DOI:
10.1039/D4SC04107K
(Review Article)
Chem. Sci., 2024,
15, 16844-16886
AI empowering traditional Chinese medicine?
Received
21st June 2024
, Accepted 22nd September 2024
First published on 23rd September 2024
Abstract
For centuries, Traditional Chinese Medicine (TCM) has been a prominent treatment method in China, incorporating acupuncture, herbal remedies, massage, and dietary therapy to promote holistic health and healing. TCM has played a major role in drug discovery, with over 60% of small-molecule drugs approved by the FDA from 1981 to 2019 being derived from natural products. However, TCM modernization faces challenges such as data standardization and the complexity of TCM formulations. The establishment of comprehensive TCM databases has significantly improved the efficiency and accuracy of TCM research, enabling easier access to information on TCM ingredients and encouraging interdisciplinary collaborations. These databases have revolutionized TCM research, facilitating advancements in TCM modernization and patient care. In addition, advancements in AI algorithms and database data quality have accelerated progress in AI for TCM. The application of AI in TCM encompasses a wide range of areas, including herbal screening and new drug discovery, diagnostic and treatment principles, pharmacological mechanisms, network pharmacology, and the incorporation of innovative AI technologies. AI also has the potential to enable personalized medicine by identifying patterns and correlations in patient data, leading to more accurate diagnoses and tailored treatments. The potential benefits of AI for TCM are vast and diverse, promising continued progress and innovation in the field.
1. Introduction
Traditional Chinese Medicine (TCM) has played a significant role in the treatment of various illnesses in China1–8 for centuries. TCM lies in the concept of Qi (vital energy) and the theory of Yin-Yang balance, which are pivotal in its approach to diagnosing and treating illnesses.9,10 TCM encompasses various practices such as acupuncture, herbal remedies, massage, and dietary therapy. These practices are rooted in ancient knowledge and have been refined over centuries of use in treating various illnesses and promoting overall wellness.11 TCM makes up a large proportion of natural products which serve as important sources for drug discovery and development. From 1981 to 2019, over 60% of the small-molecule drugs approved by the FDA were directly or indirectly derived from natural products.12 So TCM has the potential to provide a wealth of new drugs for a variety of diseases while also offering patients different options to choose from depending on their individual needs and preferences.13–16
AlphaFold3,17 a cutting-edge AI system for predicting protein structures, offers significant potential for advancing TCM research. TCM's complexity, characterized by multi-component herbal formulations, necessitates a thorough understanding of how active ingredients interact with specific target proteins in the body. Integrating AlphaFold3 into TCM research will not only accelerate drug discovery and optimize TCM formulations but also drive the modernization of TCM. This integration ensures that TCM evolves with the latest scientific advancements while preserving its traditional roots. The problems that TCM modernization faces include the insufficiency in data standardization, integrity, and precision of existing TCM-related databases.18–20 Besides, a bottleneck problem is that the TCM formulations, which often consist of multiple ingredients with synergistic effects make it difficult to isolate and understand the specific mechanisms of action for each component.21 Therefore, TCM databases have become essential in improving the effectiveness and accuracy of TCM research. By providing comprehensive information on TCM ingredients, including their chemical structures, pharmacological effects, and targets, the TCM databases offer researchers a centralized resource that significantly aids in their studies.22,23 This comprehensive resource greatly streamlines manual data collection and analysis, significantly reducing the time and resources required for TCM research. Furthermore, the availability of TCM ingredient databases has also encouraged more interdisciplinary collaborations between traditional medicine practitioners and modern scientific researchers.24 By sharing knowledge and resources, these collaborations have resulted in a better understanding of TCM's therapeutic potential and contributed to its integration into mainstream healthcare practices. Overall, the emergence of TCM databases has revolutionized the way in which TCM research is conducted. It has not only facilitated the acquisition of crucial information, but also encouraged greater collaboration among different fields of study, ultimately leading to advancements in TCM modernization and research.
With the rapid advancements in Artificial Intelligence (AI) algorithms and the increasing availability of high-quality, extensive databases, the development of AI in the field of TCM has accelerated significantly.25–28 These advancements have enabled more sophisticated analyses and enhanced the potential for AI to revolutionize TCM research, leading to more accurate diagnostics, a better understanding of complex herbal interactions, and the development of innovative treatment strategies.29,30 The integration of AI into TCM is opening new avenues for research and clinical applications, marking a transformative period in the field. As shown in Fig. 1, the integration of AI in TCM encompasses a systematic workflow starting from data mining of extensive TCM databases. These databases provide foundational knowledge that is crucial for identifying active herbal ingredients. AI technologies then analyse these ingredients to drive advancements in various domains including drug discovery, diagnosis, and understanding of pharmacological actions. The integration of AI and TCM has greatly changed the research paradigm of TCM and promoted the development of TCM in disease treatment. The application of AI in TCM spans across diverse areas such as herbal screening,31–33 new drug discovery,34 diagnostic35–37 and treatment principles,38 pharmacological mechanism39 and network pharmacology,40–43 as well as the incorporation of innovative AI technologies. AI shines in data mining, pattern recognition, and predictive analysis, skills crucial in unravelling the intricate formulations and treatment strategies unique to TCM44,45 Compared to Western medicine, TCM has advantages in personalized medicine because its diagnostic approach focuses on the individual's response to pathogenetic factors and internal maladjustments rather than solely on pathological mechanisms.46 By harnessing large databases and machine learning algorithms, AI can help identify patterns and correlations in patient data that would be difficult or impossible for human practitioners to detect.47 This can lead to more accurate diagnoses and tailored treatments that take into account each patient's unique characteristics and health history. Furthermore, AI's analysis of TCM databases sheds light on the mechanisms behind various herbal medicines and formulations. AI-based network pharmacology is employed to unravel the active ingredients and potential targets of TCM formulations in treating ailments like Type 2 diabetes and COVID-19.48,49 Meanwhile, AI can play a vital role in analyzing drug incompatibilities in TCM, which is essential for ensuring patient safety.50 Given the complexity and widespread use of herbal compounds in TCM, accurate analysis of potential interactions is critical, and AI's advanced capabilities can significantly enhance this process. AI's impact is also profound in improving diagnostic accuracy in TCM. Advanced models enhance syndrome differentiation and diagnosis in TCM, using deep learning and neural networks to analyse complex patient data – a leap from traditional subjective assessments.51,52
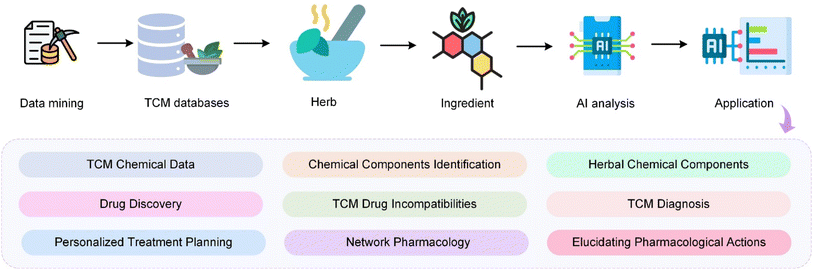 |
| Fig. 1 Workflow of the integration of AI in TCM research and applications. The process begins with data mining of TCM databases, which collate historical and contemporary data on various herbs. These herbs are then analysed to identify their key ingredients, which are subsequently subjected to AI-driven analysis for various applications including chemical component identification, drug discovery, personalized treatment planning, network pharmacology, and elucidating pharmacological actions. The outcome of these analyses informs various TCM applications such as drug compatibility, diagnostic strategies, and personalized treatment plans. | |
Despite these strides, integrating AI fully into TCM research presents challenges. The intricacy of TCM formulations, the necessity for standardized data, and ensuring AI models align with TCM philosophy are significant obstacles. As AI evolves, future research in this field aims to develop more advanced AI models tailored to the complexities of TCM data, upholding its foundational principles. The synergy of AI and TCM heralds a new era in healthcare, where ancient wisdom is enhanced by modern technology. This integration not only deepens our understanding of traditional practices but also paves the way for innovative treatments and therapies. As AI technology progresses, its role in TCM is set to grow, heralding a future where traditional methods are bolstered by cutting-edge technology for the betterment of global health.
To provide a clear roadmap for our discussion, the structure of this paper is as follows: Section 2 introduces the development status and features of TCM databases; Section 3 discusses the challenges of TCM modernization and the opportunities for integration with AI; Section 4 provides an overview of AI technologies applied in TCM research; Section 5 delves into the specific applications of AI in analyzing TCM chemical data; Sections 6–12 comprehensively detail the applications of AI across various TCM fields, including drug discovery, ingredient analysis, and diagnostic treatment; Section 13 explores AI's role in elucidating the pharmacological mechanisms of TCM; Section 14 summarizes the current challenges and future development prospects; finally, Section 15 offers a concluding summary of the entire paper.
2. TCM databases: data quality, features, and AI-driven analysis
2.1 TCM database
2.1.1 TCMbank.
Expanding on our TCM Database@Taiwan,53 we have released TCMBank54 (https://TCMBank.CN/), a comprehensive Chinese medicine database in 2023. TCMBank is free to access and offers standardized information on herbs, ingredients, targets, diseases, and various other valuable resources. At present, TCMBank is the largest free database that furnishes standardized information on targets and diseases and it is automatically updated by using an intelligent document identification module (IDIM) that continuously retrieves and recognizes TCM-related information from newly published literature and books. TCMBank offers a user-friendly interface and provides access to a vast amount of TCM-related data, including literature-driven relationship mapping between herbs/ingredients and genes/diseases. Besides, the TCMBank provides a powerful function for medicinal chemists to search for a series of traditional Chinese herbs containing some certain ingredients. Additionally, TCMBank features an ensemble learning-based drug discovery protocol for identifying potential leads and drug repurposing, aiming to accelerate drug discovery in the field of TCM. It can be accessed at https://TCMBank.CN.
2.1.2 ETCM v2.0.
The ETCM (Encyclopaedia of Traditional Chinese Medicine) v2.0 (ref. 55) is an updated TCM database. In response to the increased availability of a wider range of data, the update based on ETCM56 ensures a more extensive and integrated collection of TCM big data. ETCM v2.0 includes information on TCM formulas, Chinese patent drugs, Chinese medicinal materials, and ingredients. It also offers a method for target identification and prediction, as well as an enhanced JavaScript-based network visualization tool for exploring multi-scale biological networks. Overall, ETCM v2.0 has been designed with the overarching objective of enhancing the identification of bioactive constituents and quality markers of TCM. Additionally, it aims to provide extensive support for the research and development of novel drugs, facilitate the expedited repurposing of existing drugs, and streamline the investigation and application of TCMs in clinical settings. ETCM v2.0 is accessible at http://www.tcmip.cn/ETCM2/front/#.
2.1.3 BATMAN-TCM 2.0.
BATMAN-TCM (Bioinformatics Analysis Tool for Molecular Mechanism of Traditional Chinese Medicine) 2.0 (ref. 57) is an enhanced integrative database that focuses on known and predicted interactions between TCM ingredients and target proteins. BATMAN-TCM 2.0 collected 17
068 known and 2
319
272 predicted TTIs, together with 54
832 formulas, 8404 herbs and 39
171 ingredients, which are much larger than the previous version BATMAN-TCM 1.0.58 It is designed to address the need for a higher coverage TCM ingredient–target protein interaction (TTI) dataset and to use omics data to screen active TCM ingredients or herbs for complex disease treatment. The database boasts the most holistic known and predicted TTI dataset, and it added new features for exploring TCM ingredients and their targets simultaneously for pharmacology research, as well as TCM ingredients binding to target proteins for drug discovery. Besides, BATMAN-TCM 2.0 offers significantly increased TTI coverage and the website has been redesigned to provide a better user experience and faster speed. The database can be accessed at http://bionet.ncpsb.org.cn/batman-tcm.
2.1.4 TM-MC.
TM-MC59 is a database of medicinal materials and chemical compounds in Northeast Asian traditional medicine. It contains information on about 14
000 compounds from 536 medicinal materials, and provides links to the articles from which each medicinal material and chemical compound was extracted. The database aims to provide comprehensive information on the chemical compounds of medicinal materials used in Northeast Asia and is accessible at http://informatics.kiom.re.kr/compound.
2.1.5 LTM-TCM.
LTM-TCM (Linking of Traditional Chinese Medicine with Modern Medicine at molecular and phenotypic levels)60 is a comprehensive database that links TCM with modern medicine at both molecular and phenotypic levels. It contains high-quality data integration from fourteen TCM authoritative databases, 40
000 TCM clinical treatment records, and 213 ancient Chinese medical books. LTM-TCM provides information on symptoms, prescriptions, herbs, ingredients, targets, and their interactions. It offers online computational pipelines for virtual screening of ingredient-related targets and predictive analysis services for ADME properties. The aim of LTM-TCM is to provide a comprehensive and reliable database that aids in understanding the mechanisms of TCM at a system level. It seeks to support research, validate the effectiveness of TCM, facilitate medical research and mechanism prediction, and contribute to the modernization and popularization of TCM. It can be accessed through https://cloud.tasly.com/#/portalHome.
2.1.6 TCM-suite.
TCM-suite61 is a comprehensive platform for TCM component identification and network pharmacology analysis. It consists of two sub-databases, Holmes-suite and Watson-suite, which cover TCM biological ingredient identification and downstream network pharmacology investigation. The platform offers a user-friendly interface, search engines, and a holistic pipeline for exploring and visualizing TCM-related resources. TCM-suite aims to facilitate TCM-based drug discovery and repurposing and is freely accessible at http://TCM-Suite.AImicrobiome.cn.
2.1.7 HIT 2.0.
HIT (Herb Ingredients' Targets) 2.0 (ref. 62) was developed to focus on the targets of herbal ingredients and covers PubMed literature from 2000 to 2020. Compared to HIT 1.0 (ref. 63) released in 2011, HIT 2.0 incorporates advanced text mining algorithms and rigorous curation methods. HIT 2.0 includes an extensive update by adding literature from 2010 to 2020. This expansion has resulted in nearly double the amount of data available compared to the previous version. HIT 2.0 contains 10
031 compound–target activity pairs which have quality indicators and are derived from 2208 targets and 1237 ingredients sourced from over 1250 well-regarded herbs. It provides information on genes/proteins that are directly/indirectly activated/inhibited, protein binders, and enzyme substrates or products. Additionally, it offers features such as automatic Target-mining and My-target curation, which allow users to explore the latest literature from PubMed and retrieve abstracts containing potential targets for specific compounds. HIT 2.0 can be accessed at http://hit2.badd-cao.net.
2.1.8 SuperTCM.
SuperTCM64 is a biocultural database that combines biological pathways and historical linguistic data of Chinese Materia Medica for drug development. It provides comprehensive information on TCM derived from medicinal plants, including pharmacological recipes, chemical compounds, and active ingredients. The database covers 6516 herbs, 5372 botanical species, and 55
772 active ingredients against 543 targets in 254 KEGG pathways associated with 8634 diseases. SuperTCM exhibits the following features: it differentiates between scientific and non-scientific common names to prevent confusion arising from the use of multiple names for the same species. Additionally, the database incorporates KEGG Global Maps to identify the ingredients and targets of individual drugs and blended “herbs” or recipes, offering a comprehensive view of their impacts on pathways. SuperTCM aims to facilitate the study of TCM drugs and expand their applications and is freely available online at http://tcm.charite.de/supertcm.
2.1.9 TCMSID.
TCMSID,65 the Traditional Chinese Medicine Simplified Integrated Database, is a high-quality and comprehensive database that offers the following features: (a) integration of 499 TCM herbs and 20
015 unique herbal ingredients, which fills the gaps in previous databases; (b) in-depth information for each ingredient, including significance degree, ADME/T-related properties, structural classification, and reliability; (c) inclusion of reliable potential targets for each ingredient predicted by multiple target-prediction platforms. (d) Availability of bioassay data for herbal ingredients, enabling the study of hidden activity-related information using cheminformatics methods. (e) Establishment of an herb–component–target–drug multilevel interaction network, facilitating a deeper understanding of the mechanisms of action in TCM. Notably, the comprehensive data for each field was consolidated from other pertinent databases, as well as through text mining of published articles and the use of prediction tools like ADMETlab.66,67 Besides, TCMSID provides ingredient structural classification which is not available in most of the existing TCM ingredient databases. The ingredient structural classification of all ingredients was refined layer-by-layer using the ClassyFire web server,68 an automated chemical classification web tool. TCMSID also offers a user-friendly interface for easy access and analysis of the data, making it a valuable resource for TCM research and drug discovery. TCMSID can be accessed at https://tcm.scbdd.com.
2.1.10 TCMIO.
TCMIO69 (Traditional Chinese Medicine on Immuno-Oncology) is the first comprehensive database that integrates TCM data with immuno-oncology data. It contains over 120
000 small molecules against 400 immuno-oncology targets, extracted from public databases and literature. The database provides cheminformatics and bioinformatics tools for exploring the chemical ingredients and ligands against immuno-oncology targets, as well as pathway enrichment analysis for TCM or prescription. TCMIO aims to accelerate natural product drug discovery for cancer treatment and expand the understanding of traditional medicine. It is publicly accessible at http://tcmio.xielab.net.
2.1.11 HERB.
HERB70 is a high-throughput experiment- and reference-guided database of TCM, with its Chinese name as BenCaoZuJian. It integrates multiple TCM databases and contains a comprehensive list of herbs and ingredients. HERB reanalysed 6164 gene expression profiles from 1037 high-throughput experiments evaluating TCM herbs/ingredients and generated data-driven connections between TCM herbs/ingredients and modern drugs. By leveraging database mining and statistical inference techniques, HERB provided connections between 12
933 targets, 28
212 diseases, 7263 herbs, and 49
258 ingredients. These data-driven associations serve as valuable evidence, linking TCM and contemporary medicines. HERB outlines the evidence-based connections between TCM and modern medicines, offering compelling support for additional pharmacological research on TCM as a crucial component of contemporary drug discovery endeavors. It is accessible through http://herb.ac.cn/.
2.1.12 TCM-ID.
TCM-ID,71 the Traditional Chinese Medicine Information Database, is a comprehensive resource providing quantitative information about TCM. It includes details on TCM prescriptions, constituent herbs, herbal ingredients, molecular structures, functional properties of active ingredients, therapeutic and side effects, clinical indications, and related matters. The database contains information for 7443 prescriptions, 2751 herbs, and 7375 herbal ingredients. It serves as a valuable tool for studying and exploring TCM and is accessible online at https://bidd.group/TCMID/.
2.1.13 SymMap V2.0.
SymMap72 is an integrative database of traditional Chinese medicine enhanced by symptom mapping. It provides a comprehensive collection of information on TCM symptoms, herbs, ingredients, targets (genes), and diseases. SymMap integrates TCM with modern medicine at both the phenotypic and molecular levels, making it a valuable resource for evidence-based pharmaceutical research and drug discovery. The database can be accessed at http://www.symmap.org/and https://www.bioinfo.org/symmap.
2.1.14 TCMID 2.0.
TCMID 2.0 (ref. 73) is a comprehensive resource for Traditional Chinese Medicine. The database TCMID (Traditional Chinese Medicine Integrated Database),74 initially released in 2013, was designed as a thorough database with the goal of modernizing and standardizing TCM. It is a database that provides information on TCM prescriptions, herbs, ingredients, diseases, drugs, and targets. The latest release, TCMID 2.0, has significantly expanded the database with additional herbal ingredients, prescriptions, related targets, drugs, diseases, and new connections between them. It also includes data on herbal mass spectrometry (MS) spectra related to herbs and ingredients. TCMID 2.0 aims to facilitate TCM's modernization and enhance the exploration of the biological processes related to the pharmacologic actions of TCM. TCMID 2.0 is accessible through http://www.megabionet.org/tcmid.
2.1.15 TCMSP 2.3.
TCMSP,75 which stands for Traditional Chinese Medicine Systems Pharmacology, is a database designed to facilitate drug discovery from herbal medicines. It provides comprehensive information on Chinese herbs, their ingredients, targets, associated diseases, and ADME-related properties. The database aims to promote the integration of modern medicine and traditional medicine for drug discovery and development. TCMSP is freely available at https://tcmsp-e.com/tcmsp.php/ and offers tools for visualization and analysis of the data, making it a valuable resource for chemists, biologists, and pharmacologists.
2.2 Data quality and features
The databases vary in terms of data quality, characteristics, and features. As shown in Fig. 2, most databases contain five elements: TCM prescriptions, herbs, chemical ingredients, targets and diseases. Among them, TCMbank, HIT 2.0, SuperTCM, TCMSID, HERB, SymMap and TCMSP 2.3 lack TCM prescriptions, while ETCM v2.0, BATMAN-TCM 2.0, LTM-TCMand TCMID 2.0 have a large number of that. LTM-TCM, HIT 2.0, TCMSID and TCMIO lack data of diseases. Specifically, TCMbank has the highest number of herbs, and TCM-suite has the highest number of chemical ingredients (704
321). TCMbank, ETCM v2.0, and BATMAN-TCM 2.0 offer comprehensive and standardized information on herbs, ingredients, targets, and diseases. They use advanced algorithms and data retrieval methods to ensure data quality and accuracy. TM-MC provides information on medicinal materials and chemical compounds in Northeast Asian traditional medicine, contributing to the diversity of TCM data. LTM-TCM integrates data from fourteen TCM authoritative databases, ancient Chinese medical books, and TCM clinical treatment records, ensuring a comprehensive and reliable database. TCM-suite and HIT 2.0 offer comprehensive platforms for TCM component identification, network pharmacology analysis, and exploring TCM ingredients and their targets. SuperTCM combines biological pathways and historical linguistic data of Chinese Materia Medica for drug development, providing comprehensive information on TCM derived from medicinal plants. TCMSID offers a multilevel interaction network and in-depth information for each ingredient, ensuring data reliability and structural classification of ingredients. TCMIO integrates TCM data with immuno-oncology data, contributing to the expansion of TCM research into the field of cancer treatment. HERB provides high-throughput experiment- and reference-guided data of TCM, ensuring data-driven connections between TCM and modern drugs. SymMap V2.0 integrates TCM with modern medicine at both the phenotypic and molecular levels, providing valuable evidence for evidence-based pharmaceutical research and drug discovery. TCMID 2.0 has significantly expanded the database with additional herbal ingredients, prescriptions, related targets, drugs, diseases, and new connections between them. TCMSP 2.3 offers comprehensive information on Chinese herbs, their ingredients, targets, associated diseases, and ADME-related properties, facilitating drug discovery and development from herbal medicines.
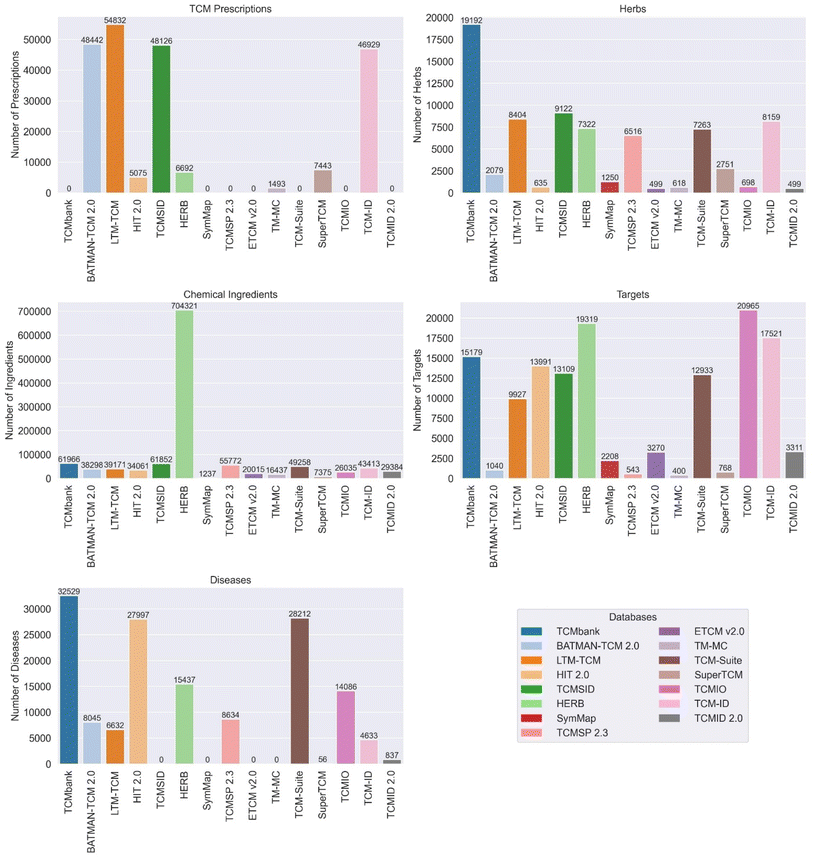 |
| Fig. 2 Comparison of the TCM prescriptions, herbs, chemical ingredients, targets, and diseases that the databases contain. | |
Each database has unique characteristics as shown in Table 1. Some databases focus on specific areas, such as integrating TCM data with immuno-oncology data (TCMIO), providing high-throughput experiment- and reference-guided data of TCM (HERB), and enhancing TCM with symptom mapping (SymMap V2.0). The databases offer a wide range of features, including comprehensive information, advanced text mining algorithms, and integration with modern medicine and advanced technologies. In summary, the databases vary in terms of data quality and characteristics, but collectively they contribute to the advancement of TCM research by providing comprehensive, reliable, and diverse information, as well as specialized tools for drug discovery and integration with modern medicine. These databases offer a wide range of features, including comprehensive information, user-friendly interfaces, advanced text mining algorithms, and integration with modern medicine and advanced technologies.
