DOI:
10.1039/D4TB00651H
(Paper)
J. Mater. Chem. B, 2024,
12, 7826-7836
Dopamine sensing by fluorescent carbon nanoparticles synthesized using artichoke extract†
Received
28th March 2024
, Accepted 8th July 2024
First published on 9th July 2024
Abstract
The practical and easy detection of dopamine levels in human fluids, such as urine and saliva, is of great interest due to the correlation of dopamine concentration with several diseases. In this work, the one-step synthesis of water-soluble carbon nanoparticles (CNPs), starting from artichoke extract, containing catechol groups, for the fluorescence sensing of dopamine is reported. Size, morphology, chemical composition and electronic structure of CNPs were elucidated by DLS, AFM, XPS, FT-IR, EDX and TEM analyses. Their optical properties were then explored by UV-vis and fluorescence measurements in water. The dopamine recognition properties of these CNPs were investigated in water through fluorescence measurements and we observed the progressive enhancement of the CNP emission intensity upon the progressive addition of dopamine, with a binding affinity value of log
K = 5.76 and a detection limit of 0.81 nM. Selectivity towards dopamine was tested over other interfering analytes commonly present in human saliva. Finally, in order to perform a solid point of care test, CNPs were adsorbed on a solid support and exposed to different concentrations of dopamine, thus observing a pseudo-linear response, using a smartphone as a detector. Therefore, the detection of dopamine in simulated human saliva was performed with excellent results, in terms of selectivity and a detection limit of 100 pM.
Introduction
Dopamine (DA, Fig. 1) is a catecholamine neurotransmitter playing a crucial role in the nervous system, participating in many emotional and physical body functions, such as the sleep-wake cycle, human feelings and behaviour, heart rate, kidney function, and many other physiological mechanisms.1,2 Under physiological conditions, the concentrations of DA are around 20 ng mL−1 in plasma,3 18.9 pg mL−1 in saliva4 and 0.2–1 mg mL−1 in human urine.5 Alteration of these values is often related to neurological dysfunctions or diseases.6,7 For these reasons, the fast and precise detection of DA in biological fluids is of great importance for the early diagnosis of nervous system-related disorders, such as Parkinson's and Alzheimer's diseases and schizophrenia.8–10 Due to the relevance of DA in the human body, several methods have been explored so far for its detection, including electrochemical detection,11 liquid chromatography (LC),12 capillary electrophoresis (CE),13 microdialysis coupled with high performance liquid chromatography (HPLC),14 fast scan cyclic voltammetry (CV),15 mass spectrometry (MS),16 Raman spectroscopy, etc.17,18 Although the above-listed techniques are all reliable, efficient, selective and sensitive, some of their limitations preclude the possibility of achieving fast and easy detection of DA, in particular, the sophisticated equipment requires specialized personnel and time-consuming sample preparation precluding the direct point-of-care (POC) sensing of DA in human fluids. To overcome these drawbacks, fluorescence-based detection systems have become the most promising alternatives, due to their cost effectiveness, real-time detection, ease of preparation, and ease of application, especially in solid devices.19–32 In this context, smartphone-based solid chemosensors have gained great interest as point-of-care and point-of-need platforms in healthcare. Smartphones are extremely easy-accessible tools, endowed with high-resolution digital cameras. When accompanied by an optically responsive solid receptor, they become accessible, easy-to-use point-of-care devices, with a wide application range.33–40
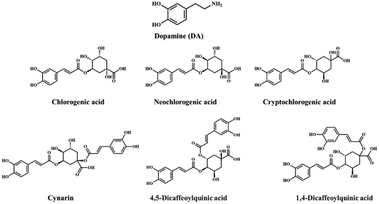 |
| Fig. 1 Chemical structures of dopamine (guest) and the main components of the artichoke extract. | |
Nanomaterials with optical properties have emerged in recent years as innovative materials in sensing applications.41,42 The driving force of the nanosensors is mainly their unique optical properties, in terms of absorption and emission of light, and the possibility of being functionalized with specific natural or synthetic receptors. Although the sensing of DA by nanomaterials can be performed by different methodologies, including electrochemical,43,44 optical detection is preferred due to its easy and fast read-out.
Among other fluorescent probes employed for the realization of solid sensors, carbon nanoparticles (CNPs) have emerged in the field of biomarker detection, due to their ease of synthesis, good biocompatibility, low cost, good water-solubility, good photostability, and remarkable optical properties.45 To date, the detection of DA using fluorescent CNPs has mainly been performed by using N-doped CNPs, obtained by adding urea to the carbonaceous source, during a solvothermal synthesis process.46 Another reported approach enables the post-functionalization of the CNPs after their synthesis, and this strategy affords new functional groups to the graphene-core of the NPs. However, these systems are mainly applied in solution, with limited use in point of care testing. To the best of our knowledge, only one literature report on a CNP-based sensor used as a point-of-care device for DA detection in plasma is available,47 while the detection of dopamine in human saliva, which could be the fastest and easiest way of screening, has not been reported. In this context, the development of a solid chemosensor containing fluorescent CNPs able to show fluorescence response to the presence of dopamine in saliva, is highly desirable, due to the easier sampling method compared to a blood sample. Moreover, the further simplification of the testing method is achievable by the use of a smartphone as the detector.
Due to the low DA concentration in human fluids, a practical sensor must be able to detect nanomolar concentration of DA. Many DA optical nanosensors have been developed in recent years;48 however, only a few of them have been realized in easy-to-use practical devices. In particular, gold nanoflowers containing the Tb3+ cation have been developed for fluorescence DA sensing, with a detection limit of 0.21 nM.49 Smartphones have also been used as detectors in combination with CuO nanoparticles, functionalized with maca extract, with a detection limit of 16.9 nM.50 Very recently, an attomolar detection limit has been achieved using an aptamer-based sensor through the use of a microfiber as a detector.51 A bacterial cellulose-based nanosensor has recently been developed for DA detection by using a smartphone as a detector, with a detection limit of 279 nM.52 A remarkably low detection (13.5 pM) limit has been lately reported, using mesoporous silica nanostructures containing o-phthalaldehyde and 2-mercapto ethanol.53 An impressive detection limit of 10−18 M has been recently obtained with gold nanoparticles, functionalized with MOF structures.54 However, these highly sensitive sensors show in some cases low portability or long synthetic steps, precluding their scalability and potential application in the field.
In this work we report the one-step synthesis of new CNPs, starting from artichoke extract powder containing chlorogenic acid and its derivatives as the carbonaceous sources for the formation of the graphitic core (Fig. 1).55,56
Chlorogenic acid and its derivatives were used as the starting materials for the CNP synthesis, and thus functional catechol groups (polyphenol) are covalently anchored to the CNP core, exploited as active groups for the non-covalent recognition of DA (Fig. 2), without any post-functionalization reaction after the synthesis of the nanoparticles. In particular, at the temperatures used during the synthesis (ca. 200 °C), sugar moieties should generate the CNP core, leading to formation of polyphenolic groups on the external shell.
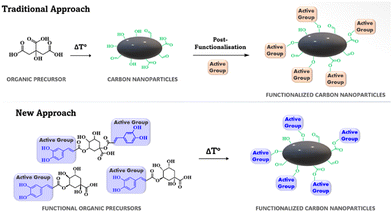 |
| Fig. 2 Schematic representation of two different synthetic approaches. | |
The new fluorescent carbon nanoparticles (CNPs_ART) were obtained by hydrothermal treatment of the artichoke extract. CNPs_ART have fully been characterized by DLS, AFM, XPS, IR EDX and TEM. DA sensing properties, including linearity, detection limits and selectivity, were studied by emission spectroscopy, both in solution and in the solid state. A solid prototype was tested for the DA sensing in an artificial saliva sample by using a smartphone as a detector. The excellent limit of detection (nM concentration), the high selectivity towards DA when compared to other common analytes contained in saliva and the efficient detection properties with artificial saliva pave the way for the realization of a real device for DA monitoring.
