DOI:
10.1039/D4SC02058H
(Edge Article)
Chem. Sci., 2024,
15, 15698-15712
Precise redesign for improving enzyme robustness based on coevolutionary analysis and multidimensional virtual screening†
Received
28th March 2024
, Accepted 27th June 2024
First published on 6th September 2024
Abstract
Natural enzymes are able to function effectively under optimal physiological conditions, but the intrinsic performance often fails to meet the demands of industrial production. Existing strategies are based mainly on the evaluation and subsequent combination of single-point mutations; however, this approach often suffers from a limited number of designable residues and from low accuracy. Here, we propose a strategy (Co-MdVS) based on coevolutionary analysis and multidimensional virtual screening for precise design to improve enzyme robustness, employing nattokinase as a model. Using this strategy, we efficiently screened 8 dual mutants with enhanced thermostability from a virtual mutation library containing 7980 mutants. After further iterative combination, the optimal mutant M6 exhibited a 31-fold increase in half-life at 55 °C, significantly enhanced acid resistance, and improved catalytic efficiency with different substrates. Molecular dynamics simulations indicated that the reduced flexibility of thermal and acid-sensitive regions resulted in a significantly increased robustness of M6. Furthermore, the potential of multidimensional virtual screening in enhancing design precision has been validated on L-rhamnose isomerase and PETase. Therefore, the Co-MdVS strategy introduced in this research may offer a viable approach for developing enzymes with enhanced robustness.
1. Introduction
Enzymes, as natural biocatalysts, have substantial potential for industrial application due to their high specificity, efficiency, and sustainability.1,2 While natural enzymes can function effectively under optimal physiological conditions, their inherent performance is often insufficient for the rigorous demands imposed by industrial processes.3,4 To overcome this limitation, several molecular engineering strategies have been employed. Directed evolution has proven to be an effective approach for enhancing enzyme performance.5–7 Nevertheless, the widespread utilization of this strategy has been impeded by the need to develop proprietary high-throughput screening systems for various enzymes and perform subsequent extensive screening work.8–10 The mechanism-driven strategy of rational or semi-rational design has been widely and effectively used in enzyme engineering,11–13 allowing for virtual screening based on extensive computations to reduce the experimental workload.14,15 However, the precise targeting of key residues for enzyme functions is a critical issue for reliable design.
Proteins from different organisms can be identified as homologs through sequence comparison, as evolutionary constraints restrict substitutions at specific positions.16 Coevolving residues often correspond to positions in a protein that have a significant impact on its stability.17,18 Therefore, utilizing the coevolutionary relationships between protein residues can more accurately reveal key residues that affect enzyme functions. Moreover, coevolving residues occur in pairs, providing a convenient method for designing combinations of mutations. Therefore, the coevolution of residues can be exploited to design algorithms for the efficient targeting of hotspot regions and contribute to the design of combinatorial mutations. However, faced with the enormous size of mutant libraries, there is an urgent need for more accurate and labor-saving methods for screening virtual mutations. The computation of free energies in biological processes, such as protein folding, protein–ligand binding, and protein–protein binding, is crucial for protein design due to its direct impact on protein stability.19–21 Introducing mutations that decrease the folding free energy (ΔΔG) has been shown to effectively increase the melting temperature, stabilize the protein structure, and therefore extend the half-life (t1/2) at high temperatures.22–24 However, extensive research has shown that using ΔΔG as a static indicator is insufficient for accurately screening highly stable mutants.23,25,26 Previous reports have demonstrated that dynamic simulation indicators, such as the root mean square deviation (RMSD), the radius of gyration (Rg) and the total hydrogen bonds (H-bonds), are related to protein structural stability,11,27,28 and mutants with a decreased RMSD, decreased Rg, or an increased number of total H-bonds in comparison to that of the wild type (WT) often exhibit greater robustness.14,26,29,30 Therefore, by combining the identification of coevolving residue pairs with multidimensional virtual screening, it is possible to systematically construct hybrid strategies that facilitate the identification of elusive combination mutations and reduce the workload.
Nattokinase (NK), a potent fibrinolytic enzyme, is a Ca2+-dependent serine protease belonging to the subtilisin family and has great potential in the food and pharmaceutical industries.29 However, NK rapidly loses activity at high temperatures (above 55 °C) and under acidic conditions, while encountering a frequent trade-off between enzymatic activity and stability during the modification process; these limitations restrict its industrial applications.11,29,31,32 Although traditional screening methods that involve a heavy workload have improved the robustness of NK to some extent, the development of novel approaches for increasing enzyme performance remains worthwhile.
In this study, we developed a strategy combining the identification of coevolving residues and multidimensional virtual screening, namely Co-MdVS, for accurate and efficient design to enhance NK robustness and greatly reduce the experimental workload. Furthermore, the MdVS method was also found to be suitable for screening for high-robustness mutants of other enzymes. Based on the successful application of Co-MdVS, we anticipate that Co-MdVS shows promise in improving the robustness of enzymes.
2. Experimental section
2.1. Bacterial strains, plasmids, and chemicals
The bacterial strains and plasmids utilized in this study are presented in Table S1.†Escherichia coli JM109 was employed for plasmid construction and propagation. B. subtilis comK was employed for the secretory expression of NK.33 The cells were cultured in Luria-Bertani (LB) medium consisting of 10 g L−1 tryptone, 5 g L−1 yeast extract, 10 g L−1 NaCl, pH 7.0. For enzyme expression, 2× YT medium containing 16 g L−1 tryptone, 10 g L−1 yeast extract, 5 g L−1 NaCl, pH 7.0 was used. The concentrations of ampicillin and kanamycin were 100 μg mL−1 and 50 μg mL−1, respectively. Tryptone and yeast extract were purchased from Thermo Fisher Scientific (Waltham, MA, USA), and ampicillin and kanamycin were purchased from Sangon Biotech (Shanghai, China). Succinyl-Ala-Ala-Pro-Phe-p-nitroanilide (Suc-AAPF-pNA) was purchased from GlpBio Technology (Montclair, CA, USA). 20 L-type amino acid standards were purchased from Sigma (Merck KGaA, Darmstadt, Germany). All other chemicals not specifically mentioned were purchased from Sinopharm Chemical Reagent Co. (Shanghai, China).
2.2. Site-directed mutagenesis
All plasmids were constructed using the Gibson assembly-based strategy previously described.34 The secretory expression plasmid of wild-type NK (pBp43-WapA-NK-ter) was used as a template to construct mutants through full-length plasmid PCR. PCR was performed using PrimeSTAR Max DNA Polymerase (Takara, Dalian, China). The primers were synthesized by GENEWIZ Co., Ltd (Suzhou, China) (Table S2†). PCR products were treated with QuickCutTM DpnI (Takara, Dalian, China) to remove the DNA templates, and then purified using a DNA Gel Extraction Kit (Cowin Biotech Co., Ltd, Jiangsu, China). The purified DNA fragments were seamlessly ligated using ABclonal MultiF Seamless Assembly Mix (ABclonal Technology Co. Ltd, China), and then transformed into E. coli JM109. The constructed plasmids were confirmed by sequencing (GENEWIZ Co., Ltd Suzhou, China).
2.3. Secretory expression and purification of NK
Single colonies were inoculated into a test tube containing 5 mL 2× YT medium. and cultured at 37 °C for 24 hours. After overnight culture at 37 °C with shaking at 200 rpm, 1 mL of seed liquid was transferred into 250 mL shake flasks containing 50 mL of 2× YT medium, and further incubated at 37 °C with shaking at 200 rpm. The supernatant was harvested by centrifugation at 12
000 rpm for 5 min. The wild-type and mutant proteins M5 and M6 were purified by precipitation with ammonium sulfate. The target protein was obtained in the 20–60% saturation range and solubilized with 100 mM Tris–HCl (pH 8.0, 0.2 mM CaCl2). The ammonium sulfate was removed by dialysis in 100 mM Tris–HCl (pH 8.0, 0.2 mM CaCl2) buffer. The purity of the purified enzymes was assessed using SDS-PAGE analysis. The protein concentration was determined using the Bradford method.
2.4. Determination of enzymatic parameters
Measurement of enzyme specific activity, the ability to degrade fiber plates and fibrinolytic activity.
The specific activity of NK was measured according to Luo et al.11 In this assay, 40 μL of the NK sample (fermentation broth supernatant or purified NK) and 80 μL of the N-Succinyl-Ala-Ala-Pro-Phe p-nitroanilide (Suc-AAPF-pNA) substrate at a final concentration of 0.4 mM were incubated in 80 μL of buffer (pH 8.0, 100 mM Tris–HCl, and 0.2 mM CaCl2). The mixture was then subjected to analysis using a microplate reader (BioTek Instruments, Inc. Winooski, Vermont, USA) at 405 nm and 25 °C for 5 minutes, with p-NA content measured every minute. One unit of enzyme activity was defined as the amount of enzyme that produced 1 μmol of p-NA per minute under the assay conditions. The specific enzyme activity, expressed as units of enzyme activity per milligram of enzyme, was calculated based on the total number of units. Protein concentration was determined using the Bradford method.
Prepare a 1% fibrinogen solution (Solarbio Life Science Biotechnology Co., Ltd Beijing, China) in 10 mL using 50 mM borate buffer (pH 8.5, 50 mM Na2B4O7, 0.15 M NaCl), and then add 0.5 mL of 20 U mL−1 thrombin. Mix well and pour the mixture into a plastic Petri dish with a 30 mm diameter. Incubate at 37 °C for 10 minutes, and then place a 5 mm inner diameter Oxford cup in each Petri dish. Subsequently, add 200 μL of a 0.3 mg mL−1 enzyme solution into each Oxford cup. Incubate at 37 °C for 12 hours, and then photograph and measure the area (ImageJ NIH, USA) of the hydrolysis zone.
