Equity-centered adaptive sampling in sub-sewershed wastewater surveillance using census data†
Received
30th June 2024
, Accepted 2nd October 2024
First published on 24th October 2024
Abstract
Sub-city, or sub-sewershed, wastewater monitoring for infectious diseases offers a data-driven strategy to inform local public health response and complements city-wide data from centralized wastewater treatment plants. Developing strategies for equitable representation of diverse populations in sub-city wastewater sampling frameworks is complicated by misalignment between demographic data and sampling zones. We address this challenge by: (1) developing a geospatial analysis tool that probabilistically assigns demographic data for subgroups aggregated by race and age from census blocks to sub-city sampling zones; (2) evaluating representativeness of subgroup populations for COVID-19 wastewater-based disease surveillance in Davis, California; and (3) demonstrating scenario planning that prioritizes vulnerable populations. We monitored SARS-CoV-2 in wastewater as a proxy for COVID-19 incidence in Davis (November 2021–September 2022). Daily city-wide sampling and thrice-weekly sub-city sampling from 16 maintenance holes covered nearly the entire city population. Sub-city wastewater data, aggregated as a population-weighted mean, correlated strongly with centralized treatment plant data (Spearman's correlation 0.909). Probabilistic assignment of demographic data can inform decisions when adapting sampling locations to prioritize vulnerable groups. We considered four scenarios that reduced the number of sampling zones from baseline by 25% and 50%, chosen randomly or to prioritize coverage of >65-year-old populations. Prioritizing representation increased coverage of >65-year-olds from 51.1% to 67.2% when removing half the zones, while increasing coverage of Black or African American populations from 67.5% to 76.7%. Downscaling had little effect on correlations between sub-city and centralized data (Spearman's correlations ranged from 0.875 to 0.917), with strongest correlations observed when prioritizing coverage of >65-year-old populations.
Water impact
Wastewater-based disease surveillance should aim to achieve equitable representation of vulnerable groups within sampling regions. The probabilistic assignment approach in this study helps determine the distribution of demographic groups within sampling areas at sub-sewershed levels. When resource constraints necessitate downscaling the number of sampling sites, the approach demonstrated herein can inform decisions to preserve spatial representation of vulnerable populations.
|
Introduction
Social determinants of health (SDOH) have been identified as the set of conditions that drive health disparities.1 SDOH include a variety of factors such as race, socioeconomic position, and age, all of which play a crucial role in determining health outcomes within a population.2 The cumulative impact of these factors drives structural inequities.3 The Coronavirus Disease-2019 (COVID-19) pandemic highlighted how social vulnerabilities can contribute to infections and subsequent community spread, with increased risk of serious illness attributed to pre-existing comorbidities and older age, and disproportionate impacts seen in low-income populations and communities of color.4
According to the Centers for Disease Control and Prevention (CDC), the three major factors affecting COVID-19's unequal distribution of impact are age, race, and ethnicity, with age cited as the main risk factor for severe COVID-19 outcomes.5 Data from the National Vital Statistics System has shown that, relative to those between ages 18–29 years old, risk of death from COVID-19 is 25 times higher for people between ages 50–64 years, 60 times higher for people between 65–74 years, 140 times higher for people between 75–84 years, and 340 times higher for those 85 years and older.6 Moreover, the COVID-19 pandemic underscored racial and ethnic health inequities. Individuals from racial and ethnic minority groups were disproportionately affected by severe acute respiratory syndrome coronavirus 2 (SARS-CoV-2) transmission, leading to increased rates of hospitalization, emergency room visits, and premature death compared to non-Hispanic White individuals.6 Since March 2020, the average daily increase in COVID-19 mortality was found to be much higher in rural U.S. counties amongst predominantly Black and Hispanic populations.7 Among rural counties, those in the top quartile of percent Black populations had an average daily increase in COVID-19 mortality rates 70% higher than counties in the bottom quartile, and counties in the top quartile of percent Hispanic populations had an average daily increase that was 50% higher.7
Wastewater-based disease surveillance (WDS), which involves analyzing community-pooled wastewater samples from centralized wastewater treatment plants or sewer collection systems for disease biomarkers, has emerged as a viable strategy to provide insight into population-level disease trends. WDS has been favored as a minimally invasive, anonymous, and cost-effective way to track virus spread compared to testing individuals within the population since more than 80% of U.S. residents are on a piped sewer system.1 Each wastewater sample can represent hundreds to over a million people depending on the sample collection location. Those infected with SARS-CoV-2, including asymptomatic, pre-symptomatic, and symptomatic individuals, can shed viral particles and associated ribonucleic acid (RNA) through fecal matter. SARS-CoV-2 RNA remains readily detectable in wastewater even though fecal–oral transmission has not been reported for this virus. WDS has thus filled gaps associated with underreporting of cases, and can serve as an early indicator of potential outbreaks.8 Moreover, WDS has proven to be a more comprehensive approach to tracking viral outbreaks and community infections since it does not rely on community members having access to clinical testing services or seeking healthcare when they are experiencing symptoms.9 WDS can be particularly useful in resource-limited settings (e.g., where clinical testing services are constrained).
Trends in wastewater data at a sub-city level (e.g., at the census block level, the most granular level at which public demographic data can be obtained) have also been used in WDS to inform public health responses.10,11 Early in the pandemic, many WDS programs were established rapidly via academic and government partnerships, and sampling locations were not always selected in a methodical way. While the utility of WDS is evident, sampling paradigms that rely on convenient points of access within the sewer network may not equitably serve the public or public health, as some populations may be underrepresented depending on where sampling occurs. There may be similar disparities in traditional monitoring efforts as there are disparities in access to clinical testing and vaccinations.12 Recent efforts in the field have acknowledged the importance of taking steps targeted at reducing inequities,13 but standardized measures for assessing performance of WDS towards improving health equity are lacking. Additional analysis is needed to evaluate whether chosen sampling locations are appropriately representative of a given community, especially in such cases as wastewater surveillance where any given sample pools together information from a broad population.
Equitable protection of public health can be guided through inclusive wastewater surveillance efforts.4 Specific considerations are needed to promote inclusion of underrepresented groups and equity in responses to public health threats, including evaluations regarding the extent to which vulnerable populations are represented in wastewater monitoring programs.14 Ultimately, the success of a WDS program relies on the assurance that there is equitable representation of high-risk and/or underserved communities in the sampling design. Evaluating demographic representation of sub-sewershed zone populations can prove difficult because data at a census block level does not align with sewer networks. In other words, flows of wastewater from populations within census blocks depend on city-wide sewer system connections and do not conform to the zones of population that are represented from wastewater samples collected from maintenance holes (MHs) within a city.
