DOI:
10.1039/D4VA00014E
(Critical Review)
Environ. Sci.: Adv., 2024,
3, 661-675
A brief review on the assessment of potential joint effects of complex mixtures of contaminants in the environment
Received
22nd January 2024
, Accepted 13th March 2024
First published on 5th April 2024
Abstract
Organisms and humans are exposed to a “cocktail” of contaminants in the environment, but methods for mixture assessment, untargeted analysis, and source identification (fingerprinting) are still lagging, which is critically reviewed in this article. Firstly, this paper briefly summarized both the direct and indirect effects of chemical contaminants at multiple levels on the biological responses of wild organisms. Secondly, the choice of a predictive model for chemical mixture assessment can greatly influence the outcome. Therefore, this review emphasizes the limitation of the main methodologies of risk assessments for chemical mixtures. Thirdly, since current environmental toxicology approaches face barriers to realizing the true potential of advances in analytical chemistry for human health or ecology risk assessment, bioanalytical methods, to screen toxic chemicals or identify unknown chemicals at environmentally relevant levels are reviewed. Lastly, Recently developed machine learning models, incorporating non-targeted screening analysis for the suspect and unknown chemicals and machine learning methods, can be trained on complex datasets to better predict interactions among identified chemicals with random combinations, quantification of similar structural chemicals without the presence of analytical standards, and transfer of chemicals based on their physicochemical properties in human tissues. To perform risk assessments for a variety of chemicals, we propose employing a framework that makes use of a range of methods from the toolbox summarized in this review.
Environmental significance
Anthropogenic contaminants are ubiquitous and are normally present in the environment as complex mixtures, where wildlife and humans can be exposed to these chemicals. Many contaminants may have long-term effects with chronic exposures of organisms at low concentrations. However, current approaches for evaluating the toxicity of chemical mixtures can be questionable and fail to adequately consider synergistic or interactive effects. New approaches for identifying the effects of chemical mixture samples have been developed, e.g., nontarget screening analysis using high-resolution mass spectrometry and machine learning models for predicting the toxicity of chemicals using previous toxicity data. Developing these new methods can help quickly identify the potential toxicity of highly complex mixtures without the initial consideration of known chemicals.
|
1. Introduction
Chemical pollution is a serious global problem that poses a threat to organisms and human health. There are currently over 350
000 registered chemicals being produced and used worldwide.1 The extensive use and production of these chemicals result in continuous exposure to chemical pollutants for wildlife, humans, and ecological systems. As a result, these chemicals can enter the bodies of wildlife and humans through various routes of exposure, such as ingestion or inhalation, leading to harmful effects on both wildlife and human health.2,3 Despite the occurrence of obvious events, such as widespread wildlife mortality, which may indicate the consequences of chemical spills, chemical pollutants can also have more subtle yet significant adverse effects on ecosystems.2 Additionally, most environmental pollutants have long-term effects when organisms are exposed to low concentrations over an extended period, which can be just as harmful as acute effects of short-term exposures.4 However, conventional methods used to assess the toxicity of chemical contaminants typically involve laboratory experiments that focus on the effects of a single pollutant on a single species under controlled conditions.5,6
Although it is important to understand the effects of a single pollutant on individual organisms, it is crucial to recognize that communities of organisms in real environments are typically exposed to multiple pollutants. For instance, when examining water, soil, air, and precipitation in aquatic and terrestrial environments, researchers have discovered complex mixtures of pesticides, such as atrazine, diazinon, and metolachlor, often at relatively low concentrations (<1 μg L−1).7–9 However, chemical analysis provides only limited insight into the complexity of these mixtures, which are expected to contain over 1000 compounds.10 Due to their negative impact on the environment and human health, regulatory authorities have established maximum concentration levels (MCLs) for various contaminants. For example, MCLs of ∼3534 pesticides in drinking water have been regulated by 130 international jurisdictions11 and the World Health Organization.12 Furthermore, the Nationwide Urban Runoff Program (U.S. Environmental Protection Agency) released MCLs of numerous contaminants in soils and groundwater.13 While these concentration limits may be effective and safe for individual contaminants at very low levels, the accumulation of multiple pollutants in the environment poses a major problem, particularly in ecosystems that contain human consumers. A previous study revealed that mixtures of different contaminants were discovered in groundwater used for public supply, and at worryingly high concentrations.14 However, there is currently limited knowledge regarding the effects of more diverse mixtures of contaminants on individuals15–17 or communities in many environmental situations.18,19
Two methodologies have been widely used for assessing the combined effects of chemical mixtures. The first is whether toxic chemicals act via common pathways such as cells oxidized and oxidative stress.20 The second approach uses the toxicity of chemical mixtures with the side effects resulting from chemical and biochemical interactions that can lead to diverse toxic effects on tissues and cells.21 Although these methodologies are being developed and applied in some cases and for specific users, such as the WHO/IPCS framework,22 there is currently no systematic and integrated method for hazard evaluation.
Evaluating the harmful effects of chemical mixtures on the ecosystem and human health, for example in relation to food, is a major concern for policymakers and regulators.23–27 Previous efforts have aimed to link specific harmful impacts, such as mortality and pathology, to the toxicity of individual components within mixtures.28 However, these attempts have been only somewhat successful, and the hypothesis that the toxicity of a complex mixture is simply the sum of the toxicity of its constituents is now seen as questionable, especially when considering potential synergistic or interactive impacts.29,30 For instance, numerous mixtures of medicines used for treatments have shown significant synergistic interactions.25,31 Additionally, there is evidence of emergent effects in the estrogen-like activity of mixed contaminants,32,33 and in the synergistic interactive effects of sucrose polyester (a harmless food additive) and anthracene, a polycyclic aromatic hydrocarbon (PAH).34 In complex mixtures, where confounding factors are present and contaminants are often associated with particle surfaces or lipid-rich coatings, catalytic reactions can occur, leading to the formation of new compounds.35 This is particularly true in a two-dimensional environment, such as on surfaces, where highly concentrated compounds are located and reactions, such as oxidation, can easily take place. For instance, debris such as microplastics can encounter a wide range of different pollutants with varying degrees of toxicity. To further complicate matters, the surface properties of plastics can be significantly altered by interactions with co-contaminants, which can in turn be exposed to organisms.36,37 Several additives used in plastic manufacturing processes have been found to produce synergistic,38–40 antagonistic,41 or additive effects when combined with other environmental pollutants, such as pesticides, pharmaceuticals, and PAHs.
There are thousands of chemical substances that may have a toxic effect on human in the environment. Current advances in high-resolution mass spectrometry (HRMS)-based non-target screening have the potential to identify hundreds of chemicals in a single batch of experiments.42 This means that the field of analytical chemistry is shifting from targeted individual chemical analysis to non-targeted exposome-wide analysis. Where there is still limited information on the toxicity of most chemical contaminants, a new strategy for toxicity screening using biological receptors has been developed.43,44 Additionally, machine learning techniques have been utilized to predict the interactions and assess the risks associated with mixture exposure and chemical fingerprinting. This review summarizes studies on the adverse effects of exposure to contaminant mixtures and current methodologies for risk assessment of chemical mixtures. It also focuses on non-target screening and machine learning methods to screen hazardous contaminants and predict the fate and toxicity of environmental mixtures.
2. Review method
The electronic databases, e.g., Web of Knowledge, ScienceDirect, and ACS Publications, were searched from 2000 to 2023, using the search terms: emerging contaminants, non-targeted analysis, NTA, chemical mixtures, toxicity, assessment, and machine learning. We only chose the recently relevant references concerning the effects/toxicity of mixture chemicals, assessment models of mixture chemicals, non-targeted screening analysis, and machine learning of emerging contaminants. We classified the chapters according to different research areas of the current assessment of known and unknown chemical mixtures in the environment.
3. Toxicity of mixture of contaminants to organisms
The proliferation of new chemicals in recent years has surged, evidenced by a staggering 37 million increase in the Chemical Abstract Service Registry within the past three years alone.45 Yet, our capacity to evaluate the associated risks remains constrained. Table 1 provides a broad overview of mixture contaminants' toxicity to organisms. For instance, only 11% of single chemicals registered in Europe and the United States have available empirical data on their toxicity to aquatic biota.46–48 Moreover, the dynamic environmental landscape, characterized by diverse pollutants like contaminated water, sediment, and soil, poses substantial threats to local flora and fauna. In light of these challenges, concerted efforts are needed to enhance our understanding and management of chemical risks in ecosystems.