Materials and methods
Dopamine, adrenaline, testosterone, uric acid, creatine, glucose, sodium hydroxide, 2,2′-azobis(2-amidinopropane) dihydrochloride (AAPH), fluorescein, potassium persulfate (K2S2O8), gallic acid, 2,2-diphenyl-1-picrylhydrazyl (DPPH˙), quercetin, 2,2′-azino-di-(3-ethylbenzthiazoline sulfonic acid), ABTS˙+, and Trolox were purchased from MERCK and used without any further purification.
Synthesis of carbon nanoparticles
By means of the extraction procedure, artichoke extract powder was obtained from fresh artichoke leaves in water (leaves/water 1
:
3 ratio) at 90 °C, followed by resin purification. 7 g of the powder were heated up to 200 °C for two hours. After cooling down the heated powder, 10 mL of a NaOH 0.2 M solution were added until neutral pH was obtained (tested by means of litmus paper). The solution was filtered and then dialyzed (cutoff 11
000 Da). The solvent was removed under vacuum and a brown solid (CNPs_ART) was obtained.
XPS-EDX measurements
X-ray photoelectron spectra (XPS) were recorded at a 45° take-off angle relative to the surface sample holder, with a PHI 5000 Versa Probe II system (ULVAC-PHI, Inc., the base pressure of the main chamber was 1 × 10−8 Pa).57,58 Samples were excited with the monochromatized Al Kα X-ray radiation using a pass energy of 5.85 eV. The instrumental energy resolution was ≤0.5 eV. The XPS peak intensities were obtained after Shirley background subtraction. Spectral calibration was achieved by fixing the Ag 3d5/2 peak of a clean sample at 368.3 eV;59 this method fixed the C 1s peak of the adventitious carbon contamination at 285.0 eV.56,57 The atomic concentration analysis was performed by considering the relevant atomic sensitivity factors.60
Morphological characterization (DLSL, AFM, and TEM)
Size and electrophoresis measurements were performed with a Zetasizer Nano ZS (Malvern Instrument, UK), equipped with a He–Ne laser at a wavelength of 632.8 nm. DLS measurements were run on disposable cuvettes by using optimal measurement times and laser attenuation settings, assuming a viscosity of 0.887 mPa s and a refractive index 1.33 for the solutions. All samples were measured at least 3 times at 25 °C using non-invasive backscatter detection at a scattering angle of 173°. CNPs_ART solutions (1 mg mL−1) were filtered through a 0.22 μm filter before the beginning of each experiment. The electrophoretic mobility of every sample was measured three times and reported as mean ± standard deviation. The z-potential (ζ) at pH 6.5 was determined using the electrophoretic mobility (u) values according to the Helmholtz-Smoluchowski equation: ζ = ηu/ε, where η is the viscosity of solution and ε is the dielectric permittivity of water.
The morphological characterization was performed using a nanoscope IIIA-MultiMode atomic force microscope (AFM), Digital Instruments (Santa Barbara, CA, USA) used in tapping mode. Images were recorded at a scan rate of 1 Hz and 512 × 512 pixels per image using Tap 300 G silicon probes (Budget sensors) mounted on cantilevers with a nominal force constant of 40 N m−1 and a resonant frequency of 300 kHz. CNP transmission electron microscopy (TEM) characterization was performed on a Jeol JEM-ARM200F at 200 kV in parallel beam mode (C-TEM). The microscope is equipped with a cold field emission gun (CFEG), which has a 0.27 eV energy spread and a CEOS Cescor probe aberration corrector. For C-TEM imaging, a Gatan Rio 2 CMOS camera with a large field of view of 10 megapixels (4k × 4k) was used. The sample for TEM characterization was prepared by drop casting a few microliters of aqueous solutions containing CNPs over a TEM grid supporting an ultra-thin carbon film (nominal thickness < 3 nm).
Determination of the total phenolic content (TPC)
The TPC of CNPs_ART was spectrophotometrically determined.61 The CNPs were suspended in water (CNPs_ART 1.7 mg mL−1) and sonicated for 5 min. Gallic acid was employed as a reference compound to obtain a calibration curve (R2 = 0.9991) with concentrations of solutions ranging from 0.05 mg mL−1 to 0.40 mg mL−1. The resulting supernatant from CNPs and the solutions of gallic acid (25 μL) were mixed in a 96-well microplate (using an Agilent BioTek Synergy H1 Multimode Reader and the Gen5 software) with Folin–Ciocalteu solution (previously diluted 1
:
10; 100 μL). Then, a 1 M Na2CO3 solution (100 μL) was added, the plate was mechanically mixed for 1 min and then incubated in the dark for 1.5 h at room temperature. Optical density (OD) was read at 765 nm. Results were reported as milligrams of gallic acid equivalents per gram of CNPs (mg GAE per g). All measurements were carried out in quintuplicate and reported as mean ± SD.
Antioxidant activity evaluation
CNPs_ART were suspended in water (2.14 mg mL−1) and sonicated for 5 min. The supernatant was employed in the antioxidant assays.
DPPH radical scavenging activity
The bleaching of the purple-coloured radical 2,2-diphenyl-1-picrylhydrazyl (DPPH˙) was employed to measure the radical-scavenging ability of CNPs.61 Briefly, a freshly prepared DPPH˙ solution (190 μM; 200 μL) was mixed with the samples (20 μL). The mixtures were incubated at 25 °C for 1.5 h in the dark. Trolox (in the range of 40–800 μM) was employed as a reference standard to obtain a calibration curve for data evaluation (R2 = 0.9992). Trolox was assayed under the same conditions as those of samples. Quercetin was used as a positive control (0.05 mg mL−1). A blank was obtained by replacing the tested samples with the same amount of water. OD was acquired at 515 nm and the antiradical activity of the extracts was expressed as micromoles of Trolox equivalents (TE) per gram of CNPs (μmol TE per g).
ABTS radical scavenging activity
The assay was performed as previously reported.62 Briefly, the radical cation 2,2′-azino-di-(3-ethylbenzthiazoline sulfonic acid), ABTS˙+, was generated by mixing 7 mM ABTS with 2.45 mM potassium persulfate (K2S2O8); this solution was stored in the dark and at room temperature for 12 h prior utilization. ABTS˙+ was diluted in ethanol to a final concentration of 70 μM and 200 μL were mixed with the CNPs (20 μL) or quercetin (0.04 mg mL−1), which was employed as a positive control. Blank solutions were obtained by replacing the samples with the same volume of water. The mixtures were incubated for 6 min and the OD was measured at 734 nm. A calibration curve of Trolox was obtained by assaying different solutions (80–800 μM) under the same conditions as those of samples. The results were evaluated by linear regression with the standard calibration curve (R2 = 0.9998) and expressed as micromoles of TE per gram of CNPs.