This project offers a strategy for WDS sub-city (or sub-sewershed) health equity evaluations using census data at a block-level with the goal of enhancing inclusivity in the design of sampling frameworks within the constraints of a sewer system. First, we developed a probabilistic assignment approach to determine the expected subgroup population that is represented by collecting samples at different locations in a city sewer system. We used sub-sewershed wastewater surveillance during the COVID-19 pandemic and demographic data in Davis, California to demonstrate the approach. Second, we compared trends in sub-sewershed wastewater data to city-wide trends. Sampling frameworks may seek to achieve representativeness of overall community disease trends in addition to achieving representation based on demographic characteristics. Finally, we evaluated scenarios in which adaptive sampling strategies are implemented to prioritize representation of high-risk or vulnerable populations under resource-constrained conditions. The overall framework offers a strategy to evaluate sub-city sampling designs for wastewater surveillance to enhance health equity goals.
Experimental
Study setting and design
This study includes a retrospective evaluation of SARS-CoV-2 wastewater concentration data collected during the COVID-19 pandemic as part of the Healthy Davis Together (HDT) program in Davis, a city with a population of approximately 66
850 in Yolo County, California.15 HDT offered wide-spread saliva-based asymptomatic and symptomatic testing for free, conducting over 1.6 million COVID-19 tests for the community and the university across 120 locations including testing sites at local schools, community centers, the university campus, and mobile clinics.16 From September 2020 to September 2022, we conducted wastewater surveillance throughout the City of Davis (COD) at the city, sub-city, and building/neighborhood levels.17Table 1 provides definitions for key terms associated with the sampling framework. At the city level, samples were collected from the influent to the COD Wastewater Treatment Plant (COD WWTP). At the sub-sewershed level, samples were collected from up to sixteen nodes that each represent a sub-sewershed within the COD. At the building/neighborhood level, samples were collected from up to seven additional nodes for building complexes or neighborhoods identified as priority areas by HDT and local officials for potential communication and/or health interventions. The number of sampling locations and frequency of sampling increased through time. By April 2021, HDT sampled daily from the COD WWTP and three times per week from MHs in each of the sub-sewershed and building/neighborhood zones. Safford et al. (2022)17 describe HDT wastewater surveillance conducted from September 2020 to June 2021, for which wastewater samples were collected at seven building/neighborhood locations, 16 sub-sewershed nodes, and the city level. These samples were analyzed using reverse transcription quantitative polymerase chain reaction (RT-qPCR) and showed good correlation with clinical test results at the city-level and sub-sewershed level. Daza-Torres et al. (2023)18 report city-level (COD WWTP) wastewater surveillance data from December 1, 2021, to March 31, 2022, which was measured using reverse transcription droplet digital PCR (RT-ddPCR). The present study describes and analyzes HDT wastewater surveillance conducted from November 22, 2021, to September 30, 2022, for which wastewater samples were collected daily from the COD WWTP and three times per week at 15 sub-sewershed nodes. All samples in this study were analyzed using RT-ddPCR as reported by Daza-Torres et al. (2023)18 and described below.
Table 1 Introductory terms and definitions
Key term |
Definition |
Sewershed |
The area that contributes wastewater to a common end point. In this study, the sewershed refers to the area whose sewers flow to the City of Davis Wastewater Treatment Plant (COD WWTP) |
Sewershed node |
A maintenance hole (MH) that serves as a wastewater sampling location |
Sub-sewershed zone |
The area represented by one or more sewershed nodes. Also referred to as sub-city zone |
Subgroup |
A subset of the overall city population that shares a specific demographic characteristic (e.g., race or age) |
Wastewater sample collection
Sample collection was previously described by Safford et al. (2022).17 Briefly, samples at each sub-sewershed node were collected using insulated Hach AS950 Portable Compact Samplers (Thermo Fisher Scientific, USA), which were programmed to collect 30 mL of sample every 15 minutes. Composite wastewater influent samples from the COD WWTP were collected using Teledyne ISCO 5800 refrigerated autosamplers. Each sampler was programmed to collect 400 mL of wastewater every 15 “pulses”. Each pulse was set at 10
000 gallons.18 Based on the influent flow of 3.6 million gallons per day, about 24 pulses were expected per day. Each sample date recorded corresponds to the date that an autosampler program was completed. The COD WWTP provided 12 mL samples in new 15 mL polypropylene centrifuge tubes. The samples were stored at 4 °C. Samples were transported to the analytical laboratory at UC Davis in coolers on ice and generally processed the same day. Samples were first pasteurized for 30 minutes at 60 °C to mitigate biohazard risk while maintaining RNA quality and equilibrated to 4 °C prior to additional processing described below.
