DOI:
10.1039/D4CS01074D
(Review Article)
Chem. Soc. Rev., 2025,
54, 2832-2880
Implantable hydrogels as pioneering materials for next-generation brain–computer interfaces
Received
28th October 2024
First published on 4th March 2025
Abstract
Use of brain–computer interfaces (BCIs) is rapidly becoming a transformative approach for diagnosing and treating various brain disorders. By facilitating direct communication between the brain and external devices, BCIs have the potential to revolutionize neural activity monitoring, targeted neuromodulation strategies, and the restoration of brain functions. However, BCI technology faces significant challenges in achieving long-term, stable, high-quality recordings and accurately modulating neural activity. Traditional implantable electrodes, primarily made from rigid materials like metal, silicon, and carbon, provide excellent conductivity but encounter serious issues such as foreign body rejection, neural signal attenuation, and micromotion with brain tissue. To address these limitations, hydrogels are emerging as promising candidates for BCIs, given their mechanical and chemical similarities to brain tissues. These hydrogels are particularly suitable for implantable neural electrodes due to their three-dimensional water-rich structures, soft elastomeric properties, biocompatibility, and enhanced electrochemical characteristics. These exceptional features make them ideal for signal recording, neural modulation, and effective therapies for neurological conditions. This review highlights the current advancements in implantable hydrogel electrodes, focusing on their unique properties for neural signal recording and neuromodulation technologies, with the ultimate aim of treating brain disorders. A comprehensive overview is provided to encourage future progress in this field. Implantable hydrogel electrodes for BCIs have enormous potential to influence the broader scientific landscape and drive groundbreaking innovations across various sectors.

Wasid Ullah Khan
| Wasid Ullah Khan obtained his master's degree in chemistry from Hazara University, Pakistan. He is currently pursuing a PhD under the supervision of Prof. Qiang Zhang at the Changchun Institute of Applied Chemistry, Chinese Academy of Sciences. His research emphasizes on the advanced synthesis strategies of nanomaterials for applications in wearable and implantable biosensors. |
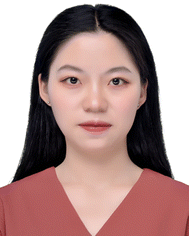
Zhenzhen Shen
| Zhenzhen Shen received her BS degree from Xinjiang University in 2021. She is currently a PhD candidate under the supervision of Prof. Qiang Zhang in the Changchun Institute of Applied Chemistry, Chinese Academy of Sciences. Her research focuses on the brain–machine interfaces including hydrogel bioelectronics, neural signal recording and neuromodulation of brain disorders. |
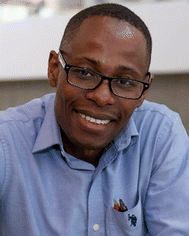
Samuel M. Mugo
| Samuel M. Mugo earned his PhD in analytical chemistry from the Memorial University of Newfoundland, Canada, in 2006. From 2007 to 2008, he held a post-doctoral fellowship at Queen's University, Kingston, ON, Canada, focusing on developing microfluidics sensor platforms for proteomics and personalized medical diagnostics. Since 2008, he has been a Professor of Analytical Chemistry at MacEwan University, Canada. His research group has over fifteen years of experience developing inexpensive Point-of-Need (PON) and wearable spectroelectrochemical sensors integrated with machine learning systems for mental health diagnostics and precision agri-food chemical tracking. Dr Mugo currently serves as the Associate Dean of Development for the Faculty of Arts & Science at MacEwan University. |
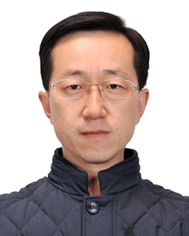
Hongda Wang
| Hongda Wang has been a principal investigator at the Changchun Institute of Applied Chemistry since 2008. He earned his bachelor's degree from the Department of Molecular Biology at Jilin University, PRC, in 1995. He received his doctorate from the Changchun Institute of Applied Chemistry, Chinese Academy of Sciences, PRC, in 2000. From 2001 to 2007, he worked as a postdoctoral research associate at the Biodesign Institute at Arizona State University, USA and served as a visiting professor there in 2008. He is also a recipient of the National Science Fund for Distinguished Young Scholars. His research focuses on studying the structure and function of cell membranes using various single-molecule techniques, particularly atomic force microscopy, molecular recognition imaging, super-resolution fluorescence microscopy, and cryo-electron microscopy. |
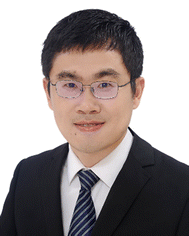
Qiang Zhang
| Qiang Zhang received his PhD from the Changchun Institute of Applied Chemistry, Chinese Academy of Science in 2012 and performed his post-doctoral studies at the University of Akron, US, from 2012 to 2013 and at the University of Alberta of Alberta, Canada, from 2013 to 2017. He joined the State Key Laboratory of Electroanalytical Chemistry, Changchun Institute of Applied Chemistry, Chinese Academy of Science as a full professor in 2017. He has been granted the Wiley 2022–2023 Top Downloaded Article Award (2024), Wiley 2021–2022 Top Cited Article Award (2023), and Materials Horizons 2019 Outstanding Review Paper Prize (2020). He is dedicated to scientific research in the field of life analysis, including brain–computer interfaces and wearable biosensors. |
1. Introduction
The brain is a highly intricate organ, composed of various regions that regulate thoughts, emotions, and behaviors.1–3 With more than 86 billion neurons and trillions of synapses transmitting information, the human brain is capable of perceiving the world, making decisions, executing complex tasks, and possessing an extraordinary ability to learn and adapt.4–9 Brain neurological disorders, marked by intricate pathophysiological mechanisms and limited treatment options, present a major challenge to human health and quality of life, emerging as a global public health concern.10 Scientists are working to unravel brain information and solve the puzzle of how neural circuits control human thoughts and behaviors. This emerging field of brain circuit research offers great potential for developing new methods to treat neurological illnesses. In recent years, brain–computer interfaces (BCIs) have been investigated as a means to directly link the brain with external devices, facilitating information exchange between them.11 These BCI systems can interact with brain neurons, record neural signals, and deliver feedback signals for neuromodulation. Signal acquisition is one of the key technologies of BCI. Non-invasive neural interfaces provide an accessible approach to capture brain signals and offer the advantage of large-scale monitoring with minimal risk. However, the dura mater, skull, and scalp act as low-pass filters, limiting signal detection to low-frequency bands such as alpha and beta waves. This filtering effect results in the loss of critical information needed for advanced BCI applications. To address this limitation, achieving multiplexed neural recording of millisecond-scale action potentials from individual neurons—the brain's fundamental computational units—is essential for developing high-performance BCIs. Moreover, precise regulation of neuronal activity in the brain remains a significant challenge. Many enduring questions in neurobiology are still unclear due to the absence of advanced technologies for accurately triggering neural signals. For instance, while deep brain stimulation has been utilized in clinical settings for several decades, its underlying mechanisms remain poorly understood. Additionally, many proposed neural prosthetic and therapeutic approaches face significant limitations due to the current inability to achieve precise stimulation, high electrode resolution, and multifunctional interactions with neuronal signals. Although BCIs have made some progress, there is still a long way to go in offering effective treatment for neurological disorders12 and helping to restore lost functions of patients.13
Neural electrodes stand as the key components of BCIs, facilitating both brain signal acquisition and neuromodulation.14–19 These technologies play a crucial role in the diagnosis and treatment of brain disorders such as stroke,20 Parkinson's disease,21 Alzheimer's,22 epilepsy,23 mental illness,24etc. To date, neural electrodes primarily have used traditional materials like metals, silicon, carbon, and conducting polymers. While these materials offer excellent conductivity, they are rigid and do not align well with the soft, flexible brain tissue. For instance, brain tissue has an elastic modulus of 1 kPa to 3 kPa, whereas metals and metal oxides have an elastic modulus greater than 100 GPa.25 When these rigid probes are implanted into brain tissue, the significant mechanical mismatch results in notable migration of glial cells encapsulating the electrode surfaces. The glial cells on the electrode interfere with efficient surface electron transmission, leading to a decrease in brain signals over time. Additionally, the mechanical mismatch creates micromotion between the rigid probes and brain tissue, which damages neural tissue and triggers immune responses. Moreover, metal-based electrodes cause photoelectric distortions during optogenetic neuromodulation because of their narrow energy band gap, resulting in signal noise and inaccurate results. In recent years, considerable efforts have been focused on developing flexible neural electrodes by depositing conductive layers on flexible substrates, such as metal nanoparticles and conductive polymers.25–28 However, these structural designs cannot address the intrinsic modulus mismatch, which still results in unwanted reactions like biofouling, fibrotic encapsulation, or fibrosis.29,30 Such foreign body response (FBR) and fibrotic separation from surrounding tissues negatively impact long-term human–computer interaction.31–33
Because of their unique similarity to biological tissues and their customizable features, hydrogels have rapidly become preferred materials for bridging the gap between humans and machines. Notably, hydrogels have found significant applications in tissue engineering and biomedicine due to their physicochemical properties closely resembling those of human tissues.34 Hydrogels' high water content provides a wet physiological environment and their soft nature reduces mechanical mismatch with biological tissues. However, pure hydrogels, almost insulators with low conductivity (<1 S m−1), cannot meet the requirements of high-quality neural signal transmission. Conductive hydrogels are typically developed by incorporating conductive additives into elastic 3D hydrogel networks. However, the mechanical and conductive stability of these hydrogels is often compromised due to phase separation between the conductive additives and polymer networks. It is essential to design the chemical structures and components of hydrogels to achieve optimal performance. Hydrogels offer the potential to match the modulus of neural tissue (1 kPa to 3 kPa) due to their adjustable Young's modulus. Mechanical damage and immune responses during implantable applications can be significantly reduced by aligning the modulus of hydrogel electrodes with that of brain tissue. Seamless interfaces between implantable electrodes and brain tissues enable to effectively acquisite neural signals and facilitate neuromodulation. Hydrogels can be feasibly designed with carboxyl, amide, or catechol groups to form covalent bonds or supramolecular interactions with brain tissues. These interactions can bring about seamless interfaces for BCIs. The transparency of hydrogels plays a crucial role in neuromodulation, particularly when combined with optogenetic techniques. Transparent hydrogels can effectively deliver laser or optical signals to target areas for precise optogenetic stimulation. Thus, hydrogels offer exceptional design flexibility in terms of mechanical, electrical, and biological properties.35–44 Because of these unique intrinsic properties45–48 and their customizable qualities,48–50 hydrogels have rapidly become preferred materials for connecting humans and machines. Studies have shown that hydrogels with tailored chemical and mechanical compositions can greatly enhance the long-term stability of implantable devices by providing highly lubricious surfaces,51–53 reducing biofouling53,54 and mitigating FBR.55–57 Implantable hydrogel electrodes have proven effective in maintaining reliable bioelectronic communication and modulation over extended periods for neurological treatment58 and neuroprosthetics.59,60
This review provides a comprehensive analysis of implantable hydrogel electrodes and their role in advancing BCI technology (Fig. 1). This review highlights the unique properties of hydrogels, such as conductivity, transparency, and modulus matching, and examines their potential applications in recording neural signals and neuromodulation for brain disorder treatment. This review discusses the key properties of implantable hydrogels and their effectiveness in recording various neural signals—ranging from single-neuron spikes to local field potentials (LFPs). Additionally, it explores various neuromodulation technologies enabled by implantable hydrogel electrodes, including optogenetics, electrical, and ultrasound methods. Finally, this review will provide numerous examples of using neuromodulation technologies in the treatment of brain disorders such as Alzheimer's disease, Parkinson's disease, epilepsy, depression, brain stroke, and chronic pain. By incorporating the latest developments and examining specific functionalities, this review seeks to be a comprehensive, timely, authoritative, and critical resource for researchers, thereby supporting ongoing advancements in the field of BCIs. This review consists of 4 sections. Section 1 offers a comprehensive examination of implantable hydrogel electrodes, focusing on their key properties such as conductivity, modulus match, adhesion, and transparency. Section 2 covers recent advancements in using implantable hydrogel electrodes for recording brain neural signals. Section 3 explores the applications of implantable hydrogel electrodes in various neuromodulation technologies. Section 4 presents successful examples of brain disorder treatments using neuromodulation technologies, investigating neural circuit modulations and therapeutic targets. This review concludes by addressing the current challenges and prospects of implantable hydrogel electrodes.
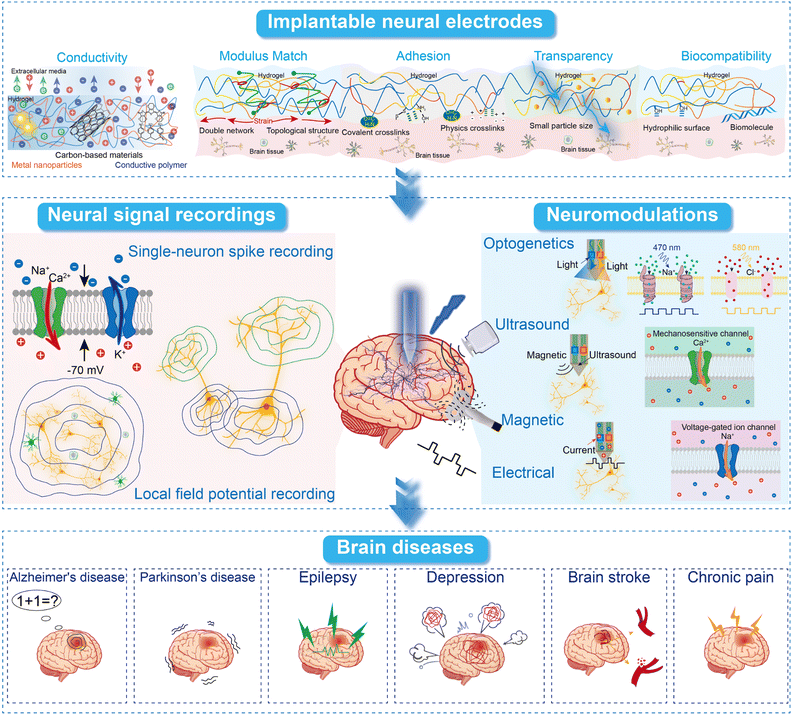 |
| Fig. 1 Schematic illustration of this review paper content, including hydrogel neural electrode properties, neural signal recording, neuromodulation, and brain disease treatment. | |
2. The critical properties of implantable hydrogel electrodes
Implantable hydrogel electrodes are surgically inserted into deep brain regions to capture detailed data or deliver precise targeted stimulation. These electrodes excel in capturing electrical activity data of neurons, providing signals with excellent signal-to-noise ratios (SNR) and high spatiotemporal resolution. Implantable hydrogel electrodes show great potential in BCIs for monitoring neural activities across different spatial scales and anatomical structures.61 To achieve effective bidirectional communications, the implantable hydrogels must possess specific properties such as conductivity, modulus match, adhesion, biocompatibility, and transparency, as depicted in Fig. 2. High conductivity allows the implantable electrodes to effectively capture electric signals from neural circuits and stimulate excitable cells through electrical inputs. For implantable applications, the modulus of electrodes must match that of brain tissue to minimize interfacial stress, enhance biocompatibility, and reduce inflammatory responses. A good modulus match ensures reliable, high-quality neural signal acquisition and stimulation, contributing to long-term functionality. Strong adhesion is also essential for creating a seamless interface that reduces interfacial resistance and improves electrical signal transmission. It also reduces micromovement between electrodes and brain tissues, minimizing the risk of mechanical damage to neural tissues. Transparency is essential for optogenetic neuromodulation, as it allows light to pass through the electrodes and reach the targeted area.
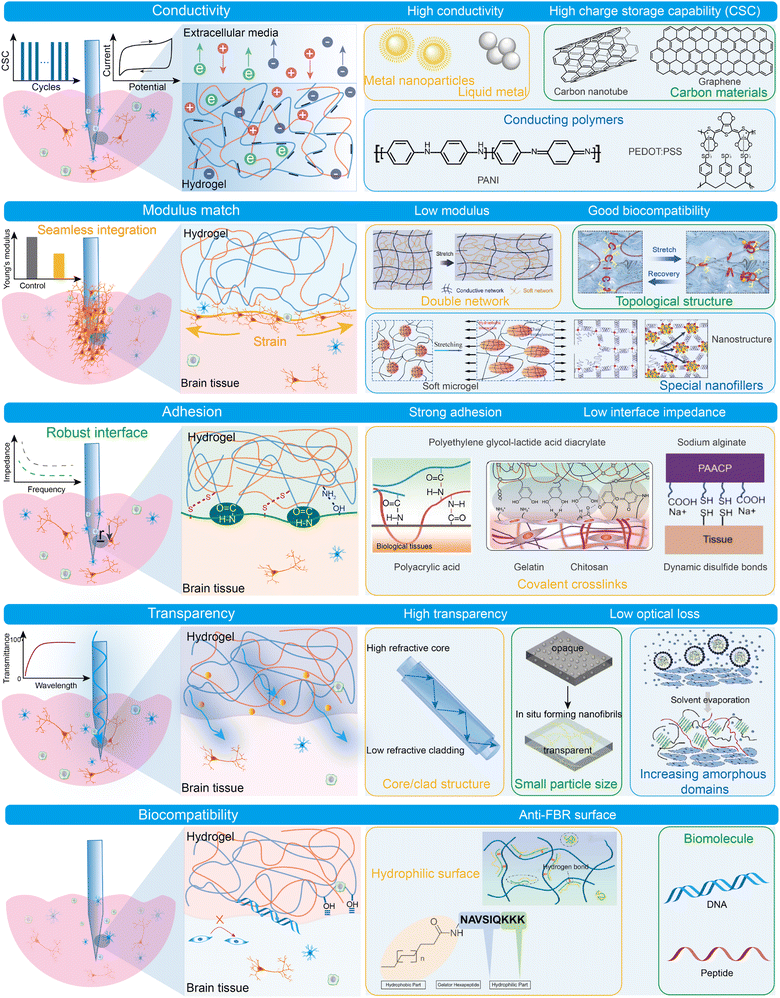 |
| Fig. 2 Characteristic properties of implantable hydrogel electrodes with strategic enhancement for advanced brain neural interfaces. | |
While implantable hydrogels show promise for neuromodulation in BCIs, significant challenges remain in optimizing their properties for practical use. Ongoing interdisciplinary research and innovation are addressing these issues, aiming to provide the necessary electrical, mechanical, and biological features for effective and reliable BCIs. Typical properties of implantable hydrogel materials reported in the literature are summarized in Table 1.
Table 1 Summary of electrochemical performance, Young's modulus, biocompatibility, and applications of the hydrogel materials
Hydrogel materials |
Electrochemical performance |
Young's modulus |
Biocompatibility |
Applications |
Ref. |
PPy/PVA-based hydrogels |
5.99 mS cm−1 |
119.8 kPa |
Skin-compatible |
Hierarchically structured supercapacitors |
62
|
β-Peptide based CNT hydrogels |
0.1345 S m−1, <0.2 MΩ |
∼1500 Pa |
Neuronal cell compatibility |
Soft neural interface |
63
|
PVA and hydroxypropyl cellulose hydrogels |
3.4 S m−1 |
1.3 MPa |
Skin-compatible |
Stretchable, artificial soft electronics |
64
|
GO and GelMA hydrogels |
9 mS cm−1 |
60 kPa |
Nerve regeneration support |
Nerve guidance conduits |
65
|
10 kΩ |
AgNCs/PAAm hydrogels |
5.61 S cm−1 |
∼7.6 ± 0.65 kPa |
Neuronal cell compatibility |
Chronic brain cortex signal recording |
66
|
2.5 kΩ |
PPy, aramid nanofibers, and PVA hydrogels |
≈80 S cm−1 |
≈9.4 MPa |
Neuronal cell compatibility |
Soft bioelectronics |
67
|
PEDOT:PSS/PVA DN hydrogels |
≈10 S cm−1 |
≈460 kPa |
Skin and muscle tissue compatibility |
Soft bioelectronics |
68
|
AgNWs–PVA hydrogels |
≈1739 S cm−1 |
240 kPa |
Neuronal cell compatibility |
Soft electronics |
69
|
EGaIn liquid metal-doped PVA hydrogels |
4628 S m−1 ≈ 6 Ω |
15.44 MPa |
Skin-compatible |
Real-time monitoring of biosignals |
70
|
PU hybrid composite hydrogels |
12.5 S cm−1 |
16.21 MPa |
Neuronal cell compatibility |
Supporting neural stem cell growth and differentiation |
71
|
GO/PAA composite hydrogels |
>30 S cm−1 |
>0.069 MPa |
Biocompatible with stem cells |
Promoting the differentiation of NSCs |
72
|
3D printable BC-CPH hydrogels |
>11 S cm−1 |
<1 MPa |
Biocompatible with various tissues |
All-hydrogel bioelectronic interfaces |
73
|
PEDOT:PSS electrically conductive hydrogels |
47.4 S cm−1 |
32 ± 5.1 kPa |
Neuronal cell compatibility |
Localized low-voltage neuromodulation |
74
|
Acid-treated PEDOT:PSS hydrogels |
880 S m−1 |
6.5 GPa |
|
Wearable energy-storage devices |
75
|
0.53 Ω cm2 |
PEDOT:PSS nanofibril hydrogels |
40 S cm−1 in deionized water |
∼2 MPa |
|
All-hydrogel bioelectronic interfaces |
76
|
Microgels/PPy hydrogels |
1.17 S m−1 ≈ 23.5 kΩ |
17–32 kPa |
Neuronal and glial cell compatibility |
Neural signal recording and neuromodulation |
39
|
Cucurbit[8] uril supramolecular hydrogels |
52 S m−1 |
<5 kPa |
|
Monitoring electromyography signals |
77
|
PAM-PEDOT conductive hydrogels |
≈0.05 S m−1 |
2 kPa |
Minimizing inflammatory reactions |
Soft bioelectronics |
78
|
Polyrotaxane topological hydrogels |
27 S m−1 |
45 kPa |
Neuronal cell compatibility |
Brain signal monitoring, neuromodulation, and stroke treatment |
43
|
25.4 kΩ |
PVA/CNF/PEDOT:PSS hydrogels |
0.3 S m−1 |
3.71 MPa |
Tendon tissue compatibility |
Implantable electronic tendon |
79
|
2.1 Conductivity
Hydrogels are typically insulating materials that require the addition of conductive fillers to achieve a high electrical conductivity. It is crucial to attain the percolation of continuous conductive phases inside hydrogel networks. Typically, conductive hydrogels showed an abrupt increase in conductivity at a certain content of conductive fillers, referred to as percolation content. The content influence of conductive fillers on conductivity can be described by percolation theory. Below a percolation threshold, conductive fillers separately dispersed within hydrogel networks and composite hydrogel conductivity moderately increased with conductive filler content. Above a percolation threshold, isolated conductive fillers established stable contacts and formed cohesive networks. The conductivity of hydrogels exhibited a sharp rise with filler content, spanning several orders of magnitude. Various conductive fillers, including metal nanoparticles (like Ag and Au),66,80,81 liquid metals,70 carbon materials,82,83 and conducting polymers,67,68,84,85 have been utilized to create electrically conductive phases within hydrogel networks. For example, a conductive hydrogel composite can be formed by combining silver nanowires (AgNWs) and poly(vinyl alcohol) (PVA) using a synergistic approach involving freezing and salting-out processes, as illustrated in Fig. 3A.69 This method produces a hierarchical hydrogel structure and increases the localized concentration of AgNWs by promoting ongoing phase separation. In this study, the resulting conductive hydrogel composites exhibited an extremely high electrical conductivity (1739 S cm−1). Remarkably, these composites also retained a high water content (87%) and demonstrated excellent extensibility (480%). The AgNWs–PVA hydrogel composites show promise for applications in underwater soft robots and bioelectronic interfaces. Liquid metals can also serve as conductive fillers for achieving highly conductive hydrogel materials. The self-sintering behavior of liquid metals on the bottom surface helps form conductive networks, resulting in extraordinarily high conductivity.86,87 Eutectic gallium indium (EGaIn) has recently gained significant attention due to its high thermal and electrical conductivity, low vapor pressure, and low viscosity.88,89 A series of EGaIn composite hydrogels were synthesized by combining EGaIn with various polymer networks, including PVA, poly(acrylic acid) (PAA), polyacrylamide (PAAm/PAM), and biopolymers such as polysaccharide and hydrocellulose.90–93 For instance, a hydrogel composed of PVA doped with EGaIn exhibits a conductivity of 217
895 S m−1, a tensile stress of 15.44 MPa, a tensile strain of 704%, and excellent fatigue resistance, as shown in Fig. 3B.70 The excellent performances of conductive hydrogels are attributed to the creation of PVA crystalline areas and the self-sintering behavior of EGaIn.
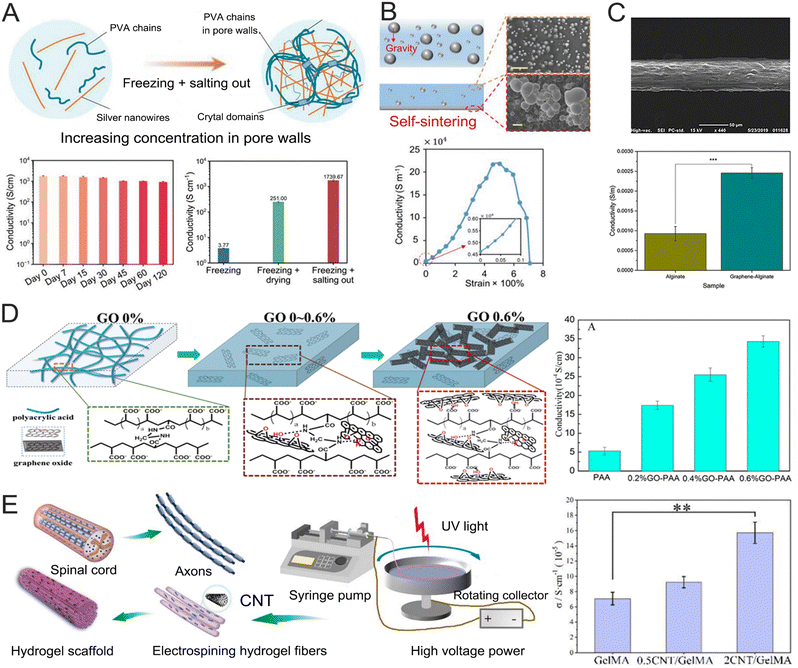 |
| Fig. 3 Metal nanoparticles, liquid metals, and carbon materials to improve the conductivity of hydrogels. (A) Schematic illustrating the fabrication procedure of AgNWs–PVA hydrogel composites by combining freezing and salting-out treatments (top) and the conductivity of hydrogel composites (bottom). Reproduced with permission from ref. 69. Copyright 2024, Wiley Online Library. (B) Schematic and SEM images of the mechanism of liquid metal self-sintering (top) and conductivity–strain curve of an EGaIn liquid metal-doped PVA hydrogel (bottom). [Reproduced with permission from ref. 70]. Copyright 2023, Wiley Online Library. (C) SEM analysis of graphene-Alg fibers (top) and the conductivity of pure Alg and graphene-Alg microfibers (bottom). Reproduced with permission from ref. 94. Copyright 2020, Frontiers. (D) The schematic model of the GO–PAA hydrogel structure (left) and the conductivity of PAA and GO–PAA hydrogels (right). Reproduced with permission from ref. 72. Copyright 2018, Elsevier. (E) Schematic representation of CNT/GelMA hydrogel fiber preparation inspired by the spinal cord and axons (left) and electrical conductivity of hydrogel fibers (right). Reproduced with permission from ref. 95. Copyright 2024, Elsevier. | |
Carbon-based nanomaterials, such as carbon nanotubes (CNTs) and graphene, are increasingly used as conductive fillers in hydrogel networks due to their high electrical conductivity and outstanding stability. The CNTs' exceptional stability in wet environments has made them a popular alternative to metallic nanomaterials in conductive nanocomposite hydrogels.96 Graphene, a carbon-based conducting filler, exhibits high in-planar electrical conductivity, with thermal conductivities and electron mobilities comparable to those of metals.97,98 Various forms of graphene are often combined with hydrogels to enhance their properties, as shown in Fig. 3C.94 Graphene oxide (GO), in particular, is highly suitable for biological platforms due to its ability to improve interactions with mammalian cells, microbes, and proteins at the interface. GO has been incorporated into PAA hydrogels to tune conductivity and mechanical properties for biomedical applications (Fig. 3D).72 The incorporation of GO significantly enhanced the hydrogel conductivity (35 × 10−4 S cm−1), porosity, and mechanical properties. The GO–PAA hydrogel demonstrated good compatibility with bone marrow-derived mesenchymal stem cells. When subjected to electrical stimulation, the hydrogel's electro-mechanical coupling could alter the morphology of adhesive cells and regulate the cell's cytoskeleton, further promoting the development of neural stem cells (NSCs). CNTs are widely favored as conductive additives in hydrogels due to their consistent electroactivity and chemical inertness. CNTs are particularly useful in applications such as biosensors, tissue scaffolds, and neuro devices.99,100 Coatings made from CNTs on neural devices have demonstrated the ability to enhance charge transfer, lower impedance, and improve neural recording both in vitro and in vivo.101 These coatings have been demonstrated to enhance signaling and cellular communication with neural cells upon electrical stimulation. For example, a conductive aligned CNT/methacrylate acylated gelatin (CNT/GelMA) hydrogel fiber was created using a rotating liquid bath electrospinning technique, as illustrated in Fig. 3E.95 The CNT-containing fibers demonstrated the micro-scale aligned structure, conductivity, and mechanics of brain axons. This work investigated how CNTs are distributed within the fibers and their impact on conductivity, water content, degradation, and mechanical properties. Additionally, this work evaluated the adhesion, proliferation, and differentiation of PC12 cells and NSC on the fibers with electrical stimulation.