Oxygen radical absorbance capacity (ORAC)
The antioxidant activity toward the peroxyl radical (ROO˙) was determined by following the ORAC method previously described.63 Briefly, a fluorescein solution freshly prepared in PBS (112 nM, pH 7.4, 150 μL) was added to each well, and then 25 μL of CNPs were added and finally 25 μL of 2,2′-azobis(2-amidinopropane) dihydrochloride (AAPH) solution (153 mM) were added. Trolox was assayed (1.5–0.2 μM) under the same conditions as those of samples and it has been employed as a standard to build a calibration curve (R2 = 0.9999). Gallic acid (0.37 mg mL−1) was employed as a positive control. Fluorescence was measured at 37 °C every minute for 120 min (λex: 485 nm; λem: 528 nm). A blank assay using phosphate buffer instead of the sample was performed. The ORAC values were calculated using the area under the fluorescence decay curves and expressed as micromoles of TE per gram of CNPs.
UV-vis measurements
The UV-vis absorption spectrum was acquired using a JASCO V-560 UV-vis spectrophotometer equipped with a 1 cm path-length cell for the UV-vis measurements (resolution 0.1 nm). A stock solution of CNPs_ART (1 mg mL−1) was prepared in MilliQ water (pH 7). The measurement was performed in a 1 × 1 cm quartz cuvette using a 0.05 mg mL−1 solution of the sample.
Fluorescence measurements
Fluorescence measurements were carried out using a Cary eclipse fluorescence spectrophotometer with a resolution of 0.5 nm, at room temperature. The emission was recorded at 90° with respect to the exciting line beam using 5
:
5 slit-widths for all measurements. Two stock solutions of the probe (CNPs_ART 1.0 mg mL−1) and dopamine (1.0 mM) in MilliQ water were prepared (pH 7). From these, different solutions with different receptor/guest ratios were prepared (in the cuvette for fluorescence analysis, the probe concentration was fixed at 0.01 mg mL−1, while increased amounts of dopamine were added in the 0–12 μM range). Emission spectra were recorded at 25 °C. The apparent binding affinity values were estimated using HypSpec (version 1.1.33),64 a software designed to extract equilibrium constants from potentiometric and/or spectrophotometric titration data. HypSpec starts with an assumed complex formation scheme and uses a least-squares approach to derive the spectra of the complexes and the stability constants. The χ2 test (chi-square) was applied, where the residuals follow a normal distribution (for a distribution approximately normal, the χ2 test value is around 12 or less). In all cases, χ2 values ≤10 were found, as obtained by 3 independent measurement sets. The limit of detection (LOD) was calculated using the calibration curve method by means of the equation 3σ/K (where σ is the standard deviation of the blank and K is the slope of the calibration curve).
FT-IR analysis of the complex CNPs_ART@DA
FT-IR spectra were acquired by using a PerkinElmer Spectrum One spectrophotometer. For the analysis of the complex, two stock solutions (1 mL), one containing DA (1 × 10−2 M in water) and the other containing CNPs_ART (2 mg mL−1 in water), were mixed, in order to promote the formation of the host–guest complex. Afterwards, 100 μL of this solution were dropped onto a transparent silica slide, the solvent was removed by nitrogen flux, and the FT-IR spectrum was recorded. The same procedure was previously performed with a solution containing DA only (1 × 10−2 M in water).
NMR experiments
The NMR experiments were carried out at 27 °C on a Varian UNITY Inova 500 MHz spectrometer (1H at 499.88 MHz and 13C NMR at 125.7 MHz) equipped with a pulse field gradient module (Z axis) and a tunable 5 mm Varian inverse detection probe (ID-PFG). Two stock solutions of the fluorescent probe (CNPs_ART 1.75 mg mL−1 in D2O, pD 7) and the analyte (DA 1 mM in D2O, pD 7) were prepared. NMR spectra of dopamine only (700 μL, 1 mM, D2O, pD 7) and the dopamine/CNPs_ART (700 μL, D2O) at different ratios were recorded, as reported in the ESI† ((a) DA 1 mM; (b) DA 1 mM + CNPs_ART 0.0625 mg mL−1; (c) DA 1 mM + CNPs_ART 0.125 mg mL−1; (d) DA 1 mM + CNPs_ART 0.187 mg mL−1; (e) DA 1 mM + CNPs_ART 0.250 mg mL−1).
Test strip
In a 3 × 10 cm aluminum oxide foil, spots of the nanoparticle solutions (1.5 μL in water, pH 7) were cast at three different concentrations: 1, 0.5, and 0.05 mg mL−1. The so-obtained solid receptor system was exposed to UV light irradiation, by using a dark chamber and a UV lamp working at 365 nm. The images of the spots at different concentrations were collected by using a smartphone camera (I-phone 9), before and after exposure to DA solution (cast using a polycellulose filter) at different concentrations (1 × 10−3 M; 1 × 10−7 M and 1 × 10−9 M). The pictures collected were then processed by the Fiji software65 and converted into RGB channel values, in order to provide the intensity value on a grey scale, following the equation: G = (R value + G value + B value)/3, thus obtaining a single value for each pixel. Normalized grey channel intensity values (considered as G − G0, where G is the measured intensity and G0 is the intensity of the blank) were employed to relate the solid array response to DA concentration (the results reported were obtained by three independent measurements). The same procedure was carried out for the evaluation of the linear response expressed in normalized grey channel intensity values of CNPs_ART on a solid support (0.5 mg mL−1), exposed to DA solution in the 1 × 10−10 M to 1 × 10−3 M concentration range.
After the evaporation of water, the response of CNPs_ART on a solid support is instantaneous, according to fluorescence measurements.
Computational methods
Ab initio and density functional calculations were performed using the Gaussia09 program package. Optimization of all involved systems was performed at the B3LYP/6-31G(d,p) level of theory in the gas phase. All structures were subjected to a full conformational search to determine the absolute minimum. Frequencies were calculated and checked to make sure that all of them were positive and no imaginary frequencies were present. Gaussview software has been used as a graphic interface to visualize the optimized structures. Zero-point energy (ZPE) was included in each result.
Results and discussion
CNP synthesis and characterization
CNPs_ART were obtained by a slightly modified hydrothermal decomposition procedure,66,67 starting from freeze-dried extract of artichoke as the carbonaceous source. In particular, the powder was heated up to its melting point at 200 °C. The subsequent treatment with NaOH solution, centrifugation and dialysis afforded CNPs as brown crystals. While traditional carbonization procedures often involve higher temperatures, our work demonstrates that a lower-temperature and use of artichoke extract are effective for synthesizing CNPs well-suited for dopamine sensing. This approach offers the potential benefits of preserving polyphenolic groups and controlling CNP properties for enhanced sensitivity.
The size and morphology of CNPs_ART were firstly explored by means of AFM imaging (Fig. 3a) performed on a dry sample, indicating the presence of several flat irregularly shaped particles. These particles show a broad thickness distribution (1.1 ± 0.8 nm) as revealed by the section analysis (Fig. 3b).
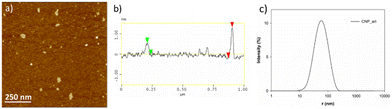 |
| Fig. 3 (a) 1 × 1 μm2 AFM height image of CNPs_ART, the height scale is 5 nm; (b) exemplificative cross-sections, used for the determination of the nanoparticle height; (c) particle size distribution of CNPs_ART (1 mg mL−1) in water at 25 °C. | |
Afterwards, the particle size, zeta potential, and polydispersity index (PDI) were analyzed as important parameters associated with the quality, stability, and other macroscopic properties of nanoparticles. The results of DLS of CNPs_ART (Fig. 3c) showed a single mode distribution with a z-average hydrodynamic radius and PDI of 50 ± 1 nm and 0.21 ± 0.02, respectively. The PDI value of prepared nanoparticles was in the acceptable range for solid nanoparticulate systems, indicating a homogeneous suspension and well-controlled particle size with a narrow dispersity.68 The zeta potential is generally used for predicting the stability and the possibility of aggregation of particles in suspension.69 In particular, CNPs_ART showed a zeta-potential value of −25 ± 2 mV. The negative zeta value might be related to the presence of acidic polyphenol groups and carboxylic acid groups, assuming that the predominant ions in the electric double layer up to the slipping plane are similar compared to the surface of the particle itself.