Concentration and nucleic acid extraction procedures
Protocols to concentrate viruses and to extract and purify nucleic acids were implemented in a biosafety level 2 (BSL2)-certified laboratory. The Environmental Microbiology Minimum Information Checklist was followed for quality assurance and quality control in the laboratory.19 Each starting sample volume of 4.875 mL was deposited into a separate well of a KingFisher 24 deep-well plate (Thermo Fisher). Nuclease-free water was included as a sample to act as a contamination control during the concentration and extraction process.19 Five μL of a stock of inactivated encapsulated whole vaccine-strain Bovine Coronavirus (BCoV, Bovilis® Coronavirus vaccine) was spiked into each well. The vaccine stock was determined by RT-ddPCR quantification to contain approximately 1.3 × 108 gc mL−1. The initial BCoV vaccine stock arrived lyophilized and was reconstituted in 20 mL of provided diluent, then divided into 1.5 mL aliquots and stored at −80 °C before use. Fifty μL of Nanotrap® Enhancement Reagent 1 (Ceres Nanosciences product ER1 SKU #10111-30) were added into each well alongside the BCoV spike. Then, 75 μL Nanotrap® Magnetic Virus Particles (Ceres Nanosciences, Inc.) were added to each well. A KingFisher Apex robot (Thermo Scientific) was used to concentrate the viruses from the samples using a protocol provided by Ceres Nanosciences, Inc.20 with minor modifications described herein. The solution was mixed 10 times at medium speed for 15 seconds with a 45 second pause between mixes. The magnet was then engaged, and the beads were collected by five three-second magnetic collection steps and transferred to 400 μL MagMAX Lysis Buffer from the MagMAX Microbiome Ultra Nucleic Acid Isolation kit (Thermo Fisher). The magnet was disengaged and mixed at medium speed for 10 seconds at the bottom of the well and another 10 seconds in the full well. The magnet was re-engaged, and beads were collected with five one-second magnetic collection steps. The beads were re-suspended with by mixing five times for 15 seconds with a pause of 1 minute 45 seconds between each mix. Beads were recollected with five-second magnetic collection steps and discarded into the original sample wells. The sample concentrates were then extracted using the KingFisher Apex according to MagMAX Microbiome Ultra Nucleic Acid Isolation kit instructions using a script provided by Thermo Fisher (MagMAX_Microbiome_Flex96_Wastewater.kfx), modified to match our input volume and 100 μL elution volume, and implemented with one of each wash step and without use of proteinase K. Specifically, the sample concentrates (400 μL) were transferred to 96 deep-well KingFisher plates that contained 500 μL MagMAX™ Viral/Pathogen Binding Solution and 20 μL MagMAX™ DNA/RNA Binding Beads in each well. The solution was mixed for five minutes, and the beads were collected and transferred to a reservoir containing one mL of MagMAX™ Viral/Pathogen Wash Solution and released. After 60 seconds of mixing, alternating between 10 seconds of bottom-mixing and 10 seconds of fast full-well mixing, the beads were collected and transferred to one mL of 80% ethanol and mixed in an identical fashion for an additional 40 seconds before being collected once more. The beads were then eluted into 100 μL of MagMAX™ Elution Solution by alternating between 15 seconds of bottom-mixing and 45 seconds of medium-speed full-well mixing over a period of six minutes before a slow-moving release of the nucleic acids from the binding beads into solution over a period of 90 seconds. Typically, extracts were stored on ice and analyzed on the same day, but extracts were stored at −80 °C to preserve RNA quality for up if same-day analysis was not possible. In this study, we did not include the recorded concentration of SARS-CoV-2 in our final dataset if more than 14 days passed between sample collection and processing.
Extract analysis by droplet digital PCR
Digital droplet polymerase chain reaction (ddPCR) was used to analyze the nucleic acid extracts. The targets analyzed were the N1 and N2 targeting regions of the nucleocapsid (N) gene of SARS-CoV-2, and Bovine Coronavirus (BCoV) and pepper mild mottle virus (PMMoV) to normalize the SARS-CoV-2 results. The primer and probe sequences for each target are listed in Table S1.† Separate duplex assays were used to quantify N1/N2 and BCoV/PMMoV. The fluorophores used for detection were carboxyfluorescein (FAM) for N1 and PMMoV, 2′-chloro-7′-phenyl-1,4-dichloro-6-carboxyfluorescein (VIC) for N2, and hexachlorofluorescein (HEX) for BCoV. The sample for the duplex for PMMoV and BCoV was diluted 40× before loading due to the high concentrations of PMMoV in wastewater. A QX ONE ddPCR System (Bio-Rad) was used to perform the ddPCR amplifications in 20 μL reactions. Each reaction contained 15 μL of master mix from the One-Step RT-ddPCR Advanced kit for probes (Bio-Rad) with primer concentrations at 900 nM of each primer and probe concentrations at 250 nM of each probe, plus a 5 μL of sample extract or control. The cycling conditions for RT-ddPCR are listed in Table S2.†
To avoid contamination, preparation and plating of the ddPCR master mix were conducted manually in a PCR hood in a separate location from sample loading, which was performed using an epMotion® 5075 (Eppendorf) liquid handler. Each reaction plate included duplicate positive controls (stock mixture of synthesized gene fragments, gBlocks™ from Integrated DNA Technologies, for the target regions) and duplicate no-template controls (nuclease-free water). Results were analyzed using the QX One Software Regular Edition (Bio-Rad) and thresholds were adjusted by visual inspection in samples and controls. The results were considered invalid if the distribution of positive or negative droplets appeared abnormal or if the total number of droplets generated was below 10
000 droplets in a well.
Wastewater ddPCR data processing
To assess the sensitivity of the assay, a limit of detection (LOD) and a limit of blank (LOB) were determined using protocols recommended by Bio-Rad.21 Fifteen wastewater samples that were negative for SARS-CoV-2 were used to determine the lowest detectable SARS-CoV-2 concentrations in apparently negative wastewater samples. These 24-hour composite samples had been collected on November 11, 2021, from building clean-outs or MHs downstream of UC Davis residential buildings. The samples originally tested negative (zero positive droplets) or very low (no greater than three positive droplets for each N target). The samples were re-analyzed by RT-ddPCR to acquire data for four additional replicates for each sample. Since the results from the blank were not normally distributed, rank order was used to select the LOB. To do so, the number of droplets from individual wells were recorded from lowest to highest, and the LOB was set at the value of the concentration measurement for the rank position corresponding to the 95th percentile, using the following equation: | Rank = 0.5 + 0.95 × (number of measurements) | (1) |
The rank position was rounded up to provide a more conservative LOB since the calculated value was a non-integer. The theoretical LOD was determined by adding two times the standard deviation of all the replicate results to the LOB. The LOD and LOB values are reported in Table S3.† The highest number of positive droplets in the merged wells of the blank samples were 6 (N1) and 8 (N2). The cutoff was set at 3 (N1) and 4 (N2) since wastewater samples were routinely analyzed in duplicate. This way, it was possible to mark samples below the droplet threshold. Additionally, if the samples had fewer N1 and N2 droplets than twice the number of droplets in the extraction control blank that was analyzed on the same day, they were considered below the droplet threshold. Furthermore, runs that had an extraction control blank with greater than 15 positive droplets (N1 or N2) were considered contaminated, and the extracts were re-processed.
