DOI:
10.1039/D4AN01573H
(Paper)
Analyst, 2025, Advance Article
Evaluating sample normalization methods for MS-based multi-omics and the application to a neurodegenerative mouse model†
Received
25th December 2024
, Accepted 13th February 2025
First published on 14th February 2025
Abstract
Mass spectrometry (MS)-based omics methods have transformed biomedical research with accurate and high-throughput analysis of diverse molecules in biological systems. Recent technological advances also enabled multi-omics to be achieved from the same sample or on a single analytical platform. Sample normalization is a critical step in MS-omics studies but is usually conducted independently for each omics experiment. To bridge this technical gap, we evaluated different sample normalization methods suitable for analyzing proteins, lipids, and metabolites from the same sample for multi-omics analysis. We found that normalizing samples based on tissue weight or protein concentration before or after extraction generated distinct quantitative results. Normalizing samples first by tissue weight before extraction and then by protein concentration after extraction resulted in the lowest sample variation to reveal true biological differences. We then applied this two-step normalization method to investigate multi-omics profiles of mouse brains lacking the GRN gene. Loss-of-function mutations in the GRN gene lead to the deficiency of the progranulin protein and eventually cause neurodegeneration. Comparing the proteomics, lipidomics, and metabolomics profiles of GRN KO and WT mouse brains revealed molecular changes and pathways related to lysosomal dysfunction and neuroinflammation. In summary, we demonstrated the importance of selecting an appropriate normalization method during multi-omics sample preparation. Our normalization method is applicable to all tissue-based multi-omics studies, ensuring reliable and accurate biomolecule quantification for biological comparisons.
1. Introduction
Mass spectrometry (MS) has become a dominant technology for proteomics, lipidomics, and metabolomics research to understand physiological and disease processes.1,2 With recent advances in analytical methods and computational tools, different omics can be integrated together to capture the complex molecular landscape in biological systems.3 Multi-omics integration can be achieved at different levels. Sample preparation methods have been developed to extract different types of molecules from the same biological sample.4–6 Analyzing different biomolecules on the same liquid chromatography-mass spectrometry (LC-MS) platform has also become possible.7,8 Various bioinformatics tools have emerged to integrate different omics datasets to associate with the same gene or pathway, revealing how different types of molecules work together to govern human health.9
Omics studies typically involve the analysis of multiple replicates across different biological groups. Normalization is a crucial step in omics studies to control systematic biases and minimize variability.10 Normalization can be conducted during sample preparation (pre-acquisition normalization) or during data analysis (post-acquisition normalization). Pre-acquisition normalization aims to standardize each sample to the same total quantity of molecules to minimize sample variability.11,12 Post-acquisition normalization adjusts the data to reduce instrument and data variations.13–16 Whenever possible, pre-acquisition normalization is preferred over post-acquisition normalization so that the same amount of starting material from each sample can be injected onto the instrumental platform for a fair comparison. However, pre-acquisition normalization methods suitable for multi-omics analysis have rarely been investigated.
Different omics experiments are traditionally analyzed with different analytical methods in different laboratories. Therefore, sample normalization is typically conducted independently for each omics experiment. Proteomics analysis employs a uniform sample normalization method that adjusts each sample to the same total protein amount based on a colorimetric assay measuring total protein concentration. For lipidomics and metabolomics, there is no accurate method to measure the total lipid or metabolite amount from complex biological samples. Thus, normalization methods for lipidomics and metabolomics vary based on sample types and study designs. For example, tissue weight, plasma volume, urine osmolality, cell count, and protein amount have been used for sample normalization for various lipidomics and metabolomics applications.11,12,17–19 Normalization methods for multi-omics need to adjust sample amounts to ensure that they are suitable for the extraction and analyses of different types of molecules from the same sample.
To address this critical gap, we evaluated different sample normalization methods for tissue-based multi-omics analysis. Normalization methods based on tissue weight and protein concentration before and after extraction were compared using mouse brain samples. The goal is to control systematic bias, reduce sample variability, minimize sample preparation steps, and maximize the separation of different biological groups. We then applied the optimal normalization method to explore the multi-omics profile of a neurodegenerative mouse model lacking the GRN gene. Mutations in the GRN gene lead to the deficiency of progranulin protein inside lysosomes and eventually cause frontotemporal dementia.20–22 Recent studies have associated progranulin deficiency with lysosomal dysfunction, innate immunity, and lipid metabolism.5,23–25 Comparing the proteome, lipidome, and metabolome profiles between GRN KO and WT mouse brains can provide us a comprehensive picture to understand progranulin deficiency and neurodegenerative diseases.
2. Experimental
2.1. Mouse brain tissue sample preparation
Mice (C57/BL6 WT and GRN KO) were obtained from the Jackson Laboratory and housed in the Weill Hall animal facility at Cornell University. All animal experiments and procedures were performed according to NIH guidelines and were approved by the Institutional Animal Care and Use Committee (IACUC) at Cornell. Two-month-old mice were euthanized with 5% isoflurane and perfused with cold phosphate buffered saline (PBS). Mouse brain hemisphere tissues were dissected and snap-frozen in liquid nitrogen and stored at −80 °C. Frozen mouse brain tissues were lyophilized briefly (2 min under 10 torr in a Labconco Lyophilizer) to remove residual PBS and cut into small pieces using a micro-scissor in 2 ml tubes kept on ice. For the comparison of different normalization methods with four biological replicates, WT mouse brain tissues were weighed and homogenized at a ratio of 800 μL of HPLC-grade water per 25 mg of tissue using a tissue grinder (Kimble) in 2 mL tubes. Tissue samples were sonicated on ice for 10 minutes using a Qsonica bath sonicator with a 1 min-on and 30 s-off cycle and then vortexed briefly. For the biological comparison of GRN KO vs. WT, mouse brain tissues were weighed, and a methanol–water mixture (5
:
2, v
:
v) was added to the tissue at a concentration of 0.06 mg of tissue per microliter of solvent mixture. Four mice were used for each group.