Table 1 Toxicity of mixture of contaminants to organisms
Target receptor |
Chemicals |
Questions/Aims |
Toxicity/Effect |
Reference |
Daphnia magna |
Organochlorine pesticides |
What are the effects of combined nutrients and pesticides on the abundance of D. magna and algal biomass? |
A significant reduction in the abundance of D. magna |
Onyango, et al.125 |
Round gobies (Neogobies melanostomus) |
Bupropion, carbamazepine, citalopram, clotrimazol, diltiazem, diphenhydramine, diphenhydramine, flecainide, risperidone, etc. |
If contaminant exposure in general reduces the competitive capacity of round gobies? |
Reduction of aggression behavior |
Sopinka, et al.50 |
Larval amphibians |
Malathion, carbaryl, chlorpyrifos, diazinon, and endosulfan, glyphosate, atrazine, acetochlor, metolachlor, and 2,4-D |
How mixtures of pesticides at low concentrations affect aquatic communities? |
Reproductive issues |
Relyea7 |
Largemouth bass (Micropterus salmoides) |
17β-estradiol, 17α-ethinyl estradiol, and nonylphenols |
If the vitellogenin receptor may be a target of selectendocrine disr upting compounds through environmental exposures? |
Induction of vitellogenin |
Dominguez, et al.126 |
Juvenile rainbow trout (Oncorhynchus mykiss) |
Estrone (E1), estradiol-17β (E2), and 17α-ethynylestradiol (EE2) |
If induction of vitellogenin in juvenile female rainbow trout can be determined to the relative potency? |
Mixture was more potent than either of the individual chemicals |
Thorpe, et al.54 |
Fathead minnows (Pimephales promelas) |
Estradiol, 17α-ethinylestradiol, bisphenol A, 4-nonylphenol, and 4-tert-octyphenol |
If the combined effects of five estrogenic chemicals on VTG induction in the fathead minnow? |
Vitellogenin induction |
Brian Jayne, et al.58 |
Pakchoi root |
Ciprofloxacin, norfloxacin, and tetracycline |
Assessing the phytotoxicity of mixed antibiotics drugs for plants |
Low-dose synergism and high-dose antagonism |
Yang, et al.59 |
Freshwater cyanobacteria (Microcystis aeruginosa) |
Spiramycin and amoxicillin |
Would the combined two target antibiotics have effects on algal growth? |
Harm of M. aeruginosa |
Liu, et al.60 |
Atlantic salmon |
Endosulfan, chlorpyrifos, phenanthrene, benzo(a)pyrene |
Screening for interaction effects of contaminants using metabolomic, lipidomic and transcriptomic profiling |
A synergistic effect on decrease of cell viability and down-regulate of CYP3A and FABP4 transcription |
Søfteland, et al.62 |
Developing zebrafish |
Bisphenol A and triclocarban (TCC) |
What are TCC's effects on transcription of known estrogen target genes? |
TCC strongly enhanced the overexpression of AroB that is induced by exogenous estrogen |
Chung, et al.61 |
Marine mussels |
Phenanthrene, anthracene, lindane, malathion, irgarol-1051, cis-permethrin and sodium dodecylbenzene sulphonate |
Whether aliphatic oils are accumulated in epithelia cells of marine mussel digestive tract? |
Lysosomal lipid storage disease (lipidosis) might be induced in the long term |
Moore, et al.34 |
Human cell |
Decabrominated diphenyl ether, bisphenol A, and acrylamide |
Assessing their combined effects in terms of additive, antagonistic or synergistic toxicity |
Oxidative DNA damage and decrease of repair capacity |
Kopp, et al.63 |
Microbenthic organisms |
PAHs |
If exposure to this mixture of organic contaminants in sediments will decrease the expression of microbial AmoA genes and nitrification rates? |
The significant effects on microbenthic community structure although the individual components are present at low concentrations |
Iburg, et al.65 |
Exposure to pollutants triggers a cascade of effects that affect various reactions controlled by specific mechanisms. In addition to direct effects on growth, survival, and reproduction, exposure to chemicals can also alter the behavior of organisms. In the real world, organisms encounter combinations of chemicals rather than individual compounds. For instance, a study conducted in Lombardy waters, Italy, examined the potential synergistic effects of mixtures of herbicides and insecticides that exceeded the legal limit in surface water.49 This study used the alga Chlamydomonas reinhardtii and found that the presence of palmelloids (phenotypic plasticity in algae as a response to environmental stress through the generation of reversible colonies) induced stress in the algae.49 Other examples for round gobies (Neogobius melanostomus) sampled from intensely polluted industrial sites50 and lab-exposed to municipal sewage effluent51 revealed the same reduction of aggression behavior despite the pollutant mixtures being significantly different.
Combined endocrine disruptors can have significant effects, particularly causing reproductive issues, even at low doses that do not individually produce observable effects.52 This phenomenon also applies to other environmental contaminants. For instance, the simultaneous application of insecticides and herbicides led to a staggering 99 percent mortality rate among amphibian larvae.7 Additionally, estrogen compounds present in discharged wastewater can disrupt the induction of vitellogenin protein in fish, consequently exerting varying degrees of influence on their reproductive behavior.53 To study some of the underlying mixture phenomena, the effects of binary mixtures of estrogenic compounds on juvenile rainbow trout (Oncorhynchus mykiss) were studied by Thorpe, et al.54 The same holds for mutagenicity, where mixture tests conducted in surface water revealed a strong synergism between aromatic amines, not only with norharman but also with carboline and 5-carboline, strongly suggesting that surface water mutagenicity is highly complex and driven by synergistic mechanisms of a complex compound mixture.55 In 2014, the United States Geological Survey conducted a study in South Carolina Piedmont and found 475 unique organic compounds, indicating widespread instream exposure to extensive contaminant mixtures and providing multiple lines of evidence for adverse effects on aquatic communities, including estrogenicity and mutagenicity.56 These examples underscore the profound impact of integrated environmental contaminants on aquatic ecosystems and emphasize the importance of considering mixed effects for effective environmental management and protection.
These findings are similar to the results of toxicity experiments conducted in the laboratory using neat chemicals. For example, the epibenthic amphipod Hyalella azteca was exposed to multiple stressors (i.e., water temperature and salinity) in the presence of mixed contaminants. This exposure resulted in negative synergistic effects on invertebrate survival, behavior, and gene response after 96 hours.57 The effects of a binary mixture of estradiol and 4-nonylphenol varied based on concentration levels. Additionally, a combination of estradiol and 17α-ethinylestradiol also had concentration additive effects.54 Furthermore, a larger group of chemicals (estradiol, 17α-ethinylestradiol, bisphenol A, 4-nonylphenol, and 4-tert-octyphenol) were studied to determine the concentration–response relationships of vitellogenin induction in fathead minnows (Pimephales promelas). The data collected from these studies were then used to predict the responses to a mixture of all five chemicals, and the results showed that the observed effects were consistent with the expected increase in concentrations.58
Different tests have been used to determine the toxic effects of chemical mixtures on plants and primary producers. In one study, a relative root elongation (RRE) test was conducted to examine the individual and combined effects of ciprofloxacin, norfloxacin, and tetracycline on pakchoi. The analysis of toxic units (TU) and synergistic ratios (SR) indicated that lower EC50 values were observed in combinations with lower antibiotic concentrations, and greater EC50 values in combinations with greater antibiotic concentrations, suggesting a low-dose synergistic effect and a high-dose antagonistic effect.59 In another experiment, the combined toxicity of spiramycin and amoxicillin was tested on Microcystis aeruginosa. The EC50 values expressed in TU values were 1.25 and 1.83 for spiramycin and amoxicillin, respectively, when mixed in rations of 1
:
7 and 1
:
1. These results suggest an antagonistic interaction at the median effect level.60 Furthermore, it was found that a mixture of the two target antibiotics with a low proportion of spiramycin (<50%) would increase the harm that M. aeruginosa itself can cause to aquatic environments by stimulating its growth and production and release of the algal biotoxin microcystin-LR at environmentally-relevant contamination levels.60 In another study, the expression of estrogen target genes in the brain of developing zebrafish embryos demonstrated that the overexpression of B-subtype aromatase was induced by the combined presence of bisphenol A and triclocarban.61
Mixture effects were also described in considerable detail using fish cellular and early-life stage models. For example, Atlantic salmon primary hepatocytes were used to examine the interaction effects of a broad variety of contaminants. In this study, the results showed that pesticides caused the strongest responses, although they had less impact on cell viability compared to PAHs. PAH-exposed hepatocytes, on the other hand, only exhibited weak molecular responses. The findings indicated that the interaction effects between the contaminants could mostly be explained as an additive effect. However, at high concentrations, the contaminants acted synergistically.62
In other in vitro toxicity tests, significant emergent synergistic interactions were observed in the lysosomal membrane stability of hemocytes from marine mussels when exposed to environmentally relevant chemicals (i.e., polycyclic aromatic hydrocarbons, pesticides, biocides, and a surfactant).10 Synergistic interactions among components were observed in mixtures compared to the response of human cells to exposure to individual chemicals. The authors speculated that this was mainly due to the simultaneous induction of oxidative DNA damage and a decrease in repair capacity, contributing to the synergistic toxic effect of these chemical mixtures.63 Mixture of organic contaminants, e.g., hexachlorobenzene, biphenyl, three polychlorinated biphenyls, and PAHs, can decrease relative abundance of sensitive taxa such as Paraplectonema and increase the abundance of resistant nematodes.64 Furthermore, other studies also showed that mixtures of these contaminants could have significant effects on microbenthic organisms,65 alkaline phosphatase activation,66 gene expressions, and cellular activities.67 All these studies indicate that the additive or synergistic mechanisms of combined contaminants posed disturbances to multiple organelles.
4. Current toxicity evaluation system of chemicals
4.1 Current individual chemical toxicity testing strategies
Currently, the most recognized approach for estimating the toxicity of chemicals is through whole-organism in vivo bioassays. However, this approach has limitations in terms of low throughput and high costs, which makes it impractical for testing a large number of chemicals. As a solution, in vitro, high-throughput screening (HTS) assays (Fig. 1a) have been recommended as a relatively low-cost method compared to in vivo bioassays. This allows for the screening of a large number of compounds for their potential toxicity.68 HTS assays can be conducted using 96-, 384-, or 1536-well plates, which allow for the screening of numerous samples in one batch.69 Over the years, various in vitro bioassays, such as those involving nuclear receptors (NRs) including the aryl hydrocarbon receptor (AhR), as well as nuclear factor-erythroid 2 related factor 2 (Nrf2), have been developed and deployed.43,69–71
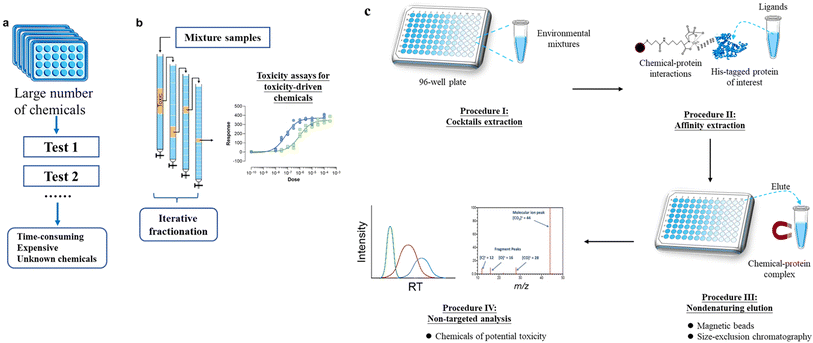 |
| Fig. 1 Illustration of processes for (a) in vitro high-throughput screening (HTS) assays, (b) effect-directed analysis (EDA), and basic workflow of the APNA approach. This figure is modified from Gong, et al.102 | |
Although these assays have been used in many studies, there are still three main disadvantages to this “bottom-up” approach, where each chemical is individually tested. Specifically, (1) no single bioassay can test all toxicity mechanisms. Despite there being over 700 bioassays used in the US Environmental Protection Agency's Toxicity Forecaster (ToxCast program),72 it can only cover a small fraction of the toxicity routes mediated by around 20
000 human proteins. (2) This approach is also very time-consuming and expensive. With a large number of chemicals (>350
000) and bioassays (>700), conducting over 245 million tests would be necessary for each chemical and endpoint. However, as of 2014, only around 4500 chemicals have been tested by the ToxCast program (https://www.epa.gov/chemical-research/toxcast-chemicals). (3) In addition, certified reference standards are not available for most chemicals, especially unknown metabolites that are formed during various environmental or biological processes.73 These metabolites might even be more toxic than their original substances.74,75 In light of these limitations of the “bottom-up” strategy, it is important to establish a complementary assay for toxicity screening that considers the seemingly limitless scale of the exposome.