Conducting polymers were incorporated as conductive fillers within hydrogel networks, which exhibited unique properties due to their high volumetric capacitance. In capacitive biosensors, the high volumetric capacitance greatly improves detection sensitivity by allowing greater charge storage.102 The electronic conductivity of these conducting polymers stems from the delocalization of π-bonded electrons along their conjugated backbone structures. To enhance their conductivity, conductive polymers are often combined with various ionic dopants. The unique structural features of these composite materials form electrical double layers (EDL) within the nanoporous channels of composite hydrogels, contributing to their high volumetric capacitance.103,104 Hydrogel electrodes comprised of percolated conducting polymers, such as poly(3,4-ethylenedioxythiophene):poly(styrene sulfonate (PEDOT:PSS), polypyrrole (PPy), and polyaniline (PANI), exhibit remarkable electrical conductivity. For instance, pure PEDOT:PSS hydrogels were prepared by treating PEDOT:PSS with concentrated sulfuric acid followed by thermal treatment (Fig. 4A).75 The acidic environment caused the PEDOT chain to expand, shifting from a coiled to a linear conformation, which extended its conjugation length. This conformation change promoted π–π stacking and hydrophobic interactions between PEDOT chains, leading to the formation of physical crosslinks and the development of PEDOT networks. Notably, the resulting hydrogels achieved a conductivity of 880 S m−1 and a volumetric capacitance of 202 F cm−3. Additionally, an annular gelatin methacrylate–polyaniline hydrogel (Gel/Pani) was prepared for electrical stimulation, displaying good conductivity (2.26 S m−1), biocompatibility, and three-dimensional (3D) porous nanostructure (Fig. 4B).105 The unique structure of the hydrogel made it an ideal candidate for neural interfaces. The implanted annular hydrogel was used as a secondary coil to enable wireless electrical stimulation via electromagnetic induction, which supported neural stem cell proliferation, neuronal differentiation, and the construction of functional neural networks. To achieve high electrical conductivity, incorporating a significant amount of conducting polymers into hydrogel networks is often necessary, but this compromises the mechanical properties, limiting their usefulness as bioelectronic interfaces. To overcome this limitation, a bi-continuous conducting polymer hydrogel (BC-CPH) was developed, offering exceptional conductivity in physiological environments without compromising mechanical properties. This hydrogel exhibits high electrical conductivity (over 11 S cm−1), stretchability (over 400%), and fracture toughness (over 3300 J m−2). Additionally, it is compatible with advanced fabrication techniques like 3D printing, as illustrated in Fig. 4C.73 This work successfully showcased the use of multi-material 3D printed hydrogels for long-term electrophysiological monitoring and stimulation of tissues and organs.
 |
| Fig. 4 Conductive polymers to improve the conductivity of the hydrogel. (A) Cross-section SEM images of a freeze-dried PEDOT:PSS hydrogel before and after the treatment with concentrated H2SO4 (left), plot of the solid content of the hydrogel versus the concentration of H2SO4 used for acid treatment (middle), and plot of the conductivity of the hydrogel versus its solid content (right). Reproduced with permission from ref. 75. Copyright 2017, Wiley Online Library. (B) Schematic diagram of hydrogel-mediated wireless electrical stimulation for accelerating the NSCs into functional neurons (left) and the conductivity of the GelMA hydrogel and Gel/Pani hydrogel (right). Reproduced with permission from ref. 105. Copyright 2024, Wiley Online Library. (C) Schematic illustration of mechanisms (left) and plots (right) for electrical conductivity of BC-CPH. Reproduced with permission from ref. 73. Copyright 2023, Nature. | |
2.2 Modulus match
Most rigid electrode materials such as silicon, gold, platinum (Pt), titanium nitride, iridium, tungsten, and tin, have high moduli (exceeding 1 GPa), which are several orders of magnitude greater than those of neural tissues (1 to 3 kPa).25,106,107 Over the past few decades, various innovative materials and lab-scale devices have been developed to address the biomechanical mismatch between electronics and biological tissues.27 Flexible polymer materials like polyimide (PI), polydimethylsiloxane (PDMS), and polyurethane (PU) have been used to reduce this modulus gap.108 However, their Young's moduli, typically ranging from 1 MPa to 1 GPa, remain significantly higher than those of brain tissues. Hydrogels, with their tunable mechanical properties, can closely replicate the softness of brain tissue.109 The modulus match is essential for developing biocompatible and high-performance neural interfaces, which enhance brain–computer communication and control. Various approaches have been employed to adjust the hydrogel modulus to align with the mechanical characteristics of the nervous system while preserving good electrical conductivity. These strategies include incorporating double networks (DNs), designing specific topological structures, and using special nanofillers. Typically, increasing crosslinking density in hydrogel networks improves their strength and stiffness but reduces their stretchability. Therefore, it is crucial to combine covalent and non-covalent crosslinking methods and carefully adjust the crosslinking density to achieve desired mechanical properties.
DN hydrogels have garnered significant interest due to their unique approach to dissipate mechanical loads. They are comprised of a tightly cross-linked network that can break to dissipate energy and a loosely cross-linked network that provides extensibility, as shown in Fig. 5A.110 Various physically cross-linked networks—such as those linked by ionic, hydrogen bonds, or hydrophobic interactions—111 have been used as sacrificial networks to enhance the reversibility of DN hydrogels. A densified DN hydrogel was developed using PVA and PEDOT:PSS, followed by an acid treatment to create highly conductive networks (Fig. 5B).68 This hydrogel exhibits remarkable stretchability (150%), a low elastic modulus (460 kPa), a low swelling ratio (0.20), and good conductivity (10 S cm−1), making it suitable for use as an implantable electrode. Additionally, molecular chain entanglement was used as a non-covalent crosslinking site in the DN structure, effectively transferring tensile force, releasing stored elastic energy, and thereby tuning its tensile strength and modulus.112 Sortase A (SrtA)-cleavable peptide sequences were used as crosslinkers in DN hydrogel networks (Fig. 5C).113 These SrtA sequences can reversibly break and reform in response to mechanical stress, thereby affecting the hydrogels' stiffness and extensibility. The hydrogels demonstrated a Young's modulus of 21 kPa, a fracture strain of 2033%, and a fracture energy of 9410 J m−2. Notably, these hydrogels can recover their mechanical properties by reassembling the cross-linkers through an enzyme-catalyzed reaction. Srt A serves a catalyst for both proteolysis reactions and to mediate peptide bond formation. The products of proteolysis can reform covalent linkages with the help of SrtA, leading to reversible bond rupture and formation.
 |
| Fig. 5 Double network to improve the stretchability of hydrogels. (A) Stretchability of the DN hydrogel containing conductive network and soft network. Reproduced with permission from ref. 110. Copyright 2003, Wiley Online Library. (B) Fabrication of the PEDOT:PSS/PVA DN hydrogel (top) and mechanical and electrical properties of the PEDOT:PSS/PVA DN hydrogel (bottom). Reproduced with permission from ref. 68. Copyright 2022, Wiley Online Library. (C) Schematic illustration of the engineering of strong and reversible double-network hydrogels based on force-coupled enzymatic reactions (top) and optical images of the stretching of the hydrogels in the absence and presence of SrtA (bottom). Reproduced with permission from ref. 113. Copyright 2022, Wiley Online Library. | |
The topological structure used in crosslinking sites offers an alternative method for adjusting the modulus and strength of hydrogels. A mechanically interlocked polyrotaxane was utilized as a cross-linker within hydrogel networks, providing significant conformational freedom through its mobile junctions.114 This polyrotaxane consists of a polyethylene glycol (PEG) backbone with tens of α-cyclodextrin (α-CD) side chains threaded onto it. Topological hydrogels were created by polymerizing polyrotaxane with N-isopropylacrylamide (Fig. 6A).114 When mechanical stress was applied to these hydrogels, the α-CD segments shifted along the PEG chains, which dissipated the applied energy. This resulted in a low elastic modulus (8.32 kPa), high stretchability (1463%), and tensile strength (31.4 kPa). The mechanical performances of these topological hydrogels are significantly different from those of conventional covalently crosslinked hydrogels. In a similar study, a topological supramolecular hydrogel was created using polyrotaxane (crosslinker), acrylamide (monomer), and PEDOT:PSS (conductive filler), as shown in Fig. 6B. This topological hydrogel exhibited an elastic modulus of as low as 45 kPa, an elongation at break of 4363%, and a conductivity of 27 S m−1.43 These distinctive properties make the hydrogels suitable as implantable electrodes for effectively recording brain neural signals and performing neuromodulation.
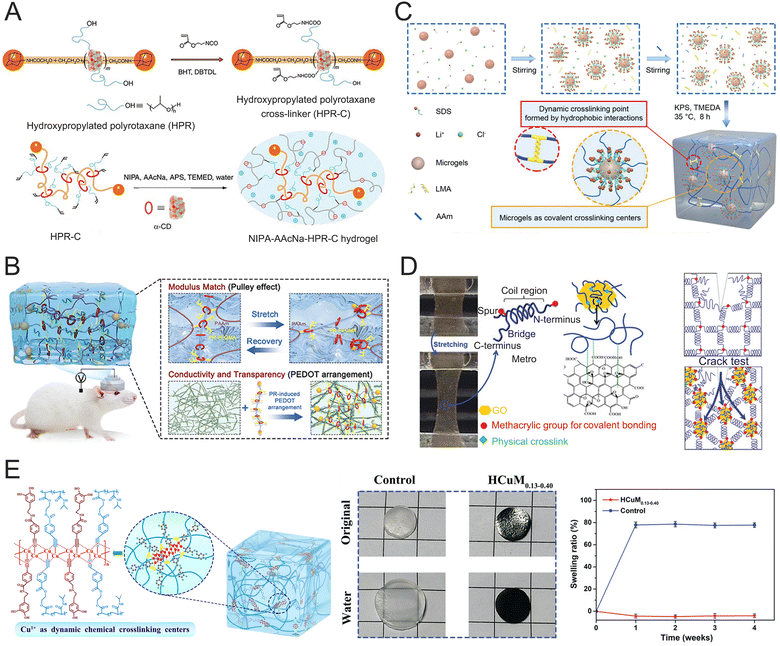 |
| Fig. 6 Hydrogel structure design to improve stretchability. (A) Preparation of the topological hydrogels using a nonionic cross-linker. Reproduced with permission from ref. 114. Copyright 2014, Nature. (B) Schematic diagram of the topological hydrogel structure and performance as an implantable neural probe. Reproduced with permission from ref. 43. Copyright 2023, Wiley Online Library. (C) The synthesis and structure of microgel-crosslinking hydrogels. Reproduced with permission from ref. 115. Copyright 2022, Wiley Online Library. (D) Formation of an MeTro/GO hybrid hydrogel (left) and the crack test on the MeTro/GO hybrid gel (right). Reproduced with permission from ref. 116. Copyright 2016, Wiley Online Library. (E) The swelling curves of the pNIPAm hydrogel and HCuM0.13–0.40 embedded in rat epidermis. Reproduced with permission from ref. 117. Copyright 2022, Wiley Online Library. | |
Soft microgels (diameter: 200 nm) are used as micro-crosslinking centers in hydrogel networks to tune modulus and strength (Fig. 6C).115 When the hydrogels are subjected to external stress, the deformation capability of microgel crosslinkers results in a low modulus (59 kPa), high stretchability (1866%), and strong tensile strength (1.01 MPa). The hydrogels exhibit excellent physicochemical compatibility with neural tissues without triggering any FBR. In addition to microgels, GO nanoparticles were also used to tune the modulus of protein hydrogels while providing good conductivity.116 Incorporating GO nanoparticles into recombinant human tropoelastin (MeTro) increased the elastic modulus from 12.6 kPa to 19.3 kPa and improved the rupture strain from 140% to 203%, as shown in Fig. 6D. Due to their tunable electrical and mechanical properties, these hydrogels hold promise for applications in tissue engineering and regenerative medicine.
Hydrogels typically suffer from serious swelling issues for long-term applications in aqueous environments.118–121 These hydrogels often underwent significant volumetric expansions, which significantly impaired their modulus and conductivity.122,123 When hydrogel electrodes were exposed to cerebrospinal or interstitial fluids, these body fluids can also cause swelling, thus affecting their Young's modulus and conductivity. Therefore, it is crucial to explore anti-swelling conductive hydrogels for implantable neural electrodes. In a study, interpenetrating polymer networks of poly(Cu-arylacetylide) and pNIPAm chain (poly(Cu-NIPAm) hydrogels were synthesized to achieve excellent electron conductivity, anti-swelling, and antibacterial properties (Fig. 6E).117 The poly(Cu-NIPAm) hydrogel exhibited a swelling ratio of 5% in DI water for four days, while a swelling ratio of 57% was observed for the pNIPAm hydrogel. Moreover, it maintained a constant size for three weeks embedded in rat subcutaneous tissue, demonstrating a superior anti-swelling performance than pNIPAm (in vivo swelling ratio: 78%). The outstanding anti-swelling property is attributed to the accumulation of poly(Cu-arylacetylide) chains within the interpenetrating networks.
2.3 Adhesion
It is crucial for implantable electrodes to possess strong adhesion for facilitating a stable and durable integration between electrodes and brain tissue.124 A seamless interface helps to prevent electrode delamination or separation from the tissues, reducing the risks of signal loss, inflammation, and tissue damage.125 Biological tissues contain various functional groups such as carboxylic acids, mercaptans, amino groups, and hydroxyl groups. Hydrogels can form strong bonds at the interface by interacting with these reactive groups on tissue surfaces, through covalent bonds, ionic interactions, hydrogen bonds, and dynamic bonds.126 For instance, when chitosan or gelatin hydrogels linked with N-hydrosuccinimidyl ester (DST) were attached to the tissue surface, a strong connection was formed between hydrogels and the tissue surface by the amidation reaction between the DST and amine groups (Fig. 7A).127 This covalent crosslinking significantly increased the adhesion strength (>650 J m−2) and enhanced the stability of the hydrogel–tissue interface. In another example, bioelectronic–tissue interfaces were created using DN hydrogels made from polyethylene glycol-lactide acid diacrylate (PEG-LA-DA) and sodium alginate (Alg) (Fig. 7B).128 Covalent amide bonds were formed through the chemical reaction between amine groups on tissue surfaces and the carboxylic acid groups of the hydrogels. These hydrogels remained stably adhered to wet tissue surfaces for durations ranging from several days to several months. Various in vivo applications were demonstrated using live animal models, including battery-free optoelectronic systems for deep-brain optogenetics and subdermal phototherapy, wireless millimeter-scale pacemakers, and flexible multielectrode epicardial arrays. In contrast to the gradual development of covalent bonds, a special interfacial interaction was developed using electrooxidation to create immediate and robust adhesion to various tissue surfaces (Fig. 7C).129 The hydrogels were made from bovine serum albumin, Alg-dopa, and PAA. The Alg-dopa can be electrically oxidized into dopaquinone groups, which form covalent bonds with amino groups on tissue surfaces for long-lasting adhesion. Additionally, PAA and unreacted dopa groups establish interfacial hydrogen bonding with the tissue surfaces. This combination of interfacial covalent bonding and hydrogen interaction results in an impressive adhesion strength of 1268 J m−2, outperforming other reported bio-adhesives. A similar design has been found in an electrical bioadhesive interface, which utilized covalent bonds, hydrogen bonding, and electrostatic interactions to achieve rapid (<5 s), strong (>400 J m−2), and detachable integration of the hydrogels on a variety of wet tissues.130 The hydrogels’ exceptional bioadhesive properties allow them to serve as implantable electrodes, capable of recording in situ epicardial electrocardiograms and electrically stimulating the sciatic nerve. However, in vivo hydrogel swelling can lead to expansion at the hydrogel–tissue interface, compressing surrounding biological tissues, which often causes tissue irritation and a FBR. To address this, anti-swelling hydrogels have been developed for hydrogel–tissue interfaces by incorporating hydrophobic segments. One example is the introduction of hydrophobic poly(vinyl butyral) into PAA-chitosan-grafted N-acetyl-L-cysteine hydrogels, which significantly reduces water infiltration while still allowing the adhesive groups to migrate to the hydrogel–tissue interface (Fig. 7D).131 Moreover, the N-acetyl-L-cysteine segment enables the hydrogels to form dynamic disulfide bonds with tissue surfaces, resulting in reversible covalent adhesion. No reduction in adhesion strength loss was observed after 8 repeated peel tests.
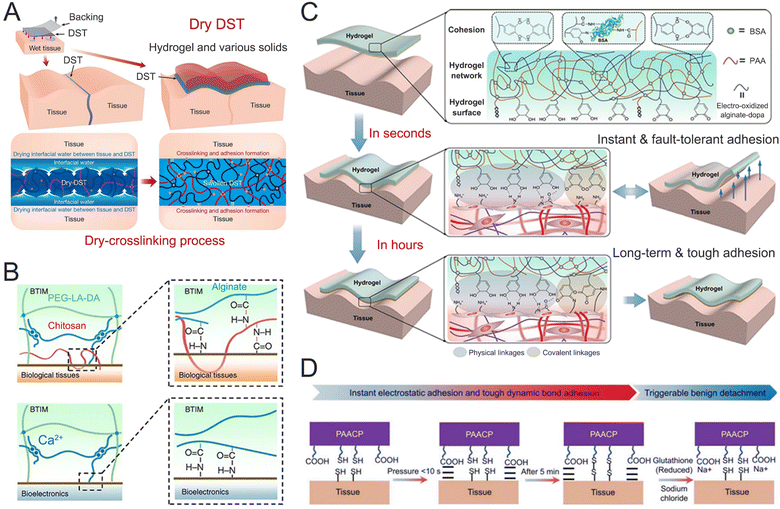 |
| Fig. 7 Covalent crosslinking to improve the adhesion of the hydrogel. (A) The mechanism for the DST integrates the drying of interfacial water by hydration and swelling of the dry DST, temporary crosslinking, and covalent crosslinking. Reproduced with permission from ref. 127. Copyright 2019, Nature. (B) Mechanisms of interfacial bonding to the surfaces of biological tissues (top) and bioelectronic devices (bottom). Reproduced with permission from ref. 128. Copyright 2021, Nature. (C) Schematic illustration of the adhesion and fault-tolerant mechanism of electro-oxidation hydrogel tape. Reproduced with permission from ref. 129. Copyright 2021, Nature. (D) Schematic of PAACP adhesion and detachment mechanisms. Reproduced with permission from ref. 131. Copyright 2023, Wiley Online Library. | |
2.4 Transparency
Optogenetic neuromodulation requires high transparency to enable effective optical stimulation. Conventional light-guiding systems typically use solid-state optical materials such as glass and inorganic plastics, which are not compatible with biological systems. As such exploring transparent hydrogels is essential for developing durable neural interfaces that seamlessly integrate optical and electrical functions. Polymers like PEG, PAAm, and PVA are commonly used to fabricate transparent hydrogels due to their low optical loss and refractive index. For instance, PEG-based hydrogels have been used for in vivo optical sensing, implanted through a 1-cm-long skin incision on the back of mice. The pigtail fiber was securely attached to the skull, allowing stable optical coupling to the hydrogel while the animal was in an awake and free-moving state. The leakage of light from the hydrogel into the surrounding tissue was easily visible through the thin skin, indicating a 40-fold increase in the illuminated area compared to using a bare laser fiber, as shown in Fig. 8A.132 Bulk hydrogel materials have a refractive index similar to that of surrounding tissues (1.33–1.51), which reduces light transmission efficiency. Typically, effective light guiding requires the elimination of light refraction at the interface between the core and cladding when the angle of incidence surpasses a critical angle. To achieve light guiding, the hydrogels were designed with core/cladding structures consisting of high refractive index cores and low refractive index cladding. This setup allows light to pass from a denser medium to a less dense one. For instance, a core/clad step-index hydrogel was prepared using 40 wt% PAAm as the core and 20 wt% PAAm with 2 wt% fixed Alg as the cladding (Fig. 8B).133 The refractive index increases progressively with higher PAAm density, allowing efficient light guidance through total internal reflection at the interface. In another example, step-index hydrogel optical fibers were fabricated using PEG hydrogels with a refractive index of 1.46 as the core and Alg hydrogels with a refractive index of 1.331 as the cladding (Fig. 8C).134 These hydrogel optical fibers were successfully used for in vivo optical sensing in response to oxygen and nitrogen fluctuations. Light transmittance is heavily affected by the degree of scattering and obstruction of incident light within materials. When hydrogel constituents exceed one-tenth of the incident light's wavelength, light scattering increases significantly leading to reduced transmittance. To enhance light transmission, the size of hydrogel components should be minimized. For instance, a transparent and conductive composite hydrogel was created using gelatin and glycerol as hydrogen bond donors/acceptors to enable topological structure reorganization of bacterial cellulose (BC), as shown in Fig. 8D.135 As a result of hydrogen bond transitions, the original BC nanofibrils generated numerous ultra-fine nanofibrils, reducing light scattering and significantly increasing the transparency of the hydrogel. The pure BC hydrogel showed a transmittance of 7% at 450 nm, 14% at 550 nm, and 22% at 650 nm. In contrast, the restructured BC hydrogel exhibited a much higher transmittance, reaching 57% at 450 nm, 69% at 550 nm, and 77% at 650 nm. This enhanced transparency was achieved through precise alignment of the hydrogel's structural components, which minimized light scattering and allowed for more efficient light transmission. Another example includes nanocellulose reinforced PVA hydrogels, which displayed an optical transparency of 90–95% at 550 nm, accomplished by reducing the size of phase-separated polymer-rich and water-rich regions and forming nanoscale semi-crystalline domains.136
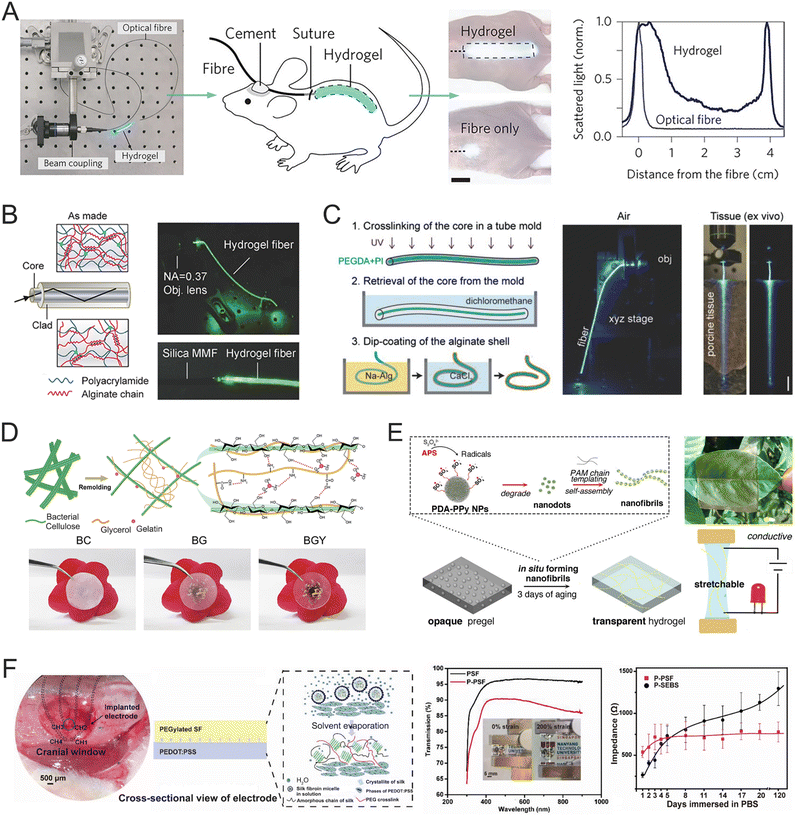 |
| Fig. 8 Different strategies to improve the transmittance of hydrogels. (A) Hydrogel implants in vivo. Reproduced with permission from ref. 132. Copyright 2013, Nature. (B) Optical properties of step-index, core/clad Alg-PAAm hydrogel fibers. Reproduced with permission from ref. 133. Copyright 2016, Wiley Online Library. (C) The fabrication of core-cladded fibers (left) and the light guidance in air (right). Reproduced with permission from ref. 134. Copyright 2015, Wiley Online Library. (D) Schematic illustration of the formation of ultra-fine BC nanofibrils and the hydrogen bonding between cellulose, gelatin, and glycerol (top) and optical images exhibiting the transparency of the pure BC hydrogel, cellulose–gelatin (BG) hydrogel, and bacterial cellulose nanofibril-reinforced (BGY) hydrogel (bottom). Reproduced with permission from ref. 135. Copyright 2023, Elsevier. (E) Schematic illustration of the formation of PDA-PPy nanofibril transparent hydrogel (left) and the transparent, conductive, and stretchable properties of this hydrogel (right). Reproduced with permission from ref. 137. Copyright 2018, ACS Publications. (F) Design of a highly transparent, stretchable, and stable hydrogel electrode based on PEDOT:PSS and silk fibroin. Reproduced with permission from ref. 138. Copyright 2021, Wiley Online Library. | |
As aforementioned, hydrogels need a significant amount of conductive components to achieve good conductivity. While transparent and conductive hydrogels can be made using free ionic electrolytes as conductive components, ion diffusion and exchange between the hydrogels and surrounding tissues can affect the stability of signals from implanted devices and potentially pose risks to the biological environments at the implantation site. Intrinsic electron conductive materials can provide stable signal transmission at the interfaces between implants and tissues. However, these black or deep-colored materials can reduce the transparency of the hydrogel rendering it opaque. Thus, balancing high transparency and good conductivity in hydrogels is a considerable challenge. Various approaches have been explored to address this tradeoff, such as inducing structural rearrangement and controlling the size of conductive components. For example, a transparent, conductive, and self-adhesive hydrogel was developed by in situ formation of polydopamine (PDA)-doped PPy nanofibrils within PAM networks (Fig. 8E).137 The hydrophilic nanofibrils were seamlessly integrated into the hydrophilic polymer phase, forming a nanomesh that provided continuous conductive channels and high intrinsic conductivity (12 S m−1). The hydrogel maintained a transmittance of 70% across the visible spectrum, even at a thickness exceeding 1 mm. This enhanced transparency is attributed to the in situ-formation of conductive nanofibrils dispersing throughout the hydrogel networks, which created nanoscale networks and improved light transmission. The PDA component effectively bonded with both hydrophobic and hydrophilic phases, ensuring proper integration of the conductive PPy into the hydrophilic PAM phase. In another example, a transparent and conductive hydrogel electrode was created using PEDOT:PSS and PEGylated silk protein, achieving over 85% transparency from visible to near-infrared regions and low interfacial impedance (Fig. 8F).138 The PEGylated silk fibroin enhances optical transparency by reducing crystalline β-sheets and increasing amorphous domains, while the strong interpenetrating interface with PEDOT:PSS reduces light scattering and reflection. This hydrogel electrode also exhibited stable electrical performance for 4 months, attributed to its stable micro-nano-structure.
2.5 Porosity
Hydrogels are composed of cross-linked polymer networks with highly porous structures. The porosity of conducting hydrogels shows significant influences on various properties such as stress relaxation, electrical conductivity, and cell migration.139–143 The porous structure of hydrogels enables stress dissipation through pore deformation when subjected to external forces. This stress–relaxation property is essential for ensuring compatibility with soft tissues, particularly in interactions with brain tissue, as it effectively mitigates chronic inflammation and minimizes tissue scarring. In addition, the size and distribution of pores directly influence the mechanical strength of hydrogels.144 Uniformly distributed microporous structures can provide a stable supporting structure, while excessively high porosity in hydrogels can significantly reduce their intrinsic mechanical modulus, leading to substantial deformation and limited load-bearing capacity. Various techniques have been developed to tailor the porous structure of hydrogels, including microfluidics,145 porogen leaching,146,147 freezing,148,149 foaming,150,151 and phase separation.152 An innovative phase separation approach was proposed to construct hydrogels with super macroporous (SMP) structures and improved mechanical toughness through enhanced polymer aggregation.153 The SMP hydrogel was prepared by crosslinking Alg using Ca2+ as a crosslinker with PVA as a phase separation enhancer (Fig. 9A). The PVA facilitated localized aggregation of the Alg during gelation, leading to the formation of larger pores and enhancing mechanical properties. The resulting SMP hydrogel exhibited Young's modulus of approximately 300 kPa and a compressive fracture strain of about 99%. The mechanical strength of highly porous hydrogels primarily depends on the robustness of their non-porous structural network (i.e., the pore walls). This characteristic offers an effective strategy for designing porous hydrogels with enhanced mechanical strength. A novel crystalline-constrained multi-solvent template method has been developed for preparing highly porous PVA hydrogels (P-exogels) with outstanding mechanical properties (Fig. 9B).154 This crystalline-constrained strategy simultaneously involves organic solvent exchange, solid-phase template removal, and pore wall network crystal enhancement. The prepared P-exogel features a hierarchical, interconnected pore structure with a high porosity of 81.69%. It demonstrates impressive mechanical properties, including a tensile breaking strength of 2.47 ± 0.53 MPa, elongation exceeding 400%, and an exceptional toughness of 5.61 ± 1.65 MJ m−3.