2.2. Multi-omics extraction
Multi-omics extraction for proteins, lipids, and metabolites was conducted using the Folch method.26 Briefly, methanol, water, and chloroform were added to the tissue sample at a volume ratio of 5
:
2
:
10 (v
:
v
:
v). The tissue sample in extraction solvents was incubated on ice for 1 hour with frequent vortexing. Samples were centrifuged at 12
700 rpm and 4 °C for 15 min. The organic solvent layer was transferred into a new tube, dried, and reconstituted in a MeOH
:
CHCl3
:
H2O mixture (18
:
1
:
1, v
:
v
:
v) for lipidomics analysis. The aqueous layer was transferred into a new tube, dried, and reconstituted in MS-grade water with 0.1% formic acid (FA) for metabolomics analysis. Internal standards (I.S.) were spiked into each tube before drying the aqueous and organic solvent layers: 13C515N folic acid (Sigma) was used for metabolomics and EquiSplash® (Avanti Polar Lipids) was used for lipidomics (Table S1†). The protein pellet was dried and reconstituted in lysis buffer (8 M urea, 50 mM ammonium bicarbonate, and 150 mM sodium chloride). Each protein sample was sonicated with a Qsonica on ice for 30 minutes and clarified by centrifugation. The protein concentration was measured using a DCA assay (Bio-Rad). Bottom-up proteomics procedures were conducted as described previously for reduction, alkylation, overnight digestion, and cleanup.5
To evaluate different multi-omics normalization methods, method A measures the protein concentration from the tissue-water slurry and normalize tissue samples by the protein concentration before multi-omics extraction. For method B, tissue samples were normalized based on tissue weight before multi-omics extraction. For method C, tissue samples were normalized based on tissue weight before multi-omics extraction. After extraction, the protein concentration from the extracted protein pellet was measured. The volumes of lipid and metabolite fractions were normalized based on protein concentration before drying.
2.3. LC-MS/MS analysis
All samples were transferred into glass LC vials with fixed inserts for LC-MS/MS analyses. Peptide samples were analyzed using a Dionex UltiMate 3000 RSLCnano system coupled with a Thermo Scientific Q-Exactive HF-X Orbitrap mass spectrometer. MS-grade water with 0.1% FA and acetonitrile (ACN) with 0.1% FA were used for mobile phases A and B, respectively. An EASY-Spray PepMap RSLC C18 column (2 μm, 100 Å, 75 μm × 50 cm) was used for peptide separation with a 210 min gradient at 55 °C and a flow rate of 0.25 μL min−1. Data independent acquisition was conducted, and the MS scan range was set as m/z 400 to 1000 in positive ion mode. The MS1 resolving power was 60 K (at m/z 200 FWHM), the automatic gain control (AGC) target value was 1 × 106, and the maximum injection time (maxIT) was 60 ms. The precursor isolation window was m/z 8.0 (overlapped) with 75 sequential data independent acquisition (DIA) MS/MS scans at an MS/MS resolving power of 15 K, an AGC target of 2 × 105, a maxIT of 50 ms, and a normalized collision energy (NCE) of 30%.
Lipid samples were analyzed using a Vanquish Duo UHPLC system coupled with a Thermo Scientific Q-Exactive HF-X MS. An ACQUITY UPLC® BEH Shield RP18 column (1.7 μm, 2.1 mm × 150 mm) was used with a 40 min total gradient, a flow rate of 0.15 mL min−1, and a column temperature of 40 °C. Mobile phase A (H2O
:
ACN, 9
:
1, v
:
v) and mobile phase B (IPA
:
MeOH
:
ACN
:
H2O, 7
:
1.5
:
1
:
0.5, v
:
v
:
v
:
v) were used for lipid separation. As the ionization modifier, 0.5 mM NH4HCO2 and 5 mM NH4OH were used in both mobile phases. Full MS scans with positive and negative ion modes were used for each sample. Data dependent acquisition (DDA) in both positive and negative ion modes was used for lipid identification using a pooled sample from different biological replicates and groups. The m/z range of the MS1 scan was from 380 to 1200 (ESI+) and 380 to 2000 (ESI−). The isolation m/z window was set as 2. The resolving power was 60 K, the AGC target was 1 × 106, and the NCE was 22.5% (ESI+) or 27.5% (ESI−) for DDA with dynamic exclusion times of 10 s and 20 s.
Metabolite samples were analyzed using the same UHPLC-ESI-MS/MS system as the lipid sample analysis. A Luna Omega Polar C18 column (1.6 μm, 100 Å, 2.1 mm × 100 mm) was used for metabolite separation with a 20 min total gradient, a flow rate of 0.3 mL min−1, and a column temperature of 30 °C. Mobile phases were the same as those used for proteomic analysis. Full MS scans for positive and negative ion modes were used for each sample, and DDA was used for metabolite identification using pooled samples. The m/z range of the MS1 scan was 70 to 800 with a resolving power of 60 K and an AGC target of 1 × 106. The isolation m/z window was set as 2. DDA used an NCE of 30% and a dynamic exclusion time of 10 s.