Another toxicity-driven approach to screen and identify chemicals in mixtures is effect-directed analysis (EDA) (Fig. 1b). EDA combines toxicity tests, fractionation of multi-component samples, and chemical analysis. It is considered a powerful tool for screening toxic chemicals in complex environmental samples.76,77 However, the process of iterative fractionation in EDA is highly time-consuming and labor-intensive. This can potentially introduce artifacts or cause the loss of important toxic components. Additionally, the co-elution of numerous chemicals in the same fraction can lead to high false positive rates. As a result, the successful application of the EDA approach for identifying new toxic chemicals in environmental samples is limited to a small number of highly qualified laboratories.78,79 A recent example of a new chemical being identified using EDA approaches is N-(1,3-dimethyl butyl)-N′-phenyl-p-phenylenediamine-quinone (6PPD-quinone), an oxidation product of the tire antioxidant 6PPD, which was found to acute mortality to coho salmon (Oncorhynchus kisutch) at threshold concentrations of ∼1 μg L−1.78
4.2 Current assessment models of mixture chemicals
4.2.1 Functions of assessment models.
Some mathematical models are employed to evaluate the toxicological impacts of chemicals. These models are based on either the individual chemical behavior through different modes of action (independent action, IA) or through a similar mode of action (concentration addition, CA).80–82 Considering real-world environmental conditions, Escher, et al.83 utilized a combined CA/IA model to evaluate the low-effect concentration and response curves for over 200 mixtures of water samples, consisting of 17 chemicals. They found that 86% of the samples fell within the effective ranges calculated by the CA/IA model, which supports the CA hypothesis that toxic effects can be aggregated. However, these joint models can only be applied to samples with low effect levels and cannot fully explain the effects of complex mixtures with chemical interactions. However, when interactions occur within a mixture, synergistic and antagonistic effects may only be observed with a few specific compounds at high concentrations, among thousands of compounds present at low concentrations in an environmental sample.23
Other models were also utilized to determine if and when a combined risk assessment is necessary. These tools can help identify the individual contribution of chemicals among the total toxicity of known chemicals in the mixture. Although these tools were not specifically designed to predict the risk of a mixture, they offer an approach for investigating data on the cumulative risk assessment of mixture chemicals to human or ecological receptors. One example is the maximum cumulative ratio (MCR), which is the ratio between the toxicity of a mixture and the toxicity of the most significant contributing chemical in the mixture. The MCR can determine whether the toxicity to a receptor is due to a single chemical or multiple chemicals in combined exposure.84 Before calculating MCR, it may be necessary to establish a screening level for mixture risk assessment to predict the combined effects that may be associated with adverse effects caused by the most contributing compounds. To address this concern, the MCR method has been proven to be a valuable tool as part of a tiered approach to prioritize and identify acute toxicity from a small subset of mixtures.85 This is particularly useful when the potentially adverse effect may be overlooked through individual chemical assessments. Similarly, the threshold of toxicological concern (TTC) approach can be used as a ranking assessment tool for chemical exposures, especially when there is a lack of available chemical data. For instance, Meek, et al.22 conducted a risk assessment by applying the TTC approach to ten chemicals detected in water. This was done for chemicals that did not have any existing health-based guidance or chronic health standards.
Current assessment models require toxicological data for each known chemical in a mixture. The toxicity of environmental mixtures is typically determined by using one of two methods: (1) testing the entire mixture using a simple in vivo or in vitro system, or (2) using toxicological data for individual chemicals along with their analytical concentrations. The second method can be used to create a mathematical model that predicts the overall toxicity of the mixture. The whole mixture test is often used for environmental mixtures, allowing for the prediction of the toxicity of unknown compositions in the mixture, even if the specific toxicity of the compounds is unknown. The component-based method is more commonly used, but it requires more information on compound identification, concentration, and toxicity for the individual chemicals.
4.2.2 Issues of assessment models.
Some methodological issues frequently arise during the process of risk assessment for chemical mixtures. The data sources used for risk assessment can be diverse and somewhat incomplete, which directly impacts the accuracy and associated uncertainties of the assessments. Furthermore, there is a significant limitation in data availability to validate the estimated results. Cumulative risk assessments often fail to account for the combined adverse effects of multiple stressors, including chemical or biological agents, and environmental conditions, as the simplifying assumptions made by these risk assessment tools are not applicable in such cases. Exposure data are typically derived from biomonitoring or published data from exposure experiments. The reliability of exposure data depends on the biomonitoring procedure used.86,87 However, assessing exposure to lipophilic and bioaccumulating compounds poses additional challenges. This is because it requires considering the kinetics of these compounds and accounting for the body burden as a starting point for risk assessment, instead of relying solely on daily intake and exposure history. This is necessary because exposure patterns can vary over time. Similarly, toxicological data are largely obtained from published databases, with missing data sometimes being filled in using a variety of methods. The gaps in data relating to exposure and toxicity for various chemicals, such as pesticides and pharmaceuticals,80,88–90 can be quite significant. This means that there is a high level of uncertainty when it comes to assessing risks using extrapolation methods. To illustrate this point, models have been developed to assess the cumulative exposure of consumers to personal care products.91 However, there is still a lack of detailed data regarding the frequency of use of those products,92 which hampers the accuracy of risk assessment.
Except for the required exposure and toxicological data, the risk assessment of chemical mixtures also relies heavily on assumptions, which greatly impact the outcome.89,93 This pattern is similar to that of a single chemical assessment, but it becomes particularly important in the assessment of chemical mixtures because the uncertainties from individual chemical assessments accumulate when assessing combined risks. It is important to exercise caution when interpreting the results in cases where different models are combined and applied to the same risk assessment, such as the differences between dietary and non-dietary exposure models. Furthermore, when assessing the combined effects of chemicals, it is necessary to consider reference values for the specific effect on common effects or common modes of action. However, toxicity values often dominate the assessment of single chemicals, which means that using the lowest reference values in the risk estimation may lead to an overestimation of the combined effects. In the case of a mixture sample containing unknown chemicals with dominant toxicity, the assessment models become ineffective without existing toxicology data and knowledge of the compound structure.
5. New approaches to assess environmental mixtures
5.1 Non-target screening analysis
With the development of HRMS, screening analysis has been applied for expected suspect chemicals based on the database or for the determination of unknown chemicals using the examination of HRMS.94,95 For example, non-target screening with LC/GC quadrupole time-of-flight (Q/TOF) confirmed 271 chemicals, 163 of which can be conducted for unambiguous identification by in silico fragmentation from 38 dust samples.96 This study found that a large number of emerging contaminants (e.g., organofluorine/polyfluorinated compounds) have been underestimated in the dust that can potentially affect humans. To avoid extensive machine-running time for non-target screening, some interesting studies conducted a pooling samples strategy to run a minimal number of samples for a wide range of chemicals. In a recent study, sixteen pooled human serum samples were randomly selected from 25 individual samples for GC-Q/TOF suspect screening analysis, where 5444 unique compounds were tentatively identified with data-based spectral matching. A similar study was conducted for prenatal exposure by non-target screening analysis of maternal and cord blood samples, which annotated 98 compounds as endogenous and exogenous sources, e.g., endogenous fatty acids (interaction between PFAS and fatty acids) in both maternal and cord samples. These non-target screening works can help with the demonstration of the presence of exposure sources among different chemicals relevant to human health.
In environmental samples, Peng, et al.97 developed a screening method using HRMS to identify unknown natural and synthetic organo-bromine compounds (NSOBCs) in lake sediment samples. This method resulted in the identification of 1593 new NSOBCs. Additionally, NSOBC peak abundances in sediment core samples can be analyzed using hierarchical cluster analysis, revealing temporal trends in peak abundance that are influenced by anthropogenic activities.98 Similar untargeted methods can also be used to compare characteristic fragments between different samples. For instance, iodinated organic compounds can be identified in both freshwater and marine sediments.99 Furthermore, an enhanced nontarget screening algorithm using the Toxic Substance Control Act (TSCA) database has been employed to screen indoor pollutants, leading to the annotation of 18 previously unrecognized chlorinated azo dyes as chlorinated analogs derived from Diperse Violet 93 and Diperse Blue 373.100 However, caution should be exercised when using large databases in non-targeted methods, as they might decrease sensitivity, considering different chemical responses in mass spectrum with several orders of magnitude for very similar compounds.
Recently, a new method called “Affinity Purification with Non-target screening (APNA)” has been developed for the “top-down” screening of toxic substances from environmental complexes.101,102 This method follows the principles of biochemistry, using a tagged protein (e.g., NR) as a bait to isolate bioactive pollutants directly from extracts of environmental samples containing thousands of compounds. Fig. 1c outlines the general APNA workflow. In summary, environmental cocktail samples are extracted using a suitable method (Procedure I), and then the extracts are incubated with Escherichia coli lysates that overexpress a polyhistidine-tagged protein of interest. Bioactive ligands bound to the polyhistidine-tagged protein are then isolated using a two-step approach: affinity purification (Procedure II) and nondenaturing elution (Procedure III). Finally, the structures of the isolated ligands are identified using HRMS-based non-target screening, combined with nuclear magnetic resonance (NMR) spectroscopy and X-ray crystallography for identification of the general structure, and ion mobility spectrometry for positional isomers and cis–trans isomers if needed (Procedure IV).