Table 1 The characteristics of major TCM databases
Database |
Latest update year |
Pros and cons |
Unique data/function |
Website |
TCMbank |
2023 |
It provides standardized information about TCMs, ingredients, diseases, targets, and their relationships. Continuously updated via intelligence text mining. But it lacks the information of TCM prescriptions |
Intelligent document identification, EL-based drug discovery protocol |
https://tcmbank.cn
|
ETCM v2.0 |
2023 |
It includes information on TCM prescriptions and 9872 Chinese patent drugs. It also offers a method for target identification and prediction |
Chinese patent drugs, drug similarity evaluation, network visualization tool |
http://www.tcmip.cn/ETCM2/front/#
|
BATMAN-TCM 2.0 |
2023 |
It focuses on known and predicted interactions between TCM ingredients and target proteins. But it lacks quantitative information about the components in each formula |
Compound–target pairs, predicted TCM ingredient–target protein interactions |
http://bionet.ncpsb.org.cn/batman-tcm
|
TM-MC |
2023 |
Provides comprehensive information on the chemical compounds of medicinal materials used in Northeast Asian traditional medicine. But the amount of herbal data is limited |
The largest number of medicinal materials using the chromatographic method |
http://informatics.kiom.re.kr/compound
|
LTM-TCM |
2022 |
It links TCM with modern medicine at both molecular and phenotypic levels. But it lacks disease data |
ADME of ingredients and target prediction |
https://cloud.tasly.com/#/portalHome
|
TCM-suite |
2022 |
It's a comprehensive platform for TCM component identification and network pharmacology analysis. It aims to facilitate TCM-based drug discovery and repurposing |
TCM biological ingredient identification and network pharmacology search |
http://tcm-suite.aimicrobiome.cn
|
HIT 2.0 |
2021 |
It focuses on the targets of herbal ingredients and covers PubMed literature from 2000 to 2020. It has incorporated advanced text mining algorithms and rigorous curation methods. But it lacks disease data |
Compound–target pairs |
https://hit2.badd-cao.net
|
SuperTCM |
2021 |
It combines biological pathways and historical linguistic data of Chinese Materia Medica for drug development. It provides comprehensive information on TCM derived from medicinal plants |
Drug–target–pathway visualization |
http://tcm.charite.de/supertcm
|
TCMSID |
2020 |
It offers integration of TCM herbs and herbal ingredients and provides in-depth information for each ingredient, including bioassay data and multilevel interaction network. But it lacks disease and TCM prescriptions data |
ADME/T properties for all ingredients, potential targets of ingredients, the structural reliability of all ingredients |
https://tcm.scbdd.com/home/index
|
TCMIO |
2020 |
It integrates TCM data with immuno-oncology data. It contains over 120 000 small molecules against 400 immuno-oncology targets. But it lacks disease data |
400 unique IO targets, 157 195 ligand–target interactions, network pharmacology analysis |
http://tcmio.xielab.net
|
HERB |
2020 |
It integrates multiple TCM databases and provides high-throughput experiment- and reference-guided data and connections between TCM herbs/ingredients and modern drugs. But it lacks TCM prescriptions data |
High-throughput experimental evidence |
http://herb.ac.cn
|
TCM-ID |
2019 |
It provides quantitative information about TCM, including details on TCM prescriptions, constituent herbs, herbal ingredients, molecular structures, and therapeutic and side effects. But it lacks updates |
Human healthy/disease samples |
https://bidd.group/TCMID
|
SymMap v2 |
2018 |
It integrates TCM with modern medicine at both the phenotypic and molecular levels. But it lacks updates |
TCM symptom-herb and TCM symptom-MM symptom associations |
https://symmap.org
|
TCMID 2.0 |
2018 |
It significantly expanded the database and includes data on herbal mass spectrometry (MS) spectra related to herbs and ingredients. But it lacks updates |
MS spectra data, computationally predicted TCM ingredient–target protein interactions |
http://47.100.169.139/tcmid
|
TCMSP 2.3 |
2014 |
It excels in identification of drug–target and drug–disease networks, and potential to uncover the mechanisms of action in Chinese herbs. But it lacks updates |
Computationally predicted TCM ingredient–target protein interactions |
https://tcmsp-e.com/tcmsp.php
|
2.3 AI-based integration and analysis of herbal chemical data
The application of machine learning and natural language processing (NLP) in integrating and analysing data from various TCM databases enables autonomous processing and interpretation of vast amounts of unstructured and semi-structured data, identifying previously unrealizable patterns, relationships, and insights. This capability is crucial for synthesizing knowledge from historical texts, experimental data, and clinical findings, thereby advancing the understanding and application of TCM.76,77 AI technologies help uncover deep correlations between chemical components, diseases, and targets within the field of herbal medicine. Through pattern recognition and predictive models, AI reveals the complex interactions and efficacy pathways of TCM components, aiding in the development of new treatment strategies and personalized medical approaches. AI-driven deep analysis not only accelerates the discovery of new compounds and formulations but also enhances the precision and effectiveness of TCM treatments.78,79
The integration of AI technologies significantly enhances the querying capabilities of TCM medicine databases. Intelligent search functions supported by machine learning algorithms provide semantic understanding and personalized recommendations, making it easier for researchers to navigate and extract relevant information. Huang et al.80 developed a TCM prescription data collection mini-program, using NLP for intelligent information extraction. Semantic search engines interpret the intent and contextual meaning of queries, thus improving the accuracy and relevance of search results. AI-based tools are revolutionizing the way TCM chemical data is analysed. These tools support advanced analytical functions such as complex querying, data visualization, and interactive analysis platforms. Utilizing deep learning and NLP, these tools can process and analyse large datasets, revealing patterns and insights that promote drug discovery and understanding of TCM pharmacological mechanisms.81,82 The integration of AI with TCM databases has led to successful chemical data analysis and drug discovery projects. Bai et al.83 utilized AI to analyse the quality markers of TCM materials using ultra-high performance liquid chromatography and near-infrared spectroscopy (NIRS), providing intelligent strategies for quality assessment based on these markers. Wang et al.24 used AI to analyse spectroscopic data, identifying and quantifying complex chemical structures and components in TCM, enhancing the reliability and accuracy of compound identification. Li et al.84 combined chatGPT technology for medical data mining, integrating machine learning with historical TCM data to analyse and identify ancient TCM prescriptions from the Song Dynasty. FordNet is an AI-based system that integrates phenotypic and molecular information for TCM formulation recommendations, significantly improving hit rates and formulation precision.43 Combining advanced liquid chromatography and mass spectrometry with AI-driven data processing significantly improves the accuracy and efficiency of TCM formulation analysis. This integrated approach provides a detailed characterization of the chemical foundations of TCM, better understanding its components and their interactions, which are crucial for quality control and formulation optimization.85 Data fusion techniques in TCM research involve integrating data from various analytical techniques (including spectroscopy and chromatography) to create a more accurate and comprehensive view of TCM compounds. This method enhances the identification and analysis of complex interactions in TCM formulations, promoting more precise and predictive models for TCM research and development.86
2.4 Challenges and solutions
The quality and standardization of data in these databases are critical for their effective use in research and development. These challenges hinder the efficiency and reliability of research outcomes, necessitating stringent quality control measures to ensure the integrity of data used in TCM research.87 The integration of AI in analysing and refining these data ensures high-quality, reliable datasets that can be used for drug discovery, clinical trials, and a broader understanding of TCM mechanisms. The application of AI in data management and analysis highlights the importance of high-quality data in obtaining meaningful insights from TCM research.88 Interoperability among different TCM databases presents challenges primarily due to differences in data formats, terminologies, and standards. These differences hinder seamless data exchange and integration, limiting comprehensive analysis of TCM components and their efficacy. The complexity arises from the diversity of data sources, including traditional texts, experimental results, and clinical observations, each encoded in various formats and structures.89,90 Enhancing interoperability and data sharing among TCM databases is critical for fostering collaborative research and maximizing the utility of collected data. Refining data sharing protocols and establishing common standards are initiatives beneficial for aggregating and comparing data from different sources, enhancing the comprehensiveness and depth of TCM research.91,92 AI facilitates these processes through automated data integration and analysis, ensuring that TCM databases remain at the forefront of modern medical research.
3. Traditional Chinese medicine modernization
3.1 The challenges and opportunities of TCM modernization
As TCM integrates more deeply with modern scientific methodologies, it becomes essential to address the broader context in which these advancements take place. The path to modernization is fraught with challenges but also brimming with opportunities that could redefine the future of TCM. In this section, we will explore the key hurdles that TCM faces in its modernization journey and the potential avenues for overcoming these challenges. Some stumbling blocks hinder the progress of TCM in a scientific manner:
(a) Some individuals opt for TCM over Western treatments due to a fear of surgical procedures or injections.
(b) The scarcity of uniformly qualified TCM practitioners has resulted in patient fatalities stemming from delayed Western medical intervention. Additionally, some TCM practitioners surreptitiously incorporate Western medications into TCM formulations.
(c) Numerous renowned TCM practitioners rely on secret family recipes, hindering transparency and standardization.
(d) Certain TCM herbs pose toxicity risks.
(e) Some TCM practitioners staunchly maintain the uniqueness of TCM, resisting modernization through scientific methods even though, many TCM educational programs now integrate Western subjects such as biochemistry and physiology.
At the same time, we think there are several chances for the modernization of TCM, which can be summarized as follows:
(a) The long history of successful TCM treatments in the East underscores the efficacy of certain formulas with robust clinical evidence.
(b) Acupuncture and specific TCM formulations show promise for modernization efforts.
(c) Collaborative efforts with Western-trained scientists, exemplified by initiatives like GP-TCM led by Professor Tai-Ping Fan of the University of Cambridge and the Globalization of Chinese Medicine (CGCM) advocated by Academician Yong-Qi Zheng of Yale University, have fostered a scientific approach to TCM in the Western world, promoting its progress.
Addressing these challenges and capitalizing on these opportunities requires a concerted effort from both the TCM and scientific communities. In the following sections, we will explore specific strategies and steps being taken to modernize TCM, with a focus on integrating modern technology and scientific methodologies.
3.2 The next step of TCM modernization
Building upon the challenges and opportunities discussed, the next phase in the modernization of TCM focuses on leveraging advanced technological frameworks to accelerate research and development. We are currently trying to construct the roadmap for TCM modernization (Fig. 3). After establishing the world's largest Traditional Chinese Medicine database (TCMbank),93 we will then proceed to build a cloud-based drug screening system (iScreen),94 and an integrated portal for TCM and systems biology (iSMART).95 iScreen is the first cloud-computing platform dedicated to virtual screening and de novo drug design based on a TCM database. This system leverages molecular docking, chemoinformatics, and virtual screening technologies to help researchers rapidly identify potentially active compounds and perform preliminary drug design. iSMART is an integrated cloud computing web server designed to merge TCM with systems biology. It supports online virtual screening, de novo evolution, and drug design. iSMART combines complex biological networks and a large-scale database of TCM components, providing a powerful tool to help researchers understand the mechanisms of TCM and develop new therapeutic approaches. Next, we will establish a TCM metabolome database, combining vast disease-related signaling pathways and the complex interactions of compounds in Traditional Chinese Medicine formulas within the body. Only by considering all three aspects can we fully understand the mechanisms of TCM. The integration of these three requires the use of modern technologies such as big data. Big data is characterized by the 4V: volume, velocity, variety, and veracity. It is evident that we need to apply big data techniques to the vast amount of formula data, which reaches up to 140
000 entries. Currently, we are working towards the integration of the latest technologies like big data, cloud computing, biochemical data, extensive TCM formula database, vast TCM ingredient database, and a large-scale TCM metabolome database. We firmly believe that these methods can be realized in the near future.
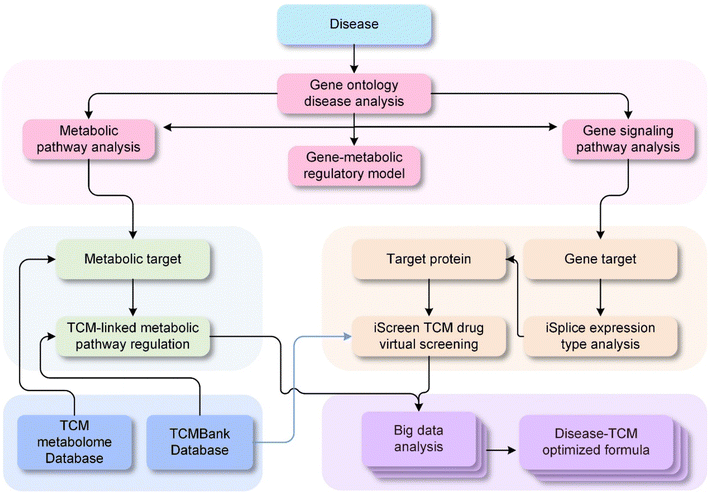 |
| Fig. 3 The road map of TCM modernization. This diagram outlines the integration of computational methods and databases in modernizing TCM. Starting with disease analysis, it utilizes gene ontology and signaling pathways to explore metabolic and gene targets. These inform the regulation of TCM-linked metabolic pathways, analyzed through TCMbank and metabolome databases. Virtual drug screenings via iScreen and big data analysis refine TCM formulas, integrating modern technology to advance TCM applications. | |
3.3 The outlook of TCM modernization
Currently, Chinese medicine network research mostly aims to prove Chinese medicine theories and is lacking in terms of defining network design and optimization principles. Rational prescription design can be divided into two types: top down and bottom up. The top down approach designs new prescriptions based on old prescriptions96 whereas the bottom up approach does not take old prescriptions into consideration when designing completely new prescriptions based on disease networks.97 We combined various feasible methods for designing networks and first defined several common top down optimization formulas models that will be most common in the future (Fig. 4). A commonality between the top down and bottom up design approaches is that they both establish relevance based on biological networks. According the analysis of degree of freedom, all the variable (N) can be calculated to be N-1 for analytical solution instead of numerical solution. Their differences lie in their considerations of current prescriptions and Chinese medicine theories. Before Chinese medicine theories are expressed quantitatively, novel formulas designed without taking old formulas into considerations will have greater chances of contradicting Chinese medicine principles than those designed using the top down approach.
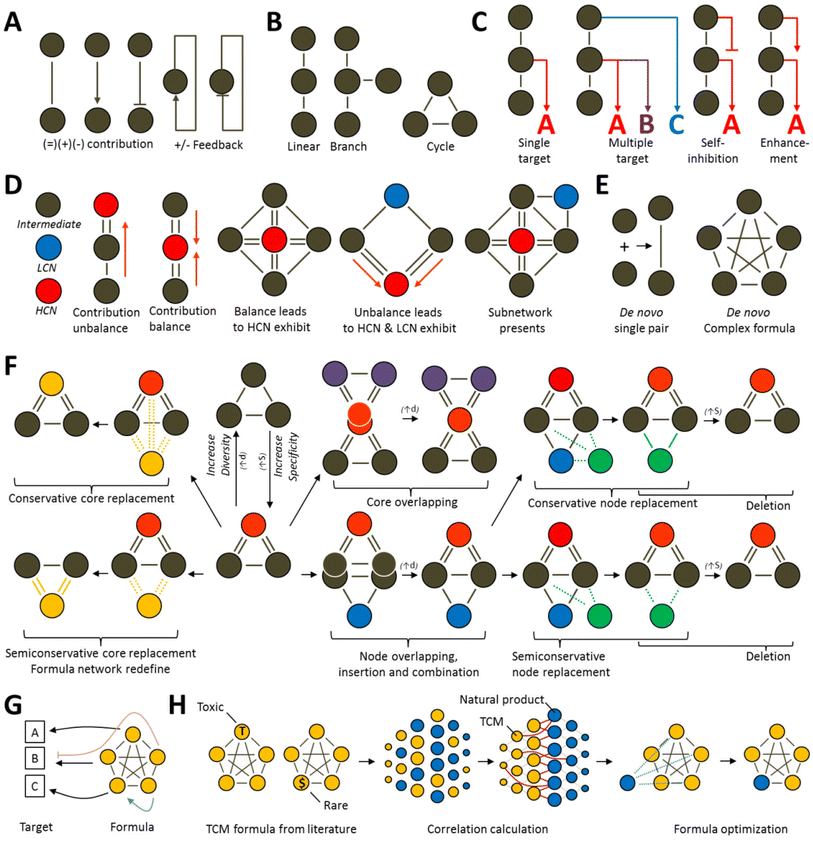 |
| Fig. 4 Overview of TCM prescription network design and optimization approaches. (A) Five basic elements that prescription networks are composed of. (B) Three fundamental natural processes that prescriptions are composed of. (C) Four basic models for operating on targets. (D) Prescriptions can be simplified into three elements in order to facilitate design: highly contributed nodes (HCN), lowly contributed nodes (LCN), and intermediate (which fall in between the previous two); two types of conditions appear in non-ideal networks: one is contributions being capable of balancing the network, and the other is trending towards HCN and not being able to become balanced; non-ideal networks can be sub-divided into various sub-networks. Prescription design is divided into (E) bottom up formulas design and (F) top down formulas design. Top down formulas design can be further divided into five basic design models: replacement, addition, enhancement, reduction, and removal. Both top down and bottom up prescription design has the potential to (G) be able to take different Chinese medicine theories into consideration when engaging the adjustment and control of different disease networks and abnormal nodes. (H) Natural product categorization and organization is capable of expanding the designable range of most TCM prescription optimizations. Biological data, providing a holistic view of patient health and enhancing the precision of TCM treatments. | |
In recent years, Chinese medicine has continually trended towards the categorization and organization of natural products. Therefore, under the support of our analysis results, TCM has much in common with natural product. In the face of over-development by humans, enormous species is endangered, it is why we suggest it is necessary to establish a method for calculating the correlation between natural products and individual entities of TCM species, as well as use highly reliable models to rapidly screen prescription ingredients that are replaceable, those that can be optimized, and those that can be removed. Therefore, a novel formula design of prescriptions will surely become a core area of research that will bring a high level of sustainability to herbal medicine.
4. AI technologies in TCM: principles and general applications
4.1 Emerging AI technologies in TCM
The integration of advanced AI technologies into TCM heralds a new era in healthcare. Cutting-edge technologies like graph neural networks (GNNs), deep learning, and multi-modal AI approaches are revolutionizing TCM, offering novel insights and enhancing efficacy. GNNs have shown great promise in TCM, particularly in understanding complex herbal interactions and patient data. GNNs are adept at processing non-Euclidean data, making them ideal for analysing the intricate networks in TCM. Some studies98,99 have employed GNNs to investigate potential inhibitors and auxiliary diagnosis in TCM, exemplifying their potential in identifying novel therapeutic pathways and enhancing diagnostic accuracy.
Deep learning, a subset of AI, is significantly contributing to TCM, especially in diagnosis and treatment planning. CNNs and recurrent neural networks (RNNs), as applied in studies,100,101 have shown efficacy in classifying TCM formulas and analysing medical records. These technologies allow for the processing of vast amounts of data, ranging from patient records to images, thus enabling more accurate and efficient TCM practices. Multi-modal AI approaches, which integrate various data types, are emerging as powerful tools in TCM. These approaches, as demonstrated in the work,102,103 combine image, text, and AI technologies have been instrumental in drug discovery and genome sequencing within the scope of TCM. The studies104,105 highlight AI's role in genome sequencing and drug development. AI's capability to rapidly process genetic data and simulate drug interactions is accelerating the discovery of new medicinal compounds from TCM. AI's application in TCM syndrome differentiation and treatment recommendation is a significant advancement. The AI models facilitate the analysis of complex symptom patterns and recommend appropriate TCM prescriptions, thereby personalizing treatment strategies.44,106 Emerging AI technologies are enhancing TCM diagnostics, with tools like automated image recognition and symptom entity classification. Some AI models has been developed for identifying herbal medicines and classifying symptoms, greatly improving diagnostic accuracy and efficiency in TCM.107,108
With the advancement of AI technologies, there is an increasing demand for high-quality data from databases to support accurate machine learning models and predictive analytics. This trend places additional pressure on database providers to enhance the quality, consistency, and standardization of the chemical structure data they offer. For instance, some databases, such as TCM-ID and TCMID 2.0, provide chemical structures in SMILES format, while others, like ETCM 2.0 and TCMSID, offer molecular formulas. TCMBank supplies data in mol2 format, which includes three-dimensional structural information, and BATMAN-TCM 2.0 provides the IUPAC names of chemical ingredients. As AI continues to evolve and play a more significant role in TCM research, improving the quality of chemical structure data will be essential for fully harnessing the potential of these technologies.
4.2 AI models in TCM chemical data analysis
Table 2 illustrates various AI models reshaping TCM research, highlighting their innovative aspects and applications. In the realm of TCM, AI models are innovating the approach to understanding complex chemical interactions within traditional practices. The application of graph attention networks (GANs) has refined the herb recommendation process, tailoring treatment plans by analysing the intricate chemical relationships between various herbal constituents. This approach underscores the importance of personalized healthcare, ensuring that patients receive chemically synergistic and personalized herbal concoctions.9 Representation learning has streamlined the process of TCM knowledge acquisition, serving as a cornerstone for constructing comprehensive TCM knowledge graphs that facilitate efficient data organization and retrieval, essential for both research and clinical practice.109 Meanwhile, the innovative use of generative adversarial networks (GANs) for TCM prescription generation represents a breakthrough in creating tailored treatment plans, taking into account the vast chemical data encoded within traditional remedies.110 Transfer learning has shown significant potential in the identification of herbal medicines, where the nuanced chemical signatures of herbs are accurately recognized, thereby improving the integrity and effectiveness of TCM treatments.111 In the realm of formula discovery, hybrid-scales graph contrastive learning has emerged as a powerful tool for uncovering the regularities in TCM formulas, revealing the underlying chemical principles that govern effective herbal combinations.112 Finally, Transformer-Based Models and Deep Crossing Neural Networks are pioneering personalized inpatient care and prescription algorithms in TCM. These models adeptly handle the vast chemical datasets to improve the quality of healthcare delivery.103,113
Table 2 Innovative AI models and techniques in TCM research. It provides an insight into the innovative AI models and techniques that are shaping the field of TCM research. The specific applications of these AI technologies in TCM are detailed, along with key studies that have explored and validated these models
AI model |
Innovative aspect |
TCM application |
Key findings |
Key study |
Graph attention networks |
Applying attention mechanisms on graph-structured data |
Herb recommendation in TCM |
Used for recommending herbs based on their interactions and effects, facilitating personalized TCM treatments |
Jin et al.9 |
Representation learning |
Learning representations of data for easier information extraction |
TCM knowledge graph construction |
Employed for building TCM knowledge graphs, enabling better data organization and retrieval for research and practice |
Shi et al.109 |
Generative adversarial network (GAN) |
AI framework for generating data using two neural networks |
TCM prescription recommendation |
Developed for generating TCM prescription recommendations, contributing to personalized treatment plans |
Rong et al.110 |
Transfer learning |
Leveraging a pre-trained model on a new, related problem |
Image recognition in TCM |
Utilized for efficient image recognition in TCM, particularly in the identification of herbal medicines |
Xing et al.111 |
Hybrid-Scales graph contrastive learning |
Learning graph representations by contrasting different scales of graph structures |
TCM formula discovery |
Used for discovering regularities in TCM formulas, facilitating the understanding of herbal combinations |
Wu et al.112 |
Transformer-based models |
Models based on transformer architecture for handling sequential data |
Inpatient TCM prescription recommendation |
Implemented for recommending inpatient TCM prescriptions, enhancing decision-making in clinical settings |
Zhang et al.113 |
Deep crossing neural network |
Architecture for handling sparse, high-dimensional categorical data |
TCM prescription algorithm |
Utilized for recommending TCM prescriptions, enabling more accurate treatment suggestions |
Zhang et al.103 |
4.3 Large language models in TCM chemical data analysis
The application of LLM in TCM represents a transformative development in the integration of AI technologies within the domain of medical herbalism. Wang et al.114 utilized various LLMs including ChatGPT, Tsinghua University's General Language Model (GLM), and Shanghai Artificial Intelligence Laboratory-InternLM, ChatGLM-6b and ChatGLM2-6b to classify TCM prescriptions into predefined efficacy groups, as depicted in Fig. 5. The process involves fine-tuning these models using specialized prompt templates that encapsulate complex TCM prescription information, including prescription name, ingredients, efficacy, and indications. They compiled an extensive dataset of 2308 TCM formulas derived from authoritative sources like the Chinese National Medical Insurance Catalog and various TCM textbooks. LLMs were initially trained on a common dataset to learn from a broad language background and subsequently fine-tuned on a specialized dataset of TCM prescriptions. The fine-tuned model showed a significant improvement in accuracy, reaching 71% accuracy for the ChatGLM2-6b model. These results highlight the potential of the LLM to transform TCM practice by providing a more precise and efficient tool for classifying prescriptions. The ZhongJing model115 is a custom-developed LLM that operates effectively within the TCM context, finely tuned to integrate domain-specific knowledge crucial for medical diagnosis and educational training. The introduction of the TCMEval method has enabled this model to demonstrate significant enhancements in performance by generating responses that are highly relevant to TCM, thereby addressing some of the unique challenges associated with the integration of such domain-specific information. TCM-GPT116 leverages a vast corpus of TCM-focused texts to foster a deeper understanding and categorization of complex medical literature typical of TCM. This model's domain-specific pre-training allows for substantial improvements in task performance, illustrating the critical role of tailored pre-training in enhancing the accuracy and reliability of medical applications within TCM. The Qibo model117 emerges as an innovative solution specifically designed for the unique theoretical framework of TCM compared to modern medicine. Through stages from pre-training to supervised fine-tuning, the model uses a specialized corpus to encapsulate the extensive knowledge and nuanced details of TCM, making it invaluable in clinical and educational settings.
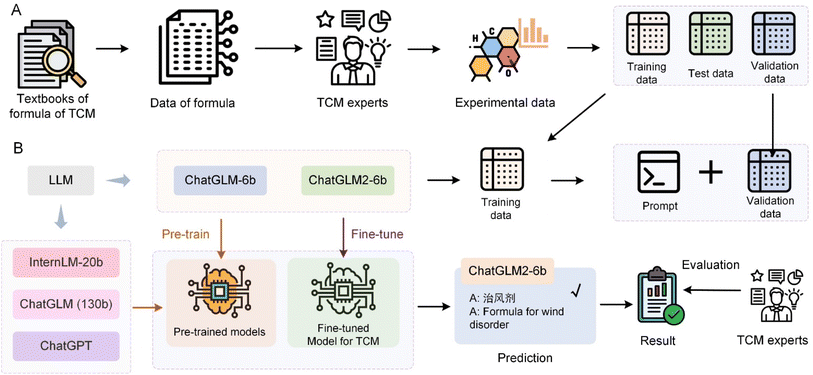 |
| Fig. 5 Case study of AI integration in TCM formula classification using LLM. (A) Illustrates the data collection from textbooks of TCM formulas, input from TCM experts, and compilation of experimental data into training, test, and validation datasets. (B) Depicts the utilization of various LLMs, including pre-trained models such as ChatGLM-130b and InternLM-20b, and fine-tuning specific models like ChatGLM-6b and ChatGLM2-6b for TCM application. The workflow demonstrates how experimental data feeds into model training and validation, with TCM experts evaluating the final outcomes. | |
Furthermore, in China, the “Shuzhibencao” LLM developed by Tasly Pharmaceutical Group Co., Ltd and Huawei Cloud, harnesses a vast array of data including 100
000 TCM formulas and over 40 million literature summaries. This model demonstrates groundbreaking advances in mining and summarizing large-scale TCM texts, significantly improving the chemical data analysis and optimization of TCM formulations. The “Bencaozhiku” LLM initiative represents a collaborative effort led by the Chengdu University of TCM, creating a digital repository that holds over 15 million genetic records of herbal species and 40 million compounds. This platform is integral in bridging the gap between core TCM research data and key industrial processes, enhancing the overall quality and safety of TCM products. Lastly, the “Shuzhiqihuang” LLM, a collaborative development by prestigious institutions such as East China Normal University, uses pre-training and fine-tuning techniques along with enhanced retrieval capabilities. It serves key roles in TCM by supporting functionalities such as prescription recommendations and syndrome diagnosis, thus fostering innovation and the preservation of TCM cultural heritage. These models illustrate the profound impact that specialized LLMs can have on the field of TCM, transforming traditional practices by leveraging modern AI capabilities to improve data processing, diagnostic accuracy, and the educational dissemination of TCM knowledge. Through focused adaptation and advanced training within the TCM domain, these models not only enhance current practices but also ensure the continuity and evolution of TCM in the modern era.
