HIS41 residues located at a cleft nearby domain I (residues 15–99) and domain II (residues 1–14, 100–197) protomers.62 In this context shown in Fig. 5b, the nitrogen center group of azetidine-2-one attacked the carbonyl moiety of HIS41 catalytic base, while depronated CYS145 could covalently form with the nitrile head of KS2 inhibitors as described in eqn (1) with a demonstration in Video S2† (simulation parameters listed in Fig. S9†). The existence of a hydrophobic Pi–Pi interaction is also found at HIS164 toward the cyclopentanone ring. The attribute of PRO168 hydroxyl tails provides a weak interaction with the cyclobutene terminal. With the consideration that the conserved catalytic triad sties of CYS111, HIS272, and ASP286 exist in the PLpro protease,63 the prediction of binding activity was exhibited in Fig. 5c. The binding position at this catalytic site relied on the deactivation of CYS111 with the acetonitrile head. The hydrogen bond of cyclopentanone tails defuse HIS272 and ASP286 as well as the Pi–Pi interaction to neutralize HIS272 residues.
|
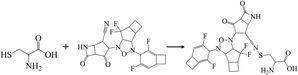 | (1) |
In addition, halogen-forming interactions have been also seen at ALA114 and GLY287. While the catalytic sites of SARS-CoV-2 RdRp lied down with ASP 760, ASP761, and ASP618 residues,64 this negative polar contact site was neutralized with KS2 inhibitor as it entered the pocket gate, given in Fig. 5d. The deprotonated azetidine-2-one terminal attacked the carbonyl group of ASP760, while the acetonitrile head was oriented to form ASP761. In addition, the cyclopentanone ring and cyclobutene terminal also deactivate ASP761 and ASP618 through Pi–cation/Pi–anion interactions, respectively. To further neutralize the polar contact site, the neighboring residues of ASP623, LYS62, GLU811, TRP617, ALA625, and PRO627 contribute hydrogen formation to the catalytic site. Effectively, antiviral treatment could aim for the methyltransferase (MTase) that caps viral mRNAs because the capping entity enhances the translation of viral proteins and keeps the host immune system from realizing viral mRNAs. To achieve this, the optimized binding conformation of the KS2 inhibitor activating on the allosteric MTase site is shown in Fig. 5e. The acetonitrile head is oriented toward the phenol ring of TYR4329, while the azetidine-2-one head induces Pi–Pi interaction with the 1H-indole ring of TRP4376. The nearby CYS4343, LEU4328, GLY4362, LYS4366, LEU4345, and PRO4369 residues triggered the activation of hydrogen and carbon–hydrogen formation. Notably, RNA replicase (nsp 9) is the only viral protomer and protease that can be effectively defused by several hypothesized, repurposed, and current herbal inhibitors given in Table 1. This obstacle is addressed by KS1 and KS2 inhibitors, which achieve upper vina docking scores of −8.0 and −8.8 kcal mol−1, respectively. As shown in Fig. 5f, the KS2 inhibitor relied on the electrophilic acetonitrile head to covalently form with ASP60, as revealed by eqn (2).
|
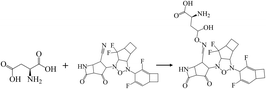 | (2) |
The neighboring ARG39, PHE40, VAL41, and PHE56 occupied pi–alkyl interactions in deactivating the allosteric gate. It is evident that the SARS-CoV-2 inhibitors selected in the table offer encouraging docking scores in neutralizing the endoribonuclease that processes viral RNA to avoid the host defense system's identification. Fig. 5g provides the optimum binding pose of KS2 inhibitor exhibiting TRP87 and ALA55, which are lined with the acetonitrile head and an azetidine-2-one plane. This metabolically labile pocket further blocked GLU42, ASN46, and ASP92 with fluorinated bonding. Despite SARS-CoV-2 modulators such as Saquinavir, Indinavir, Zafirlukast, Jun_9_62_2R, S217622, and 13B achieving high docking scores for ADP ribose phosphatase inhibition, they are constrained by a Lipinski drug violation. With the design of the KS2 inhibitor, the acetonitrile head could catalytically form with the carbonyl group of VAL49, and pi–alkyl bond interactions exist within ALA52, ALA129, and PHE156 residues, given in Fig. 5h. In addition, water-bridging hydrogen and carbon–hydrogen formation also occurs at ASP22, ILE23, VAL31, PRO136, and ALA154 residues, attaining the optimal docking score of −9.5 kcal mol−1.