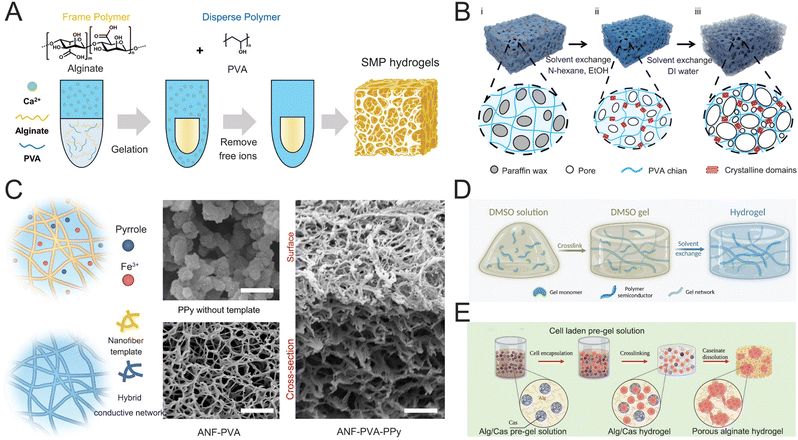 |
| Fig. 9 (A) One-step preparation of SMP hydrogels using PVA as the disperse polymer and Alg as the frame polymer. Reproduced with permission from ref. 153. Copyright 2024, Wiley Online Library. (B) Preparation process and network formation mechanism of Pexogel. Reproduced with permission from ref. 154. Copyright 2024, Wiley Online Library. (C) Schematics of the synthesis of a percolated PPy network guided by the nanofiber template. Reproduced with permission from ref. 155. Copyright 2023, Nature. (D) Preparation method of the hydro-SC, which decouples the gelation step and water swelling step through the solvent exchange. Reproduced with permission from ref. 156. Copyright 2024, AAAS. (E) Schematic showing the fabrication process of porous cell-laden Alg hydrogels based on Alg/Cas emulsions. Reproduced with permission from ref. 157. Copyright 2023, Wiley Online Library. | |
Meanwhile, the porosity also shows a significant influence on hydrogel conductivity.158,159 Highly porous structures can dilute the distribution of conductive fillers disturbing the percolation routes required for efficient electron transport and thereby reducing bulk electrical conductivity. A 3D hyperconnective aramid/PVA nanofiber network was used as a template to guide the assembly of PPy, achieving an ultralow electrical percolation threshold (approximately 1 wt%).155 The resulting hydrogels (CNHs) combine high mechanical strength with excellent electronic conductivity (Fig. 9C). Semiconductor hydrogels are widely regarded as some of the most promising materials with a broader range of electronic and optoelectronic functionalities, which are comprised of percolated 3D networks enabling efficient charge transport. A novel processing approach was proposed to prepare semiconductor hydrogels through a solvent-induced phase transformation method (Fig. 9D).156 Conventional polythiophene derivatives were processed into semiconductor hydrogels featuring a well-percolated network of polymer semiconductors integrated into a porous hydrogel matrix. Thus, the semiconductor hydrogels demonstrated tissue-like softness with moduli as low as 81 kPa, a stretchability of up to 150% strain, and charge-carrier mobility reaching 1.4 cm2 V−1 s−1.
Cell migration plays a vital role in tissue engineering, significantly relying on extracellular matrix (ECM) microstructures or microcracks.160–162 Hydrogels are a class of water-swollen 3D polymer networks with tunable physicochemical properties, capable of closely mimicking the ECM.163,164 The most critical characteristics of 3D hydrogels for cell migration are pore size and pore connectivity, as these factors can either physically hinder or facilitate cell migration. Larger pores in hydrogels allow the nucleus to freely migrate, while smaller pores can press the nuclear envelope and extrude chromatin into the cytoplasm.165 In a study, a simple method was developed to create highly porous, cell–laden Alg hydrogels for improving cell viability (Fig. 9E).157 The hydrogels were prepared by cross-linking immiscible Alg and caseinate (Cas) with Ca2+. Its porous structure was achieved by removing the Cas phase from the ionically cross-linked Alg hydrogels. The porous Alg hydrogels feature heterogeneous pore sizes of approximately 100 μm and interconnected pathways. Cells encapsulated within these hydrogels can self-assemble into spheroids, which improved viability, proliferation, and adipogenic differentiation compared with those in non-porous constructs.
Future research ought to focus on the combination of multiscale porosity to enhance the functionality of hydrogels. Stimuli-responsive hydrogels can vary in their porosity or stiffness in response to environmental cues such as pH, temperature, and electrical fields, which may offer adaptable solutions for tissue engineering applications. Long-term in vivo applications also required to assess these hydrogels' capacity to sustain conductivity and biocompatibility over time.
2.6 Biocompatibility
Hydrogels can be designed to mimic the ECM of the native tissue, which can effectively mitigate inflammation, suppress immunological responses, and alleviate the risk of tissue rejection. Recently, various hydrogels were created by incorporating biomolecular structures to achieve remarkable biocompatibility and other desirable functions. For example, peptide-based hydrogels have been demonstrated to possess inherent biocompatibility and exhibit notable antimicrobial properties. Inflammation and hyperpigmentation are effectively alleviated upon the implantation of the peptide-based hydrogels in the body (Fig. 10A).166 In addition, nucleobase, a fundamental constituent of genetic material, was incorporated into conductive PAAm–PEDOT hydrogels to enhance biocompatibility and conductivity, as well as reduce inflammatory reactions (Fig. 10B).78 An effective method was established by coating conductive hydrogels on traditional rigid electrodes to promote the biocompatibility of traditional electrodes.167 The streamlined fabrication technique involves the chemical modification of metallic surfaces using poly(styrene sulfonate-co-4-vinyl pyridine) (poly(SS–4VP)), followed by in situ electrochemical formation of conductive PEDOT hydrogels. The PEDOT:poly(SS–4VP) interpenetrating networks exhibited similar physicochemical properties to biological tissues (Fig. 10C). The survivability of neuronal cells was conducted by growing primary cells from the mouse hippocampus on a PEDOT:poly(SS–4VP)-coated substrate. The hydrogel coating significantly promoted neuronal development and attachment, which generated a significantly greater density and more organized growth of neurites.
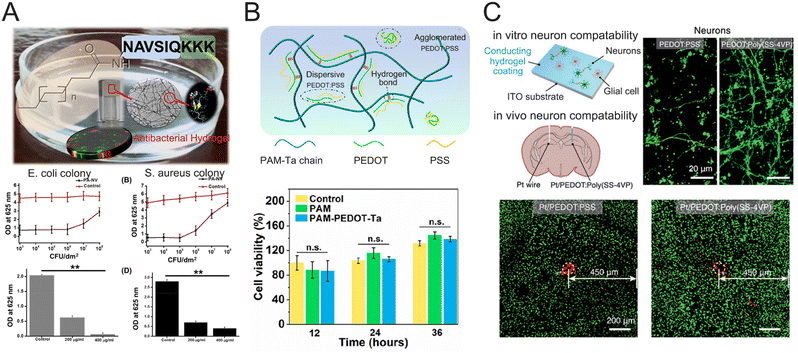 |
| Fig. 10 Various hydrogels exhibit remarkable biocompatibility. (A) Peptide-based antibacterial hydrogel. Reproduced with permission from ref. 166. Copyright 2019, ACS Publications. (B) Nucleobase-schematic diagram of hydrogel composition and proliferation of cells evaluated by the CCK-8 method. Reproduced with permission from ref. 78. Copyright 2023, ACS Publications. (C) In vitro and In vivo biocompatibility of the neural electrodes with conducting hydrogel layers. Reproduced with permission from ref. 167. Copyright 2023, Wiley Online Library. | |
Recent research has shown that hydrogels can efficiently support cell growth and proliferation with low cytotoxicity. Hydrogels have favorable interactions with biological tissues, making them intriguing candidates for enhanced neuroelectronic interfaces and other biomedical applications. The biocompatibility of hydrogels is closely associated with their chemical structures and components. Cross-linking agents and surface chemistry, in particular, possibly cause toxicity or inflammation. To solve this issue, physical cross-linking methods like freeze-drying and thermal gelation can be utilized, allowing for hydrogel production through supramolecular interactions, hence significantly reducing toxicity. Natural biomacromolecules such as Alg are another option to generate biocompatible materials conducive to long-term implantation applications. In addition, the immobilization of anti-inflammatory components on hydrogel surfaces may aid in reducing immune responses.
Although various desirable properties of hydrogels can be achieved through different synthetic strategies, hydrogel-based BCIs require a comprehensive understanding of the interplay between mutually restrictive factors. It is a significant challenge to balance the conductivity, modulus, transparency, and adhesiveness of hydrogels for BCI applications. In future research, the combination of modern synthetic strategies and emerging fabrication techniques is essential to meet the special requirements of BCI applications. The design of supramolecular hydrogels such as topological hydrogels provides a feasible method to balance the tradeoff between modulus and conductivity. Mechanically interlocked hydrogels contain massive mobile junctions to yield large conformational freedom. The dynamic junctions can break molecular chain accumulation and induce molecular chain reorientation of conducting polymers, which can increase the conductivity and meanwhile maintain a low elastic modulus. High transparency of hydrogel electrodes is desirable for optogenetic modulation, which is often influenced by the high content of conductive fillers. Emerging nanotechnologies are required to balance the conductivity and transparency of implantable hydrogel electrodes. For example, conducting polymers can be incorporated into microgels with diameters of <200 nm. The soft conductive microgels can be used as crosslinkers and conductive fillers in hydrogels. The small sizes allow microgels with minimal radiation interaction to generate excellent transparency and maintain a high conductivity. Conductive hydrogels with high volumetric capacitance should be developed to mitigate the impedance at the electrode–tissue interfaces. Emerging fabrication techniques, such as inkjet printing, stereolithography, and direct ink writing, allow the creation of intricate hydrogel architectures to increase biocompatibility and reduce foreign rejection reactions.
3. Brain neural signal recording
The networks of our nervous systems generate and transmit neural signals that regulate emotions, movements, perception, and learning. When the membrane potential of neurons is sufficiently stimulated, it results in a temporary and distinct pulse of membrane potential pulse, known as an action potential (AP), which serves as a fundamental component of bioelectronic activity at the nanoscale.168,169 APs are produced by specific types of voltage-gated ion channels embedded in the plasma membrane. In the resting state, the transmembrane potential of a neuron remains negative (∼−60 mV to −75 mV) due to an ion imbalance, with excess intracellular K+ and extracellular Na+. When voltage-gated Na+ channels open, Na+ rapidly enters the neuron from the extracellular environment, causing the membrane potential to rise to a threshold voltage (∼−50 mV to −55 mV). This triggers the opening of more channels, generating rapid local ionic currents and potentials. At cellular scales, extracellular potentials decrease quickly with distance from the source (e.g., neuron soma or axon). The superposition of simultaneous neuronal activities results in tissue-scale oscillations and rhythms of electrical potentials, referred to as LFPs.170
Implantable electrodes have been employed to create electrical connections between brain tissues and external electronics, enabling the transmission of signals and information between cells and electronics.171,172 A variety of ionic and electronic interactions across a wide range of length scales are involved in bioelectronic activities at the tissue–electrode interfaces. Extracellular potentials result from the sum of transmembrane currents across neuronal surfaces. Single-neuron spikes originate from individual neurons, which produce APs at specific time intervals. LFPs, characterized by continuous signals, are generated by neurons with long, directed dendritic arbors. Temporally synchronized synaptic inputs at restricted dendritic locations can significantly contribute to LFPs. Typically, circuit models are used to quantitatively explain complex bioelectronic processes (eqn (1)). In its simple form, the electrolyte–electrode interface is modeled as a parallel circuit comprising the electrical double-layer capacitance (Ce) and the leakage resistance (Re).168 The interconnection resistance (Ric) represents the resistance of higher-order electrical circuits connected to the electrode. Ve denotes the LFP, while the distance between the target cells and the electrode surface is expressed as r. Additionally, σ represents the conductivity of the extracellular media, and s refers to the complex frequency of APs. The equation governing the recording of electrical potential (Vrec) and ionic current (IAP) is expressed as:
| 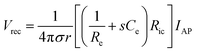 | (1) |
From
eqn (1), the amplitude of
Vrec is directly proportional to
Ce and
Ric and inversely proportional to
Re,
r, and
σ. Thus, for high-efficiency electrodes, it should be essential to have characteristics such as low
Re, high
Ce, high
Ric, and low
r.
172 Considering the complexity and variability of tissue–electrode interfaces, a strategically guided approach is crucial for the successful development of next-generation bioelectronic materials and devices (
Fig. 11). In this section, we will apply these design principles to explore the unique advantages of hydrogels in recording brain neural signals and review their recent advancements. Specifically, we provide an in-depth discussion on implantable hydrogels, focusing on their role in detecting brain signals such as single-neuron spikes and LFPs.
 |
| Fig. 11 Schematic overview of brain neural signal recording. | |
3.1 Single-neuron spike recording
Understanding the brain's inner workings depends on observing the spatiotemporal actions of individual neurons, which serve as the nervous system's structural and functional units. Various types of microelectrodes such as patch clamps, complementary metal-oxide–semiconductor (CMOS) nanoelectrode arrays, microelectromechanical (MEMS) probes, and nanowire-based field effect transistors, are frequently used to record the electrophysiological activities of biological neurons both intracellularly and intercellularly.173,174 The brain is a dynamic system with neural activity and connectivity fluctuating across different time scales, from the millisecond duration of individual APs to months or even years for circuit-level changes that occur during learning and long-term memory formation. The limited interface contact affects signal conduction. By reducing the distance between tissues and sensory electrodes, highly stretchy hydrogels can form biocompatible hydrogel-neural tissue interfaces that enhance signal transduction. In one study, a hybrid multifunctional electrode was fabricated by embedding microscale fibers into a soft hydrogel matrix made of PAAm–Alg for long-term recording of neuronal activity in the mouse brain (Fig. 12A).175 The mechanical and chemical properties of PAAm–Alg minimized damage to surrounding tissue while offering capabilities useful for neuroscience research. These electrodes were able to record individual neuron APs for up to 6 months (168 days) post-implantation. AP waveforms from two distinct neurons were captured at different time intervals (3 days, 1 week, 2 weeks, and every month between 1 and 6 months). The separation and isolation distance of the primary component clusters remain consistent throughout these recordings. The clusters representing the separate units largely overlapped, suggesting that the waveform shapes and amplitudes were stable. These various units exhibited their typical and statistically distinct interspike intervals. Axons, composed of neurofilament proteins and α-internexin are enclosed in a liquid-rich axoplasm, providing remarkable flexibility. Inspired by the axonal unique structure, a soft-fiber neural electrode (FND) was developed using PEDOT:PSS networks as conductive blocks and a glycerol–water mixture as the liquid matrix (Fig. 12B).176 This approach gave the fiber neural device a low modulus (300 kPa), low specific impedance (579 kΩ μm2), and high electrical conductivity (32
700 S m−1). The electrode successfully tracked single-unit spikes from individual neurons in the brain of a running cat. PEDOT:PSS hydrogels can also be crafted into biocompatible digital micropatterns via laser-induced phase separation (Fig. 12C).177 The laser scanning process significantly improved the conductivity and aqueous stability of PEDOT:PSS. The resulting PEDOT:PSS hydrogels demonstrated an electrical conductivity of 670 S cm−1 with 6-μm resolution in water, maintaining excellent conductivity for 6 months without notable degradation under physiological conditions. This hydrogel microelectrode array successfully recorded extracellular APs from mouse brain slices, showing stable, consecutive AP spikes. In another study, the laser-induced process was used to create a PEDOT:PSS hydrogel microelectrode array on PET substrates (Fig. 12D).178 The array was carefully inserted into the somatosensory cortex (S1) of rats, which is capable of recording extracellular APs. The microelectrode array recorded APs in rats at rest, anesthesia, and mechanical stimulation, demonstrating long-term stable signal acquisition for up to 3 weeks.
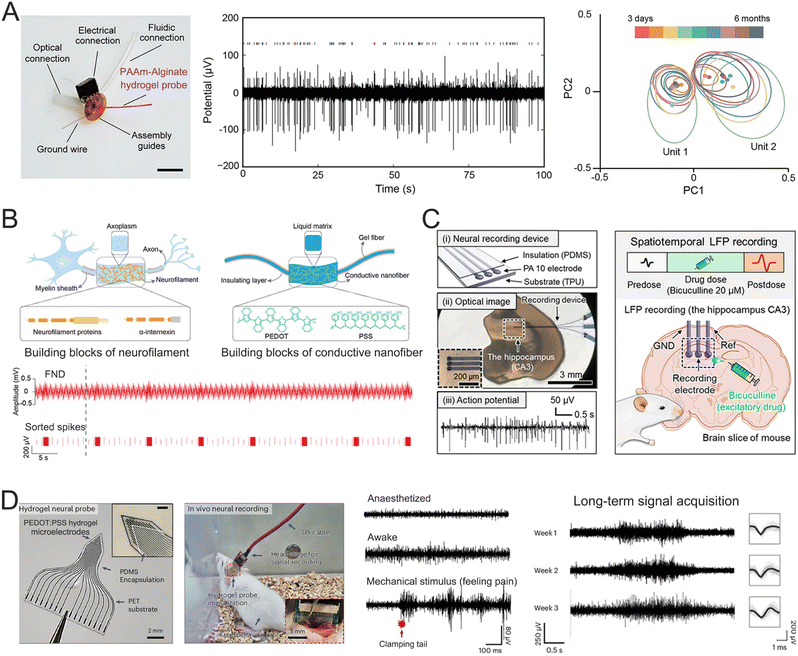 |
| Fig. 12 (A) The composition of the hydrogel neural probe (left), the recording of representative spontaneous electrophysiological activity (middle), and principle component analysis of the single-unit potentials (spikes) recorded 3 days, 1 week, 2 weeks, and 1–6 months following implantation (right). Closed circles indicate centers and ellipses represent two standard deviation (2σ) contours of the principle component distributions. Reproduced with permission from ref. 175. Copyright 2021, Nature. (B) Schematic of network-in-liquid structure in neuron axon and FND (top) and band-pass filtered waveforms and sorted spikes of input and recording signals when gels containing FND changed from static to shaking states (bottom). Reproduced with permission from ref. 176. Copyright 2024, Wiley Online Library. (C) The APs (left) and in situ LFPs (right) recording of the mouse. Reproduced with permission from ref. 177. Copyright 2022 AAAS. (D) The set-up for the neural recording of the free-behaving rat (left) and APs of the rat's brain under various physiological conditions (right). Reproduced with permission from ref. 178. Copyright 2024, Nature. | |
3.2 The local field potential recording
LFPs provide a broad overview of the collective neural activity within a specific brain region. In contrast to APs, which represent the rapid firing of individual neurons, LFPs arise from the combined excitatory and inhibitory synaptic inputs of a neuronal population. Neurons in the brain are interconnected by synapses, and when activated, they generate APs—rapid and short-lived electrical fluctuations across the neuron's membrane. As the AP reaches the presynaptic terminal, neurotransmitters are released into the synaptic clefts. These neurotransmitters bind to receptors on the postsynaptic membrane, initiating changes in ion channel conductance and electrical conductivity. The simultaneous neurotransmitter release from multiple neurons leads to the summation of electrical signals, culminating in the formation of LFPs. LFPs provide important insights into the synchronized activity of neural circuits and the brain's functional state. By reflecting the collective dynamics of neuronal populations, LFPs can reveal levels of neural excitability, coordination, and connectivity in specific brain areas. For instance, distinct oscillatory patterns in LFPs, such as alpha waves (8–12 Hz), beta waves (13–30 Hz), and gamma waves (30–100 Hz), are linked to critical brain functions like motor control, sensory processing, and cognitive tasks such as attention and memory.179,180 These oscillations not only signify brain activity but also contribute to communication between brain regions, supporting complex behaviors and cognitive processes.181–184 In BCIs, LFPs are used to decode neural signals associated with motor intentions or other brain functions, enabling users to control external devices, such as robotic arms or computers, through their thoughts. This technology offers great promise for individuals with motor impairments, providing them with new ways to interact with their environment.
Hydrogel neural electrodes have gained recognition as suitable candidates for monitoring LFPs due to their unique physicochemical properties. In one study, a PEG-ACSF hydrogel neural interface was developed to mimic the natural neuronal environment, enabling the successful recording of high-quality LFPs from freely moving mice while significantly reducing immunochemical rejection (Fig. 13A).185 This electrode was employed to investigate LFPs in mice during both awake and sleep states. During sleep, the LFPs exhibited lower-frequency, higher-amplitude waveforms, which were attributed to the synchronous activation of large numbers of neurons. The PEG-ACSF hydrogel allowed continuous high-quality LFP recordings for up to two weeks. Another approach involved using soft microgels as large crosslinking centers within hydrogel networks to simulate the physicochemical properties of brain tissues.115 These microgel centers act as deformation and mechanical buffers, resulting in a low modulus (59 kPa) while maintaining a high strength (1.01 MPa). The close modulus match reduces micromotion between the electrode and brain tissue, enhances signal transmission across the biohybrid interface, and minimizes the FBR. Using this hydrogel electrode implanted in the hippocampus CA1 region of rats, LFP signals were successfully recorded for 4 weeks (Fig. 13B). By the third week, the hydrogel electrode demonstrated stronger LFP signals than Pt electrodes due to its reduced FBR. In another example, a 3D-printed neural electrode was constructed using conducting PEDOT:PSS hydrogels for neural signal recording. The device comprised nine PEDOT:PSS electrodes, each with a diameter of 30 μm (Fig. 13C).186 The PEDOT:PSS hydrogel electrodes displayed an impedance range of 50–150 kΩ at 1 kHz, making them suitable for in vivo neural activity recording. When implanted into the mouse dorsal hippocampus, the soft neural electrode successfully recorded continuous neural activities over two weeks, including LFP signals at 1 kHz and APs at 40 kHz.
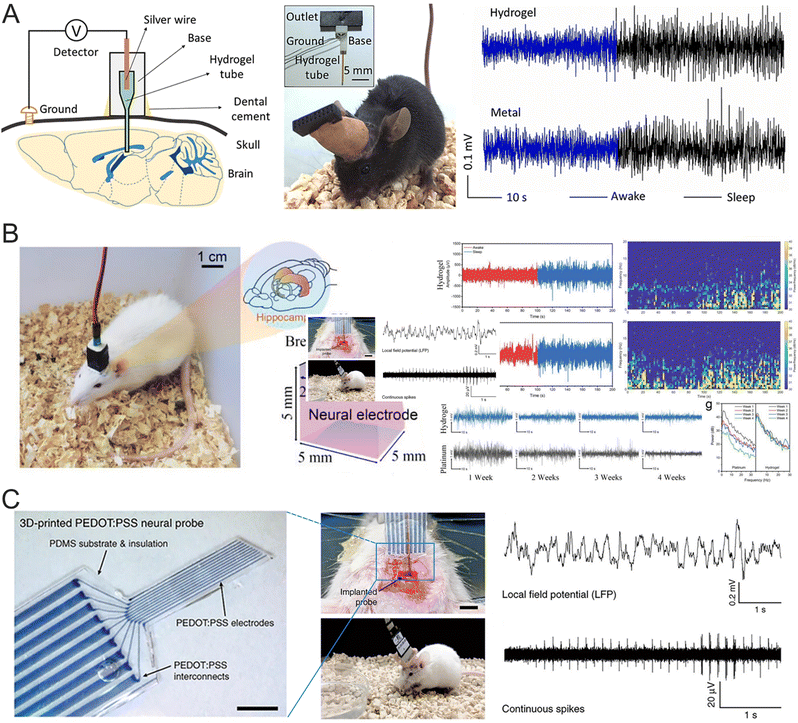 |
| Fig. 13 (A) A schematic of the experimental setup to detect LFPs of mouse in vivo (left), photos of a cap and an implanted mouse (middle), and LFPs in vivo detected using a hydrogel and metal (right). Reproduced with permission from ref. 185. Copyright 2019, Elsevier. (B) The indication of the implant position of the hydrogel electrode (left) and the LFP recording by hydrogel and Pt electrodes (right). Reproduced with permission from ref. 115. Copyright 2022, Wiley Online Library. (C) Images of the implanted 3D-printed soft neural probe (left) and representative electrophysiological recordings (right) by the 3D-printed soft neural probe. Reproduced with permission from ref. 186. Copyright 2020, Nature. | |
Electrocorticography (ECoG) is frequently employed in clinical neurology for purposes such as detecting and controlling epileptic seizures, as well as long-term neural monitoring. ECoG signals can be captured by placing electrodes on the surface of the cerebral cortex. This semi-invasive method reduces surgical risks and recovery time while providing relatively high signal quality. In one study, a conductive β-peptide-based hydrogel (betaVhex/CNTs) was developed to create a soft, biocompatible neural interface that integrates fully with neural tissues (Fig. 14A).63 The controlled interaction of non-biodegradable β-peptides with CNTs resulted in the formation of a 3D conducting network that facilitates signal transmission. The betaVhex/CNT electrode, placed on the cortical surface, recorded ECoG during seizure episodes, showing a clear enhancement of high-frequency signal components (β and γ). Subsequently, an implantable electrode was created by injecting the betaVhex/CNTs into a glass pipet, which was then implanted into the S1 of chronic epileptic mice to record LFP signals from deep cortical tissue. The betaVhex/CNT electrode successfully recorded spontaneous seizures and consistently captured neural signals across all frequency ranges, with higher signal augmentation observed in LFP recordings compared to ECoG. In another research, a flexible neural biointerface was fabricated using silver nanocubes (AgNCs) embedded in PAAm composite hydrogels. This hydrogel was encapsulated in a biocompatible structure composed of PDMS and soft skin adhesive, the latter providing stable adhesion to the skull, ensuring consistent contact while recording neural cortex signals.66 The upper PDMS layer prevented the neural acquisition system from sticking to the skin of the mouse's head post-surgery. These layers worked together to ensure reliable signal acquisition from targeted areas of the brain cortex. The composite hydrogel demonstrated low electrical impedance (<3 kΩ at 10 Hz) and Young's modulus (<10 kPa), which align with the flexibility and softness of neural tissues. The PAAm/AgNC-based neural biointerface promptly captured ECoG signals, as shown in Fig. 14B, with consistent amplitude across 6 weeks of chronic monitoring. Additionally, another bioadhesive ultrasoft BCI was developed using PDA–PAAm hydrogels, which incorporated dopamine-hybridized PEDOT nanoparticles and carrageenan-interpenetrated PDA–PAAm networks.187 The hydrogel's modulus aligns with that of brain tissue, significantly reducing the mechanical mismatch between the hydrogel and the brain and helping to prevent fibrous tissue encapsulation and neuroinflammation. The ECoG signals recorded by the PDA–PAAm probe were further evaluated using the Pearson correlation coefficient (r), as shown in Fig. 14C. The electrode achieved a remarkable correlation coefficient of 0.9, demonstrating enhanced performance compared to commercial screw electrodes. This enhanced performance is partially due to the conformal interfacial contact, which minimizes environmental noise.
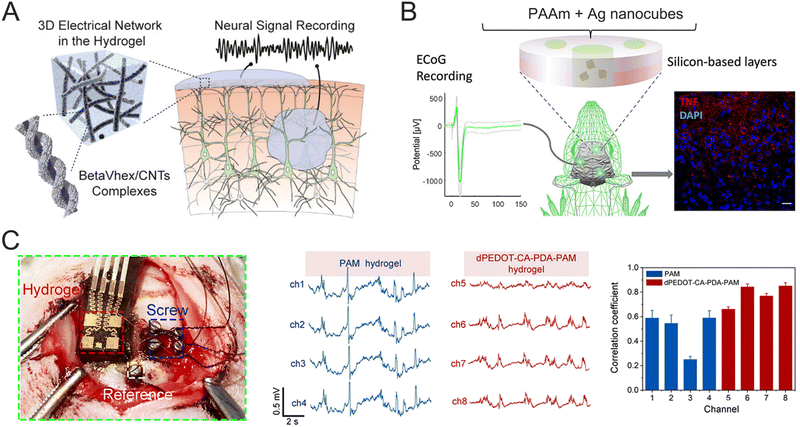 |
| Fig. 14 (A) Schematic illustration of a supramolecular peptide-based hydrogel and its applications in neural signal recording. Reproduced with permission from ref. 63. Copyright 2020, ACS Publications. (B) Schematic illustration of nanostructured hydrogels for ECoG recording. Reproduced with permission from ref. 66. Copyright 2022, ACS Publications. (C) The location of a bioadhesive ultrasoft neural electrode and commercial screw electrode (left), and the representative ECoG signals recorded by the PAM and dPEDOT-CA-PDA-PAM hydrogel-integrated BCI (right). Reproduced with permission from ref. 187. Copyright 2022, Elsevier. | |
Although hydrogel neural electrodes offer unique properties to record brain signals, they still suffer from some critical technical challenges such as swelling and micromotion between neural electrodes and brain tissues. Hydrogels consist of hydrophilic networks with high water contents, which typically experience significant volumetric expansions in body fluids. The volumetric expansions often lead to conductivity decreases and neural signal attenuation. Meanwhile, the swelling hydrogels often oppressed brain tissues causing undesirable FBRs. The swelling feature of hydrogels can be restrained using hydrophobic association, high density of crosslinking sites, and crystallization aggregation with the compromise of modulus match. Therefore, it is incredibly important to balance the anti-swelling property and modulus match. Micromotions between neural electrodes and brain tissues often occur during long-term applications due to weak interfacial interaction and modulus mismatch. Micromotions can damage surrounding neural tissues and lead to chronic inflammatory reactions. These micromotions can be alleviated by creating supramolecular interactions on the interfaces between neural electrodes and brain tissues.
4. Brain neuromodulation
Neuromodulation is an emerging technology used to treat neurological and neuropsychiatric disorders by influencing the nervous system through various external stimuli, such as optical, electrical, and acoustic inputs.188 The nervous system regulates bodily functions through complex patterns of APs in neural networks, involving communication among interconnected cells, axons, dendrites, and synapses. Neuromodulation can modify the membrane potential of neurons, thereby controlling their functional state and the release of neurotransmitters. This process can lead to long-term activation, inhibition, or regulation of neural network behaviors.189–192 By selectively targeting dysfunctional neural circuits with precise stimuli, neuromodulation can adjust their activity to improve disease outcomes, making it a powerful tool for restoring or enhancing motor, sensory, and cognitive functions.193–195 One of the major challenges in neuromodulation is delivering stimuli with high spatial and temporal precision while minimizing damage to surrounding tissues. Additionally, combining neural recording capabilities with neuromodulation techniques is essential for real-time brain activity monitoring, ensuring safe and effective modulation.18,196,197 Ongoing material innovations are aimed at ensuring the effectiveness, safety, and long-term stability of the neuromodulation process.198,199 Hydrogels have emerged as highly suitable materials for neuromodulation due to their similar physico-chemical properties, which closely resemble brain tissues.200–202 Hydrogels can also be engineered to deliver a range of neuromodulatory agents, such as optical signals, electrical impulses, and ultrasound, making them a versatile platform for integrating with neuromodulation techniques (Fig. 15). While hydrogels offer considerable advantages in this field, research is still in its early stages, and only a limited number of examples have been reported in recent years. Most instances of hydrogel-based neuromodulation have been linked to optogenetic modulation, with fewer cases of hydrogel-based electric stimulation and ultrasound neuromodulation being documented. This section summarizes recent advances in this field, aiming to draw more attention and inspire further interest, which could accelerate breakthroughs and help bridge the gap between hydrogel-based materials and neuromodulation techniques.
 |
| Fig. 15 Schematic illustration of neuromodulation. | |
4.1. Optogenetic modulation
Optogenetic modulation utilizes external optical signals to manipulate neural circuits in the brain, triggering specific physiological responses. When exposed to light of a particular wavelength, photosensitive proteins (voltage–gated ion channels) located on neuron membranes are activated, allowing ions to flow through the membrane. This ion flow results in APs that can either activate or inhibit particular neurons and neural circuits.203–205 Optogenetic modulation can target specific neuron types and circuits with exceptional temporal and spatial precision.206–208 Various photosensitive proteins have been explored for optogenetic modulation, including channel channelrhodopsin-2 (ChR2), inhibitory ancient rhodopsin (Arch), and inhibitory halorhodopsin (NpHR), which are activated by light at wavelengths of 470 nm, 570 nm, and 580 nm, respectively.203 By manipulating these proteins, researchers can investigate the biological mechanisms and functions underlying neurological disorders at the cellular, circuit, and organ levels. In addition to biocompatibility and conductivity, electrode materials used for optogenetic modulation require excellent light transmittance. The scattering and absorption of light by electrode materials reduce the efficiency of the optogenetic stimulation and generate heat (photo-thermal effect), potentially damaging brain tissue. Hydrogel optrodes have garnered significant attention recently due to their excellent transparency and biocompatibility, enabling safe and effective optogenetic modulation.