4.4 AI-driven TCM application scenarios in chemical data analysis
Table 3 provides a comprehensive overview of AI applications across different aspects of TCM. AI technology is being skilfully applied in various scenarios of TCM, from herbal identification to syndrome differentiation and treatment. Each scenario affects the chemical basis of this field. In herbal identification, automated image recognition significantly improves the speed and accuracy of herbal classification, which is an integral part of formulating chemically accurate TCM solutions.107 Syndrome differentiation benefits from the customized methods provided by BiLSTM-CRF and Transformer models, which analyse patient data to classify TCM syndromes, directly affecting personalized treatment by considering the chemical composition of the patient's condition.52,118 This precise classification is critical for chronic disease and cancer treatment, where chemical data can guide the selection and combination of herbs to develop effective personalized medicine strategies.
Table 3 AI-based TCM application scenarios and fields. It provides a comprehensive summary of AI-based application scenarios and fields within TCM, highlighting the specific diseases or conditions focused on and the advantages brought by AI applications. The table showcases how various AI models and methods are being employed across different aspects of TCM, including herbal medicine identification, syndrome differentiation, and prescription recommendation
Application scenario |
AI model |
Disease/condition focus |
Advantages of AI application |
Key study |
Herbal medicine identification |
Automated image recognition |
General TCM practice |
Enhances accuracy and speed in identifying various herbs, leading to more precise formulations |
Chen et al.107 |
Syndrome differentiation |
BiLSTM-CRF, transformer models |
Chronic diseases, cancer |
Facilitates personalized treatment by accurately categorizing TCM syndromes based on symptoms and patient data |
Deng et al.;118 Liu et al.52 |
Prescription recommendation |
GAN, deep crossing neural network |
Various chronic and acute conditions |
Enables tailored herbal prescriptions by analysing patient profiles and historical treatment data |
Rong et al.;110 Zhang et al.103 |
Pharmacological analysis |
Network pharmacology, FordNet |
Drug discovery, complex diseases |
Aids in understanding the multi-target and multi-pathway actions of herbal compounds in TCM |
Jin et al.;9 Zhou et al.119 |
Knowledge graph construction |
Representation learning |
General TCM knowledge |
Enhances the organization, retrieval, and interpretation of vast TCM knowledge, supporting research and education |
Shi et al.109 |
TCM formula discovery |
Hybrid-scales graph learning |
Herbal formula optimization |
Assists in discovering effective herbal combinations and understanding their synergistic effects |
Wu et al.112 |
Prescription recommendation is another area where AI has been greatly enhanced, using models such as GANs and deep cross neural networks. These AI tools consider historical treatment data and patient profiles (often including detailed chemical and pharmacological information) to recommend customized herbal prescriptions. Such AI applications ensure that proposed treatments are not only chemically suitable for patients but also consistent with past successful interventions.110 In pharmacological analysis, AI models such as network pharmacology and FordNet help describe the multi-target and multi-pathway effects of herbal compounds. They navigated the complex chemical data involved in TCM drug discovery, elucidating the complex interactions of herbal compounds in treating complex diseases.9,43 Finally, in knowledge graph construction, representation learning enhances the organization, retrieval, and interpretation of large amounts of chemical data in TCM knowledge bases, supporting ongoing research and education.109 Likewise, mixed-scale graph learning provides valuable insights into the optimization of herbal formulations, helping to discover effective herbal combinations by analysing their chemical composition and interactions.112
5. AI analysis of TCM chemical data
5.1 AI processing of chemical data
Herbal medicine databases are crucial for TCM research and applications, offering deep insights into the history and global influence of TCM and facilitating drug discovery and pharmacological research. Particularly in preserving information on medicinal materials, chemical components, and efficacy, these databases have made significant contributions to herbal research and application.70Fig. 6 shows the general process and application of TCM chemistry data analysis. Due to the complexity and diversity of herbal chemical components, these databases face challenges that require sophisticated data analysis methods to extract meaningful information.120 AI technologies, especially machine learning and neural networks, have been applied to pre-process, extract features from, and analyse herbal chemical data (Fig. 6A). This includes data cleansing, normalization, and transformation to facilitate subsequent analysis, as well as feature extraction and complexity reduction for more effective analysis.121 Furthermore, AI models can identify patterns and cluster similar compounds, which aids in predicting biological activity and identifying new drug candidates.122 Predictive models built using AI can forecast the pharmacological activities of compounds in TCM, thus facilitating the discovery of novel therapeutic drugs. These models utilize the vast and complex datasets in TCM databases, applying advanced analytics to identify potential drug candidates with desired biological activities.43
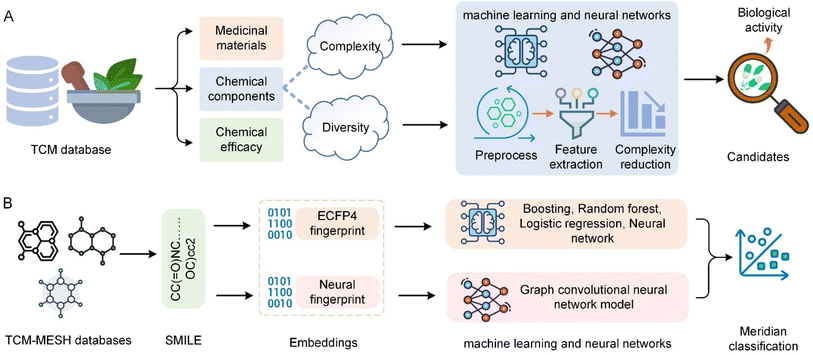 |
| Fig. 6 AI analysis of TCM chemical data and analysis of application examples. (A) AI-driven TCM chemical data analysis workflow. For the complexity and diversity of herbal chemical components, machine learning and neural networks have been applied to the preprocessing, feature extraction and analysis of herbal chemical data, ultimately predicting biological activities and identifying new drug candidates. (B) Case study. Predicting associations between meridians and TCM using cost-sensitive graph convolutional neural networks. | |
5.2 Case studies and challenges
Deep learning technologies like convolutional neural networks (CNNs) have significantly advanced the field of chemical structure analysis. These technologies assist in understanding the functions of compounds through accurate chemical structure analysis. CNNs can process images of chemical structures to identify patterns and features that are difficult to discern using traditional methods, thereby enhancing the drug discovery process.123,124 NLP techniques, including advanced models like Generative Pre-trained Transformers (GPT), help mine chemical and pharmacological information from TCM literature and databases. These AI models can extract valuable data from textual information, thus facilitating a deeper understanding of TCM compounds and their potential therapeutic effects.81,125 Yeh et al.126 explored the application of graph convolutional neural networks (GCNs) to classify TCM meridians by analysing the topological features of molecular compounds (Fig. 6B). This study utilized TCM-MESH database information where compounds were encoded into Simplified Molecular Input Line Entry Specification (SMILES) and transformed into binary and neural fingerprints. Extended-connectivity fingerprints (ECFPs) were used for traditional machine learning methods, which included a variety of models such as boosting and random forests to construct feature matrices and predict meridian associations. Deep learning approaches were employed using GCNs to analyse the neural fingerprints derived from SMILES. This dual approach allowed for comprehensive modelling of TCM compound interactions and significantly improved the prediction of TCM meridian classifications, demonstrating the efficacy of combining traditional and advanced AI methods in TCM research. Zhao et al.127 proposed PreGenerator, a hybrid neural network architecture for TCM prescription recommendation based on retrieval and generation methods. It combines a novel hierarchical retrieval mechanism and deep neural networks, demonstrating its ability to recommend effective TCM prescriptions, including useful herbs not listed on prescription labels. Bai et al.128 enhanced TCM entity relation extraction by combining CNNs with a segmented attention mechanism, improving the ability to extract local semantic features from TCM texts.
The integration of AI in the analysis of herbal chemical data faces challenges related to data quality, model accuracy, and interpretability.129 Addressing these issues requires the development of more sophisticated AI models and data processing techniques. Furthermore, interdisciplinary collaboration between AI technicians and TCM researchers is crucial for fully utilizing AI's potential to uncover new insights from TCM databases.130,131 The application of AI in TCM chemical data analysis not only enhances understanding of traditional formulations but also paves the way for new therapeutic discoveries. As AI technology continues to evolve, its application in TCM research is expected to expand, offering new opportunities for the integration of traditional and modern medical practices. The synergistic interaction between TCM and AI has the potential to unlock the full potential of ancient wisdom through the lens of contemporary science, fostering innovations that could significantly impact global healthcare. The future of AI in TCM research is poised for advances in algorithm innovation and computational platforms. These developments will enable more comprehensive analysis of TCM compounds, thereby discovering new drugs and therapeutic strategies.
6. AI in the analysis and identification of TCM chemical components
6.1 AI analysis of TCM chemical components
Advancements in AI have had a significant impact on the analysis of chemical components, offering sophisticated tools for deciphering the complex chemical matrix inherent in TCM. These AI-driven methods are crucial for enhancing the precision and efficiency of chemical analyses, thereby aiding in a deeper understanding of the pharmacological actions and mechanisms of TCM.129,132 The integration of AI technologies in herbal medicine research is expected to significantly enhance the analysis of chemical components, providing pathways for the discovery of new compounds and elucidating the complex interactions within TCM formulations. This potential is rooted in AI's ability to handle large datasets, identify patterns, and make high-precision predictions, thus opening new avenues for drug discovery and personalized medicine.82Fig. 7 shows the general process machine and its application cases for TCM chemical component identification. CNNs have shown exceptional promise in identifying complex chemical structures from spectroscopic and chromatographic data. These models excel in analysing chemical images and spectroscopic data, assisting in the high-precision, rapid identification of TCM components.133 AI's role in analysing spectroscopic data, such as mass spectrometry and nuclear magnetic resonance spectra, underscores its ability to decipher complex chemical signals, enabling the rapid and accurate identification of TCM compounds.134 AI aids in the analysis and comparison of chemical fingerprints, a key aspect of identifying and classifying specific components in TCM formulations (Fig. 7A). This application of AI enhances the ability to distinguish similar compounds and identify unique chemical markers.135
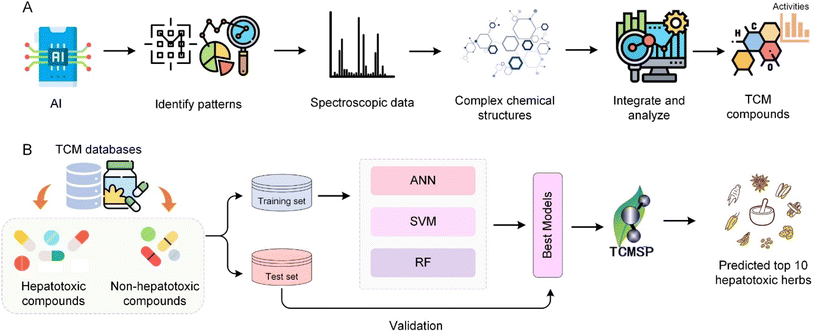 |
| Fig. 7 AI analysis and application cases of TCM chemical composition identification. (A) AI-driven pattern recognition workflow for TCM compound analysis. From data collection to integration and analysis of complex chemical structures and their biological activities. (B) Case analysis. An example of computer identification of hepatotoxic components of TCM, including the process of data collection from the TCM database to model validation and virtual screening of hepatotoxic herbs. | |
6.2 Structure–activity relationships in TCM chemical components
AI's application in establishing relationships between chemical structures and their biological activities is a key research area. Machine learning methods, including deep learning, have been used to predict the pharmacological activity of compounds, thus deepening the understanding of their therapeutic potential.136 AI technologies facilitate data mining to reveal previously unknown relationships between chemical components and their activities. This approach not only aids in new drug discovery but also enhances the understanding of TCM's multifaceted effects on biological systems.137 The fusion of different AI models and algorithms provides a robust framework for a more comprehensive analysis of TCM chemical components. Integrative learning approaches that combine the strengths of various AI technologies can improve the accuracy of identifying and understanding the multifaceted nature of TCM compounds. This interdisciplinary approach explores the complex relationships between chemical structures and their biological activities, aiding in a deeper understanding of TCM's therapeutic potential.138 AI's role in merging chemical data from different techniques, such as mass spectrometry, nuclear magnetic resonance spectroscopy, and chromatography, enhances the accuracy and reliability of identifying TCM components. Through data fusion, AI algorithms effectively integrate and analyse data from multiple sources, refining the identification process and providing a more comprehensive understanding of the chemical components in TCM formulations. This approach highlights AI's potential to overcome traditional barriers in data analysis, providing pathways for more precise and reliable insights into TCM's complex chemical landscape.139
6.3 Case studies and challenges
Machine learning and deep learning are employed to screen complex TCM chemical components to identify those with potential therapeutic effects. By using predictive models to assess the biological activity of compounds, AI aids in the rapid screening of a large number of substances, highlighting those with significant medicinal value. This process not only accelerates the discovery of bioactive components but also enhances the efficiency of drug development efforts, showcasing AI's key role in modernizing research and application of TCM.140 AI's application in TCM formula screening underscores its transformative impact on the discovery of pharmacologically active compounds and new drug development. Through high-throughput data analysis, AI significantly improves research efficiency and can quickly identify promising drug candidates. This streamlined drug discovery approach exemplifies the synergy between AI and TCM, paving the way for utilizing the rich heritage of traditional medicine in innovative therapies.141
Wu et al.142 utilized AI and machine learning to enhance the prediction and analysis of hepatotoxic compounds within TCM. Using a dataset comprised of 619 hepatotoxic and 1857 non-hepatotoxic compounds sourced from comprehensive adverse reactions databases like SIDER, the researchers employed consensus modelling techniques integrating artificial neural network (ANN), support vector machine (SVM), random forest (RF) and k-nearest neighbors (kNN) algorithms. This approach was applied to scrutinize the TCMSP database, effectively identifying compounds with potential hepatotoxic effects. The machine learning models, refined through rigorous training and testing phases as depicted in Fig. 7B, enabled a deeper exploration into the hepatotoxic mechanisms of TCM, culminating in a systems pharmacology analysis that identified the top 10 potentially hepatotoxic herbs. This study highlights the power of integrating AI to predict and understand complex pharmacological phenomena in TCM, offering a robust framework for safety evaluations in herbal medicine. Liu et al.143 performed UPLC/Q-Orbitrap-MS/MS to identify the preliminary chemical characterization of Kangshuai Lao Pian. A total of 138 compounds were identified based on retention time, accurate mass, and characteristic MS/MS fragment patterns, including ginsenosides, phenylethanol glycosides, iridoids, alkaloids, ionones, and others. Gao et al.136 explored key biomarkers for quality control of Lianhua Qingwen capsules, a TCM formulation used to treat H1N1 influenza virus infections, through a bioassay-based approach. This study emphasizes the integration of AI with traditional medicine to ensure quality and efficacy. Lu et al.134 developed a method for analysing chemical third-order data (such as fluorescence excitation–emission time data), enhancing the analysis of complex samples in TCM research. The method was applied to fluorescence excitation–emission time data to analyse epinephrine and norepinephrine in urine samples and data fusion of excitation–emission matrices, NMR and liquid chromatography to determine the mass spectra of five target components.
The primary challenges in using AI for TCM chemical component analysis include the quality of TCM data and the interpretability of AI models. Techniques such as transfer learning and novel data representation methods are being explored to address these issues. Interdisciplinary collaboration among AI researchers, chemists, and TCM practitioners is crucial to fully realizing AI's potential in TCM research. This collaborative approach can help tackle the complexities of TCM and fully leverage AI's potential for innovative discoveries. The prospects for AI in TCM chemical component analysis are broad, with potential advancements including the development of more complex AI models to improve prediction accuracy, the creation of comprehensive TCM databases for AI training, and the integration of AI into personalized medical approaches.
7. Applications of AI in herbal medicine
7.1 Analysis of herbal chemical components
AI has become a key technology in enhancing the research and application of herbal medicine, particularly in chemical component analysis, pharmacological research, and new drug discovery. AI's ability to handle complex datasets, recognize patterns, and conduct predictive analyses surpasses the limitations of traditional methods. This advanced technology, combined with the knowledge of TCM, not only unleashes the therapeutic potential of herbal treatments but also significantly enhances our understanding and utilization of these natural resources for more effective therapeutic purposes.
The complexity of herbal medicine, involving multiple components with intricate interactions affecting the human body, makes AI an essential tool. The complexity highlights the necessity of integrating AI with traditional herbal knowledge, as herbs typically involve mixtures with complex interactions and effects on the human body.144Fig. 8A illustrates the overall process of AI-based analysis of TCM herbal medicine. AI is essential for the identification and classification of herbal chemical components. In particular, machine learning techniques are highly effective in analyzing and interpreting the complex chemical properties of herbs. These technologies enable detailed analysis of herbal components, deepening understanding of their potential therapeutic effects, and aiding in the standardization and quality control of herbal products.145 Additionally, the application of AI effectively addresses the challenges of managing the vast and diverse data involved in herbal medicine research, showing unique strengths in addressing complex chemical issues in herbs.146
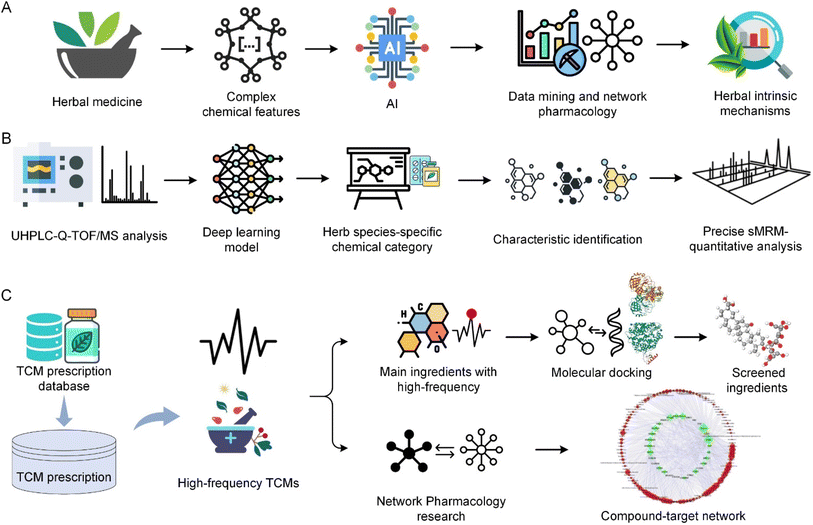 |
| Fig. 8 AI analysis and application cases of TCM herbal ingredients. (A) AI-based TCM herbal medicine analysis process. AI starts from the initial herbal input to complex chemical profile analysis and finally the intrinsic mechanism of the herbal medicine through AI and data mining. (B) Case study of Qiang Huoshengshi decoction – intelligent chemical analysis strategy guides the accurate quantification of multiple components of TCM compound. Apply UHPLC-Q-TOF/MS analysis to detailed steps for identifying herbal species-specific chemical classes using deep learning models, enabling precise chemical analysis quantitation. (C) Case analysis of using a data-driven approach to identify potential treatments for COVID-19 from TCM. Through molecular docking and network pharmacology research, the utilization process of TCM prescription database to high-frequency TCM and main drugs is demonstrated. | |
AI's integration extends beyond the chemical analysis of herbs; it also impacts the classification and recognition of herbs, bringing a new dimension to the field of herbal medicine. By adopting systematic, precise, and data-driven approaches, AI significantly improves the accuracy and effectiveness of diagnostics and treatments. Advances in AI, especially in classifying based on the physical and chemical properties of herbs, play a crucial role in revealing deeper insights into the therapeutic potentials of herbs.147 Furthermore, AI technologies such as machine learning and deep learning enable researchers to identify complex data patterns that might not be immediately apparent to human researchers. These technologies play a key role in understanding complex herbal combinations, and through data mining and network pharmacology approaches, AI-driven analyses help reveal the intrinsic mechanisms of herbal combinations, showing how different herbs interact and collectively contribute to therapeutic effects.148,149 Additionally, AI-assisted network pharmacology provides a comprehensive framework for understanding the multi-target and multi-pathway actions of herbal formulations, particularly important in treating complex diseases involving multiple physiological systems.49,150
7.2 Case studies and challenges
Guo et al.151 leveraged advanced deep learning and UHPLC-Q-TOF/MS methodologies to refine the chemical profiling and quantification of the Qiang Huosheng Stone Soup (QHSS). Their process, illustrated in Fig. 8B, began with the development of a deep-learning model tailored for mass defect-based classification of QHSS compounds. This model facilitated the herb-specific categorization of coumarins and chromones, crucial for distinguishing compounds with similar structures. Subsequently, characteristic ions and neutral losses were analysed to identify multiple phytochemicals, enhancing the precision of the herbal analysis. The chemical fingerprints derived from these steps enabled the accurate, multi-target quantitative analysis using a sensitive UHPLC-sMRM technique. This comprehensive strategy significantly advanced the quality assessment of QHSS, proving essential for ensuring the efficacy and safety of this traditional formula. Lee et al.152 utilized systems pharmacology to understand the pharmacological actions of herbal treatments, employing AI to explore the gene expression profiles induced by these treatments. This approach provided a comprehensive view of the molecular mechanisms of action of Chinese medicine, showcasing AI's capability to interpret complex biological data. By identifying potential interaction networks of drugs, this method enhanced understanding of the multi-target efficacy of herbal medicine in treating complex diseases. Zhang et al.153 developed a novel computational pipeline to assist in de novo identification of protein targets of herbal components. This method, combining pharmacophore comparison and reverse ligand–protein docking simulations, effectively identified known targets of Chinese medicine components, facilitating the discovery of new molecular structures and elucidating the mechanisms of action, significantly aiding drug discovery from natural products. Tang et al.154 first developed a network-based approach to quantify the interactions among herbal combinations in TCM. By constructing a protein–protein interaction network, it analyzes 349
197 pairs of herbs, revealing that commonly used herb pairs have shorter network distances, indicating a higher likelihood of influencing adjacent proteins. The research highlights the significance of central components in herbal interactions, providing a theoretical foundation for the modernization of herbal combinations. Zuo et al.155 developed a robust integrated strategy based on LC-MS systems for the comprehensive characterization and quantification of multiple components in herbal medicine. Using Gouji as an example, 147 components were identified, demonstrating the power of AI in enhancing the understanding of herbal components. Yang et al.146 focused on Gouji extract, using an integrated analysis strategy independent of real standards to explore the pharmacokinetics of herbal medicine in vivo. It demonstrated the capability to evaluate herbal pharmacokinetics through novel analytical methods, providing insights into the bioactive components of Gouji. Herbal omics is an approach that combines metabolomics with herbal medicine, using AI to assess the efficacy of herbal treatments, providing a holistic view of their impacts on biological systems.156 Wang et al.157 quantified interactions within herbal components by constructing protein–protein interaction networks, aiding in more effectively exploring herbal combinations. This method utilized network topology to identify synergistic compound interactions, greatly aiding in understanding the complex relationships within Chinese medicine formulations. Yi et al.158 used hyperspectral imaging and machine learning algorithms including support vector machine, extreme learning machine, CNN, and recurrent neural network to identify counterfeit herbs and determine the content of bioactive components. This approach addressed issues of quality control in Chinese medicine by making predictions for large sample analyses more reliable. Guo et al.159 focused on Clematis, developing an integrated platform that uses metabolomics and chemometrics to characterize and predict Chinese medicinal materials with multiple plant sources. This approach employed genetic algorithms and support vector machines for accurate species prediction, enhancing the traceability and quality control of Chinese medicine products.
Herbal medicine played a significant role in COVID-19. Ren et al.160 developed a robust screening system to identify potential TCM-based treatments for COVID-19, as depicted in Fig. 8C. Their approach utilized data mining to extract prevalent medicinal materials and prescriptions from ancient texts. This was followed by molecular docking studies to assess the interactions between the main active ingredients of frequently used TCMs and the vital targets of the SARS-COV-2 virus. The integration of network pharmacology allowed for the exploration of these interactions at the molecular level, uncovering potential mechanisms of action against the virus. This methodology not only highlighted key compounds in common remedies like licorice and skullcap but also facilitated a deeper understanding of their potential as natural therapeutic agents against COVID-19, underscoring the capability of AI-enhanced network pharmacology in advancing TCM research and application. Zhang et al.161 evaluated the adjunctive therapeutic efficacy of Natural Herbal Medicine (NHM) combined with Western medicine in treating COVID-19. The results indicated that NHM in combination with standard Western medicine significantly shortened the duration of fever and improved other clinical symptoms, demonstrating the effectiveness of NHM in treating COVID-19 patients. Shi et al.162 conducted a systematic review and meta-analysis to assess the impact of oral traditional medicine on immunogenicity during COVID-19. The study found that traditional medicine as an adjunctive drug could improve immune responses and shorten the time for clinical symptom relief, highlighting the potential of traditional medicine as an adjunctive immunotherapy for disease mitigation and symptom relief. Ang et al.163 conducted a systematic review and meta-analysis to evaluate the efficacy and safety of herbal medicine in treating COVID-19. The study indicated that adding herbal medicine to standard treatment produced beneficial effects, suggesting potential benefits in treating COVID-19 symptoms.
Despite these advancements, challenges such as data heterogeneity, the complexity of herbal formulations, and the need for clinical trials to validate AI predictions remain. Future research should focus on enhancing AI models to improve accuracy, integrating different data sources for comprehensive analysis, and conducting rigorous clinical validations to ensure the safety and efficacy of AI-predicted herbal treatments. The application of AI in herbal research not only simplifies the discovery and analysis of herbal components but also opens new avenues for understanding complex mechanisms and potential synergistic actions within herbal formulations. As AI technology continues to evolve, its integration with herbal research is expected to usher in an innovative era, offering profound insights into ancient practices through the lens of modern science.