NK fibrinolytic activity was measured according to Liu et al.29 In this assay, 1.4 mL of Tris–HCl (50 mM, pH 8.0, 0.2 mM CaCl2) and 0.4 mL of a 0.72% fibrinogen solution were preincubated at 37 °C for 5 minutes. Then, 0.1 mL of a 20 U mL−1 thrombin solution (Solarbio Biotechnology Co., Ltd Beijing, China) was added, followed by the addition of 0.1 mL of purified NK after 10 minutes. Fibrinogen and thrombin were solubilized with 0.05 mol L−1 borate buffer (pH 8.5). The mixture was incubated at 37 °C for one hour. After that, a trichloroacetic acid solution (0.2 M) was added, and the reaction was stopped by incubating at 37 °C for 20 minutes. The mixture was then centrifuged (15
000 rpm, 5 min), and the absorbance of the supernatant was measured at 275 nm. One unit (1 FU) of fibrinolytic activity was defined as the amount of enzyme that increased the absorbance of the filtrate at 275 nm by 0.01 per minute. Fibrinolytic activity was analyzed independently in triplicate, and the data are presented as mean ± standard deviation.
Determination of the hydrolysis degree of casein.
Initially, 10 g of casein sample (J & K Scientific, Beijing, China) is accurately weighed, followed by the addition of a solution containing either WT or mutant NK. This solution is composed of 100 mM Tris–HCl (pH 8.0, 0.2 mM CaCl2), to reach a final volume of 50 mL. It is essential to maintain the ratio of NK to casein in the mixture at 0.5% (w/w). The mixture was subjected to enzymatic digestion by incubating at a constant temperature of 37 °C and 55 °C with agitation at 250 rpm in a shaking incubator for 6 hours. The samples were taken every hour and treated with a final concentration of 0.2 mM PMSF to inactivate the NK. Subsequently, the mixture is centrifuged at 4 °C and 12
000 rpm for 20 minutes to collect the supernatant for further analysis.
In this study, the degree of protein hydrolysis (DH) is quantified using the ninhydrin colorimetric method.35 The specific protocol is as follows: 1% ninhydrin reagent solution is prepared using anhydrous ethanol as the solvent. A 200 mM amino acid standard mixture solution is then prepared and serially diluted to final concentrations of 0, 1, 2, 3, 4, and 5 mM. Next, 100 μL of each hydrolysis solution is taken and combined with 50 μL of the 1% ninhydrin reagent, thoroughly mixed, and then heated at 100 °C for a duration of 10 minutes to initiate the color development reaction. The absorbance of the reaction mixture is measured at a wavelength of 570 nm immediately following the heating process. The quantity of newly formed peptide bonds in the hydrolysate is calculated by comparison with the amino acid standard curve. This experimental value is compared to the innate peptide bond content of casein (8.2 mM g−1) to ascertain the DH. This ratio indicates the proportion of new peptide bonds formed through the hydrolysis of casein relative to the original peptide bonds, thereby reflecting the extent of protein degradation achieved.
Measurement of residual activity, half-life, and apparent melting temperatures.
The residual activity was defined as the percentage of crude NK activity that remained after incubation at 65 °C for 30 minutes. Briefly, 1 mL of crude enzyme supernatant was incubated at 65 °C for 30 minutes. After incubation, the crude enzyme activity of the supernatant was measured, and the percentage of residual enzyme activity was calculated.
For the determination of thermostability, the half-life period (t1/2) of purified WT, M5 and M6 mutants was determined by monitoring the time quantum of the half residual activity after incubation at 55 °C. 1 mL Tris–HCl (100 mM, pH 8.0, 0.2 mM CaCl2) containing 0.2 mg mL−1 purified WT and mutants were incubated at 55 °C, and the total enzyme activity was detected every 30 minutes until no enzyme activity was detected.
Apparent melting temperatures were determined using a fluorescence-based thermal stability assay.11 The purified NK solution (20 μL) was added to buffer (100 mM Tris–HCl and 0.2 mM CaCl2, pH 8.0) for a final concentration of 0.2 mg mL−1. The solution was mixed with 5 μL of 100-fold diluted SYPRO Orange dye (Molecular Probes, Life Technologies, USA). The solution was mixed in low-profile PCR tubes (8-tube strips, white) with optical flat 8 caps (Bio-Rad Laboratories, Inc, USA) and heated in a StepOnePlus™ PCR (Thermo Fisher Scientific, Applied Biosystems, USA) from 40 to 85 °C at a heating rate of 1% per minute. The changes in fluorescence were monitored with a built-in detector, and the data from the melting region derivative were obtained using a StepOnePlus™ Real-Time PCR system.
Determination of the tolerance of NK to different pH values.
Use seven gradient reaction solutions with pH values of 2.5, 3.0, 3.5, 4.0, 4.5, 5.0, and 5.5, to create NK (0.5 mg mL−1) working solutions. After incubating the solutions at 37 °C for 30 minutes, adjust the pH of each reaction solution to 8.0. Subsequently, measure the enzymatic activity of fibrinogen in the reaction solutions subjected to different pH treatments and calculate the residual enzymatic activity.
Kinetics characterization.
The model substrate Suc-AAPF-pNA was prepared at ten different concentrations (0.08, 0.16, 0.32, 0.64, 0.96, 1.28, 1.60, 3.20, 6.40, 9.6, and 12.8 mM) and used to evaluate the Michaelis–Menten kinetics of WT and variants. In addition, the specific activity of each substrate was measured at 25 °C after maintaining the concentration of the purified enzyme at 0.005 mg mL−1 (100 mM Tris–HCl and 0.2 mM CaCl2, pH 8.0). The experimental data were fitted using GraphPad Prism 8.00 (GraphPad Software Co., USA).
2.5. Evolutionary coupling analysis
Evolutionary coupling amino acid residue pairs were predicted by an online software EVcouplings server (http://evfold.org).36 In this study, EVcouplings was utilized for homologous sequence searches using the UniRef90 database. UniRef90 is a widely used non-redundant sequence database, comprising sequences with less than 90% similarity, thereby reducing redundancy and enhancing alignment quality. Iterative searches were conducted using JackHMMER for five iterations. Subsequently, homologous sequences were filtered based on an E-value threshold of 1 × 10−5, the minimum sequence coverage threshold: 80%, and the maximum sequence redundancy threshold: 90%. Eventually, evolutionarily coupled residue pairs were identified from the 62
032 filtered sequences. Based on the computational results, residues with a Bitscore of 0.5 are considered reliable coevolutionary analysis data. Therefore, we selected the top 20 residue pairs based on the strength of coevolutionary coupling from this dataset for subsequent analysis.
2.6. Virtual screening based on folding free energy evaluation
Three-dimensional structures of NK and its mutants were obtained using the Schrödinger software Maestro module based on the crystal structure of NK (PDB: 4DWW)37 and the structures were optimized by using the Rosetta FastRelax module.38,39 Prediction of changes in the ΔΔG of mutations was performed by a customizable Python wrapper RosettaDDGPrediction based on the Rosetta cartesian_ddg module.40,41 For each residue, mutagenesis to the other 19 typical amino acids was allowed, resulting in a total of 399 saturated combinatorial mutations that need to be screened for each coevolutionary residue pair. Candidate mutants were screened out based on ΔΔG (Rosetta Energy Unit, REU), and those of less than −1.6 REU were considered as potential positive mutations. The scoring weights of ref2015_cart were used to calculate the ΔΔG.42 The iterations were set to 5 (5 independent mutation calculations were performed), and the results were the average of the 5 calculations to ensure the accuracy of the predictions (SI_Scripts_for_ddG_Prediction).
2.7. MD simulation and analysis
In this study, MD simulations were performed using NAMD 2.14 software with the CHARMM36m force field.43,44 The initial structures (PDB ID:4DWW45) were optimized using the FastRelax module of Rosetta 2021-16 and used as the input for the MD simulations. PDB2PQR (https://server.poissonboltzmann.org/) was used to determine the protonation state of residues at different pH values.46 The MD simulations were carried out in a rectangular TIP3P explicit solvent box with a distance of 10 Å between the solute and the box edge. To neutralize the system, 0.15 M Na+ and Cl− ions were added. A time step of 2 fs was used, and a cutoff of 12 Å was applied for non-bonded interactions. Periodic boundary conditions and particle mesh Ewald were used during the MD simulation. The complete MD simulation protocol consisted of a 1 ns equilibration under an NVT ensemble, heating the system from 0 K to the target temperature with a gradient of 5 K, and a 50 ns production MD simulation under the NPT ensemble. For MdVS, 20 ns production MD simulations at 400 K were used to reduce the computational cost.
Determination of the protonation state of residues.
The online tool PDB2PQR (https://server.poissonboltzmann.org/pdb2pqr)47 was used to predict the protonation state of Asp, Glu, and His at pH 3 or 7. The CHARMM force field was applied, with the output also in the CHARMM force field format. PROPKA48 was used to assign protonation states at provided pH values. Details of the protonation state of each residue at pH 3 or 7 are listed in Table S8.†
RMSD analysis.
The RMSD of the protein backbone was calculated using the RMSD Trajectory Tool, a plugin for VMD, using the initial structure of the MD simulation as a reference.
R
g analysis.
The Rg of the protein backbone was calculated using a set of homemade scripts that were generously provided by Professor Lukasz Peplowski at Nicolaus Copernicus University in Toruń, Poland (SI_Scripts_for_Rg_calculation).
H-bond analysis.
H-bond formation in the MD simulation was evaluated using hydrogen bonds, a plugin for VMD. Only polar atoms (N, O, S, and F) were included; the donor–acceptor distance cut-off was set to 3.0 angstroms and the angular cut-off to 20°.