The APNA method has been used to screen for major contributors to peroxisome proliferator-activated receptor γ (PPARγ) activity in oil sands process-affected water (OSPW). Although the toxic effects of OSPW exposure have been reported,103 little was known about the specific toxic chemicals causing adverse effects due to the complexity of OSPW.104 By applying the APNA method, hydroxylated carboxylic and sulfonic acids were identified as major drivers of the PPARγ-mediated effects of OSPW (Fig. 2a).101 In aqueous film-forming foam (AFFF) products and AFFF-contaminated surface waters, 31 unknown polyfluoroalkyl ligands of human liver fatty acid binding protein (hL-FABP) were identified (Fig. 2b).105 While most of these ligands do not have standard substances, tentative identities were determined based on extract mass, secondary mass spectra, and retention time. The APNA method could explain the unknown toxicity of known contaminants. For instance, the liver X receptor α (LXRα) antagonist activity of triphenyl phosphate (TPHP) (Fig. 2c) was found in vivo by promoting the development of atherosclerotic lesions in ApoE-/- model mice, independently validating the APNA method. This method was also applied to other chemicals.106,107 Another example is the potent antibacterial compound 6-OH-BDE-47 (6-hydroxy-2,2′,4,4′-tetrabromodiphenyl ether), which acts by inhibiting enoyl-acyl carrier protein reductase (FabI) (Fig. 2d) as determined by APNA combined with chemical proteomics. Furthermore, 6-OH-BDE-47 was found to function as a chemical defense molecule in marine sponges by selectively killing the sponge microbiome carrying FabI.108
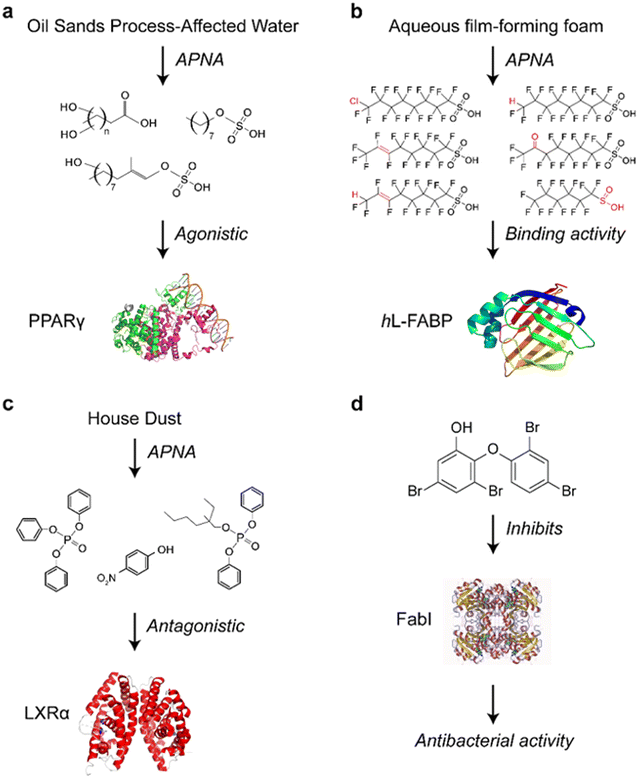 |
| Fig. 2 Examples of the application of the APNA method: (a) unknown hydroxylated carboxylic and sulfonic acids were identified as the major drivers for the PPARγ activity from complex OSPW samples; (b) identification of hL-FABP ligands in AFFF with the absence of standard substances; (c) toxicological mechanism of TPHP in LXRα antagonism; (d) 6-OH-BDE47 was found to be an inhibitor of FabI. This graph is modified from previous publications.101,102,105,107,108 | |
5.2 Machine-learning approaches
Nowadays, machine learning has been used in spheres of prediction of the risk of chemical mixture, qualification of suspect compounds in the absence of analytical standards, and chemical behavior for human health (Fig. 3).
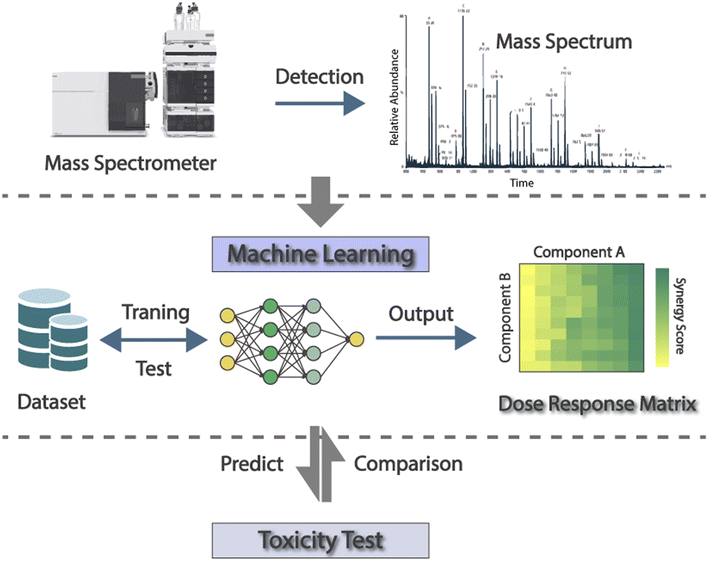 |
| Fig. 3 The general workflow of machine learning approaches for the toxicity assessment of. | |
The small molecules, e.g., emerging contaminants and their metabolites, have been largely elucidated by HRMS-based suspect and non-targeted screening analysis, whereas the confidence depended on the HRMS identification may not always be possible for different substances, which causes a not accurate semi-quantification for these suspects. Traditionally, analytical standards are used to quantify different chemicals in LC-HRMS as compensation for a broad range of ionization efficiency (IE). IE can be quite variable among the similar structures of chemicals such as polychlorinated biphenyls.109 Therefore, even if some compounds can be quantified based on their homologs, the signal of the homolog can be significantly higher than the estimated compound under the same conditions of the machine.109 Recently, machine learning models combining molecular and eluent descriptors showed the potential to predict IE.110 Lauria, et al.110 used a gradient-boosted tree-based model to train the absolute response factors in negative mode for prediction of IE with the validation of 33 per- and polyfluoroalkyl substances (PFAS). This method was first applied to the liver of marine mammals (pilot whales and white-beaked dolphins) to estimate the concentrations of unidentified suspect PFAS. Another artificial neural network also used physicochemical descriptors of database-matched chemicals to predict the transplacental transfer of PFAS as a function based on their physicochemical properties.111
Furthermore, another machine learning method has recently been developed for diagnostic purposes in chemical forensic analyses using non-target chemical data.112–114 The underlying hypothesis in chemical forensic analysis is that the chemical composition of a sample, at least to some extent, contains information about its source or the environmental conditions it was exposed to.115 This selection process for a subset of chemical features is often referred to as “chemical fingerprints.” However, choosing a subset of diagnostic chemical features from a pool of thousands of potential features can be challenging. Dávila-Santiago, et al.112 proposed a forensic workflow to overcome this obstacle. The critical thresholds in this workflow are data-dependent and serve the needs of the investigation. Dávila-Santiago, et al.112 suggested two general rules: (1) the initial tuning should achieve a balanced accuracy of >50%.; (2) when using only the diagnostic features, the balanced accuracy should increase when using the top n diagnostic features compared to using all the features. This workflow enables the simultaneous quantification of processes occurring among thousands of organic molecules within a single sample, such as dissolved organic contaminants in a water sample. It provides diagnostic chemical signatures for each process, allowing for better detection of pollution sources at trace levels in the environment. However, non-targeted screening methods still rely on the different large chemical databases and putative structures; and the high-throughput data from non-targeted screening methods cannot be quantified by off-the-shelf detectors, wherein the machine learning may not fully consider their transformation, which might show a quite different toxicity compared to their parent compounds.
A framework was to create a robust machine learning model that can accurately predict the risk of algal toxicity caused by mixtures of contaminants.116 This model examines the nonlinear relationship between the effect sizes of contaminant number, temperature, water quality parameters, and land use metrics on the ecological functions of algae, invertebrates, and fish. The tests were carried out under various lab conditions and field sites, totaling more than 900 locations worldwide. The results of this study confirmed that machine learning methods can serve as excellent and flexible tools for toxicologists and policy-makers. A smaller scale of machine learning was developed to discriminate mixtures of EDCs using capacitance spectra obtained by a two-dimensional molybdenum disulfide (MoS2)-based impedimetric electronic tongue (e-tongue).117 Three models, namely the multilayer perceptron (MLP), random forest, and extreme gradient boosting (XGBoost), were employed for multi-targeted regression to predict the levels of individual compounds and their mixtures. The study found that XGBoost outperformed random forest and neural networks, largely due to its basis in Gradient Boosting Machines (GBMs), which boost weak learners through optimizations and algorithmic enhancements.118 In a similar study, artificial neural networks, and random forest models were used to predict temporal changes in the bioavailability and toxicity of complex chemical mixtures in soils contaminated with petroleum hydrocarbons and metal(loid)s.119 The models were trained using empirical data such as soil type, bioavailable concentration, and time. However, it should be noted that this model may not accurately predict toxicity beyond 180 days due to limitations in the dataset and other input variables. To overcome this limitation, the use of more contaminant toxicity datasets or additional empirical data is recommended when applying machine learning methods to predict toxicity.
6. Conclusion and future directions
Due to the highly complex mixtures of emerging contaminants in the environment, either individually or through their interactions, make it challenging to establish model specifications, exposure levels, and toxic reference values. The current lack of analytical standards for different compounds makes the difficult to determine the chemical groups and sources. Although the current studies in recent years have been focused on different methods of non-target screen analysis for identification, semi-quantification of similar structure chemicals, and machine learning of chemical behavior, it is still essential to carefully interpret the results without adequate information on chemicals. Exposure to toxic chemicals can cause health problems by interacting with biomolecules, known as molecular initiating events, which can disrupt various downstream physiological processes.120 Although small molecular chemicals, such as PAHs121 and aromatic amines,122 attack nucleotides, and others, e.g., halogenated benzene,123 target cell membranes, proteins also play a role in mediating adverse effects of contaminants.124 However, the current non-target screening method still focuses on PFAS-relevant compounds while the interactions between emerging chemicals and proteins are still not clear by the current methods. Besides, current assessment methods and frameworks for mixture chemicals need to further consider incorporating unknown or suspect chemicals into machine learning algorithms for predicting the fate and behavior in environmental and biological samples.