Stability analysis of the inhibitor-SARS-CoV-2 complex
Further analysis was carried out with stability tests. The KS2 inhibitor interacting with SARS-CoV-2 protein targets were simulated utilizing MD at 100 ns. To evaluate how simulations of the complex might be affected in the absence of inhibitors, the unligated SARS-CoV-2 complex was considered for analysis. Frequent factors, including inhibitor conformation, water molecules, nearby ions, association–disassociation factors, inhibiting protonation state, and inhibitor-protein and solvation entropies, can unexpectedly influence simulation predictions. The optimized structure of the simulated docking complexes realized strong stereochemical geometries of the protomer, polymerase, and replicase residues, as depicted in the Ramachandran diagram in Fig. 6a. The diagram states that there are strong interactions, as seen with the unligated SARS-CoV-2 complex given in Fig. S10,† and the inhibitor stabilizes multiple SARS-CoV-2 protomers, polymerases, and replicases at the active sites through water bridges, hydrophobic, hydrophilic, and hydrogen bond interactions.
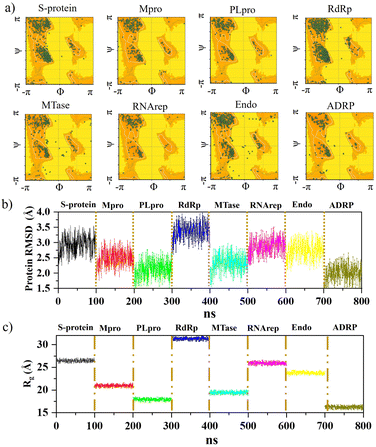 |
| Fig. 6 Ramachandran diagram illustrating stereochemical geometry for (a) complexes of the KS2 inhibitor bound with S-protein, Mpro, PLpro, RdRp, MTase, RNArep, Endo, and ADRP. Plots of (b) RMSD and (c) Rg of the KS2 inhibitor complexed with all 8 protein targets. | |
Hence, the MD study strongly validated the molecular docking data of SARS-CoV-2 inhibitor interactions. Further MD simulation was performed, where the stability of the KS2 inhibitor was evaluated using the root mean square deviation (RMSD) of backbone atoms with all 8 protein targets. As depicted in Fig. 6b, the RMSD traces of S-protein, Mpro, PLpro, RdRp, MTase, RNArep, Endo, and ADRP complexed with the KS2 inhibitor exhibit an average RMSD of 2.3–3.5 Å, 1.8–3.1 Å, 1.5–2.6 Å, 2.7–3.9 Å, 1.6–3.0 Å, 2.3–3.5 Å, 2.0–3.4 Å, and 1.5–2.6 Å, respectively. It is remarkable to note that all the protomers, polymerases, and replicases complexed with the KS2 inhibitor, achieving their stable conformations at the end of the 40 ns time scale simulation, as seen by their convergence and stable RMSD values. The Rg values of all viral protein target-inhibitor complexes extracted in Fig. 6c did not significantly change over the simulated period, implying that the inhibitors did not separate from the complex and that the compactness of the viral proteins did not alter.
Stability of acetonitrile catalytic head
To confirm the affinity and specificity of KS inhibitors with the viral Mpro target, MD simulations were carried out to investigate three different KS1, KS2, and KS12 compounds. Evidently, the molecular interaction between the acetonitrile head and the Mpro active dyad site is a result of an unaltered covalent bonding interaction (within a cut-off distance of 0.15 nm) for the KS1 and KS2 inhibitors shown in Fig. 7a and b, respectively. As a result, the fluorinated placement of tetrafluorocyclohexa-1,4-diene, difluorobicyclo[3.2.0]heptane, and difluorocyclo[4.2.0]octa-1,4-diene termini enhances the catalytic attachment, providing optimum docking scores in the table.