Root mean square fluctuation (RMSF) analysis.
The RMSF of the Cα atom of the protein was analyzed using the Bio3D package, an R package for structural bioinformatics.49
2.8. Statistical analysis
All experiments were performed in triplicate by independent investigators;the results are expressed as mean ± SD (n = 3). The significance of the experimental test data was analysed using SPSS19.0 (IBM Corporation, New York. USA), and one-way ANOVA analysis was performed using Duncan's test at the 5% level. The parameter of MD simulations employed the Kruskal–Wallis test for analysis at the 5% level.
3. Results
3.1. Proposal and general procedure for Co-MdVS
Mechanism-based rational design is an important approach for enhancing enzyme properties. In contrast to directed evolution, rational design does not rely on expensive high-throughput screening equipment and can reduce labor costs through efficient calculations.25,50–52 However, there are still several bottlenecks associated with effective rational design, including efficient targeting of functional residues, rapid and accurate evaluation of the effects of mutations, and effective iterative combinations. Here, we present a strategy Co-MdVS for the direct design of combinatorial mutations that begins with identifying hotspot residue pairs for enzyme engineering based on coevolutionary analysis, followed by multidimensional virtual screening of combinatorial saturation mutations and, finally, validation and further combination of the screened mutants (Fig. 1).
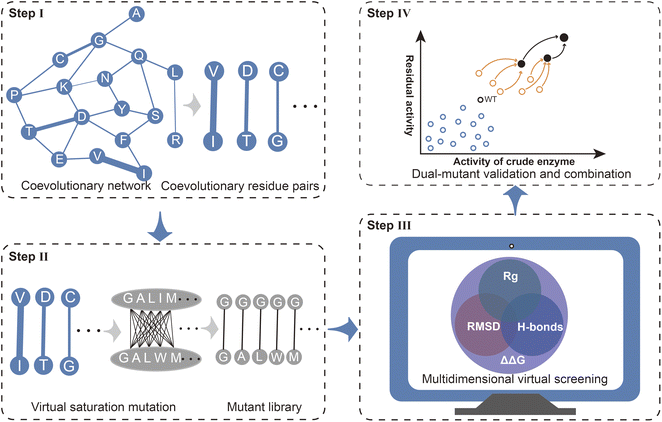 |
| Fig. 1 General procedure of the strategy used in this study. (Step I) Analysis of the coevolutionary residue pairs. (Step II) Virtual saturation mutant library generation based on the coevolutionary residue pairs. (Step III) Potential positive mutants screened through the multidimensional virtual screening method. (Step IV) Experimental verification and further combination of dual-mutants. | |
Coevolutionary analysis is an important method for identifying functional residues and predicting the protein structure by extracting information about residue pairs that are spatially and functionally closely related.17,53 Therefore, in this study, coevolutionary analysis was used to identify hotspot residue pairs in Step I. Virtual saturation mutagenesis was subsequently performed on the residue pairs with strong evolutionary coupling, yielding a virtual mutant library in Step II. Although several tools have been developed for the evaluation of mutations in proteins, high-precision design remains a challenge. The calculation of ΔΔG based on energy functions tends to be limited on a spatial scale by considering only interactions between mutated residues and nearby residues. MD simulation is a useful method for resolving the effects of mutations on structural dynamics at the cost of high computational requirements. To balance design efficiency and accuracy, in Step III, we used a MdVS strategy of first filtering out undesirable mutations using ΔΔG, followed by identifying beneficial mutations using multiple indicators (RMSD, Rg, and H-bonds) derived from MD simulations. In Step IV, we conducted mutant validation and iterative combination.
3.2. Construct a virtual mutant library and determine potentially beneficial mutations
The EVcoupling server was utilized for the identification of coevolving residue pairs in NK.36 Coevolutionary information was derived from the analysis of 62
032 sequences. In total, 253 residues of NK were analyzed (Fig. 2a and b), and the top 20 coevolving residue pairs were selected based on their score values (Table S3†). By mapping these 20 residue pairs onto the 3D structure of NK, we observed that 11 residue pairs were situated near the catalytic triad (Asp32, His64, and Ser221), while the remaining 9 pairs were located on the opposite side (Fig. 2c). Notably, the residues belonging to each pair were close in space, with distances between the Cα atoms ranging from 4.1 to 8.4 Å (Table S3†). The interactions between these residue pairs were further examined, and the results are shown in Fig. S1.† Specifically, T22-S87 and D140-S173 formed a hydrogen bond (H-bond), and E112-K141 formed both an H-bond and a salt bridge. Additionally, V28-I72, V93-W113, A169-A176, A179-F189, A187-V203, A230-I268, and A230-A270 engaged in nonpolar interactions. The remaining residue pairs did not exhibit well-defined interactions. Virtual saturation mutagenesis was performed on the 20 selected coevolving residue pairs to construct a mutant library.
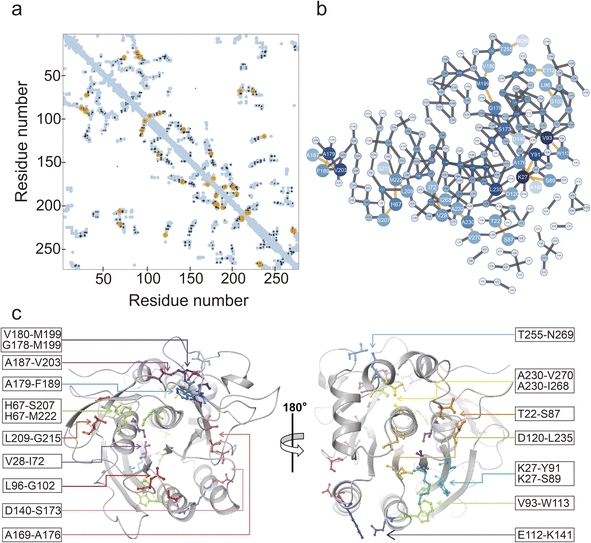 |
| Fig. 2 Characterization of coevolutionary residue pairs. (a) Contact map of the coevolutionary residue pairs in the NK sequence. Yellow represented the top 20 residue pairs with a strong coevolution relationship. (b) Coevolution relationship network among the residues. Yellow lines represented the top 20 residue pairs with a strong coevolution relationship. (c) The specific positions of the 20 coevolutionary residue pairs in the three-dimensional structure of NK. | |
Experimental validation of the sheer number of possible combinations resulting from saturated combinatorial mutations on 20 coevolving residue pairs, 7980 in total, would be prohibitive. Because screening potential stability-enhancing mutations based on a single indicator has limitations, we combined a static indicator (ΔΔG) with dynamic indicators (RMSD, Rg, and total H-bonds) to guide the MdVS of potentially beneficial mutants.11,27,30,54 To facilitate this process, virtual saturation mutagenesis and calculation of the ΔΔG (Cartesian_ddg) values for the variants were performed with a high-throughput mutational scan python wrapper called RosettaDDGPrediction, which utilizes the Rosetta software Cartesian_ddg module.40 Subsequently, the ΔΔG values were calculated for 20 pairs of coevolving residues, resulting in a comprehensive assessment of their potential effects on stability (Fig. S2†). Based on the calculation results and previous reports, a threshold of −1.6 REU was set to identify potentially beneficial mutations, and any mutations below this value were considered for further analysis.11,24 Consequently, a total of 80 mutants from 8 pairs of coevolving residues were selected based on their ΔΔG values (Fig. S2 and Table S4†). MD simulation was next proposed to evaluate the effect of mutations on NK thermostability from a structural dynamic perspective. However, since it is computationally intensive, we excluded mutations with similar polarity and spatial hindrance,55 and finally focused on 40 mutants. (Table S4†). Afterwards, we performed 20 ns conventional MD simulations on 40 selected mutants at 400 K and calculated the RMSD, Rg, and total H-bonds of the NK structure during the simulation process (Fig. 3a and Table S5†). The ΔΔG, RMSD, Rg, and total H-bonds are often used to characterize the stability of protein structures;11,19,28,56 surprisingly, however, further analysis revealed no significant correlations among them (Fig. S3†). These results demonstrate that it is difficult to accurately evaluate mutants based on a single indicator; therefore, by combining the four indicators, beneficial mutants can be predicted multidimensionally, greatly increasing the accuracy and efficiency of screening. Based on the differences in these three dynamic indicators, we categorized the 40 mutants into three groups for further analysis. The first group consisted of the top 20 mutants with a decreased RMSD, the second group consisted of the top 20 mutants with decreased Rg, and the third group consisted of the top 20 mutants with increased total H-bonds. We constructed a Venn diagram to analyze the similarities and differences among these three groups of mutants (Fig. 3b). Notably, 8 dual mutants overlapped in three groups, namely, T22FS87L, T22FS87W, T22YS87L, T22YS87Y, K27NS89Y, K27YS89L, K27YS89Y, and H67AS207V (Fig. 3b and Table S6†). These eight mutants were considered to have high potential to increase robustness. According to the Venn diagram, 8 mutants that did not belong to any of the three groups were excluded (Fig. 3b and Table S6†). To further evaluate whether this strategy can accurately predict a mutant with increased robustness, the remaining 24 mutants included in a single group or in only two of the three groups were also constructed and characterized (Fig. 3b and Table S6†).