Author contributions
Yu Cheng wrote the major part of this review. Jue Ding wrote chapter 4.2 and did the visualization. Catherine Estefany Davila Arenas helped reference collection and revised chapters 3 and 6. Markus Brinkmann provided the concept and revised the manuscript. Xiaowen Ji designed the frame of this review, gathered the references, and revised the manuscript.
Conflicts of interest
There are no conflicts to declare.
Acknowledgements
Dr Markus Brinkmann is currently a faculty member of the Global Water Futures (GWF) program, which received funds from the Canada First Research Excellence Funds (CFREF). Dr Xiaowen Ji was supported through a GWF PhD Excellence Scholarship. This work was funded by the National Key Research and Development Program of China (No 2023YFC3208900 & 2023YFC3208902), the National Natural Science Foundation of China (No 52039003 & 52209097), and the Natural Science Foundation of Jiangsu Province (BK20210953 & BK20220208).
References
- Z. Wang, G. W. Walker, D. C. G. Muir and K. Nagatani-Yoshida, Toward a Global Understanding of Chemical Pollution: A First Comprehensive Analysis of National and Regional Chemical Inventories, Environ. Sci. Technol., 2020, 54(5), 2575–2584 CrossRef CAS PubMed.
- J. Hellou, Behavioural ecotoxicology, an “early warning” signal to assess environmental quality, Environ. Sci. Pollut. Res., 2011, 18(1), 1–11 CrossRef CAS PubMed.
- Z. Tang, J. Zhang, Q. Zhou, S. Xu, Z. Cai and G. Jiang, Thyroid Cancer “Epidemic”: A Socio-Environmental Health Problem Needs Collaborative Efforts, Environ. Sci. Technol., 2020, 54(7), 3725–3727 CrossRef CAS PubMed.
- M. Saaristo, T. Brodin, S. Balshine, M. G. Bertram, B. W. Brooks, S. M. Ehlman, E. S. McCallum, A. Sih, J. Sundin, B. B. M. Wong and K. E. Arnold, Direct and indirect effects of chemical contaminants on the behaviour, ecology and evolution of wildlife, Proc. R. Soc. B, 2018, 285(1885), 20181297 CrossRef PubMed.
- V. Ducrot, E. Billoir, A. R. R. Péry, J. Garric and S. Charles, From Individual to Population Level Effects of Toxicants in the Tubicifid Branchiura sowerbyi Using Threshold Effect Models in a Bayesian Framework, Environ. Sci. Technol., 2010, 44(9), 3566–3571 CrossRef CAS PubMed.
- J. Klaminder, G. Hellström, J. Fahlman, M. Jonsson, J. Fick, A. Lagesson, E. Bergman and T. Brodin, Drug-Induced Behavioral Changes: Using Laboratory Observations to Predict Field Observations, Front. Environ. Sci., 2016, 4, 81 Search PubMed.
- R. A. Relyea, A cocktail of contaminants: how mixtures of pesticides at low concentrations affect aquatic communities, Oecologia, 2009, 159(2), 363–376 CrossRef PubMed.
- G. L. Daly, Y. D. Lei, C. Teixeira, D. C. G. Muir, L. E. Castillo and F. Wania, Accumulation of Current-Use Pesticides in Neotropical Montane Forests, Environ. Sci. Technol., 2007, 41(4), 1118–1123 CrossRef CAS PubMed.
- K. J. Hageman, S. L. Simonich, D. H. Campbell, G. R. Wilson and D. H. Landers, Atmospheric Deposition of Current-Use and Historic-Use Pesticides in Snow at National Parks in the Western United States, Environ. Sci. Technol., 2006, 40(10), 3174–3180 CrossRef CAS PubMed.
- M. N. Moore, R. J. Wedderburn, K. R. Clarke, I. R. B. McFadzen, D. M. Lowe and J. W. Readman, Emergent synergistic lysosomal toxicity of chemical mixtures in molluscan blood cells (hemocytes), Environ. Pollut., 2018, 235, 1006–1014 CrossRef CAS PubMed.
- Z. Li and A. Jennings, Worldwide Regulations of Standard Values of Pesticides for Human Health Risk Control: A Review, Int. J. Environ. Res. Public Health, 2017, 14(7), 826 CrossRef PubMed.
-
World Health O., Guidelines for Drinking-Water Quality, World Health Organization, 2004, vol. 1 Search PubMed.
-
United States. Environmental Protection Agency, Water Planning, D., Results of the Nationwide Urban Runoff Program, Water Planning Division, US Environmental Protection Agency, 1982, vol. 1 Search PubMed.
- P. L. Toccalino, J. E. Norman and J. C. Scott, Chemical mixtures in untreated water from public-supply wells in the U.S. — Occurrence, composition, and potential toxicity, Sci. Total Environ., 2012, 431, 262–270 CrossRef CAS PubMed.
- M.-S. Christin, A. D. Gendron, P. Brousseau, L. Ménard, D. J. Marcogliese, D. Cyr, S. Ruby and M. Fournier, Effects of agricultural pesticides on the immune system of Rana pipiens and on its resistance to parasitic infection, Environ. Toxicol. Chem., 2003, 22(5), 1127–1133 CrossRef CAS PubMed.
- M. S. Christin, L. Ménard, A. D. Gendron, S. Ruby, D. Cyr, D. J. Marcogliese, L. Rollins-Smith and M. Fournier, Effects of agricultural pesticides on the immune system of Xenopus laevis and Rana pipiens, Aquat. Toxicol., 2004, 67(1), 33–43 CrossRef CAS PubMed.
- B. Hayes Tyrone, P. Case, S. Chui, D. Chung, C. Haeffele, K. Haston, M. Lee, P. Mai Vien, Y. Marjuoa, J. Parker and M. Tsui, Pesticide Mixtures, Endocrine Disruption, and Amphibian Declines: Are We Underestimating the Impact?, Environ. Health Perspect., 2006, 114(suppl 1), 40–50 CrossRef PubMed.
- R. P. A. van Wijngaarden, J. G. M. Cuppen, G. H. P. Arts, S. J. H. Crum, M. W. van den Hoorn, P. J. van den Brink and T. C. M. Brock, Aquatic risk assessment of a realistic exposure to pesticides used in bulb crops: A microcosm study, Environ. Toxicol. Chem., 2004, 23(6), 1479–1498 CrossRef CAS PubMed.
- L. Wendt-Rasch, P. J. Van den Brink, S. J. H. Crum and P. Woin, The effects of a pesticide mixture on aquatic ecosystems differing in trophic status: responses of the macrophyte Myriophyllum spicatum and the periphytic algal community, Ecotoxicol. Environ. Saf., 2004, 57(3), 383–398 CrossRef CAS PubMed.
- L. Gross, Diverse Toxic Chemicals Disrupt Cell Function through a Common Path, PLoS Biol., 2007, 5(2), e41 CrossRef PubMed.
- A. Lahiani, E. Yavin and P. Lazarovici, The Molecular Basis of Toxins’ Interactions with Intracellular Signaling via Discrete Portals, Toxins, 2017, 9(3), 107 CrossRef PubMed.
- M. E. Meek, A. R. Boobis, K. M. Crofton, G. Heinemeyer, M. Van Raaij and C. Vickers, Risk assessment of combined exposure to multiple chemicals: A WHO/IPCS framework, Regul. Toxicol. Pharmacol., 2011, 60(2), S1–S14 CrossRef CAS PubMed.
- N. Cedergreen, Quantifying Synergy: A Systematic Review of Mixture Toxicity Studies within Environmental Toxicology, PLoS One, 2014, 9(5), e96580 CrossRef PubMed.
- A. Kienzler, S. K. Bopp, S. van der Linden, E. Berggren and A. Worth, Regulatory assessment of chemical mixtures: Requirements, current approaches and future perspectives, Regul. Toxicol. Pharmacol., 2016, 80, 321–334 CrossRef CAS PubMed.
- R. J. Tallarida, Revisiting the Isobole and Related Quantitative Methods for Assessing Drug Synergism, J. Pharmacol. Exp. Ther., 2012, 342(1), 2 CrossRef CAS PubMed.
- R. J. Tallarida, Drug Combinations: Tests and Analysis with Isoboles, Curr. Protoc. Pharmacol., 2016, 72(1), 9.19.1–9.19.19 Search PubMed.
- J. Y. M. Tang, F. Busetti, J. W. A. Charrois and B. I. Escher, Which chemicals drive biological effects in wastewater and recycled water?, Water Res., 2014, 60, 289–299 CrossRef CAS PubMed.
- A. LeBlanc Gerald and W. Olmstead Allen, Evaluating the Toxicity of Chemical Mixtures, Environ. Health Perspect., 2004, 112(13), A729–A730 CAS.
- K. A. Heys, R. F. Shore, M. G. Pereira, K. C. Jones and F. L. Martin, Risk assessment of environmental mixture effects, RSC Adv., 2016, 6(53), 47844–47857 RSC.
- R. Altenburger, M. Nendza and G. Schüürmann, Mixture toxicity and its modeling by quantitative structure-activity relationships, Environ. Toxicol. Chem., 2003, 22(8), 1900–1915 CrossRef CAS PubMed.
- M. Prins, P. J. Veugelers and for the European Seroconverter Study the Tricontinental Seroconverter S., Comparison of progression and non-progression in injecting drug users and homosexual men with documented dates of HIV-1 seroconversion, AIDS, 1997, 11(5), 621–631 CrossRef CAS PubMed.
- L. Song, W. Xia, Z. Zhou, Y. Li, Y. Lin, J. Wei, Z. Wei, B. Xu, J. Shen, W. Li and S. Xu, Low-level phenolic estrogen pollutants impair islet morphology and β-cell function in isolated rat islets, J. Endocrinol., 2012, 215(2), 303–311 CAS.
- L. Filby Amy, T. Neuparth, L. Thorpe Karen, R. Owen, S. Galloway Tamara and R. Tyler Charles, Health Impacts of Estrogens in the Environment, Considering Complex Mixture Effects, Environ. Health Perspect., 2007, 115(12), 1704–1710 CrossRef CAS PubMed.
- M. N. Moore, D. M. Lowe, C. Soverchia, S. D. Haigh and S. G. Hales, Uptake of a non-calorific, edible sucrose polyester oil and olive oil by marine mussels and their influence on uptake and effects of anthracene, Aquat. Toxicol., 1997, 39(3), 307–320 CrossRef CAS.