If the samples satisfied the criteria above, the relative concentration of N gene was calculated. This was done by merging the duplicate results for each target and calculating the concentration of each target in the RT-ddPCR reaction, assuming a Poisson distribution using the QXOne Software 1.1.1 Standard Edition. To obtain the average SARS-CoV-2 RNA concentration in the initial wastewater sample, the N1 and N2 results were averaged after correcting for the sample and reagent volumes used. The resultant value was reported as genome copies (gc) per mL wastewater. Concentrations of targets were not corrected for BCoV recovery efficiency. A threshold BCoV recovery value of 10% was used to retain the recorded SARS-CoV-2 concentrations that had a recovery rate equal to or greater than the threshold value. High variability is to be expected among BCoV recovery values due to variability of sample characteristics. Other recovery analyses have reported average BCoV recovery values ranging from 4.8% to 36.1%, depending on the virus concentration method employed.22 Targets were excluded from the average concentration if N1 or N2 merged droplet counts were below the minimum droplet threshold, and the concentration was reported as 0 if both the N1 and N2 targets were below the droplet threshold. We use N/PMMoV (the average SARS-CoV-2 RNA concentration (N) divided by the concentration of PMMoV) as the wastewater signal for subsequent analysis.
Wastewater dataset
The dataset used in this study comprises normalized SARS-CoV-2 concentrations recorded between November 22, 2021, and September 30, 2022. The SARS-CoV-2 concentrations were normalized with PMMoV concentrations. PMMoV was universally present and in high concentrations in wastewater samples, making it a suitable biomarker for process control.23 For visualization purposes, a 10-day trimmed right-aligned moving average was applied to the data. To do so, 10% of the values on either end of the dataset were removed prior to calculating the mean of the current day and the previous nine days. This effectively smoothed out short-term variations of the normalized N gene metric. Fig. 1 depicts the wastewater data retrieved from one sub-sewershed zone (SR-A), showing the raw wastewater surveillance data (red) and the 10-day trimmed right-aligned moving average (black) of the data. In the figure, we see that the peaks in the data coincide with the surge in infections when the Omicron BA.1 variant was predominant in the region between December 2021 and March 2022, and a similar surge between April 2022 and September 2022 when the BA.2, BA.4, and BA.5 subvariants were prevalent.24 Similar patterns can be observed in the data available for the other sub-sewershed zones.
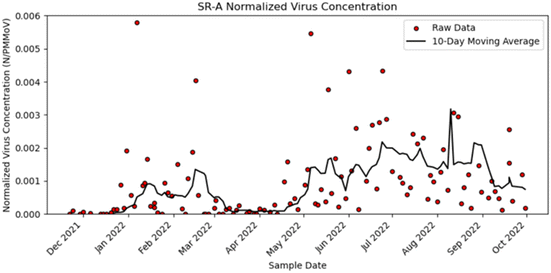 |
| Fig. 1 Normalized virus concentration for sub-sewershed zone A (SR-A). | |
Sub-sewershed zones
The wastewater treatment plant area serviced by the COD WWTP was divided into sub-sewershed zones, which were delineated according to Fig. 1 in Safford et al. (2022).17 While HDT conducted wastewater monitoring at both the sub-sewershed and building/neighborhood level, this study focuses on assessing trends across only the sub-sewershed zones because most of the building/neighborhood zones are encompassed within the sub-sewershed zones. We aimed at selecting zones that spatially covered as much of the city as possible with minimal to no overlap of census block boundaries. Sub-sewershed zones were defined by a list of census block geographic identifiers (GEOIDs), which are numeric codes that uniquely identify each census block.25 Each zone definition comprises several GEOIDs. For the geospatial analysis conducted in this study, significant overlap of census blocks was observed for some sub-sewershed zones when defining them as they were delineated in Safford et al. (2022).17 In these cases, the zones experiencing overlap were merged to form a larger sub-sewershed zone to prevent double counting populations for demographic analysis. This merging was performed by conglomerating the zone definitions for the smaller zones, and keeping all unique GEOIDs in the new, larger sub-sewershed zone definition. This is the case for SR-B (which merges SR-B1, SR-B2, SR-B3, and SR-B4), SR-C (merges SR-C1, SR-C2, and SR-C3), and SR-F (merges SR-F1 and SR-F2). The maintenance holes associated with each zone were then assigned to the overarching sub-sewershed zone from which the wastewater samples were being collected. The sub-sewershed zones and maintenance hole sampling sites are illustrated in Fig. 2 below. These zones show how wastewater contributions from different parts of the city are isolated at the sub-city scale. The sampling locations (brown) can be seen atop the census blocks (lines). We then aligned the delineated zone boundaries with the respective census block boundaries across the city to be able to compare the demographics of the people residing in each zone.
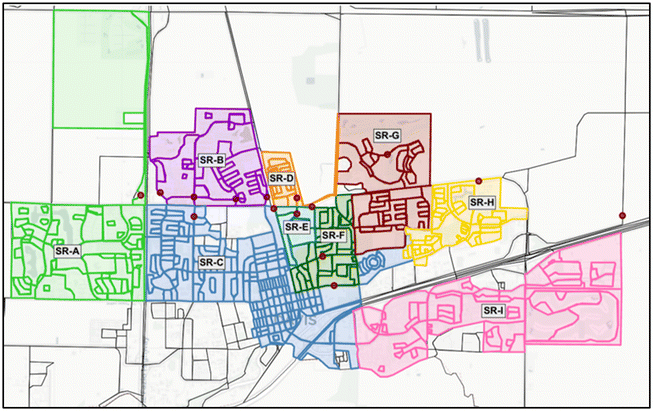 |
| Fig. 2 Map of the nine sub-sewershed zones for the SARS-CoV-2 wastewater-based epidemiology efforts in Davis (census blocks outlined). | |
Demographic data
Demographic data was gathered from the U.S. Census Bureau (USCB), which includes census-block-level population data from 2020.26 To prepare the census data for analysis, we filtered the data to include only the census blocks within the COD, in addition to two areas within the catchment area that are designated as spheres of influence and thus should be included in our analysis.27 We intersected the respective sub-sewershed zone areas with the census block boundaries. The total population within each sub-sewershed zone is listed in Table S4.†
Because of the high spatial granularity of census-block-level data, it is important to note that only certain demographic factors had sufficient data available for this analysis. Additionally, increased margins of error were reported in the census data collected in 2020 due to the difficulty of collecting responses during the COVID-19 pandemic.28 Consequently, this study focuses on the following two demographic factors: race and age. No other demographic variables were available in the 2020 census data at the block level during the time of our analysis.