2.4. Multi-omics data analysis
Proteomics raw data were analyzed using Spectronaut software (v19, Biognosys) with the DirectDIA mode and default settings. The Swiss-Prot Mus musculus (Mouse) reviewed database and our custom contaminant libraries (https://github.com/HaoGroup-ProtContLib) were included for protein identification.27 For protein identification, up to 2 missed cleavages and 3 variable modifications were allowed. Carbamidomethyl C was set as the fixed modification, and methionine oxidation and protein N-terminal acetylation were set as variable modifications. False discovery rate (FDR) cutoffs for precursors and proteins were set as 1%. DIA precursors with intensities below 1.0 × 103 arbitrary units (a.u.) were removed. Normalization to total spectral abundance was selected for the GRN KO vs. WT dataset.
Lipidomics data were analyzed using LipidMatch software (v4.0).28 For lipid identification, a 5 ppm mass tolerance for precursor ions and 10 ppm for product ions were used. Each lipid peak area was normalized by both the lipid I.S. and the total protein amount after extraction. The semi-absolute quantification (pmol lipid per μg of protein) was used for the calculation of lipid concentration.29–31 For lipid annotation, an underbar “_” is used to separate the acyl chain composition for glycerophospholipid (GP) and glycerolipid (GL) without indicating the exact acyl chain location. For the sphingolipid (SP), a “/” separates the sphingosine backbone and acyl chain composition with the exact acyl chain location. The abundance can be calculated using individual lipid concentration per total lipid concentration within each lipid class.
Metabolomics data were analyzed using Compound Discoverer software (v3.3, Thermo) based on MS1 and MS/MS database searches with the built-in MzCloud, ChemSpider, HMDB, and KEGG libraries and our in-house metabolite standard spectral library.6,32 A max retention time shift of 0.5 min, a mass tolerance of 10 ppm, and a minimum peak intensity of 1.0 × 105 a.u. were used. Quantification of metabolites in our in-house library was further confirmed using Skyline software for targeted peak extraction and integration.33 Metabolite concentrations were normalized by both the I.S. and the total protein amount.
Statistical analysis was conducted using the Student's t-test for each proteomics, lipidomics, and metabolomics dataset. Several website-based software packages were used for additional data analysis: VolcaNoseR34 (volcano plots), ShinyGO35 (protein GO-enrichment analysis), and Metaboanalyst36 (principal component analysis (PCA) and joint pathway analysis).
3. Results and discussion
3.1 Evaluation of sample normalization methods for multi-omics analysis
Three different sample normalization methods were evaluated here for multi-omics analysis. The overall workflow is shown in Fig. 1. Tissues were briefly freeze-dried to remove residue buffer from dissection and then weighed. Protein, lipid, and metabolite fractions were obtained from the same mouse brain tissue sample by methanol–water–chloroform extraction, followed by LC-MS and data analysis. Example base peak chromatograms for proteomics, lipidomics, and metabolomics are shown in Fig. S1.† Method A measured the protein concentration from the tissue–water slurry and then normalized tissue samples by protein concentration before extraction. Method B normalized samples by tissue weight before extraction. Method C first normalized samples by tissue weight before extraction and then by protein concentration after extraction. All samples are normalized by the protein amount from the corresponding protein fraction during the data analysis step.
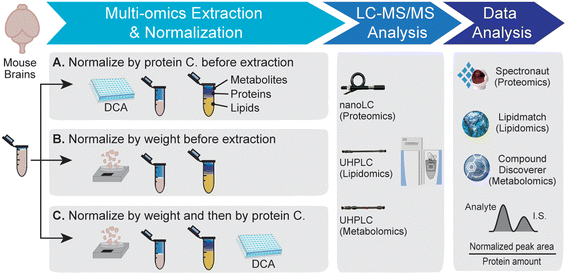 |
| Fig. 1 Overall workflow to evaluate normalization methods for multi-omics. | |
First, three different sample normalization methods were evaluated by principal component analysis (PCA) using proteomics, lipidomics, and metabolomics datasets (Fig. 2A). Method A displayed a distinct proteome profile compared to methods B and C. Consistent with the PCA plot, over 100 proteins were significantly changed in method A compared to the other two methods (p-value <0.05 and fold change >1.5), while only 22 proteins were significantly different between B and C (Fig. 2B). This is likely because method A normalizes samples based on the protein concentration from the tissue–water slurry before extraction, which is not an accurate measurement of protein amount. For lipidomics, biological replicates in method A did not cluster as tightly as in methods B and C (Fig. 2A), and many lipids showed over 80% coefficient of variation (CV) among biological replicates (Fig. 2C). For metabolomics, method C showed the lowest sample variation among replicates (Fig. 2C). A volcano plot comparison of the three methods for lipidomics and metabolomics also demonstrated that the quantitative omics profile can be largely influenced by the sample normalization method (Fig. S2 and S3†).