8. Applications of AI in drug discovery in TCM
8.1 AI analysis and screening of TCM chemical components
AI plays a transformative role in the field of drug discovery, particularly accelerating the research of TCM chemical components and the development of new drugs. The potential and value of combining TCM databases and chemical analysis with AI in drug discovery processes are immense. Fig. 9A shows the general process of AI-based TCM drug discovery. AI technologies can quickly identify novel compounds and elucidate their mechanisms, offering a promising avenue for innovative drug development.164,165 AI utilizes deep learning to process complex chemical data, facilitating the rapid identification and classification of chemical components in TCM. The application of AI in high-throughput screening processes enhances the efficacy of identifying chemical components with potential therapeutic effects. This method enables rapid screening of large compound libraries against various targets, significantly accelerating the discovery phase of drug development.166
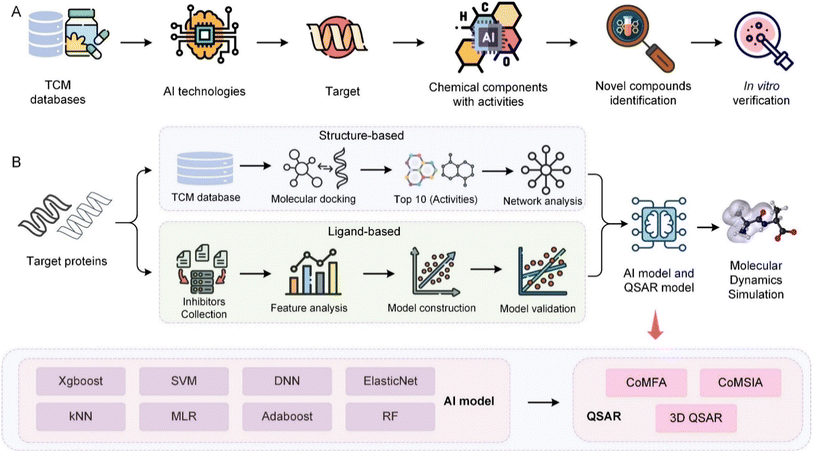 |
| Fig. 9 AI analysis of TCM drug discovery and its application cases. (A) AI-based TCM drug discovery process. Apply AI technology to perform virtual screening from Chinese medicine databases to target and identify active chemical ingredients, followed by new compound identification and in vitro validation. (B) Case study of effective clues to gain insights into Alzheimer's disease through the use of multiple AI algorithms. Screening is carried out using structure-based and ligand-based methods, then AI and QSAR models are developed to continue screening, and finally validated through molecular dynamics simulations. | |
AI aids in predicting potential targets of TCM chemical components, using machine learning models to analyse and forecast interactions between molecules and biological targets. This predictive capability is crucial for identifying new therapeutic pathways and understanding the pharmacodynamics of TCM compounds.167 AI also plays a role in the validation of identified targets, using bioinformatics and data analysis to support experimental validation efforts. This ensures that predicted targets are biologically relevant and can lead to effective therapeutic interventions.168 AI technologies are fundamentally changing the field of drug discovery, especially within the realm of TCM. By leveraging TCM databases and advanced chemical analysis methods, AI can rapidly identify, classify, and validate bioactive compounds and their targets.
8.2 Pharmacological mechanisms and pathways
AI plays a key role in revealing the action mechanisms and pharmacological pathways of TCM chemical components. Particularly, network pharmacology, which uses AI to integrate multi-source data, provides a holistic view of how TCM compounds interact within biological systems. This approach aids in understanding complex interactions, offering insights into the synergistic effects of TCM components and their therapeutic potential.169 AI assists the drug design process, using computational chemistry and molecular modelling to optimize drug structures for enhanced efficacy. By predicting how molecules interact with biological targets, AI can fine-tune drug properties, developing more effective and safer therapeutic drugs.170 Utilizing AI to integrate data from various TCM databases, including chemical, pharmacological, and clinical data, significantly enhances the drug discovery process. AI algorithms can analyse and synthesize data from diverse sources, identifying new candidate drugs and clarifying their potential therapeutic applications.171 AI's role in merging herbal chemical, genetic, and pharmacological data is crucial for advancing drug discovery. By analysing multidimensional data, AI aids in a comprehensive understanding of herbs, improving the accuracy and efficiency of identifying new therapeutic compounds.140 The integration of AI in the drug discovery process, especially under a TCM context, represents a paradigm shift in pharmaceutical research. Utilizing TCM databases and chemical analyses, AI helps to gain deeper insights into complex herbal compounds, simplifying the drug design process, and paving new pathways for the discovery of novel therapeutic drugs.
8.3 Applications in predicting TCM drug toxicity and side effects
AI plays a crucial role in predicting the toxicity and side effects of TCM components, which is essential for ensuring safety in drug development. AI models, including machine learning algorithms and deep learning architectures, analyze vast datasets to predict the toxic effects and adverse reactions of TCM compounds, significantly reducing the risk of late-stage failures in drug development. Recent advancements in AI-based drug toxicity prediction have improved the accuracy and efficiency of identifying potentially toxic effects of new compounds before they reach clinical trials, thereby saving time and resources.172 AI methods have also been developed to predict severe toxicities, such as drug-induced liver injury (DILI), by integrating gene expression data and other biological information.173 Moreover, AI models employing deep learning techniques have demonstrated superior performance in predicting adverse drug reactions (ADRs), further enhancing the safety assessment process in TCM drug discovery.174 These models can predict a wide range of side effects by analyzing drug chemical structures and integrating diverse datasets.175 In summary, the integration of AI in TCM drug discovery not only accelerates the identification of bioactive compounds but also enhances safety assessments by predicting potential toxicities and side effects early in the drug development process. This approach ensures that TCM compounds are both effective and safe for therapeutic use.
8.4 Case studies and challenges
He et al.176 utilized a series of machine learning and deep learning models to screen and analyse the bioactivity of potential compounds derived from TCM for treating Alzheimer's Disease (AD). Employing molecular docking techniques, the study pinpointed active compounds targeting GSNOR, C3b, Factor D, and PERK proteins. This approach, as shown in Fig. 9B, integrates AI-driven predictions with ligand-based feature analysis to refine the selection of multi-target inhibitors. The use of 3D-QSAR models facilitated the examination of molecular interactions, enhancing the understanding of potential inhibition mechanisms. Furthermore, molecular dynamics (MD) simulations confirmed the stability of these ligand–target complexes throughout the simulation period, with particular attention to the cleft closure mechanism observed in the Nodifloridin A-PERK complex. This comprehensive use of AI and molecular modelling tools not only validated the potential therapeutic effects of the identified compounds but also underscored the effectiveness of integrating AI in the traditional drug discovery process, showcasing a novel therapeutic approach for AD using TCM ingredients. Li et al.177 combined network pharmacology, machine learning, deep learning and molecular dynamics simulation to study long-lived and effective lead drugs, and successfully screened GS-3, conanine, and isofebrigine as candidate compounds. Antipyretic bicolor grass, antidysentery rhubarb grass, and Gelsemium eternalis containing these compounds may be effective TCMs targeting two targets. Gong et al.178 used machine learning regression models of regression algorithms such as support vector machines, bagging, and random forests, as well as deep neural network models to predict candidate compounds targeting dipeptidyl peptidase 4 protein. The 2007_4105 compound obtained from the final screening was finally verified by molecular dynamics simulation. The fine fruity aroma of this compound as its main component may be a potential and effective herbal medicine for the treatment of diabetes. Feng et al.179 developed a new strategy using ultra-high-performance liquid chromatography tandem mass spectrometry to discover pharmacologically active components in complex biological samples. By effectively identifying metabolites, this method enhanced understanding of the mechanisms of action of TCM, showcasing the potential of AI in elucidating the pharmacodynamics of herbal medicine. Hassanzadeh et al.170 highlighted the key role of AI in analysing biological or genetic information, advancing drug discovery, and predicting the behaviour of small molecule modulators or rare molecules. They noted significant improvements in therapeutic outcomes brought by AI, especially through reverse target identification and predictive modelling, emphasizing AI's expanding role in TCM and modern pharmacology.
The application of AI in new drug discovery in TCM faces challenges related to data quality, model interpretability, and the complexity of interdisciplinary collaboration. The development of new algorithms and technological innovations, along with innovative interdisciplinary research approaches, provides significant opportunities for AI in the TCM discovery field. AI has the potential to modernize and internationalize TCM, enhancing its global acceptance and application. This transformative impact could revolutionize traditional medicine, providing more effective and personalized medical solutions. The application of AI in the development of new drugs in TCM exemplifies the synergy between traditional knowledge and modern technology, offering a hopeful path for the development of new therapeutic drugs. Despite some challenges, AI's potential to fundamentally change TCM and contribute to the global pharmaceutical landscape remains vast.
9. AI in the chemical analysis of drug incompatibilities in TCM
9.1 AI-based prediction of TCM drug incompatibilities
In TCM, the concept of drug–drug interactions (DDI) is crucial for ensuring patient safety in clinical practice. Given the complexity and widespread use of herbal compounds, understanding and predicting potential DDIs is essential to mitigate adverse effects.180,181 Drug interactions in TCM, particularly given the holistic approach often involving combinations of multiple herbs, are a critical issue. The concept of drug incompatibility highlights potential adverse reactions from specific herbal combinations, emphasizing the need for careful selection and combination of TCM ingredients to avoid negative interactions.182Fig. 10A shows the general workflow for analysing drug incompatibilities in TCM using AI. The inherent complexity of multi-herbal combinations in TCM, often involving the concept of incompatibility underscores the urgent need to carefully select and combine herbal ingredients to prevent adverse interactions.50 Due to the multifaceted nature of TCM formulations, traditional methods face significant challenges in accurately predicting DDIs. These formulations typically involve numerous bioactive compounds with potential synergistic or antagonistic effects, complicating the understanding of their interactions at the molecular level.183 The use of modern tools such as systems pharmacology provides a more systematic approach to elucidate bioactive compounds, predict their targets, and clarify the molecular mechanisms of action, which is crucial for understanding interactions within TCM.167 These modern integrative approaches, leveraging computational models capable of handling large datasets and complex interaction networks, address the limitations of traditional methods, offering a promising path for more accurate DDI prediction and safer clinical application of TCM.184
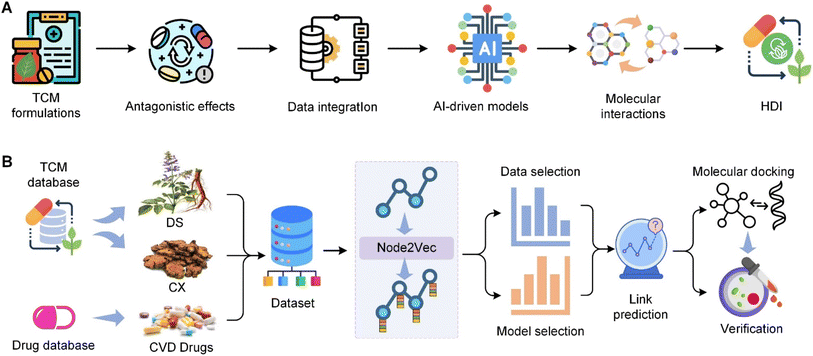 |
| Fig. 10 Application of AI in analysing HDIs. (A) General workflow for analysing drug incompatibilities in TCM using AI. It illustrates the steps from TCM formulations to the prediction of herb–drug interactions (HDI) through AI-driven models, emphasizing the identification of antagonistic effects and the integration of diverse data. (B) Case study of AI application in HDI analysis. It outlines the process from data collection to molecular docking and verification, focusing on the application of graph embedding techniques like node2vec to predict new potential targets within HDIs. | |
AI, especially machine learning and deep learning, is revolutionizing the field of chemical data analysis, making significant advances in predicting drug interactions (DDI) and optimizing drug safety. AI's role in enhancing the accuracy of predicting complex interactions within drug combinations—including drug–drug, drug–food, and drug–microbiome interactions. Advanced AI algorithms utilize large datasets to predict potential interactions and outcomes, refining drug compatibility and safety profiles more accurately than traditional methods.185 These AI-driven models are particularly beneficial in TCM, where formulations typically include multiple herbs that may interact in unpredictable ways. By integrating data on chemical properties, genetic information, and clinical data, AI models provide a robust framework to predict DDIs, enhance understanding of molecular interactions, and improve the overall safety of drug prescriptions.186,187 Additionally, AI applications in TCM not only predict interactions but also help design safer, more effective herbal formulations, potentially reducing adverse reactions and enhancing therapeutic outcomes.188
9.2 Prediction of interactions between Chinese and Western medicines
Advancements in herbal–drug interaction (HDI) models and the development of comprehensive databases are crucial for improving the safety and efficacy of integrated Chinese and Western medicine. TCM formulations often contain multiple herbs, whose complexity and interactions with synthetic drugs pose significant challenges. The use of AI and the compilation of extensive databases help address these challenges. AI models tailored for HDI use machine learning algorithms to predict potential interactions between herbal and pharmaceutical compounds. These models analyse historical data, chemical properties, and biological pathways to predict interactions that could lead to adverse reactions or therapeutic inefficacy.189 The PHYDGI database integrates HDI's scientific data in a well-structured form, including citations to scientific sources. It provides the strength level of herbal entities and their interactions as well as pharmacokinetic interactions. This database can be used by health practitioners, botanical medicine and food supplement manufacturers and should help reduce potential HDI and develop safe use of botanical therapies or supplements.190 Martins et al.191 designed an original hybrid decision support system to identify herb–drug interactions, applying AI technology to identify new possible interactions. The system enables better and more accurate treatment decisions and mitigates possible adverse events. The ongoing development of HDI models and databases is essential for safely integrating TCM into the global healthcare system. As AI technologies advance, these tools will become increasingly sophisticated, providing deeper insights and more reliable predictions, thereby enhancing patient safety and optimizing therapeutic outcomes. The challenge remains to ensure that these models and databases keep pace with the rapid development of herbal and pharmaceutical therapies, maintaining an up-to-date and accurate repository of information.
9.3 Case studies and challenges
Integrating AI into the analysis of drug antagonisms in TCM provides significant insights into complex pharmacological interactions. A key breakthrough in the field is the application of AI models to predict potential adverse drug–drug interactions (DDI), making substantial contributions to patient safety and treatment efficacy. Zhang et al.192 utilized node2vec graph embedding technology to expand potential targets of herbal chemicals in the context of HDIs. As shown in Fig. 10B, taking Salvia miltiorrhiza (Danshen, DS) and Ligusticum chuanxiong (Chuanxiong, CX) and related approved drugs for cardiovascular diseases (CVD) as examples, the results were verified through molecular docking and pharmacological experiments. Their methodology transformed target identification into a link prediction problem, utilizing node2vec along with other algorithms like Adamic–Adar and Jaccard similarity coefficient to enhance the predictive accuracy of chemical-target associations. This approach was rigorously validated through tenfold cross-validation, achieving robust AP and AUROC scores. This study effectively expanded the interaction edges by incorporating node2vec into an existing dataset, revealing new targets for 32 herbal chemicals, particularly volatile oils and diterpenoids at low levels. The findings not only demonstrate the efficacy of node2vec in scaling HDI networks, but also provide a comprehensive methodological framework for predicting and understanding complex interactions between herbs and drugs. This can serve as an important tool for clinical risk management and elucidation of the mechanisms of action of traditional drugs. Zhu et al.193 proposed a novel supervised learning framework with attribute regularization for prediction of patient absorption of incompatible drug pairs. In this framework, they model the prediction task as a nonnegative matrix three-factorization problem, where two important herbal properties (potency and flavor) and their correlations are combined to characterize the incompatible relationships between herbs. This model is crucial for identifying potential hazardous combinations before clinical application, essential for preventing adverse reactions. Zhang et al.194 evaluated the inhibitory potency of Jingyin granules (HEJG) on human drug metabolizing enzymes and elucidated whether HEJG can modulate the pharmacokinetic characteristics of Western medicines in vivo. HEJG regulates the pharmacokinetics of CYP substrate drugs by inactivating CYP3A, providing critical information for clinicians and patients to use herbal–drug combinations for antiviral therapy in a scientifically sound manner. Ge et al.195 explained the DDI and potential incompatibility mechanisms between Aconitum carmichaelii Debx (Chuanwu, CW) and Pinellia ternata (Thunb.) Breit (Banxia, BX) by using pharmacokinetic and cocktail-based methods. The results showed that BX not only enhanced the absorption of aconitine and benzoyl aconitine, but also accelerated the metabolism of neoconitine, benzoyl neoconitine, songolin and fuzilin.
Despite these advancements, integrating AI into TCM DDI research still faces significant challenges. The heterogeneity of TCM data, the variability of herbal components, and the non-standardized formulations of herbal compounds complicate the training of robust and universal AI models. Additionally, ethical issues related to data privacy, informed consent, and the transparency of AI algorithms need to be addressed to gain broader acceptance. The future of AI in TCM DDI research lies in further integrating omics data, enhancing the interpretability of AI models, and developing real-time DDI prediction tools that can seamlessly integrate into clinical workflows. Advances in AI can lead to more personalized, safe, and effective TCM treatments, broadening the scope of integrated Chinese and Western medicine.196
9.4 Proposal of integrated clinical study of HDIs
In the field of TCM, understanding the interactions between herbal medicines and conventional drugs is crucial for ensuring patient safety and treatment efficacy. This article integrates TCM into clinical research and proposes an TCM-based automated clinical research proposal. This proposal outlines an integrated clinical study system designed to explore these interactions comprehensively. As shown in Fig. 11, this system is structured into three main modules: clinical study system, integrated system, and fundamental study, each focusing on different aspects of chemical analysis and data integration to predict and analyze drug incompatibilities.
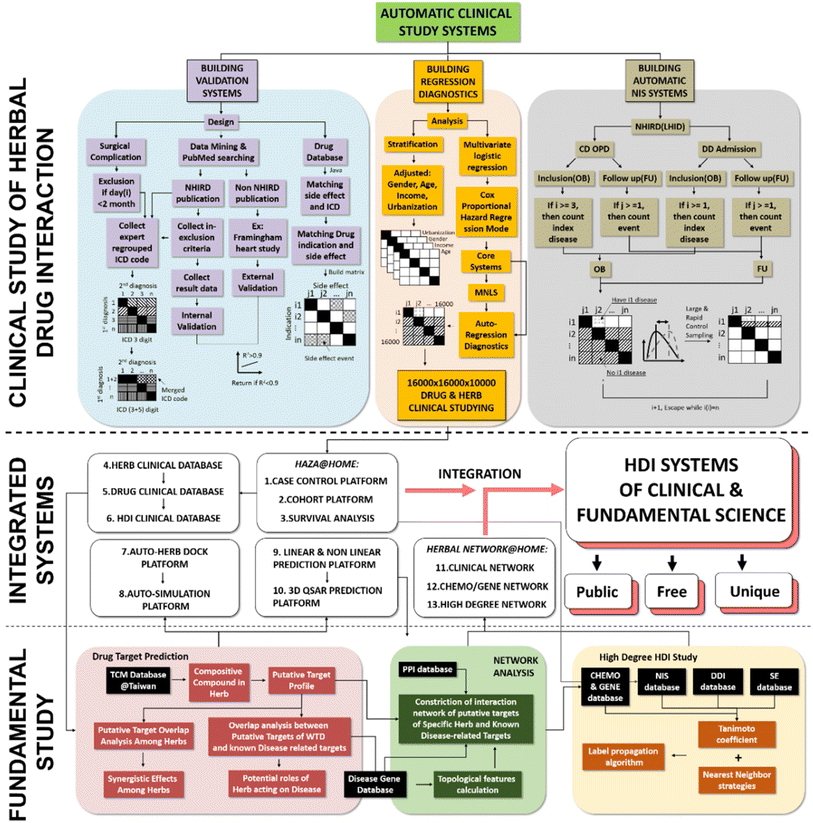 |
| Fig. 11 A comprehensive proposal for automatic clinical study system. The framework is divided into three main modules: clinical study system, integrated system and fundamental study system. The clinical study system module includes stages such as surgical sample extraction, chemical analysis, and data mining. This workflow aims to build a validation system to rigorously test and confirm observed interactions between herbal medicines and pharmacological substances. The integrated system serves as a link between clinical and basic science data, facilitating the integration of disparate data sets through platforms such as HAZA@home. It supports comprehensive analysis at home, allowing users to perform preliminary disease assessments using integrated network pharmacology tools. The fundamental study system focuses on the theory and mechanism of drug interactions. The module employs a variety of computational and experimental methods, including target prediction, ADMET simulations, and construction of molecular databases. Aims to elucidate the molecular basis of drug interactions through in-depth chemical analysis and simulation modeling. | |
The clinical study system primarily handles the collection and analysis of clinical data to assess the pharmacokinetic and pharmacodynamic properties of herbal and pharmaceutical drug combinations. Utilizing advanced chemical extraction methods and analytical techniques, this module aims to identify potential adverse interactions through rigorous clinical testing and chemical analysis. Data mining and machine learning algorithms are employed to process complex clinical data, identifying patterns that predict the outcomes of drug interactions.
Central to the integrated approach is the integrated system, which features the HAZA@home platform. This innovative system combines data from clinical studies and fundamental research to provide real-time health monitoring and predictive analyses directly to patients at home. It uses an extensive database network and simulation tools to personalize health assessments and drug interaction warnings, leveraging AI to adapt recommendations based on individual patient data.
The fundamental study system module supports the integrated system by focusing on the scientific basis behind drug interactions. It involves in-depth chemical analysis and computational modeling to predict drug targets and simulate drug interactions. This includes using TCM and clinical drug databases, ADMET prediction models, and the creation of small molecule databases. These tools are instrumental in understanding the biochemical mechanisms of drug interactions, which is vital for developing preventive strategies against potential adverse effects.
This proposal exemplifies the integration of clinical and fundamental scientific approaches in the study of herbal drug interactions within TCM. By employing a combination of chemical analysis, real-time data monitoring, and AI-driven predictive modeling, the system not only enhances the understanding of complex drug interactions but also pioneers new methodologies for patient safety in the integration of herbal and pharmaceutical treatments. This approach sets a benchmark for future studies and applications in the realm of AI-enhanced pharmacology, particularly within the unique context of TCM.
10. Applications of AI-assisted TCM diagnosis and treatment
10.1 TCM diagnostic data analysis
The integration of AI with TCM diagnostics is transforming the landscape of medical diagnostics, enhancing the interpretation of chemical components and their correlation with symptoms, improving symptom analysis and disease prediction, and significantly increasing diagnostic efficiency and accuracy. This synergistic action enables a deeper understanding of the complex patterns of TCM and the relationships between chemical components and specific diseases, thus providing a more refined and precise diagnostic process.35
Advances in TCM research highlight the significant role of chemical components in diagnosing specific diseases. Understanding these relationships lays the foundation for TCM diagnostics, helping to identify disease biomarkers and therapeutic targets.140 The complexity of analysing chemical data in TCM diagnostics presents several challenges. AI technology helps overcome these challenges by using advanced algorithms to process and interpret complex datasets, making diagnostics more efficient and accurate.197Fig. 12A shows the general process of AI-based TCM diagnostic analysis. Using machine learning algorithms to identify disease characteristics and biomarkers demonstrates its utility in diagnosing diseases. This approach can identify subtle patterns that traditional analysis methods may not reveal.198 AI predicts disease progression by analysing chemical data, including the application of deep learning technologies for disease prediction and risk assessment, highlighting its potential in proactive healthcare measures. These models greatly assist in early detection and intervention strategies, customizing treatment plans based on individual patient conditions.199 The combination of AI with TCM diagnostics represents a significant advancement in medical diagnostics, offering a more detailed and comprehensive approach to disease detection and understanding. By leveraging large datasets in TCM databases and employing AI for chemical data analysis, the medical community can enhance diagnostic processes and improve patient treatment outcomes.
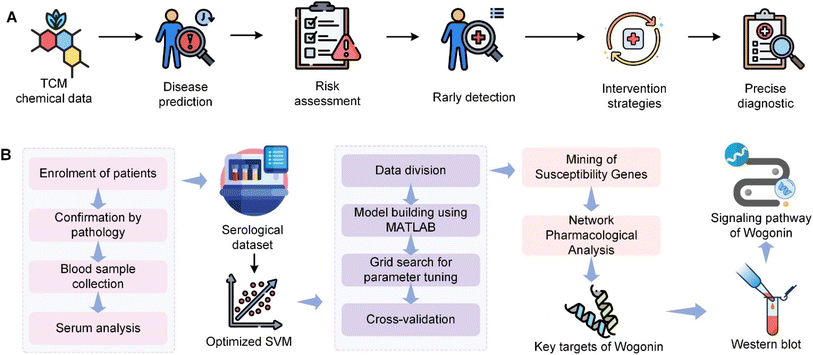 |
| Fig. 12 Application of AI in TCM diagnosis. (A) General workflow for AI applications in TCM diagnosis. It illustrates the process of utilizing AI to enhance TCM diagnosis through disease prediction, risk assessment, early detection, intervention strategies, and precise diagnostics, leveraging chemical data from TCM sources. (B) Case study on lung cancer diagnosis and therapeutic insights. It shows the integration of an optimized SVM for serological data analysis, network pharmacology, and experimental techniques to uncover the mechanisms of wogonin in lung cancer. | |
10.2 AI-based personalized TCM diagnostics
The combination of AI with chemical data analysis provides a breakthrough method for delivering personalized TCM diagnostics and treatment recommendations. By analysing patients' unique chemical signatures and health data, AI algorithms can tailor treatment strategies that better match individual health needs and conditions. This personalized approach not only improves treatment efficacy but also minimizes potential side effects, thereby enhancing patient treatment outcomes.197 AI's role in analysing patient historical data, lifestyle habits, and chemical sensitivities is crucial for devising more precise treatment plans. By utilizing machine learning algorithms to sift through large amounts of data, AI can identify patterns and correlations that may not be immediately apparent, allowing for highly customized treatment plans that consider the unique health conditions and predispositions of patients.200 Using AI to integrate data from various TCM databases, including chemical, clinical, and pharmacological data, significantly enhances the comprehensiveness and accuracy of diagnostics. This holistic data analysis approach ensures that all relevant aspects of patient health are considered, resulting in more informed and effective diagnostic outcomes. The integration process involves advanced data mining techniques and AI algorithms capable of handling the complexity and diversity of TCM data.201 Multiple AI-driven TCM diagnostic tools and platforms have been developed to assist healthcare professionals in making more accurate disease diagnoses and treatment plans. These tools use AI's capability to analyse and interpret complex data, providing valuable insights that can guide physicians' decision-making processes. The emergence of these tools represents a significant advancement in the field of TCM, providing a more objective and effective approach to diagnosis and treatment based on comprehensive analysis of patient health data.80 The integration of AI with TCM diagnostics heralds a new era of personalized medicine, driven by the comprehensive analysis of chemical data and patient information, tailoring treatment plans to individuals' unique health conditions. This method not only increases the accuracy of TCM diagnostics but also paves the way for more effective, patient-centered treatments. By harnessing the power of AI and the rich data contained in TCM databases, healthcare providers can gain deeper insights into diseases and their treatments, ultimately leading to better patient care and outcomes.