Fig. 4a shows a C-TEM image of CNPs_ART. Its structure consists of a crystalline scaffold formed by several graphene layers folded to form a closed shell. Externally, the CNP is coated by a layer of amorphous carbon. Fig. 4b is a magnified C-TEM image of the area enclosed in the black box in Fig. 4a; the lower panel shows the relative 2-D integrated intensity profile. Fig. 4b shows in detail the CNP's multilayer structure, formed by more than 20 graphene sheets. The CNP's rim is covered by an amorphous carbon layer having a thickness of about 4 nm. Fig. 4c shows the fast Fourier transform (FFT) image of the C-TEM image shown in Fig. 4a, showing several spots that originate from the atomic planes of the CNP and highlighting its polycrystalline nature. The (002) planes, which lie along the stacking direction of graphene layers, have a measured distance of 0.339 ± 0.03 nm.
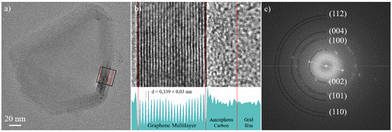 |
| Fig. 4 (a) C-TEM image of CNPs_ART; (b) C-TEM magnified image of the black box in (a) with its relative intensity profile (lower panel), showing the multilayer structure of CNPs_ART with an interplanar distance of 0.339 ± 0.03 nm and the amorphous carbon coating on its surface; (c) FFT image of (a) with indexed CNP's atomic planes. | |
Planes (100), (101), (110) and (112) show characteristic distances of 0.211 nm, 0.203 nm, 0.121 nm and 0.115 nm, respectively. The electronic structure of CNPs_ART was investigated by X-ray photoelectron spectroscopy. This technique gives information on the oxidation states and on the chemical environment of the studied elements and allows estimation of the surface elemental composition, once the relevant atomic sensitivity factors have been considered.58
Fig. 5a shows the high-resolution XP spectrum of the CNPs in the C 1s binding energy region. According to the related literature, the two signals at 285.0 and 287.6 eV are due to the aliphatic/aromatic carbon and to the –C
O groups, respectively.58 The overall carbon signal accounts for a 40.3% atomic concentration.
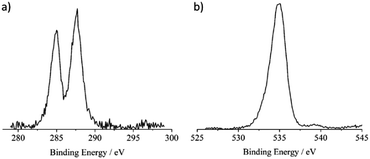 |
| Fig. 5 (a) Al Kα excited XPS of the CNPs in the C 1s binding energy region. (b) Al Kα excited XPS of the CNPs in the O 1s binding energy region. | |
Fig. 5b shows the high-resolution XP spectrum of the CNPs in the O 1s binding energy region. The main peak at 534.9 eV agrees with the presence of abundant water on the CNP surface.70,71
On the lower binding energy side of this main peak an asymmetry whose flex is at about 533 eV is evident and is consistent with the –C
O group. The oxygen signal accounts for a 59.0% atomic concentration.
Fig. S1 (ESI†) shows the high-resolution XP spectrum of the CNPs in the N 1s binding energy region and shows the absence of any nitrogen signal.72 Fig. S2–S4 (ESI†) show the high-resolution XP spectra of the CNPs in the Mn, Fe and Co 2p binding energy regions. While no Mn 2p nor Co 2p signals were detected, a very low Fe 2p content (0.7% of the overall CNP atomic concentration) was observed, probably due to the natural origin of these nanoparticles.
In order to investigate the functional groups present in the natural carbon nanoparticles, FT-IR analysis was performed. The FT-IR spectrum of CNPs_ART displays a broad intense band at ∼3400 cm−1, corresponding to free OH stretching vibration. The C–H vibration is observed at ∼2920 cm−1, and the C
C vibration, ascribed to the graphitic core (Fig. S5, ESI†), appears in the 1630–1600 cm−1 range. The spectrum of CNPs_ART also shows the typical pattern of carboxyl groups, as can be observed from the C
O vibration band at 1726 cm−1. Moreover, the intense band at 1270 cm−1 (catecholic O–H bending vibration) clearly suggests the presence of catechol groups.
The optical properties of CNPs_ART were investigated by recording its UV-vis and fluorescence spectra in water at pH 7 (Fig. 6a and b, respectively). The absorption spectrum of CNPs_ART displays an absorption band centered at 285 nm, ascribed to the π–π* electronic transition of the conjugated C
C domains of the aromatic carbon cores. The shoulder at 325 nm is related to the n–π* transition of the carbonyl- and carboxyl-functional groups on the surface.73
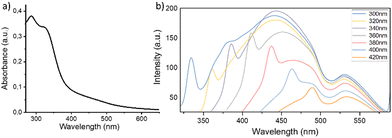 |
| Fig. 6 (a) UV-vis spectrum of CNPs_ART (0.05 mg mL−1 in MilliQ water). (b) Fluorescence emission spectra of CNPs_ART (0.05 mg mL−1 in MilliQ water, pH 7) at different excitation wavelengths (from 300 to 420 nm, see the inset). | |
The emission spectra of a 0.05 mg mL−1 CNPs_ART water solution (pH 7), recorded at different excitation wavelengths, show an emission band dependent on the excitation wavelength employed, and in particular it shifts from 430 nm to 470 nm upon increasing the excitation wavelength from 300 to 420 nm. In addition, an emission band fixed at 530 nm can be found in all spectra. These peculiar photoluminescence properties suggest two sources of fluorescence emission within the same nanosystem. In particular, the excitation-dependent photoluminescence is due to the graphene core,74,75 while the excitation-independent emission is more likely due to molecular species bound to the graphitic core. These data, supported by the above-mentioned FT-IR profile and TEM morphology studies, suggest the presence of catechol groups, covalently linked to the graphitic carbon network.
To support this hypothesis, the total phenolic content (TPC) of CNPs_ART was determined as detailed in the experimental section and the data are reported in Table 1. Despite the treatment being carried out with the extract, the resulting CNPs still show a good content of phenolic compounds (89.7 mg GAE per g of CNPs). The antioxidant activity was assessed using three methodologies, where the involved reactions can occur by hydrogen atom transfer (HAT) processes as for ORAC or single electron transfer (SET) processes for DPPH and ABTS. The data highlighted the good antioxidant activity of CNPs_ART considering the quite similar results obtained for quercetin and gallic acid, naturally occurring polyphenols frequently employed as positive references. Moreover, the values obtained for the antioxidant activity and TPC indicated that the phenolic residues within the CNPs significantly contribute to their antioxidant activity.