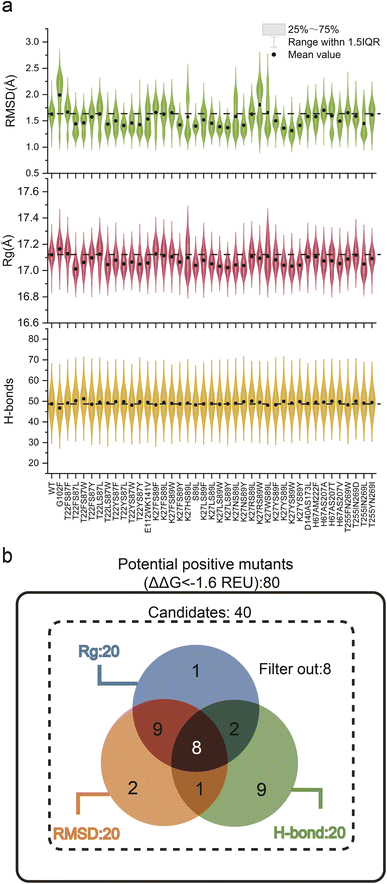 |
| Fig. 3 Multidimensional analysis of the dynamic indicators of the selected 40 mutants. (a) Comparison of the calculated RMSD, Rg, and H-bonds among the WT and the mutants. The closed circles showed the mean values of each indicator. The black dashed line marked the value of WT. (b) Analysis of the commonalities among three groups by using a Venn diagram. The top 20 mutants of the Rg group, RMSD group and H-bond group were shown in a blue circle, orange circle and green circle, respectively, and the number of the mutants overlapped in two and three groups was also shown. 8 mutants were not included in any group. | |
3.3. Characterization and iteration of combinatorial mutations
To assess the potential for increased catalytic activity and robustness, the activity of the crude enzyme and the residual activity after heat treatment were measured for the mutants. All of the eight variants identified by the intersection of three dynamic indicators exhibited higher thermostability than the WT (Fig. 4a). Specifically, the T22FS87L and T22YS87L mutants exhibited significantly higher residual activity than did the WT (Fig. 4a). Surprisingly, none of the remaining 24 mutants displayed a thermostability that was significantly greater than that of the WT. The T255IN269D mutant exhibited a marked increase in enzymatic activity compared to the WT, while its thermostability remained comparable (Fig. 4a). These findings demonstrate the effectiveness and feasibility of MdVS to identify thermostability-increasing mutations.
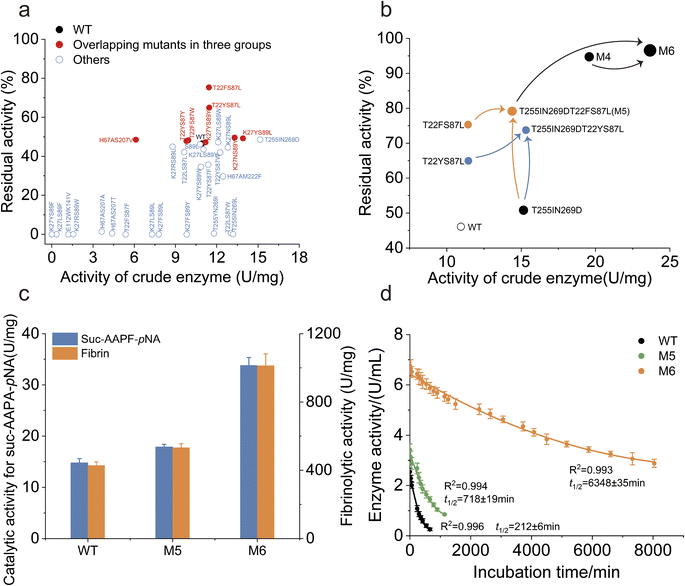 |
| Fig. 4 Validation iterative combination and characterization of mutants. (a) Determination of the thermostability and activity. The overlapping mutants in three groups were shown in red dots, and the others were shown in black circles. (b) Characterization of the mutants and the combination schedule. (c) Comparison of the enzymatic activities of purified WT and mutants catalyzing suc-AAPF-pNA and fibrin. (d) Characterization of the half-life of purified mutants. | |
Since T22FS87L and T22YS87L involve the same pair of coevolving residues, they were separately combined with the mutant T255IN269D to further increase the performance of NK. Between the two combined mutants, T22FS87LT255IN269D (referred to as M5) had greater residual activity than T22YS87LT255IN269D (Fig. 4b). By combining coevolutionary analysis with multidimensional virtual screening, we obtained the quadruple point mutant M5 after only one round of combination. The specific activity of purified M5 toward N-Succinyl-Ala-Ala-Pro-Phe-p-nitroanilide (Suc-AAPF-pNA) was approximately 16% higher than that of the WT (Fig. 4c). Compared to the WT, M5 showed a significant increase by a factor of 3.5 in the t1/2 of the purified enzyme at 55 °C (Fig. S4† and 4d). Additionally, the melting temperature (Tm) of M5 increased to 72.9 °C, which was much higher than the Tm of the WT (63.0 °C) (Fig. S4 and S5†). Notably, the Tm and t1/2 of the M5 mutant did not correspond exactly. We hypothesize that mutations distant from the catalytic triad site contribute less to the t1/2 of the enzyme. Tm measures the protein unfolding process, while the t1/2 measures the loss of biological activity, focusing on active site inactivation. Therefore, the Tm and t1/2 are not always correlated,55 as a slight perturbation of the active site may fully inactivate the protein without significantly affecting the overall folding of the protein.57,58 Overall, we successfully obtained the highly robust mutant M5 using the Co-MdVS strategy. Additionally, all mutations in M5 were different from those in the previously reported mutant M4, which was obtained through classical strategies11(Table S7†). To determine whether Co-MdVS-directed mutations could synergize with those directed by classical strategies to further improve enzyme robustness, we combined M4 and M5 to obtain mutant M6 (Table S7†). The purified M6 exhibited a 2.2-fold increase in catalytic activity towards Suc-AAPF-pNA compared to the WT, and correspondingly, the M6 showed a 2.5-fold increase in catalytic activity towards the natural substrate fibronectin compared to the WT (Fig. S4† and 4c). Comparatively, the t1/2 of purified M6 at 55 °C is 31.0 times that of WT, and the catalytic efficiency (kcat/Km) of purified M6 is approximately 3.2 times that of WT (Fig. S4, 4d, S6a and c†).
3.4. Validation of the catalytic properties of M6
To evaluate the performance of the M6 engineered in this study for applications, a comparative analysis of fibrinolytic efficiency between the WT and M6 was conducted. Fibrin plate degradation assays revealed that, degradation by M6 was noted to be 54.9%, as opposed to 19.6% for the WT (Fig. 5a). In order to investigate the protein hydrolysis effectiveness of the WT and M6 in industrial applications, we conducted experiments using casein as the substrate on a 50 mL scale, while monitoring the catalytic process. The hydrolysis degree (DH) was utilized as a metric to assess the effectiveness of hydrolysis (Fig. 5b). Given that protein hydrolysis in industrial settings typically requires elevated temperatures,59 our experiment was carried out at 37 °C and 55 °C. The results showed that M6 had a better DH of casein than WT at both temperatures. Following a 6-hour digestion at 55 °C, the DH of M6 reached 27.7%, representing a fourfold increase compared to that of the WT. These results indicate that, compared to the WT, M6 exhibits higher efficiency in protein hydrolysis, and due to its excellent thermostability, it achieves a higher DH during high-temperature and long-term enzymatic digestion. NK belongs to the family of alkaline proteases, which typically exhibit low stability in acidic environments.29 However, given the potential application of NK as a food additive and a pharmaceutical ingredient, its acid resistance is a crucial characteristic.32,60 Therefore, this study investigated the acid resistance of M6. The tolerance of both WT and M6 under various pH conditions was compared, finding that at pH 4.5, M6 retained 61.3% residual activity, whereas the WT's was 2.5%, and even at a pH of 2.5, M6 is able to retain more than 15% residual activity (Fig. 5c). In summary, M6 displayed superior robustness and catalytic efficiency compared to the WT and these findings corroborate the advantages of M6 for industrial application.