10.3 Case studies and challenges
Wang et al.202 employed an optimized SVM to develop a serology-based diagnostic model for lung cancer (Fig. 12B). They further explored the therapeutic impacts of wogonin, derived from the herb Scutellaria baicalensis, known for its potential anticancer properties. The research integrated serological data analysis using SVM, network pharmacology to identify and analyse key targets of wogonin, and Western blot techniques to confirm these targets. This multi-disciplinary approach enabled the construction of a molecular regulation model showcasing how wogonin interacts within lung cancer pathways. Key findings from the study demonstrated the ability of wogonin to modulate significant pathways such as PI3K-Akt, contributing to its efficacy in inducing apoptosis in lung cancer cells. The study underscores the effective use of AI and machine learning in enhancing the precision of lung cancer diagnostics and deepening the understanding of herbal medicine's role in treatment, presenting a novel integration of technology and traditional medicine for tackling complex diseases like lung cancer. Lin et al.121 used neural network analysis to recommend clinical prescriptions through big data resource analysis. The applied DeepMedic software identified medicinal materials with the effect of replenishing qi and removing dampness as recommended prescriptions, which accurately corresponded to the TCM prescriptions used to treat colorectal cancer in the clinic. This method can be used to provide accurate TCM diagnosis and suggest prescriptions for the treatment of colorectal cancer. To analyse the clinical and case characteristics of herb-induced liver injury, Teschke et al.203 conducted a selective literature search in the PubMed database. It was found that HILI caused by TCM is rare and, similar to drugs, can be caused by unpredictable specific reactions or predictable intrinsic reactions. The clinical characteristics of liver injury caused by TCM products vary, and specific diagnostic biomarkers such as microsomal epoxide hydrolase, pyroprotein adducts, metabolomics, and microRNA are available for only a few TCM products. Ji et al.204 developed a model based on latent Dirichlet allocation to analyse symptoms and corresponding herbs, revealing TCM pathogenesis related to TCM theory. This approach supports more accurate diagnosis and treatment planning by revealing hidden relationships in clinical data. Jafari et al.205 integrated unsupervised learning and network models to stratify TCM patients by clustering herbal ingredients based on chemical properties. This approach provides insights into the effectiveness of herbal treatments and supports the development of personalized medicine strategies in TCM.
The main challenges in applying AI to TCM diagnostics include ensuring data privacy protection and addressing issues of model interpretability. Developing models that practitioners can transparently understand and trust is crucial. The future of AI in TCM diagnostics is promising, with opportunities to improve diagnostic accuracy, develop personalized treatment approaches, and promote the internationalization of TCM. New algorithm developments and innovative interdisciplinary research methods are essential. Collaboration between AI technologies, TCM knowledge, and chemical data analysis is crucial for driving innovation and development in TCM diagnostics. Through collaborative efforts, significant progress can be made in creating more effective, efficient, and personalized diagnostic and treatment methods. The integration of AI with TCM diagnostics represents a significant advancement in personalized medicine, offering the potential to transform traditional practices with modern technology.
11. Applications of AI in personalized treatment planning
11.1 Chemical data in personalized medicine
The concept of personalized medicine represents a paradigm shift in healthcare, moving from a one-size-fits-all approach to customizing medical care based on an individual's unique genetic makeup, lifestyle, and environmental factors. This approach is particularly significant in the field of TCM, where treatment is inherently personalized. Fig. 13A reveals the general process for AI applications in personalized treatment planning. The role of AI in analysing chemical data and utilizing TCM databases is crucial for developing personalized treatment plans and achieving more precise and effective medical interventions.206 The analysis of chemical components in TCM plays a vital role in personalized treatment, where AI models predict treatment outcomes based on chemical composition. Considering patients' unique physiological responses allows for the identification of compounds most effective for individual patients.207 Patient-specific data, including genetic information, medical history, and lifestyle choices, are integral in crafting personalized treatment plans. AI algorithms analyse this data to formulate treatment strategies that align with patients' unique health conditions, thereby enhancing the efficacy of TCM interventions. AI technology identifies patterns in TCM chemical data, aiding in disease characterization and biomarker identification. This capability is crucial for diagnosing complex conditions that traditional methods may fail to address.198 AI's predictive modelling capabilities, by analysing chemical and patient-specific data, predict disease progression and treatment outcomes. This approach greatly contributes to proactive and personalized healthcare management.208 AI combines chemical data analysis with patient-specific information, providing tailored TCM diagnostic and treatment recommendations. This process involves a thorough analysis of genetic and clinical data to determine the best treatment paths specifically designed for individual health needs.197 AI-enhanced analysis of patients' historical data, lifestyle habits, and chemical sensitivities leads to precise, personalized treatment plans. AI's ability to handle complex datasets and identify key health indicators facilitates this personalized approach.200 The integration of AI with TCM personalized treatment plans represents a significant advance towards more effective, efficient, and patient-centered healthcare. By utilizing AI for chemical data analysis and integrating patient-specific information, healthcare providers can devise truly personalized treatment plans, thereby enhancing the potential for successful outcomes and patient satisfaction.
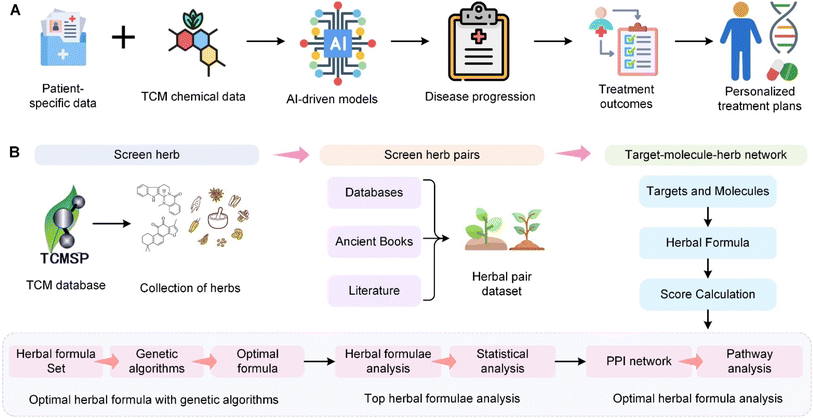 |
| Fig. 13 AI-enhanced personalized TCM treatment planning. (A) General process for AI applications in personalized treatment planning. This workflow illustrates how AI integrates patient-specific and TCM chemical data to model disease progression and predict treatment outcomes, ultimately guiding the development of personalized treatment plans. (B) Case study on optimizing herbal formulas using AI for personalized treatment planning. It depicts the use of genetic algorithms to analyse and derive optimal herbal formulas from TCM datasets, validating their effectiveness and functional impact through systematic analysis. | |
11.2 Personalized TCM treatment tools
AI plays a key role in customizing treatment plans based on specific patient conditions and chemical data. It aids in selecting appropriate medications and adjusting dosages to optimize treatment outcomes. By analysing vast amounts of data, AI algorithms can identify patterns associated with effective treatment responses, thus facilitating the tailoring of treatment strategies for individuals.207 AI-driven personalized TCM treatment decision-support systems help healthcare professionals make more accurate treatment decisions. These systems analyse patient data, including genetic information and medical history, as well as TCM principles, to recommend personalized treatment plans. They play a crucial role in bridging traditional knowledge with modern technology, enhancing the accuracy and effectiveness of TCM practices.209 AI technology is an indispensable part of patient health management and follow-up care, providing platforms for ongoing monitoring and analysis of patient data to adjust treatment plans as needed. These platforms ensure continuous assessment of patients' responses to treatments, enabling real-time adjustments and personalized care. By utilizing AI, these platforms provide dynamic support for patient management, promoting the adoption of more responsive and adaptive approaches to treatment planning and execution.210 The integration of AI with personalized treatment plans and TCM highlights the transformative shift towards more personalized and effective healthcare solutions. By harnessing the power of AI to analyse chemical data and patient-specific information, healthcare providers can create personalized treatment plans that significantly improve patient treatment outcomes.
11.3 Case studies and challenges
Niu et al.211 introduced a novel method for predicting herbal formulas called TCMFP, which leverages TCM expertise, AI, and network science (Fig. 13B). This method was applied to devise treatments for Alzheimer's disease, asthma, and atherosclerosis. Using a network-based approach, TCMFP integrates patient and herbal data to predict and validate the most effective herbal combinations. The process involves constructing a “Target–Molecule–Herb” network, identifying high-value herbal pairs, and calculating scores to evaluate herbal formulations. A genetic algorithm optimizes the selection, focusing on those with the highest efficacy scores. The effectiveness of these formulas is then substantiated through detailed validity and functional analyses, encompassing pathways and gene ontology enrichments. This innovative approach underscores the potential of integrating AI with traditional herbal practices to refine and enhance treatment strategies for complex diseases. Ahmed et al.207 proposed an enhanced deep learning model for personalized cancer treatment that combines deep learning with genetic and drug response data to predict individual responses to cancer treatment, emphasizing personalization in oncology. The model provides high accuracy in predicting drug response, which is critical for effective and customized treatment strategies in cancer care. Tian et al.212 constructed the largest drug transcriptome platform ITCM based on active ingredients of TCM to date, including 496 active ingredients of TCM in unified high-throughput experiments. Multi-scale biological analysis was performed to reveal the mechanism of each active ingredient, and six state-of-the-art feature search methods were integrated into the TCM-Query screening strategy to identify potential active ingredients for special diseases and achieve personalized TCM recommendation. Gao et al.213 defined a new concept of “active metabolite combination therapy (AMCT)” rules to clarify how bioactive metabolites of TCM produce synergistic effects. AMCT rules clarify the mechanism of action of these combination therapies in disease treatment by integrating multidisciplinary technologies such as molecular biology, biochemistry, pharmacology, analytical chemistry and pharmacodynamics. AMCT rules provide new ideas for the development of new active metabolite combination drugs and promote personalized treatment of TCM.
While AI shows tremendous promise in personalized treatment plans, challenges remain in data privacy, algorithm transparency, and the integration of AI systems with clinical workflows. Future advancements should aim to improve AI algorithms for more accurate predictions, ensure ethical use of patient data, and promote interdisciplinary collaboration to fully realize AI's potential in personalized healthcare. The integration of AI into personalized treatment plans represents a significant step towards more detailed and effective healthcare approaches, offering customized treatments that consider genetic, lifestyle, and pathological differences among individuals. As AI technology continues to evolve, its application in personalized medicine is expected to deepen, bringing new possibilities for patient care and treatment outcomes.
12. Applications of AI in network pharmacology
12.1 Network pharmacology chemical data analysis
Network pharmacology, leveraging systems biology and computational tools, has become a key method for elucidating the complex mechanisms of TCM. It shifts the research paradigm from “single target, single drug” to “network targets, multi-component therapy”, particularly suitable for analysing the multi-component, multi-target treatment strategies of TCM. The role of AI in this area is profound, enabling the analysis of complex chemical data, unveiling mechanisms of action, and optimizing therapeutic approaches with unprecedented precision and efficiency.214 Drug target networks aid in understanding how TCM compounds interact with biological targets, thus deepening our understanding of the synergistic effects of TCM formulations. By mapping these interactions, researchers can reveal the complex interplay between TCM components and biological pathways, guiding the development of more effective and balanced therapeutic solutions.215 Disease–gene–drug networks play a crucial role in identifying the genetic basis of diseases and potential TCM compounds that can modulate these genetic factors. By integrating disease, gene, and drug data, network pharmacology helps to gain a deeper understanding of disease mechanisms and identify new therapeutic targets for TCM.216
Fig. 14A illustrates the general process for AI applications in network pharmacology. AI excels at screening large chemical datasets to identify and categorize active TCM compounds. This process lays the foundation for building detailed pharmacological networks containing a wide range of potential drug targets and therapeutic pathways.217 Utilizing AI to predict and verify drug–target interactions enhances the efficiency of network pharmacology studies. By employing algorithms capable of handling complex biological data, researchers can predict how TCM compounds interact with specific targets, facilitating the discovery of new therapeutic pathways and optimizing TCM formulations for increased efficacy and safety.140 The rich network pharmacology facilitated by AI provides a comprehensive framework for understanding the multifaceted nature of TCM. Through the construction and analysis of complex biological networks, this approach enables a thorough understanding of drug actions, supports the discovery of new therapeutic targets, and promotes the design of multi-target synergistic drug combinations.
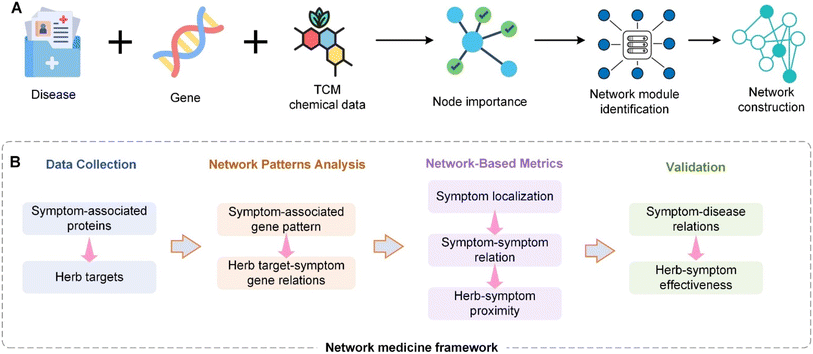 |
| Fig. 14 Applications of AI in network pharmacology. (A) General process for AI applications in network pharmacology. This workflow demonstrates how AI integrates disease, genetic, and TCM chemical data to identify node importance, network modules, and ultimately construct a comprehensive network model. (B) Case study on network medicine framework. It illustrates the use of a network medicine framework to analyse the relationships between herbal prescriptions, patient symptoms, and genetic data to localize symptoms, identify key patterns, and establish a network-based medicinal strategy. | |
12.2 Chemical component network modeling and analysis
AI technologies are crucial for constructing more accurate drug–target interaction networks and disease-related networks. These models can analyse network topologies to identify key nodes and connections representing therapeutic targets or disease mechanisms. This detailed network construction allows for a finer understanding of the complex interactions within TCM formulations and their impacts on biological pathways.218,219 The application of AI in network pharmacology extends to network analysis, utilizing techniques such as node importance evaluation and network module identification to unveil the complex mechanisms of drug action. Through these methods, AI helps clarify how different components of TCM act synergistically within various biological networks, aiding in a deeper understanding of their therapeutic effects.215,220 AI plays a crucial role in designing TCM treatment plans based on network pharmacology principles. By integrating data from various sources, AI can help devise treatment strategies addressing multiple aspects of a disease, enhancing the efficacy and balance of TCM treatments. This approach aligns with the holistic nature of TCM, aiming to treat diseases by addressing both the root causes and manifestations within the entire biological network.169 Utilizing AI for personalized TCM healthcare, by analysing vast amounts of data including specific patient conditions and network pharmacology analysis results, allows for treatment plans customized to individual patient needs. This personalization ensures more effective treatment plans with fewer side effects, significantly improving patient treatment outcomes. AI's ability to process and analyse complex datasets makes it a valuable tool for personalized TCM treatment, making treatments more targeted and efficient.221 The integration of AI with network pharmacology represents a significant advancement in TCM research and application. By constructing detailed networks and using sophisticated AI techniques to analyse them, researchers can uncover the complex interactions and mechanisms behind TCM formulations.
12.3 Case studies and challenges
AI technology has made significant contributions to network pharmacology, particularly in revealing the complex mechanisms of action of TCM and optimizing treatment strategies. Gan et al.40 developed a network medicine framework to decipher the efficacy of TCM through the lens of network pharmacology (Fig. 14B). By analysing the interconnectedness of disease symptoms and herbal targets within the human protein interactome, they established a clear topological relationship that underpins TCM treatment principles. This framework identifies clusters of symptom-associated proteins and examines their proximity to herbal targets on the protein network. Their findings suggest that the closer a herb's targets to these symptom clusters, the more effective the herb in treating the associated symptoms. The study utilized both empirical data and patient records to validate the framework, demonstrating its potential in streamlining herb discovery and repurposing. This innovative approach offers a scientific basis for the traditional practice of symptom-focused diagnosis and treatment in TCM, showcasing the integration of modern network analysis with ancient herbal wisdom. Peng et al.222 used network pharmacology, machine learning, molecular docking, and experimental evaluation to explore the potential mechanism of Qizhu Tangshen Recipe (QZTS) in the treatment of diabetic kidney disease (DKD). The results show that the therapeutic effect of QZTS on DKD involves multiple targets and pathways, and is mainly concentrated in glucose and lipid metabolism disorders, inflammation and angiogenesis. Li et al.223 screened potential biomarkers in different parts of coconut from the perspectives of measurability and effectiveness based on fingerprinting, machine learning, and network pharmacology. Network pharmacology results show that 11 ingredients, including dehydropurocosic acid and 16α-hydroxydehydroapernic acid, have high connectivity in the “component–target–pathway” network and are the main active ingredients. The network pharmacology approach provides a new strategy for exploring potential biomarkers and explains the clinical application and rational development and utilization of Poria cocos and Pi Poria cocos. He et al.224 used gas chromatography-mass spectrometry (GC-MS), ultra performance liquid chromatography coupled with quadrupole time-of-flight mass spectrometry (UPLC-Q-TOF/MS), network pharmacology analysis, and experimental validation to elucidate the potential pharmacodynamic substances and mechanisms of Wuwei Musk Pill (WPW) in treating rheumatoid arthritis (RA). The results show that 11 ingredients, including sungolamine, collantine, sarconine C, ursolic acid, arjunoic acid, piperacin, ellagic acid, and arjunogen ligand, may be the main active ingredients of WPW in treating RA.
Challenges in applying AI to network pharmacology research commonly involve integrating data and ensuring model accuracy. Addressing these challenges requires standardized research processes and assessment metrics. The future of AI in network pharmacology looks promising to handle larger datasets and enhance model interpretability. This advancement can significantly improve the precision and depth of TCM research. Collaboration between AI technologies, TCM knowledge, and network pharmacology is crucial for innovation and advancing TCM research. This interdisciplinary approach can propel TCM from an empirically-based practice to an evidence-based science, contributing to its modernization and global acceptance.
13. Applications of AI in elucidating pharmacological actions
13.1 Analysis of chemical components in pharmacology
The integration of AI with pharmacology, particularly TCM, marks significant advancements in analysing complex chemical data and unveiling the mechanisms of drug actions. Utilizing TCM databases and chemical data analysis, AI technologies have the potential to revolutionize the study of pharmacological actions, aiding in a deeper understanding of drug interactions, actions, and therapeutic outcomes.217Fig. 15A illustrates the general process for AI applications in elucidating pharmacological actions. The complexity of TCM, characterized by its multi-component and multi-target approach, presents unique challenges in understanding pharmacological actions. The diversity and complexity of chemical components in TCM compounds require advanced analytical techniques to decipher their pharmacological potential.214 Traditional methods often fall short in handling the vast, complex datasets associated with TCM. The limitations in processing large-scale chemical data and elucidating complex pharmacological actions underscore the need for AI-driven methods to enhance the efficiency and accuracy of pharmacological research.225 Machine learning and deep learning models, aid in identifying TCM chemical components and predicting their potential activities. This application of AI facilitates the exploration of new therapeutic compounds, enhancing the drug discovery and development process.226 AI extends to analysing the relationships between chemical components and their pharmacological activities. Utilizing data mining and pattern recognition technologies, AI helps reveal the mechanisms through which TCM compounds exert their therapeutic effects, paving the way for new drug development and optimization strategies.227
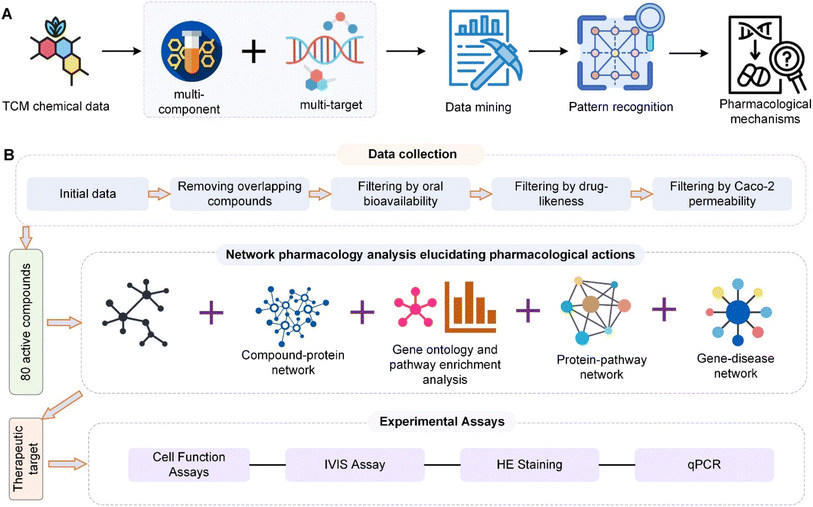 |
| Fig. 15 Utilization of AI in elucidating pharmacological actions. (A) General process for AI applications in elucidating pharmacological actions. This showcases the workflow where AI integrates TCM chemical data to analyse multi-component and multi-target interactions, employ data mining and pattern recognition techniques, and ultimately elucidate pharmacological mechanisms. (B) Case study on elucidating pharmacological actions through network pharmacology analysis. This diagram illustrates the detailed steps in network pharmacology analysis starting from the TCMS database to the integration of various networks including herb-compound, compound-protein, gene ontology, and pathway enrichment, contributing to the understanding of pharmacological actions. | |
13.2 AI-driven virtual bioequivalence prediction in TCM research
AI-driven virtual bioequivalence (VBE) prediction offers a transformative approach to the sustainable development and modernization of TCM. By leveraging machine learning, deep learning algorithms, chemoinformatics, and network pharmacology, AI can effectively screen for potential alternatives to rare or expensive TCM materials. This approach is critical in identifying bioequivalent compounds that are more accessible and cost-effective, ensuring the continued availability and efficacy of TCM therapies without over-reliance on scarce herbal resources. Recent advancements have demonstrated the potential of AI to identify and predict bioequivalent substitutes by analyzing the molecular structure, chemical components, and targets of herbs. Liang et al. employed a totality-of-the-evidence approach to evaluate the quality consistency of Scutellariae Radix, highlighting how AI can be used to ensure bioequivalence between traditional decoctions and dispensing granules.228 This study underlines the importance of maintaining therapeutic efficacy while exploring cost-effective alternatives. Similarly, Li et al. used a network pharmacology and bioactive equivalence assessment strategy to discover quality markers in Da-Cheng-Qi decoction, demonstrating how AI can identify bioequivalent alternatives for expensive ingredients, ensuring the formula's effectiveness against intestinal obstruction.229 In the broader context of drug development, Bego et al. highlighted the role of AI in virtual bioequivalence studies, emphasizing its capability to predict pharmacokinetic parameters and screen for viable alternatives to traditional materials.230 Similarly, Jereb et al. demonstrated the effectiveness of AI-driven physiologically-based biopharmaceutics modeling (PBBM) in predicting bioequivalence across different formulations, paving the way for identifying cost-effective substitutes.231 In TCM, where many medicinal plants are rare or endangered, AI's ability to analyze complex datasets and predict molecular interactions is invaluable. Ren et al. illustrated this by using AI to discover bioequivalent small molecules that could replace expensive or hard-to-source herbal components, demonstrating how AI can maintain therapeutic efficacy while ensuring sustainability.232 Furthermore, Loisios-Konstantinidis et al. explored how AI can establish clinically relevant bioequivalence, further illustrating the capability of AI to identify and validate economic alternatives in TCM.233 The integration of these AI-driven approaches into TCM research not only enhances the drug development process but also supports the sustainable use of herbal resources. By identifying bioequivalent substitutes that are both effective and economical, AI contributes to the modernization of TCM, ensuring its global relevance and accessibility.
13.3 Case studies and challenges
Huang et al.234 employed a novel approach that synergizes network pharmacology and experimental methodologies to investigate the therapeutic effects of the TCM formula Huanglian Jiedu Decoction (HLJDD) on liver cancer (Fig. 15B). The research began with the construction of comprehensive networks mapping herb–compound interactions and their downstream effects on proteins, pathways, and genes. This predictive model pinpointed liver cancer, specifically hepatocellular carcinoma, as a primary therapeutic target for HLJDD, highlighting the significant role of inflammation-related genes. Subsequent validation of these network pharmacology predictions was conducted through rigorous experimental procedures using both in vitro cell models and in vivo mouse models. These experiments demonstrated HLJDD's capacity to inhibit tumor growth, proliferation, and migration, and to induce apoptosis in liver cancer cells. This integrative method of combining computational predictions with empirical evidence effectively underscores the potential of HLJDD in treating liver cancer and validates the practical utility of AI-driven network pharmacology in TCM research. Zhang et al.167 proposed a systems pharmacology approach to explore the molecular mechanisms of TCM. This model integrated the identification of bioactive compounds, target prediction, and elucidation of molecular mechanisms through network pharmacology, highlighting its utility in revealing synergistic effects and formulation analysis in TCM. Wang et al.235 used computational systems pharmacology to focus on the TCM formula Tianma Gouteng Yin, revealing its potential targets and pathways for treating Alzheimer's disease. This approach highlighted the neuroprotective and anti-inflammatory actions of the formula, providing a new perspective on the correlation between TCM and specific diseases. Yang et al.236 developed a machine learning model to identify neuroprotective compounds in Xiaoxuming Tang, a traditional formula used for stroke treatment. This innovative application of phenotype-based machine learning models for compound screening represents a significant advancement in the integration of AI with TCM research. Qin et al.237 conducted a study on Shenkang, a TCM formula, using network pharmacology to predict and validate its effects on chronic kidney disease. This study illustrated how AI can help elucidate the complex interactions of TCM components and predict their impacts on chronic diseases.
Integrating different data types and ensuring the accuracy of AI models are major obstacles that need to be addressed. To overcome these issues, standardized research protocols and robust evaluation metrics are crucial. This standardization not only enhances the reproducibility of results but also strengthens the validation process, ensuring that research findings are reliable and applicable in a clinical setting. The future of using AI in network pharmacology research appears promising to handle larger datasets and enhance model interpretability. These advancements could significantly improve the precision and depth of TCM research, providing insights into the complex mechanisms of multi-component herbal formulations. As AI technologies mature, they will be able to explore vast pharmacological networks, revealing subtle interactions between herbal compounds that were previously obscured. Collaboration between AI technologies, TCM knowledge, and network pharmacology is essential for fostering innovation and advancing the field. By bridging these disciplines, TCM has the potential to transition from an empirically-based practice to an evidence-based scientific practice. This transformation is expected to promote the modernization and global acceptance of TCM, fostering a more integrated approach to health that combines ancient wisdom with contemporary scientific practice.
14. Challenges and prospects
14.1 Challenges
The integration of AI in TCM presents both remarkable opportunities and significant challenges. Understanding and addressing these challenges is crucial for the successful application of AI in TCM and for harnessing its full potential.
Current challenges in AI integration in TCM are as follow:
• Data quality and standardization: one of the primary challenges in AI integration into TCM is the quality and standardization of data. TCM practices involve a wide range of herbs, practices, and individualized treatments, which can lead to inconsistencies in data. Xu et al.238 and Zhou et al.239 highlight the need for standardized data collection methods to improve the accuracy of AI applications in TCM.