The tabular census data was grouped according to the categories specified on the California Department of Public Health (CDPH) Health Equity Dashboard.29 The census data presenting the racial composition of each census block was filtered to include the following seven groups: White, Black or African American, American Indian and Alaska Native, Asian, Native Hawaiian and Other Pacific Islander, Other, and Multi-Race. The census data presenting the composition of people by age in each census block was grouped into the following ten age categories: less than 5 years, 5–17 years, 18–34 years, 35–49 years, 50–59 years, 60–64 years, 65–69 years, 70–74 years, 75–79 years, and greater than or equal to 80 years.
All analyses were performed using Python version 3.11.5 (the Python script used for implementation is available at https://tinyurl.com/HealthEquityWBE).
Probabilistic assignment tool
We adapted Python code from Safford et al. (2022)17 to combine information on municipal wastewater flows (provided as a File Geodatabase by the City of Davis Public Works Department) with census data. The resultant probabilistic assignment tool assigns census data to sewershed sampling zones based on geospatial probability. Each sampling zone spans several census blocks and may have different boundaries than the census blocks (e.g., in the case of overlap at neighboring zone boundaries). The probabilistic assignment tool performs the following tasks. First, the geospatial coordinates of all the MHs in the Davis sewer system and information about the relative upstream or downstream position of each MH were used to build a “connection graph” capturing directional connections among all the MHs. Second, the 2020 USCB population data was used to estimate the number of people living in each census block within the sampling zone of interest. Populations were spatially analyzed by age and race. Several key assumptions were made when using this tool. We assumed that each person in each census block produces the same amount of wastewater every day regardless of demographics, and that each person has an equal probability of discharging that wastewater to each MH within the block. We used the connection graph to probabilistically assign demographic data from census blocks to sewershed nodes. Thus, we extrapolated information from the census block level to each sub-sewershed zone. See Fig. 3 for an illustrative example of this approach.
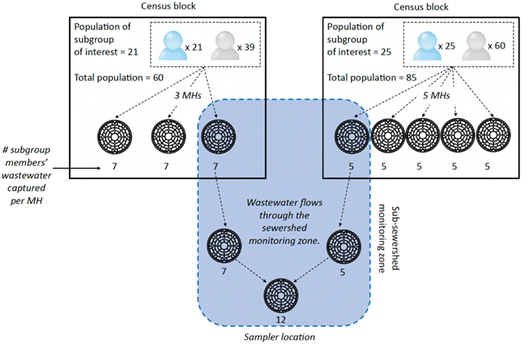 |
| Fig. 3 Simplified illustration of the probabilistic assignment method adopted from Safford et al. (2022),17 demonstrating how demographic data is distributed from a census block to a sub-sewershed zone. | |
Fig. 3 illustrates how the locations of the MHs in the COD sewershed can be used to probabilistically assign demographic data from census blocks to sub-sewershed monitoring zones, whereas Safford et al. (2022)17 probabilistically assigned clinical case count data to sampling zones. This approach allows us to assess whether sampling locations were chosen in a way that appropriately represents subgroups within the overall population. The probabilistic assignment determines the expected subgroup population members whose wastewater is captured by sampling at a specific location. In the example outlined in Fig. 3, the sampler location depicted at the bottom covers a sub-sewershed monitoring zone that spans two census blocks. The census block on the left has a total population of 60, of which 21 individuals belong to the subgroup of interest (e.g., >65 year olds). There are three MHs in this census block. The census block on the right has a total population of 85, of which 25 individuals belong to the subgroup of interest. There are five MHs in this census block. The tool's objective is to determine a predicted number of subgroup members captured by each MH in the census block. To calculate this value, we divided the subgroup population by the number of MHs, under the assumptions noted above. In the case of the census block on the left, we divided the 21 individuals' wastewater contributions across all three MHs, which results in a value of seven. This value is the probabilistically assigned subgroup population represented by a sample taken at a given MH in the census block on the left. For the census block on the right, we split the 25 individuals' wastewater contributions across all five MHs, resulting in a probabilistically assigned value of five individuals. The wastewater flow was tracked through the dataset containing the directional connections between all the MHs in the city and summed at the sampler location. In this example, we obtained a predicted population of 12 people who belong to the subgroup of interest. Note that this is a simplified representation of the methodology behind the probabilistic tool. It is possible to obtain decimal resultant values using this method, but tool outputs can be rounded to whole numbers and still provide meaningful insight.
The output of the probabilistic tool for a single run can be generated in the format of a summary table, shown in Table 2. The table displays the number of community members from the subgroup of interest who are represented by the sample taken at a node under the given sampling zone boundaries. Additional columns denote sampling nodes by their MH identification (ID) number, and additional rows of the output table represent data from additional census blocks within the city. The values within each column under a MH ID number are the probabilistically assigned subgroup populations represented by the wastewater sample. We ran the probabilistic distribution tool for each of the seven racial groups and each of the ten age categories. Then, the output table was filtered for the census blocks within each sub-sewershed zone to be able to calculate summary statistics by sub-sewershed zone rather than discrete census blocks.
Table 2 Example outputs from the probabilistic assignment tool for each sewershed node (truncated for visualization purposes)
Census block |
Total subgroup population |
Sewershed node |
M16-011 |
N13-045 |
N11-062 |
N11-072 |
N12-066 |
O13-002 |
O20-001 |
… |
061130104012002 |
13 |
0 |
0 |
0 |
0 |
0 |
0 |
13.0 |
… |
061130107041016 |
8 |
0 |
4.0 |
0 |
0 |
0 |
4.0 |
0 |
… |
… |
… |
… |
… |
… |
… |
… |
… |
… |
… |
We then compared the results of the probabilistic assignment tool to “manually derived” subgroup populations for each sub-sewershed zone. We obtained the manually derived population values by visually assigning the census-reported subgroup population value to a given census block under the delineated zone boundaries. We calculated the absolute percent difference (APD) between the probabilistically assigned subgroup population value and the manually derived subgroup population value using the following equation:
| 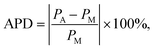 | (2) |
where
PA is the probabilistically assigned population value of a subgroup within a sub-sewershed zone, and
PM is the manually derived population value of a subgroup within a sub-sewershed zone.