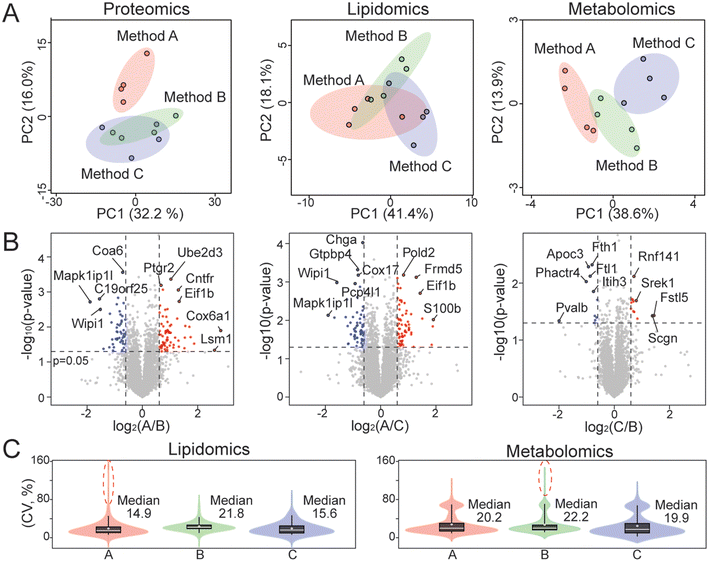 |
| Fig. 2 Evaluation of different normalization methods for multi-omics analysis. (A) Principal component analysis using proteomics, lipidomics, and metabolomics datasets with four replicates per group. (B) Proteomics volcano plots for comparing different normalization methods. Volcano plots for lipidomics and metabolomics are provided in Fig. S2 and S3.† Dashed lines denote p-value of 0.05 and fold change of 1.5. (C) Violin plots showing the distribution of coefficients of variation (CV) in lipidomics and metabolomics datasets. | |
Since method A did not perform well for multi-omics analysis, we then evaluated methods B and C using biological comparison groups with and without the GRN gene in mouse brains. Method C achieved complete separation of GRN KO vs. WT groups for both lipidomics and metabolomics data (Fig. 3A and C). Method C also quantified more lipids and metabolites compared to method B (Fig. 3B and D). Therefore, method C was selected as the optimal method to normalize multi-omics sample preparation. This is likely because normalizing samples first by tissue weight before extraction can reduce variability in the amount of starting material. Protein concentration can be more accurately measured after extraction in the protein lysis buffer compared to the measurement before extraction and there is no accurate way to measure the total amount of lipids and metabolites. Therefore, further normalizing samples based on the total protein amount after extraction can scale lipids, metabolites, and proteins to achieve the same LC-MS injection amount per sample, avoiding systematic error and controlling instrumental variation.
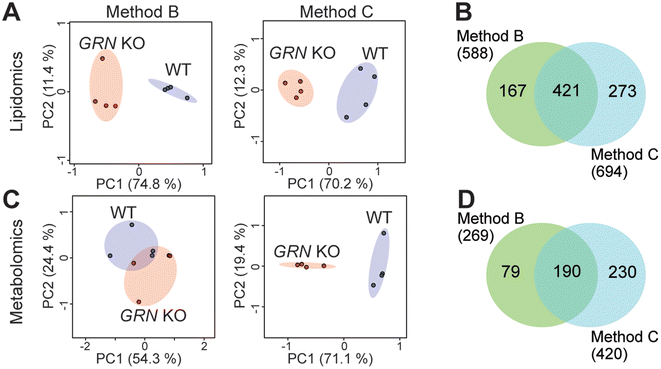 |
| Fig. 3 Lipidomics and metabolomics analyses of GRN KO vs. WT groups using different normalization methods. (A) Principal component analysis of GRN KO vs. WT lipidomics data with four biological replicates from each group. (B) Venn diagram showing the comparison of identified and quantified lipids using normalization Method B and Method C. (C) Principal component analysis of GRN KO vs. WT metabolomics data. (D) Venn diagram showing the comparison of identified and quantified metabolites using normalization Method B and Method C. | |
3.2 Multi-omics evaluation of progranulin deficiency in mouse brain
After selecting the optimal sample normalization method C, we compared the proteome, lipidome, and metabolome profiles of 2-month-old mouse brain tissues with and without the GRN gene. The GRN gene encodes the progranulin protein, whose deficiency has been associated with various neurodegenerative diseases.20–22 Mice lacking the GRN gene can already exhibit neurodegenerative phenotypes by a few months of age. The subtle molecular changes in 2-month-old GRN KO and WT mice can be used to test the robustness of our normalization method. As shown in Fig. 4A, a PCA plot demonstrates a clear separation between 2-month-old GRN KO and WT groups. Many lysosomal proteins (Ctsh, Lamtor4, Ank3, Scarb2, Tmem192, Fuca1, Lgmn, Ppt1, Naaa, Asah1, Smpd1, and Tmem106b) were significantly changed as shown in the volcano plot (Fig. 4B). GO-enrichment analysis of significantly increased proteins revealed biological processes related to the complement component C1 complex and lysosomes. Significantly down-regulated proteins were enriched for myelin sheath, synaptic activity and neuron projection. This is consistent with our and others’ previous findings of lysosomal dysfunction caused by the lack of the GRN gene.22,24,37 We then compared these protein changes with our previous result of lysosomal proximity labeling in the mouse brain.24 Two-month-old young mice in this study exhibited consistent protein changes but with smaller fold changes compared to the 20-month-old mice used in our previous proteomics study (Fig. 4D).24 Lysosomal proteins such as TMEM106B, TPP1, and CTSH were consistently upregulated in both studies. Another significantly altered pathway is related to innate immunity and neuroinflammation with proteins like C1qa, Gfap, and Srr.38,39 Additionally, Pip4k2c plays a role in regulating the immune system, and the depletion of Pip4k2c is relevant to inflammation.40 Prkar2a regulates inflammation via its catalytic subunit and reduced Prkar2a can cause neuronal apoptosis.41