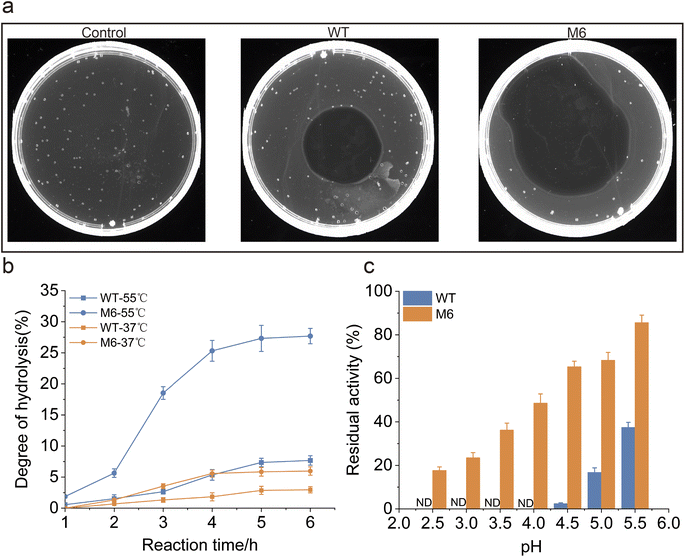 |
| Fig. 5 Validation of the robustness of M6. (a) Comparison of the ability of the WT and M6 to degrade fibrin on a flat plate. (b) Comparison of the ability of the WT and M6 to hydrolyze casein at different temperatures in practical application scenarios. On the 50 mL scale (100 Mm Tris–HCl, pH 8.0, 0.2 mM CaCl2), the substrate concentration is 20% casein, and the NK concentration is 0.5% of the substrate (w/w). (c) Comparison of the tolerance of WT and M6 to an acid environment. ND means not detectable. | |
3.5. Mechanistic analysis of enhanced performance of M6
MD simulations at different temperatures (300 K and 400 K) and pH values (3 and 7) were used to investigate the mechanism that gives M6 high thermostability and acid resistance. RMSD analysis showed that WT and M6 possessed similar overall structural flexibility at 300 K and pH 7. At high temperature and low pH, the structural variations of M6 were significantly lower than those of WT (Fig. S7a and b†). By means of RMSF analysis, we targeted region 202–225 in the WT that were sensitive to both high temperature and low pH, and this region was rigidified in M6 (Fig. 6a and b). The increased rigidity of this region was helpful for improving thermostability, and its help in improving the adaptability of NK to low pH was characterized in this study. Besides, the flexibility of the N-terminal region of M6 was found to be enhanced compared to that of the WT both at high temperature and low pH, indicating a structural flexibility enhancement that allows M6 to maintain backbone flexibility while ensuring stability and catalytic activity. This conversion of the flexible region was demonstrated to maintain high activity and stability of the enzyme.11
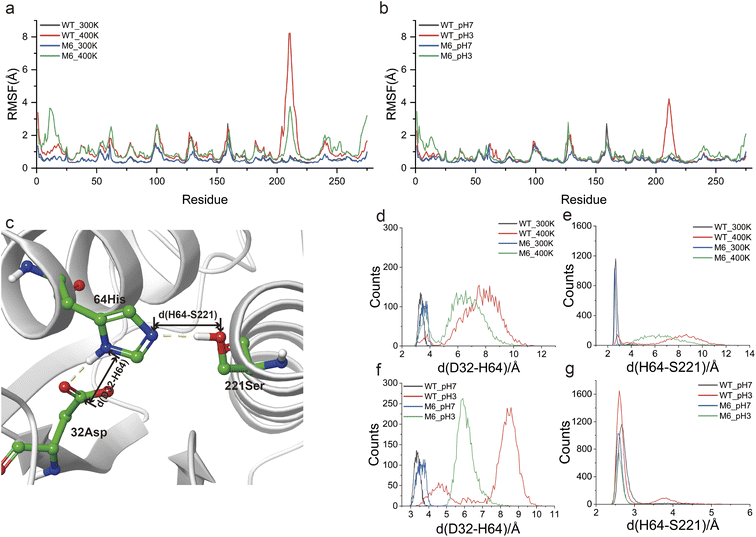 |
| Fig. 6 Analysis of the mechanism for the enhanced performance of mutant M6. Comparison of the RMSF of WT and M6 under different temperature (a) and pH (b) conditions using MD simulations. (c) The static structure of the NK catalytic triad. Yellow dashed lines showed hydrogen bonds. d(D32–H64) showed the distance between the carbon atom of the β-carboxyl group of residue D32 and the δ nitrogen atom of residue H64. d(H64–S221) showed the distance between the ε nitrogen atom of residue H64 and the hydroxyl oxygen atom of residue S221. Comparison of the d(D32–H64) (d) and d(H64–S221) (e) of WT and M6 at different temperatures during MD simulations. Comparison of d(D32–H64) (f) and d(H64–S221) (g) of WT and M6 at different pH values during MD simulations. | |
The catalytic triad (D32, H64 and S221) forms the active site of NK. D32 positively charges H64 through electrostatic interactions (Fig. 6c) and H64 acts as a general base, deprotonating S221; then the deprotonated S221 performs nucleophilic attack on the carbonyl carbon of the amide bond in peptides. The tight spatial structure among the catalytic triad ensures a suitable interaction among them. Notably, region 202–225 is spatially close to the catalytic triad and its fluctuations may lead to structural collapse of the catalytic triad. To validate this speculation, the distances between D32 and H64 (d(D32–H64)), and D64 and S221 (d(H64–S221)) in the last 20 ns of the MD simulation were statistically represented to reflect the structural stability of the catalytic triad (Fig. 6c). Under mild conditions (300 K and pH 7), d(D32–H64) was distributed in the range of 3–4 Å, both in WT and in M6 (Fig. 6d and f). The d(D64–S221) of WT and the mutant M6 was also distributed within a smaller range (2.5–3 Å) (Fig. 6e and g). High temperature and low pH lengthened d(D32–H64) and d(D64–S221), which may disrupt the structure of the catalytic triad. However, in M6, the increase in d(D32–H64) and d(D64–S221) was significantly smaller than those in WT. Based on these results, it was reasonable to believe that a more stable catalytic triad structure was an important factor contributing to the robustness of M6.
3.6. Exploring the potential application of MdVS
To explore the potential application of the MdVS approach proposed in this study, reverse validation was conducted using two enzymes, L-rhamnose isomerase61 and PETase,23 selected from previous reports (Fig. S8a and b†). Both of these previous studies employed initial screening of mutant variants based on a reduction in ΔΔG, followed by a subsequent combination of variants. Consequently, we randomly selected six pre-validated mutants (including both beneficial and undesirable mutants) with reduced ΔΔG values for each enzyme from the mutation library. Conventional MD simulations were then performed at 400 K for 20 ns for each of the selected variants. We calculated the RMSD, Rg, and total H-bonds of the enzyme structure for each mutant during the simulation process and calculated the differences from those of the WT (Fig. 7a and b). According to the results of the MdVS, we screened three L-rhamnose isomerase mutants (G238R, S239L and L375Y) (Fig. 7a). Notably, all three of these variants had been previously validated as beneficial mutants. The remaining mutants (G57I, S60R and S239F), which do not satisfy the screening criteria, had been previously validated as undesirable mutants. Simultaneously, the violin diagram representing the RMSD distribution for the undesirable mutants showed considerable variability (Fig. 7a), suggesting an inability to maintain a stable structure under the 400 K conditions. For PETase, the intersection of the three indicators revealed three variants (Q119Y, I168R and S187W), all of which had been previously validated as beneficial mutants (Fig. 7b). Similarly, the remaining mutants (S54W R100F and T286V), which did not satisfy the screening criteria, were previously confirmed to be undesirable mutants. A further violin diagram displaying the RMSD distribution for the undesirable mutants revealed a significant increase (Fig. 7b), indicating the inability to maintain a stable structure at high temperatures. Collectively, these results demonstrated the potential applicability of MdVS in protein design.
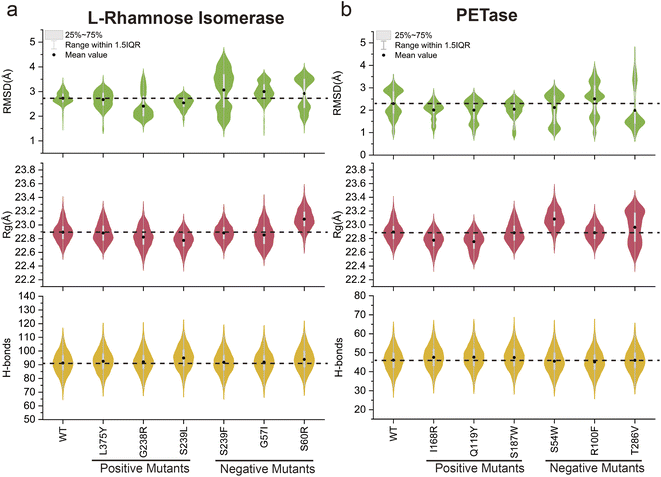 |
| Fig. 7 Examination of the application prospect of multiple indicator screening. Comparison of the calculated RMSD, Rg, and H-bonds among the WT and the mutants of L-rhamnose isomerase (a) and PETase (b). The black dots showed the mean values of each indicator. The black dashed line marked the value of WT. The positive mutants and the negative mutants were shown respectively. All of the positive mutants both in L-rhamnose isomerase and PETase exhibited reduced RMSD and Rg, increasing H-bonds, and the negative mutants could not satisfy the three conditions. | |
4. Discussion
Various strategies have been developed to design mutations to enhance protein stability or activity, such as FireProt and PROSS, whose effectiveness has been widely proven.62,63 However, the low accuracy of designed mutations remains an insurmountable obstacle. For instance, we previously employed FireProt for thermostability improved NK, in which 11 mutations were recommended; however, more than half of them showed lower thermostability than WT11. PROSS can be used to design multipoint mutations with mutation rates typically ranging from 2% to 12%.64 However, excessively high mutation rates may be detrimental to enzyme function, necessitating stepwise revertant mutations for optimization.65 Targeting key residues and the subsequent evaluation of mutants are critical factors that influence the accuracy and efficiency of the design. The Co-MdVS strategy proposed in this study employed coevolutionary analysis to target crucial residues and multidimensional virtual screening to accurately evaluate mutations. Based on Co-MdVS, a handful of NK mutants with increased thermostability and activity were efficiently screened from thousands of theoretical mutations, demonstrating the accuracy and effectiveness of this strategy.
We believe that coevolutionary analysis is of great use in the design of protein mutations. Coevolving residue pairs usually exhibit tight interactions that can be disrupted by single point mutations. For this reason, the conventional evaluation of single-point mutations rarely yields useful results involving coevolving residue pairs. Thus, the application of coevolutionary analysis greatly expands the scope of screening for potential beneficial mutations. As demonstrated in this study, although NK has been previously engineered with satisfactory results, we nevertheless obtained beneficial mutations at previously unaltered residues (T22, S87, T255 and N269). Classically, optimized mutants are obtained based on the combination of single point mutations, but this approach is inefficient because it requires multiple rounds of iterative combinations. Combinatorial mutations can be directly designed on the basis of coevolutionary analysis, which can greatly accelerate iterative evolution. We directly designed double point mutations in NK, and the optimal four-point mutation M5 was obtained in only one round of combination.