A sponge-like hydrogel scaffold composed of PAA, PVA, and PEG was developed to support neuron growth at an organism level, stimulating the structure and function of brain tissue.209 Glutamatergic hippocampal cells were cultured within this hydrogel scaffold for in vitro optogenetic modulation (Fig. 16A). A custom optrode array was integrated into the hydrogel scaffold for optogenetic modulation. When blue light pulses (473 nm, 20 Hz, duration 5 ms) were applied to the glutamatergic hippocampal cells, continuous and frequency-modulated APs were generated, indicating successful neuron activation. In another case, an implantable neural electrode was fabricated to enable spatiotemporal gene delivery, optogenetic modulation, and simultaneous monitoring of neural signals.210 This neural electrode was functionalized with a biotic–abiotic neural interface (PG) composed of amphiphilic chitosan, RGO, and PEDOT. The hydrogels self-assembled into a nanogel-based structure, mimicking tissue environments to promote cell interaction and neural signal transduction. Additionally, nonviral gene vectors were encapsulated within the hydrogel matrix for gene transfection. PG's high charge storage capacity enabled rapid gene vector release with minimal current (125 μA), and the gene vector was successfully transfected into localized rat adrenal pheochromocytoma cells, producing a strong expression of green fluorescent protein. Photoevoked extracellular spikes were observed by applying a 473 nm laser in the thalamic ventrobasal region (Fig. 16B). The increased spike counts through repeated optogenetic modulation demonstrated its potential capability for treating neural disorders. Transparency is a crucial property for implantable probes used in optogenetic modulation. An Alg–PAAm composite hydrogel was designed with low light-propagation loss, low Young's modulus, and high tensile strength.211 A hydrogel-optrode array was prepared to deliver blue light pulses to the hippocampus region, allowing for the observation of frequency-dependent neuronal responses and the modulation of animal behavior, such as right-turning and rotating movements. Hydrogel optrodes significantly reduce tissue reactivity compared to silica optrodes. In an optogenetic study, an adeno-associated virus (AAV) carrying the CaMKIIα-ChR2-mCherry gene was injected into the hippocampus region of mice. Unprocessed spike data recorded using the hydrogel array showed substantial electrophysiological responses from stimulated neurons during light pulse sequences (Fig. 16C). The analysis of these data, including 3D unit cluster images and averaged spike waveforms, demonstrated that the hydrogel optical fibers were excellent waveguides for in vivo optogenetic stimulation.
 |
| Fig. 16 (A) Schematic illustration of the culture of glutamatergic hippocampal cells (top) and signals changes of hChR2-expressing hippocampal neurons before and during light stimulation (bottom). Reproduced with permission from ref. 209. Copyright 2023, Elsevier. (B) Setup for the in vivo optogenetic experiment (top) and the optoelectronic probe provided a recording of neuronal activity during optogenetic stimulation (bottom). Reproduced with permission from ref. 210. Copyright 2019, ACS Publications. (C) Optogenetic modulations in vivo. Reproduced with permission from ref. 211. Copyright 2018, Wiley Online Library. (D) Optogenetic stimulation for brain–interface communications. Reproduced with permission from ref. 212. Copyright 2021, ACS Publications. (E) Hydrogel as both a neuroelectronic and a neuro-optic interface in an optogenetic experiment. Reproduced with permission from ref. 185. Copyright 2019, Elsevier. | |
Additionally, a core-sheath structure was developed to improve the optical propagation properties of hydrogel fibers (Fig. 16D).212 A core-sheath hydrogel optical fiber was created using a wet spinning process, comprising a poly(PEGDA-co-AAm) core (339 ± 26 μm) and a Ca–Alg sheath (776 ± 44 μm). These hydrogel optical fibers displayed an optical attenuation of 0.18 ± 0.01 dB cm−1 under 650 nm laser illumination, one of the lowest reported data for hydrogel materials, and possessed high biocompatibility and a tissue-like Young's modulus. The hydrogel fibers were implanted into the primary visual cortex (V1) of mice expressing ChR2 in parvalbumin-positive inhibitory neurons. Blue light pulses successfully passed through the hydrogel fiber, suppressing pyramidal neuron activity. The 3D visualization of the united clusters revealed spiking activity from two distinct neurons. PEG hydrogels have been reported to function as optical fibers and ionic conductors with minimal immunological rejection.185 These hydrogels can capture LFPs and individual spikes in vivo. Implanted in the laterodorsal tegmentum region of mice to control fear, GABAergic neurons were activated using 470 nm laser pulses (5 mW, 20 ms), generating clear neural signals. The hydrogel fibers modulate the neural circuit in the lateral hypothalamus to regulate sleep. Fig. 16E shows the activation of GABAergic cells during the mouse's sleep state, with the mouse waking rapidly after light stimulation. The power density further confirmed the sleep-wake transition.
In situ neural signal monitoring and neuromodulation play a significant role in investigating the function mechanism of neural circuits and treating neurological disorders. However, in situ neural signal monitoring and neuromodulation were restricted by the tradeoff between the conductivity and transparency of implantable electrodes. In general, neural signal monitoring and neuromodulation were conducted using separated electrodes and optrodes with a distance of a millimeter scale. The first in situ neural signal monitoring and neuromodulation were realized by Zhang and coworkers who addressed the tradeoff between the conductivity and transparency of hydrogels by tuning conductive components with minimal radiation interactions and designing dynamic topological structures.39,43 For instance, conductive PPy has been incorporated into microgels with a diameter of 200 nm, which act as large crosslinking centers to form hydrogel networks through polymer chain entanglements and covalent bonds.39 Since the microgels are much smaller than the wavelength of visible light, it minimizes the interaction between optical waves and conductive components. This unique structure provides the hydrogel with excellent optical transparency and electron conductivity. Additionally, the microgel crosslinking sites serve as mechanical buffers, allowing the hydrogel to have an elastic modulus similar to brain neural tissues, resulting in negligible FBR. This hydrogel electrode was successfully used for in situ optogenetic neuromodulation and simultaneously neural signal monitoring over 8 weeks (Fig. 17A). Throughout this time, the LFP signals exhibited minimal degradation, confirming the hydrogel electrode's potential for long-term optogenetic applications. Notably, the electrode was used to modulate the neural circuit in the right primary motor cortex (M1), which controlled rat behaviors such as lifting the left forepaw and rotating counterclockwise. The relationship between these behaviors and neural signals was established through optogenetic neuromodulation. Similar research has been conducted using polyrotaxane-based topological hydrogels.43 In this case, polyrotaxane, composed of a PEG backbone and 15 cyclodextrins (CDs), allows the CD rings to slide along the PEG chains like pulleys. This sliding effect provides the hydrogel with low elastic modulus, high conductivity, and excellent optical transparency. Using this topological hydrogel probe, continuous optogenetic neuromodulation at 473 nm was achieved in the M1 for four weeks. Moreover, the amplitude and frequency of the induced LFP signals could be adjusted by controlling the power and frequency of the applied laser (Fig. 17B). Previous studies have used sophisticated molecular structure designs to achieve low elastic modulus, high conductivity, and optical transparency for in situ neuromodulation and neural signal recording. A universal method has also been developed that incorporates electrical, optical, and microfluidic fibers into PU-PEG hydrogels.213 This neural device was chronically implanted in the nucleus accumbens of Thy1-ChR2 mice, enabling optical stimulation and simultaneous recording of evoked and spontaneous neural activity in the nucleus accumbens region (Fig. 17C). The evoked LFP signals remained stable for 4 weeks. In another study conducted by the same group, microelectrode arrays containing seven tin microwires and optical fibers were integrated within a soft PAAm–Alg hydrogel matrix (Fig. 17D).175 This PAAm–Alg hydrogel mimicked brain tissue, minimizing tissue damage and FBR after implantation. The hybrid device was implanted in the ventral hippocampal projection cortex (vHPC) to record induced LFP signals in freely moving mice in response to optical stimulation (473 nm) for up to six months. Induced LFP signals were first detected after 1–2 weeks, with their amplitudes gradually increasing until the eighth week, after which they remained stable for four months. This device enabled the investigation of electrophysiological, optogenetic, and behavioral responses in freely moving rats for 6 months. Various cross-linking chemistries and micro-nano fabrication techniques have been employed to optimize hydrogel properties for optogenetic neuromodulation. PVA is a well-known semi-crystalline polymer, and its crystalline domain size can be adjusted to control mechanical strength and light transmission. A step-index hydrogel electrode was fabricated, consisting of a PVA hydrogel optical core and a CNTs–PVA microelectrode sheath (Fig. 17E).214 These evoked LFP signals remained stable for 10 weeks. Reducing the crystal size to 3.5 nm increased the hydrogels’ refractive indices while maintaining relatively low elastic moduli. The PVA hydrogel optical core exhibited a refractive index of 1.37–1.40 (480 nm), a light transmission of >96%, a stretchability of 139–169%, and an elastic modulus of 2.8–9.3 MPa. The increased refractive index contrast between the PVA hydrogel core (ncore = 1.40) and the CNTs–PVA sheath (ncladding = 1.34) ensured light transmission and, consequently, successful optogenetic neuromodulation. The hydrogel electrode was implanted into the mouse ventral tegmental area, where it optically manipulated neurons while simultaneously recording evoked LFP signals during behavioral assays. The evoked LFP signals remained stable and consistent for a period of 10 weeks.
 |
| Fig. 17 (A) The optogenetically evoked LFPs recorded by hydrogel electrodes for 8 weeks (top) and the optogenetically evoked neuronal spike recording in vivo (bottom). Reproduced with permission from ref. 39. Copyright 2022, Wiley Online Library. (B) Optogenetically evoked LFP signals tuned by light frequencies (1–20 Hz) (top) and the recording of the optogenetically evoked LFPs by a topological hydrogel neural probe for four weeks (bottom). Reproduced with permission from ref. 43. Copyright 2023, Wiley Online Library. (C) Image of optogenetically evoking APs in anesthetized transgenic Thy1-ChR2 mouse (top) and chronic recording of optogenetically evoked APs in a Thy1-ChR2 mouse (bottom). Reproduced with permission from ref. 213. Copyright 2021, ACS Publications. (D) An illustration of optogenetic modulation and electrophysiological recording (left) and representative electrophysiological recordings during optical stimulation using the hydrogel hybrid probes 3 days, 1–2 weeks, and 1–6 months following implantation (right). Reproduced with permission from ref. 175. Copyright 2021, Nature. (E) Schematic illustrations and representative photographs of a core hydrogel fiber (left) and representative in vivo electrical signals recorded with optrodes upon optical stimulation (right). Reproduced with permission from ref. 214. Copyright 2024, Nature. | |
4.2 Electrical stimulation modulation
Electrical stimulation generates an electric potential across the outer membrane of targeted neurons, facilitating processes such as neural differentiation of stem cells, neurite outgrowth, and the reconstruction of neural circuits.215 Electronic stimulation offers diverse applications, ranging from precise neuronal stimulation with implanted electrodes to broader stimulation using large-area epidermal electrodes. The interfaces between brain tissues and electrodes can be represented by equivalent circuit models, as shown in Fig. 18. In contrast to electrical recording, stimulation applications involve electrical signals traveling from electrodes into brain tissues, creating an electric potential (Ve) in the electrolytic medium that affects the outer membrane of the targeted neurons, leading to depolarization of the transmembrane potential. When Ve reaches a sufficient level to depolarize the membrane to the threshold (∼−55 mV), the neuron is triggered to fire APs. A key criterion for effective electronic stimulation is the ability to inject more ionic current at the electrolyte–electrode interface without causing negative outcomes like electrode degradation, water hydrolysis, or tissue damage. Currently, two major mechanisms facilitate ionic current injection at these interfaces: (i) faradaic charge injection, which relies on surface-confined electrochemical reactions (oxidation and reduction), and (ii) capacitive charge injection, which involves the charging and discharging of the electrolyte–electrode interface. The capacitive mechanism is generally favored for electronic stimulation. The governing equation relating Ve and Vsti in bioelectronic stimulation is given by: |  | (2) |
According to this equation, the amplitude of Ve is proportional to Ce and Vsti, while inversely proportional to Re, Ric, and r. Therefore, achieving high efficiency in electronic stimulation requires electrode characteristics, including high values of Ce and Vsti, alongside low values of Re, Ric, and r.
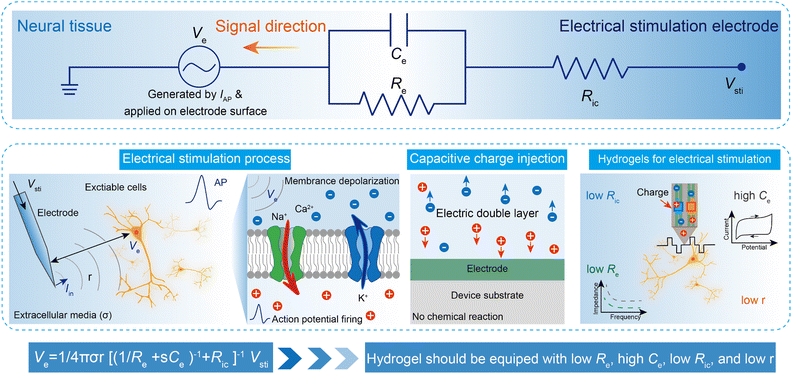 |
| Fig. 18 Schematic overview of electrical stimulation. | |
Electrical stimulation can greatly enhance neural differentiation of stem cells, promote neurite outgrowth, and facilitate neural circuit reconstruction.215 It is one of the primary neuromodulation techniques used to regulate neural activity and provide precise treatments for diseased neural circuits. Recently, conductive hydrogels have been investigated as neural electrodes for electrical stimulation due to their adaptability, biocompatibility, and high charge capacity. For example, a composite hydrogel made of aloe vera gel and PEDOT:PSS was used as a transcranial electrical stimulation electrode (Fig. 19A).216 This composite hydrogel demonstrated a higher charge capacity and lower vivo interfacial impedance than traditional metal-based transcranial electrical stimulation electrodes. These electrodes were able to modulate neuronal activity, induce epileptic activity, and simultaneously monitor neural activity across multiple brain regions. However, current electrical stimulations require wired connections between the electrodes and the stimulator, limiting mobility and increasing the risk of infection, which hinders their widespread clinical use. There is a growing interest in developing wireless electrical stimulation probes to eliminate these wired connections.217 Wireless electrical signals can be generated by a rotating magnetic field through electromagnetic induction. A wireless electrical stimulation electrode was developed using an annular Gel/Pani hydrogel, which served as a secondary coil to achieve wireless electrical stimulation through electromagnetic induction with an external primary coil (Fig. 19B).105 This innovative design capitalized on the unique properties of conductive hydrogels—such as biocompatibility, biodegradability, and conductivity, to ensure safe and effective operation within biological systems. The annular conductive hydrogel electrode promoted the growth and differentiation of NSCs, contributing to the formation of functional neural networks. The hydrogel electrode was implanted beneath the skull around the lateral cortical and striatal infarct regions in stroke-affected rats to facilitate wireless electrical stimulation. This wireless electrical stimulation significantly reduced infarct volume, organized disordered neurons, and improved neurological functions.
 |
| Fig. 19 (A) Hydrogel-based transcranial electrical stimulation electrodes enable focal seizure stimulation in vivo. Reproduced with permission from ref. 216. Copyright 2020, Wiley Online Library. (B) Schematic diagram of hydrogel-mediated wireless electrical stimulation for accelerating the NSCs into functional neurons. Reproduced with permission from ref. 105. Copyright 2024, Wiley Online Library. | |
4.3 Ultrasound modulation
The integration of ultrasound technology with genetic engineering has given rise to the field of sonogenetics, which utilizes genetically encoded ultrasound-sensitive elements alongside proteins.218 Some studies have identified mechanically sensitive ion channels, such as Piezo1,219 the large-conductance mechanosensitive ion channel (MscL),220 the mechanosensory ion channel (MEC-4),221 hsTRPA1,222 TRP-4,223 Nav1.5,224 voltage-gated calcium channels,225 and members of the two-pore potassium channel family226 as crucial mediators of ultrasound-induced neuromodulation. Generally, ultrasound exerts its effects on neurons primarily through two mechanisms: mechanical stress and thermal effect.227 The mechanical effect involves the generation of mechanical forces, which directly influence cell membranes and mechanosensitive ion channels, altering their states of openness. The thermal effect occurs through the energy conversion of ultrasound into heat, leading to a localized temperature increase and activating temperature-sensitive ion channels. However, the thermal effect may yield permanent tissue changes and damage. More recent research has focused on harnessing the mechanical effects of ultrasound modulation. Precise ultrasound neurostimulation was conducted using microbubbles that leveraged significant differences in acoustic impedance between the surrounding medium and gas inside the microbubbles (Fig. 20A).228 Localized mechanical forces were generated by microbubble oscillation driven by acoustic stimulation, activating nearby mechanosensitive ion channels. In addition, the microbubble oscillation can trigger Ca2+ influx through mechanosensitive ion channels to evoke reversible neural calcium responses in the mouse brain (Fig. 20B).229
 |
| Fig. 20 (A) A general scheme of mechanosensitive ion channels. Reproduced with permission from ref. 228. Copyright 2024, Wiley Online Library. (B) Schematic illustration of ultrasonic mechanical force amplified locally by gas vesicles (GVs, depicted in yellow) inducing neuronal activation. Reproduced with permission from ref. 229. Copyright 2024, Elsevier. | |
Moreover, the depth and resolution of ultrasound can be efficiently adjusted to meet the system's requirements across various spatial and temporal scales by modulating its frequency. Low-frequency ultrasounds (<3.5 MHz) can penetrate biological tissues, including bones and brains. Because of their deep penetration and spatiotemporal resolution, ultrasounds can non-invasively reach deep brain regions for neuromodulation. However, the relatively low frequencies of the ultrasound result in spatial precision that ranges from millimeters and centimeters, making it difficult to precisely target specific brain cells. To address this, hydrogels were used as media for sound wave transmission due to matched acoustic impedance, which reduced reflection and attenuation at the tissue–device interface, thereby enhancing the efficiency of ultrasound signal conduction.230 This combination of hydrogels and ultrasound devices provides a more advanced method for modulating brain activity with greater spatial precision than current ultrasound techniques. A recent study introduced a shape-morphing cortex-adhesive (SMCA) hydrogel sensor for closed-loop transcranial focused ultrasound (tFUS) neurostimulation, aimed at treating drug-resistant epilepsy (Fig. 21A).231 This sensor consists of a catechol-conjugated Alg hydrogel adhesive, a stretchable thin (∼3 μm) 16-channel electrode array, and isophorone bisurea-functionalized PDMS, combined with a pulse-controlled tFUS device. The catechol-conjugated Alg hydrogel adheres strongly to the brain's curved surface, ensuring stable contact during stimulation. In addition, a customized head stage system was developed for a closed-loop BCI, integrating a tFUS transducer with the implanted SMCA sensor. The SMCA hydrogel sensor can record high-frequency oscillations (HFOs) (80–500 Hz) generated by epileptogenic tissues. The seizure-preceding HFOs acts as biomarkers, providing neurophysiological feedback to the closed-loop tFUS neurostimulation system. The tFUS stimulation, triggered by these HFOs, can influence the right CA3 region of the hippocampus before the ictal activity begins. Using this technique, the system can accurately predict seizure spikes and deliver a proactive therapeutic intervention. In another research, a bioadhesive ultrasound (BAUS) device was proposed featuring a thin, rigid ultrasound probe securely attached to the skin using a bioadhesive hydrogel-elastomer hybrid (Fig. 21B).232 The hydrogel consisted of chitosan-PAAm interpenetrating polymer networks, which were encased in a thin PU membrane (less than 40 mm in thickness) to prevent hydrogel dehydration. The bioadhesive hydrogel–elastomer hybrid ensured stable positioning even during dynamic body movements and exceptional reliability for long-term applications. The BAUS device efficiently transmitted acoustic waves and shielded the probe from skin deformation.
 |
| Fig. 21 (A) SMCA sensor for ultrastable brain interfacing enables closed-loop tFUS neurotherapy. Reproduced with permission from ref. 231. Copyright 2024, Nature. (B) Design and imaging performances of the BAUS device. Reproduced with permission from ref. 232. Copyright 2022, AAAS. | |
Ultrasonic neuromodulation provides a non-invasive, deep-tissue technique for brain activation. Hydrogels play a crucial role in maintaining microbubble-mediated neurostimulation, improving ultrasonic signal transmission, and ensuring long-term implant integration. However, the limited spatial resolution of ultrasonic neuromodulation remains a significant challenge. To address this, more work should be devoted to reducing acoustic impedance by enhancing the interfacial adhesion of hydrogels or developing soft acoustic metamaterials. Additional challenges include the instability of gas vesicles and microbubbles, variations in ultrasonic wave propagation, and potential thermal effects. To overcome these constraints, innovative approaches should be developed, such as designing hydrogels with tunable acoustic impedance to optimize wave transmission and coating microbubbles with hydrogels to delay premature degradation. Furthermore, temperature-sensitive hydrogels hold promise for dynamically controlling ultrasonic wave intensity to mitigate overheating risks. In the future, piezoelectric nanoparticles and mechano-responsive materials can be incorporated into hybrid hydrogels to improve ultrasound-mediated neuromodulation. In addition, the neuromodulation effect can be enhanced by designing hybrid hydrogels with multifunctional synergistic stimulations.
4.4 Magnetogenetic modulation
Magnetogenetics can use magnetic stimuli to modulate neural circuits via magnetoreceptors in a non-invasive way. The magnetogenetic modulation depends on transducers, known as mediators, to convert magnetic fields into recognizable signals (thermal and mechanical signals) by biological receptors. Based on the transducer types, magnetic modulation techniques can be classified into several categories: magneto-thermal,233 where magnetic fields are converted into heat; magneto-chemical,234 which releases chemical species by magnetic stimulations; magneto-mechanical,235,236 which converts magnetic fields into mechanical force; and magnetoelectric,237 where magnetic stimulations are transduced into electrical potential (Fig. 22).235,238 Magneto-thermal and magneto-chemical transductions utilized the hysteretic losses of magnetic mediators exposed to quickly changing magnetic fields, enabling the activation of heat-sensitive ion channels or the release of chemical species, respectively. Magneto-mechanical transduction activated pressure-sensitive ion channels through the mechanical stress generated by a changing magnetic field. The mechanical attraction of magnetic mediators was utilized to induce protein aggregation, triggering biological signaling cascades. Magnetoelectric transduction yielded an electric dipole between a magnetostrictive core and a piezoelectric shell under alternating magnetic fields. This dipole can modulate membrane potential and activate voltage-gated ion channels. In past research, magneto-mechanical techniques attracted more attention, achieving the level of technical maturity required for their applications as essential tools in fundamental research.
 |
| Fig. 22 Magnetic transduction mechanisms. Reproduced with permission from ref. 235 and 238. Copyright 2021, 2024, Nature. | |
In a study, magnetic particles bonded to neuron membranes, which can stretch the lipid bilayer to enhance the unfolding probability of endogenous mechanosensitive channels. A 3D magnetic hyaluronic acid (HA) hydrogel was used to stimulate primary dorsal root ganglion (DRG) neurons through magnetogenetics (Fig. 23A).239 The magnetic HA hydrogel mimicked the biochemical and biophysical characteristics of the ECM, which facilitated the healthy development of neurites producing essential ion channels. Magnetomechanical stimulation can activate natural mechanosensitive TRPV4 and PIEZO2 channels on primary DRG neurons to induce Ca2+ influx. This work provided a general strategy to remote magneto-mechanically modulate excitable neurons using 3D magnetic hydrogels. Superparamagnetic iron oxide (SPIO) nanoparticles exhibited great potential in magnetic neuro-stimulations due to good biocompatibility, clear pharmacokinetics, and high spatial resolution. However, the SPIO nanoparticles diffused and were metabolized rapidly in vivo, reducing spatial resolution and modulation effectiveness. In a study, injectable microgels packed with SPIO nanoparticles (80–160 μm) were prepared to improve nanoparticle stability, magnetic characteristics, and stimulation efficacy (Fig. 23B).240 The magnetic microgels can reside in the cortex region of rats for up to 22 weeks. A single magnetic microgel can produce a mechanical force of 7–8 pN under 10 Hz magnetic stimulation. Electrophysiological measurements revealed that basal-synaptic transmission in the left frontal cortex was significantly enhanced by the magnetic microgels under 10 Hz magnetic stimulation (100 mT).
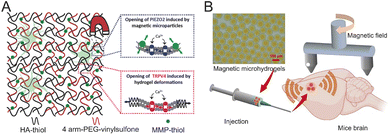 |
| Fig. 23 (A) Mechanism of magneto-mechanical stimulation of DRG neurons with magnetic HA hydrogels. Mechanosensitive PIEZO2 channels and mechanosensitive TRPV4 channels are activated by magnetic force-induced HA hydrogel deformation. Reproduced with permission from ref. 239. Copyright 2018, Wiley Online Library. (B) Schematic of a magnetic microhydrogel for improvement of magnetic neuro-stimulation. Reproduced with permission from ref. 240. Copyright 2023, Springer Nature. | |
Magnetic neuromodulation could provide a remote, deep-tissue, and non-invasive way of controlling neuronal activity. However, it still suffers some serious limitations including restricted magnetic field focusing, low magnetogenetic construct efficacy, long-term magnetic stability, and the possible cytotoxicity of magnetic nanoparticles. The potential solutions include embedding superparamagnetic nanoparticles into hydrogel scaffolds to create localized magnetic gradients, increasing the expression of highly responsive magnetosensitive ion channels and developing magnetic hydrogels that retain their properties over time. In addition, the cytotoxicity of magnetic nanoparticles can be reduced by coating them with a thin hydrogel layer, while the complex nanoparticles retain excellent magnetic responsiveness. More efforts should be devoted to improving ion channel sensitivity to magnetic fields. The long-term effects of magnetic stimulation on neuroplasticity and cognitive function should also be investigated. The long-term impact of magnetic stimulation on magneto-mechanical sensitive ion channel overexpression remains uncertain. Further research is essential to evaluate the potential cumulative effects of magnetic neuromodulation, such as neuroadaptation or neurotoxicity.
Understanding brain functions and treating neurological disorders necessitates the precise identification and targeted manipulation of specific neurons. A series of neuromodulation techniques have been developed to address this need. Hydrogels play a pivotal role in these neuromodulation techniques as supporting materials. Hydrogels can be tailored with high transparency and low optical loss to ensure effective light transmission for optogenetics. Conductive hydrogels serve as key interface materials to regulate cellular activities by applying electrical currents. Although hydrogels exhibited great advantages for neuromodulation, there are limited examples in this field and the related studies are still in the starting stages. There are numerous research gaps in the field for further exploration in the future. For example, in situ neural signal monitoring and neuromodulation are highly desirable for investigating the function mechanism of neural circuits and treating neurological disorders, which still remain significant challenges. Magnetogenetics utilizes superparamagnetic nanoparticles to conduct precise and effective neurostimulations. However, the concentrated magnetic nanoparticles will diffuse readily and be metabolized soon in brain tissues. The resident period and biocompatibility can be significantly prolonged by wrapping these magnetic nanoparticles with hydrogel networks. Hydrogels also exhibit minimal acoustic attenuation, which leads to great potential in sonogenetic neuromodulation.
5. Brain disease treatment
Brain diseases are among the most complex and difficult medical conditions, impacting millions of people worldwide including conditions such as Alzheimer's disease and Parkinson's disease, epilepsy, and stroke.241,242 These disorders lead to substantial cognitive, motor, emotional, and sensory impairments, primarily due to dysfunction in neural circuits. Current clinical treatments for brain diseases typically involve a mix of medications, surgery, and supportive therapies. While these methods can alleviate some symptoms, they cannot achieve complete recovery, and the conditions often deteriorate over time. Neuromodulation offers valuable tools for exploring the complexities of brain function and regulating dysfunctional neural circuits, which may slow the progression of brain diseases.243–246 Specifically, neuromodulation can precisely modulate abnormal neural circuits by either generating or inhibiting APs, helping to restore or enhance brain function.247,248 This capability to directly influence neural circuits allows for an unprecedented level of control over brain activity, making neuromodulation a highly promising area for treating brain diseases. In this context, we aim to present a thorough overview of how brain diseases can be treated through neuromodulation, with a particular emphasis on the role of hydrogel neural electrodes in these therapies. Given the limited examples available, we will also discuss alternative electrode materials for neuromodulation to create a more systematic framework that connects neuromodulation with brain disease treatment. This section seeks to explore new possibilities in neuromodulation, ultimately moving toward more precise and effective treatments for brain diseases, and inspiring the scientific community to gain fresh insights and pursue further research in this area (Table 2).