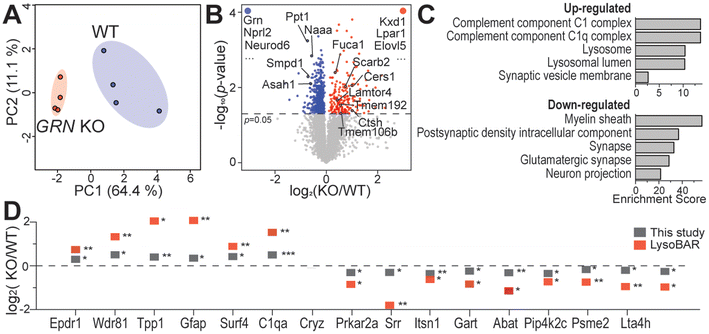 |
| Fig. 4 Proteomics analysis of mouse brains from GRN KO and WT groups. (A) Principal component analysis of GRN KO vs. WT proteomics data. (B) Volcano plot of GRN KO vs. WT proteomics data. Proteins that were reproducibly quantified in only one group are labeled at the upper left and right corners of the graph. (C) GO-Enrichment analysis showing enriched biological processes using significantly changed proteins from the GRN KO vs. WT comparison. Enrichment score was calculated as log2(fold enrichment) × −log10(enrichment FDR). (D) Significantly changed proteins overlapped between this study and our previous mouse brain lysosomal proximity labeling (LysoBAR) proteomics data.24 *p < 0.05; **p < 0.01; and ***p < 0.001. | |
Alterations in lipid metabolism have recently been associated with progranulin deficiency in frontotemporal dementia.25,42 Lipidomics analysis of mouse brain tissues revealed 39 significantly changed lipid species in 2-month-old GRN KO vs. WT groups (Fig. 5A). As shown in Fig. 5B, many sphingolipids were significantly changed, consistent with recent reports in progranulin-deficient mouse brains.25 Sphingolipid is highly abundant in the central nervous system and is crucial for brain development.43 Other key lipid changes are shown in Fig. 5C and Fig. S4.† Ceramide (Cer) is known to regulate inflammation and binds to cathepsin.43,44 Ganglioside (GM1) gangliosidosis is a rare inherited neurodegenerative disease with deficient lysosomal enzyme β-galactosidase.45 Increased hexosylceramide (HexCer) induces the expression of sphingosine kinase, causing neuroinflammation.46 Sulfatide (SHexCer) is a lipid component of the myelin sheath that plays a key role in myelination, the process of wrapping a myelin sheath around axonal bundles.47 Interestingly, eight dimethyl-phosphatidylethanolamine (DMPE) species were significantly decreased in the GRN KO group (Fig. 5C and S4†). Anti-inflammatory treatment has been found to lead to an increase in DMPE.48 Ether lipids (PE(P-18
:
1/18
:
1), PS(P-18
:
0/22
:
6), and PS(P-20
:
0/20
:
1)) were significantly decreased in the GRN KO group (Fig. 5C and S4†). They are known for their antioxidant properties in the central nervous system, especially plasmalogen lipids.49
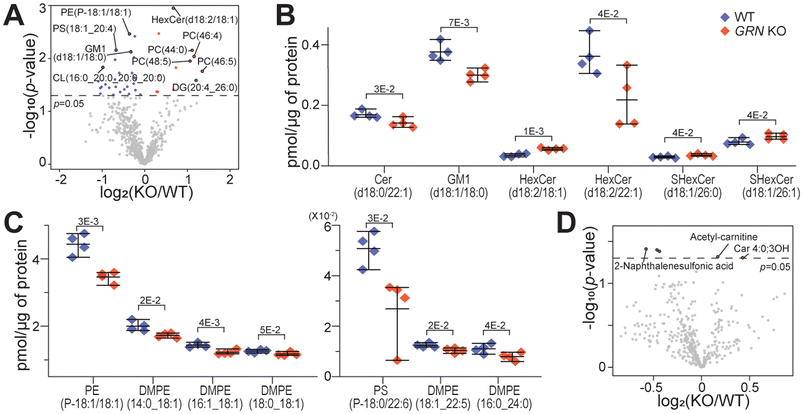 |
| Fig. 5 Lipidomics and metabolomics analyses of mouse brains from GRN KO and WT groups. (A) Volcano plot of GRN KO vs. WT lipidomics data. (B) Sphingolipid concentrations (pmol μg−1 of protein) in GRN KO vs. WT mouse brains. (C) Other key lipid changes in GRN KO vs. WT mouse brains. (D) Volcano plot of GRN KO vs. WT metabolomics data. | |
In the metabolomics dataset, there were not many significant changes in the GRN KO vs. WT comparison (Fig. 5D). This is likely due to the young age of mice (2-month-old). Among a handful of changed metabolites, two acyl-carnitines were significantly increased, which are known to be associated with mitochondrial metabolism and neuroinflammation.50 In particular, acetyl carnitine is known for its neuroprotective function.51 Joined KEGG pathway analysis using significantly changed proteins and metabolites showed enrichment in lysosomes, sphingolipid related pathways, the GABAergic synapse, and the Hippo signaling pathway (Fig. 6). Neuroinflammation can alter GABAergic neurotransmission, which can impair cognitive and motor function.52 Also, the Hippo signaling pathway has been associated with neurodegeneration, where its activation can lead to excessive neuronal cell death by promoting apoptosis under oxidative stress.53 Most studies for neurodegenerative diseases use aged mice. Here we showed that the molecule profiles of GRN-null 2-month-old mice already exhibit profound changes related to lysosomal dysfunction and neuroinflammation.