- H. Li, J. Xiao, Q. Fu and X. Bao, Confined catalysis under two-dimensional materials, Proc. Natl. Acad. Sci. U. S. A., 2017, 114(23), 5930–5934 CrossRef CAS PubMed.
- Z.-l. Zhu, S.-c. Wang, F.-f. Zhao, S.-g. Wang, F.-f. Liu and G.-z. Liu, Joint toxicity of microplastics with triclosan to marine microalgae Skeletonema costatum, Environ. Pollut., 2019, 246, 509–517 CrossRef CAS PubMed.
- B. Huang, Z.-B. Wei, L.-Y. Yang, K. Pan and A.-J. Miao, Combined Toxicity of Silver Nanoparticles with Hematite or Plastic Nanoparticles toward Two Freshwater Algae, Environ. Sci. Technol., 2019, 53(7), 3871–3879 CrossRef CAS PubMed.
- Y. Zhou, X. Liu and J. Wang, Ecotoxicological effects of microplastics and cadmium on the earthworm Eisenia foetida, J. Hazard. Mater., 2020, 392, 122273 CrossRef CAS PubMed.
- R. Qiao, K. Lu, Y. Deng, H. Ren and Y. Zhang, Combined effects of polystyrene microplastics and natural organic matter on the accumulation and toxicity of copper in zebrafish, Sci. Total Environ., 2019, 682, 128–137 CrossRef CAS PubMed.
- W. S. Lee, H.-J. Cho, E. Kim, Y. H. Huh, H.-J. Kim, B. Kim, T. Kang, J.-S. Lee and J. Jeong, Bioaccumulation of polystyrene nanoplastics and their effect on the toxicity of Au ions in zebrafish embryos, Nanoscale, 2019, 11(7), 3173–3185 RSC.
- M. Oliveira, A. Ribeiro, K. Hylland and L. Guilhermino, Single and combined effects of microplastics and pyrene on juveniles (0+ group) of the common goby Pomatoschistus microps (Teleostei, Gobiidae), Ecol. Indic., 2013, 34, 641–647 CrossRef CAS.
- E. L. Schymanski, J. Jeon, R. Gulde, K. Fenner, M. Ruff, H. P. Singer and J. Hollender, Identifying Small Molecules via High Resolution Mass Spectrometry: Communicating Confidence, Environ. Sci. Technol., 2014, 48(4), 2097–2098 CrossRef CAS PubMed.
- S. Wang, H. Zhang, W. Zheng, X. Wang, M. E. Andersen, J. Pi, G. He and W. Qu, Organic Extract Contaminants from Drinking Water Activate Nrf2-Mediated Antioxidant Response in a Human Cell Line, Environ. Sci. Technol., 2013, 47(9), 4768–4777 CrossRef CAS PubMed.
- M. Ema, N. Ohe, M. Suzuki, J. Mimura, K. Sogawa, S. Ikawa and Y. Fujii-Kuriyama, Dioxin binding activities of polymorphic forms of mouse and human arylhydrocarbon receptors, J. Biol. Chem., 1994, 269(44), 27337–27343 CrossRef CAS PubMed.
- S. Sauban Ghani, A comprehensive review of database resources in chemistry, Ecletica Quim., 2020, 45(3), 57–68 CrossRef.
- P. P. Egeghy, R. Judson, S. Gangwal, S. Mosher, D. Smith, J. Vail and E. A. Cohen Hubal, The exposure data landscape for manufactured chemicals, Sci. Total Environ., 2012, 414, 159–166 CrossRef CAS PubMed.
- A. C. Johnson, X. Jin, N. Nakada and J. P. Sumpter, Learning from the past and considering the future of chemicals in the environment, Science, 2020, 367(6476), 384–387 CrossRef CAS PubMed.
- S. Strempel, M. Scheringer, C. A. Ng and K. Hungerbühler, Screening for PBT Chemicals among the “Existing” and “New” Chemicals of the EU, Environ. Sci. Technol., 2012, 46(11), 5680–5687 CrossRef CAS PubMed.
- C. A. M. La Porta, M. R. Fumagalli, S. Gomarasca, M. C. Lionetti, S. Zapperi and S. Bocchi, Synergistic effects of contaminants in Lombardy waters, Sci. Rep., 2021, 11(1), 13888 CrossRef CAS PubMed.
- N. M. Sopinka, J. R. Marentette and S. Balshine, Impact of contaminant exposure on resource contests in an invasive fish, Behavioral Ecology and Sociobiology, 2010, 64(12), 1947–1958 CrossRef.
- E. S. McCallum, E. Krutzelmann, T. Brodin, J. Fick, A. Sundelin and S. Balshine, Exposure to wastewater effluent affects fish behaviour and tissue-specific uptake of pharmaceuticals, Sci. Total Environ., 2017, 605–606, 578–588 CrossRef CAS PubMed.
- A. Kortenkamp, Ten Years of Mixing Cocktails: A Review of Combination Effects of Endocrine-Disrupting Chemicals, Environ. Health Perspect., 2007, 115(suppl 1), 98–105 CrossRef PubMed.
- J. M. Leese, J. McMahon and J. C. Colosi, Effects of Wastewater Treatment Plant Effluent in a Receiving Stream on Reproductive Behavior of Fathead Minnows (Pimephales promelas), Fishes, 2021, 6(2), 14 CrossRef.
- K. L. Thorpe, R. I. Cummings, T. H. Hutchinson, M. Scholze, G. Brighty, J. P. Sumpter and C. R. Tyler, Relative Potencies and Combination Effects of Steroidal Estrogens in Fish, Environ. Sci. Technol., 2003, 37(6), 1142–1149 CrossRef CAS PubMed.
- M. Muz, M. Krauss, S. Kutsarova, T. Schulze and W. Brack, Mutagenicity in Surface Waters: Synergistic Effects of Carboline Alkaloids and Aromatic Amines, Environ. Sci. Technol., 2017, 51(3), 1830–1839 CrossRef CAS PubMed.
- P. M. Bradley, C. A. Journey, J. P. Berninger, D. T. Button, J. M. Clark, S. R. Corsi, L. A. DeCicco, K. G. Hopkins, B. J. Huffman, N. Nakagaki, J. E. Norman, L. H. Nowell, S. L. Qi, P. C. VanMetre and I. R. Waite, Mixed-chemical exposure and predicted effects potential in wadeable southeastern USA streams, Sci. Total Environ., 2019, 655, 70–83 CrossRef CAS PubMed.
- S. Hasenbein, H. Poynton and R. E. Connon, Contaminant exposure effects in a changing climate: how multiple stressors can multiply exposure effects in the amphipod Hyalella azteca, Ecotoxicology, 2018, 27(7), 845–859 CrossRef CAS PubMed.
- V. Brian Jayne, A. Harris Catherine, M. Scholze, T. Backhaus, P. Booy, M. Lamoree, G. Pojana, N. Jonkers, T. Runnalls, A. Bonfà, A. Marcomini and P. Sumpter John, Accurate Prediction of the Response of Freshwater Fish to a Mixture of Estrogenic Chemicals, Environ. Health Perspect., 2005, 113(6), 721–728 CrossRef CAS PubMed.
- L. Yang, Y.-X. Feng, H. Zhang and X.-Z. Yu, Estimating the synergistic and antagonistic effects of dual antibiotics on plants through root elongation test, Ecotoxicology, 2021, 30(8), 1598–1609 CrossRef CAS PubMed.
- Y. Liu, J. Zhang, B. Gao and S. Feng, Combined effects of two antibiotic contaminants on Microcystis aeruginosa, J. Hazard. Mater., 2014, 279, 148–155 CrossRef CAS PubMed.
- E. Chung, M. C. Genco, L. Megrelis and J. V. Ruderman, Effects of bisphenol A and triclocarban on brain-specific expression of aromatase in early zebrafish embryos, Proc. Natl. Acad. Sci. U. S. A., 2011, 108(43), 17732–17737 CrossRef CAS PubMed.
- L. Søfteland, J. A. Kirwan, T. S. F. Hori, T. R. Størseth, U. Sommer, M. H. G. Berntssen, M. R. Viant, M. L. Rise, R. Waagbø, B. E. Torstensen, M. Booman and P. A. Olsvik, Toxicological effect of single contaminants and contaminant mixtures associated with plant ingredients in novel salmon feeds, Food Chem. Toxicol., 2014, 73, 157–174 CrossRef PubMed.
- B. Kopp, P. Sanders, I. Alassane-Kpembi, V. Fessard, D. Zalko, L. Le Hégarat and M. Audebert, Synergic toxic effects of food contaminant mixtures in human cells, Mutagenesis, 2020, 35(5), 415–424 CrossRef CAS PubMed.
- J. Yuan, S. Che, L. Zhang, X. Li, J. Yang, X. Sun and Z. Ruan, Assessing the combinatorial cytotoxicity of the exogenous contamination with BDE-209, bisphenol A, and acrylamide via high-content analysis, Chemosphere, 2021, 284, 131346 CrossRef CAS PubMed.
- S. Iburg, I. Nybom, S. Bonaglia, A. M. Karlson, A. Sobek and F. J. Nascimento, Organic Contaminant Mixture Significantly Changes Microbenthic Community Structure and Increases the Expression of PAH Degradation Genes, Front. Environ. Sci., 2020, 8, 128 CrossRef.
- K. Vejdovszky, K. Hahn, D. Braun, B. Warth and D. Marko, Synergistic estrogenic effects of Fusarium and Alternaria mycotoxins in vitro, Arch. Toxicol., 2017, 91(3), 1447–1460 CrossRef CAS PubMed.
- Y. Zhang, M. Liu, B. Peng, S. Jia, D. Koh, Y. Wang, H. S. Cheng, N. S. Tan, B. Warth, D. Chen and M. Fang, Impact of Mixture Effects between Emerging Organic Contaminants on Cytotoxicity: A Systems Biological Understanding of Synergism between Tris(1,3-dichloro-2-propyl)phosphate and Triphenyl Phosphate, Environ. Sci. Technol., 2020, 54(17), 10722–10734 CrossRef CAS PubMed.
- B. I. Escher, H. M. Stapleton and E. L. Schymanski, Tracking complex mixtures of chemicals in our changing environment, Science, 2020, 367(6476), 388–392 CrossRef CAS PubMed.