Table 2 Brain disease treatments with various neuromodulations
Brain diseases |
Neuromodulation mechanisms |
Stimulation parameters |
Target brain areas |
Electrode materials |
Ref. |
Stroke |
Optogenetic neuromodulation for regulating brain neural circuits and reducing infarct regions |
Optogenetics at 473 nm, 20 Hz, 5 ms, stimulation for 14 days |
iM1 |
Topological hydrogel |
43
|
Stroke |
Optogenetics-based circuit modulation and neuroplasticity enhancement |
Optogenetics at 472 nm, 10 Hz, 20 ms, three sessions/day for 18 days |
iM1 |
Amylopectin-PAAm hydrogel |
258
|
Stroke |
Optogenetic stimulation to enhance plasticity and neurotrophin expression |
Optogenetics at 473 nm, 10 Hz, 20 ms light pulses, stimulation for 10 days |
iM1 |
|
259
|
Stroke |
Restoration of corticothalamic and corticocortical circuits |
Optogenetics at 473 nm, 10 Hz, 1 min day−1 repeated for 14 days |
Corticothalamic, peri-infarct regions |
|
260
|
Alzheimer's disease |
SuM-induced enhancement of neurogenesis and ABNs |
Optogenetic stimulation at 10 Hz for 32 days, 1-min intervals |
SuM |
|
266
|
Alzheimer's disease |
Optogenetic stimulation |
Optogenetics at 473 nm, 10 Hz, 1-min pulses for 14 days |
Hippocampus |
|
267
|
Alzheimer's disease |
TrkA receptor activation without p75NTR |
MRIgFUS-assisted D3 delivery to basal forebrain, repeated weekly |
Basal forebrain |
|
268
|
Alzheimer's disease |
Ultrasound opening of blood–brain barrier for amyloid clearance |
Focused ultrasound, continuous |
Brain regions involved in amyloid plaque deposition |
|
269
|
Parkinson's disease |
Chemogenetic and optogenetic stimulation of caudal PPN neurons |
Optogenetics at 473 nm, 40 Hz, 10 ms pulses |
PPN |
|
278
|
Parkinson's disease |
Optogenetic activation of CnF glutamatergic neurons to control locomotion |
Optogenetics at 473 nm, 20 Hz |
CnF |
|
279
|
Parkinson's disease |
Direct-pathway activation increases locomotion; indirect pathway causes bradykinesia |
Optogenetics at 470 nm, direct-pathway excitation at 1 mW |
SNr, globus pallidus |
|
280
|
Parkinson's disease |
Wireless MMG-DBS system activation via magnetic torque for motor recovery |
Rotating magnetic field, continuous |
STN |
Magnetic nanoparticles (m-Torquers) |
281
|
Epilepsy |
Optogenetic stimulation of astrocytes to modulate K+ buffering and attenuate seizures |
Optogenetics at 473 nm, 20 Hz |
M1 |
|
299
|
Epilepsy |
Optogenetic inhibition of FC neurons in mesial temporal lobe epilepsy models |
Blue light pulses, closed-loop system |
FC, hippocampus |
|
300
|
Epilepsy |
NIR-assisted neuromodulation and neuroprotection against seizures |
NIR light at 980 nm, short pulses |
Hippocampus |
TiS2/Pt nanotransducers |
301
|
Epilepsy |
Closed-loop optogenetic seizure suppression |
Optogenetics at 530 nm, 31.2–35.8 mW mm−2, 10 s illumination |
DG, CA1 |
|
303
|
Epilepsy |
Wireless DBS via magnetoelectric nanochains |
Pulse magnetic stimulation |
ANT |
Magnetoelectric Fe3O4@BaTiO3 nanochains |
302
|
Depression |
Bidirectional modulation of depression-like behaviors |
Optogenetic inhibition at 593 nm light |
VTA |
|
305
|
Depression |
Orbitofrontal cortex-hippocampus pathway potentiation |
10 Hz rTMS, 1800 pulses per session, 2 weeks |
DLPFC, OFC, HPC |
|
306
|
Depression |
Astrocyte-driven functional connectivity enhancement |
Optogenetic activation, 473 nm, 10 Hz |
mPFC |
|
307
|
Depression |
Personalized biomarker-driven closed-loop neuromodulation |
Bipolar stimulation at 100 Hz, 120 μs, 1–2 mA |
VC/VS, amygdala |
|
308
|
Neuropathic pain |
Optogenetic activation of PFC pyramidal neurons for pain relief |
Optogenetics stimulation upon detected pain |
ACC, PFC |
|
311
|
Neuropathic pain |
Real-time pain detection with automated PFC neuromodulation |
Optogenetics-based PFC neuromodulation linked to pain decoding |
S1, ACC, PFC |
|
312
|
Neuropathic pain |
Brain-to-spleen vagal signaling modulating immune responses |
Optogenetics at 470 nm; chemogenetics (hM4Di/hM3Dq) for vagus nerve |
S1, central amygdala, vagus nerve to Spleen |
Hydrogel-based neuromodulation system |
313
|
5.1 Brain stroke
Stroke is often caused by the rapid rupture or blockage of cerebral blood vessels, leading to hemiplegic paralysis or even death.249 Current clinical treatment strategies primarily focus on thrombolysis and intra-arterial thrombectomy. While modern clinical treatments are effective in managing stroke, achieving complete recovery remains difficult, and hemiplegic paralysis and related impairments typically worsen over time.250,251 Recent developments in neuromodulation enable the recording and regulation of neural signals, which helps to reconstruct neural circuits in affected brain areas and restore physiological functions.252–257 Infarcted regions in stroke-affected brain tissues disrupt neural signal transmission, leading to brain dysfunctions. In one study, optogenetic neuromodulation was applied in the M1 of stroke rats using a transparent and conductive topological hydrogel neural electrode.43 The excellent transparency and conductivity of neural electrodes allowed for simultaneous in situ recording of neural signals during the neuromodulation process. After 12 days of optogenetic modulation, the infarct volume in stroke rats was significantly reduced from 22% to 4% (Fig. 24A). Furthermore, the creep speed of the stimulated stroke rats recovered to 80% of its original level after the same duration. A seamless brain–electrode interface was created using amylopectin-based hydrogels to enhance neural signal recording and neuromodulation with a negligible immunological response (Fig. 24B).258 Optogenetic stimulation (472 nm, 10 Hz, 20 ms duration) was utilized to modulate neural circuits in rat M1, resulting in improved synaptic plasticity and functional motor recovery in stroke models. The study found that optogenetic activation consistently changed neuronal excitability and LFP responses, with robust relationships between evoked neuronal activity and motor performance. In stroke-affected rats, amylopectin hydrogel-based neuromodulation drastically reduced infarct size (from 36% to 8%), boosted BDNF and PSD95 expression, and restored locomotor activity, indicating a promising neuroprotective technique. Another investigation explored the recovery mechanism in stroke rats by selectively activating neurons in the ipsilesional primary motor cortex (iM1).259 The pyramidal neurons in the iM1 region were selectively activated to assess the effect of repeated neuronal activations on stroke recovery (Fig. 24C). The optogenetic neuromodulation notably enhanced cerebral blood flow and the neurovascular coupling response. Additionally, the stimulated stroke rats exhibited a significant increase in GAP43 protein levels in the ipsilesional somatosensory cortex (iS1) and contralesional M1 (cM1) regions, indicating synaptic plasticity and reorganization following stroke. This suggests that neuronal stimulation can facilitate synaptic plasticity.
 |
| Fig. 24 (A) Optogenetic stimulation paradigm and location in promoting the recovery of locomotor function in stroke rats. Reproduced with permission from ref. 43. Copyright 2023, Wiley Online Library. (B) Optogenetic stimulation paradigm and location in promoting the recovery of locomotor function in stroke rats. Reproduced with permission from ref. 258. Copyright 2024, Wiley Online Library. (C) iM1 neuronal stimulation activates peri-infarct areas and the contralesional cortex, increasing the GAP43 expression of neurotrophins after stroke. Reproduced with permission from ref. 259. Copyright 2014, PNAS. (D) The experimental timeline and procedures and selective stimulation of the corticothalamic circuit to improve functional recovery after stroke. Reproduced with permission from ref. 260. Copyright 2021, AAAS. | |
While optogenetic stimulation in the iM1 region has been established as an effective treatment method for stroke, effects on overall network dynamics and the specific downstream circuits involved in recovery remain unclear. To investigate these mechanisms further, optogenetic functional magnetic resonance imaging (ofMRI) was employed to analyze neural circuit dynamics across the entire brain (Fig. 24D).260 This innovative approach combined optogenetic activation with functional magnetic resonance imaging, allowing for longitudinal assessments of brain circuitry dynamics during the recovery phase. By selectively manipulating specific neurons and circuits through optogenetic stimulation, researchers were able to investigate their roles in post-stroke recovery in detail. The study revealed that optogenetic stimulation in the iM1 region effectively reinstated the activation of the corticothalamic neurons on the side of the brain affected by the stroke, with activation levels correlating directly to the functional recovery of stroke-affected rats. Additionally, the study specifically activated the neural circuit from iM1 to the thalamus, which enhanced sensorimotor recovery, evidenced by a significant increase in the creep speed of stroke rats. These findings underscore the critical role of the corticothalamic circuit in facilitating early poststroke recovery. Overall, this research represents a significant advancement in neural electrode technology, enabling the capture of neural signals, neuromodulation, and stroke treatment, and makes an important step toward understanding brain information processing and addressing brain function disorders.
5.2 Alzheimer's disease
Alzheimer's disease, the foremost cause of dementia, has swiftly become one of the most widespread and fatal diseases of this century, with no effective treatment currently available. Projections suggest that by 2050, the global number of Alzheimer's disease individuals could exceed 150 million.261 This situation brings considerable challenges and distress for both patients and healthcare providers, along with significant financial implications.262 The formation of amyloid-beta (Aβ) plaques is viewed as the primary pathological event in Alzheimer's disease.263,264 This event triggers the deposition of tau, neuroinflammation, neuronal and synaptic loss, and cognitive decline. Both neuropathological and genetic evidence support this pathology.265 The exploration of treatment options for this incurable condition is a high priority in medical and neuroscience research, given the profound challenges involved. The dentate gyrus (DG) contains NSCs that persist in producing adult-born neurons (ABNs) throughout an individual's life, a phenomenon referred to as adult hippocampal neurogenesis (AHN). Typically, Alzheimer's disease is associated with reduced AHN and a marked decline in activity. Recently, neuromodulation has emerged as a novel strategy to alter disrupted neural circuits, presenting a promising pathway for Alzheimer's disease therapy. Enhancing AHN activity in the Alzheimer's disease-affected brain tissues is essential for restoring the functionalities of the hippocampus, a crucial area for memory and learning. One study demonstrated that optogenetic neuromodulation significantly boosted AHN activity, leading to functional recovery in Alzheimer's disease mouse models (Fig. 25A).266 The optogenetic stimulation targeted the supra mammillary nucleus (SuM) in a key subcortical region of the hypothalamus throughout the various stages of Alzheimer's disease stages. This stimulation notably increased the number of adult hippocampal neurons, improving memory function and reducing anxiety and depression-like behaviors. It has been noted that microglia play a vital role in clearing pathological Aβ plaques, helping to maintain brain homeostasis.267 By utilizing optogenetic methods to selectively manipulate microglia, researchers enhanced the clearance of Aβ plaques in brain tissue (Fig. 25B). However, synapse elimination occurred during this process, attributed to an increase in complement C1q due to optogenetic neuromodulation. Consequently, a synergistic strategy was proposed to eliminate Aβ plaques while preserving sprouting, combining optogenetic stimulation with C1q antibody treatment to inhibit complement C1q.
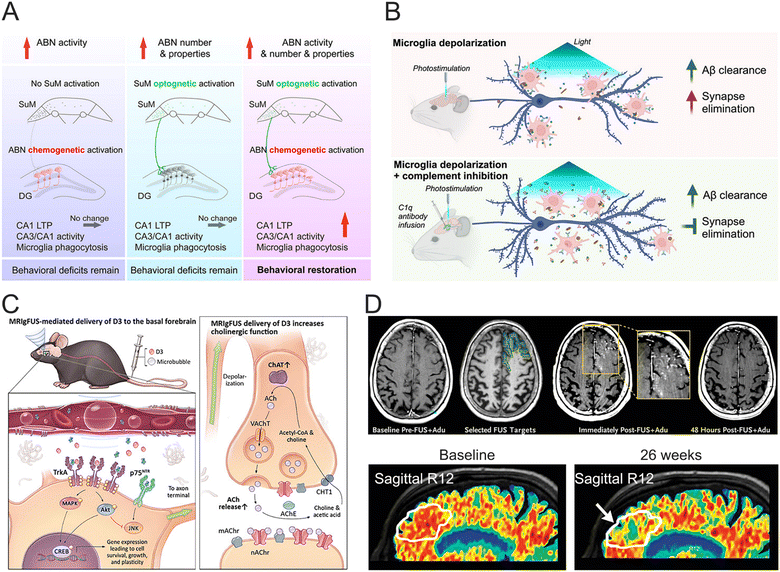 |
| Fig. 25 (A) Patterned stimulation of SuM enhances hippocampal neurogenesis in Alzheimer's disease. Reproduced with permission from ref. 266. Copyright 2023, Elesevier. (B) Targeted manipulation of microglia by optogenetics. Reproduced with permission from ref. 267. Copyright 2023, Elesevier. (C) MRIgFUS delivery of D3, a selective TrkA agonist, to the basal forebrain rescued neurotrophin signaling and cholinergic function in the TgCRND8 model of Alzheimer's disease. Reproduced with permission from ref. 268. Copyright 2020, AAAS. (D) BBB opening with focused ultrasound (FUS) to reduce Aβ levels. Reproduced with permission from ref. 269. Copyright 2024, NEJM Group. | |
The extensive loss of neurons and synapses, along with the degeneration of basal forebrain cholinergic neurons and decreased cholinergic innervation in both the cortex and hippocampus, contributes to cognitive decline. During the progression of Alzheimer's disease, cholinergic neurons undergo trophic changes, which involve alterations in nerve growth factor (NGF) processing, NGF receptor expression, and activation of downstream elements in the NGF signal pathway. Typically, NGF supports neuronal survival, growth, and synaptic plasticity through its interaction with tropomyosin receptor kinase A (TrkA) and p75 neurotrophin receptor. Tailored ligands can selectively activate TrkA, providing a promising therapeutic approach to enhance cholinergic functions. For instance, one study illustrated the noninvasive administration of D3 (a TrkA peptidomimetic agonist) to the basal forebrain in an AD mouse model using magnetic resonance imaging-guided focused ultrasound (MRIgFUS).268 This localized delivery of D3 activated TrkA signaling, effectively restoring cholinergic function even in the presence of Aβ pathology. The long-term administration of D3 via MRIgFUS significantly improved cognitive function in AD rats by modulating cholinergic neurotransmission (Fig. 25C). Pharmacological treatment remains a core strategy in managing Alzheimer's disease, crucial for delaying its progression.270 Targeted pharmacological interventions can effectively reduce cognitive decline and manage behavioral symptoms. However, the blood–brain barrier (BBB) poses a significant hurdle for optimizing Alzheimer's disease pharmacological therapy. Ultrasound stimulation can facilitate the opening of the BBB, thereby enhancing the efficacy of anti-Aβ therapeutic interventions. For example, aducanumab was combined with focused ultrasound to breach the BBB in specific brain regions (Fig. 25D).269 An MRI-guided system was used to direct ultrasound to the designated brain areas, introducing microbubbles to temporarily open the BBB. This combined ultrasound and pharmacological approach led to a significant reduction in Aβ levels in targeted brain regions. This research represents a critical advancement in the quest for combination therapies for AD.
5.3 Parkinson's disease
Parkinson's disease is a significant degenerative neurological disorder characterized by the degeneration of dopamine-producing neurons in the brain. The underlying pathophysiological mechanisms involve a complex interplay of various factors, such as the abnormal aggregation of α-synuclein, mitochondrial dysfunction, lysosomal and vesicular transport impairment, disruption in synaptic transmission, and neuroinflammation, all contributing to the accelerating loss of dopaminergic neurons.271,272 While pharmacological treatments remain the primary approach, there is an ongoing exploration of alternative strategies to modify the disease's progression and alleviate its symptoms.273 Research indicates that deep brain stimulation (DBS) targeting the mesencephalic locomotor region can facilitate rehabilitation of Parkinson's disease.223–233 This region includes the pontine nucleus and the wedge nuclei, with the pedunculopontine nucleus (PPN) being for locomotion functions due to its glutamatergic neurons, which initiate and sustain movement.274–277 A study showed that stimulating caudal glutamatergic PPN neurons through combined chemo- and optogenetic neuromodulation successfully normalized severe locomotor impairments in Parkinson's disease rats (Fig. 26A).278 Another study examined the effects of wedge nucleus stimulation on Parkinson's disease (Fig. 26B).279 Here, optogenetic stimulation selectively activated Vglut2-positive glutamatergic neurons in the wedge nucleus, with channelrhodopsin expressed in a core-dependent manner. This activation increased the frequency of motor initiations, prolonged the duration of motor activity, and modulated movement speed, highlighting the cuneiform nucleus glutamatergic neurons as a relevant target for enhancing locomotor functions in Parkinson's disease.
 |
| Fig. 26 (A) Chemogenetic activation of glutamatergic PPN neurons rescues motor suppression induced by D1-receptor antagonism (left) and optical excitation of glutamatergic PPN neurons acutely alleviates D1- and D2-mediated akinesia (middle and right). Reproduced with permission from ref. 278. Copyright 2022, Nature. (B) Optogenetic stimulation of the cuneiform nucleus (CnF) increases locomotor activity in Vglut2-ChR2-EYFP mice lesioned with 6-OHDA. Reproduced with permission from ref. 279. Copyright 2021, PNAS. (C) Direct-pathway activation rescues motor deficits of Parkinson's disease. Reproduced with permission from ref. 280. Copyright 2010, Nature. (D) Schematic illustration of the overall processes of magneto-mechanical genetics-DBS treatment in the Parkinson's disease mouse model (left) and assessment of immediate and prolonged therapeutic effects of magneto-mechanical genetics-DBS on Parkinson's disease mice (right). Reproduced with permission from ref. 281. Copyright 2023, ACS Publications. | |
The neuronal circuits within the basal ganglia are essential for motor planning and action decision-making. There are two primary pathways: the ‘direct’ and ‘indirect’ pathways, which have opposing effects on movement. The motor deficits associated with Parkinson's disease are linked to heightened activity in the indirect pathway and diminished activity in the direct pathway.282–285 Optogenetic neuromodulation demonstrated that stimulating the 'indirect' pathway exacerbates Parkinson's disease symptoms, while activation of the 'direct' enhances motor function (Fig. 26C).280 Parkinson's disease mouse models with bilateral lesions in the dorsomedial striatum were created using 6-hydroxydopamine (6-OHDA), resulting in nearly complete loss of dopaminergic innervation and significant motor deficits. Efforts to alleviate these symptoms by activating both sides of the direct pathway in the dorsomedial striatum, even without dopamine supply, revealed that optogenetic stimulation of the direct pathway restored motor behavior to pre-injury levels. This activation alleviated bradykinesia, increased locomotor initiations, and reduced freezing, suggesting that ‘direct’ pathway stimulations offer an effective therapeutic strategy for managing Parkinson's disease.
A notable limitation of current DBS technology is its dependence on a wired, permanently implanted system.286 Recent research explored the therapeutic potential of remote magnetic nanoparticle neuromodulation technology, eliminating the need for physical connections between the external stimulator and the brain.287 Magnetothermal deep brain stimulation (mDBS) activates thermosensitive ion channels like the transient receptor potential cation channel subfamily V member 1 (TRPV1). The mDBS opens TRPV1 channels, facilitating Ca2+ influx and inducing neuronal excitation. This process activates specific neuronal circuits, effectively reversing forelimb deficits, enhancing treadmill performance and improving locomotion speed. Magneto-mechanical genetics offers unique advantages for wireless DBS, allowing operation over long distances while maintaining cellular and anatomical precision. This magneto-mechanical genetics-based DBS approach was used to increase neuronal activity in the subthalamic nucleus (STN) and alleviate motor symptoms in Parkinson's disease mouse model, as shown in Fig. 26D.281 Nanoscale magnetic force actuators (m-Torquer) were used to generate torque to activate pre-encoded Piezo1 ion channels in specific neurons. This wireless STN-specific DBS approach eliminates the need for implants and resolves the issue of wiring, demonstrating a significant 2-fold increase in locomotor activity and motor balance in both late- and early-stage Parkinson's disease animals compared to untreated Parkinson's disease mice.
5.4 Epilepsy
Epilepsy is characterized by abnormal neuronal discharges in the brain, leading to recurrent seizures. This condition can persist for extended periods and is linked to a high rate of disability. Surgical interventions have become a major focus in epilepsy treatment. While 70% of cases can be managed with antiepileptic drugs or surgical methods, 30% of patients experience drug-resistant epilepsy that remains difficult to treat with conventional approaches. Recent advances in neuromodulation aim to decrease the frequency and intensity of seizures by stimulating the nervous system to better regulate brain electrical activity.288–294 Optogenetic neuromodulation enables precise and temporary control of specific neuronal populations using light-sensitive opsins, making it a promising option for seizure management. Emerging research underscores the significant role of astrocytes in epilepsy due to their various functions, including buffering extracellular ions, clearing neurotransmitters, releasing gliotransmitters, and producing inflammatory mediators.295–298 Through optogenic manipulation of astrocyte-to-neuron signaling, researchers have been able to examine how astrocytes can directly impact neuronal excitability and network dynamics.299 In a study involving the GFAP-Cre mice, a Cre-dependent ChR2-EYFP AAV virus was used to selectively target astrocytes in the M1 region. Optogenetic stimulation of these astrocytes during neocortical seizures (473 nm, 20 Hz, 5 mW, 10 ms), significantly reduced seizure progression, evidenced by a decrease in seizure severity and delay in onset (Fig. 27A). Furthermore, even early-phase stimulation of astrocytes consistently lowered seizure severity across all phases, indicating a reduction in seizure stages and delayed onset. This antiseizure effect was attributed to the astrocytic Na+-K+-ATPase-mediated K+ buffering, which suppressed the activity of hyperactive pyramidal neurons during seizures. Another investigation identified the fasciola cinereum neurons in the posterior hippocampal tail as a promising target for intervention, as shown in Fig. 27B.300 Closed-loop optogenetic inhibition of fasciola cinereum neurons led to a significant reduction in seizure duration. This study revealed the notable role of fasciola cinereum neurons during seizures in a group of six epilepsy patients, particularly noting that targeted lesioning of the fasciola cinereum resulted in decreased seizure burden following the ablation of anterior mesial temporal structures.
 |
| Fig. 27 (A) Optogenetic stimulation of ChR2-expressing astrocytes attenuates kainic acid-induced neocortical seizures. Reproduced with permission from ref. 299. Copyright 2022, Nature. (B) The fasciola cinereum in mice is an intervenable target for the treatment of epilepsy. Reproduced with permission from ref. 300. Copyright 2024, Nature. (C) Nongenetic precise neuromodulation and spatiotemporal neuroprotection for epilepsy therapy. Reproduced with permission from ref. 301. Copyright 2024, ACS Publications. (D) Deep wireless magnetoelectric brain therapy platform in anti-epilepsy. Reproduced with permission from ref. 302. Copyright 2023, ACS Publications. (E) Optogenetic device for HD-MEAs. Hardware components: the MaxWell CMOS HD-MEA system is augmented with an optogenetic platform with a cross-section of optogenetic well insert showing main components, a close-up of the optical fiber creating illumination, and software modules. Reproduced with permission from ref. 303. Copyright 2024, Nature. | |
Additionally, a novel nano neuromodulation strategy with neuroprotective properties was proposed for the effective and minimally invasive treatment of epilepsy (Fig. 27C).301 This involved an engineered nanotransducer with a Pt coating on titanium disulfide (TiS2), referred to as TiS2/Pt. This device converts near-infrared (NIR) light into localized heat, allowing for high temporal and spatial resolution in non-genetic neuromodulation. Although DBS has shown promise in treating drug-resistant epilepsy, the development of wireless DBS remains a significant challenge. The enzyme-mimicking capabilities of TiS2/Pt facilitate the efficient clearance of harmful free radicals, thereby shielding neurons from oxidative damage. The combination of non-genetic NIR neuromodulation and neuroprotection was effective in reducing abnormal high-frequency electrical activity linked to epilepsy and alleviating oxidative stress caused by hyperexcitability. A conceptual platform for wireless epilepsy was created to enable localized electrical stimulation using 1D-structured magnetoelectric Fe3O4@BaTiO3 nanochains (FBC) (Fig. 27D).302 The FBC exhibited enhanced electrical output due to increased local strain induced by their anisotropic structure and strain coherence. Initially, in vitro wireless magnetoelectric neuromodulation was successfully performed by activating voltage-gated ion channels, aided mechanosensitive ion channels. Subsequently, FBCs were delivered into the anterior nucleus of the thalamus (ANT), leading to significant inhibition of both acute and continuous seizures. This approach demonstrated remarkable effectiveness in suppressing the propagation of high-voltage electroencephalogram signals and mitigating behavioral seizure stages. This research introduces a new strategy for epilepsy treatment and provides insights for addressing other neurological disorders. A recent study found that AAV-mediated optogenetic suppression of excitatory CAMK2A+ neurons in human hippocampus slices effectively decreased epileptiform activity (Fig. 27E).303 Using HcKCR1, a K+-selective inhibitory channelrhodopsin, light activation at 530 nm hyperpolarized excitatory pyramidal neurons, significantly lowering neuron excitability while preserving inhibitory interneurons. The study used high-density microelectrode arrays (HD-MEAs) to investigate the effects of optogenetic neuromodulation on LFPs and neuronal firing rates. Optical stimulation at 31.2–35.8 mW mm−2 for 10 s significantly reduced theta-frequency oscillations, a key factor in seizure propagation. The viability of closed-loop optogenetic neuromodulation for seizure management was demonstrated by integrating the system with a real-time spike detection algorithm that initiated optogenetic stimulation when abnormal neuronal activity was beyond a predetermined threshold. These results show an important milestone for the creation of implantable optogenetic BCIs for the treatment of epilepsy.
5.5 Depression
Major depressive disorder (MDD) is a serious condition characterized by various symptoms, including a (Table 2) persistent sense of sadness, suicidal thoughts, slowed or restless movements, and a diminished interest in pleasurable activities.304 Current medical treatments for depression often fall short of producing effective outcomes for many patients and can lead to significant side effects. It is necessary to achieve a more profound comprehension of depression mechanisms and corresponding treatment methods. Optogenetic neuromodulation, known for its high spatiotemporal resolution and efficient selectivity, has been utilized to explore potential targets and the underlying mechanisms of depression. For instance, midbrain dopamine neurons were selectively manipulated using an optogenetic technique to address depressive behaviors in both positive and negative contexts (Fig. 28A).305 In this study, a Cre-dependent AAV was used to transfect the ventral tegmental area of rats, allowing for the expression of ChR2 within these neurons. Optogenetic stimulation at 473 nm was employed to selectively activate ventral tegmental area dopamine neurons, effectively reversing the reduction in sucrose preference induced by chronic mild stress and improving depression behaviors. These results indicate that the ventral tegmental area dopamine neurons play a crucial role in influencing various depression-related behaviors. Additionally, repeated transracial magnetic stimulation (rTMS) was applied to specifically target the dorsolateral prefrontal cortex (DLPFC) of patients with major depressive disorder.306 This technique stimulates neural activity in the hippocampus (HPC) and orbitofrontal cortex (OFC), enhancing connectivity between these regions, as illustrated in Fig. 28B. This improved connectivity is critical for alleviating depression symptoms. Furthermore, the role of astrocytes in MDD and their mechanisms were examined using optogenetic techniques, revealing that astrocyte dysfunction results in abnormal resting-state functional connectivity (rsFC) in MDD.307 By activating astrocytes in the medial prefrontal cortex (mPFC) with optogenetic stimulation (473 nm, 10 Hz, 3.5 mW), researchers were able to enhance rsFC in depression-related networks. The activation of mPFC neurons and the pathway connecting the mPFC to the striatum restored the disrupted rsFC, thereby alleviating depressive-like behaviors (Fig. 28C).
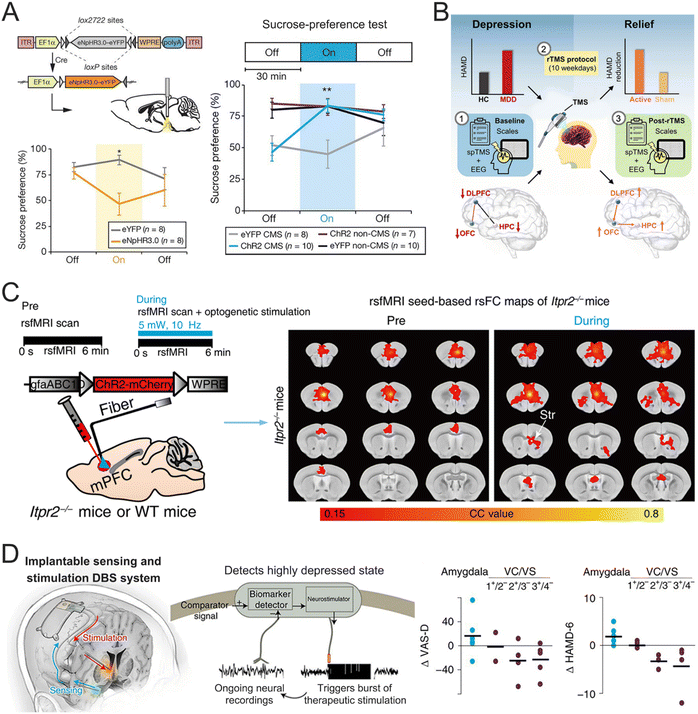 |
| Fig. 28 (A) Selective inhibition of ventral tegmental area dopamine neurons induces a depression-like phenotype (left) and temporally sparse phasic photoactivation of ventral tegmental area dopamine neurons rescues stress-induced depression-like phenotype (right). Reproduced with permission from ref. 305. Copyright 2012, Nature. (B) Patients with MDD have impaired regional excitability and inter-regional connectivity and DLPFC-targeted rTMS treatment relieves depression. Reproduced with permission from ref. 306. Copyright 2023, Elsevier. (C) Optogenetic stimulation of mPFC astrocytes increases rsFC within depression-related networks. Reproduced with permission from ref. 307. Copyright 2022, AAAS. (D) Implementation of closed-loop neuromodulation for depression treatment. Reproduced with permission from ref. 308. Copyright 2021, Nature. | |
A systematic evaluation of acute responses in patients with severe depression was carried out using multi-site intracranial electrodes.309 These electrodes enabled electrical neuromodulation to explore the link between changes in intracranial brain stimulation and the patient's psychological state. Closed-loop DBS systems can modify stimulation parameters based on real-time neural activity, offering more precise and effective treatments for chronic depression. Another notable development is closed-loop neuromodulation aimed at identifying individualized biomarkers related to symptoms, which helps determine the most effective stimulation sites.308 The NeuroPace System was designed to continuously monitor neural activity and was implanted unilaterally in the right hemisphere of the brain, targeting two specific areas: the amygdala and the ventral caudate/ventral subthalamic nucleus (VC/VS), respectively, as illustrated in Fig. 28D. When the NeuroPace System detects predefined patterns in neural activity, it initiates electrical stimulation through specific electrodes in these regions. Bipolar stimulation at the VC/VS contacts resulted in acute, dose-dependent improvements in symptoms across most trials. Additionally, this closed-loop therapy was applied to patients with chronic depression. Following 6 seconds of stimulation triggered by the automatic detection of biomarkers, there was a rapid reduction in both symptom severity and depression levels. This study highlighted a significant advancement in depression treatment through biomarker-driven closed-loop therapy.