Sub-sewershed zone and COD WWTP alignment
The following strategy was applied to aggregate sub-city level wastewater data to the city level. First, a set of population-weighted moving average values (PWMA) was calculated for all fifteen sub-sewershed zones. This was done by multiplying the 10-day trimmed moving average N/PMMoV values by the sub-sewershed zone population and dividing those values by the total city population. These PWMA values were then plotted, as can be seen in Fig. 4. A city-aggregated N/PMMoV metric was determined by summing the PWMA values for the sub-sewershed zones and creating a set of combined PWMA values. These values were then plotted against the unweighted COD WWTP moving average values (see Fig. 5). Note that, in this summation, SR-C3 was not included since it is encompassed within the SR-C2 sub-sewershed zone. To assess the strength of the correlation between the combined sub-sewershed values and the city-level wastewater data, we calculated and reported the Spearman's rank correlation coefficient. This metric was chosen because it measures strength and direction of any monotonic relationship rather than solely a linear one.30
 |
| Fig. 4 Population-weighted, 10-day right-aligned trimmed moving average of the normalized SARS-CoV-2 concentration in wastewater (N/PMMoV) for each sub-sewershed monitoring zone in the study area. | |
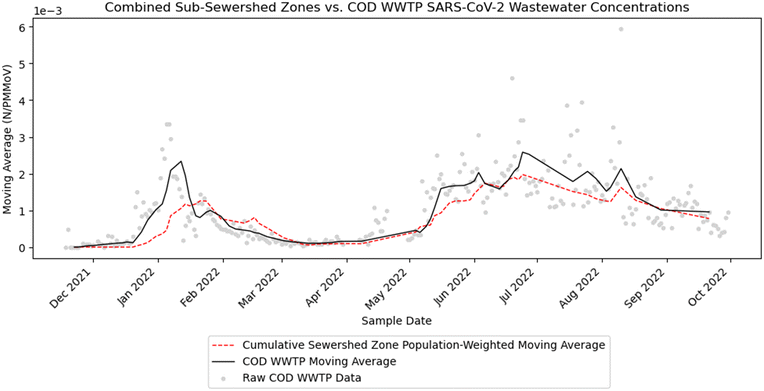 |
| Fig. 5 Aggregated wastewater data from the City of Davis sub-sewershed zones using a right-aligned population-weighted moving average (red, dashed line) and wastewater data from the City of Davis WWTP using a right-aligned moving average (black, solid line). | |
To quantify the error associated with the cumulative population-weighted moving average values, we calculated the mean absolute error (MAE) for each sub-sewershed zone's set of population-weighted moving averages, using the following equation:
| 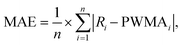 | (3) |
where
Ri is the raw wastewater data value for a given sub-sewershed zone, PWMA
i is the associated population-weighted moving average value for the zone, and
n is the total number of data points. Since these resultant error values are then added together to generate the cumulative population-weighted moving average values, error propagation must be considered.
31 The additive formula for error propagation was used to combine these uncertainties:
|  | (4) |
where MAE
i is a given mean absolute error value in the set of error values for a sub-sewershed zone.
Scenario planning construct
We consider four artificial conditions that would necessitate scale-back of the number of sampling sites compared to baseline. We define baseline as the sampling framework established for this study, which covers a population of 63
826 (95.5% of the census-reported total population in 2020). Scenarios 1 and 2 evaluate impacts for a minor (∼25%) reduction in the number of sampling nodes, while scenarios 3 and 4 evaluate a reduction of sampling nodes by approximately 50%. In scenarios 1 and 3, the sampling sites removed were chosen at random. In scenarios 2 and 4, the sampling sites removed were selected such that the coverage (total subgroup population in the included zones divided by the total subgroup population in Davis) of the >65-year-old population was prioritized. In other words, the nodes that were removed corresponded to sub-sewershed zones with the fewest number of >65-year-olds. For each scenario we determined: the percentage of the total city population covered by the scenario, the percentage of the >65-year-old subgroup population covered by the sampling regime, the percentage of Black or African American subgroup population covered under that same regime, and a Spearman's rank correlation coefficient that reports the strength of correlation between the aggregated wastewater signals for the included zones (PWMA values) and the COD WWTP signals.
Results and discussion
Demographics of sub-sewershed zones
An important aspect of considering health equity in the design of sub-city sampling strategies for wastewater monitoring includes evaluating the percent of subgroup populations represented by a set of sub-sewershed zones selected. Yet demographic data needed to perform this assessment are available for census blocks, which do not directly align with sub-sewershed zones. We compare two approaches—one manual and one probabilistic—to determine subgroup population demographics in sub-sewershed zones using census block data as inputs. The absolute percent difference for the two approaches for each sub-sewershed zone in the City of Davis are listed in Tables 3 and 4. The APD values serve as a metric for detecting differences between the subgroup population demographics generated manually by visual inspection and the subgroup population values generated using probabilistic assignment within each sub-sewershed. An APD close to 0% indicates that there is minimal difference between the two approaches when devising a monitoring strategy. High APDs suggest that there are large boundary effects for the subgroup population of interest. In these cases, population distributions overlapping multiple census blocks should be more carefully considered when evaluating the overall coverage of subgroup populations in a city's sampling strategy.
Table 3 Absolute percent differences (APDs) between probabilistic and manual assignment of census block data for race into sub-sewershed zones. Cells are shaded gray to flag when the APD exceeded 30%
Table 4 Absolute percent differences (APDs) between probabilistic and manual assignment of census block data for age into sub-sewershed zones. Cells are shaded gray to flag when the APD exceeded 30%
We assessed the APD values spatially (sub-sewershed zones) and by demographics (race and age). APDs values across all sub-sewershed zones in Davis for race categories (Table 3) ranged from approximately 0% to 43%. Relatively low percentage differences were observed by race category in SR-A, SR-D, and SR-I, while SR-B and SR-E showed consistently higher APD values. APDs across all sub-sewershed zones in Davis for age categories (Table 4) ranged from approximately 0% to 60%. Relatively low percent difference values were observed in SR-A, SR-D, SR-H, and SR-I. Consistently higher APD values were observed in SR-B, SR-C, and SR-E.