- D. L. Villeneuve, K. Coady, B. I. Escher, E. Mihaich, C. A. Murphy, T. Schlekat and N. Garcia-Reyero, High-throughput screening and environmental risk assessment: State of the science and emerging applications, Environ. Toxicol. Chem., 2019, 38(1), 12–26 CrossRef CAS PubMed.
- J. Lundqvist, A. Andersson, A. Johannisson, E. Lavonen, G. Mandava, H. Kylin, D. Bastviken and A. Oskarsson, Innovative drinking water treatment techniques reduce the disinfection-induced oxidative stress and genotoxic activity, Water Res., 2019, 155, 182–192 CrossRef CAS PubMed.
- W. Brack, S. A. Aissa, T. Backhaus, V. Dulio, B. I. Escher, M. Faust, K. Hilscherova, J. Hollender, H. Hollert, C. Müller, J. Munthe, L. Posthuma, T.-B. Seiler, J. Slobodnik, I. Teodorovic, A. J. Tindall, G. de Aragão Umbuzeiro, X. Zhang and R. Altenburger, Effect-based methods are key. The European Collaborative Project SOLUTIONS recommends integrating effect-based methods for diagnosis and monitoring of water quality, Environ. Sci. Eur., 2019, 31(1), 10 CrossRef.
- R. Kavlock, K. Chandler, K. Houck, S. Hunter, R. Judson, N. Kleinstreuer, T. Knudsen, M. Martin, S. Padilla, D. Reif, A. Richard, D. Rotroff, N. Sipes and D. Dix, Update on EPA's ToxCast Program: Providing High Throughput Decision Support Tools for Chemical Risk Management, Chem. Res. Toxicol., 2012, 25(7), 1287–1302 Search PubMed.
- B. I. Escher and K. Fenner, Recent Advances in Environmental Risk Assessment of Transformation Products, Environ. Sci. Technol., 2011, 45(9), 3835–3847 CrossRef CAS PubMed.
- C. H. Hurst and D. J. Waxman, Activation of PPARα and PPARγ by Environmental Phthalate Monoesters, Toxicol. Sci., 2003, 74(2), 297–308 CrossRef CAS PubMed.
- F. Li, Q. Xie, X. Li, N. Li, P. Chi, J. Chen, Z. Wang and C. Hao, Hormone Activity of Hydroxylated Polybrominated Diphenyl Ethers on Human Thyroid Receptor-β: In Vitro and In Silico Investigations, Environ. Health Perspect., 2010, 118(5), 602–606 CrossRef CAS PubMed.
- H. Dong, A. A. Cuthbertson and S. D. Richardson, Effect-Directed Analysis (EDA): A Promising Tool for Nontarget Identification of Unknown Disinfection Byproducts in Drinking Water, Environ. Sci. Technol., 2020, 54(3), 1290–1292 CrossRef CAS PubMed.
- W. Brack, S. Ait-Aissa, R. M. Burgess, W. Busch, N. Creusot, C. Di Paolo, B. I. Escher, L. Mark Hewitt, K. Hilscherova, J. Hollender, H. Hollert, W. Jonker, J. Kool, M. Lamoree, M. Muschket, S. Neumann, P. Rostkowski, C. Ruttkies, J. Schollee, E. L. Schymanski, T. Schulze, T.-B. Seiler, A. J. Tindall, G. De Aragão Umbuzeiro, B. Vrana and M. Krauss, Effect-directed analysis supporting monitoring of aquatic environments — An in-depth overview, Sci. Total Environ., 2016, 544, 1073–1118 CrossRef CAS PubMed.
- Z. Tian, H. Zhao, K. T. Peter, M. Gonzalez, J. Wetzel, C. Wu, X. Hu, J. Prat, E. Mudrock, R. Hettinger, A. E. Cortina, R. G. Biswas, F. V. C. Kock, R. Soong, A. Jenne, B. Du, F. Hou, H. He, R. Lundeen, A. Gilbreath, R. Sutton, N. L. Scholz, J. W. Davis, M. C. Dodd, A. Simpson, J. K. McIntyre and E. P. Kolodziej, A ubiquitous tire rubber–derived chemical induces acute mortality in coho salmon, Science, 2021, 371(6525), 185–189 CrossRef CAS PubMed.
- E. Simon, M. van Velzen, S. H. Brandsma, E. Lie, K. Løken, J. de Boer, J. Bytingsvik, B. M. Jenssen, J. Aars, T. Hamers and M. H. Lamoree, Effect-Directed Analysis To Explore the Polar Bear Exposome: Identification of Thyroid Hormone Disrupting Compounds in Plasma, Environ. Sci. Technol., 2013, 47(15), 8902–8912 CrossRef CAS PubMed.
- T. Backhaus, Å. Arrhenius and H. Blanck, Toxicity of a Mixture of Dissimilarly Acting Substances to Natural Algal Communities: Predictive Power and Limitations of Independent Action and Concentration Addition, Environ. Sci. Technol., 2004, 38(23), 6363–6370 CrossRef CAS PubMed.
- T. Backhaus and M. Faust, Predictive Environmental Risk Assessment of Chemical Mixtures: A Conceptual Framework, Environ. Sci. Technol., 2012, 46(5), 2564–2573 CrossRef CAS PubMed.
- A. Kortenkamp and M. Faust, Combined exposures to anti-androgenic chemicals: steps towards cumulative risk assessment, Int. J. Androl., 2010, 33(2), 463–474 CrossRef CAS PubMed.
- B. Escher, G. Braun and C. Zarfl, Exploring the Concepts of Concentration Addition and Independent Action Using a Linear Low-Effect Mixture Model, Environ. Toxicol. Chem., 2020, 39(12), 2552–2559 CrossRef CAS PubMed.
- X. Han and P. S. Price, Determining the Maximum Cumulative Ratios for Mixtures Observed in Ground Water Wells Used as Drinking Water Supplies in the United States, Int. J. Environ. Res. Public Health, 2011, 8, 4729–4745 CrossRef CAS PubMed.
- N. Vallotton and P. S. Price, Use of the Maximum Cumulative Ratio As an Approach for Prioritizing Aquatic Coexposure to Plant Protection Products: A Case Study of a Large Surface Water Monitoring Database, Environ. Sci. Technol., 2016, 50(10), 5286–5293 CrossRef CAS PubMed.
- L. Dewalque, C. Charlier and C. Pirard, Estimated daily intake and cumulative risk assessment of phthalate diesters in a Belgian general population, Toxicol. Lett., 2014, 231(2), 161–168 CrossRef CAS PubMed.
- E. Malaj, P. C. von der Ohe, M. Grote, R. Kühne, C. P. Mondy, P. Usseglio-Polatera, W. Brack and R. B. Schäfer, Organic chemicals jeopardize the health of freshwater ecosystems on the continental scale, Proc. Natl. Acad. Sci. U. S. A., 2014, 111(26), 9549–9554 CrossRef CAS PubMed.
- M. Junghans, T. Backhaus, M. Faust, M. Scholze and L. H. Grimme, Application and validation of approaches for the predictive hazard assessment of realistic pesticide mixtures, Aquat. Toxicol., 2006, 76(2), 93–110 CrossRef CAS PubMed.
- M. C. Kennedy, C. R. Glass, B. Bokkers, A. D. M. Hart, P. Y. Hamey, J. W. Kruisselbrink, W. J. de Boer, H. van der Voet, D. G. Garthwaite and J. D. van Klaveren, A European model and case studies for aggregate exposure assessment of pesticides, Food Chem. Toxicol., 2015, 79, 32–44 CrossRef CAS PubMed.
- L. H. Nowell, J. E. Norman, P. W. Moran, J. D. Martin and W. W. Stone, Pesticide Toxicity Index—A tool for assessing potential toxicity of pesticide mixtures to freshwater aquatic organisms, Sci. Total Environ., 2014, 476–477, 144–157 CrossRef CAS PubMed.
- C. Delmaar, B. Bokkers, W. ter Burg and G. Schuur, Validation of an aggregate exposure model for substances in consumer products: a case study of diethyl phthalate in personal care products, J. Exposure Sci. Environ. Epidemiol., 2015, 25(3), 317–323 CrossRef CAS PubMed.
- I. Gosens, C. J. E. Delmaar, W. ter Burg, C. de Heer and A. G. Schuur, Aggregate exposure approaches for parabens in personal care products: a case assessment for children between 0 and 3 years old, J. Exposure Sci. Environ. Epidemiol., 2014, 24(2), 208–214 CrossRef CAS PubMed.
- P. E. Boon, G. van Donkersgoed, D. Christodoulou, A. Crépet, L. D'Addezio, V. Desvignes, B.-G. Ericsson, F. Galimberti, E. Ioannou-Kakouri, B. H. Jensen, I. Rehurkova, J. Rety, J. Ruprich, S. Sand, C. Stephenson, A. Strömberg, A. Turrini, H. van der Voet, P. Ziegler, P. Hamey and J. D. van Klaveren, Cumulative dietary exposure to a selected group of pesticides of the triazole group in different European countries according to the EFSA guidance on probabilistic modelling, Food Chem. Toxicol., 2015, 79, 13–31 CrossRef CAS PubMed.
- M. Krauss, H. Singer and J. Hollender, LC–high resolution MS in environmental analysis: from target screening to the identification of unknowns, Anal. Bioanal. Chem., 2010, 397(3), 943–951 CrossRef CAS PubMed.
- J. E. Rager, M. J. Strynar, S. Liang, R. L. McMahen, A. M. Richard, C. M. Grulke, J. F. Wambaugh, K. K. Isaacs, R. Judson, A. J. Williams and J. R. Sobus, Linking high resolution mass spectrometry data with exposure and toxicity forecasts to advance high-throughput environmental monitoring, Environ. Int., 2016, 88, 269–280 CrossRef CAS PubMed.
- C. Moschet, T. Anumol, B. M. Lew, D. H. Bennett and T. M. Young, Household Dust as a Repository of Chemical Accumulation: New Insights from a Comprehensive High-Resolution Mass Spectrometric Study, Environ. Sci. Technol., 2018, 52(5), 2878–2887 CrossRef CAS PubMed.