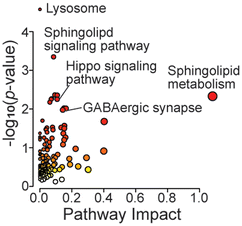 |
| Fig. 6 Multi-omics KEGG pathway analysis of mouse brains from GRN KO and WT groups. | |
4. Conclusions
In summary, we evaluated different sample normalization methods for MS-based multi-omics analysis using tissue samples. We found that sample normalization significantly impacts quantitative results in proteomics, lipidomics, and metabolomics. To reduce variations between replicates and reveal biological differences, we recommend normalizing tissue samples first by tissue weight before extraction and then by protein concentration after extraction. Briefly drying tissue samples before weighing also helps reduce variation by removing the remaining buffer from dissection. With this two-step sample normalization method, sample variation from starting materials was minimized. The same total amount of samples was injected into the LC-MS to control systematic error for multi-omics analysis. As expected, pre-acquisition normalization to protein concentration performed better than post-acquisition normalization. Thanks to this normalization method, subtle biological differences can be revealed with great confidence. We discovered key molecular changes in just 2-month-old mice from a neurodegenerative mouse model lacking the GRN gene. The loss of the GRN gene leads to multi-omics alterations related to lysosomal dysfunction and neuroinflammation. Our normalization method is applicable to all MS-based multi-omics studies using tissue samples. Same principle would apply to cell culture experiments: each sample should start from the same total cell count and be further normalized to the protein concentration after extraction to achieve the best accuracy and precision for multi-omics analysis. We urge researchers to take the normalization method into consideration when designing omics studies to ensure reliable and accurate biomolecule quantification for biological comparisons.
Author contributions
G.L. and L.H. designed the study. C.Y. and F.H. provided mouse brain samples. G.L. conducted sample preparation and LC-MS analysis. G.L. analyzed the data and wrote the manuscript with edits from L.H., C.Y., and F.H. All authors have read and approved the final manuscript.
Data availability
Raw LC-MS/MS data from this manuscript are available through the MassIVE repository (Identifier: MSV000096533). Full omics datasets are available in the Supplementary data in this manuscript.†
Conflicts of interest
The authors declare no competing financial interests.
Acknowledgements
This study is supported by the NIH grants: R01NS121608 (L. H.) and R01NS095954 (F. H.). C. Y. acknowledges the Weill Institute Fleming Postdoctoral Fellowship. We thank all Hao Lab members for their support and friendship.
References
- B. F. Cravatt, G. M. Simon and J. R. Yates III, Nature, 2007, 450, 991–1000 CrossRef CAS PubMed.
- G. J. Patti, O. Yanes and G. Siuzdak, Nat. Rev. Mol. Cell Biol., 2012, 13, 263–269 CrossRef CAS PubMed.
- M. Babu and M. Snyder, Mol. Cell. Proteomics, 2023, 22, 100561 CrossRef CAS PubMed.
- L. K. Muehlbauer, A. Jen, Y. Zhu, Y. He, E. Shishkova, K. A. Overmyer and J. J. Coon, Anal. Chem., 2023, 95, 659–667 CAS.
- G. Lee, W. N. A. B. Mazli and L. Hao, J. Proteome Res., 2024, 23, 3149–3160 CrossRef CAS PubMed.
- H. Li, M. Uittenbogaard, R. Navarro, M. Ahmed, A. Gropman, A. Chiaramello and L. Hao, Mol. Omics, 2022, 18, 196–205 RSC.
- L. Hao, J. Johnson, C. B. Lietz, A. Buchberger, D. Frost, W. J. Kao and L. Li, Anal. Chem., 2017, 89, 1138–1146 CrossRef CAS PubMed.
- Y. He, E. H. Rashan, V. Linke, E. Shishkova, A. S. Hebert, A. Jochem, M. S. Westphall, D. J. Pagliarini, K. A. Overmyer and J. J. Coon, Anal. Chem., 2021, 93, 4217–4222 CrossRef CAS PubMed.
- M. D. Ritchie, E. R. Holzinger, R. Li, S. A. Pendergrass and D. Kim, Nat. Rev. Genet., 2015, 16, 85–97 CrossRef CAS PubMed.
- T. G. Brooks, N. F. Lahens, A. Mrčela and G. R. Grant, Nat. Rev. Genet., 2024, 25, 326–339 CrossRef CAS PubMed.
- Y. Wu and L. Li, J. Chromatogr. A, 2016, 1430, 80–95 CrossRef CAS PubMed.
- J. Wang, C. Wang and X. Han, Anal. Chim. Acta, 2019, 1061, 28–41 CrossRef CAS PubMed.
- B. Low, Y. Wang, T. Zhao, H. Yu and T. Huan, ACS Meas. Sci. Au, 2024, 4, 702–711 CrossRef CAS PubMed.
- J. Walach, P. Filzmoser and K. Hron, Data Normalization and Scaling: Consequences for the Analysis in Omics Sciences, 2018, vol. 82 Search PubMed.
- S. J. Callister, R. C. Berry, J. N. Adkins, E. T. Johnson, W. Qian, B.-J. M. Webb-Robertson, R. D. Smith and M. S. Lipton, J. Proteome Res., 2006, 2, 277–286 CrossRef PubMed.
- P. Cuevas-Delgado, D. Dudzik, V. Miguel, S. Lamas and C. Barbas, Anal. Bioanal. Chem., 2020, 412, 6391–6405 CrossRef CAS PubMed.