Table 1 Total phenolic content and antioxidant activity of CNPs_ART
Samples |
TPC (GAE ± SD)a |
Antioxidant activity |
DPPH ± SDb |
ABTS ± SDb |
ORAC ± SDb |
Results are the average of 4 replicates. GAE = gallic acid equivalents (expressed as milligrams per gram of CNPs). Expressed as Trolox equivalents, TE (micromoles per gram of CNPs). Quercetin and gallic acid have been assayed as positive references, expressed as Trolox equivalent TEs (μM). |
CNPs_ART |
89.7 ± 4.2 |
13.5 ± 0.8 |
9.5 ± 1.9 |
1.9 ± 0.1 |
Quercetinc |
— |
3.7 ± 0.8c |
2.7 ± 0.3c |
5.1 ± 0.7c |
Gallic acid |
— |
1.85 ± 0.2c |
3.7 ± 0.1c |
7.4 ± 0.2c |
Dopamine sensing
Due to the observed remarkable fluorescence, stable also after 4 hours (see ESI,† Fig. S9), the recognition properties of CNPs_ART towards DA in water were studied by fluorescence titrations, by observing the change in the fluorescence emission of CNPs_ART 0.01 mg mL−1 solution in water (pH 7), upon the progressive addition of DA in the 0–12 μM concentration range. Interestingly, the fluorescence spectrum of CNPs_ART shows an increase of the emission intensity upon the addition of DA (Fig. 7), displaying a binding constant value of log
K = 5.76 ± 0.02 (calculated using HypSpec software),40,64 which is the highest reported in the literature concerning the sensing of DA by carbon nanoparticles. Notably the limit of detection of 0.83 nM, calculated using the calibration curve method, is in the range of DA concentrations in human fluids (0.11 μM in plasma,3 100 pM in saliva4 and 1–5.3 mM in human urine5).
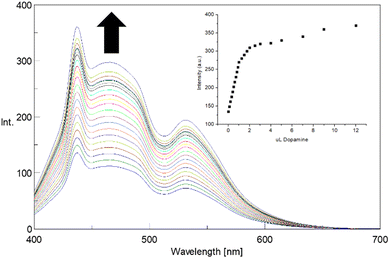 |
| Fig. 7 Fluorescence emission spectra of CNPs_ART solution (0.01 mg mL−1 in MilliQ water, λex = 380 nm) upon addition of DA (0–12 μM). Inset shows the calibration curve. | |
Optical recognition studies highlighted a remarkable affinity of CNPs_ART towards DA. To shed light on the recognition mechanism, 1H NMR titration of a DA solution (1 × 10−3 M in D2O) was performed, upon the progressive addition of CNPs_ART.
The 1H NMR spectrum of DA shows the typical pattern of the aromatic protons in the region 6.75–7.95 ppm and of two aliphatic methylene protons as two triplets at 3.25 and 2.89 ppm, respectively. Interestingly, upon the progressive addition of CNPs_ART, the aromatic signals undergo a slight downfield shift (see Fig. S7, ESI†), thus suggesting that dopamine is placed at the rim of the graphene core of the nanoparticles, being subjected to the ring current effect.
To further elucidate the recognition mechanism, FT-IR spectra of DA and the DA@CNPs_ART complex were recorded (Fig. 8).
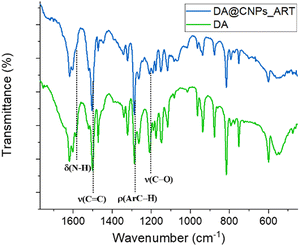 |
| Fig. 8 FT-IR spectra of DA and the DA@CNPs_ART complex. | |
The DA spectrum shows the diagnostic signals, including the stretching vibration peak of the catechol structure at a wavenumber of 1287 cm−1, the stretching vibration peak of the C
C bond in the dopamine benzene ring at around 1616 cm−1 (Fig. 8), and the N–H bond stretching vibration at 3157 cm−1 and 3332 cm−1 (see Fig. S8, ESI†).76
Comparing the FT-IR spectra of DA@CNPs_ART and neat DA (Fig. 8), except for the peaks at 1616, 1502 and 1287 cm−1, relative to the aromatic C
C/C–H bond stretching and C–H rocking vibrational modes, respectively, which do not show any change, almost all the signals are either absent or less intense. This may exclude, in accordance with NMR data, stacking interaction involving the aromatic moiety of the guest and the graphene core of the nanoparticles. Moreover, the C–O stretching at 1205 cm−1 in the spectrum of DA disappears in the spectrum of the complex, thus indicating the involvement of at least one hydroxyl group in the interaction with the nanoparticles. In addition, the peak at 1584 cm−1, relative to the scissoring vibrational mode of the NH2 group of DA, is no more visible in the spectrum of the complex. This last datum, together with the shift at lower frequency of the NH2 stretching peak at 3250 cm−1 (see Fig. S8, ESI†), is consistent with the formation of hydrogen bonds involving also the amino group of dopamine, meaning the formation of hydrogen bonds between either the hydroxyl or the amine group of dopamine and hydroxyl groups of the carbon nanoparticles.
A computational study has been performed to further highlight the interaction(s) between DA and CNPs_ART at the B3LYP/6-31G(d,p) level of theory in the gas phase.77–79 A graphene surface of 1.1 × 1.3 nm was functionalized with two catechol units, far from each other by 0.3 nm (see Fig. S9, ESI†). We want to underline that the proposed model system (host nanoparticles) and the relative distances between the functional groups are in agreement with the XPS and TEM analyses related to the pristine CNPs_ART sample. Multiple hydrogen bonds (HBs) have been found in the host–guest complex between DA and the functionalized CNPs_ART. In more detail, the OH groups of the functionalized CPNs_ART form three HBs with the DA's catechol unit, while the terminal amino group of DA is involved in two HBs, as suggested by the NMR and IR analyses. Moreover, the entire host–guest complex is further stabilized by an intramolecular HB between the OH groups of the host's catechol unit, bringing the total number of HBs in the host–guest complex to six (Fig. S10, ESI†). The HBs formed with the DA's catechol unit are relatively shorter (1.87–1.99 Å) compared to the ones formed with the DA's terminal amino group (1.79–2.51 Å), highlighting stronger interactions with the hydroxyl groups compared to the amino group of DA (Fig. S10, ESI†). A high complexation energy (Ecomplex) for the DA@CNPs_ART complex is hypothesized due to the high number of HBs involved in the complex formation. Indeed, a complexation energy of 19.8 kcal mol−1 has been calculated for the DA@CNPs_ART complex, which is 13.9 kcal mol−1 higher than the complexation energy between two water molecules, used as a reference model (Table S1, ESI†). This value is in agreement with the experimental high binding constant found for the DA@CNPs_ART complex.
Detection of DA for biomedical purpose requires a highly selective response; for this reason, the selectivity of CNPs_ART towards DA was investigated by observing the response in the fluorescence emission spectrum of CNPs_ART solution (0.05 mg mL−1 in water) upon addition of several interfering analytes commonly present in human saliva.4 In particular, we measured the emission of CNPs_ART after the selective addition of 1 μM adrenaline, testosterone, uric acid, creatine, and glucose and 0.1 μM DA. The results reported in Fig. 9 show a remarkable selectivity of the nanoparticles towards DA over the other analytes, also in large excess (10 times), in particular in terms of turn-on of the emission. Taking into account all these results obtained in solution, CNPs_ART were tested as a probe for DA sensing also in the solid phase.
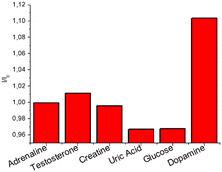 |
| Fig. 9 Selectivity test: normalized emission response (I/I0, where I and I0 are the emission intensities of the nanoparticles at 437 nm, λexc 380 nm, after and before the addition of the analytes, respectively) of CNPs_ART towards dopamine (0.1 μM in water) over interfering analytes (1 μM in water, pH 7). | |
DA sensing by strip test
As mentioned, the fast detection of DA in human fluids would be helpful for the development of point-of-care devices for biomedical applications. In addition, the possibility of easy and fast read-out determination of the DA level in saliva by using a simple smartphone camera would represent a great advantage. Indeed a smartphone, normally equipped with a high-resolution digital camera and endowed with data processing software, is an extremely accessible tool with great potential use in the biomedical field. To this aim, we selected an appropriate inert support (aluminium oxide layer) to perform the strip test, by using a black chamber to acquire the image under UV excitation at 365 nm. In particular, the smartphone is located on the top of the black chamber in a fixed position. Images acquired are processed using Fiji software, thus obtaining RGB and gray channel values (see the Materials and methods section).