- H. Peng, C. Chen, D. M. V. Saunders, J. Sun, S. Tang, G. Codling, M. Hecker, S. Wiseman, P. D. Jones, A. Li, K. J. Rockne and J. P. Giesy, Untargeted Identification of Organo-Bromine Compounds in Lake Sediments by Ultrahigh-Resolution Mass Spectrometry with the Data-Independent Precursor Isolation and Characteristic Fragment Method, Anal. Chem., 2015, 87(20), 10237–10246 CrossRef CAS PubMed.
- H. Peng, C. Chen, J. Cantin, D. M. V. Saunders, J. Sun, S. Tang, G. Codling, M. Hecker, S. Wiseman, P. D. Jones, A. Li, K. J. Rockne, N. C. Sturchio and J. P. Giesy, Untargeted Screening and Distribution of Organo-Bromine Compounds in Sediments of Lake Michigan, Environ. Sci. Technol., 2016, 50(1), 321–330 CrossRef CAS PubMed.
- H. Peng, C. Chen, J. Cantin, D. M. V. Saunders, J. Sun, S. Tang, G. Codling, M. Hecker, S. Wiseman, P. D. Jones, A. Li, K. J. Rockne, N. C. Sturchio, M. Cai and J. P. Giesy, Untargeted Screening and Distribution of Organo-Iodine Compounds in Sediments from Lake Michigan and the Arctic Ocean, Environ. Sci. Technol., 2016, 50(18), 10097–10105 CrossRef CAS PubMed.
- S. Kutarna, S. Tang, X. Hu and H. Peng, Enhanced Nontarget Screening Algorithm Reveals Highly Abundant Chlorinated Azo Dye Compounds in House Dust, Environ. Sci. Technol., 2021, 55(8), 4729–4739 CrossRef CAS PubMed.
- H. Peng, J. Sun, H. A. Alharbi, P. D. Jones, J. P. Giesy and S. Wiseman, Peroxisome Proliferator-Activated Receptor γ is a Sensitive Target for Oil Sands Process-Affected Water: Effects on Adipogenesis and Identification of Ligands, Environ. Sci. Technol., 2016, 50(14), 7816–7824 CrossRef CAS PubMed.
- Y. Gong, D. Yang, H. Barrett, J. Sun and H. Peng, Building the Environmental Chemical-Protein Interaction Network (eCPIN): An Exposome-Wide Strategy for Bioactive Chemical Contaminant Identification, Environ. Sci. Technol., 2023, 57(9), 3486–3495 CrossRef CAS PubMed.
- C. Li, L. Fu, J. Stafford, M. Belosevic and M. Gamal El-Din, The toxicity of oil sands process-affected water (OSPW): A critical review, Sci. Total Environ., 2017, 601–602, 1785–1802 CrossRef CAS PubMed.
- A. S. Pereira, S. Bhattacharjee and J. W. Martin, Characterization of Oil Sands Process-Affected Waters by Liquid Chromatography Orbitrap Mass Spectrometry, Environ. Sci. Technol., 2013, 47(10), 5504–5513 CrossRef CAS PubMed.
- D. Yang, J. Han, D. R. Hall, J. Sun, J. Fu, S. Kutarna, K. A. Houck, C. A. LaLone, J. A. Doering, C. A. Ng and H. Peng, Nontarget Screening of Per- and Polyfluoroalkyl Substances Binding to Human Liver Fatty Acid Binding Protein, Environ. Sci. Technol., 2020, 54(9), 5676–5686 CrossRef CAS PubMed.
- Y. Jia, H. Zhang, W. Hu, L. Wang, Q. Kang, J. Liu, T. Nakanishi, Y. Hiromori, T. Kimura, S. Tao and J. Hu, Discovery of contaminants with antagonistic activity against retinoic acid receptor in house dust, J. Hazard. Mater., 2022, 426, 127847 CrossRef CAS PubMed.
- Y. Sun, H. Cui, T. Li, S. Tao, J. Hu and Y. Wan, Protein-affinity guided identification of chlorinated paraffin components as ubiquitous chemicals, Environ. Int., 2020, 145, 106165 CrossRef CAS PubMed.
- J. Sun, H. Barrett, D. R. Hall, S. Kutarna, X. Wu, Y. Wang and H. Peng, Ecological Role of 6OH-BDE47: Is It a Chemical Offense Molecule Mediated by Enoyl-ACP Reductases?, Environ. Sci. Technol., 2022, 56(1), 451–459 CrossRef CAS PubMed.
- S. Khabazbashi, J. Engelhardt, C. Möckel, J. Weiss and A. Kruve, Estimation of the concentrations of hydroxylated polychlorinated biphenyls in human serum using ionization efficiency prediction for electrospray, Anal. Bioanal. Chem., 2022, 414(25), 7451–7460 CrossRef CAS PubMed.
- M. Z. Lauria, H. Sepman, T. Ledbetter, M. Plassmann, A. M. Roos, M. Simon, J. P. Benskin and A. Kruve, Closing the Organofluorine Mass Balance in Marine Mammals Using Suspect Screening and Machine Learning-Based Quantification, Environ. Sci. Technol., 2024, 58(5), 2458–2467 CrossRef CAS PubMed.
- D. Abrahamsson, A. Siddharth, J. F. Robinson, A. Soshilov, S. Elmore, V. Cogliano, C. Ng, E. Khan, R. Ashton, W. A. Chiu, J. Fung, L. Zeise and T. J. Woodruff, Modeling the transplacental transfer of small molecules using machine learning: a case study on per- and polyfluorinated substances (PFAS), J. Exposure Sci. Environ. Epidemiol., 2022, 32(6), 808–819 CrossRef CAS PubMed.
- E. Dávila-Santiago, C. Shi, G. Mahadwar, B. Medeghini, L. Insinga, R. Hutchinson, S. Good and G. D. Jones, Machine Learning Applications for Chemical Fingerprinting and Environmental Source Tracking Using Non-target Chemical Data, Environ. Sci. Technol., 2022, 56(7), 4080–4090 CrossRef PubMed.
- G. W. Johnson and R. Ehrlich, State of the Art Report on Multivariate Chemometric Methods in Environmental Forensics, Environ. Forensics, 2002, 3(1), 59–79 CAS.
- J. E. Schollée, E. L. Schymanski, S. E. Avak, M. Loos and J. Hollender, Prioritizing Unknown Transformation Products from Biologically-Treated Wastewater Using High-Resolution Mass Spectrometry, Multivariate Statistics, and Metabolic Logic, Anal. Chem., 2015, 87(24), 12121–12129 CrossRef PubMed.
- K. Purschke, M. Vosough, J. Leonhardt, M. Weber and T. C. Schmidt, Evaluation of Nontarget Long-Term LC–HRMS Time Series Data Using Multivariate Statistical Approaches, Anal. Chem., 2020, 92(18), 12273–12281 CrossRef CAS PubMed.
- J. Chen, B. Wang, J. Huang, S. Deng, Y. Wang, L. Blaney, G. L. Brennan, G. Cagnetta, Q. Jia and G. Yu, A machine-learning approach clarifies interactions between contaminants of emerging concern, One Earth, 2022, 5(11), 1239–1249 CrossRef.
- W. A. Christinelli, F. M. Shimizu, M. H. M. Facure, R. Cerri, O. N. Oliveira Jr, D. S. Correa and L. H. C. Mattoso, Two-dimensional MoS2-based impedimetric electronic tongue for the discrimination of endocrine disrupting chemicals using machine learning, Sens. Actuators, B, 2021, 336, 129696 CrossRef CAS.
- T. Chen and C. Guestrin, XGBoost: A Scalable Tree Boosting System, 2016, 785–794 Search PubMed.
- S. Cipullo, B. Snapir, G. Prpich, P. Campo and F. Coulon, Prediction of bioavailability and toxicity of complex chemical mixtures through machine learning models, Chemosphere, 2019, 215, 388–395 CrossRef CAS PubMed.
- G. T. Ankley, R. S. Bennett, R. J. Erickson, D. J. Hoff, M. W. Hornung, R. D. Johnson, D. R. Mount, J. W. Nichols, C. L. Russom, P. K. Schmieder, J. A. Serrrano, J. E. Tietge and D. L. Villeneuve, Adverse outcome pathways: A conceptual framework to support ecotoxicology research and risk assessment, Environ. Toxicol. Chem., 2010, 29(3), 730–741 CrossRef CAS PubMed.
-
F. Henkler, K. Stolpmann and A. Luch, Exposure to Polycyclic Aromatic Hydrocarbons: Bulky DNA Adducts and Cellular Responses, in Molecular, Clinical and Environmental Toxicology: Volume 3: Environmental Toxicology, ed. A. Luch, Springer, Basel: Basel, 2012, pp. 107–131 Search PubMed.
- R. W. Kung, T. K. Deak, C. A. Griffith-Salik, N. A. Takyi and S. D. Wetmore, Impact of DNA Adduct Size, Number, and Relative Position on the Toxicity of Aromatic Amines: A Molecular Dynamics Case Study of ANdG- and APdG-Containing DNA Duplexes, J. Chem. Inf. Model., 2021, 61(5), 2313–2327 CrossRef CAS PubMed.
- M. Ogata, T. Hasegawa and B. Inoue, Effects of environmental pollutants on the mitochondrial and red cell membranes, J. Toxicol. Sci., 1983, 8(3), 205–212 CrossRef CAS PubMed.
- D. R. Hall and H. Peng, Characterizing physical protein targets of chemical contaminants with chemical proteomics: Is it time to fill a crucial environmental toxicology knowledge gap?, Comp. Biochem. Physiol., Part D: Genomics Proteomics, 2020, 34, 100655 CAS.
- J. Onyango, J. J. A. van Bruggen, N. Kitaka, J. Simaika and K. Irvine, Effects of combined nutrient and pesticide exposure on algal biomass, and Daphnia magna abundance, Environmental Systems Research, 2024, 13(1), 1 CrossRef.
- G. A. Dominguez, J. H. Bisesi Jr, K. J. Kroll, N. D. Denslow and T. Sabo-Attwood, Control of Transcriptional Repression of the Vitellogenin Receptor Gene in Largemouth Bass (Micropterus Salmoides) by Select Estrogen Receptors Isotypes, Toxicol. Sci., 2014, 141(2), 423–431 CrossRef CAS PubMed.
|
This journal is © The Royal Society of Chemistry 2024 |