5.6 Neuropathic pain
Currently, chronic pain management primarily depends on continuous drug administration. This ongoing pharmaceutical treatment can lead to side effects such as drowsiness, desensitization, and a risk of addiction.310 Neuropathic pain involves complex brain-immune connections that influence pain perception and immune system reactions. It has been reported that neuropathic pain controls splenic immune function through neuronal circuits including the S1, central amygdala, and vagus nerve projections to the spleen (Fig. 29A).311 Two different pain-state-dependent neuromodulatory pathways are identified in this study: chronic pain suppresses immune responses through inhibitory GABAergic projections from the central amygdala (GABACeA) to AChDMV neurons, whereas acute pain improves immune function through excitatory glutamatergic projections from the primary somatosensory cortex (GluS1HL) to cholinergic neurons in the dorsal motor nucleus of the vagus (AChDMV). This bidirectional modulation of immune function emphasizes the importance of the brain–spleen axis in chronic pain-induced immunological dysfunction. Retrograde tracing and optogenetic activation of AChDMV neurons confirmed a direct vagal projection from the brainstem to the spleen. Using chemogenetic inhibition of AChDMV neurons, blocking vagal output reduced splenic T-helper 2 immune cell activity in acute pain situations, whereas activating AChDMV neurons reversed immunological suppression in chronic pain models. The neuromodulation mechanism stresses the function of the vagus nerve in modulating systemic immunological responses to pain. According to these results, neuromodulation treatments offer a fresh approach to treat immunological dysregulation and pain.
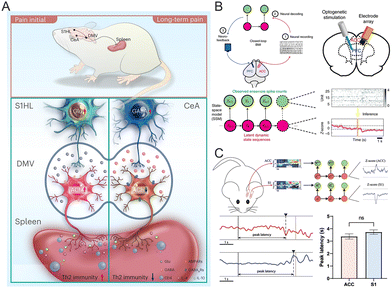 |
| Fig. 29 (A) Brain circuits mediate spleen immune responses upon neuropathic pain. Reproduced with permission from ref. 311. Copyright 2024, Nature. (B) Design of a closed-loop BMI to detect and treat pain. Reproduced with permission from ref. 312. Copyright 2021, Nature. (C) The design of a multi-region LFP-based neural interface for pain (top) and pain-evoked event-related potentials and pain decoder did not show a clear temporal sequence in cortical processing of a thermal pain stimulus (bottom). Reproduced with permission from ref. 313. Copyright 2023, Springer Nature. | |
A significant challenge in managing persistent pain is the need for targeted therapies that minimize the negative effects associated with excessive medication use. Recently, closed-loop BCI has emerged, providing demand-based pain relief by automatically monitoring neural activity to identify pain episodes and delivering therapeutic brain stimulation. A noteworthy advancement in pain management has been achieved through the use of closed-loop BCIs, which can manipulate sensory and emotional experiences in real-time in freely moving rats (Fig. 29B).312 These systems employ a state-space model to interpret the beginning of pain sensations by analyzing neural spike activity. The pain detection triggers optogenetic activation of the prelimbic prefrontal cortex (PFC), enabling top–down control of nociception. This innovative approach has effectively suppressed both sensory and emotional responses associated with various types of pain, including sudden physical pain, heat-induced pain, and persistent inflammatory or nerve-related pain. Recent research has enhanced our understanding of how pain signals are processed temporally in the brain's primary S1 and anterior cingulate cortex (ACC). For example, a study highlighted the distinct roles and timing of the S1 and ACC regions in processing the sensory and emotional dimensions of pain (Fig. 29C).313 Neurons in the S1 region primarily encode the sensory aspects of pain, while the ACC predominantly manages the emotional experience of pain. By simultaneously recording LFP signals from the SI and ACC through BCIs, researchers were able to conduct an in-depth analysis of temporal pain processing. Utilizing event-related potentials and a machine-learning pain decoder, the study found that mechanical pain stimuli elicited changes in brain activity in the S1 region, whereas thermal pain exhibited a similar processing timeline in both S1 and ACC. These insights into the temporal dynamics of pain are crucial for developing more efficient pain management strategies and our understanding of the interplay between the sensory and emotional components of pain.
Implantable hydrogel electrodes have shown transformative potential in the treatment of neurological disorders through precise neuromodulation. Despite their promising roles in neurological treatments, they still suffer from several challenges in maximizing their efficacy such as hydrogel swelling, mechanical instability, and limited electrical conductivity. Furthermore, the complexity of neural circuits necessitates advanced strategies for precise hydrogel integration and modulation. Future research needs to concentrate on creating disease-specific hydrogel systems that integrate neuromodulation and real-time biosensing capabilities, enabling adaptable and individualized therapy options for patients with brain diseases. Hydrogel-based brain interfaces can be further enhanced for clinical and research applications by combining innovative material designs, cutting-edge fabrication techniques, and biofunctional improvements, ultimately advancing the fields of neuroscience and neuroengineering.
6. Conclusions and future perspectives
BCIs present a valuable opportunity for exploring neural circuits and treating brain disorders. Central to BCIs are the technologies for monitoring and modulating neural signals, which are essential for understanding the mechanisms behind brain disorders and developing treatment strategies. However, the advancement of implantable neural electrodes is significantly hindered by the adverse FBR and performance degradation over time, stemming from the chemo-mechanical mismatch between electrodes and brain tissue. To create the next generation of neural interfaces, it is crucial to carefully evaluate the biological, electrical, chemical, and mechanical properties of electrode materials. This approach aims to minimize physical stress at the electrode–tissue interface and prevent rejection reactions. Currently, extensive research is underway focusing on the innovative design, material advancements, and functional modifications of neural electrodes. Hydrogel-based BCIs show considerable promise in neural signal monitoring and modulation due to their physicochemical properties that closely resemble those of brain tissue. Unlike conventional rigid electrodes, hydrogel neural electrodes exhibit unique tunable attributes, such as adjustable conductivity, modulus compatibility, adhesion, transparency, and biocompatibility. These characteristics render them particularly effective for recording brain signals and modulating neural activity. The biocompatibility and modulus alignment of hydrogels facilitates long-term implantation, enabling chronic neural signal recording. Hydrogel neuronal electrodes can manipulate neural activity by delivering optical, electrical, and acoustic stimuli to specific neural tissues, providing valuable insights into brain function and adjustment of dysfunctional neural circuits. Neuromodulation has emerged as a novel approach for manipulating neuronal activity through various stimuli—electrical, optical, chemical, acoustic, or magnetic—targeted at specific neural tissues. This technique holds promise in the treatment of brain diseases such as stroke, drug-resistant epilepsy, and Parkinson's. A widely accepted perspective is that neuromodulation enhances the secretion and transmission of neurotrophic factors, cytokines, and other nutrients, leading to changes in neural plasticity and the reorganization of neural circuits. Furthermore, neuromodulation can be a valuable tool for investigating neural circuits in brain diseases, elucidating the neural pathways involved and identifying therapeutic targets.
While hydrogels show great promise for BCIs due to their biocompatibility, flexibility, and ability to reduce immune reactions, various hurdles must be overcome before practical applications in clinical settings. The long-term stability and dependability of hydrogel-based implants in the brain's extremely reactive and dynamic environment is one of the main challenges. To ensure effective implementation, degradation rate, mechanical characteristics, and the possibility of chronic inflammation must all be carefully considered. Furthermore, hydrogels face the tradeoffs between electrical conductivity and modulus/transparency, which are crucial for chronic signal recording and conducting in situ signal recording and neuromodulations. How to balance the conductivity, modulus match, transparency, and biocompatibility of hydrogels will be an important direction for future research. These hydrogels often incorporate conductive fillers to improve electrical conductivity. However, a major challenge faced by these systems is incremental modulus and uneven electrical percolation, which can significantly impair their modulus match with brain tissues. This phenomenon is associated with several factors, including filler distribution, the interaction between conductive fillers and hydrogel matrix, and their dynamic chemical structures. It is possible to solve the tradeoff by employing special chemical modification strategies such as tuning the size and composition of fillers and designing dynamic topological structures of hydrogels. Another challenge is the difficulty of scaling up hydrogel electrode fabrication techniques, which are generally complicated, restricting their broad use. Future research should develop novel methods to fabricate hydrogel electrodes that are reproducible on a large scale. More research should be conducted to investigate interactions between hydrogels and neural tissues, such as hydrogel effects on neuronal growth, synaptic plasticity, and overall neural health. While both theoretical and clinical evidence supports the efficacy of neuromodulation in treating brain disorders, the exploration of its underlying mechanisms remains nascent. Genetic modifications can make cells responsive to magnetic and acoustic stimuli, enhancing spatial specificity; however, the response times of magnetogenetic and sonogenetic neuroregulation need to advance to match the temporal resolution and precision of cutting-edge optical techniques. Additionally, there is currently minimal application of hydrogels in magnetogenetics and sonogenetics, highlighting the potential for hydrogel-based neuromodulation technologies as promising trends for future development. Despite these challenges, advancements in material design and micro-nano-fabrication present an optimistic outlook for hydrogel-based BCIs to transform neural signal recording, neuromodulation, and the treatment of brain diseases. The remarkable potential of hydrogels lies in their capacity to overcome the limitations of existing BCIs and to unlock new possibilities in brain–computer interaction.
Abbreviations
3D | Three-dimensional |
6-OHDA | 6-Hydroxydopamine |
AAV | Adeno-associated virus |
ABNs | Adult-born neurons |
ACC | Anterior cingulate cortex |
AchDMV | Cholinergic neurons in the dorsal motor nucleus of the vagus |
AgNCs | Silver nanocubes |
AgNWs | Silver nanowires |
AHN | Adult hippocampal neurogenesis |
Alg | Alginate |
ANT | Thalamus |
AP | Action potential |
Arch | Ancient rhodopsin |
Aβ | Amyloid-beta |
BBB | Blood–brain barrier |
BAUS | Bioadhesive ultrasound |
BC | Bacterial cellulose |
BC-CPH | Bi-continuous conducting polymer hydrogel |
BCIs | Brain–computer interfaces |
BG | Bacterial cellulose–gelatin |
BGY | Bacterial cellulose nanofibril-reinforced |
Cas | Caseinate |
CDs | Cyclodextrins |
ChR2 | Channelrhodopsin-2 |
cM1 | Contralesional M1 |
CMOS | Complementary metal-oxide–semiconductor |
CnF | Cuneiform nucleus |
CNTs | Carbon nanotubes |
DBS | Deep brain stimulation |
DG | Dentate gyrus |
DLPFC | Dorsolateral prefrontal cortex |
DN | Double network |
DRG | Dorsal root ganglion |
ECoG | Electrocorticography |
ECM | Extracellular matrix |
EDL | Electrical double layers |
EGaIn | Eutectic gallium indium |
FBR | Foreign-body response |
GABACEA | GABAergic projections from the central amygdala |
GelMA | Methacrylate acylated gelatin |
GluS1HL | Glutamatergic projections from the primary somatosensory cortex |
GO | Graphene oxide |
GV | Gas vesicle |
HA | Hyaluronic acid |
HFOs | High-frequency oscillations |
HPC | Hippocampus |
iM1 | Ipsilesional primary motor cortex |
iS1 | Ipsilesional somatosensory cortex |
LFP | Local field potential |
M1 | Primary motor cortex |
mDBS | Magnetothermal deep brain stimulation |
MDD | Major depressive disorder |
MEMS | Microelectromechanical |
mPFC | Medial prefrontal cortex |
MRIgFUS | Magnetic resonance imaging-guided focused ultrasound |
NGF | Nerve growth factor |
NSCs | Neural stem cells |
OFC | Orbitofrontal cortex |
ofMRI | Optogenetic functional magnetic resonance imaging |
PAA | Poly(acrylic acid) |
PAAm/PAM | Polyacrylamide |
PANI | Polyaniline |
PDA | Polydopamine |
PDMS | Polydimethylsiloxane |
PEDOT:PSS | Poly(3,4-ethylenedioxythiophene):poly(styrene sulfonate) |
PEG | Polyethylene glycol |
PEGDA | Poly(ethylene glycol) diacrylate) |
PEG-LA-DA | Polyethylene glycol-lactide acid diacrylate |
PFC | Prefrontal cortex |
PI | Polyimide |
PPN | Pedunculopontine nucleus |
PPy | Polypyrrole |
Pt | Platinum |
PU | Polyurethane |
PVA | Poly(vinyl alcohol) |
RGO | Reduced graphene oxide |
rsFC | Resting-state functional connectivity |
rTMS | Transracial magnetic stimulation |
S1 | Somatosensory cortex |
SMCA | Shape-morphing cortex-adhesive |
SMP | Super macroporous |
SPIO | Super paramagnetic iron oxide |
SNR | Signal-to-noise |
STN | Subthalamic nucleus |
SuM | Supra mammillary nucleus |
TES | Transcranial electrical stimulation |
tFUS | Transcranial focused ultrasound |
V1 | Primary visual cortex |
VC/VS | The ventral caudate/ventral subthalamic nucleus |
vHPC | Ventral hippocampal projection cortex |
α-CD | α-Cyclodextrin |
Data availability
No primary research results, software, or code have been included and no new data were generated or analyzed as part of this review.
Conflicts of interest
There are no conflicts to declare.
Acknowledgements
The authors gratefully acknowledge the funding from the National Natural Science Foundation of China (22377122 and U22A20183), the Jilin Province Science and Technology Development Plan (SKL202402018), the International Partnership Program of the Chinese Academy of Sciences (029GJHZ2024038FN), and the Changchun City Science and Technology Development Plan (2024GZZ14).
References
- Y. Zhou, H. Song and G. L. Ming, Nat. Rev. Genet., 2024, 25, 26–45 Search PubMed.
- N. C. Rust and J. E. LeDoux, Trends Neurosci., 2023, 46, 3–4 CrossRef CAS PubMed.
- J. A. Lecoq, R. Boehringer and B. F. Grewe, Nat. Methods, 2023, 20, 495–496 Search PubMed.
- A. Greenberg, A. Cohen and M. Grewal, Nat. Biotechnol., 2021, 39, 1194–1199 CrossRef CAS.
- M. S. Willsey, S. R. Nason-Tomaszewski, S. R. Ensel, H. Temmar, M. J. Mender, J. T. Costello, P. G. Patil and C. A. Chestek, Nat. Commun., 2022, 13, 6899 CrossRef CAS PubMed.
- W. Heng, S. Solomon and W. Gao, Adv. Mater., 2022, 34, 2107902 CrossRef CAS PubMed.
- L. Drew, Nat. Electron., 2023, 6, 90–95 Search PubMed.
- U. Raghavendra, U. R. Acharya and H. Adeli, Eur. Neurol., 2020, 82, 41–64 CrossRef PubMed.
- N. V. Thakor, Sci. Transl. Med., 2013, 5, 210ps17 Search PubMed.
- N. Obidin, F. Tasnim and C. Dagdeviren, Adv. Mater., 2020, 32, 1901482 CrossRef CAS PubMed.
- M. M. Fouad, K. M. Amin, N. El-Bendary and A. E. Hassanien, Intell. Syst. Ref. Libr, 2015, 74, 3–30 Search PubMed.
- S. R. Patel and C. M. Lieber, Nat. Biotechnol., 2019, 37, 1007–1012 CrossRef CAS PubMed.
- M. Filippi, A. Balciunaite and R. Katzschmann, Nano Today, 2024, 54, 102094 CrossRef CAS.
- H. Acarón Ledesma, X. Li, J. L. Carvalho-de-Souza, W. Wei, F. Bezanilla and B. Tian, Nat. Nanotechnol., 2019, 14, 645–657 CrossRef PubMed.
- Y. Duan, S. Wang, Q. Yuan, Y. Shi, N. Jiang, D. Jiang, J. Song, P. Wang and L. Zhuang, Small, 2023, 19, 2205768 CrossRef CAS PubMed.
- G. T. Go, Y. Lee, D. G. Seo and T. W. Lee, Adv. Mater., 2022, 34, 2201864 CrossRef CAS PubMed.
- H. Song, M. Kim, E. Kim, J. Lee, I. Jeong, K. Lim, S. Y. Ryu, M. Oh, Y. Kim and J. Park, BMEMat, 2024, 2, e12048 CrossRef CAS.
- H. Li, J. Wang and Y. Fang, Microsyst. Nanoeng., 2023, 9, 4 CrossRef PubMed.
- A. Bansal, S. Shikha and Y. Zhang, Nat. Biomed. Eng., 2023, 7, 349–369 CrossRef PubMed.
- A. Ramos-Murguialday, D. Broetz, M. Rea, L. Läer, Ö. Yilmaz, F. L. Brasil, G. Liberati, M. R. Curado, E. Garcia-Cossio, A. Vyziotis, W. Cho, M. Agostini, E. Soares, S. Soekadar, A. Caria, L. G. Cohen and N. Birbaumer, Ann. Neurol., 2013, 74, 100–108 CrossRef.
- R. Gilron, S. Little, R. Perrone, R. Wilt, C. de Hemptinne, M. S. Yaroshinsky, C. A. Racine, S. S. Wang, J. L. Ostrem, P. S. Larson, D. D. Wang, N. B. Galifianakis, I. O. Bledsoe, M. San Luciano, H. E. Dawes, G. A. Worrell, V. Kremen, D. A. Borton, T. Denison and P. A. Starr, Nat. Biotechnol., 2021, 39, 1078–1085 CrossRef CAS PubMed.
- M. Soula, A. Martín-Ávila, Y. Zhang, A. Dhingra, N. Nitzan, M. J. Sadowski, W. B. Gan and G. Buzsáki, Nat. Neurosci., 2023, 26, 570–578 CrossRef CAS PubMed.
- E. Salari, Z. V. Freudenburg, M. P. Branco, E. J. Aarnoutse, M. J. Vansteensel and N. F. Ramsey, Sci. Rep., 2019, 9, 14165 CrossRef CAS PubMed.
- M. M. Shanechi, Nat. Neurosci., 2019, 22, 1554–1564 CrossRef CAS PubMed.
- C. F. Guimarães, L. Gasperini, A. P. Marques and R. L. Reis, Nat. Rev. Mater., 2020, 5, 351–370 CrossRef.
- D. H. Kim, J. Xiao, J. Song, Y. Huang and J. A. Rogers, Adv. Mater., 2010, 22, 2108–2124 CrossRef CAS PubMed.
- D. H. Kim, R. Ghaffari, N. Lu and J. A. Rogers, Annu. Rev. Biomed. Eng., 2012, 14, 113–128 CrossRef CAS PubMed.
- E. Song, J. Li, S. M. Won, W. Bai and J. A. Rogers, Nat. Mater., 2020, 19, 590–603 CrossRef CAS PubMed.
- R. D. Bierman-Duquette, G. Safarians, J. Huang, B. Rajput, J. Y. Chen, Z. Z. Wang and S. K. Seidlits, Adv. Healthcare Mater., 2022, 11, 2101577 CrossRef CAS PubMed.
- J. M. Anderson, A. Rodriguez and D. T. Chang, Semin. Immunol., 2008, 20, 86–100 CrossRef CAS PubMed.
- G. Voskerician, M. S. Shive, R. S. Shawgo, H. Von Recum, J. M. Anderson, M. J. Cima and R. Langer, Biomaterials, 2003, 24, 1959–1967 Search PubMed.
- G. Wick, C. Grundtman, C. Mayerl, T.-F. Wimpissinger, J. Feichtinger, B. Zelger, R. Sgonc and D. Wolfram, Annu. Rev. Immunol., 2013, 31, 107–135 CrossRef CAS PubMed.
- K. Sadtler, A. Singh, M. T. Wolf, X. Wang, D. M. Pardoll and J. H. Elisseeff, Nat. Rev. Mater., 2016, 1, 16040 CrossRef CAS.
- X. Xia, Q. Liang, X. Sun, D. Yu, X. Huang, S. M. Mugo, W. Chen, D. Wang and Q. Zhang, Adv. Funct. Mater., 2022, 32, 2208024 CrossRef CAS.
- F. Wang, Y. Xue, X. Chen, P. Zhang, L. Shan, Q. Duan, J. Xing, Y. Lan, B. Lu and J. Liu, Adv. Funct. Mater., 2024, 34, 2314471 CrossRef CAS.
- W. Lei, C. Peng, S. Chiu, H. Lu, C. Wu, T. Cheng and W. Huang, Adv. Funct. Mater., 2024, 34, 2307365 CrossRef CAS.
- W. Duan, U. A. Robles, L. Poole-Warren and D. Esrafilzadeh, Adv. Sci., 2024, 11, 2306275 CrossRef CAS PubMed.
- K. C. Spencer, J. C. Sy, K. B. Ramadi, A. M. Graybiel, R. Langer and M. J. Cima, Sci. Rep., 2017, 7, 1952 CrossRef PubMed.
- Q. Liang, Z. Shen, X. Sun, D. Yu, K. Liu, S. M. Mugo, W. Chen, D. Wang and Q. Zhang, Adv. Mater., 2023, 35, 2211159 CrossRef CAS PubMed.
- W. Wang, H. Zhou, Z. Xu, Z. Li, L. Zhang and P. Wan, Adv. Mater., 2024, 36, 2401035 CrossRef CAS.
- J. Goding, A. Gilmour, P. Martens, L. Poole-Warren and R. Green, Adv. Healthcare Mater., 2017, 6, 1601177 CrossRef PubMed.
- S. Lin, H. Yuk, T. Zhang, G. A. Parada, H. Koo, C. Yu and X. Zhao, Adv. Mater., 2016, 28, 4497–4505 CrossRef CAS.
- Z. Shen, Q. Liang, Q. Chang, Y. Liu and Q. Zhang, Adv. Mater., 2024, 36, 2310365 CrossRef CAS PubMed.
- W. Huang, X. C. Ong, I. S. Kwon, C. Gopinath, L. E. Fisher, H. Wu, G. K. Fedder, R. A. Gaunt and C. J. Bettinger, Adv. Funct. Mater., 2018, 28, 1801059 CrossRef.
- O. Wichterle and D. Lím, Nature, 1960, 185, 117–118 CrossRef.
- K. Y. Lee and D. J. Mooney, Chem. Rev., 2001, 101, 1869–1879 CrossRef CAS.
- N. A. Peppas, J. Z. Hilt, A. Khademhosseini and R. Langer, Adv. Mater., 2006, 18, 1345–1360 CrossRef CAS.
- X. Zhao, X. Chen, H. Yuk, S. Lin, X. Liu and G. Parada, Chem. Rev., 2021, 121, 4309–4372 CrossRef CAS PubMed.
- P. Calvert, Adv. Mater., 2009, 21, 743–756 CrossRef CAS.
- X. Zhao, Soft Matter, 2014, 10, 672–687 RSC.
- M. E. Freeman, M. J. Furey, B. J. Love and J. M. Hampton, Wear, 2000, 241, 129–135 CrossRef CAS.
- J. P. Gong, T. Kurokawa, T. Narita, G. Kagata, Y. Osada, G. Nishimura and M. Kinjo, J. Am. Chem. Soc., 2001, 123, 5582–5583 CrossRef CAS PubMed.
- Y. Yu, H. Yuk, G. A. Parada, Y. Wu, X. Liu, C. S. Nabzdyk, K. Youcef-Toumi, J. Zang and X. Zhao, Adv. Mater., 2019, 31, 1807101 CrossRef PubMed.
- G. Parada, Y. Yu, W. Riley, S. Lojovich, D. Tshikudi, Q. Ling, Y. Zhang, J. Wang, L. Ling, Y. Yang, S. Nadkarni, C. Nabzdyk and X. Zhao, Adv. Healthcare Mater., 2020, 9, 2001116 Search PubMed.
- A. J. Vegas, O. Veiseh, J. C. Doloff, M. Ma, H. H. Tam, K. Bratlie, J. Li, A. R. Bader, E. Langan, K. Olejnik, P. Fenton, J. W. Kang, J. Hollister-Locke, M. A. Bochenek, A. Chiu, S. Siebert, K. Tang, S. Jhunjhunwala, S. Aresta-Dasilva, N. Dholakia, R. Thakrar, T. Vietti, M. Chen, J. Cohen, K. Siniakowicz, M. Qi, J. McGarrigle, S. Lyle, D. M. Harlan, D. L. Greiner, J. Oberholzer, G. C. Weir, R. Langer and D. G. Anderson, Nat. Biotechnol., 2016, 34, 345–352 CrossRef CAS PubMed.
- M. A. Bochenek, O. Veiseh, A. J. Vegas, J. J. McGarrigle, M. Qi, E. Marchese, M. Omami, J. C. Doloff, J. Mendoza-Elias, M. Nourmohammadzadeh, A. Khan, C.-C. Yeh, Y. Xing, D. Isa, S. Ghani, J. Li, C. Landry, A. R. Bader, K. Olejnik, M. Chen, J. Hollister-Lock, Y. Wang, D. L. Greiner, G. C. Weir, B. L. Strand, A. M. A. Rokstad, I. Lacik, R. Langer, D. G. Anderson and J. Oberholzer, Nat. Biomed. Eng., 2018, 2, 810–821 CrossRef CAS PubMed.
- Y. Liu, J. Li, S. Song, J. Kang, Y. Tsao, S. Chen, V. Mottini, K. McConnell, W. Xu, Y. Q. Zheng, J. B. H. Tok, P. M. George and Z. Bao, Nat. Biotechnol., 2020, 38, 1031–1036 CrossRef CAS PubMed.
- M. S. Okun, N. Engl. J. Med., 2012, 367, 1529–1538 CrossRef CAS PubMed.
- D. Farina, I. Vujaklija, M. Sartori, T. Kapelner, F. Negro, N. Jiang, K. Bergmeister, A. Andalib, J. Principe and O. C. Aszmann, Nat. Biomed. Eng., 2017, 1, 0025 Search PubMed.
- M. OrtizCatalan, E. Mastinu, P. Sassu, O. Aszmann and R. Brånemark, N. Engl. J. Med., 2020, 382, 1732–1738 Search PubMed.
- S. Waldert, Front. Neurosci., 2016, 10, 295 Search PubMed.
- Y. Zhao, B. Zhang, B. Yao, Y. Qiu, Z. Peng, Y. Zhang, Y. Alsaid, I. Frenkel, K. Youssef, Q. Pei and X. He, Matter, 2020, 3, 1196–1210 CrossRef.
- J. Nam, H. K. Lim, N. H. Kim, J. K. Park, E. S. Kang, Y. T. Kim, C. Heo, O. S. Lee, S. G. Kim, W. S. Yun, M. Suh and Y. H. Kim, ACS Nano, 2020, 14, 664–675 CrossRef CAS PubMed.
- Y. Zhou, C. Wan, Y. Yang, H. Yang, S. Wang, Z. Dai, K. Ji, H. Jiang, X. Chen and Y. Long, Adv. Funct. Mater., 2019, 29, 1806220 CrossRef.
- J. Park, J. Jeon, B. Kim, M. S. Lee, S. Park, J. Lim, J. Yi, H. Lee, H. S. Yang and J. Y. Lee, Adv. Funct. Mater., 2020, 30, 2003759 CrossRef CAS.
- C. Rinoldi, Y. Ziai, S. S. Zargarian, P. Nakielski, K. Zembrzycki, M. A. Haghighat Bayan, A. B. Zakrzewska, R. Fiorelli, M. Lanzi, A. Kostrzewska-Księżyk, R. Czajkowski, E. Kublik, L. Kaczmarek and F. Pierini, ACS Appl. Mater. Interfaces, 2023, 15, 6283–6296 CrossRef CAS PubMed.
- H. He, H. Li, A. Pu, W. Li, K. Ban and L. Xu, Nat. Commun., 2023, 14, 759 CrossRef CAS PubMed.
- G. Li, K. Huang, J. Deng, M. Guo, M. Cai, Y. Zhang and C. F. Guo, Adv. Mater., 2022, 34, 2200261 CrossRef CAS PubMed.
- Z. Wang, X. Xu, R. Tan, S. Zhang, K. Zhang and J. Hu, Adv. Funct. Mater., 2024, 34, 2312667 CrossRef CAS.
- Q. Zhang, H. Lu, G. Yun, L. Gong, Z. Chen, S. Jin, H. Du, Z. Jiang and W. Li, Adv. Funct. Mater., 2024, 34, 2308113 CrossRef CAS.
- M. Javadi, Q. Gu, S. Naficy, S. Farajikhah, J. M. Crook, G. G. Wallace, S. Beirne and S. E. Moulton, Macromol. Biosci., 2018, 18, 1700270 CrossRef PubMed.
- K. Qiao, S. Guo, Y. Zheng, X. Xu, H. Meng, J. Peng, Z. Fang and Y. Xie, Mater. Sci. Eng., C, 2018, 93, 853–863 CrossRef CAS PubMed.