- L. Hao, T. Greer, D. Page, Y. Shi, C. M. Vezina, J. A. Macoska, P. C. Marker, D. E. Bjorling, W. Bushman and W. A. Ricke, et al., Sci. Rep., 2016, 6, 30869 CrossRef CAS PubMed.
- B. M. Warrack, S. Hnatyshyn, K. H. Ott, M. D. Reily, M. Sanders, H. Y. Zhang and D. M. Drexler, J. Chromatogr. B:Anal. Technol. Biomed. Life Sci., 2009, 877, 547–552 CrossRef CAS PubMed.
- E. M. Gallagher, G. M. Rizzo, R. Dorsey, E. S. Dhummakupt, T. S. Moran, P. M. Mach and C. C. Jenkins, Toxicol. in Vitro, 2023, 88, 105540 CrossRef CAS PubMed.
- M. Baker, I. R. Mackenzie, S. M. Pickering-Brown, J. Gass, R. Rademakers, C. Lindholm, J. Snowden, J. Adamson, A. D. Sadovnick, S. Rollinson, A. Cannon, E. Dwosh, D. Neary, S. Melquist, A. Richardson, D. Dickson, Z. Berger, J. Eriksen, T. Robinson, C. Zehr, C. A. Dickey, R. Crook, E. McGowan, D. Mann, B. Boeve, H. Feldman and M. Hutton, Nature, 2006, 442, 916–919 CrossRef CAS PubMed.
- A. W. Kao, A. McKay, P. P. Singh, A. Brunet and E. J. Huang, Nat. Rev. Neurosci., 2017, 18, 325–333 CrossRef CAS PubMed.
- D. H. Paushter, H. Du, T. Feng and F. Hu, Acta Neuropathol., 2018, 136, 1–17 CrossRef CAS PubMed.
- J. Zhang, D. Velmeshev, K. Hashimoto, Y. H. Huang, J. W. Hofmann, X. Shi, J. Chen, A. M. Leidal, J. G. Dishart, M. K. Cahill, K. W. Kelley, S. A. Liddelow, W. W. Seeley, B. L. Miller, T. C. Walther, R. V. Farese, J. P. Taylor, E. M. Ullian, B. Huang, J. Debnath, T. Wittmann, A. R. Kriegstein and E. J. Huang, Nature, 2020, 588, 459–465 CrossRef CAS PubMed.
- S. Hasan, M. S. Fernandopulle, S. W. Humble, A. M. Frankenfield, H. Li, R. Prestil, K. R. Johnson, B. J. Ryan, R. Wade-Martins, M. E. Ward and L. Hao, Mol. Neurodegener., 2023, 18, 96 CrossRef PubMed.
- S. Boland, S. Swarup, Y. A. Ambaw, P. C. Malia, R. C. Richards, A. W. Fischer, S. Singh, G. Aggarwal, S. Spina, A. L. Nana, L. T. Grinberg, W. W. Seeley, M. A. Surma, C. Klose, J. A. Paulo, A. D. Nguyen, J. W. Harper, T. C. Walther and R. V. Farese, Nat. Commun., 2022, 13, 5924 CrossRef CAS PubMed.
- J. Folch, M. Lees and G. H. Sloane, J. Biol. Chem., 1957, 226, 497–509 CrossRef CAS PubMed.
- A. M. Frankenfield, J. Ni, M. Ahmed and L. Hao, J. Proteome Res., 2022, 21, 2104–2113 CrossRef CAS PubMed.
- J. P. Koelmel, N. M. Kroeger, C. Z. Ulmer, J. A. Bowden, R. E. Patterson, J. A. Cochran, C. W. W. Beecher, T. J. Garrett and R. A. Yost, BMC Bioinf., 2017, 18, 331 CrossRef PubMed.
- K. L. Bidne, C. Uhlson, C. Palmer, K. Zemski-Berry and T. L. Powell, Clin. Sci., 2022, 136, 1389–1404 CrossRef CAS PubMed.
- M. Kiamehr, L. Viiri, T. Vihervaara, K. Koistin, M. Hilvo, K. Ekroos and K. Aalto-Setälä, Atherosclerosis, 2017, 263, e104 CrossRef.
- M. D. Cas, A. Zulueta, A. Mingione, A. Caretti, R. Ghidoni, P. Signorelli and R. Paroni, Cells, 2020, 9, 1197 CrossRef CAS PubMed.
- L. Hao, T. Greer, D. Page, Y. Shi, C. M. Vezina, J. A. Macoska, P. C. Marker, D. E. Bjorling, W. Bushman, W. A. Ricke and L. Li, Sci. Rep., 2016, 6, 30869 CrossRef CAS PubMed.
- B. MacLean, D. M. Tomazela, N. Shulman, M. Chambers, G. L. Finney, B. Frewen, R. Kern, D. L. Tabb, D. C. Liebler and M. J. MacCoss, Bioinformatics, 2010, 26, 966–968 CrossRef CAS PubMed.
- J. Goedhart and M. S. Luijsterburg, Sci. Rep., 2020, 10, 20560 CrossRef CAS PubMed.
- S. X. Ge, D. Jung and R. Yao, Bioinformatics, 2020, 36, 2628–2629 CrossRef CAS PubMed.
- Z. Pang, J. Chong, G. Zhou, D. A. De Lima Morais, L. Chang, M. Barrette, C. Gauthier, P. É. Jacques, S. Li and J. Xia, Nucleic Acids Res., 2021, 49, W388–W396 CrossRef CAS PubMed.