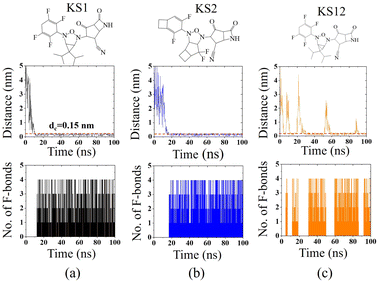 |
| Fig. 7 Recognition interaction time with separation distance and several fluorinated bonds between (a) KS1, (b) KS2, and (c) KS12 inhibitors with the SARS-CoV-2 Mpro active site. The dashed line indicates a cutoff distance of 0.15 nm to define covalent binding. | |
Nevertheless, the 3-oxa-2,4-diazatricyclo[3.1.1.01,5]heptane plane of KS12, shown in Fig. 7c, destabilizes the catalytic attack of the acetonitrile head. It ascertains that the fluorinated placement as well as the carbon planes of cyclic compounds contribute to optimize binding potency.
Accuracy predictions of KS inhibitors defusing SARS-CoV-2 protein targets
This portion further examined the coherence accuracy of all selected primary 12 KS inhibitors using artificial intelligence, while the binding efficacy of the developed inhibitors was verified using MD simulations. Overall, all protein targets have Vina docking scores of at least R2 = 0.97 and RMSE ranging (0.20–0.40), as shown in Fig. 8a obtained using METEORITE-PROOF deep learning, indicating that the validated ML model is accurate. The KS1 inhibitor's confusion matrices, which are displayed in Fig. 8b–d, demonstrate great prediction accuracy in each ML score interval when bound to the SARS-CoV-2 S-protein, Mpro, and RNA replicase. Thus, the proper inhibitor structure in the viral pockets could be predicted by deep learning. The KS1 and KS2 ligands' accuracy radar plots, which show how they inhibit all protein targets, are shown in Fig. 8e and f. These plots show the high accuracy of the ML model as well as high accuracy precision-recall plot (Fig. S11†) in predicting the dynamics of protein binding at allosteric pockets caused by these compounds' physicochemical interactions. Furthermore, as Fig. 8g illustrates, the ML model predicts with great accuracy the structural selection of KS1–KS12 inhibitors onto numerous RNA replicase pockets.
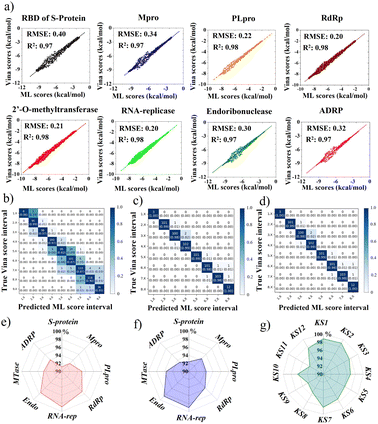 |
| Fig. 8 (a) Parity plot of Vina docking and ML sensing scores, showing the high prediction fitting attained by ML models. RMSE: root mean square error; R2: correlation coefficient. Confusion matrices of KS1 inhibitor modulating (b) spike-protein, (c) main protease, and (d) RNA replicase. Accuracy radar plots of (e) KS1 inhibitors, (f) KS2 inhibitors deactivating protein targets, and (g) KS1 to KS12 inhibitors neutralizing RNA replicase. | |
Selectivity of KS1 and KS2 inhibitors toward protomer targets relative to human protein domains
Investigating the selectivity of the developed compounds towards the viral protomers relative to human protein domains is another crucial part of creating a lab-on-a-chip to speed up the drug development process, as an oral medication shouldn't have any negative effects on humans. In the molecular dynamics study, human lung protein that plays a role in host defense against infection and human serum albumin that transports hormones, fatty acids, and other important compounds in blood stream were chosen as human protein domains. As given in Fig. 9, KS1 and KS2 compounds show an excellent inhibitory action toward all viral subunit targets relative to human protein domains. When compared to non-target protein binding energies (−5.7 to −6.3 kcal mol−1), it is evident that all viral subunits have lower binding energies toward the inhibitors.
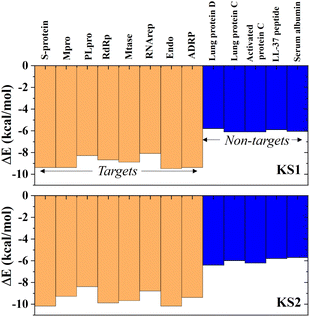 |
| Fig. 9 Selectivity of KS1 and KS2 inhibitors toward viral subunits relative to selected human protein domains. | |
Real-time obtaining lab-on-chip sensing scores
To achieve a quick drug design search in the lab-on-a-chip, real-time sensing score output is required for the closed-loop drug discovery process with minimized trial errors. The sensing scores of KS1 and KS2 inhibitors binding to protein targets were obtained from the lab-on-a-chip, as shown in Fig. 10a and b. Video S3† provides additional detail on how to activate the lab-on-a-chip and reveal KS inhibitors.