The identification and screening of mutants with improved properties, such as increased thermostability, require the use of appropriately selected screening indicators. Additionally, to ensure the accuracy of the screening process, it is crucial to ensure that the selected indicators are not significantly correlated with each other. Only in this way can beneficial mutants with performance-enhancing mutations be accurately and efficiently screened using multiple dimensions. The Rosetta ΔΔG is an energy term that describes the stability of protein folding after amino acid mutations in the protein structure.40 The calculation of ΔΔG based on energy functions tends to be limited on a spatial scale. The RMSD and Rg of the protein structure backbone, as well as the total H-bonds, produced from MD simulation, to some extent better characterize the stability of the protein structure.11,28,66 However, long-term MD simulations require massive computational resource consumption. To balance design efficiency and accuracy, we integrated four different dimensional indicators (ΔΔG, RMSD, Rg and total H-bonds) to screen for potentially beneficial mutants. First, the ddG predicted by the static structure was used to exclude mutations that were clearly detrimental to structural stability. Multiple indicators that characterize the structural dynamics of the protein were then used to identify the desired mutation. The application of these indicators, which have no significant correlation with each other (Fig. S3†), ensures the accuracy of the design. Moreover, harnessing computational capacity as an alternative to experimental testing allowed us to complete the screening of a virtual library containing 7980 mutants in a few weeks in this study. In fact, the widespread use of computational capacity has become a major trend in protein design, encompassing areas such as structure and function prediction,67–69 deep learning of beneficial mutations,70,71 and sequence and conformational ensemble generation,72–74 among others.
Obviously, Co-MdVS can be flexibly embedded in other protein design strategies. On the one hand, coevolutionary analysis can be used to greatly expand the targeting of crucial residues that significantly affect protein function,75 followed by the design of mutations for these residues. On the other hand, MdVS can be applied to the evaluation of mutations that are designed by diverse strategies. In this study, MdVS was tested on the previously reported mutations in L-rhamnose isomerase and PETase, demonstrating that it contributes to the accuracy of mutation design. It is reasonable to believe that MdVS can be applied to the enhancement of catalytic activity, substrate selectivity, stereoselectivity, and other enzyme properties if broader types of indicators are introduced, such as protein-ligand binding free energy, and the distance and angle between the active site and the attacked group of the substrate.
We found that certain mutants, such as T22LS87L, K27RS89L, and H67AS207V, though they had reasonable MdVS indicators, exhibited thermostability comparable to that of the WT but showed decreased activity (Fig. 4a). Further RMSF analysis indicated that these mutants exhibited enhanced rigidity near the active site (region 210–220) compared to the WT (Fig. S9a and b†). These results suggested that increased rigidity near the active site may negatively impact enzymatic activity. This may be due to the fact that enzymes generally undergo conformational changes during catalysis,76–78 so maintaining an appropriate degree of flexibility in the enzyme structure is key to preserving catalytic activity.
5. Conclusion
Overall, in this study, we integrated coevolutionary information on residues and multiple indicators of mutant robustness to develop a strategy, Co-MdVS, for accurate and efficient design and screening of combined mutants to increase enzyme robustness. Using this strategy, we accurately enhanced the robustness of NK. Moreover, we have demonstrated the potential of this strategy in enhancing enzyme robustness across various enzymes. By incorporating indicators that can encompass other protein properties, this strategy holds the promise of achieving broader objectives.
Data availability
The data supporting this article have been included as part of the ESI.†
Author contributions
LC. H., J. L. and ZM. Z. designed the experiments and supervised the project. J. L., CS. S. and Q. W. performed the experiments. J. L., LC. H. and WJ. C. performed the data analysis. J. L., LC. H. and ZM. Z. wrote the manuscript. All authors reviewed the manuscript.
Conflicts of interest
There are no conflicts of interest to declare.
Acknowledgements
This work was financially supported by the National Key Research and Development Program of China (2023YFC3402400), the National Natural Science Foundation of China (32201034 and 32271301), and the Fundamental Research Funds for the Central Universities (JUSRP124019). The MD simulation and structural analysis in this study were supported by the high-performance computing cluster platform of the School of Biotechnology, Jiangnan University.
References
- I. V. D. Amatto, N. G. da Rosa-Garzon, F. A. D. Simoes, F. Santiago, N. P. D. Leite, J. R. Martins and H. Cabral, Enzyme engineering and its industrial applications, Biotechnol. Appl. Biochem., 2022, 69, 389–409 CrossRef PubMed.
- S. Gaseidnes, B. Synstad, J. E. Nielsen and V. G. H. Eijsink, Rational engineering of the stability and the catalytic performance of enzymes, J. Mol. Catal. B: Enzym., 2003, 21, 3–8 CrossRef CAS.
- P. A. Dalby, Strategy and success for the directed evolution of enzymes, Curr. Opin. Struct. Biol., 2011, 21, 473–480 CrossRef CAS PubMed.
- U. T. Bornscheuer, B. Hauer, K. E. Jaeger and U. Schwaneberg, Directed Evolution Empowered Redesign of Natural Proteins for the Sustainable Production of Chemicals and Pharmaceuticals, Angew. Chem., Int. Ed., 2019, 58, 36–40 CrossRef CAS PubMed.
- H. M. Zhao and F. H. Arnold, Directed evolution converts subtilisin E into a functional equivalent of thermitase, Protein Eng., 1999, 12, 47–53 CrossRef CAS PubMed.
- F. H. Arnold and A. A. Volkov, Directed evolution of biocatalysts, Curr. Opin. Chem. Biol., 1999, 3, 54–59 CrossRef CAS PubMed.
- G. Qu, A. T. Li, C. G. Acevedo-Rocha, Z. T. Sun and M. T. Reetz, The Crucial Role of Methodology Development in Directed Evolution of Selective Enzymes, Angew. Chem., Int. Ed., 2020, 59, 13204–13231 CrossRef CAS PubMed.
- H. Suzuki, J. Kobayashi, K. Wada, M. Furukawa and K. Doi, Thermoadaptation-Directed Enzyme Evolution in an Error-Prone Thermophile Derived from Geobacillus kaustophilus HTA426, Appl. Environ. Microbiol., 2015, 81, 149–158 CrossRef PubMed.
- W. L. Zheng, H. R. Yu, S. Fang, K. T. Chen, Z. Wang, X. L. Cheng, G. Xu, L. R. Yang and J. P. Wu, Directed Evolution of L-Threonine Aldolase for the Diastereoselective Synthesis of beta-Hydroxy-alpha-amino Acids, ACS Catal., 2021, 11, 3198–3205 CrossRef CAS.
- A. P. Green, N. J. Turner and E. O'Reilly, Chiral Amine Synthesis Using ω-Transaminases: An Amine Donor that Displaces Equilibria and Enables High-Throughput Screening, Angew. Chem., Int. Ed., 2014, 53, 10714–10717 CrossRef CAS PubMed.
- J. Luo, C. S. Song, W. J. Cui, L. C. Han and Z. M. Zhou, Counteraction of stability-activity trade-off of Nattokinase through flexible region shifting, Food Chem., 2023, 423 DOI:10.1016/j.foodchem.2023.136241.
- D. Ma, Z. Y. Cheng, L. Peplowski, L. C. Han, Y. Y. Xia, X. D. Hou, J. L. Guo, D. J. Yin, Y. J. Rao and Z. M. Zhou, Insight into the broadened substrate scope of nitrile hydratase by static and dynamic structure analysis, Chem. Sci., 2022, 13, 8417–8428 RSC.
- G. Rabbani, E. Ahmad, A. Ahmad and R. H. Khan, Structural features, temperature adaptation and industrial applications of microbial lipases from psychrophilic, mesophilic and thermophilic origins, Int. J. Biol. Macromol., 2023, 225, 822–839 CrossRef CAS PubMed.
- N. M. Ashraf, A. Krishnagopal, A. Hussain, D. Kastner, A. M. M. Sayed, Y. K. Mok, K. Swaminathan and N. Zeeshan, Engineering of serine protease for improved thermostability and catalytic activity using rational design, Int. J. Biol. Macromol., 2019, 126, 229–237 CrossRef CAS PubMed.
- Y. L. Cui, Y. H. Wang, W. Y. Tian, Y. F. Bu, T. Li, X. X. Cui, T. Zhu, R. F. Li and B. Wu, Development of a versatile and efficient C-N lyase platform for asymmetric hydroamination via computational enzyme redesign, Nat. Catal., 2021, 4, 364–373 CrossRef CAS.
- D. S. Marks, L. J. Colwell, R. Sheridan, T. A. Hopf, A. Pagnani, R. Zecchina and C. Sander, Protein 3D Structure Computed from Evolutionary Sequence Variation, PLoS One, 2011, 6 DOI:10.1371/journal.pone.0028766.
- F. Colizzi and M. Orozco, Probing allosteric regulations with coevolution-driven molecular simulations, Sci. Adv., 2021, 7 DOI:10.1126/sciadv.abj0786.
- Y. Liu, P. Palmedo, Q. Ye, B. Berger and J. Peng, Enhancing Evolutionary Couplings with Deep Convolutional Neural Networks, Cell Syst., 2018, 6, 65 CrossRef CAS PubMed.
- Z. Zhang, L. Wang, Y. Gao, J. Zhang, M. Zhenirovskyy and E. Alexov, Predicting folding free energy changes upon single point mutations, Bioinformatics, 2012, 28, 664–671 CrossRef CAS PubMed.
- A. N. Naganathan and A. Kannan, A hierarchy of coupling free energies underlie the thermodynamic and functional architecture of protein structures, Curr. Res. Struct. Biol., 2021, 3, 257–267 CrossRef CAS PubMed.
- J. T. Zhao, Y. Cao and L. Zhang, Exploring the computational methods for protein-ligand binding site prediction, Comput. Struct. Biotechnol. J., 2020, 18, 417–426 CrossRef CAS PubMed.