- T. Zhou, H. Yuk, F. Hu, J. Wu, F. Tian, H. Roh, Z. Shen, G. Gu, J. Xu, B. Lu and X. Zhao, Nat. Mater., 2023, 22, 895–902 CrossRef CAS PubMed.
- Y. Liu, J. Liu, S. Chen, T. Lei, Y. Kim, S. Niu, H. Wang, X. Wang, A. M. Foudeh, J. B. H. Tok and Z. Bao, Nat. Biomed. Eng., 2019, 3, 58–68 CrossRef CAS PubMed.
- B. Yao, H. Wang, Q. Zhou, M. Wu, M. Zhang, C. Li and G. Shi, Adv. Mater., 2017, 29, 1700974 CrossRef PubMed.
- B. Lu, H. Yuk, S. Lin, N. Jian, K. Qu, J. Xu and X. Zhao, Nat. Commun., 2019, 10, 1043 CrossRef PubMed.
- S. J. K. O’Neill, Z. Huang, M. H. Ahmed, A. J. Boys, S. Velasco-Bosom, J. Li, R. M. Owens, J. A. McCune, G. G. Malliaras and O. A. Scherman, Adv. Mater., 2023, 35, 2207634 CrossRef PubMed.
- S. Sun, M. Xu, Y. Zhao, T. Cheng, Y. Xiang, X. Liu, J. Wang and R. Pei, ACS Appl. Nano Mater., 2023, 6, 21226–21235 CrossRef CAS.
- N. Li, Q. Yu, S. Duan, Y. Du, X. Shi, X. Li, T. Jiao, Z. Qin and X. He, Adv. Funct. Mater., 2024, 34, 2309500 CrossRef CAS.
- T. Dvir, B. P. Timko, M. D. Brigham, S. R. Naik, S. S. Karajanagi, O. Levy, H. Jin, K. K. Parker, R. Langer and D. S. Kohane, Nat. Nanotechnol., 2011, 6, 720–725 CrossRef CAS PubMed.
- Y. Ahn, H. Lee, D. Lee and Y. Lee, ACS Appl. Mater. Interfaces, 2014, 6, 18401–18407 CrossRef CAS PubMed.
- H. S. Song, O. S. Kwon, J.-H. Kim, J. Conde and N. Artzi, Biosens. Bioelectron., 2017, 89, 187–200 CrossRef CAS PubMed.
- S. R. Shin, S. M. Jung, M. Zalabany, K. Kim, P. Zorlutuna, S. B. Kim, M. Nikkhah, M. Khabiry, M. Azize, J. Kong, K.-T. Wan, T. Palacios, M. R. Dokmeci, H. Bae, X. Tang and A. Khademhosseini, ACS Nano, 2013, 7, 2369–2380 CrossRef CAS PubMed.
- J. Wang, Q. Li, K. Li, X. Sun, Y. Wang, T. Zhuang, J. Yan and H. Wang, Adv. Mater., 2022, 34, 2109904 CrossRef CAS PubMed.
- U. A. Aregueta-Robles, A. J. Woolley, L. A. Poole-Warren, N. H. Lovell and R. A. Green, Front. Neuroeng., 2014, 7, 15 CAS.
- J. Zhang, J. Liao, Z. Liu, R. Zhang and M. Sitti, Adv. Funct. Mater., 2023, 34, 2308238 CrossRef.
- K. Park, S. Park, Y. Jo, S. A. Kim, T. Y. Kim, S. Kim and J. Seo, Ind. Chem. Mater., 2024, 2, 361–377 RSC.
- Y. Zhao, Y. Ohm, J. Liao, Y. Luo, H. Y. Cheng, P. Won, P. Roberts, M. R. Carneiro, M. F. Islam, J. H. Ahn, L. M. Walker and C. Majidi, Nat. Electron., 2023, 6, 206–215 CrossRef CAS.
- S. Chen, H.-Z. Wang, R.-Q. Zhao, W. Rao and J. Liu, Matter, 2020, 2, 1446–1480 CrossRef.
- M. Liao, H. Liao, J. Ye, P. Wan and L. Zhang, ACS Appl. Mater. Interfaces, 2019, 11, 47358–47364 CrossRef CAS PubMed.
- Y. Xu, R. Rothe, D. Voigt, S. Hauser, M. Cui, T. Miyagawa, M. Patino Gaillez, T. Kurth, M. Bornhäuser, J. Pietzsch and Y. Zhang, Nat. Commun., 2021, 12, 2407 CrossRef CAS PubMed.
- X. Wang, J. Zhou, Y. Zhu, W. Cheng, D. Zhao, G. Xu and H. Yu, Chem. Eng. J., 2020, 392, 123644 Search PubMed.
- H. Peng, Y. Xin, J. Xu, H. Liu and J. Zhang, Mater. Horiz., 2019, 6, 618–625 RSC.
- M. C. McNamara, A. E. Niaraki-Asli, J. Guo, J. Okuzono, R. Montazami and N. N. Hashemi, Front. Mater., 2020, 7, 500340 Search PubMed.
- S. Yao, Y. Yang, C. Li, K. Yang, X. Song, C. Li, Z. Cao, H. Zhao, X. Yu, X. Wang and L.-N. Wang, Bioact. Mater., 2024, 35, 534–548 CAS.
- C. Cha, S. R. Shin, N. Annabi, M. R. Dokmeci and A. Khademhosseini, ACS Nano, 2013, 7, 2891–2897 CrossRef CAS PubMed.
- K. S. Novoselov, V. I. FalKo, L. Colombo, P. R. Gellert, M. G. Schwab and K. Kim, Nature, 2012, 490, 192–200 Search PubMed.
- N. O. Weiss, H. Zhou, L. Liao, Y. Liu, S. Jiang, Y. Huang and X. Duan, Adv. Mater., 2012, 24, 5782–5825 Search PubMed.
- L. He, D. Lin, Y. Wang, Y. Xiao and J. Che, Colloids Surf., B, 2011, 87, 273–279 Search PubMed.
- A. Vashist, A. Kaushik, A. Vashist, V. Sagar, A. Ghosal, Y. K. Gupta, S. Ahmad and M. Nair, Adv. Healthcare Mater., 2018, 7, 1701213 CrossRef PubMed.
- E. W. Keefer, B. R. Botterman, M. I. Romero, A. F. Rossi and G. W. Gross, Nat. Nanotechnol., 2008, 3, 434–439 CrossRef CAS PubMed.
- X. Li, T. Zhao, Q. Chen, P. Li, K. Wang, M. Zhong, J. Wei, D. Wu, B. Wei and H. Zhu, Phys. Chem. Chem. Phys., 2013, 15, 17752 RSC.
- C. M. Proctor, J. Rivnay and G. G. Malliaras, J. Polym. Sci., Part B: Polym. Phys., 2016, 54, 1433–1436 CrossRef CAS.
- B. D. Paulsen, K. Tybrandt, E. Stavrinidou and J. Rivnay, Nat. Mater., 2020, 19, 13–26 CrossRef CAS PubMed.
- J. Hong, Z. Zhu, Z. Wang, J. Li, Z. Liu, R. Tan, Y. Hao and G. Cheng, Adv. Healthcare Mater., 2024, 13, 2400624 CrossRef CAS PubMed.
- R. Feiner and T. Dvir, Nat. Rev. Mater., 2017, 3, 17076 Search PubMed.
- S. Lacour, S. P. Lacour, G. Courtine, G. Courtine, J. Guck and J. Guck, Nat. Rev. Mater., 2016, 1, 16063 Search PubMed.
- J. W. Jeong, J.-W. Jeong, G. Shin, G. Shin, G. Shin, S. I. Park, S. I. Park, K. J. Yu, K. J. Yu, L. Xu, L. Xu, J. A. Rogers and J. A. Rogers, Neuron, 2015, 86, 175–186 CrossRef CAS PubMed.
- M. Hu, J. Ren and Y. Pan, Adv. Funct. Mater., 2024, 34, 2407926 Search PubMed.
- J. P. Gong, Y. Katsuyama, T. Kurokawa and Y. Osada, Adv. Mater., 2003, 15, 1155–1158 Search PubMed.
- W. Zhang, R. Wang, Z. Sun, X. Zhu, Q. Zhao, T. Zhang, A. Cholewinski, F. (Kuo) Yang, B. Zhao, R. Pinnaratip, P. K. Forooshani and B. P. Lee, Chem. Soc. Rev., 2020, 49, 433–464 Search PubMed.
- J. Kim, G. Zhang, M. Shi and Z. Suo, Science, 2021, 374, 212–216 CrossRef CAS PubMed.
- G. He, H. Lei, W. Sun, J. Gu, W. Yu, D. Zhang, H. Chen, Y. Li, M. Qin, B. Xue, W. Wang and Y. Cao, Angew. Chem., Int. Ed., 2022, 61, e202201765 CrossRef CAS PubMed.
- A. Bin Imran, K. Esaki, H. Gotoh, T. Seki, K. Ito, Y. Sakai and Y. Takeoka, Nat. Commun., 2014, 5, 5124 CrossRef CAS PubMed.
- Q. Liang, X. Xia, X. Sun, D. Yu, X. Huang, G. Han, S. M. Mugo, W. Chen and Q. Zhang, Adv. Sci., 2022, 9, 2201059 CrossRef CAS PubMed.
- N. Annabi, S. R. Shin, A. Tamayol, M. Miscuglio, M. A. Bakooshli, A. Assmann, P. Mostafalu, J.-Y. Sun, S. Mithieux, L. Cheung, X. S. Tang, A. S. Weiss and A. Khademhosseini, Adv. Mater., 2016, 28, 40–49 CrossRef CAS PubMed.
- X. Xia, Q. Liang, X. Sun, D. Yu, X. Huang, S. M. Mugo, W. Chen, D. Wang and Q. Zhang, Adv. Funct. Mater., 2022, 32, 2208024 CrossRef CAS.
- Y. Li, Q. Cheng, Z. Deng, T. Zhang, M. Luo, X. Huang, Y. Wang, W. Wang and X. Zhao, Polym., 2024, 16, 971 CAS.
- S. Pan, C. Xiang, Z. Liu, G. Tong, C. Zhang and X. Zhu, J. Mater. Chem. C, 2024, 12, 8813–8824 RSC.
- Z. Zhao, X. Qin, L. Cao, J. Li and Y. Wei, Int J. Bio. Macro., 2022, 212, 123–133 CrossRef CAS PubMed.
- S. Zhang, F. Guo, X. Gao, M. Yang, X. Huang, D. Zhang, X. Li, Y. Zhang, Y. Shang and A. Cao, Adv. Sci., 2024, 11, 2405880 Search PubMed.
- X. Cheng, Z. Zhang, H. Ren, Z. Zou, Y. Zhang, Y. Qu, X. Chen, J. Zhao and C. He, Nat. Sci. Rev., 2024, 11, nwae160 CrossRef PubMed.
- Z. Zhang, A. Yao and P. Raffa, Adv. Funct. Mater., 2024, 34, 2407529 CrossRef CAS.
- C. M. Tringides, N. Vachicouras, I. De Lázaro, H. Wang, A. Trouillet, B. R. Seo, A. Elosegui-Artola, F. Fallegger, Y. Shin, C. Casiraghi, K. Kostarelos, S. P. Lacour and D. J. Mooney, Nat. Nanotechnol., 2021, 16, 1019–1029 CrossRef CAS PubMed.
- L. Teng, Z. Shao, Q. Bai, X. Zhang, Y. He, J. Lu, D. Zou, C. Feng and C. Dong, Adv. Funct. Mater., 2021, 31, 2105628 CrossRef CAS.
- Y. Cong and J. Fu, Langmuir, 2022, 38, 11503–11513 CrossRef CAS PubMed.
- H. Yuk, C. E. Varela, C. S. Nabzdyk, X. Mao, R. F. Padera, E. T. Roche and X. Zhao, Nature, 2019, 575, 169–174 Search PubMed.
- Q. Yang, T. Wei, R. T. Yin, M. Wu, Y. Xu, J. Koo, Y. S. Choi, Z. Xie, S. W. Chen, I. Kandela, S. Yao, Y. Deng, R. Avila, T. L. Liu, W. Bai, Y. Yang, M. Han, Q. Zhang, C. R. Haney, K. Benjamin Lee, K. Aras, T. Wang, M. H. Seo, H. Luan, S. M. Lee, A. Brikha, N. Ghoreishi-Haack, L. Tran, I. Stepien, F. Aird, E. A. Waters, X. Yu, A. Banks, G. D. Trachiotis, J. M. Torkelson, Y. Huang, Y. Kozorovitskiy, I. R. Efimov and J. A. Rogers, Nat. Mater., 2021, 20, 1559–1570 CrossRef CAS PubMed.
- B. Xue, J. Gu, L. Li, W. Yu, S. Yin, M. Qin, Q. Jiang, W. Wang and Y. Cao, Nat. Commun., 2021, 12, 7156 CrossRef CAS PubMed.
- J. Deng, H. Yuk, J. Wu, C. E. Varela, X. Chen, E. T. Roche, C. F. Guo and X. Zhao, Nat. Mater., 2021, 20, 229–236 Search PubMed.
- G. Tian, D. Yang, C. Liang, Y. Liu, J. Chen, Q. Zhao, S. Tang, J. Huang, P. Xu, Z. Liu and D. Qi, Adv. Mater., 2023, 35, 2212302 CrossRef CAS PubMed.
- M. Choi, J. W. Choi, S. Kim, S. Nizamoglu, S. K. Hahn and S. H. Yun, Nat. Photonics, 2013, 7, 987–994 CrossRef CAS PubMed.
- J. Guo, X. Liu, N. Jiang, A. K. Yetisen, H. Yuk, C. Yang, A. Khademhosseini, X. Zhao and S.-H. Yun, Adv. Mater., 2016, 28, 10244–10249 CrossRef CAS PubMed.
- M. Choi, M. Humar, S. Kim and S.-H. Yun, Adv. Mater., 2015, 27, 4081–4086 Search PubMed.
- T. Zhou, Z. Qiao, M. Yang, K. Wu, N. Xin, J. Xiao, X. Liu, C. Wu, D. Wei, J. Sun and H. Fan, Biosens. Bioelectron., 2023, 231, 115288 CrossRef CAS PubMed.
- G. K. Tummala, T. Joffre, R. Rojas, C. Persson and A. Mihranyan, Soft Matter, 2017, 13, 3936–3945 RSC.
- L. Han, L. Yan, M. Wang, K. Wang, L. Fang, J. Zhou, J. Fang, F. Ren and X. Lu, Chem. Mater., 2018, 30, 5561–5572 CrossRef CAS.
- Y. Cui, F. Zhang, G. Chen, L. Yao, N. Zhang, Z. Liu, Q. Li, F. Zhang, Z. Cui, K. Zhang, P. Li, Y. Cheng, S. Zhang and X. Chen, Adv. Mater., 2021, 33, 2100221 CrossRef CAS PubMed.
- L. Li, Z. Ai, J. Wu, Z. Lin, M. Huang, Y. Gao and H. Bai, Nat. Commun., 2024, 15, 6591 CrossRef CAS PubMed.
- L. Yang, Y. Zhang, W. Cai, J. Tan, H. Hansen, H. Wang, Y. Chen, M. Zhu and J. Mu, Chem. Soc. Rev., 2024, 53, 5956–6010 RSC.
- J. K. Wychowaniec, H. Saini, B. Scheibe, D. P. Dubal, A. Schneemann and K. Jayaramulu, Chem. Soc. Rev., 2022, 51, 9068–9126 RSC.
- R. Foudazi, R. Zowada, I. Manas-Zloczower and D. L. Feke, Langmuir, 2023, 39, 2092–2111 CrossRef CAS PubMed.
- Y. Yuan, Q. Zhang, S. Lin and J. Li, Prog. Mater. Sci., 2025, 148, 101378 CrossRef CAS.
- Y. Alsaid, S. Wu, D. Wu, Y. Du, L. Shi, R. Khodambashi, R. Rico, M. Hua, Y. Yan, Y. Zhao, D. Aukes and X. He, Adv. Mater., 2021, 33, 2008235 CrossRef CAS PubMed.
- B. Ahmad, E. Stride and M. Edirisinghe, Food Biopr. Technol., 2012, 5, 2848–2857 Search PubMed.
- N. Huebsch, E. Lippens, K. Lee, M. Mehta, S. T. Koshy, M. C. Darnell, R. M. Desai, C. M. Madl, M. Xu, X. Zhao, O. Chaudhuri, C. Verbeke, W. S. Kim, K. Alim, A. Mammoto, D. E. Ingber, G. N. Duda and D. J. Mooney, Nat. Mater., 2015, 14, 1269–1277 CrossRef CAS PubMed.
- M. V. Badiger, M. E. McNeill and N. B. Graham, Biomaterials, 1993, 14, 1059–1063 CrossRef CAS PubMed.
- S. A. Bencherif, R. W. Sands, O. A. Ali, W. A. Li, S. A. Lewin, T. M. Braschler, T.-Y. Shih, C. S. Verbeke, D. Bhatta, G. Dranoff and D. J. Mooney, Nat. Commun., 2015, 6, 7556 CrossRef CAS PubMed.
- S. Van Vlierberghe, V. Cnudde, P. Dubruel, B. Masschaele, A. Cosijns, I. De Paepe, P. J. S. Jacobs, L. Van Hoorebeke, J. P. Remon and E. Schacht, Biomaterials, 2007, 8, 331–337 CAS.
- P. Eiselt, J. Yeh, R. K. Latvala, L. D. Shea and D. J. Mooney, Biomaterials, 2000, 21, 1921–1927 CrossRef CAS PubMed.
- J. Chen, H. Park and K. Park, J. Biomed. Mater. Res., 1999, 44, 53–62 CrossRef CAS PubMed.
- N. Broguiere, A. Husch, G. Palazzolo, F. Bradke, S. Madduri and M. Zenobi-Wong, Biomaterials, 2019, 200, 56–65 CrossRef CAS PubMed.
- Y. Guo, Y. Han, Y. Cao, Y. Chen, J. Xie, H. Ding, S. Liang, X. Liu, W. Sun, J. Tang, S. Shao, J. Xiang and Y. Shen, Adv. Funct. Mater., 2025, 35, 2412412 CrossRef CAS.
- H. Yuan, X. Zhao, W. Zhao, Y. Zhang, Q. Ye, S. Liu, B. Yu, S. Ma and F. Zhou, Adv. Funct. Mater., 2024, 2416898 CrossRef.
- H. He, H. Li, A. Pu, W. Li, K. Ban and L. Xu, Nat. Commun., 2023, 14, 759 CrossRef CAS PubMed.
- Y. Dai, S. Wai, P. Li, N. Shan, Z. Cao, Y. Li, Y. Wang, Y. Liu, W. Liu, K. Tang, Y. Liu, M. Hua, S. Li, N. Li, S. Chatterji, H. C. Fry, S. Lee, C. Zhang, M. Weires, S. Sutyak, J. Shi, C. Zhu, J. Xu, X. Gu, B. Tian and S. Wang, Science, 2024, 386, 431–439 CrossRef CAS PubMed.
- W. Xue, D. Lee, Y. Kong, M. Kuss, Y. Huang, T. Kim, S. Chung, A. T. Dudley, S. Ro and B. Duan, Adv. Funct. Mater., 2023, 33, 2214129 CrossRef CAS PubMed.
- Q. He, Y. Cheng, Y. Deng, F. Wen, Y. Lai and H. Li, Adv. Funct. Mater., 2024, 34, 2308974 CrossRef CAS.
- F. Fu, J. Wang, H. Zeng and J. Yu, ACS Mater. Lett., 2020, 2, 1287–1301 CrossRef CAS.
- Y. Xu, W. Zhu, J. Wu, L. Liu, L. Yue, X. Zhang, J. Li, P. She, J. Yang, C. Sun, X. Xu and L. Rao, Adv. Funct. Mater., 2024, 34, 2400507 CrossRef CAS.
- B. A. Nerger, K. Kashyap, B. T. Deveney, J. Lou, B. F. Hanan, Q. Liu, A. Khalil, T. Lungjangwa, M. Cheriyan, A. Gupta, R. Jaenisch, D. A. Weitz, L. Mahadevan and D. J. Mooney, Proc. Natl. Acad. Sci. U. S. A., 2024, 121, e2410806121 CrossRef CAS PubMed.
- S. SenGupta, C. A. Parent and J. E. Bear, Nat. Rev. Mol. Cell Biol., 2021, 22, 529–547 CrossRef CAS PubMed.
- A. A. Solbu, D. Caballero, S. Damigos, S. C. Kundu, R. L. Reis, Ø. Halaas, A. S. Chahal and B. L. Strand, Mater. Today Bio., 2023, 18, 100537 CrossRef CAS PubMed.
- S. Lu, X. Wang, W. Li, Y. Zu and J. Xiao, Adv. Funct. Mater., 2023, 33, 2303368 CrossRef CAS.
- K. M. Yamada and M. Sixt, Nat. Rev. Mol. Cell Biol., 2019, 20, 738–752 CrossRef CAS PubMed.
- A. Adak, S. Ghosh, V. Gupta and S. Ghosh, Biomacromolecules, 2019, 20, 1889–1898 CrossRef CAS PubMed.
- J. Zhang, L. Wang, Y. Xue, I. M. Lei, X. Chen, P. Zhang, C. Cai, X. Liang, Y. Lu and J. Liu, Adv. Mater., 2023, 35, 2209324 CrossRef CAS PubMed.
- J. Rivnay, H. Wang, L. Fenno, K. Deisseroth and G. G. Malliaras, Sci. Adv., 2017, 3, e1601649 CrossRef PubMed.
- K. Liu, H. Zhang, M. Hu, Z. Li, K. Xu, D. Chen, W. Cui, C. Lv, R. Ding, X. Geng and S. Wei, Mater. Adv., 2024, 5, 4958–4973 RSC.
- N. R. Provenza, S. A. Sheth, E. M. Dastin-van Rijn, R. K. Mathura, Y. Ding, G. S. Vogt, M. Avendano-Ortega, N. Ramakrishnan, N. Peled, L. F. F. Gelin, D. Xing, L. A. Jeni, I. O. Ertugrul, A. Barrios-Anderson, E. Matteson, A. D. Wiese, J. Xu, A. Viswanathan, M. T. Harrison, K. R. Bijanki, E. A. Storch, J. F. Cohn, W. K. Goodman and D. A. Borton, Nat. Med., 2021, 27, 2154–2164 CrossRef CAS PubMed.
- S. M. Wellman, J. R. Eles, K. A. Ludwig, J. P. Seymour, N. J. Michelson, W. E. McFadden, A. L. Vazquez and T. D. Y. Kozai, Adv. Funct. Mater., 2018, 28, 1701269 CrossRef PubMed.
- M. E. J. Obien, K. Deligkaris, T. Bullmann, D. J. Bakkum and U. Frey, Front. Neurosci., 2015, 8, 423 Search PubMed.
- Z. M. Khan, E. Wilts, E. Vlaisavljevich, T. E. Long and S. S. Verbridge, Macromol. Biosci., 2022, 22, 2100355 CrossRef CAS PubMed.
- J. J. Jun, N. A. Steinmetz, J. H. Siegle, D. J. Denman, M. Bauza, B. Barbarits, A. K. Lee, C. A. Anastassiou, A. Andrei, Ç. Aydın, M. Barbic, T. J. Blanche, V. Bonin, J. Couto, B. Dutta, S. L. Gratiy, D. A. Gutnisky, M. Häusser, B. Karsh, P. Ledochowitsch, C. M. Lopez, C. Mitelut, S. Musa, M. Okun, M. Pachitariu, J. Putzeys, P. D. Rich, C. Rossant, W. Sun, K. Svoboda, M. Carandini, K. D. Harris, C. Koch, J. O’Keefe and T. D. Harris, Nature, 2017, 551, 232–236 CrossRef CAS PubMed.
- S. Park, H. Yuk, R. Zhao, Y. S. Yim, E. W. Woldeghebriel, J. Kang, A. Canales, Y. Fink, G. B. Choi, X. Zhao and P. Anikeeva, Nat. Commun., 2021, 12, 3435 Search PubMed.
- C. Tang, Z. Han, Z. Liu, W. Li, J. Shen, K. Zhang, S. Mai, J. Li, X. Sun, X. Chen, H. Li, L. Wang, J. Liang, M. Liao, J. Feng, C. Wang, J. Wang, L. Ye, Y. Yang, S. Xie, X. Shi, K. Zeng, X. Zhang, X. Cheng, K. Zhang, Y. Guo, H. Yang, Y. Xu, Q. Tong, H. Yu, P. Chen, H. Peng and X. Sun, Adv. Mater., 2024, 36, 2407874 CrossRef CAS PubMed.
- D. Won, J. Kim, J. Choi, H. Kim, S. Han, I. Ha, J. Bang, K. K. Kim, Y. Lee, T.-S. Kim, J.-H. Park, C.-Y. Kim and S. H. Ko, Sci. Adv., 2022, 8, eabo3209 CrossRef CAS PubMed.
- D. Won, H. J. Kim, J. Kim, H. Kim, M. W. Kim, J. Ahn, K. Min, Y. Lee, S. Hong, J. Choi, C. Y. Kim, T. S. Kim and S. H. Ko, Nat. Electron., 2024, 7, 475–486 CrossRef CAS.
- B. Pesaran, M. Vinck, G. T. Einevoll, A. Sirota, P. Fries, M. Siegel, W. Truccolo, C. E. Schroeder and R. Srinivasan, Nat. Neurosci., 2018, 21, 903–919 Search PubMed.
- G. Buzsaki and A. Draguhn, Science, 2004, 304, 1926–1929 CrossRef CAS PubMed.
- G. Buzsáki, C. A. Anastassiou and C. Koch, Nat. Rev. Neurosci., 2012, 13, 407–420 CrossRef PubMed.
-
J. Malmivuo and R. Plonsey, Bioelectromagnetism: Principles and Applications of Bioelectric and Biomagnetic Fields, Oxford University Press, London, 1995 Search PubMed.
- J. R. Greco and J. W. Clark, IEEE Trans. Biomed. Eng., 1977, BME-24, 18–23 Search PubMed.
- P. C. Miranda, A. Mekonnen, R. Salvador and G. Ruffini, NeuroImage, 2013, 70, 48–58 CrossRef PubMed.
- H. Sheng, X. Wang, N. Kong, W. Xi, H. Yang, X. Wu, K. Wu, C. Li, J. Hu, J. Tang, J. Zhou, S. Duan, H. Wang and Z. Suo, Extreme Mech. Lett., 2019, 30, 100510 CrossRef.
- H. Yuk, B. Lu, S. Lin, K. Qu, J. Xu, J. Luo and X. Zhao, Nat. Commun., 2020, 11, 1604 CrossRef CAS PubMed.
- X. Wang, X. Sun, D. Gan, M. Soubrier, H.-Y. Chiang, L. Yan, Y. Li, J. Li, S. Yu, Y. Xia, K. Wang, Q. Qin, X. Jiang, L. Han, T. Pan, C. Xie and X. Lu, Matter, 2022, 5, 1204–1223 CrossRef CAS.
- E. Sivanesan, R. B. North, M. A. Russo, R. M. Levy, B. Linderoth, S. M. Hayek, S. Eldabe and S. F. Lempka, Neuromodulation Technol. Neural Interface, 2024, 27, 1–12 CrossRef PubMed.
- F. B. Wagner, J.-B. Mignardot, C. G. Le Goff-Mignardot, R. Demesmaeker, S. Komi, M. Capogrosso, A. Rowald, I. Seáñez, M. Caban, E. Pirondini, M. Vat, L. A. McCracken, R. Heimgartner, I. Fodor, A. Watrin, P. Seguin, E. Paoles, K. Van Den Keybus, G. Eberle, B. Schurch, E. Pralong, F. Becce, J. Prior, N. Buse, R. Buschman, E. Neufeld, N. Kuster, S. Carda, J. von Zitzewitz, V. Delattre, T. Denison, H. Lambert, K. Minassian, J. Bloch and G. Courtine, Nature, 2018, 563, 65–71 CrossRef CAS PubMed.
- B. L. Roth, Neuron, 2016, 89, 683–694 CrossRef CAS PubMed.
- W. Legon, T. F. Sato, A. Opitz, J. Mueller, A. Barbour, A. Williams and W. J. Tyler, Nat. Neurosci., 2014, 17, 322–329 Search PubMed.
- A. Sadeghi, E. Afshari, M. Hashemi, D. Kaplan and M. Mozafari, Curr. Opin. Biomed. Eng., 2023, 25, 100420 Search PubMed.
- Translating neuromodulation, Nat. Biotechnol., 2019, 37, 967 Search PubMed.
- L. Wang, S. Liu, W. Zhao, J. Li, H. Zeng, S. Kang, X. Sheng, L. Wang, Y. Fan and L. Yin, Adv. Healthcare Mater., 2024, 13, 2303316 CrossRef CAS PubMed.
- S. M. Won, L. Cai, P. Gutruf and J. A. Rogers, Nat. Biomed. Eng., 2023, 7, 405–423 Search PubMed.
- S. M. Won, E. Song, J. T. Reeder and J. A. Rogers, Cell, 2020, 181, 115–135 CrossRef CAS PubMed.
- X. Yang, E. McGlynn, R. Das, S. P. Paşca, B. Cui and H. Heidari, Adv. Mater., 2021, 33, 2103208 CrossRef CAS PubMed.
- R. Chen, A. Canales and P. Anikeeva, Nat. Rev. Mater., 2017, 2, 16093 CrossRef CAS PubMed.
- A. Vázquez-Guardado, Y. Yang, A. J. Bandodkar and J. A. Rogers, Nat. Neurosci., 2020, 23, 1522–1536 CrossRef PubMed.
- Q. Zeng and Z. Huang, Adv. Funct. Mater., 2023, 33, 2301223 CrossRef CAS.
- S. Cheng, R. Zhu and X. Xu, Commun. Mater., 2024, 5, 99 CrossRef CAS.
- H. Chen and Y. Fang, MRS Bull., 2023, 48, 484–494 CrossRef.
- A. Erofeev, O. Zakharova, S. Terekhin, P. Plotnikova, I. Bezprozvanny and O. Vlasova, Opera Med. Physiol., 2016, 2, 117–121 Search PubMed.