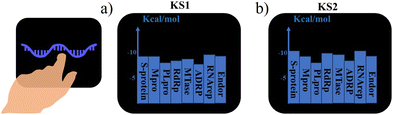 |
| Fig. 10 Real-time output screen of the on-wrist lab-on-a-chip for (a) KS1 and (b) KS2 inhibitors. | |
A snapshot of the on-wrist lab-on-a-chip that can accommodate dual microprocessors is shown in Fig. S12.† All twelve main KS inhibitors with their SMILES string output as well as their primary KS inhibiting analogs are given in Fig. S13 and S14,† respectively.
Conclusions
Time constraints are a challenge in the quest for novel drugs, particularly in the presence of long-term COVID or post-COVID conditions that escalate impaired health functions, leading to low work productivity in the modern world. Considering viral polymerase pocket dynamics with reduced-dimensional Euclidean descriptors can increase the accuracy of pocket identification with a reduced cost of deep learning and reveal more viral inhibitors with bi- and tricyclic structures. The work proposes the lab-on-a-chip that restores a reserve of machine learning nodes and utilizes an automated descriptor reduction approach that notably reduces the complexity of the interaction map and simplifies the learning effect. With a limited capability of repurposing and herbal remedies to confine viral RNA replication, multi-level cyclic compounds stood as a candidate to manage the Long COVID. The precise placement of halogen terminals in the cyclic compound enhances the acetonitrile catalytic head to deactivate multiple viral protein targets. The reported multilevel cyclic compounds are highly notable as inhibitors of multiple viral protein targets as they are novel and nonpeptidic with Lipinski attributes. Currently, drug purposing using the artificial intelligence-aiding lab-on-a-chip could greatly aid in the development of therapeutic inhibitors for effective management of Long COVID. Furthermore, many lab-on-a-chip technologies have only been employed extremely limited for illness diagnoses. This is a significant step toward developing the lab-on-a-chip to speed up the drug development process and supplement the ageing labor force in the event of impending pandemics.
Data availability
All data is available within the manuscript and ESI† (including video simulation and video demonstration).
Author contributions
S. K. carried out the lab-on-a-chip assembly and wrote the manuscript. S. K. and W. S. designed this deep learning-integrated lab-on-a-chip. S. K. conceived the original idea. S. K. and W. S. edited and reviewed the manuscript.
Conflicts of interest
The authors declare no competing financial interests regarding the publication of this paper.
Acknowledgements
The authors gratefully acknowledge financial support from the Fundamental Fund (FF2566 and FF2567) for smart healthcare theme and national PM 2.5 grant provided by Thailand Science Research and Innovation (TSRI) and National Research Council of Thailand (NRCT) for King Mongkut's University of Technology Thonburi.
References
- M. T. Osterholm, in The COVID-19 Reader, Routledge, 2020, pp. 11–20 Search PubMed.
- W. H. O. Organization, https://covid19.who.int/, accessed 2024-08-12.
- C. Pozzi, A. Vanet, V. Francesconi, L. Tagliazucchi, G. Tassone, A. Venturelli, F. Spyrakis, M. Mazzorana, M. P. Costi and M. Tonelli, J. Med. Chem., 2023, 66, 3664–3702, DOI:10.1021/acs.jmedchem.2c01229.
- D. R. Owen, C. M. Allerton, A. S. Anderson, L. Aschenbrenner, M. Avery, S. Berritt, B. Boras, R. D. Cardin, A. Carlo and K. J. Coffman, Science, 2021, 374, 1586–1593, DOI:10.1126/science.abl4784.
- Y. Duan, H. Zhou, X. Liu, S. Iketani, M. Lin, X. Zhang, Q. Bian, H. Wang, H. Sun and S. J. Hong, Nature, 2023, 622, 376–382, DOI:10.1038/s41586-023-06609-0.
- S. Malesevic, N. A. Sievi, P. Baumgartner, K. Roser, G. Sommer, D. Schmidt, F. Vallelian, I. Jelcic, C. F. Clarenbach and M. Kohler, Sci. Rep., 2023, 13, 7717, DOI:10.1038/s41598-023-34678-8.