- S. F. Xiao, V. Patsalo, B. Shan, Y. Bi, D. F. Green and D. P. Raleigh, Rational modification of protein stability by targeting surface sites leads to complicated results, Proc. Natl. Acad. Sci. USA, 2013, 110, 11337–11342 CrossRef CAS PubMed.
- Y. L. Cui, Y. C. Chen, X. Y. Liu, S. J. Dong, Y. E. Tian, Y. X. Qiao, R. Mitra, J. Han, C. L. Li, X. Han, W. D. Liu, Q. Chen, W. Q. Wei, X. Wang, W. B. Du, S. Y. Tang, H. Xiang, H. Y. Liu, Y. Liang, K. N. Houk and B. Wu, Computational Redesign of a PETase for Plastic Biodegradation under Ambient Condition by the GRAPE Strategy, ACS Catal., 2021, 11, 1340–1350 CrossRef CAS.
- H. Park, P. Bradley, P. Greisen, Y. Liu, V. K. Mulligan, D. E. Kim, D. Baker and F. DiMaio, Simultaneous Optimization of Biomolecular Energy Functions on Features from Small Molecules and Macromolecules, J. Chem. Theory Comput., 2016, 12, 6201–6212 CrossRef CAS PubMed.
- M. Musil, H. Konegger, J. Hong, D. Bednar and J. Damborsky, Computational Design of Stable and Soluble Biocatalysts, ACS Catal., 2019, 9, 1033–1054 CrossRef CAS.
- R. Qing, S. L. Hao, E. Smorodina, D. V. Jin, A. Zalevsky and S. G. Zhang, Protein Design: From the Aspect of Water Solubility and Stability, Chem. Rev., 2022, 122(18), 14085–14179, DOI:10.1021/acs.chemrev.1c00757.
- H. R. Yu and P. A. Dalby, Exploiting correlated molecular-dynamics networks to counteract enzyme activity-stability trade-off, Proc. Natl. Acad. Sci. USA, 2018, 115, E12192–E12200 CAS.
- L. Y. Zhang, S. Y. Zhao, C. Chang, J. A. Wang, C. Yang and Z. Y. Cheng, N-terminal loops at the tetramer interface of nitrile hydratase act as “hooks” determining resistance to high amide concentrations, Int. J. Biol. Macromol., 2023, 245 DOI:10.1016/j.ijbiomac.2023.125531.
- Z. M. Liu, H. Zhao, L. C. Han, W. J. Cui, L. Zhou and Z. M. Zhou, Improvement of the acid resistance, catalytic efficiency, and thermostability of nattokinase by multisite-directed mutagenesis, Biotechnol. Bioeng., 2019, 116, 1833–1843 CrossRef CAS PubMed.
- P. H. Yang, X. L. Wang, J. C. Ye, S. Q. Rao, J. W. Zhou, G. C. Du and S. Liu, Enhanced Thermostability and Catalytic Activity of Streptomyces mobaraenesis Transglutaminase by Rationally Engineering Its Flexible Regions, J. Agric. Food Chem., 2023, 71, 6366–6375 CrossRef CAS PubMed.
- Y. Li, L. Q. Chen, X. Y. Tang and J. Y. Li, Biotechnology, Bioengineering and Applications of Bacillus Nattokinase, Biomolecules, 2022, 12 DOI:10.3390/biom12070980.
- Y. Li, X. Tang, L. Chen, A. Ma, W. Zhu, W. Huang and J. Li, Improvement of the fibrinolytic activity, acid resistance and thermostability of nattokinase by surface charge engineering, Int. J. Biol. Macromol., 2023, 253 DOI:10.1016/j.ijbiomac.2023.127373.
- L. Han, W. J. Cui, F. Y. Suo, S. N. Miao, W. L. Hao, Q. Q. Chen, J. L. Guo, Z. M. Liu, L. Zhou and Z. M. Zhou, Development of a novel strategy for robust synthetic bacterial promoters based on a stepwise evolution targeting the spacer region of the core promoter in Bacillus subtilis, Microb. Cell Fact., 2019, 18 DOI:10.1186/s12934-019-1148-3.
- D. G. Gibson, L. Young, R. Y. Chuang, J. C. Venter, C. A. Hutchison and H. O. Smith, Enzymatic assembly of DNA molecules up to several hundred kilobases, Nat. Methods, 2009, 6 DOI:10.1038/nmeth.1318.
- R. Panasiuk, R. Amarowicz, H. Kostyra and L. Sijtsma, Determination of α-amino nitrogen in pea protein hydrolysates:: a comparison of three analytical methods, Food Chem., 1998, 62, 363–367 CrossRef CAS.
- T. A. Hopf, A. G. Green, B. Schubert, S. Mersmann, C. P. I. Scharfe, J. B. Ingraham, A. Toth-Petroczy, K. Brock, A. J. Riesselman, P. Palmedo, C. Kang, R. Sheridan, E. J. Draizen, C. Dallago, C. Sander and D. S. Marks, The EVcouplings Python framework for coevolutionary sequence analysis, Bioinformatics, 2019, 35, 1582–1584 CrossRef CAS PubMed.
- Y. Yanagisawa, T. Chatake, K. Chiba-Kamoshida, S. Naito, T. Ohsugi, H. Sumi, I. Yasuda and Y. Morimoto, Purification, crystallization and preliminary X-ray diffraction experiment of nattokinase from Bacillus subtilis natto, Acta Crystallogr., Sect. F: Struct. Biol. Cryst. Commun., 2010, 66, 1670–1673 CrossRef CAS PubMed.
- P. Conway, M. D. Tyka, F. DiMaio, D. E. Konerding and D. Baker, Relaxation of backbone bond geometry improves protein energy landscape modeling, Protein Sci., 2014, 23, 47–55 CrossRef CAS PubMed.
- F. Khatib, S. Cooper, M. D. Tyka, K. F. Xu, I. Makedon, Z. Popovic, D. Baker and F. Players, Algorithm discovery by protein folding game players, Proc. Natl. Acad. Sci. USA, 2011, 108, 18949–18953 CrossRef CAS PubMed.
- V. Sora, A. O. Laspiur, K. Degn, M. Arnaudi, M. Utichi, L. Beltrame, D. De Menezes, M. Orlandi, U. K. Stoltze, O. Rigina, P. W. Sackett, K. Wadt, K. Schmiegelow, M. Tiberti and E. Papaleo, RosettaDDGPrediction for high-throughput mutational scans: From stability to binding, Protein Sci., 2023, 32 DOI:10.1002/pro.4527.
- B. Frenz, S. M. Lewis, I. King, F. DiMaio, H. Park and Y. F. Song, Prediction of Protein Mutational Free Energy: Benchmark and Sampling Improvements Increase Classification Accuracy, Front. Bioeng. Biotechnol., 2020, 8 DOI:10.3389/fbioe.2020.558247.
- R. F. Alford, A. Leaver-Fay, J. R. Jeliazkov, M. J. O'Meara, F. P. DiMaio, H. Park, M. V. Shapovalov, P. D. Renfrew, V. K. Mulligan, K. Kappel, J. W. Labonte, M. S. Pacella, R. Bonneau, P. Bradley, R. L. Dunbrack, R. Das, D. Baker, B. Kuhlman, T. Kortemme and J. J. Gray, The Rosetta All-Atom Energy Function for Macromolecular Modeling and Design, J. Chem. Theory Comput., 2017, 13, 3031–3048 CrossRef CAS PubMed.
- J. C. Phillips, D. J. Hardy, J. D. C. Maia, J. E. Stone, J. V. Ribeiro, R. C. Bernardi, R. Buch, G. Fiorin, J. Henin, W. Jiang, R. McGreevy, M. C. R. Melo, B. K. Radak, R. D. Skeel, A. Singharoy, Y. Wang, B. Roux, A. Aksimentiev, Z. Luthey-Schulten, L. V. Kale, K. Schulten, C. Chipot and E. Tajkhorshid, Scalable molecular dynamics on CPU and GPU architectures with NAMD, J. Chem. Phys., 2020, 153 DOI:10.1063/5.0014475.
- R. B. Best, X. Zhu, J. Shim, P. E. M. Lopes, J. Mittal, M. Feig and A. D. MacKerell, Optimization of the Additive CHARMM All-Atom Protein Force Field Targeting Improved Sampling of the Backbone phi, psi and Side-Chain chi(1) and chi(2) Dihedral Angles, J. Chem. Theory Comput., 2012, 8, 3257–3273 CrossRef CAS PubMed.
- Y. Yanagisawa, T. Chatake, K. Chiba-Kamoshida, S. Naito, T. Ohsugi, H. Sumi, I. Yasuda and Y. Morimoto, Purification, crystallization and preliminary X-ray diffraction experiment of nattokinase from, Acta Crystallogr., Sect. F: Struct. Biol. Cryst. Commun., 2010, 66, 1670–1673 CrossRef CAS PubMed.
- T. J. Dolinsky, J. E. Nielsen, J. A. McCammon and N. A. Baker, PDB2PQR: an automated pipeline for the setup of Poisson-Boltzmann electrostatics calculations, Nucleic Acids Res., 2004, 32, W665–W667 CrossRef CAS PubMed.
- E. Jurrus, D. Engel, K. Star, K. Monson, J. Brandi, L. E. Felberg, D. H. Brookes, L. Wilson, J. H. Chen, K. Liles, M. J. Chun, P. Li, D. W. Gohara, T. Dolinsky, R. Konecny, D. R. Koes, J. E. Nielsen, T. Head-Gordon, W. H. Geng, R. Krasny, G. W. Wei, M. J. Holst, J. A. McCammon and N. A. Baker, Improvements to the APBS biomolecular solvation software suite, Protein Sci., 2018, 27, 112–128 CrossRef CAS PubMed.