- X. Zhou, D. H. Paushter, T. Feng, L. Sun, T. Reinheckel and F. Hu, Mol. Neurodegener., 2017, 12, 62 CrossRef PubMed.
- X. Zheng, T. Mi, R. Wang, Z. Zhang, W. Li, J. Zhao, P. Yang, H. Xia and Q. Mao, Glia, 2022, 70, 1317–1336 CrossRef CAS PubMed.
- H. Lui, J. Zhang, S. R. Makinson, M. K. Cahill, K. W. Kelley, H. Y. Huang, Y. Shang, M. C. Oldham, L. H. Martens, F. Gao, G. Coppola, S. A. Sloan, C. L. Hsieh, C. C. Kim, E. H. Bigio, S. Weintraub, M. M. Mesulam, R. Rademakers, I. R. MacKenzie, W. W. Seeley, A. Karydas, B. L. Miller, B. Borroni, R. Ghidoni, R. V. Farese, J. T. Paz, B. A. Barres and E. J. Huang, Cell, 2016, 165, 921–935 CrossRef CAS PubMed.
- H. Shim, C. Wu, S. Ramsamooj, K. N. Bosch, Z. Chen, B. M. Emerling, J. Yun, H. Liu, R. Choo-Wing, Z. Yang, G. M. Wulf, V. K. Kuchroo and L. C. Cantley, Proc. Natl. Acad. Sci. U. S. A., 2016, 113, 7596–7601 CrossRef CAS PubMed.
- R. Huang, W. Li, D. Han, Y. Gao, D. Zheng and G. Bi, Cell Death Discovery, 2022, 8, 247 CrossRef CAS PubMed.
- B. M. Evers, C. Rodriguez-Navas, R. J. Tesla, J. Prange-Kiel, C. R. Wasser, K. S. Yoo, J. McDonald, B. Cenik, T. A. Ravenscroft, F. Plattner, R. Rademakers, G. Yu, C. L. White and J. Herz, Cell Rep., 2017, 20, 2565–2574 CrossRef CAS PubMed.
- Y. A. Hannun and L. M. Obeid, Nat. Rev. Mol. Cell Biol., 2018, 19, 175–191 CrossRef CAS PubMed.
- J. L. Stith, F. N. Velazquez and L. M. Obeid, J. Lipid Res., 2019, 60, 913–918 CrossRef CAS PubMed.
- M. Y. Son, J. E. Kwak, B. Seol, D. Y. Lee, H. Jeon and Y. S. Cho, J. Pathol., 2015, 237, 98–110 CrossRef CAS PubMed.
- A. Dehghan, R. C. Pinto, I. Karaman, J. Huang, B. R. Durainayagam, M. Ghanbari, A. Nazeer, Q. Zhong, S. Liggi, L. Whiley, R. Mustafa, M. Kivipelto, A. Solomon, T. Ngandu, T. Kanekiyo, T. Aikawa, C. I. Radulescu, S. J. Barnes, G. Graça, E. Chekmeneva, S. Camuzeaux, M. R. Lewis, M. R. Kaluarachchi, M. A. Ikram, E. Holmes, I. Tzoulaki, P. M. Matthews, J. L. Griffin and P. Elliott, Proc. Natl. Acad. Sci. U. S. A., 2022, 119, e2206083119 CrossRef CAS PubMed.
- T. Takahashi and T. Suzuki, J. Lipid. Res., 2012, 53, 1437–1450 CrossRef CAS PubMed.
- I. Yadav, N. Sharma, R. Velayudhan, Z. Fatima and J. S. Maras, Life, 2023, 13, 1877 CrossRef CAS PubMed.
- F. Dorninger, S. Forss-Petter and J. Berger, FEBS Lett., 2017, 591, 2761–2788 CrossRef CAS PubMed.
- M. R. McCann, M. V. G. De la Rosa, G. R. Rosania and K. A. Stringer, Metabolites, 2021, 11, 1–21 CrossRef PubMed.
- F. Kazak and G. F. Yarim, Neurosci. Lett., 2017, 658, 32–36 CrossRef CAS PubMed.
- M. Llansola, Y. M. Arenas, M. Sancho-Alonso, G. Mincheva, A. Palomares-Rodriguez, M. Doverskog, P. Izquierdo-Altarejos and V. Felipo, Front. Pharmacol., 2024, 15, 1358323 CrossRef CAS PubMed.
- M. R. Sahu and A. C. Mondal, J. Neurosci. Res., 2020, 98, 796–814 CrossRef CAS PubMed.
Footnote |
† Electronic supplementary information (ESI) available: Supplementary tables and figures: the spiked internal standards for lipidomics and metabolomics and their concentration (Table S1); example LC-MS chromatograms from (A) proteomics, (B) lipidomics, and (C) metabolomics for comparison sample normalization. LC-MS chromatograms from (D) proteomics, (E) lipidomics, and (F) metabolomics for the GRN KO mouse brain (Fig. S1); volcano plots showing the influence of normalization methods on lipidomics (Fig. S2); volcano plots showing the influence of normalization methods on metabolomics (Fig. S3); example lipid concentrations measured from GRN KO vs. WT mouse brains (Fig. S4). Supplementary data (Excel): the complete datasets for quantitative proteomics, lipidomics and metabolomics in three different sample normalization methods and the GRN KO mouse brain. See DOI: https://doi.org/10.1039/d4an01573h |
|
This journal is © The Royal Society of Chemistry 2025 |
Click here to see how this site uses Cookies. View our privacy policy here.