- D. Buonsenso, L. Martino, R. Morello, F. Mariani, K. Fearnley and P. Valentini, Lancet Microbe, 2023, 4, e745–e756, DOI:10.1016/S2666-5247(23)00115-5.
- Y. Kaku, K. Okumura, M. Padilla-Blanco, Y. Kosugi, K. Uriu, A. A. Hinay, L. Chen, A. Plianchaisuk, K. Kobiyama and K. J. Ishii, Lancet Infect. Dis., 2024, 24, e84, DOI:10.1016/S1473-3099(23)00813-7.
- L.-C. Yang, Y.-N. Wang, R. Liu, Y. Luo, X. Q. Ng, B. Yang, Z.-Q. Rong, Y. Lan, Z. Shao and Y. Zhao, Nat. Chem., 2020, 12, 860–868, DOI:10.1038/s41557-020-0503-7.
- T. B. Hughes, G. P. Miller and S. J. Swamidass, ACS Cent. Sci., 2015, 1, 168–180, DOI:10.1021/acscentsci.5b00131.
- A. Savarino, J. R. Boelaert, A. Cassone, G. Majori and R. Cauda, Lancet Infect. Dis., 2003, 3, 722–727, DOI:10.1016/S1473-3099(03)00806-5.
- R. Alonso, A. Cuevas and P. Mata, Core Evidence, 2019, 19–30, DOI:10.2147/CE.S174169.
- Y. Hu, E. M. Lewandowski, H. Tan, X. Zhang, R. T. Morgan, X. Zhang, L. M. Jacobs, S. G. Butler, M. V. Gongora and J. Choy, ACS Cent. Sci., 2023, 9, 1658–1669, DOI:10.1021/acscentsci.3c00538.
- V. S. Nunes, D. F. Paschoal, L. A. S. Costa and H. F. D. Santos, J. Biomol. Struct. Dyn., 2022, 40, 8989–9003, DOI:10.1080/07391102.2021.1921033.
- B. Tan, R. Joyce, H. Tan, Y. Hu and J. Wang, Acc. Chem. Res., 2022, 56, 157–168, DOI:10.1021/acs.accounts.2c00735.
- R. P. Joshi, K. J. Schultz, J. W. Wilson, A. Kruel, R. A. Varikoti, C. J. Kombala, D. W. Kneller, S. Galanie, G. Phillips and Q. Zhang, J. Chem. Inf. Model., 2023, 63, 1438–1453, DOI:10.1021/acs.jcim.2c01377.
- H. Willems, S. De Cesco and F. Svensson, J. Med. Chem., 2020, 63, 10158–10169, DOI:10.1021/acs.jmedchem.9b02126.
- M. W. Mullowney, K. R. Duncan, S. S. Elsayed, N. Garg, J. J. van der Hooft, N. I. Martin, D. Meijer, B. R. Terlouw, F. Biermann and K. Blin, Nat. Rev. Drug Discovery, 2023, 1–22, DOI:10.1038/s41573-023-00774-7.
- Y. Song, R. Y. Tay, J. Li, C. Xu, J. Min, E. Shirzaei Sani, G. Kim, W. Heng, I. Kim and W. Gao, Sci. Adv., 2023, 9, eadi6492, DOI:10.1126/sciadv.adi6492.
- S. Kalasin and W. Surareungchai, Anal. Chem., 2023, 95, 1773–1784, DOI:10.1021/acs.analchem.2c02642.
- J. R. Sempionatto, J. A. Lasalde-Ramírez, K. Mahato, J. Wang and W. Gao, Nat. Rev. Chem, 2022, 6, 899–915, DOI:10.1038/s41570-022-00439-w.
- C. Ma, Z. Xia, M. D. Sacco, Y. Hu, J. A. Townsend, X. Meng, J. Choza, H. Tan, J. Jang and M. V. Gongora, J. Am. Chem. Soc., 2021, 143, 20697–20709, DOI:10.1021/jacs.1c08060.
- M. K. Shave, S. Kalasin, E. Ying and M. M. Santore, ACS Appl. Mater. Interfaces, 2018, 10, 29058–29068 CrossRef CAS PubMed.
- S. Kalasin and M. M. Santore, Macromolecules, 2016, 49, 334–343, DOI:10.1021/acs.macromol.5b01977.
- S. Kalasin and M. M. Santore, ACS Nano, 2015, 9, 4706–4716, DOI:10.1021/nn505322m.