• Complexity of TCM practices: the inherently complex nature of TCM, with its emphasis on holistic treatment and the balance of bodily systems, poses a challenge for AI models. AI systems must be sophisticated enough to understand and interpret the nuances of TCM, including the interactions between different herbal compounds and their effects on the human body.98,217
• Integration with clinical practice: successfully integrating AI into TCM clinical practice is a significant hurdle. Practitioners need to trust and understand AI outputs for them to be effectively used in treatment planning. The importance of developing user-friendly AI tools that can be seamlessly integrated into the daily practice of TCM should be emphasized.240,241
• Ethical considerations and patient privacy: as with all applications of AI in healthcare, ethical considerations, particularly regarding patient privacy, are paramount. The increasing use of patient data for AI applications in TCM necessitates robust privacy protections and ethical guidelines.101,242
To address the challenges, future outlook and trends in AI-enhanced TCM research should be given attention:
• Advanced AI models and algorithms: future trends in TCM research will likely see the development of more advanced AI models and algorithms capable of handling the complexities of TCM data. These models will be more adept at pattern recognition, predictive analytics, and simulating the holistic approach of TCM.100,243
• Greater emphasis on personalized medicine: AI's role in facilitating personalized medicine within TCM is set to grow. With the capacity to analyse vast datasets and patient histories, AI will enable more tailored treatment strategies.244,245 AI models facilitate the analysis of complex symptom patterns and recommend appropriate TCM prescriptions, thereby personalizing treatment strategies.44,106
• Integrative approaches combining TCM and Western medicine: there will be an increasing trend towards integrative approaches that combine TCM and Western medicine, leveraging the strengths of both. AI will play a crucial role in this integration, helping to bridge the gap between these two systems.246,247
• Expanding the scope of AI applications in TCM: future research will likely expand the scope of AI applications in TCM beyond diagnosis and treatment to include areas like drug discovery, preventive medicine, and patient education.106,248
The integration of AI in TCM research is poised for significant growth despite the current challenges. By addressing issues related to data quality, complexity, clinical integration, and ethics, AI can profoundly enhance TCM practices. The future outlook for AI in TCM is promising, with advancements in AI technology offering new opportunities for personalized medicine, integrative approaches, and expanded applications. As AI continues to evolve, it will undoubtedly play an increasingly vital role in the development and modernization of TCM, offering exciting possibilities for future healthcare innovations.
14.2 Perspectives and prospects
The integration of AI in TCM represents a significant stride in modernizing ancient practices with contemporary technology. This fusion promise enhanced diagnostic precision, personalized treatment plans, and a deeper understanding of pharmacological mechanisms, as seen in the advancements of TCM diagnosis using AI tools.122,249
AI's capability to process and analyse large datasets has led to significant improvements in TCM diagnosis. AI's application in treatment strategies, particularly in herbal medicine prescription and syndrome differentiation, is noteworthy. AI models have been instrumental in identifying effective herb combinations, thus aiding in developing more targeted treatment strategies.40,250
The role of AI in elucidating the pharmacological actions of TCM formulations is particularly compelling. By employing network pharmacology and AI algorithms, researchers have gained insights into the complex interactions of herbal compounds and their therapeutic pathways.148,224 These advancements are pivotal in understanding the multi-target and multi-pathway actions of TCM formulations, thereby contributing to a more scientific basis for TCM practices.
Despite these advancements, the integration of AI into TCM is not without challenges. The complexity of TCM formulations, the need for standardized data, and the integration of AI into clinical practice pose significant hurdles.52,56 Furthermore, ethical considerations, particularly in terms of patient data privacy and the interpretability of AI models, remain critical concerns that need addressing.113,240 The future of AI in TCM is promising, with trends pointing towards more personalized medicine and integrative approaches combining TCM and Western medicine. The development of more sophisticated AI models capable of interpreting the holistic and individualized nature of TCM treatments is essential.119,251 Additionally, expanding AI applications beyond diagnosis and treatment to include preventive medicine and patient education is a potential area for growth.
The intersection of AI and TCM opens up new horizons in healthcare, offering innovative approaches to diagnosis, treatment, and pharmacological research. While challenges remain, particularly in data standardization, model interpretability, and ethical considerations, the potential benefits of this integration are substantial. Future research should focus on developing AI tools that are more attuned to the nuances of TCM, thereby ensuring that this ancient practice evolves in harmony with modern technological advancements. The synergy between AI and TCM not only enhances the efficacy and precision of TCM but also contributes significantly to the broader field of personalized medicine.
15. Conclusions
The exploration of TCM databases and the integration of AI represent a transformative era in medical research and practice. This review has delved into the vast potential and significant advancements that AI has brought to TCM, a field steeped in history and rich in holistic therapeutic wisdom. The union of AI and TCM has not only redefined our understanding of ancient practices but has also unlocked new avenues in research methodologies and treatment strategies.
A standout achievement in this fusion is AI's enhanced ability to sift through and make sense of the intricate data housed in TCM databases. These repositories, brimming with age-old herbal knowledge, treatment techniques, and patient histories, have become the bedrock for AI systems to learn and predict with remarkable efficacy. The spectrum of AI applications, from machine learning algorithms to sophisticated neural networks, has been instrumental in pioneering drug discovery, refining diagnostic accuracy, honing in on syndrome differentiation, and crafting personalized treatment plans. Notably, AI's role in network pharmacology has shed light on the intricate dance of herbal components, unraveling their pharmacological secrets and therapeutic pathways.
Looking ahead, the horizon of research in this melding of TCM and AI is as broad as it is fascinating. One promising direction lies in crafting AI algorithms specifically tailored to the unique diagnostic and treatment ethos of TCM. These could involve advanced pattern recognition and predictive modeling that honor TCM's holistic essence. Equally thrilling is the potential integration of AI with cutting-edge fields like genomics and metabolomics, deepening our understanding of TCM's interaction at the molecular and cellular levels. Expanding and enriching TCM databases with more diverse and comprehensive data will be crucial. This expansion is key not only to enhancing the precision and relevance of AI applications but also to ensuring their adaptability across various clinical scenarios and patient backgrounds. As we tread this path, maintaining data integrity, standardizing practices, and upholding ethical AI use in healthcare will be of utmost importance.
The application of AI in TCM transcends technological progress; it's a cultural symphony, harmonizing age-old wisdom with modern scientific innovation. As AI continues to evolve, its synergy with TCM is poised to significantly influence the landscape of personalized medicine, offering treatment solutions that are more effective, efficient, and tailored to individual needs. This convergence of AI and TCM is poised to revolutionize not just our perception of traditional medicine but also our broader approach to healthcare. We stand at the beginning of a thrilling journey where AI's role in elevating TCM research and practice holds limitless potential for future breakthroughs.
Data availability
No primary research results, no new data were generated or analysed as part of this review.
Author contributions
Zhilin Song: methodology, writing – original draft preparation. Guanxing Chen: methodology, and data curation, writing – original draft preparation. Calvin Yu-Chian Chen: conceptualization of this study, methodology and writing – original draft preparation.
Conflicts of interest
The authors declare no competing interests.
Acknowledgements
This work was supported by the National Natural Science Foundation of China (Grant No. 62176272), Research and Development Program of Guangzhou Science and Technology Bureau (No. 2023B01J1016), and Key-Area Research and Development Program of Guangdong Province (No. 2020B1111100001).
Notes and references
- H. D. Ma, Y. R. Deng, Z. Tian and Z. X. Lian, Traditional Chinese medicine and immune regulation, Clin. Rev. Allergy Immunol., 2013, 44, 229–241 CrossRef PubMed.
- Z. Yan, Z. Lai and J. Lin, Anticancer Properties of Traditional Chinese Medicine, Comb. Chem. High Throughput Screening, 2017, 20, 423–429 CrossRef CAS PubMed.
- D. Cyranoski, Why Chinese medicine is heading for clinics around the world, Nature, 2018, 561, 448–450 CrossRef CAS PubMed.
- H. Yuan, Q. Ma, L. Ye and G. Piao, The Traditional Medicine and Modern Medicine from Natural Products, Molecules, 2016, 21, 559 CrossRef PubMed.
- Y. Cai, Q. Luo, M. Sun and H. Corke, Antioxidant activity and phenolic compounds of 112 traditional Chinese medicinal plants associated with anticancer, Life Sci., 2004, 74, 2157–2184 CrossRef CAS PubMed.
- Y. Tu, The discovery of artemisinin (qinghaosu) and gifts from Chinese medicine, Nat. Med., 2011, 17, 1217–1220 CrossRef CAS PubMed.
- Y. Zhao, L. Xu, Z. Qiao, L. Gao, S. Ding, X. Ying, Y. Su, N. Lin, B. He and J. Pu, YiXin-Shu, a ShengMai-San-based traditional Chinese medicine formula, attenuates myocardial ischemia/reperfusion injury by suppressing mitochondrial mediated apoptosis and upregulating liver-X-receptor alpha, Sci. Rep., 2016, 6, 23025 CrossRef CAS PubMed.
- Z. Zhang, Y. Ji, N. Hu, Q. Yu, X. Zhang, J. Li, F. Wu, H. Xu, Q. Tang and X. Li, Ferroptosis-induced anticancer effect of resveratrol with a biomimetic nano-delivery system in colorectal cancer treatment, Asian J. Pharm. Sci., 2022, 17, 751–766 CrossRef PubMed.
- Y. Jin, W. Ji, Y. Shi, X. Wang and X. Yang, Meta-path guided graph attention network for explainable herb recommendation, Health Inf. Sci. Syst., 2023, 11, 5 CrossRef PubMed.
- G.-P. Li, L.-N. Wu, K. Li, F. Xu, J.-R. Wu, L.-L. Zhao, H.-L. Guo and T.-W. Zhu, Exploring the mechanism of Xingnao Kaiqiao acupuncture method for ischemic stroke based on EEG dynamic changes: a study protocol of an exploratory, prospective, interventional trial, TMR Integr. Med., 2022, 6, e22033 Search PubMed.
- A. Lu, M. Jiang, C. Zhang and K. Chan, An integrative approach of linking traditional Chinese medicine pattern classification and biomedicine diagnosis, J. Ethnopharmacol., 2012, 141, 549–556 CrossRef PubMed.
- D. J. Newman and G. M. Cragg, Natural Products as Sources of New Drugs over the Nearly Four Decades from 01/1981 to 09/2019, J. Nat. Prod., 2020, 83, 770–803 CrossRef CAS PubMed.
- E. Chan, M. Tan, J. N. Xin, S. Sudarsanam and D. E. Johnson, Interactions between traditional Chinese medicines and Western therapeutics, Curr. Opin. Drug Discovery Dev., 2010, 13, 50–65 CAS.
- C. Keji and X. U. Hao, The integration of traditional Chinese medicine and Western medicine, Eur. Rev., 2003, 11, 225–235 CrossRef.
- A. L. Harvey, Natural products in drug discovery, Drug Discovery Today, 2008, 13, 894–901 CrossRef CAS PubMed.
- A. L. Harvey, R. Edrada-Ebel and R. J. Quinn, The re-emergence of natural products for drug discovery in the genomics era, Nat. Rev. Drug Discovery, 2015, 14, 111–129 CrossRef CAS PubMed.
- J. Abramson, J. Adler, J. Dunger, R. Evans, T. Green, A. Pritzel, O. Ronneberger, L. Willmore, A. J. Ballard, J. Bambrick, S. W. Bodenstein, D. A. Evans, C. C. Hung, M. O'Neill, D. Reiman, K. Tunyasuvunakool, Z. Wu, A. Zemgulyte, E. Arvaniti, C. Beattie, O. Bertolli, A. Bridgland, A. Cherepanov, M. Congreve, A. I. Cowen-Rivers, A. Cowie, M. Figurnov, F. B. Fuchs, H. Gladman, R. Jain, Y. A. Khan, C. M. R. Low, K. Perlin, A. Potapenko, P. Savy, S. Singh, A. Stecula, A. Thillaisundaram, C. Tong, S. Yakneen, E. D. Zhong, M. Zielinski, A. Zidek, V. Bapst, P. Kohli, M. Jaderberg, D. Hassabis and J. M. Jumper, Accurate structure prediction of biomolecular interactions with AlphaFold 3, Nature, 2024, 630, 493–500 CrossRef CAS PubMed.
- W. F. Li, J. G. Jiang and J. Chen, Chinese medicine and its modernization demands, Arch. Med. Res., 2008, 39, 246–251 CrossRef PubMed.
- Q. Xu, R. Bauer, B. M. Hendry, T. P. Fan, Z. Zhao, P. Duez, M. S. Simmonds, C. M. Witt, A. Lu, N. Robinson, D. A. Guo and P. J. Hylands, The quest for modernisation of traditional Chinese medicine, BMC Complementary Altern. Med., 2013, 13, 132 CrossRef PubMed.
- Q. Wang, N. Wei, L. Xu, H. Hua, J. Li, Y. Jiang and L. Chen, TCM Fingerprint database: A digital approach to scientifically reflect the internal quality of traditional Chinese medicine, Pharmacol. Res. – Mod. Chin. Med., 2023, 7, 100261 CrossRef.
-
X. Wang, A. Zhang, H. Sun, G. Yan, P. Wang and Y. Han, in Serum Pharmacochemistry of Traditional Chinese Medicine, 2017, pp. 1–6, DOI:10.1016/b978-0-12-811147-5.00001-7.
- Y. Wang, M. Liu, M. Jafari and J. Tang, A critical assessment of Traditional Chinese Medicine databases as a source for drug discovery, Front. Pharmacol, 2024, 15, 1303693 CrossRef PubMed.
- T. Xue, S. Gong, T.-H. Xie, W.-J. Li and J.-P. Huang, Development status and trend of traditional Chinese medicine database, TMR Mod. Herb. Med., 2022, 5, 19 CrossRef.
- Y. Wang, X. Shi, L. Li, T. Efferth and D. Shang, The Impact of Artificial Intelligence on Traditional Chinese Medicine, Am. J. Chin. Med., 2021, 49, 1297–1314 CrossRef PubMed.
- S. Ma, J. Liu, W. Li, Y. Liu, X. Hui, P. Qu, Z. Jiang, J. Li and J. Wang, Machine learning in TCM with natural products and molecules: current status and future perspectives, Chin. Med., 2023, 18, 43 CrossRef PubMed.
- Z. Tian, D. Wang, X. Sun, Y. Fan, Y. Guan, N. Zhang, M. Zhou, X. Zeng, Y. Yuan, H. Bu and H. Wang, Current status and trends of artificial intelligence research on the four traditional Chinese medicine diagnostic methods: a scientometric study, Ann. Transl. Med., 2023, 11, 145 CrossRef PubMed.
- S. Zhang, W. Wang, X. Pi, Z. He and H. Liu, Advances in the Application of Traditional Chinese Medicine Using Artificial Intelligence: A Review, Am. J. Chin. Med., 2023, 51, 1067–1083 CrossRef CAS PubMed.
- R. Zhang, X. Zhu, H. Bai and K. Ning, Network Pharmacology Databases for Traditional Chinese Medicine: Review and Assessment, Front. Pharmacol, 2019, 10, 123 CrossRef CAS PubMed.
- W. Li, X. Ge, S. Liu, L. Xu, X. Zhai and L. Yu, Opportunities and challenges of traditional Chinese medicine doctors in the era of artificial intelligence, Front. Med., 2023, 10, 1336175 CrossRef PubMed.
- E. Zhou, Q. Shen and Y. Hou, Integrating artificial intelligence into the modernization of traditional Chinese medicine industry: a review, Front. Pharmacol, 2024, 15, 1181183 CrossRef CAS PubMed.
- S. Li, B. Zhang and N. Zhang, Network target for screening synergistic drug combinations with application to traditional Chinese medicine, BMC Syst. Biol., 2011, 5(Suppl 1), S10 CrossRef PubMed.
- Z. Chen, M. Zhao, L. You, R. Zheng, Y. Jiang, X. Zhang, R. Qiu, Y. Sun, H. Pan, T. He, X. Wei, Z. Chen, C. Zhao and H. Shang, Developing an artificial intelligence method for screening hepatotoxic compounds in traditional Chinese medicine and Western medicine combination, Chin. Med., 2022, 17, 58 CrossRef CAS PubMed.
- K. Hioki, T. Hayashi, Y. Natsume-Kitatani, K. Kobiyama, B. Temizoz, H. Negishi, H. Kawakami, H. Fuchino, E. Kuroda, C. Coban, N. Kawahara and K. J. Ishii, Machine Learning-Assisted Screening of Herbal Medicine Extracts as Vaccine Adjuvants, Front. Immunol., 2022, 13, 847616 CrossRef CAS PubMed.
- F. I. Saldivar-Gonzalez, V. D. Aldas-Bulos, J. L. Medina-Franco and F. Plisson, Natural product drug discovery in the artificial intelligence era, Chem. Sci., 2022, 13, 1526–1546 RSC.
- C. Feng, Y. Shao, B. Wang, Y. Qu, Q. Wang, Y. Li and T. Yang, Development and Application of Artificial Intelligence in Auxiliary TCM Diagnosis, J. Evidence-Based Complementary Altern. Med., 2021, 2021, 6656053 Search PubMed.
- H. Zhang, W. Ni, J. Li and J. Zhang, Artificial Intelligence-Based Traditional Chinese Medicine Assistive Diagnostic System: Validation Study, JMIR Med. Inform., 2020, 8, e17608 CrossRef PubMed.
- D. Sui, L. Zhang and F. Yang, Data-driven based four examinations in TCM: a survey, Digital Chin. Med., 2022, 5, 377–385 CrossRef.
- J. Wang, L. Duan, H. Li, J. Liu and H. Chen, Construction of an Artificial Intelligence Traditional Chinese Medicine Diagnosis and Treatment Model Based on Syndrome Elements and Small-Sample Data, Engineering, 2022, 8, 29–32 CrossRef.
- F. Guo, X. Tang, W. Zhang, J. Wei, S. Tang, H. Wu and H. Yang, Exploration of the mechanism of traditional Chinese medicine by AI approach using unsupervised machine learning for cellular functional similarity of compounds in heterogeneous networks, XiaoErFuPi granules as an example, Pharmacol. Res., 2020, 160, 105077 CrossRef CAS PubMed.
- X. Gan, Z. Shu, X. Wang, D. Yan, J. Li, S. Ofaim, R. Albert, X. Li, B. Liu, X. Zhou and A.-L. Barabási, Network medicine framework reveals generic herb-symptom effectiveness of traditional Chinese medicine, Sci. Adv., 2023, 9, eadh0215 CrossRef CAS PubMed.
- C. Zhu, T. Cai, Y. Jin, J. Chen, G. Liu, N. Xu, R. Shen, Y. Chen, L. Han, S. Wang, C. Wu and M. Zhu, Artificial intelligence and network pharmacology based investigation of pharmacological mechanism and substance basis of Xiaokewan in treating diabetes, Pharmacol. Res., 2020, 159, 104935 CrossRef CAS PubMed.
- X. Liang, H. Li and S. Li, A novel network pharmacology approach to analyse traditional herbal formulae: the Liu-Wei-Di-Huang pill as a case study, Mol. BioSyst., 2014, 10, 1014–1022 RSC.
- W. Zhou, K. Yang, J. Zeng, X. Lai, X. Wang, C. Ji, Y. Li, P. Zhang and S. Li, FordNet: recommending traditional Chinese medicine formula via deep neural network integrating phenotype and molecule, Pharmacol. Res., 2021, 173, 105752 CrossRef CAS PubMed.
- A. Gong, L. Guo, Y. Yu, Y. Xia, X. Deng and Z. Hu, Spectrum-image dual-modality fusion empowered accurate and efficient classification system for traditional Chinese medicine, Inf. Fusion, 2024, 101, 101981 CrossRef.
-
G. Huang, H. Lu, X. Zhu and J. Luo, in 2022 IEEE International Conference on Image Processing (ICIP), IEEE, Bordeaux, France, 2022, pp. 871–875 Search PubMed.
- W. Y. Jiang, Therapeutic wisdom in traditional Chinese medicine: a perspective from modern science, Trends Pharmacol. Sci., 2005, 26, 558–563 CrossRef CAS PubMed.
- A. Zhang, H. Sun, P. Wang, Y. Han and X. Wang, Future perspectives of personalized medicine in traditional Chinese medicine: a systems biology approach, Complement. Ther. Med., 2012, 20, 93–99 CrossRef PubMed.
- D. He, J. H. Huang, Z. Y. Zhang, Q. Du, W. J. Peng, R. Yu, S. F. Zhang, S. H. Zhang and Y. H. Qin, A Network Pharmacology-Based Strategy For Predicting Active Ingredients And Potential Targets Of LiuWei DiHuang Pill In Treating Type 2 Diabetes Mellitus, Drug Des., Dev. Ther., 2019, 13, 3989–4005 CrossRef CAS PubMed.
- D. H. Zhang, K. L. Wu, X. Zhang, S. Q. Deng and B. Peng, In silico screening of Chinese herbal medicines with the potential to directly inhibit 2019 novel coronavirus, J. Integr. Med., 2020, 18, 152–158 CrossRef PubMed.
- J. Su, G. Sun, J. An, Y. Ao, J. Li, Z. Shen, L. Zhang, S. Zhang, Y. Yang and Y. Shi, Efficacy and safety of the integration of traditional Chinese medicine and western medicine in the treatment of diabetes-associated cognitive decline: a systematic review and meta-analysis, Front. Pharmacol, 2023, 14, 1280736 CrossRef CAS PubMed.
- Z. Chen, D. Zhang, C. Liu, H. Wang, X. Jin, F. Yang and J. Zhang, A Traditional Chinese Medicine Diagnostic Prediction Model for Holistic Syndrome Differentiation Based on Deep Learning, Integr. Med. Res., 2023, 101019, DOI:10.1016/j.imr.2023.101019.
- Z. Liu, H. He, S. Yan, Y. Wang, T. Yang and G. Z. Li, End-to-End Models to Imitate Traditional Chinese Medicine Syndrome Differentiation in Lung Cancer Diagnosis: Model Development and Validation, JMIR Med. Inform., 2020, 8, e17821 CrossRef PubMed.
- C. Y. Chen, TCM Database@Taiwan: the world's largest traditional Chinese medicine database for drug screening in silico, PLoS One, 2011, 6, e15939 CrossRef CAS PubMed.
- Q. Lv, G. Chen, H. He, Z. Yang, L. Zhao, H. Y. Chen and C. Y. Chen, TCMBank: bridges between the largest herbal medicines, chemical ingredients, target proteins, and associated diseases with intelligence text mining, Chem. Sci., 2023, 14, 10684–10701 RSC.
- Y. Zhang, X. Li, Y. Shi, T. Chen, Z. Xu, P. Wang, M. Yu, W. Chen, B. Li, Z. Jing, H. Jiang, L. Fu, W. Gao, Y. Jiang, X. Du, Z. Gong, W. Zhu, H. Yang and H. Xu, ETCM v2.0: An update with comprehensive resource and rich annotations for traditional Chinese medicine, Acta Pharm. Sin. B, 2023, 13, 2559–2571 CrossRef CAS PubMed.
- H. Y. Xu, Y. Q. Zhang, Z. M. Liu, T. Chen, C. Y. Lv, S. H. Tang, X. B. Zhang, W. Zhang, Z. Y. Li, R. R. Zhou, H. J. Yang, X. J. Wang and L. Q. Huang, ETCM: an encyclopaedia of traditional Chinese medicine, Nucleic Acids Res., 2019, 47, D976–D982 CrossRef CAS PubMed.
- X. Kong, C. Liu, Z. Zhang, M. Cheng, Z. Mei, X. Li, P. Liu, L. Diao, Y. Ma, P. Jiang, X. Kong, S. Nie, Y. Guo, Z. Wang, X. Zhang, Y. Wang, L. Tang, S. Guo, Z. Liu and D. Li, BATMAN-TCM 2.0: an enhanced integrative database for known and predicted interactions between traditional Chinese medicine ingredients and target proteins, Nucleic Acids Res., 2023, D1110–D1120, DOI:10.1093/nar/gkad926.
- T. Li, Y. Zhong, T. Tang, J. Luo, H. Cui, R. Fan, Y. Wang and D. Wang, Formononetin induces vasorelaxation in rat thoracic aorta via regulation of the PI3K/PTEN/Akt signaling pathway, Drug Des., Dev. Ther., 2018, 12, 3675–3684 CrossRef CAS PubMed.
- S. K. Kim, S. Nam, H. Jang, A. Kim and J. J. Lee, TM-MC: a database of medicinal materials and chemical compounds in Northeast Asian traditional medicine, BMC Complementary Altern. Med., 2015, 15, 218 CrossRef PubMed.
- X. Li, J. Ren, W. Zhang, Z. Zhang, J. Yu, J. Wu, H. Sun, S. Zhou, K. Yan, X. Yan and W. Wang, LTM-TCM: A comprehensive database for the linking of Traditional Chinese Medicine with modern medicine at molecular and phenotypic levels, Pharmacol. Res., 2022, 178, 106185 CrossRef CAS PubMed.
- P. Yang, J. Lang, H. Li, J. Lu, H. Lin, G. Tian, H. Bai, J. Yang and K. Ning, TCM-Suite: A comprehensive and holistic platform for Traditional Chinese Medicine component identification and network pharmacology analysis, iMeta, 2022, 1, e47 CrossRef PubMed.
- D. Yan, G. Zheng, C. Wang, Z. Chen, T. Mao, J. Gao, Y. Yan, X. Chen, X. Ji, J. Yu, S. Mo, H. Wen, W. Han, M. Zhou, Y. Wang, J. Wang, K. Tang and Z. Cao, HIT 2.0: an enhanced platform for Herbal Ingredients' Targets, Nucleic Acids Res., 2022, 50, D1238–D1243 CrossRef CAS PubMed.
- H. Ye, L. Ye, H. Kang, D. Zhang, L. Tao, K. Tang, X. Liu, R. Zhu, Q. Liu, Y. Z. Chen, Y. Li and Z. Cao, HIT: linking herbal active ingredients to targets, Nucleic Acids Res., 2011, 39, D1055–D1059 CrossRef CAS PubMed.
- Q. Chen, L. Springer, B. O. Gohlke, A. Goede, M. Dunkel, R. Abel, K. Gallo, S. Preissner, A. Eckert, L. Seshadri and R. Preissner, SuperTCM: A biocultural database combining biological pathways and historical linguistic data of Chinese Materia Medica for drug development, Biomed. Pharmacother., 2021, 144, 112315 CrossRef CAS PubMed.
- L. X. Zhang, J. Dong, H. Wei, S. H. Shi, A. P. Lu, G. M. Deng and D. S. Cao, TCMSID: a simplified integrated database for drug discovery from traditional chinese medicine, J. Cheminf., 2022, 14, 89 Search PubMed.
- J. Dong, N.-N. Wang, Z.-J. Yao, L. Zhang, Y. Cheng, D. Ouyang, A.-P. Lu and D.-S. Cao, ADMETlab: a platform for systematic ADMET evaluation based on a comprehensively collected ADMET database, J. Cheminf., 2018, 10, 29 Search PubMed.