- L. A. Gunaydin, O. Yizhar, A. Berndt, V. S. Sohal, K. Deisseroth and P. Hegemann, Nat. Neurosci., 2010, 13, 387–392 CrossRef CAS PubMed.
- M. Scanziani and M. Häusser, Nature, 2009, 461, 930–939 CrossRef CAS PubMed.
- F. Zhang, L. P. Wang, M. Brauner, J. F. Liewald, K. Kay, N. Watzke, P. G. Wood, E. Bamberg, G. Nagel, A. Gottschalk and K. Deisseroth, Nature, 2007, 446, 633–639 CrossRef CAS PubMed.
- K. M. Tye and K. Deisseroth, Nat. Rev. Neurosci., 2012, 13, 251–266 CrossRef CAS PubMed.
- M. Häusser, Nat. Methods, 2014, 11, 1012–1014 CrossRef PubMed.
- M. Yan, L. Wang, Y. Wu, L. Wang and Y. Lu, Acta Biomater., 2023, 157, 252–262 CrossRef CAS PubMed.
- W. C. Huang, H. S. Chi, Y. C. Lee, Y. C. Lo, T. C. Liu, M. Y. Chiang, H. Y. Chen, S. J. Li, Y. Y. Chen and S. Y. Chen, ACS Appl. Mater. Interfaces, 2019, 11, 11270–11282 CrossRef CAS PubMed.
- L. Wang, C. Zhong, D. Ke, F. Ye, J. Tu, L. Wang and Y. Lu, Adv. Opt. Mater., 2018, 6, 1800427 CrossRef.
- G. Chen, G. Wang, X. Tan, K. Hou, Q. Meng, P. Zhao, S. Wang, J. Zhang, Z. Zhou, T. Chen, Y. Cheng, B. S. Hsiao, E. Reichmanis and M. Zhu, Natl. Sci. Rev., 2021, 8, nwaa209 CrossRef CAS PubMed.
- A. Tabet, M. J. Antonini, A. Sahasrabudhe, J. Park, D. Rosenfeld, F. Koehler, H. Yuk, S. Hanson, J. Stinson, M. Stok, X. Zhao, C. Wang and P. Anikeeva, ACS Cent. Sci., 2021, 7, 1516–1523 CrossRef CAS PubMed.
- S. Huang, X. Liu, S. Lin, C. Glynn, K. Felix, A. Sahasrabudhe, C. Maley, J. Xu, W. Chen, E. Hong, A. J. Crosby, Q. Wang and S. Rao, Nat. Commun., 2024, 15, 3525 CrossRef CAS PubMed.
- J. Wang, H. Wang, X. Mo and H. Wang, Adv. Mater., 2020, 32, 2004555 CrossRef CAS PubMed.
- G. D. Spyropoulos, J. Savarin, E. F. Gomez, D. T. Simon, M. Berggren, J. N. Gelinas, E. Stavrinidou and D. Khodagholy, Adv. Mater. Technol., 2020, 5, 1900652 CrossRef CAS.
- L. Liang, C. Liu, P. Cai, S. Han, R. Zhang, N. Ren, J. Wang, J. Yu, S. Shang, W. Zhou, J. Qiu, C. Mao, X. Chen, C. Sun and H. Liu, Nano Energy, 2022, 100, 107483 CrossRef CAS.
- M. A. O’Reilly, Science, 2024, 385, eadp7206 CrossRef PubMed.
- M. L. Prieto, K. Firouzi, B. T. Khuri-Yakub and M. Maduke, Ultrasound Med. Biol., 2018, 44, 1217–1232 CrossRef PubMed.
- Z. Qiu, S. Kala, J. Guo, Q. Xian, J. Zhu, T. Zhu, X. Hou, K. F. Wong, M. Yang, H. Wang and L. Sun, Cell Rep., 2020, 32, 108033 CrossRef CAS PubMed.
- J. Kubanek, P. Shukla, A. Das, S. A. Baccus and M. B. Goodman, J. Neurosci., 2018, 38, 3081–3091 CrossRef CAS PubMed.
- M. Duque, C. A. Lee-Kubli, Y. Tufail, U. Magaram, J. Patel, A. Chakraborty, J. Mendoza Lopez, E. Edsinger, A. Vasan, R. Shiao, C. Weiss, J. Friend and S. H. Chalasani, Nat. Commun., 2022, 13, 600 CrossRef CAS PubMed.
- S. Ibsen, A. Tong, C. Schutt, S. Esener and S. H. Chalasani, Nat. Commun., 2015, 6, 8264 CrossRef CAS PubMed.
- J. Kubanek, J. Shi, J. Marsh, D. Chen, C. Deng and J. Cui, Sci. Rep., 2016, 6, 24170 CrossRef CAS PubMed.
- S. R. Burks, R. M. Lorsung, M. E. Nagle, T. W. Tu and J. A. Frank, Theranostics, 2019, 9, 5517–5531 CrossRef CAS PubMed.
- B. Sorum, R. A. Rietmeijer, K. Gopakumar, H. Adesnik and S. G. Brohawn, Proc. Natl. Acad. Sci. U. S. A., 2021, 118, e2006980118 CrossRef CAS PubMed.
- M. Zhu, Y. Fang, Y. Sun, S. Li, J. Yu, B. Xiong, C. Wen, B. Zhou, B. Huang, H. Yin and H. Xu, Adv. Sci., 2024, 11, 2407373 CrossRef CAS PubMed.
- J. Hahmann, A. Ishaqat, T. Lammers and A. Herrmann, Angew. Chem., Int. Ed., 2024, 63, e202317112 CrossRef CAS PubMed.
- X. Hou, L. Liu and L. Sun, Biomaterials, 2025, 314, 122857 CrossRef CAS PubMed.
- X. Hou, J. Jing, Y. Jiang, X. Huang, Q. Xian, T. Lei, J. Zhu, K. F. Wong, X. Zhao, M. Su, D. Li, L. Liu, Z. Qiu and L. Sun, Nat. Commun., 2024, 15, 2253 CrossRef CAS PubMed.
- S. Lee, J. Kum, S. Kim, H. Jung, S. An, S. J. Choi, J. H. Choi, J. Kim, K. J. Yu, W. Lee, H. Kim, H.-S. Han, M. Shin, H. Kim and D. Son, Nat. Electron., 2024, 7, 800–814 CrossRef.
- C. Wang, X. Chen, L. Wang, M. Makihata, H. C. Liu, T. Zhou and X. Zhao, Science, 2022, 377, 517–523 CrossRef CAS PubMed.
- R. Chen, G. Romero, M. G. Christiansen, A. Mohr and P. Anikeeva, Science, 2015, 347, 1477–1480 CrossRef CAS PubMed.
- S. Rao, R. Chen, A. A. LaRocca, M. G. Christiansen, A. W. Senko, C. H. Shi, P. H. Chiang, G. Varnavides, J. Xue, Y. Zhou, S. Park, R. Ding, J. Moon, G. Feng and P. Anikeeva, Nat. Nanotechnol., 2019, 14, 967–973 CrossRef CAS PubMed.
- S. H. Choi, J. Shin, C. Park, J. Lee, J. Lee, Y. Ambo, W. Shin, R. Yu, J.-Y. Kim, J. D. Lah, D. Shin, G. Kim, K. Noh, W. Koh, C. J. Lee, J.-H. Lee, M. Kwak and J. Cheon, Nat. Nanotechnol., 2024, 19, 1333–1343 CrossRef CAS PubMed.
- J. Lee, W. Shin, Y. Lim, J. Kim, W. R. Kim, H. Kim, J. H. Lee and J. Cheon, Nat. Mater., 2021, 20, 1029–1036 CrossRef CAS PubMed.
- Y. J. Kim, N. Kent, E. Vargas Paniagua, N. Driscoll, A. Tabet, F. Koehler, E. Malkin, E. Frey, M. Manthey, A. Sahasrabudhe, T. M. Cannon, K. Nagao, D. Mankus, M. Bisher, G. De Nola, A. Lytton-Jean, L. Signorelli, D. Gregurec and P. Anikeeva, Nat. Nanotechnol., 2024, 20, 121–131 CrossRef CAS PubMed.
- G. Romero, J. Park, F. Koehler, A. Pralle and P. Anikeeva, Nat. Rev. Methods Primers, 2022, 2, 92 CrossRef CAS PubMed.
- A. Tay, A. Sohrabi, K. Poole, S. Seidlits and D. Di Carlo, Adv. Mater, 2018, 30, 1800927 CrossRef PubMed.
- L. Xue, Q. Ye, L. Wu, D. Li, S. Bao, Q. Lu, S. Liu, D. Sun, Z. Sheng, Z. Zhang, N. Gu and J. Sun, Nano Res., 2023, 16, 7393–7404 CrossRef CAS.
- C. O. Oluigbo and A. R. Rezai, IEEE Trans. Biomed. Eng., 2011, 58, 1907–1917 Search PubMed.
- M. D. Johnson, H. H. Lim, T. I. Netoff, A. T. Connolly, N. Johnson, A. Roy, A. Holt, K. O. Lim, J. R. Carey, J. L. Vitek and B. He, IEEE Trans. Biomed. Eng., 2013, 60, 610–624 Search PubMed.
-
E. S. Krames, P. Hunter Peckham, A. Rezai and F. Aboelsaad, in Neuromodulation, ed. E. S. Krames, P. H. Peckham and A. R. Rezai, Academic Press, San Diego, 2009, pp. 3–8 Search PubMed.
- A. M. Lozano, A. M. Lozano, N. Lipsman, N. Lipsman, N. Lipsman, H. Bergman, H. Bergman, P. Brown, P. Brown, S. Chabardès, S. Chabardes, J. W. Chang, J. W. Chang, K. Matthews, K. Matthews, C. C. McIntyre, C. C. McIntyre, T. E. Schläepfer, T. E. Schlaepfer, M. Schulder, M. Schulder, Y. Temel, Y. Temel, J. Volkmann, J. Volkmann, J. K. Krauss and J. K. Krauss, Nat. Rev. Neurol., 2019, 15, 148–160 CrossRef PubMed.
- M. D. Fox, R. L. Buckner, H. Liu, M. M. Chakravarty, A. M. Lozano and A. Pascual-Leone, Proc. Natl. Acad. Sci. U. S. A., 2014, 111, E4367–75 CrossRef CAS PubMed.
- S. A. Quadri, M. Waqas, I. Khan, M. A. Khan, S. S. Suriya, M. Farooqui and B. Fiani, Neurosurg. Focus, 2018, 44, E16 Search PubMed.
- M. Berggren, E. D. Głowacki, D. T. Simon, E. Stavrinidou and K. Tybrandt, Chem. Rev., 2022, 122, 4826–4846 CrossRef CAS PubMed.
- X. Yang, E. McGlynn, R. Das, S. P. Paşca, B. Cui and H. Heidari, Adv. Mater., 2021, 33, 2103208 CrossRef CAS PubMed.
- Z. Lyu, J. Park, K. M. Kim, H. J. Jin, H. Wu, J. Rajadas, D. H. Kim, G. K. Steinberg and W. Lee, Nat. Biomed. Eng., 2021, 5, 847–863 CrossRef CAS PubMed.
- J. W. Squair, M. Gautier, L. Mahe, J. E. Soriano, A. Rowald, A. Bichat, N. Cho, M. A. Anderson, N. D. James, J. Gandar, A. V. Incognito, G. Schiavone, Z. K. Sarafis, A. Laskaratos, K. Bartholdi, R. Demesmaeker, S. Komi, C. Moerman, B. Vaseghi, B. Scott, R. Rosentreter, C. Kathe, J. Ravier, L. McCracken, X. Kang, N. Vachicouras, F. Fallegger, I. Jelescu, Y. L. Cheng, Q. Li, R. Buschman, N. Buse, T. Denison, S. Dukelow, R. Charbonneau, I. Rigby, S. K. Boyd, P. J. Millar, E. M. Moraud, M. Capogrosso, F. B. Wagner, Q. Barraud, E. Bezard, S. P. Lacour, J. Bloch, G. Courtine and A. A. Phillips, Nature, 2021, 590, 308–314 CrossRef CAS PubMed.
- C. Kathe, M. A. Skinnider, T. H. Hutson, N. Regazzi, M. Gautier, R. Demesmaeker, S. Komi, S. Ceto, N. D. James, N. Cho, L. Baud, K. Galan, K. J. E. Matson, A. Rowald, K. Kim, R. Wang, K. Minassian, J. O. Prior, L. Asboth, Q. Barraud, S. P. Lacour, A. J. Levine, F. Wagner, J. Bloch, J. W. Squair and G. Courtine, Nature, 2022, 611, 540–547 CrossRef CAS PubMed.
- T. Kim, H. J. Kim, W. Choi, Y. M. Lee, J. H. Pyo, J. Lee, J. Kim, J. Kim, J. H. Kim, C. Kim and W. J. Kim, Nat. Biomed. Eng., 2023, 7, 149–163 CrossRef CAS PubMed.
- D. Farina, I. Vujaklija, R. Brånemark, A. M. J. Bull, H. Dietl, B. Graimann, L. J. Hargrove, K. P. Hoffmann, H. (Helen) Huang, T. Ingvarsson, H. B. Janusson, K. Kristjánsson, T. Kuiken, S. Micera, T. Stieglitz, A. Sturma, D. Tyler, R. F. ffWeir and O. C. Aszmann, Nat. Biomed. Eng., 2023, 7, 473–485 CrossRef PubMed.
- C. Kathe, F. Michoud, P. Schönle, A. Rowald, N. Brun, J. Ravier, I. Furfaro, V. Paggi, K. Kim, S. Soloukey, L. Asboth, T. H. Hutson, I. Jelescu, A. Philippides, N. Alwahab, J. Gandar, D. Huber, C. I. De Zeeuw, Q. Barraud, Q. Huang, S. P. Lacour and G. Courtine, Nat. Biotechnol., 2022, 40, 198–208 CrossRef CAS PubMed.
- B. Barra, S. Conti, M. G. Perich, K. Zhuang, G. Schiavone, F. Fallegger, K. Galan, N. D. James, Q. Barraud, M. Delacombaz, M. Kaeser, E. M. Rouiller, T. Milekovic, S. Lacour, J. Bloch, G. Courtine and M. Capogrosso, Nat. Neurosci., 2022, 25, 924–934 CrossRef CAS PubMed.
- W. Ouyang, W. Lu, Y. Zhang, Y. Liu, J. U. Kim, H. Shen, Y. Wu, H. Luan, K. Kilner, S. P. Lee, Y. Lu, Y. Yang, J. Wang, Y. Yu, A. J. Wegener, J. A. Moreno, Z. Xie, Y. Wu, S. M. Won, K. Kwon, C. Wu, W. Bai, H. Guo, T. Li Liu, H. Bai, G. Monti, J. Zhu, S. R. Madhvapathy, J. Trueb, M. Stanslaski, E. M. Higbee-Dempsey, I. Stepien, N. Ghoreishi-Haack, C. R. Haney, T. il Kim, Y. Huang, R. Ghaffari, A. R. Banks, T. C. Jhou, C. H. Good and J. A. Rogers, Nat. Biomed. Eng., 2023, 7, 1252–1269 CrossRef PubMed.
- K. Kwon, J. U. Kim, S. M. Won, J. Zhao, R. Avila, H. Wang, K. S. Chun, H. Jang, K. H. Lee, J. H. Kim, S. Yoo, Y. J. Kang, J. Kim, J. Lim, Y. Park, W. Lu, T. il Kim, A. Banks, Y. Huang and J. A. Rogers, Nat. Biomed. Eng., 2023, 7, 1215–1228 CrossRef PubMed.
- Y. Qin, H. Zhao, Q. Chang, Y. Liu, Z. Jing, D. Yu, S. M. Mugo, H. Wang and Q. Zhang, Adv. Mater., 2024, 2416926 Search PubMed.
- M. Y. Cheng, E. H. Wang, W. J. Woodson, S. Wang, G. Sun, A. G. Lee, A. Arac, L. E. Fenno, K. Deisseroth and G. K. Steinberg, Proc. Natl. Acad. Sci. U. S. A., 2014, 111, 12913–12918 CrossRef CAS PubMed.
- S. Vahdat, A. V. Pendharkar, T. Chiang, S. Harvey, H. Uchino, Z. Cao, A. Kim, M. Choy, H. Chen, H. J. Lee, M. Y. Cheng, J. H. Lee and G. K. Steinberg, Sci. Adv., 2021, 7, eabd9465 CrossRef CAS PubMed.
- H. Hampel, R. Au, S. Mattke, W. M. van der Flier, P. Aisen, L. Apostolova, C. Chen, M. Cho, S. De Santi, P. Gao, A. Iwata, R. Kurzman, A. J. Saykin, S. Teipel, B. Vellas, A. Vergallo, H. Wang and J. Cummings, Nat. Aging, 2022, 2, 692–703 CrossRef PubMed.
- G. Livingston, J. Huntley, A. Sommerlad, D. Ames, C. Ballard, S. Banerjee, C. Brayne, A. Burns, J. Cohen-Mansfield, C. Cooper, S. G. Costafreda, A. Dias, N. Fox, L. N. Gitlin, R. Howard, H. C. Kales, M. Kivimäki, E. B. Larson, A. Ogunniyi, V. Orgeta, K. Ritchie, K. Rockwood, E. L. Sampson, Q. Samus, L. S. Schneider, G. Selbæk, L. Teri and N. Mukadam, The Lancet, 2020, 396, 413–446 CrossRef PubMed.
- P. C. Ke, M. A. Sani, F. Ding, A. Kakinen, I. Javed, F. Separovic, T. P. Davis and R. Mezzenga, Chem. Soc. Rev., 2017, 46, 6492–6531 RSC.
- S. J. C. Lee, E. Nam, H. J. Lee, M. G. Savelieff and M. H. Lim, Chem. Soc. Rev., 2017, 46, 310–323 RSC.
- E. Karran and B. De Strooper, Nat. Rev. Drug Discovery, 2022, 21, 306–318 CrossRef CAS PubMed.
- Y.-D. Li, Y. J. Luo, L. Xie, D. S. Tart, R. N. Sheehy, L. Zhang, L. G. Coleman, X. Chen and J. Song, Cell Stem Cell, 2023, 30, 415–432.e6 CrossRef CAS PubMed.
- Z. Lv, L. Chen, P. Chen, H. Peng, Y. Rong, W. Hong, Q. Zhou, N. Li, B. Li, R. C. Paolicelli and Y. Zhan, Neuron, 2024, 112, 740–754.e7 CrossRef CAS PubMed.
- K. Xhima, K. Markham-Coultes, H. Nedev, S. Heinen, H. U. Saragovi, K. Hynynen and I. Aubert, Sci. Adv., 2020, 6, eaax6646 CrossRef CAS PubMed.
- A. R. Rezai, P. F. D’Haese, V. Finomore, J. Carpenter, M. Ranjan, K. Wilhelmsen, R. I. Mehta, P. Wang, U. Najib, C. Vieira Ligo Teixeira, T. Arsiwala, A. Tarabishy, P. Tirumalai, D. O. Claassen, S. Hodder and M. W. Haut, N. Engl. J. Med., 2024, 390, 55–62 CrossRef CAS PubMed.
- P. Scheltens, B. De Strooper, M. Kivipelto, H. Holstege, G. Chételat, C. E. Teunissen, J. Cummings and W. M. Van Der Flier, The Lancet, 2021, 397, 1577–1590 CrossRef CAS PubMed.
- J. Parkinson, J. Neuropsychiatry Clin. Neurosci., 2002, 14, 223–236 CrossRef PubMed.
- N. Pankratz and T. Foroud, Genet. Med., 2007, 9, 801–811 CrossRef PubMed.
- H. Murakami, T. Shiraishi, T. Umehara, S. Omoto and Y. Iguchi, Intern. Med., 2023, 62, 33–42 CrossRef CAS PubMed.
- M. Sandler, R. D. Johnson, C. R. J. Ruthven, J. L. Reid and D. B. Calne, Nature, 1974, 247, 364–366 CrossRef CAS PubMed.
- J. M. Miyasaki, W. Martin, O. Suchowersky, W. J. Weiner and A. E. Lang, Neurology, 2002, 58, 11–17 CrossRef CAS PubMed.
- N. Singh, V. Pillay and Y. E. Choonara, Prog. Neurobiol., 2007, 81, 29–44 CrossRef CAS PubMed.
- G. Porras, P. De Deurwaerdere, Q. Li, M. Marti, R. Morgenstern, R. Sohr, E. Bezard, M. Morari and W. G. Meissner, Sci. Rep., 2014, 4, 3730 CrossRef PubMed.
- D. Masini and O. Kiehn, Nat. Commun., 2022, 13, 504 CrossRef CAS PubMed.
- M. Fougère, C. I. Van Der Zouwen, J. Boutin, K. Neszvecsko, P. Sarret and D. Ryczko, Proc. Natl. Acad. Sci. U. S. A., 2021, 118, e2110934118 CrossRef PubMed.
- A. V. Kravitz, B. S. Freeze, P. R. L. Parker, K. Kay, M. T. Thwin, K. Deisseroth and A. C. Kreitzer, Nature, 2010, 466, 622–626 CrossRef CAS PubMed.
- W. Shin, Y. Lee, J. Lim, Y. Lee, J. D. Lah, S. Lee, J. Lee, R. Yu, P. H. Lee, J.-H. Lee, M. Kwak and J. Cheon, Nano Lett., 2024, 24, 270–278 CrossRef CAS PubMed.
- F. Tecuapetla, X. Jin, S. Q. Lima and R. M. Costa, Cell, 2016, 166, 703–715 CrossRef CAS PubMed.
- P. Calabresi, B. Picconi and A. Tozzi, Nat. Neurosci., 2014, 17, 1022–1030 CrossRef CAS PubMed.
- J. H. Shin, M. Song, S. B. Paik and M. W. Jung, Proc. Natl. Acad. Sci. U. S. A., 2020, 117, 27004–27015 CrossRef CAS PubMed.
- B. R. Isett, K. P. Nguyen, J. C. Schwenk, J. R. Yurek, C. N. Snyder, M. V. Vounatsos, K. A. Adegbesan, U. Ziausyte and A. H. Gittis, Neuron, 2023, 111, 2218–2231.e4 CrossRef CAS PubMed.
- M. Lange, J. Mauerer, J. Schlaier, A. Janzen, F. Zeman, U. Bogdahn, A. Brawanski and A. Hochreiter, Acta Neurochir., 2017, 159, 771–778 CrossRef PubMed.
- S.-A. Hescham, P. H. Chiang, D. Gregurec, J. Moon, M. G. Christiansen, A. Jahanshahi, H. Liu, D. Rosenfeld, A. Pralle, P. Anikeeva and Y. Temel, Nat. Commun., 2021, 12, 5569 CrossRef CAS PubMed.
- M. Wiseman, I. J. Sewell, S. M. Nestor, P. Giacobbe, C. Hamani, N. Lipsman and J. S. Rabin, Nat. Rev. Psychol, 2024, 3, 242–260 CrossRef.
- O. Devinsky, A. Vezzani, T. J. O’Brien, N. Jette, I. E. Scheffer, M. De Curtis and P. Perucca, Nat. Rev. Dis. Primers, 2018, 4, 18024 CrossRef PubMed.
- C. Yang, Z. Cheng, P. Li and B. Tian, Acc. Chem. Res., 2024, 57, 1398–1410 CrossRef CAS PubMed.
- P. Ryvlin, S. Rheims, L. J. Hirsch, A. Sokolov and L. Jehi, Lancet Neurol., 2021, 20, 1038–1047 CrossRef PubMed.
- O. Karatum, M. J. Gwak, J. Hyun, A. Onal, G. R. Koirala, T. Kim and S. Nizamoglu, Chem. Soc. Rev., 2023, 52, 3326–3352 RSC.
- R. J. Piper, R. M. Richardson, G. Worrell, D. W. Carmichael, T. Baldeweg, B. Litt, T. Denison and M. M. Tisdall, Brain, 2022, 145, 3347–3362 CrossRef PubMed.
- C. Marar, Y. Jiang, Y. Li, L. Lan, N. Zheng, G. Chen, C. Yang and X. Cheng, Sci. Adv., 2024, 10, eado5560 CrossRef CAS PubMed.
- D. C. Patel, B. P. Tewari, L. Chaunsali and H. Sontheimer, Nat. Rev. Neurosci., 2019, 20, 282–297 CrossRef CAS PubMed.
- S. Robel and H. Sontheimer, Nat. Neurosci., 2016, 19, 28–33 CrossRef CAS PubMed.
- O. Devinsky, A. Vezzani, S. Najjar, N. C. De Lanerolle and M. A. Rogawski, Trends Neurosci., 2013, 36, 174–184 CrossRef CAS PubMed.
- B. S. Khakh and M. V. Sofroniew, Nat. Neurosci., 2015, 18, 942–952 CrossRef CAS PubMed.
- J. Zhao, J. Sun, Y. Zheng, Y. Zheng, Y. Shao, Y. Li, F. Fei, C. Xu, X. Liu, S. Wang, Y. Ruan, J. Liu, S. Duan, Z. Chen and Y. Wang, Nat. Commun., 2022, 13, 7136 CrossRef PubMed.
- R. M. Jamiolkowski, Q.-A. Nguyen, J. S. Farrell, R. J. McGinn, D. A. Hartmann, J. J. Nirschl, M. I. Sanchez, V. P. Buch and I. Soltesz, Nat. Med., 2024, 30, 1292–1299 CrossRef CAS PubMed.
- Q. Ren, H. Wu, Y. Zhang, J. Dai, Z. Chang, J. Nie, B. Wang and Y. Fang, ACS Nano, 2024, 18, 16853–16866 CrossRef CAS PubMed.
- Y. Zhang, X. Wu, J. Ding, B. Su, Z. Chen, Z. Xiao, C. Wu, D. Wei, J. Sun, F. Luo, H. Yin and H. Fan, ACS Nano, 2023, 17, 15796–15809 CrossRef CAS PubMed.
- J. P. Andrews, J. Geng, K. Voitiuk, M. A. T. Elliott, D. Shin, A. Robbins, A. Spaeth, A. Wang, L. Li, D. Solis, M. G. Keefe, J. L. Sevetson, J. A. Rivera De Jesús, K. C. Donohue, H. H. Larson, D. Ehrlich, K. I. Auguste, S. Salama, V. Sohal, T. Sharf, D. Haussler, C. R. Cadwell, D. V. Schaffer, E. F. Chang, M. Teodorescu and T. J. Nowakowski, Nat. Neurosci., 2024, 27, 2487–2499 CrossRef CAS PubMed.
- R. C. Kessler, W. T. Chiu, O. Demler and E. E. Walters, Arch. Gen. Psychiatry, 2005, 62, 617 CrossRef PubMed.
- K. M. Tye, J. J. Mirzabekov, M. R. Warden, E. A. Ferenczi, H.-C. Tsai, J. Finkelstein, S.-Y. Kim, A. Adhikari, K. R. Thompson, A. S. Andalman, L. A. Gunaydin, I. B. Witten and K. Deisseroth, Nature, 2013, 493, 537–541 CrossRef CAS PubMed.
- S. Han, X. X. Li, S. Wei, D. Zhao, J. Ding, Y. Xu, C. Yu, Z. Chen, D. S. Zhou and T. F. Yuan, Cell Rep. Med., 2023, 4, 101060 CrossRef PubMed.
- J. Liu, J. W. Mo, X. Wang, Z. An, S. Zhang, C. Y. Zhang, P. Yi, A. T. L. Leong, J. Ren, L.-Y. Chen, R. Mo, Y. Xie, Q. Feng, W. Chen, T. M. Gao, E. X. Wu, Y. Feng and X. Cao, Sci. Adv., 2022, 8, eabo2098 CrossRef CAS PubMed.
- K. W. Scangos, A. N. Khambhati, P. M. Daly, G. S. Makhoul, L. P. Sugrue, H. Zamanian, T. X. Liu, V. R. Rao, K. K. Sellers, H. E. Dawes, P. A. Starr, A. D. Krystal and E. F. Chang, Nat. Med., 2021, 27, 1696–1700 CrossRef CAS PubMed.
- K. W. Scangos, G. S. Makhoul, L. P. Sugrue, E. F. Chang and A. D. Krystal, Nat. Med., 2021, 27, 229–231 CrossRef CAS PubMed.
- J. W. Busse, L. Wang, M. Kamaleldin, S. Craigie, J. J. Riva, L. Montoya, S. M. Mulla, L. C. Lopes, N. Vogel, E. Chen, K. Kirmayr, K. De Oliveira, L. Olivieri, A. Kaushal, L. E. Chaparro, I. Oyberman, A. Agarwal, R. Couban, L. Tsoi, T. Lam, P. O. Vandvik, S. Hsu, M. M. Bala, S. Schandelmaier, A. Scheidecker, S. Ebrahim, V. Ashoorion, Y. Rehman, P. J. Hong, S. Ross, B. C. Johnston, R. Kunz, X. Sun, N. Buckley, D. I. Sessler and G. H. Guyatt, JAMA, 2018, 320, 2448 CrossRef PubMed.
- X. Zhu, J. Y. Huang, W. Y. Dong, H. D. Tang, S. Xu, Q. Wu, H. Zhang, P.-K. Cheng, Y. Jin, M. Y. Zhu, W. Zhao, Y. Mao, H. Wang, Y. Zhang, H. Wang, W. Tao, Y. Tian, L. Bai and Z. Zhang, Nat. Neurosci., 2024, 27, 471–483 CrossRef CAS PubMed.
- Q. Zhang, S. Hu, R. Talay, Z. Xiao, D. Rosenberg, Y. Liu, G. Sun, A. Li, B. Caravan, A. Singh, J. D. Gould, Z. S. Chen and J. Wang, Nat. Biomed. Eng., 2021, 7, 533–545 CrossRef PubMed.
- G. Sun, M. McCartin, W. Liu, Q. Zhang, G. Kenefati, Z. S. Chen and J. Wang, Mol. Brain, 2023, 16, 3 CrossRef PubMed.
Footnote |
† These authors contributed equally. |
|
This journal is © The Royal Society of Chemistry 2025 |
Click here to see how this site uses Cookies. View our privacy policy here.