- Y. Wen, X. Guo, S. Kalasin and M. M. Santore, Langmuir, 2014, 30, 2019–2027, DOI:10.1021/la404235g.
- S. Kalasin, S. Martwiset, E. B. Coughlin and M. M. Santore, Langmuir, 2010, 26, 16865–16870, DOI:10.1021/la103023t.
- N. H. Bhuiyan, J. H. Hong, M. J. Uddin and J. S. Shim, Anal. Chem., 2022, 94, 3872–3880, DOI:10.1021/acs.analchem.1c04827.
- L. Wang, P. Jia, T. Huang, S. Duan, J. Yan and L. Wang, Sensors, 2016, 16, 1275, DOI:10.3390/s16081275.
- S. Zare Harofte, M. Soltani, S. Siavashy and K. Raahemifar, Small, 2022, 18, 2203169, DOI:10.1002/smll.202203169.
- A. Isozaki, J. Harmon, Y. Zhou, S. Li, Y. Nakagawa, M. Hayashi, H. Mikami, C. Lei and K. Goda, Lab Chip, 2020, 20, 3074–3090, 10.1039/D0LC00521E.
- Z. Xing, S. Zhao, W. Guo, F. Meng, X. Guo, S. Wang and H. He, Energy, 2023, 285, 128771, DOI:10.1016/j.energy.2023.128771.
- Y. Wu, S. Zhao, Z. Xing, Z. Wei, Y. Li and Y. Li, IEEE Trans. Power Delivery, 2023, 38, 3551–3560, DOI:10.1109/TPWRD.2023.3279891.
- F. Gentile, V. Agrawal, M. Hsing, A.-T. Ton, F. Ban, U. Norinder, M. E. Gleave and A. Cherkasov, ACS Cent. Sci., 2020, 6, 939–949, DOI:10.1021/acscentsci.0c00229.
- D. M. Anstine and O. Isayev, J. Am. Chem. Soc., 2023, 145, 8736–8750, DOI:10.1021/jacs.2c13467.
- M. S. Cooper, L. Zhang, M. Ibrahim, K. Zhang, X. Sun, J. Röske, M. Göhl, M. Brönstrup, J. K. Cowell and L. Sauerhering, J. Med. Chem., 2022, 65, 13328–13342, DOI:10.1021/acs.jmedchem.2c01131.
- K. Arafet, N. Serrano-Aparicio, A. Lodola, A. J. Mulholland, F. V. González, K. Świderek and V. Moliner, Chem. Sci., 2021, 12, 1433–1444, 10.1039/D0SC06195F.
- Y. Unoh, S. Uehara, K. Nakahara, H. Nobori, Y. Yamatsu, S. Yamamoto, Y. Maruyama, Y. Taoda, K. Kasamatsu and T. Suto, J. Med. Chem., 2022, 65, 6499–6512, DOI:10.1021/acs.jmedchem.2c00117.
- E. Arutyunova, A. Belovodskiy, P. Chen, M. B. Khan, M. Joyce, H. Saffran, J. Lu, Z. Turner, B. Bai and T. Lamer, ACS Bio Med Chem Au, 2023, 3, 528–541, DOI:10.1021/acsbiomedchemau.3c00039.
- B. Boras, R. M. Jones, B. J. Anson, D. Arenson, L. Aschenbrenner, M. A. Bakowski, N. Beutler, J. Binder, E. Chen and H. Eng, Nat. Commun., 2021, 12, 6055, DOI:10.1038/s41467-021-26239-2.
- K. Sa-Ngiamsuntorn, A. Suksatu, Y. Pewkliang, P. Thongsri, P. Kanjanasirirat, S. Manopwisedjaroen, S. Charoensutthivarakul, P. Wongtrakoongate, S. Pitiporn and J. Chaopreecha, J. Nat. Prod., 2021, 84, 1261–1270, DOI:10.1021/acs.jnatprod.0c01324.
- P. Kanjanasirirat, A. Suksatu, S. Manopwisedjaroen, B. Munyoo, P. Tuchinda, K. Jearawuttanakul, S. Seemakhan, S. Charoensutthivarakul, P. Wongtrakoongate and N. Rangkasenee, Sci. Rep., 2020, 10, 19963, DOI:10.1038/s41598-020-77003-3.
- M. J. Abraham, T. Murtola, R. Schulz, S. Páll, J. C. Smith, B. Hess and E. Lindahl, SoftwareX, 2015, 1, 19–25, DOI:10.1016/j.softx.2015.06.001.