Greater percent differences were observed more often for minority subgroup populations compared to White populations. This highlights how boundary effects can be significant for subgroups present in low absolute numbers. In these cases, some corrections may need to be made for census block populations at sub-sewershed zone boundaries. This could be done by intersecting census data with unique boundaries (i.e., the sub-sewershed zone boundaries) and using apportionment to divide the census block population between two neighboring zones. However, the accuracy of apportioning area would be challenging to validate. Low-population zones (e.g., SR-E) will tend to exhibit high APDs when small absolute changes in zone reassignment lead to a large percent change. The higher populous zones (e.g., SR-A) tended to yield low APDs overall. Locally relevant infrastructure conditions can help to interpret in congruencies and modify planning strategies for sampling designs. For instance, zone SR-D showed no differences between the probabilistic assignment and manual derivation methods (APD = 0%) but also has the lowest total population amongst the seven zones. SR-D the area encompasses a relatively new development with distinct, non-overlapping census blocks. We selected to use the probabilistic assignment tool to assess the representation of subgroup populations across Davis under different planning scenarios.
Sub-sewershed zone and WWTP data alignment
In the selection of sewershed nodes for wastewater-based disease surveillance, a decision-maker may seek to consider how disease dynamics in sub-sewershed zones compare to overall city disease trends. Sampling frameworks could then be adapted, for example, to capture sub-sewershed zones with highly differing disease dynamics than the city overall. Alternatively, sampling frameworks that adequately capture overall trends for the city may be prioritized. Here, we evaluate whether wastewater surveillance data collected at the sub-sewershed level in Davis correlated in aggregate with the COD WWTP wastewater surveillance data. We then assess how the correlations would be impacted when downscaling to fewer nodes in an example scenario planning exercise for adaptive sampling. Analysis of this type can be useful to identify anomalies at the sub-city scale relative to the city-level data, revealing potentially important health vulnerabilities.
Individual sub-sewershed zones exhibited variability in the magnitude and timing of wastewater signals (Fig. 4). As expected, the propagated error for the aggregated sub-sewershed zone data (6.48 × 10−3) was much higher than that of the COD WWTP moving averages (3.60 × 10−4) due to the greater number of operations performed to generate the data. Nevertheless, the cumulative population-weighted mean average of the sub-sewershed zones exhibited similar magnitudes and patterns compared to the COD WWTP moving average wastewater data (Fig. 5). While the population in Davis is known to fluctuate during the summer and early winter months, due to the movement of students in and out of the city, there was no clear impact of these mobility trends on the correlations between sub-sewershed and city-wide wastewater data. The Spearman's rank correlation coefficient over the study period was 0.909, with a statistically significant positive correlation (p-value of 5.88 × 10−28). We also calculated a Spearman's rank correlation coefficient between each sub-sewershed zone and the COD WWTP (Table S5†). Correlation coefficients ranged from approximately 0.732 to 0.935, and all p-values were less than 0.05. This suggests that many combinations of sub-sewershed zones may offer reasonable representation of wastewater disease dynamics at the city-level. Moreover, results for wastewater data at the sub-sewershed level indicate that the data can provide utility when alerting health professionals to potential surges. For instance, wastewater data from four sub-sewershed zones (SR-A, SR-B3, SR-C1, and SR-C2) tended to rise earliest amongst all the zones, pointing to potential regions for early health interventions. Greater fluctuation in sub-sewershed zone data compared to city-level aggregated results provides finer resolution when tailoring local interventions. In fact, HDT actively utilized wastewater data from sub-sewershed zones at the time of the data collection to target communications as a component to a multi-faceted strategy for precision public health.16,17
Scenario planning for adaptive sampling
We designed the baseline scenario of sub-sewershed sites in this study to capture wastewater from as much of the sewershed as possible, while providing sufficient resolution within the city boundaries to inform public health communication interventions. This objective achieved 95.5% coverage of the population overall, 86.9% coverage of the >65-year-old population, and 82.9% coverage of the Black or African American populations within the city. Future wastewater-based disease surveillance efforts—whether ongoing or launched in response to emerging health threats—may require operation under resource-constrained conditions (e.g., logistical or economic) that limit the number of sampling nodes. Here we offer a scenario-planning example of how subgroup population assignment and wastewater data aggregation can be used to consider equity in the design of sub-sewershed sampling strategies for a city operating under such resource constraints.
Downscaling sampling may impact the representativeness of the data to the city overall, introducing tradeoffs between resource constraints and equitable representation of population subgroups. In this example, we stratified the sub-sewershed sampling by age, retaining sites to over-sample the high-risk population of individuals ages 65 and older. We evaluated the impact of this prioritization scheme on the representation of two subgroups disproportionately impacted by COVID-19 (elderly populations and Black or African American populations). In each of 4 scenarios considered, we evaluate the impact of sampling node selections on: (1) the representation of the >65-year-old population (who comprise about 14.5% of the COD population), (2) the representation of the Black or African American populations (who comprise about 2.31% of the COD population), and (3) correlations between aggregated sub-sewershed zone wastewater data with city-level data.
In scenarios 1 and 2, we examined the city-level effect of artificially pausing sampling at approximately 25% of the sampling sites, reducing the number of nodes from 15 to 11. In scenario 1, we removed nodes at random (SR-A, SR-D, SR-F1, and SR-F2 removed). The resulting coverage of the >65-year-old population decreased to 57.5%. The coverage of the Black or African American population declined to 70.3%. For comparison, scenario 2 maximized coverage of the >65-year-old population under the same resource constraints (SR-D, SR-E, SR-F1, and SR-F2 were removed). As expected, coverage of the >65-year-old population improved relative to scenario 1, to 80.5%. The coverage of Black or African American populations also improved in this scenario, though unexpectedly, to 89.7%. The aggregated wastewater signal in both scenarios was minimally affected by the exclusion of sampling nodes. Spearman's rank correlation coefficients of the aggregated wastewater signals from sub-city zones in each scenario relative to the COD WWTP moving average remained high and significant (Table 5), reflecting minimal change from baseline.