Firstly, we optimized the CNPs_ART concentration to drop onto the solid support, demonstrating also the absence of mechanochromism (see ESI,† Fig. S10). In particular, Fig. 10a shows the results obtained with three different concentrations of nanoparticles (1, 0.5 and 0.05 mg mL−1 in water, respectively) at different concentrations of DA (from 1 nM to 1 mM), indicating the optimal sensing response of 0.5 mg mL−1 CNPs_ART. Indeed, at this concentration, we observed a linear progressive increase of the response upon increasing the amount of dopamine. Furthermore, Fig. 10b shows a linear sensing response to DA in the 10−10–10−3 M range, with an experimental detection limit of 0.1 nM, which is close to the physiological DA concentration in saliva.4
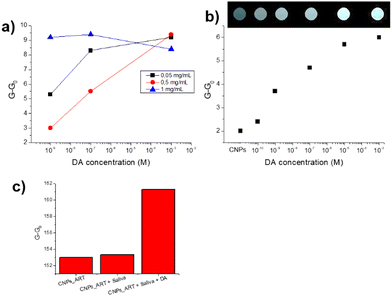 |
| Fig. 10 (a) Optical response expressed in normalised grey channel intensity values (G and G0 are the gray channel values after and before exposure to the analyte, respectively) of CNPs_ART on a solid support at three different concentrations (0.05, 0.5 and 1 mg mL−1 in water, respectively), exposed to three concentrations of DA (1 × 10−3 M, 1 × 10−7 M and 1 × 10−9 M in water, pH 7). (b) Optical response expressed in normalised grey channel intensity values of CNPs_ART on a solid support (0.5 mg mL−1), exposed to DA solution in a concentration range of 1 × 10−10 M to 1 × 10−3 M. (c) Selectivity test: variation of grey channel intensity values of CNPs_ART in the presence of water (control), artificial saliva containing uric acid (20 μM), adrenaline (0.1 nM), testosterone (0.1 pM), glucose (72 μM), creatinine (1 μM) and some metal cations (Mg2+, Ca2+, Mn2+, Fe2+, Cu2+, and Zn2+)26 and artificial saliva containing 0.1 nM DA. | |
The strip can be restored by simple washing with chloroform, in which DA (dropped onto the solid support) is soluble, while CNPs_ART are not (see ESI,† Fig. S11). In particular, we demonstrated the possibility of reusing the device for 5 cycles, with a slight loss of efficiency.
In addition, DA sensing was performed in an artificial saliva sample,25 containing typical analytes present in real human saliva at the physiological concentration, specifically: uric acid 20 μM, adrenaline 0.1 nM, testosterone 0.1 pM, glucose 72 μM, creatine 1 μM and some metal cations. In particular, Fig. 10c shows the CNPs_ART response to the as-prepared artificial saliva and to the artificial saliva containing 0.1 nM DA. We observed a slight increase of the gray channel intensity in the presence of simple saliva, while a strong increase of the response can be detected in the presence of 0.1 nM DA. This result suggests that CNPs_ART can detect DA at physiological concentrations also in the presence of the other analytes commonly present in saliva.
Table 2 reports the comparison of LOD, detection methods and matrix data between our device and the other nanosystems used for DA sensing. In particular, our sensor device detects DA at lower concentration values compared to the other sensors able to detect DA in urine.50,52 Sensors able to detect lower DA concentrations53,54 have not yet been used with practical samples.
Table 2 Comparison between our work and other nanosystems used for DA sensing
Ref. |
LOD |
Detection method |
Matrix/source |
47
|
2.7 nM |
Fluorescence |
Human cervical carcinoma |
49
|
0.21 nM |
Fluorescence |
Human serum samples |
50
|
16.9 nM |
Smartphone |
Human urine |
51
|
1 mM |
Microfiber |
Extracellular DA levels released by the nervous system |
52
|
279 nM |
Smartphone |
Real human urine, blood and serum |
53
|
13.5 pM |
UV-vis |
Real time released DA from PC12 cells |
54
|
10−18 M |
Optical fiber |
— |
This work |
100 pM |
Smartphone |
Artificial saliva |
Conclusions
Real time and easy monitoring of dopamine concentrations in human fluids is of pivotal importance, due to the correlation between dopamine and many important diseases. In this context, a new fluorescent nanosensor based on carbon nanoparticles obtained in a single synthetic step using artichoke extract has been reported here.
The size and morphology of these nanoparticles have been fully characterized by DLS, AFM, XPS, FT-IR, EDX and TEM analyses. Dopamine sensing properties were explored in water through fluorescence spectroscopy, obtaining a turn-on of the emission, with a binding affinity value of log
K = 5.76 and a detection limit of 0.81 nM. We demonstrated the selectivity of CNPs_ART towards dopamine over other important interferents, commonly present in human fluids. Test strip experiments demonstrated the possibility of using this prototype in real life. In particular, we detected 0.1 nM–1 mM concentrations of dopamine on a solid support by using a smartphone as a detector. In addition, our device recognizes dopamine also in artificial saliva, in the presence of common analytes contained in the human samples. This work paves the way for the realization of practical point-of-care devices able to self-monitor dopamine in human fluids, such as urine and saliva.
Author contributions
The manuscript was written through contributions of all authors. All authors have given approval to the final version of the manuscript. Conceptualization: R. Puglisi, N. Tuccitto, V. Zaccaria, A. Pappalardo, G. Trusso Sfrazzetto; validation: A. Gulino, G. Li Destri, G. Nicotra, V. Muccilli; formal analysis: G. Sfuncia, G. Nicotra, R. Puglisi, L. Mancuso, R. Santonocito, F. Pappalardo, R. Ruffino, N. Cardullo, M. Petroselli; investigation: L. Mancuso, F. Pappalardo, R. Santonocito, V. Oliveri, R. Ruffino, N. Cardullo, G. Sfuncia, A. Pappalardo M. Petroselli; data curation: A. Gulino, V. Oliveri, G. Li Destri, V. Muccilli, G. Nicotra, V. Zaccaria, M. Petroselli; writing – original draft: R. Puglisi, G. Trusso Sfrazzetto; and supervision: R. Puglisi, G. Trusso Sfrazzetto.
Data availability
Compounds and original data are available from the authors upon request.
Conflicts of interest
There are no conflicts to declare.
Acknowledgements
The authors thank the University of Catania for the support. This work has been partially funded by the European Union (NextGeneration EU), through the MUR-PNRR project SAMOTHRACE (ECS00000022). MP thanks Dr Corrado Bacchiocchi at the University of Camerino for the technical support.
References
- Y. Leng, K. Xie, L. Ye, G. Li, Z. Lu and J. He, Talanta, 2015, 139, 89–95 CrossRef CAS PubMed.
- Y. Zhang, S. Qi, Z. Liu, Y. Shi, W. Yue and C. Yi, J. Mater. Sci. Eng., 2016, 61, 207–213 CrossRef CAS.
- D. Türkmen, M. Bakhshpour, I. Göktürk, S. Aşır, F. Yılmaz and A. Denizli, New J. Chem., 2021, 45, 18296–18306 RSC.
- T. Okumura, Y. Nakajima, M. Matsuoka and T. Takamatsu, J. Chromatogr., 1997, 694, 305–316 CrossRef CAS PubMed.