- J. Tirado-Rives and W. L. Jorgensen, J. Chem. Theory Comput., 2008, 4, 297–306, DOI:10.1021/ct700248k.
- A. Nash, T. Collier, H. L. Birch and N. H. de Leeuw, J. Mol. Model., 2018, 24, 1–11, DOI:10.1007/s00894-017-3530-6.
- A. Pavlova, J. M. Parks and J. C. Gumbart, J. Chem. Theory Comput., 2018, 14, 784–798, DOI:10.1021/acs.jctc.7b01236.
- M. Biasini, S. Bienert, A. Waterhouse, K. Arnold, G. Studer, T. Schmidt, F. Kiefer, T. G. Cassarino, M. Bertoni and L. Bordoli, Nucleic Acids Res., 2014, 42, W252–W258, DOI:10.1093/nar/gku340.
- N. K. Manaswi and N. K. Manaswi, Deep Learning with Applications Using Python: Chatbots and Face, Object, and Speech Recognition with TensorFlow and Keras, 2018, pp. 31–43, DOI:10.1007/978-1-4842-3516-4_2.
- X. Chen, L. Yang, R. P. Dick, L. Shang and H. Lekatsas, IEEE Trans. Very Large Scale Integr. Syst., 2009, 18, 1196–1208, DOI:10.1109/TVLSI.2009.2020989.
- A. Kotobi, K. Singh, D. Höche, S. Bari, R. H. Meißner and A. Bande, J. Am. Chem. Soc., 2023, 145, 22584–22598, DOI:10.1021/jacs.3c07513.
- G. I. Premarathna and L. Ellingson, PLoS One, 2021, 16, e0244905, DOI:10.1371/journal.pone.0244905.
- J. Eberhardt, D. Santos-Martins, A. F. Tillack and S. Forli, J. Chem. Inf. Model., 2021, 61, 3891–3898, DOI:10.1021/acs.jcim.1c00203.
- A. Kabylda, V. Vassilev-Galindo, S. Chmiela, I. Poltavsky and A. Tkatchenko, Nat. Commun., 2023, 14, 3562, DOI:10.1038/s41467-023-39214-w.
- Z. Zou, Y. Zhang, L. Liang, M. Wei, J. Leng, J. Jiang, Y. Luo and W. Hu, Nat. Comput. Sci., 2023, 3, 957–964, DOI:10.1038/s43588-023-00550-y.
- A. Daina, O. Michielin and V. Zoete, Sci. Rep., 2017, 7, 42717, DOI:10.1038/srep42717.
- D. E. Pires, T. L. Blundell and D. B. Ascher, J. Med. Chem., 2015, 58, 4066–4072, DOI:10.1021/acs.jmedchem.5b00104.
- D. F. Veber, S. R. Johnson, H.-Y. Cheng, B. R. Smith, K. W. Ward and K. D. Kopple, J. Med. Chem., 2002, 45, 2615–2623, DOI:10.1021/jm020017n.
- C. A. Lipinski, Adv. Drug Delivery Rev., 2016, 101, 34–41, DOI:10.1016/j.addr.2016.04.029.
- A. K. Ghose, V. N. Viswanadhan and J. J. Wendoloski, J. Comb. Chem., 1999, 1, 55–68, DOI:10.1021/cc9800071.
- W. J. Egan, K. M. Merz and J. J. Baldwin, J. Med. Chem., 2000, 43, 3867–3877, DOI:10.1021/jm000292e.
- I. Muegge, S. L. Heald and D. Brittelli, J. Med. Chem., 2001, 44, 1841–1846, DOI:10.1021/jm015507e.
- N. Sawang, S. Phongphanphanee, J. Wong-Ekkabut and T. Sutthibutpong, J. Phys. Chem. B, 2023, 127, 2331–2343, DOI:10.1021/acs.jpcb.2c08312.
- S. Rajpoot, M. Alagumuthu and M. S. Baig, Curr. Res. Struct. Biol., 2021, 3, 9–18, DOI:10.1016/j.crstbi.2020.12.001.
- A. Mishra and A. S. Rathore, J. Biomol. Struct. Dyn., 2022, 40, 6039–6051, DOI:10.1080/07391102.2021.1875886.
|
This journal is © The Royal Society of Chemistry 2024 |
Click here to see how this site uses Cookies. View our privacy policy here.