- G. Xiong, Z. Wu, J. Yi, L. Fu, Z. Yang, C. Hsieh, M. Yin, X. Zeng, C. Wu, A. Lu, X. Chen, T. Hou and D. Cao, ADMETlab 2.0: an integrated online platform for accurate and comprehensive predictions of ADMET properties, Nucleic Acids Res., 2021, 49, W5–W14 CrossRef CAS PubMed.
- Y. Djoumbou Feunang, R. Eisner, C. Knox, L. Chepelev, J. Hastings, G. Owen, E. Fahy, C. Steinbeck, S. Subramanian, E. Bolton, R. Greiner and D. S. Wishart, ClassyFire: automated chemical classification with a comprehensive, computable taxonomy, J. Cheminf., 2016, 8, 61 Search PubMed.
- Z. Liu, C. Cai, J. Du, B. Liu, L. Cui, X. Fan, Q. Wu, J. Fang and L. Xie, TCMIO: A Comprehensive Database of Traditional Chinese Medicine on Immuno-Oncology, Front. Pharmacol, 2020, 11, 439 CrossRef CAS PubMed.
- S. Fang, L. Dong, L. Liu, J. Guo, L. Zhao, J. Zhang, D. Bu, X. Liu, P. Huo, W. Cao, Q. Dong, J. Wu, X. Zeng, Y. Wu and Y. Zhao, HERB: a high-throughput experiment- and reference-guided database of traditional Chinese medicine, Nucleic Acids Res., 2021, 49, D1197–D1206 CrossRef CAS PubMed.
- X. Chen, H. Zhou, Y. B. Liu, J. F. Wang, H. Li, C. Y. Ung, L. Y. Han, Z. W. Cao and Y. Z. Chen, Database of traditional Chinese medicine and its application to studies of mechanism and to prescription validation, Br. J. Pharmacol., 2006, 149, 1092–1103 CrossRef CAS PubMed.
- Y. Wu, F. Zhang, K. Yang, S. Fang, D. Bu, H. Li, L. Sun, H. Hu, K. Gao, W. Wang, X. Zhou, Y. Zhao and J. Chen, SymMap: an integrative database of traditional Chinese medicine enhanced by symptom mapping, Nucleic Acids Res., 2019, 47, D1110–D1117 CrossRef PubMed.
- L. Huang, D. Xie, Y. Yu, H. Liu, Y. Shi, T. Shi and C. Wen, TCMID 2.0: a comprehensive resource for TCM, Nucleic Acids Res., 2018, 46, D1117–D1120 CrossRef CAS PubMed.
- R. Xue, Z. Fang, M. Zhang, Z. Yi, C. Wen and T. Shi, TCMID: Traditional Chinese Medicine integrative database for herb molecular mechanism analysis, Nucleic Acids Res., 2013, 41, D1089–D1095 CrossRef CAS PubMed.
- J. Ru, P. Li, J. Wang, W. Zhou, B. Li, C. Huang, P. Li, Z. Guo, W. Tao, Y. Yang, X. Xu, Y. Li, Y. Wang and L. Yang, TCMSP: a database of systems pharmacology for drug discovery from herbal medicines, J. Cheminf., 2014, 6, 13 Search PubMed.
- A. Durrant, M. Markovic, D. Matthews, D. May, J. Enright and G. Leontidis, The role of cross-silo federated learning in facilitating data sharing in the agri-food sector, Comput. Electron. Agric., 2022, 193, 106648 CrossRef.
- M. Müllner, Molecular polymer bottlebrushes in nanomedicine: therapeutic and diagnostic applications, Chem. Commun., 2022, 58, 5683–5716 RSC.
- X. Zheng and Z. Cai, Privacy-preserved data sharing towards multiple parties in industrial IoTs, IEEE J. Sel. Area. Commun., 2020, 38, 968–979 Search PubMed.
- Y. Zhu and Y. Li, A data sharing and integration technology for heterogeneous databases, Int. J. Circuits Syst. Signal Process., 2022, 16, 232–238 Search PubMed.
-
T.-T. Huang, X.-F. Wang, C.-D. Yuan, F. Wang, X.-Y. Wang, Z.-L. Huang, J.-H. Li and Z.-G. Shao, in 2022 3rd Asia Conference on Computers and Communications (ACCC), IEEE, Shanghai, China, 2022, pp. 13–18 Search PubMed.
- W. P. Walters and R. Barzilay, Critical assessment of AI in drug discovery, Expert Opin. Drug Discovery, 2021, 16, 937–947 CrossRef CAS PubMed.
- Y. A. Ivanenkov, D. Polykovskiy, D. Bezrukov, B. Zagribelnyy, V. Aladinskiy, P. Kamya, A. Aliper, F. Ren and A. Zhavoronkov, Chemistry42: an AI-driven platform for molecular design and optimization, J. Chem. Inf. Model., 2023, 63, 695–701 CrossRef CAS PubMed.
- G. Bai, T. Zhang, Y. Hou, G. Ding, M. Jiang and G. Luo, From quality markers to data mining and intelligence assessment: A smart quality-evaluation strategy for traditional Chinese medicine based on quality markers, Phytomedicine, 2018, 44, 109–116 CrossRef PubMed.
- M. Li and X. Zheng, Identification of ancient Chinese medical prescriptions and case data analysis under artificial intelligence Gpt algorithm: a case study of song dynasty medical literature, IEEE Access, 2023, 131453–131464 Search PubMed.
- Y. Yu, C. Yao and D.-a. Guo, Insight into chemical basis of traditional Chinese medicine based on the state-of-the-art techniques of liquid chromatography – mass spectrometry, Acta Pharm. Sin. B, 2021, 11, 1469–1492 CrossRef CAS PubMed.
- R. Huang, S. Ma, S. Dai and J. Zheng, Application of Data Fusion in Traditional Chinese Medicine: A Review, Sensors, 2023, 24, 106 CrossRef PubMed.
- J. Yang, Y. Li, Q. Liu, L. Li, A. Feng, T. Wang, S. Zheng, A. Xu and J. Lyu, Brief introduction of medical database and data mining technology in big data era, J. Evid. Base Med., 2020, 13, 57–69 CrossRef PubMed.
- M. S. Gal and D. L. Rubinfeld, Data standardization, N. Y. Univ. Law Rev., 2019, 94, 737 Search PubMed.
- Y. Sang and S. Tian, J. Phys.: Conf. Ser., 2021, 1941, 012020 CrossRef.
-
Y. Zhao, Y. Fan and Y. Yang, in 2022 7th International Conference on Cyber Security and Information Engineering (ICCSIE), IEEE, Brisbane, Australia, 2022, pp. 94–97 Search PubMed.
- K. Wang, J. Dong, Y. Wang and H. Yin, Securing data with blockchain and AI, IEEE Access, 2019, 7, 77981–77989 Search PubMed.
- O. Borgogno and G. Colangelo, Data sharing and interoperability: Fostering innovation and competition through APIs, Comput. Law Secur. Rev., 2019, 35, 105314 CrossRef.
- Q. Lv, G. Chen, H. He, Z. Yang, L. Zhao, K. Zhang and C. Y. Chen, TCMBank-the largest TCM database provides deep learning-based Chinese-Western medicine exclusion prediction, Signal Transduction Targeted Ther., 2023, 8, 127 CrossRef PubMed.
- T. Y. Tsai, K. W. Chang and C. Y. Chen, iScreen: world's first cloud-computing web server for virtual screening and de novo drug design based on TCM database@Taiwan, J. Comput.-Aided Mol. Des., 2011, 25, 525–531 CrossRef CAS PubMed.
- K. W. Chang, T. Y. Tsai, K. C. Chen, S. C. Yang, H. J. Huang, T. T. Chang, M. F. Sun, H. Y. Chen, F. J. Tsai and C. Y. Chen, iSMART: an integrated cloud computing web server for traditional Chinese medicine for online virtual screening, de novo evolution and drug design, J. Biomol. Struct. Dyn., 2011, 29, 243–250 CrossRef CAS PubMed.
- L. Wang, G. B. Zhou, P. Liu, J. H. Song, Y. Liang, X. J. Yan, F. Xu, B. S. Wang, J. H. Mao, Z. X. Shen, S. J. Chen and Z. Chen, Dissection of mechanisms of Chinese medicinal formula Realgar-Indigo naturalis as an effective treatment for promyelocytic leukemia, Proc. Natl. Acad. Sci. U. S. A., 2008, 105, 4826–4831 CrossRef CAS PubMed.
-
C. Y.-C. Chen, When Modern Computational Systems Biology Meets Traditional Chinese Medicine, Traditional Chinese Medicine, RSC Publication, 2013, ch. 5 Search PubMed.
- Z. Zhao, X. Ren, K. Song, Y. Qiang, J. Zhao, J. Zhang and P. Han, PreGenerator: TCM Prescription Recommendation Model Based on Retrieval and Generation Method, IEEE Access, 2023, 11, 103679–103692 Search PubMed.
- W. Zhong, L. Zhao, Z. Yang and C. Yu-Chian Chen, Graph convolutional network approach to investigate potential selective Limk1 inhibitors, J. Mol. Graph. Model., 2021, 107, 107965 CrossRef CAS PubMed.
- N. Cheng, Y. Chen, W. Gao, J. Liu, Q. Huang, C. Yan, X. Huang and C. Ding, An Improved Deep Learning Model: S-TextBLCNN for Traditional Chinese Medicine Formula Classification, Front. Genet., 2021, 12, 807825 CrossRef PubMed.
-
Y. Zhang, L. Wang, J. Song, Z. Ji and Q. Wu, in 2021 36th Youth Academic Annual Conference of Chinese Association of Automation (YAC), IEEE, Nanchang, China, 2021, pp. 424–429 Search PubMed.
- J. Si, Z. Tian, D. Li, L. Zhang, L. Yao, W. Jiang, J. Liu, R. Zhang and X. Zhang, A multi-modal clustering method for traditional Chinese medicine clinical data via media convergence, CAAI Trans. Intell. Technol., 2023, 8, 390–400 CrossRef.
-
Z. Zhang, Y. Wang, S. Liu, L. Wang and M. Zhang, in Cognitive Systems and Information Processing, 2024, ch. 23, pp. 309–321, DOI:10.1007/978-981-99-8018-5_23.
- S. Abubaker Bagabir, N. K. Ibrahim, H. Abubaker Bagabir and R. Hashem Ateeq, Covid-19 and Artificial Intelligence: Genome sequencing, drug development and vaccine discovery, J. Infect. Public Health, 2022, 15, 289–296 CrossRef PubMed.
- S. Vatansever, A. Schlessinger, D. Wacker, H. Ü. Kaniskan, J. Jin, M. M. Zhou and B. Zhang, Artificial intelligence and machine learning-aided drug discovery in central nervous system diseases: State-of-the-arts and future directions, Med. Res. Rev., 2020, 41, 1427–1473 CrossRef PubMed.
- Q. Xu, W. Tang, F. Teng, W. Peng, Y. Zhang, W. Li, C. Wen and J. Guo, Intelligent Syndrome Differentiation of Traditional Chinese Medicine by ANN: A Case Study of Chronic Obstructive Pulmonary Disease, IEEE Access, 2019, 7, 76167–76175 Search PubMed.
- W. Chen, J. Tong, R. He, Y. Lin, P. Chen, Z. Chen and X. Liu, An easy method for identifying 315 categories of commonly-used Chinese herbal medicines based on automated image recognition using AutoML platforms, Inform. Med. Unlocked, 2021, 25, 100607 CrossRef.
- H. Hu, C. Cheng, Q. Ye, L. Peng and Y. Shen, Enhancing traditional Chinese medicine diagnostics: Integrating ontological knowledge for multi-label symptom entity classification, Math. Biosci. Eng., 2023, 21, 369–391 Search PubMed.
-
D. Shi, F. Lin, Y. Li, Q. Chen, Y. Lin, W. Zhu, D. Li and X. Zhang, in 2023 IEEE/ACIS 21st International Conference on Software Engineering Research, Management and Applications (SERA), IEEE, Orlando, FL, USA, 2023, pp. 423–427 Search PubMed.
- C. Rong, X. Li, X. Sun and H. Sun, Chinese Medicine Prescription Recommendation Using Generative Adversarial Network, IEEE Access, 2022, 10, 12219–12228 Search PubMed.
-
C. Xing, Y. Huo, X. Huang, C. Lu, Y. Liang and A. Wang, in 2020 International Conference on Artificial Intelligence and Electromechanical Automation (AIEA), IEEE, Tianjin, China, 2020, pp. 140–146 Search PubMed.
-
Y. Wu, Z. Yin, K. Zhou, R. Wang, Y. Yang, Z. Yin, C. Ruan and Y. Zhang, in 2021 IEEE International Conference on Bioinformatics and Biomedicine (BIBM), IEEE, Houston, TX, USA, 2021, pp.
1104–1111 Search PubMed.
- H. Zhang, J. Zhang, W. Ni, Y. Jiang, K. Liu, D. Sun and J. Li, Transformer- and Generative Adversarial Network–Based Inpatient Traditional Chinese Medicine Prescription Recommendation: Development Study, JMIR Med. Inform., 2022, 10, e35239 CrossRef PubMed.
-
Z. Wang, K. Li, Q. Ren, K. Yao and Y. Zhu, in 2023 IEEE International Conference on Bioinformatics and Biomedicine (BIBM), IEEE, Istanbul, Turkiye, 2023, pp. 4647–4654 Search PubMed.
-
J. Zhu, Q. Gong, C. Zhou and H. Luan, in Proceedings of the 2023 4th International Symposium on Artificial Intelligence for Medicine Science, ACM, Chengdu, China, 2023, pp. 1036–1042 Search PubMed.
- G. Yang, X. Liu, J. Shi, Z. Wang and G. Wang, Comput. Methods Programs Biomed. Update, 2024, 6, 100158 CrossRef.
-
H. Zhang, X. Wang, Z. Meng, Z. Chen, P. Zhuang, Y. Jia, D. Xu and W. Guo, Qibo: A Large Language Model for Traditional Chinese Medicine, arXiv, 2024, preprint, arXiv:2403.16056, DOI:10.48550/arXiv.2403.16056.
- N. Deng, H. Fu, X. Chen and A. K. Sangaiah, Named Entity Recognition of Traditional Chinese Medicine Patents Based on BiLSTM-CRF, Wireless Commun. Mobile Comput., 2021, 2021, 1–12 Search PubMed.
- W. Zhou, K. Yang, J. Zeng, X. Lai, X. Wang, C. Ji, Y. Li, P. Zhang and S. Li, FordNet: Recommending traditional Chinese medicine formula via deep neural network integrating phenotype and molecule, Pharmacol. Res., 2021, 173, 105752 CrossRef CAS PubMed.
- Y. Yang, Q. Li, Z. Liu, F. Ye and K. Deng, Understanding traditional Chinese medicine via statistical learning of expert-specific Electronic Medical Records, Quant. Biol., 2019, 7, 210–232 CrossRef CAS.
- Y.-C. Lin, W.-T. Huang, S.-C. Ou, H.-H. Hung, W.-Z. Cheng, S.-S. Lin, H.-J. Lin and S.-T. Huang, Neural network analysis of Chinese herbal medicine prescriptions for patients with colorectal cancer, Compl. Ther. Med., 2019, 42, 279–285 CrossRef PubMed.
- X. Chen, C. Ruan, Y. Zhang and H. Chen, Heterogeneous information network based clustering for precision traditional Chinese medicine, BMC Med. Inf. Decis. Making, 2019, 19, 1–12 CrossRef PubMed.
- A. Raza, T. A. Chohan, M. Buabeid, E.-S. A. Arafa, T. A. Chohan, B. Fatima, K. Sultana, M. S. Ullah and G. Murtaza, Deep learning in drug discovery: a futuristic modality to materialize the large datasets for cheminformatics, J. Biomol. Struct. Dyn., 2023, 41, 9177–9192 CrossRef CAS PubMed.
- M. K. Tripathi, A. Nath, T. P. Singh, A. Ethayathulla and P. Kaur, Evolving scenario of big data and Artificial Intelligence (AI) in drug discovery, Mol. Diversity, 2021, 25, 1439–1460 CrossRef CAS PubMed.
- A. Lavecchia, Deep learning in drug discovery: opportunities, challenges and future prospects, Drug discovery today, 2019, 24, 2017–2032 CrossRef PubMed.
- H.-Y. Yeh, C.-T. Chao, Y.-P. Lai and H.-W. Chen, Predicting the associations between meridians and Chinese traditional medicine using a cost-sensitive graph convolutional neural network, Int. J. Environ. Res. Public Health, 2020, 17, 740 CrossRef PubMed.
- Z. Zhao, X. Ren, K. Song, Y. Qiang, J. Zhao, J. Zhang and P. Han, PreGenerator: TCM prescription recommendation model based on retrieval and generation method, IEEE Access, 2023, 103679–103692 Search PubMed.
- T. Bai, H. Guan, S. Wang, Y. Wang and L. Huang, Traditional Chinese medicine entity relation extraction based on CNN
with segment attention, Neural Comput. Appl., 2022, 1–10 CAS.
- A. C. Mater and M. L. Coote, Deep learning in chemistry, J. Chem. Inf. Model., 2019, 59, 2545–2559 CrossRef CAS PubMed.
- C. F. Lipinski, V. G. Maltarollo, P. R. Oliveira, A. B. Da Silva and K. M. Honorio, Advances and perspectives in applying deep learning for drug design and discovery, Front. Robot. AI., 2019, 6, 108 CrossRef PubMed.
- C. Isert, K. Atz and G. Schneider, Structure-based drug design with geometric deep learning, Curr. Opin. Struct. Biol., 2023, 79, 102548 CrossRef CAS PubMed.
- S. Wu, K. Roberts, S. Datta, J. Du, Z. Ji, Y. Si, S. Soni, Q. Wang, Q. Wei and Y. Xiang, Deep learning in clinical natural language processing: a methodical review, J. Am. Med. Inf. Assoc., 2020, 27, 457–470 CrossRef PubMed.
- R. Zuo, Y. Xiong, J. Wang and E. J. M. Carranza, Deep learning and its application in geochemical mapping, Earth Sci. Rev., 2019, 192, 1–14 CrossRef.
- S. H. Lu, H. L. Zhai, B. Q. Zhao, B. Yin and L. Zhu, Novel approach to the analysis of chemical third-order data, J. Chem. Inf. Model., 2020, 60, 4750–4756 CrossRef CAS PubMed.
- Q. M. Bui, H. N. M. Nguyen, V. N. Le, T. T. Nguyen, N. M. Truong, N. T. Nguyen, Q. H. Le and Q. T. Nguyen, AIQS-DB: Revolutionizing the Simultaneous Analysis of Organic Compounds, Appl. Sci., 2023, 13, 8031 CrossRef CAS.
- D. Gao, M. Niu, S.-z. Wei, C.-e. Zhang, Y.-f. Zhou, Z.-w. Yang, L. Li, J.-b. Wang, H.-z. Zhang and L. Zhang, Identification of a pharmacological biomarker for the bioassay-based quality control of a thirteen-component TCM formula (Lianhua Qingwen) used in treating influenza A virus (H1N1) infection, Front. Pharmacol, 2020, 11, 746 CrossRef CAS PubMed.
- G.-F. Gong, Y.-Y. Chen, Y.-M. Zhang, Y.-Q. Fan, Q. Zhou, H.-L. Yang, Q.-P. Zhang, H. Yao, T.-B. Wei and Q. Lin, A novel bis-component AIE smart gel with high selectivity and sensitivity to detect CN−, Fe3+ and H2PO4−, Soft Matter, 2019, 15, 6348–6352 RSC.
- L. David, A. Thakkar, R. Mercado and O. Engkvist, Molecular representations in AI-driven drug discovery: a review and practical guide, J. Cheminf., 2020, 12, 56 CAS.
- N. Biswas and S. Chakrabarti, Artificial intelligence (AI)-based systems biology approaches in multi-omics data analysis of cancer, Front. Oncol., 2020, 10, 588221 CrossRef PubMed.
- W. Zhang, Y. Chen, H. Jiang, J. Yang, Q. Wang, Y. Du and H. Xu, Integrated strategy for accurately screening biomarkers based on metabolomics coupled with network pharmacology, Talanta, 2020, 211, 120710 CrossRef CAS PubMed.
-
B. Yingngam, A. Navabhatra and P. Sillapapibool, in Using Traditional Design Methods to Enhance AI-Driven Decision Making, IGI Global, 2024, pp. 1–63 Search PubMed.
- Q. Wu, C. Cai, P. Guo, M. Chen, X. Wu, J. Zhou, Y. Luo, Y. Zou, A. L. Liu, Q. Wang, Z. Kuang and J. Fang, In silico Identification and Mechanism Exploration of Hepatotoxic Ingredients in Traditional Chinese Medicine, Front. Pharmacol., 2019, 10, 458 CrossRef CAS PubMed.
- C. Liu, Q. Jiang, Z. Zhou, P. Lei, P. Zhang, X. Chai, G. Pan, Y. Wang and M. Jiang, Studies on chemical profiling and pharmacokinetics of traditional Chinese medicine Formula Kang Shuai Lao Pian, Arabian J. Chem., 2024, 17, 105398 CrossRef CAS.
- M.-C. Chung, L.-J. Su, C.-L. Chen and L.-C. Wu, AI-assisted literature exploration of innovative Chinese medicine formulas, Front. Pharmacol, 2024, 15, 1347882 CrossRef CAS PubMed.
- X. J. Wang, J. L. Ren, A. H. Zhang, H. Sun, G. L. Yan, Y. Han and L. Liu, Novel applications of mass spectrometry-based metabolomics in herbal medicines and its active ingredients: current evidence, Mass Spectrom. Rev., 2019, 38, 380–402 CrossRef CAS PubMed.
- K. Yang, X.-M. Long, J.-J. Cao, Y.-J. Li, Y. Wu, X. Bai, Z.-L. Sun and Z.-Y. Liu, An analytical strategy to explore the multicomponent pharmacokinetics of herbal medicine independently of standards: Application in Gelsemium elegans extracts, J. Pharm. Biomed. Anal., 2019, 176, 112833 CrossRef CAS PubMed.
- X. Liu, W. Jiang, M. Su, Y. Sun, H. Liu, L. Nie and H. Zang, Quality evaluation of traditional Chinese medicines based on fingerprinting, J. Sep. Sci., 2020, 43, 6–17 CrossRef CAS PubMed.
- S. Bi, L. Xu, S. Chen, S. Bu, Y. Xu and J. Zhao, Detection of Herbal Combinations and Pharmacological Mechanisms of Clinical Prescriptions for Coronary Heart Disease Using Data Mining and Network Pharmacology, J. Evidence-Based Complementary Altern. Med., 2021, 2021, 1–20 Search PubMed.
- Y. Chai, Y. Huang, J. Han, F. Chen, J. Zhu, H. Zeng, W. Li and X. Zhang, To Explore the Herbal Combinations and Pharmacological Mechanisms of Yourong Huang in the Treatment of Cervical Spondylosis Based on Data Mining and Network Pharmacology, Nat. Prod. Commun., 2023, 18, 1934578X2311804 CrossRef.
- L. Ren, X. Zheng, J. Liu, W. Li, W. Fu, Q. Tang, J. Wang and G. Du, Network pharmacology study of traditional Chinese medicines for stroke treatment and effective constituents screening, J. Ethnopharmacol., 2019, 242, 112044 CrossRef CAS PubMed.
- J. Guo, L. Zhang, Y. Shang, X. Yang, J. Li, J. He, X. Gao and Y.-x. Chang, A strategy for intelligent chemical profiling-guided precise quantitation of multi-components in traditional Chinese medicine formulae-QiangHuoShengShi decoction, J. Chromatogr. A, 2021, 1649, 462178 CrossRef CAS PubMed.
- M. Lee, H. Shin, M. Park, A. Kim, S. Cha and H. Lee, Systems pharmacology approaches in herbal medicine research: a brief review, BMB Rep., 2022, 55, 417 CrossRef CAS PubMed.
- H. Zhang, J. Pan, X. Wu, A.-R. Zuo, Y. Wei and Z.-L. Ji, Large-scale target identification of herbal medicine using a reverse docking approach, ACS Omega, 2019, 4, 9710–9719 CrossRef CAS PubMed.
- Y. Wang, H. Yang, L. Chen, M. Jafari and J. Tang, Network-based modeling of herb combinations in traditional Chinese medicine, Briefings Bioinf., 2021, 22, bbab106 CrossRef PubMed.
- M.-t. Zuo, Y.-c. Liu, Z.-l. Sun, L. Lin, Q. Tang, P. Cheng and Z.-y. Liu, An integrated strategy toward comprehensive characterization and quantification of multiple components from herbal medicine: An application study in Gelsemium elegans, Chin. Herb. Med., 2021, 13, 17–32 Search PubMed.
- Y. Han, H. Sun, A. Zhang, G. Yan and X.-j. Wang, Chinmedomics, a new strategy for evaluating the therapeutic efficacy of herbal medicines, Pharmacol. Ther., 2020, 216, 107680 CrossRef CAS PubMed.
- Y. Wang, H. Yang, L. Chen, M. Jafari and J. Tang, Network-based modeling of herb combinations in traditional Chinese medicine, Briefings Bioinf., 2021, 22, bbab106 CrossRef PubMed.
- T. Yi, C. Lin, J. En-Ci and Y. Ji-Zhong, Application and prospects of hyperspectral imaging and deep learning in traditional Chinese medicine in context of AI and industry 4.0, China J. Chin. Mater. Med., 2020, 45, 5438–5442 Search PubMed.
- L.-X. Guo, R. Li, K. Liu, J. Yang, H.-J. Li, S.-L. Li, J.-Q. Liu, L.-F. Liu and G.-Z. Xin, Structural characterization and discrimination of Chinese medicinal materials with multiple botanical origins based on metabolite profiling and chemometrics analysis: Clematidis Radix et Rhizoma as a case study, J. Chromatogr. A, 2015, 1425, 129–140 CrossRef CAS PubMed.
- X. Ren, X. X. Shao, X. X. Li, X. H. Jia, T. Song, W. Y. Zhou, P. Wang, Y. Li, X. L. Wang, Q. H. Cui, P. J. Qiu, Y. G. Zhao, X. B. Li, F. C. Zhang, Z. Y. Li, Y. Zhong, Z. G. Wang and X. J. Fu, Identifying potential treatments of COVID-19 from Traditional Chinese Medicine (TCM) by using a data-driven approach, J. Ethnopharmacol., 2020, 258, 112932 CrossRef CAS PubMed.
- H.-T. Zhang, M.-X. Huang, X. Liu, X.-C. Zheng, X.-H. Li, G.-Q. Chen, J.-Y. Xia and Z.-S. Hong, Evaluation of the adjuvant efficacy of natural herbal medicine on COVID-19: a retrospective matched case-control study, Am. J. Chin. Med., 2020, 48, 779–792 CrossRef CAS PubMed.