- M. H. M. Olsson, C. R. Søndergaard, M. Rostkowski and J. H. Jensen, PROPKA3: Consistent Treatment of Internal and Surface Residues in Empirical pKa Predictions, J. Chem. Theory Comput., 2011, 7, 525–537 CrossRef CAS PubMed.
- X. Q. Yao, G. Scarabelli, L. Skjrven and B. J. Grant, The Bio3D Package: New Interactive Tools for Structural Bioinformatics, Biophys. J., 2014, 106, 406a CrossRef.
- T. J. Magliery, Protein stability: computation, sequence statistics, and new experimental methods, Curr. Opin. Struct. Biol., 2015, 33, 161–168 CrossRef CAS PubMed.
- U. Markel, K. D. Essani, V. Besirlioglu, J. Schiffels, W. R. Streit and U. Schwaneberg, Advances in ultrahigh-throughput screening for directed enzyme evolution, Chem. Soc. Rev., 2020, 49, 233–262 RSC.
- M. Dörr, M. P. C. Fibinger, D. Last, S. Schmidt, J. Santos-Aberturas, D. Böttcher, A. Hummel, C. Vickers, M. Voss and U. T. Bornscheuer, Fully automatized high-throughput enzyme library screening using a robotic platform, Biotechnol. Bioeng., 2016, 113, 1421–1432 CrossRef PubMed.
- F. Morcos, A. Pagnani, B. Lunt, A. Bertolino, D. S. Marks, C. Sander, R. Zecchina, J. N. Onuchic, T. Hwa and M. Weigt, Direct-coupling analysis of residue coevolution captures native contacts across many protein families, Proc. Natl. Acad. Sci. USA, 2011, 108, E1293–E1301 CrossRef CAS PubMed.
- H. R. Yu, Y. H. Yan, C. Zhang and P. A. Dalby, Two strategies to engineer flexible loops for improved enzyme thermostability, Sci. Rep., 2017, 7 DOI:10.1038/srep41212.
- C. Chen, B. H. Guan, Q. Geng, Y. C. Zheng, Q. Chen, J. Pan and J. H. Xu, Enhanced Thermostability of Ketoreductase by Computation-Based Cross-Regional Combinatorial Mutagenesis, ACS Catal., 2023, 13, 7407–7416 CrossRef CAS.
- Y. Kajiwara, S. Yasuda, Y. Takamuku, T. Murata and M. Kinoshita, Identification of thermostabilizing mutations for a membrane protein whose three-dimensional structure is unknown, J. Comput. Chem., 2017, 38, 211–223 CrossRef CAS PubMed.
- O. Miyawaki, T. Kanazawa, C. Maruyama and M. Dozen, Static and dynamic half-life and lifetime molecular turnover of enzymes, J. Biosci. Bioeng., 2017, 123, 28–32 CrossRef CAS PubMed.
- J. K. Kranz and C. Schalk-Hihi, Protein Thermal Shifts to Identify Low Molecular Weight Fragments, Methods Enzymol., 2011, 493, 277–298 CAS.
- C. L. A. de Menezes, R. D. Santos, M. V. Santos, M. Boscolo, R. da Silva, E. Gomes and R. R. da Silva, Industrial sustainability of microbial keratinases: production and potential applications, World J. Microbiol. Biotechnol., 2021, 37 DOI:10.1007/s11274-021-03052-z.
- A. M. M. Ali and S. C. B. Bavisetty, Purification, physicochemical properties, and statistical optimization of fibrinolytic enzymes especially from fermented foods: A comprehensive review, Int. J. Biol. Macromol., 2020, 163, 1498–1517 CrossRef PubMed.
- M. J. Wei, X. Gao, W. Zhang, C. Li, F. P. Lu, L. J. Guan, W. D. Liu, J. W. Wang, F. H. Wang and H. M. Qin, Enhanced Thermostability of L-Rhamnose Isomerase for D-Allose Synthesis by Computation-Based Rational Redesign of Flexible Regions, J. Agric. Food Chem., 2023, 71, 15713–15722 CrossRef CAS PubMed.
- Y. F. Ming, W. K. Wang, R. Yin, M. Zeng, L. Tang, S. Z. Tang and M. Li, A review of enzyme design in catalytic stability by artificial intelligence, Briefings Bioinf., 2023, 24 CAS.
- M. Scherer, S. J. Fleishman, P. R. Jones, T. Dandekar and E. Bencurova, Computational Enzyme Engineering Pipelines for Optimized Production of Renewable Chemicals, Front. Bioeng. Biotechnol., 2021, 9 Search PubMed.
- A. Goldenzweig, M. Goldsmith, S. E. Hill, O. Gertman, P. Laurino, Y. Ashani, O. Dym, T. Unger, S. Albeck, J. Prilusky, R. L. Lieberman, A. Aharoni, I. Silman, J. L. Sussman, D. S. Tawfik and S. J. Fleishman, Automated Structure- and Sequence-Based Design of Proteins for High Bacterial Expression and Stability (vol 63, pg 337, 2016), Mol. Cell, 2018, 70, 380 CrossRef CAS PubMed.
- M. Li, D. Ma, J. Qiao, Z. Cheng, Q. Wang, Z. Zhou and L. Han, Development of high-performance nitrile hydratase whole-cell catalyst by automated structure- and sequence-based design and mechanism insights, Syst. Microbiol. Biomanuf., 2024, 4(2) DOI:10.1007/s43393-024-00239-x.
- J. Damnjanovic, H. Nakano and Y. Iwasaki, Deletion of a Dynamic Surface Loop Improves Stability and Changes Kinetic Behavior of Phosphatidylinositol-Synthesizing Phospholipase D, Biotechnol. Bioeng., 2014, 111, 674–682 CrossRef CAS PubMed.
- T. H. Yu, H. Y. Cui, J. C. Li, Y. A. Luo, G. D. Jiang and H. M. Zhao, Enzyme function prediction using contrastive learning, Science, 2023, 379, 1358 CrossRef CAS PubMed.
- R. Chowdhury, N. Bouatta, S. Biswas, C. Floristean, A. Kharkare, K. Roye, C. Rochereau, G. Ahdritz, J. Zhang, G. M. Church, P. K. Sorger and M. AlQuraishi, Single-sequence protein structure prediction using a language model and deep learning, Nat. Biotechnol., 2022, 40, 1617 CrossRef CAS PubMed.
- R. Krishna, J. Wang, W. Ahern, P. Sturmfels, P. Venkatesh, I. Kalvet, G. R. Lee, F. S. Morey-Burrows, I. Anishchenko, I. R. Humphreys, R. McHugh, D. Vafeados, X. Li, G. A. Sutherland, A. Hitchcock, C. N. Hunter, A. Kang, E. Brackenbrough, A. K. Bera, M. Baek, F. DiMaio and D. Baker, Generalized biomolecular modeling and design with RoseTTAFold All-Atom, Science, 2024, 384, eadl2528 CrossRef CAS PubMed.
- F. Zhang, M. Naeem, B. Yu, F. Liu and J. Ju, Improving the enzymatic activity and stability of N-carbamoyl hydrolase using deep learning approach, Microb. Cell Fact., 2024, 23, 164 CrossRef CAS PubMed.
- R. Hu, L. Fu, Y. Chen, J. Chen, Y. Qiao and T. Si, Protein engineering via Bayesian optimization-guided evolutionary algorithm and robotic experiments, Briefings Bioinf., 2023, 24 DOI:10.1093/bib/bbac570.
- W. Zheng, Q. Q. G. Wuyun, Y. Li, C. X. Zhang, P. L. Freddolino and Y. Zhang, Improving deep learning protein monomer and complex structure prediction using DeepMSA2 with huge metagenomics data, Nat. Methods, 2024, 21(2), 279–289, DOI:10.1038/s41592-023-02130-4.
- G. Janson, G. Valdes-Garcia, L. Heo and M. Feig, Direct generation of protein conformational ensembles via machine learning, Nat. Commun., 2023, 14 DOI:10.1038/s41467-023-36443-x.
- K. H. Sumida, R. Núñez-Franco, I. Kalvet, S. J. Pellock, B. I. M. Wicky, L. F. Milles, J. Dauparas, J. Wang, Y. Kipnis, N. Jameson, A. L. Kang, J. De La Cruz, B. Sankaran, A. K. Bera, G. Jiménez-Osés and D. Baker, Improving Protein Expression, Stability, and Function with ProteinMPNN, J. Am. Chem. Soc., 2024, 146, 2054–2061 CrossRef CAS PubMed.
- X. Y. Wang, X. R. Jing, Y. Deng, Y. Nie, F. Xu, Y. Xu, Y. L. Zhao, J. F. Hunt, G. T. Montelione and T. Szyperski, Evolutionary coupling saturation mutagenesis: Coevolution-guided identification of distant sites influencing Bacillus naganoensis pullulanase activity, FEBS Lett., 2020, 594, 799–812 CrossRef CAS PubMed.
- C. S. Karamitros, K. Murray, B. Winemiller, C. Lamb, E. M. Stone, S. D’Arcy, K. A. Johnson and G. Georgiou, Leveraging intrinsic flexibility to engineer enhanced enzyme catalytic activity, Proc. Natl. Acad. Sci. USA, 2022, 119 DOI:10.1073/pnas.2118979119.
- Z. Yuan, J. Zhao and Z. X. Wang, Flexibility analysis of enzyme active sites by crystallographic temperature factors, Protein Eng., 2003, 16, 109–114 CrossRef CAS PubMed.
- B. M. Nestl and B. Hauer, Engineering of Flexible Loops in Enzymes, ACS Catal., 2014, 4, 3201–3211 CrossRef CAS.
|
This journal is © The Royal Society of Chemistry 2024 |
Click here to see how this site uses Cookies. View our privacy policy here.