- D. Ji, Z. Liu, L. Liu, S. S. Low, Y. Lu, X. Yu, L. Zhu, C. Li and Q. Liu, Biosens. Bioelectron., 2018, 119, 55–62 CrossRef CAS PubMed.
- J. Zhou, W. Wang, P. Yu, E. Xiong, X. Zhang and J. Chen, RSC Adv., 2014, 4, 52250–52255 RSC.
- B. R. Li, Y. J. Hsieh, Y. X. Chen, Y. T. Chung, C. Y. Pan and Y. T. Chen, J. Am. Chem. Soc., 2013, 135, 16034–16037 CrossRef CAS.
- M. Levite, Acta Physiol., 2015, 216, 42–89 CrossRef PubMed.
- O. Howes, R. McCutcheon and J. Stone, J. Psychopharmacol., 2015, 29, 97–115 CrossRef PubMed.
- A. Meyer-Lindenberg, R. S. Miletich, P. D. Kohn, G. Esposito, R. E. Carson, M. Quarantelli, D. R. Weinberger and K. F. Berman, Nat. Neurosci., 2002, 5, 267–271 CrossRef CAS.
- X. Sun, L. Zhang, X. Zhang, X. Liu, J. Jian, D. Kong, D. Zeng, H. Yuan and S. Feng, Biosens. Bioelectron., 2020, 153, 112045 CrossRef CAS PubMed.
- K. Syslová, L. Rambousek, M. Kuzma, V. Najmanová, V. Bubeníková-Valešová, R. Šlamberová and P. Kačer, J. Chromatograph. A, 2011, 1218, 3382–3391 CrossRef PubMed.
- B. Claude, R. Nehmé and P. Morin, Anal. Chim. Acta, 2011, 699, 242–248 CrossRef CAS.
- T. Yoshitake, J. Kehr, K. Todoroki, H. Nohta and M. Yamaguchi, Biomed. Chromatogr., 2005, 20, 267–281 CrossRef PubMed.
- J. E. Koehne, M. Marsh, A. Boakye, B. Douglas, I. Y. Kim, S. Y. Chang, D. P. Jang, K. E. Bennet, C. Kimble, R. Andrews, M. Meyyappan and K. H. Lee, Analyst, 2011, 136, 1802–1805 RSC.
- W. Shu, Y. Wang, C. Liu, R. Li, C. Pei, W. Lou, S. Lin, W. Di and J. Wan, Small Methods, 2019, 4, 1900469 CrossRef.
- Y. Zhang, B. Li and X. Chen, Microchim. Acta, 2009, 168, 107–113 CrossRef.
- J. Liu, C. Cai, Y. Wang, Y. Liu, L. Huang, T. Tian, Y. Yao, J. Wei, R. Chen, K. Zhang, B. Liu and K. Qian, Adv. Sci., 2020, 7, 1903730 CrossRef CAS PubMed.
- S. Rostami, A. Mehdinia, A. Jabbari, E. Kowsari, R. Niroumand and T. J. Booth, Sens. Actuators, B, 2018, 271, 64–72 CrossRef CAS.
- A. Yildirim and M. Bayindir, Anal. Chem., 2014, 86, 5508–5512 CrossRef CAS PubMed.
- S. Baluta, K. Malecha, D. Zając, J. Sołoducho and J. Cabaj, Sens. Actuators, B, 2017, 252, 803–812 CrossRef CAS.
- N. Li, C. Nan, X. Mei, Y. Sun, H. Feng and Y. Li, Microchim. Acta, 2020, 187, 496 CrossRef CAS PubMed.
- S. Ghosh, N. Nagarjun, S. Nandi, A. Dhakshinamoorthy and S. Biswas, J. Mater. Chem. C, 2022, 10, 6717–6727 RSC.
- S. Gajendar, K. Amisha and S. Manu, CrystEngComm, 2021, 23, 599–616 RSC.
- R. Santonocito, N. Tuccitto, A. Pappalardo and G. Trusso Sfrazzetto, Molecules, 2022, 27, 7503 CrossRef CAS PubMed.
- A. Kumar, A. Kumari, P. Mukherjee, T. Saikia, K. Pal and S. K. Sahu, Microchem. J., 2020, 159, 105590 CrossRef CAS.
- F. Moghzi, J. Soleimannejad, E. C. Sañudo and J. Janczak, ACS Appl. Mater. Interfaces, 2020, 12, 44499–44507 CrossRef CAS PubMed.
- S. Zhuo, Y. Guan, H. Li, J. Fang, P. Zhang, J. Du and C. Zhu, Analyst, 2019, 144, 656–662 RSC.
- Z. Tang, K. Jiang, S. Sun, S. Qian, Y. Wang and H. Lin, Analyst, 2019, 144, 468–473 RSC.
- K. Wang, J. Song, X. Duan, J. Mu and Y. Wang, New J. Chem., 2017, 41, 8554–8560 RSC.
- X. P. He, Y. L. Zeng, X. Y. Tang, N. Li, D. M. Zhou, G. R. Chen and H. Tian, Angew. Chem., Int. Ed., 2016, 55, 13995–13999 CrossRef CAS PubMed.
- W. T. Dou, H. H. Han, A. C. Sedgwick, G. B. Zhu, Y. Zang, X. R. Yang, J. Yoon, T. D. James, J. Li and X. P. He, Sci. Bull., 2022, 67, 853–878 CrossRef CAS.
- E. Climent, M. Biyikal, D. Gröninger, M. G. Weller, R. Martínez-Máñez and K. Rurack, Angew. Chem., Int. Ed., 2020, 59, 23862–23869 CrossRef CAS.
- Y. Liu, J. Li, G. Wang, B. Zu and X. Dou, Anal. Chem., 2020, 92, 13980–13988 CrossRef CAS.
- R. Puglisi, R. Santonocito, E. Butera, G. L. Mendola, A. Pappalardo and G. Trusso Sfrazzetto, ACS Omega, 2023, 8, 38038–38044 CrossRef CAS PubMed.
- R. Santonocito, M. Spina, R. Puglisi, A. Pappalardo, N. Tuccitto and G. Trusso Sfrazzetto, Chemosensors, 2023, 11, 503 CrossRef CAS.
- R. Santonocito, R. Parlascino, A. Cavallaro, R. Puglisi, A. Pappalardo, F. Aloi, A. Licciardello, N. Tuccitto, S. O. Cacciola and G. Trusso Sfrazzetto, Sens. Actuators, B, 2023, 393, 134305 CrossRef CAS.
- R. Santonocito, N. Tuccitto, V. Cantaro, A. B. Carbonaro, A. Pappalardo, V. Greco, V. Buccilli, P. Maida, D. Zavattaro, G. Sfuncia, G. Nicotra, G. Maccarrone, A. Gulino, A. Giuffrida and G. Trusso Sfrazzetto, ACS Omega, 2022, 7, 37122–37132 CrossRef CAS PubMed.
- N. Tuccitto, G. Catania, A. Pappalardo and G. Trusso Sfrazzetto, Chem. – Eur. J., 2021, 27, 13715–13718 CrossRef CAS.
- N. Tuccitto, L. Riela, A. Zammataro, L. Spitaleri, G. Li-Destri, G. Sfuncia, G. Nicotra, A. Pappalardo, G. Capizzi and G. Trusso Sfrazzetto, ACS Appl. Nano Mater., 2020, 3, 8182–8191 CrossRef CAS.
- S. Dugam, S. Nangare, P. Patil and N. Jadhav, Ann. Pharm. Fr., 2021, 79, 335–345 CrossRef CAS.