- S. Shi, F. Wang, H. Yao, S. Kou, W. Li, B. Chen, Y. Wu, X. Wang, C. Pei and D. Huang, Oral Chinese herbal medicine on immune responses during coronavirus disease 2019: A systematic review and meta-analysis, Front. Med., 2022, 8, 685734 CrossRef PubMed.
- L. Ang, E. Song, X.-Y. Hu, H. W. Lee, Y. Chen and M. S. Lee, Herbal medicine intervention for the treatment of COVID-19: a living systematic review and cumulative meta-analysis, Front. Pharmacol, 2022, 13, 906764 CrossRef CAS PubMed.
- X. Yang, Y. Wang, R. Byrne, G. Schneider and S. Yang, Concepts of artificial intelligence for computer-assisted drug discovery, Chem. Rev., 2019, 119, 10520–10594 CrossRef CAS PubMed.
- N. E. Thomford, D. A. Senthebane, A. Rowe, D. Munro, P. Seele, A. Maroyi and K. Dzobo, Natural products for drug discovery in the 21st century: innovations for novel drug discovery, Int. J. Mol. Sci., 2018, 19, 1578 CrossRef PubMed.
- J. Deng, Z. Yang, I. Ojima, D. Samaras and F. Wang, Artificial intelligence in drug discovery: applications and techniques, Briefings Bioinf., 2022, 23, bbab430 CrossRef PubMed.
- W. Zhang, Y. Huai, Z. Miao, A. Qian and Y. Wang, Systems pharmacology for investigation of the mechanisms of action of traditional Chinese medicine in drug discovery, Front. Pharmacol., 2019, 10, 743 CrossRef CAS PubMed.
- G. Amendola and S. Cosconati, PyRMD: a new fully automated ai-powered ligand-based virtual screening tool, J. Chem. Inf. Model., 2021, 61, 3835–3845 CrossRef CAS PubMed.
- P. Zhang, D. Zhang, W. Zhou, L. Wang, B. Wang, T. Zhang and S. Li, Network pharmacology: towards the artificial intelligence-based precision traditional Chinese medicine, Briefings Bioinf., 2024, 25, bbad518 CrossRef PubMed.
- P. Hassanzadeh, F. Atyabi and R. Dinarvand, The significance of artificial intelligence in drug delivery system design, Adv. Drug Deliv. Rev., 2019, 151, 169–190 CrossRef PubMed.
- F. Guo, X. Tang, W. Zhang, J. Wei, S. Tang, H. Wu and H. Yang, Exploration of the mechanism of traditional Chinese medicine by AI approach using unsupervised machine learning for cellular functional similarity of compounds in heterogeneous networks, XiaoErFuPi granules as an example, Pharmacol. Res., 2020, 160, 105077 CrossRef CAS PubMed.
- T. T. V. Tran, A. Surya Wibowo, H. Tayara and K. T. Chong, Artificial Intelligence in Drug Toxicity Prediction: Recent Advances, Challenges, and Future Perspectives, J. Chem. Inf. Model., 2023, 63, 2628–2643 CrossRef CAS PubMed.
- T. Adeluwa, B. A. McGregor, K. Guo and J. Hur, Predicting Drug-Induced Liver Injury Using Machine Learning on a Diverse Set of Predictors, Front. Pharmacol, 2021, 12, 648805 CrossRef CAS PubMed.
- O. C. Uner, H. I. Kuru, R. G. Cinbis, O. Tastan and A. E. Cicek, DeepSide: A Deep Learning Approach for Drug Side Effect Prediction, IEEE/ACM Trans. Comput. Biol. Bioinf., 2023, 20, 330–339 CAS.
- D. Galeano, S. Li, M. Gerstein and A. Paccanaro, Predicting the frequencies of drug side effects, Nat. Commun., 2020, 11, 4575 CrossRef CAS PubMed.
- X. He, L. Zhao, W. Zhong, H.-Y. Chen, X. Shan, N. Tang and C. Y.-C. Chen, Insight into potent leads for alzheimer's disease by using several artificial intelligence algorithms, Biomed. Pharmacother., 2020, 129, 110360 CrossRef CAS PubMed.
- J.-Y. Li, H.-Y. Chen, W.-j. Dai, Q.-J. Lv and C. Y.-C. Chen, Artificial Intelligence Approach To Investigate the Longevity Drug, J. Phys. Chem. Lett., 2019, 10, 4947–4961 CrossRef CAS PubMed.
- J.-N. Gong, L. Zhao, G. Chen, X. Chen, Z.-D. Chen and C. Y.-C. Chen, A novel artificial intelligence protocol to investigate potential leads for diabetes mellitus, Mol. Diversity, 2021, 25, 1375–1393 CrossRef CAS PubMed.
- G. Feng, Y. Sun, S. Liu, F. Song, Z. Pi and Z. Liu, Stepwise targeted matching strategy from in vitro to in vivo based on ultra–high performance liquid chromatography tandem mass spectrometry technology to quickly identify and screen pharmacodynamic constituents, Talanta, 2019, 194, 619–626 CrossRef CAS PubMed.
- Z. Xian, S. S. Wang, C. Dennis, K. Hosen, R. N. Valentina, C. Kelvin and B. Alan, Synergistic Effects of Chinese Herbal Medicine: A Comprehensive Review of Methodology and Current Research, Front. Pharmacol, 2016, 7, 201 Search PubMed.
- Y. Zhang, C. M. Ip, Y. S. Lai and Z. Zuo, Overview of current herb–drug interaction databases, Drug Metab. Dispos., 2022, 50, 86–94 CrossRef CAS PubMed.
- Y. Gao, A. Liang, X. Fan, L. Hu, F. Hao and Y. Li, Safety research in traditional Chinese medicine: methods, applications, and outlook, Engineering, 2019, 5, 76–82 CrossRef CAS.
- N. Ai, X. Fan and S. Ekins, In silico methods for predicting drug–drug interactions with cytochrome P-450s, transporters and beyond, Adv. Drug Deliv. Rev., 2015, 86, 46–60 CrossRef CAS PubMed.
- Y.-N. Zhao, Y.-K. Chen, X. Chen, L.-S. Qiao, J.-F. Zhang, L.-Y. Cai, Y.-L. Pei and Y.-L. Zhang, Discovery of cytochrome P450 enzymes-inhibiting components in traditional Chinese medicine, China J. Chin. Mater. Med., 2020, 45, 923–931 Search PubMed.
- E. L. Romm and I. F. Tsigelny, Artificial Intelligence in Drug Treatment, Annu. Rev. Pharmacol. Toxicol., 2020, 60, 353–369 CrossRef CAS PubMed.
- Y. Zhang, Z. Deng, X. Xu, Y. Feng and S. Junliang, Application of Artificial Intelligence in Drug–Drug Interactions Prediction: A Review, J. Chem. Inf. Model., 2023, 2158–2173 Search PubMed.
- S. Chen, T. Li, L. Yang, F. Zhai, X. Jiang, R. Xiang and G. Ling, Artificial intelligence-driven prediction of multiple drug interactions, Briefings Bioinf., 2022, 23, bbac427 CrossRef PubMed.
- Z. Ke, M. Liu, J. Liu, Z. Su, L. Li, M. Qian, X. Zhang, L. Cao, T. Wang and Z. Wang, The Application of Artificial Intelligence in the Research and Development of Traditional Chinese Medicine, Int. J. Drug Discov. Pharmacol., 2024, 100001 CrossRef.
- S. P. Borse, D. P. Singh and M. Nivsarkar, Understanding the relevance of herb-drug interaction studies with special focus on interplays: a prerequisite for integrative medicine, Porto Biomed. J., 2019, 4, e15 CrossRef PubMed.
- J. Perrot, C. Bennetau-Pelissero, G. Miremont-Salamé, F. Petitet, S. Cluzet, H. Peyrouzet and L. Letinier, Herb-drug interaction resources: The PHYDGI database, Phytomed. Plus, 2022, 2, 100324 CrossRef.
-
A. Martins, E. Maia and I. Praça, in 2022 IEEE International Conference on E-health Networking, Application & Services (HealthCom), IEEE, Genoa, Italy, 2022, pp. 1–6 Search PubMed.
- D.-y. Zhang, W.-q. Cui, L. Hou, J. Yang, L.-y. Lyu, Z.-y. Wang, K.-G. Linghu, W.-b. He, H. Yu and Y.-j. Hu, Expanding potential targets of herbal chemicals by node2vec based on herb–drug interactions, Chin. Med., 2023, 18, 64 CrossRef CAS PubMed.
- J. Zhu, Y. Liu, Y. Zhang, Z. Chen, Q. Li, S. Yang, X. Liu, S. Zhai, Y. Zhang and C. Wen, IHPreten: A novel supervised learning framework with attribute regularization for prediction of incompatible herb pair in traditional Chinese medicine, Neurocomputing, 2019, 338, 207–221 CrossRef.
- F. Zhang, W. Liu, J. Huang, Q.-l. Chen, D.-d. Wang, L.-w. Zou, Y.-f. Zhao, W.-d. Zhang, J.-g. Xu, H.-z. Chen and G.-b. Ge, Inhibition of drug-metabolizing enzymes by Jingyin granules: implications of herb–drug interactions in antiviral therapy, Acta Pharmacol. Sin., 2022, 43, 1072–1081 CrossRef CAS PubMed.
- M. Ge, H. Ouyang, Y. Shang, A. M. Biu, X. Wu, C. Li, F. Zuo, Y. Zhu, Z. Xue, J. Hao and J. He, Investigation of the drug–drug interaction and incompatibility mechanism between Aconitum carmichaelii Debx and Pinellia ternata (Thunb.) Breit, J. Ethnopharmacol., 2024, 330, 118212 CrossRef CAS PubMed.
- J. Zeng and X. Jia, Systems Theory-Driven Framework for AI Integration into the Holistic Material Basis Research of Traditional Chinese Medicine, Engineering, 2024, 28–50 CrossRef.
- L.-R. Li, B. Du, H.-Q. Liu and C. Chen, Artificial intelligence for personalized medicine in thyroid cancer: current status and future perspectives, Front. Oncol., 2021, 10, 604051 CrossRef PubMed.
- A. Serag, A. Ion-Margineanu, H. Qureshi, R. McMillan, M.-J. Saint Martin, J. Diamond, P. O'Reilly and P. Hamilton, Translational AI and deep learning in diagnostic pathology, Front. Med., 2019, 6, 185 CrossRef PubMed.
- Z. Ahmed, K. Mohamed, S. Zeeshan and X. Dong, Artificial intelligence with multi-functional machine learning platform development for better healthcare and precision medicine, Database, 2020, 2020, baaa010 CrossRef PubMed.
-
J. Wu, R. Zhang, T. Gong, H. Zhang, C. Wang and C. Li, A Personalized Diagnostic Generation Framework Based on Multi-source Heterogeneous Data, arXiv, 2021, preprint, arXiv:2110.13677, DOI:10.48550/arXiv.2110.13677.
-
R. Mucheng, H. Heyan, Z. Yuxiang, C. Qianwen, B. Yuan and G. Yang: TCM-SD: A Benchmark for Probing Syndrome Differentiation via Natural Language Processing, arXiv, 2022, preprint, arXiv:2203.10839, DOI:10.48550/arXiv.2203.10839.
- L. Wang, J. Zhang, G. Shan, J. Liang, W. Jin, Y. Li, F. Su, Y. Ba, X. Tian, X. Sun, D. Zhang, W. Zhang and C. l. Chen, Establishment of a Lung Cancer Discriminative Model Based on an Optimized Support Vector Machine Algorithm and Study of Key Targets of Wogonin in Lung Cancer, Front. Pharmacol, 2021, 12, 728937 CrossRef CAS PubMed.
- R. Teschke, D. Larrey, D. Melchart and G. Danan, Traditional Chinese Medicine (TCM) and Herbal Hepatotoxicity: RUCAM and the Role of Novel Diagnostic Biomarkers Such as MicroRNAs, Medicines, 2016, 3, 18 CrossRef PubMed.
- W. Ji, Y. Zhang, X. Wang and Y. Zhou, Latent semantic diagnosis in traditional chinese medicine, World Wide Web, 2017, 20, 1071–1087 CrossRef.
- M. Jafari, Y. Wang, A. Amiryousefi and J. Tang, Unsupervised Learning and Multipartite Network Models: A Promising Approach for Understanding Traditional Medicine, Front. Pharmacol, 2020, 11, 1319 CrossRef PubMed.
- A.-D. E. Parekh, O. A. Shaikh, F. Simran, S. Manan and M. Al Hasibuzzaman, AI in personalized medicine: AI-generated personalized therapy regimens based on genetic and medical history, short communication, Ann. med. surg., 2023, 5831–5833, DOI:10.1097/MS9.0000000000001320.
- H. Ahmed, S. Hamad, H. A. Shedeed and A. S. Hussein, Enhanced deep learning model for personalized cancer treatment, IEEE Access, 2022, 10, 106050–106058 Search PubMed.
- C. Xiaotong, Y.-L. A. Leung and S. Jiangang, Artificial intelligence and its application for cardiovascular diseases in Chinese medicine, Digital Chin. Med., 2022, 5, 367–376 CrossRef.
- A.-D. E. Parekh, O. A. Shaikh, S. Manan and M. Al Hasibuzzaman, Artificial intelligence (AI) in personalized medicine: AI-generated personalized therapy regimens based on genetic and medical history, Ann. med. surg., 2023, 85, 5831–5833 CrossRef PubMed.
- J. Resnikoff, Y. Giraldo and L. Williamson, Report: Evaluating the ability of the TMA Clinical Decision Support platform to identify interventions in a clinical setting, medRxiv, 2021, 21257617 Search PubMed.
- Q. Niu, H. Li, L. Tong, S. Liu, W. Zong, S. Zhang, S. Tian, J. Wang, J. Liu, B. Li, Z. Wang and H. Zhang, TCMFP: a novel herbal formula prediction method based on network target's score integrated with semi-supervised learning genetic algorithms, Briefings Bioinf., 2023, 24, bbad102 CrossRef PubMed.
- S. Tian, J. Zhang, S. Yuan, Q. Wang, C. Lv, J. Wang, J. Fang, L. Fu, J. Yang, X. Zu, J. Zhao and W. Zhang, Exploring pharmacological active ingredients of traditional Chinese medicine by pharmacotranscriptomic map in ITCM, Briefings Bioinf., 2023, 24, bbad027 CrossRef PubMed.
- Q. Gao, H. Wu, M. Chen, X. Gu, Q. Wu, T. Xie and X. Sui, Active metabolites combination therapies: towards the next paradigm for more efficient and more scientific Chinese medicine, Front. Pharmacol, 2024, 15, 1392196 CrossRef CAS PubMed.
- R. Zhang, X. Zhu, H. Bai and K. Ning, Network pharmacology databases for traditional Chinese medicine: review and assessment, Front. Pharmacol., 2019, 10, 428993 Search PubMed.
- F. Noor, M. Asif, U. A. Ashfaq, M. Qasim and M. Tahir ul Qamar, Machine learning for synergistic network pharmacology: a comprehensive overview, Briefings Bioinf., 2023, 24, bbad120 CrossRef PubMed.
- Z. Li, Y. Liu, H. Li, W. Li, X. Wu and Z. Li, Application of network pharmacology in the prevention and treatment of COVID-19 by traditional Chinese medicine, Tradit. Med. Res., 2022, 7, 21, DOI:10.53388/TMR20220225266.
- W. Xin, W. Zi-Yi, J.-H. Zheng and L. Shao, TCM network pharmacology: A new trend towards combining computational, experimental and clinical approaches, Chin. J. Nat. Med., 2021, 19, 1–11 Search PubMed.
- J. Peng, Y. Wang, J. Guan, J. Li, R. Han, J. Hao, Z. Wei and X. Shang, An end-to-end heterogeneous graph representation learning-based framework for drug-target interaction prediction, Briefings Bioinf., 2021, 22, bbaa430 CrossRef PubMed.
- Y. You, X. Lai, Y. Pan, H. Zheng, J. Vera, S. Liu, S. Deng and L. Zhang, Artificial intelligence in cancer target identification and drug discovery, Signal Transduction Targeted Ther., 2022, 7, 156 CrossRef PubMed.
- W. Zhou, X. Li, L. Han and S. Fan, Application of network pharmacology based on artificial intelligence algorithms in drug development, Network Pharmacol., 2021, 35–73 Search PubMed.
- D. Sahoo, L. Swanson, I. M. Sayed, G. D. Katkar, S. R. Ibeawuchi, Y. Mittal, R. F. Pranadinata, C. Tindle, M. Fuller, D. L. Stec, J. T. Chang, W. J. Sandborn, S. Das and P. Ghosh, Artificial intelligence guided discovery of a barrier-protective therapy in inflammatory bowel disease, Nat. Commun., 2021, 12, 4246 CrossRef CAS PubMed.
- J. Peng, K. Yang, H. Tian, Y. Lin, M. Hou, Y. Gao, X. Zhou, Z. Gao and J. Ren, The mechanisms of Qizhu Tangshen formula in the treatment of diabetic kidney disease: Network pharmacology, machine learning, molecular docking and experimental assessment, Phytomedicine, 2023, 108, 154525 CrossRef CAS PubMed.
- L. Li, Z. Zuo and Y. Wang, Practical Qualitative Evaluation and Screening of Potential Biomarkers for Different Parts of Wolfiporia cocos Using Machine Learning and Network Pharmacology, Front. Microbiol., 2022, 13, 931967 CrossRef PubMed.
- Q. He, X. Tan, S. Geng, Q. Du, Z. Pei, Y. Zhang, S. Wang and Y. Zhang, Network analysis combined with pharmacological evaluation strategy to reveal the mechanism of Tibetan medicine Wuwei Shexiang pills in treating rheumatoid arthritis, Front. Pharmacol, 2022, 13, 941013 CrossRef CAS PubMed.
- M. Johnson, M. Patel, A. Phipps, M. van der Schaar, D. Boulton and M. Gibbs, The potential and pitfalls of artificial intelligence in clinical pharmacology, CPT Pharmacometrics Syst. Pharmacol., 2023, 12, 279–284 CrossRef CAS PubMed.
- W. Chen, X. Liu, S. Zhang and S. Chen, Artificial intelligence for drug discovery: Resources, methods, and applications, Mol. Ther. Nucleic Acids, 2023, 31, 691–702 CrossRef CAS PubMed.
- S. K. Bhattamisra, P. Banerjee, P. Gupta, J. Mayuren, S. Patra and M. Candasamy, Artificial intelligence in pharmaceutical and healthcare research, Big Data Cogn. Comput., 2023, 7, 10 CrossRef.
- D. Liang, Y. H. Yin, L. Y. Miao, X. Zheng, W. Gao, X. D. Chen, M. Wei, S. J. Chen, S. Li, G. Z. Xin, P. Li and H. J. Li, Integrating chemical similarity and bioequivalence: A pilot study on quality consistency evaluation of dispensing granule and traditional decoction of Scutellariae Radix by a totality-of-the-evidence approach, J. Pharm. Biomed. Anal., 2019, 169, 1–10 CrossRef CAS PubMed.
- D. Li, B. Lv, D. Wang, D. Xu, S. Qin, Y. Zhang, J. Chen, W. Zhang, Z. Zhang and F. Xu, Network Pharmacology and Bioactive Equivalence Assessment Integrated Strategy Driven Q-markers Discovery for Da-Cheng-Qi Decoction to Attenuate Intestinal Obstruction, Phytomedicine, 2020, 72, 153236 CrossRef CAS PubMed.
- M. Bego, N. Patel, R. Cristofoletti and A. Rostami-Hodjegan, Proof of Concept in Assignment of Within-Subject Variability During Virtual Bioequivalence Studies: Propagation of Intra-Subject Variation in Gastrointestinal Physiology Using Physiologically Based Pharmacokinetic Modeling, AAPS J., 2022, 24, 21 CrossRef PubMed.
- R. Jereb, A. Kristl and A. Mitra, Prediction of fasted and fed bioequivalence for immediate release drug products using physiologically based biopharmaceutics modeling (PBBM), Eur. J. Pharm. Sci., 2020, 155, 105554 CrossRef CAS PubMed.
- F. Ren, X. Ding, M. Zheng, M. Korzinkin, X. Cai, W. Zhu, A. Mantsyzov, A. Aliper, V. Aladinskiy, Z. Cao, S. Kong, X. Long, B. H. Man Liu, Y. Liu, V. Naumov, A. Shneyderman, I. V. Ozerov, J. Wang, F. W. Pun, D. A. Polykovskiy, C. Sun, M. Levitt, A. Aspuru-Guzik and A. Zhavoronkov, AlphaFold accelerates artificial intelligence powered drug discovery: efficient discovery of a novel CDK20 small molecule inhibitor, Chem. Sci., 2023, 14, 1443–1452 RSC.
- I. Loisios-Konstantinidis, R. Cristofoletti, N. Fotaki, D. B. Turner and J. Dressman, Establishing virtual bioequivalence and clinically relevant specifications using in vitro biorelevant dissolution testing and physiologically-based population pharmacokinetic modeling. case example: Naproxen, Eur. J. Pharm. Sci., 2020, 143, 105170 CrossRef CAS PubMed.
- J. Huang, W. Guo, F. Cheung, H. Y. Tan, N. Wang and Y. Feng, Integrating Network Pharmacology and Experimental Models to Investigate the Efficacy of Coptidis and Scutellaria Containing Huanglian Jiedu Decoction on Hepatocellular Carcinoma, Am. J. Chin. Med., 2020, 48, 161–182 CrossRef CAS PubMed.
- T. Wang, Z. Wu, L. Sun, W. Li, G. Liu and Y. Tang, A Computational Systems Pharmacology Approach to Investigate Molecular Mechanisms of Herbal Formula Tian-Ma-Gou-Teng-Yin for Treatment of Alzheimer's Disease, Front. Pharmacol, 2018, 9, 668 CrossRef PubMed.
- S. Yang, Y. Shen, W. Lu, Y. Yang, H. Wang, L. Li, C. Wu and G. Du, Evaluation and Identification of the Neuroprotective Compounds of Xiaoxuming Decoction by Machine Learning: A Novel Mode to Explore the Combination Rules in Traditional Chinese Medicine Prescription, BioMed Res. Int., 2019, 2019, 6847685 Search PubMed.
- T. Qin, L. Wu, Q. Hua, Z. Song, Y. Pan and T. Liu, Prediction of the mechanisms of action of Shenkang in chronic kidney disease: A network pharmacology study and experimental validation, J. Ethnopharmacol., 2020, 246, 112128 CrossRef CAS PubMed.
- Q. Xu, Q. Guo, C. X. Wang, S. Zhang, C. B. Wen, T. Sun, W. Peng, J. Chen and W. H. Li, Network differentiation: A computational method of pathogenesis diagnosis in traditional Chinese medicine based on systems science, Artif. Intell. Med., 2021, 118, 102134 CrossRef PubMed.
- Z. Zhou, B. Chen, S. Chen, M. Lin, Y. Chen, S. Jin, W. Chen and Y. Zhang, Applications of Network Pharmacology in Traditional Chinese Medicine Research, J. Evidence-Based Complementary Altern. Med., 2020, 2020, 1646905 CrossRef PubMed.
- Z. Ning, Y. Wang, L. Wang, L. Wu and J. Song, Research on a Vehicle-Mounted Intelligent TCM Syndrome Differentiation System Based on Deep Belief Network, IEEE Access, 2021, 9, 118019–118030 Search PubMed.
- G. Tian, K. Qian, X. Li, M. Sun, H. Jiang, W. Qiu, X. Xie, Z. Zhao, L. Huang, S. Luo, T. Guo, R. Cai, Z. Wang and B. W. Schuller, Can a Holistic View Facilitate the Development of Intelligent Traditional Chinese Medicine? A Survey, IEEE Trans. Comput. Soc. Syst., 2023, 10, 700–713 Search PubMed.
- Y. Jin, X. Ren and L. Yu, Artificial intelligence for the development and implementation guidelines for traditional Chinese medicine and integrated traditional Chinese and western medicine, TMR Mod. Herb. Med., 2021, 4, 15–28 CrossRef.
- Y. Zhou, Y. Zhang, X. Lian, F. Li, C. Wang, F. Zhu, Y. Qiu and Y. Chen, Therapeutic target database update 2022: facilitating drug discovery with enriched comparative data of targeted agents, Nucleic Acids Res., 2022, 50, D1398–D1407 CrossRef CAS PubMed.
- L. Li, L. Yang, L. Yang, C. He, Y. He, L. Chen, Q. Dong, H. Zhang, S. Chen and P. Li, Network pharmacology: a bright guiding light on the way to explore the personalized precise medication of traditional Chinese medicine, Chin. Med., 2023, 18, 146 CrossRef PubMed.
- B. Guo, C. Zhao, C. Zhang, Y. Xiao, G. Yan, L. Liu and H. Pan, Elucidation of the anti-inflammatory mechanism of Er Miao San by integrative approach of network pharmacology and experimental verification, Pharmacol. Res., 2022, 175, 106000 CrossRef CAS PubMed.
- Y. Xiong, L. Yan, X. Li, N. Duan, L. Lin, C. Lu and A. Lyu, Pattern classification in traditional Chinese medicine and precision medicine: a narrative review, Longhua Chin. Med., 2021, 4, 38 CrossRef.
- S. Wang, Y. Hou, X. Li, X. Meng, Y. Zhang and X. Wang, Practical Implementation of Artificial Intelligence-Based Deep Learning and Cloud Computing on the Application of Traditional Medicine and Western Medicine in the Diagnosis and Treatment of Rheumatoid Arthritis, Front. Pharmacol, 2021, 12, 765435 CrossRef CAS PubMed.
- L. Zhang, J. Song, L. Kong, T. Yuan, W. Li, W. Zhang, B. Hou, Y. Lu and G. Du, The strategies and techniques of drug discovery from natural products, Pharmacol. Ther., 2020, 216, 107686 CrossRef CAS PubMed.
-
H. Gong, M. Jin, L. Zhou, H. Chen, L. Zhang and Y. Liu, in 2021 IEEE International Conference on Bioinformatics and Biomedicine (BIBM), IEEE, Houston, TX, USA, 2021, pp. 3805–3811 Search PubMed.
- Z. Dou, Y. Xia, J. Zhang, Y. Li, Y. Zhang, L. Zhao, Z. Huang, H. Sun, L. Wu, D. Han and Y. Liu, Syndrome Differentiation and Treatment Regularity in Traditional Chinese Medicine for Type 2 Diabetes: A Text Mining Analysis, Front. Endocrinol., 2021, 12, 728032 CrossRef PubMed.
- X. Lai, X. Wang, Y. Hu, S. Su, W. Li and S. Li, Editorial: Network Pharmacology and Traditional Medicine, Front. Pharmacol, 2020, 11, 1194 CrossRef PubMed.
Footnote |
† These authors contributed equally to this work. |
|
This journal is © The Royal Society of Chemistry 2024 |
Click here to see how this site uses Cookies. View our privacy policy here.