- M. J. Molaei, RSC Adv., 2019, 9, 6460–6481 RSC.
- L. S. De Bortoli, C. R. Vanoni, C. L. Jost, D. Z. Mezalira and M. C. Fredel, J. Electroanal. Chem., 2023, 947, 117744 CrossRef CAS.
- P. Narasimhappa and P. C. Ramamurthy, Electrochim. Acta, 2023, 469, 143187 CrossRef CAS.
- R. Santonocito, M. Intravaia, I. M. Caruso, A. Pappalardo, G. Trusso Sfrazzetto and N. Tuccitto, Nanoscale Adv., 2022, 4, 1926–1948 RSC.
- L. Wang, J. Jana, J. S. Chung and S. H. Hur, Dyes Pigm., 2021, 186, 109028 CrossRef CAS.
- R. Das, R. Pal, S. Bej, M. Mondal, K. Kundu and P. Banerjee, Mater. Adv., 2022, 3, 4421–4459 RSC.
- G. Chellasamy, S. R. Ankireddy, K. N. Lee, S. Govindaraju and K. Yun, Mater. Today Bio, 2021, 12, 100168 CrossRef PubMed.
- X. Li, X. Liu, Y. Liu, R. Gao, X. Wu and X. Gao, Talanta, 2022, 249, 123700 CrossRef CAS.
- M. Ilgar, G. Baytemir, N. Taşaltın, S. Güllülü, I. S. Yeşilyurt and S. Karakuş, J. Photochem. Photobiol., A, 2022, 431, 114075 CrossRef CAS.
- Y. Huang, P. Chen, L. Zhou, J. Zheng, H. Wu, J. Liang, A. Xiao, J. Li and B. O. Guan, Adv. Mater., 2023, 35, 2304116 CrossRef CAS.
- M. Mahdavi, H. Emadi and S. R. Nabavi, Nanoscale Adv., 2023, 5, 4782–4797 RSC.
- R. M. Qaisi, A. Akhdhar, J. W. Choi and W. Ahmed El-Said, Spectrochim. Acta, Part A, 2023, 287, 122109 CrossRef CAS PubMed.
- N. La Ngoc Tran, B. T. Phan, H. K. T. Ta, T. T. K. Chi, B. T. T. Hien, N. T. T. Phuong, C. C. Nguyen, T. L. H. Doan and N. H. T. Tran, Sens. Actuators, A, 2022, 347, 113932 CrossRef CAS.
- X. Lin, X. K. Lu, K. H. Zhu, Y. C. Jiang, J. C. Chen, P. Z. Yan and D. S. Zhao, Molecules, 2022, 27, 8962 CrossRef CAS PubMed.
- G. Ozkan, J. Sci. Food Agric., 2023, 104, 2744–2749 CrossRef PubMed.
- J. Matthew, IMPublications, Chichester, UK and SurfaceSpectra, Manchester, UK, 2003. 900 pp., ISBN 1-901019-04-7, 900 pp, Surf. Interface Anal., 2004, 36, 1647 CrossRef CAS.
- A. Gulino, Anal. Bioanal. Chem., 2012, 405, 1479–1495 CrossRef PubMed.
- G. Greczynski and L. Hultman, Angew. Chem., Int. Ed., 2020, 59, 5002–5006 CrossRef CAS PubMed.
- S. Li, H. Zhang, Z. Liu, J. Xu, G. Fan, W. Li, Q. Li, X. Hu and G. Jing, Appl. Sci., 2022, 12, 1245 CrossRef CAS.
- N. Cardullo, V. Muccilli, V. Cunzolo and C. Tringali, Molecules, 2020, 25, 3257 CrossRef CAS.
- A. E. Maccarronello, N. Cardullo, A. Margarida Silva, A. Di Francesco, P. C. Costa, F. Rodrigues and V. Muccilli, Food Chem., 2024, 443, 138504 CrossRef.
- N. Cardullo, V. Muccilli, R. Saletti, S. Giovando and C. Tringali, Food Chem., 2018, 268, 585–593 CrossRef CAS.
- R. Puglisi, F. P. Ballistreri, C. M. A. Gangemi, R. M. Toscano, G. A. Tomaselli, A. Pappalardo and G. Trusso Sfrazzetto, New J. Chem., 2017, 41, 911–915 RSC.
- J. Schindelin, I. Arganda-Carreras, E. Frise, V. Kaynig, M. Longair, T. Pietzsch, S. Preibisch, C. Rueden, S. Saalfeld, B. Schmid, J. Y. Tinevez, D. J. White, V. Hartenstein, K. Eliceiri, P. Tomancak and A. Cardona, Nat. Methods, 2012, 9, 676–682 CrossRef CAS PubMed.
- G. Li-Destri, L. Fichera, A. Zammataro, G. Trusso Sfrazzetto and N. Tuccitto, Nanoscale, 2019, 11, 14203–14209 RSC.
- A. Zammataro, C. M. A. Gangemi, A. Pappalardo, R. M. Toscano, R. Puglisi, G. Nicotra, M. E. Fragalà, N. Tuccitto and G. Trusso Sfrazzetto, Chem. Commun., 2019, 55, 5255–5258 RSC.
- J. Stetfeld, S. A. McKenna and T. R. Patel, Biophys. Rev., 2016, 8, 409–427 CrossRef.
- S. Bhattacharjee, J. Controlled Release, 2016, 235, 337–351 CrossRef CAS.
- A. Gulino, G. G. Condorelli, P. Mineo and I. Fragalà, Nanotechnology, 2005, 16, 2170–2175 CrossRef CAS.
- M. Zimbone, G. Cacciato, M. Boutinguiza, A. Gulino, M. Cantarella, V. Privitera and M. G. Grimaldi, Catal. Today, 2019, 321–322, 146–157 CrossRef CAS.
- N. Graf, E. Yegen, T. Gross, A. Lippitz, W. Weigel, S. Krakert, A. Terfort and W. E. S. Unger, Surf. Sci., 2009, 603, 2849–2860 CrossRef CAS.
- M. Sudolská, M. Dubecký, S. Sarkar, C. J. Reckmeier, R. Zbořil, A. L. Rogach and M. Otyepka, J. Phys. Chem. C, 2015, 119, 13369–13373 CrossRef.
- P. Anilkumar, X. Wang, L. Cao, S. Sahu, J. H. Liu, P. Wang, K. Korch, K. N. Tackett
Ii, A. Parenzan and Y. P. Sun, Nanoscale, 2011, 3, 2023–2027 RSC.
- M. Mondal and S. Pramanik, Mater. Lett.: X, 2023, 18, 100195 CAS.
- T. Yadav and V. Mukherjee, J. Mol. Struct., 2018, 1160, 256–270 CrossRef CAS.
- M. Caruso, M. Petroselli and M. Cametti, ChemistrySelect, 2021, 6(45), 12975–12980 CrossRef CAS.
- M. Petroselli, Y.-Q. Chen, M.-K. Zhao, J. Rebek and Y. Yu, Chin. Chem. Lett., 2023, 34(5), 107834 CrossRef CAS.
- M. Petroselli and C. Bacchiocchi, Org. Chem. Front., 2022, 9(22), 6205–6212 RSC.
Footnote |
† Electronic supplementary information (ESI) available: XPS, FT-IR, NMR, fluorescence measurements, DFT details, recovery and stability. See DOI: https://doi.org/10.1039/d4tb00651h |
|
This journal is © The Royal Society of Chemistry 2024 |
Click here to see how this site uses Cookies. View our privacy policy here.