Table 5 Scenario planning example to adapt sampling strategies under resource constraints and differing priorities
Scenario |
Description |
% of total city population represented |
% of >65-year-old subgroup population covered |
% of Black or African American subgroup population covered |
Wastewater signal correlation (p-value) |
Baseline |
Includes all sewershed nodes monitored in this study |
95.5% |
86.9% |
82.9% |
0.909 (5.88 × 10−28) |
1 |
Baseline minus 25% of sites (randomly selected) |
69.1% |
57.5% |
70.3% |
0.907 (1.19 × 10−27) |
2 |
Baseline minus 25% of sites (prioritized to maximize coverage of >65-year-old population) |
84.9% |
80.5% |
89.7% |
0.900 (1.19 × 10−26) |
3 |
Baseline minus 50% of sites (randomly selected) |
61.8% |
51.1% |
67.5% |
0.875 (1.87 × 10−23) |
4 |
Baseline minus 50% of sites (prioritized to maximize coverage of >65-year-old population) |
70.5% |
67.2% |
76.7% |
0.917 (2.83 × 10−29) |
In scenarios 3 and 4, we examined the effect of pausing sampling at approximately 50% of the sampling sites, reducing the number of nodes from 15 to 9. A random selection of nodes in scenario 3 (SR-B1, SR-B2, SR-B3, SR-B4, SR-H, and SR-I removed) corresponded to a decline in the coverage of the >65-year-old population to 51.1%. The coverage of the Black or African American population decreased to 67.5%. In scenario 4, which once again maximized coverage of >65-year-old populations (SR-D, SR-E, SR-F1, SR-F2, SR-G, and SR-H removed), coverage of both >65-year-olds (67.2%) and Black or African American individuals (76.7%) improved relative to scenario 3. For both scenarios, the Spearman's rank correlation coefficients of the aggregated wastewater signals from sub-city zones relative to the COD WWTP moving average remained strong and significant. Notably, the aggregated wastewater signal in scenario 4 correlated most strongly with the city overall amongst all scenarios considered. This finding suggests that the zones removed in scenario 4 deviated from the city-level results during the study period.
Our scenario planning example, though rather simplistic in nature, demonstrates a strategy for assessing the impact of sampling design decisions on the coverage of vulnerable populations. As expected, demographic comparison revealed that greater coverage of >65-year-olds is achieved when scale-back of sampling is methodical rather than random. We illustrate how tailoring the sampling plan to prioritize one vulnerable population impacts another, and we recommend that decision-makers consider potential impacts of prioritization schemes on subsequent health intervention strategies. After all, prioritization of certain vulnerable groups may lead to underrepresentation of others. To balance these competing priorities in real-world settings, public health agencies may refer to general trends in wastewater surveillance data in a sampling area to identify areas of high virus concentration, irrespective of demographic factors. Another way to contend with this impact may be to utilize a combination of this study's approach and the conventional approach of placing sites for maximal spatial coverage. Randomly selecting sample sites to remove may be appropriate situationally, especially if the scale-back efforts are modest (e.g., removing less than 25% of sites) and impacts on data equity are evaluated. In our study, removal of sub-city zones had little effect on aggregated wastewater signal correlations with data collected directly at the centralized wastewater treatment plant. Site-specific variations in the timing and magnitude of wastewater virus concentrations were observed, illuminating the utility of sub-sewershed monitoring to inform targeted interventions. In any scenario, it remains important to evaluate the impact of prioritization schemes across multiple demographics and disease dynamics, to assess how these changes will impact a precision public health approach. When contending with health disparities, wastewater disease surveillance can support a precision public health approach for greater health equity.
Decision-makers hold the ultimate responsibility to determine whether the coverages of varying demographics under different sampling scenarios are sufficiently representative of the city population. When making decisions related to improved health interventions within a city, it is simultaneously important to avoid targeting specific populations and creating additional stigmas associated with disease transmission.1 Decision-makers must balance perspectives of officials at the city and county levels, health and medical professionals from public and private organizations, and other diverse stakeholders. Stakeholders may factor in the demographic representation fluctuations in specific sub-sewershed zones into decision-making instead of opting for the highest subgroup population percentage at the city level.
Limitations
We acknowledge several limitations to this study and approach. First, the approach relies on the availability and integrity of the underlying census data. As noted previously, few demographic factors had sufficient data available for our analysis at the block level, and increased margins of error were reported for the 2020 census data.28 Although there was not a statistically significant overcount or undercount for the total population, some historically undercounted groups were underrepresented, including children and older adults.32 Undercounting at-risk populations for COVID-19 may lead to inaccuracies in subgroup population estimates made by the probabilistic distribution tool. Cross-validation with alternative data sources and local knowledge may improve results. Adaptations may include inclusion of schools or long-term care facilities, where there are known higher populations of certain demographics. The Census Bureau has also incorporated statistical noise into its datasets in its efforts to implement differential privacy.33 Noise infusion is beneficial for addressing privacy concerns, as it maintains the essence of the data while ensuring that the data cannot be traced to an individual. However, noise infusion may have introduced uncertainties in our probabilistic assignment of census block data to sewershed zones. We are unable to evaluate the magnitude of this impact on our results or validate the probabilistic approach due to data privacy required for greater spatial resolutions. When working with census data, especially at its most granular level, privacy protection is crucial to help maintain public trust and to avoid reinforcing health disparities.
Second, we note that our approach may need to be modified when extended to other locations, as differences will arise from regional variations. This study specifically examines monitoring a suburban town with institutional support for its rigorous wastewater testing program during the pandemic. Differences including sewer system layout and coverage, access to digitized sewer system data, and population density may necessitate modifications to the approach in other locations.
Third, this study does not account for the mobility of populations. Assignment of demographic metrics to wastewater data does not take into account differences between individuals' place of residency and place of work or study.8 Davis also has a large student population from the University. Demographics for sub-sewershed zones with more students may change more often than areas with long-term residents. Application of the approach outlined in this study to other regions would offer opportunities for cross-comparisons.
Fourth and finally, this study analyzes race and age separately, as though their distribution of impact is mutually exclusive. In reality, there are populations (e.g., Black or African American population above the age of 65) that are especially vulnerable to COVID-19. Evaluating health equity on the basis of single characteristics is inherently limiting and risks homogenizing marginalized groups.
Conclusions
Scenarios of sampling strategies for wastewater-based disease surveillance assessed in this study showcase the utility of sub-sewershed demographics analysis. Investigating sub-sewershed demographics and trends can reveal data gaps under varied sampling designs and foster more informed decision-making for within-city monitoring efforts. Characterizing demographics within sub-sewershed zones helps place wastewater trends in the context of the subgroup populations represented (e.g., higher age brackets and/or non-White populations). Analysis of demographics can also inform adaptive selection of sampling locations to achieve more equitable representation and inclusivity of high-risk populations. The approach presented is applicable at varying scales of deployment for wastewater-based disease surveillance (e.g., building, neighborhood, city, county, or regional).
Several reasonable extensions of this project arise as we continue to understand community transmission of infectious diseases using wastewater. Health disparities are driven by a combination of many factors, including socioeconomic status, healthcare coverage, immigrant status, native language, and educational attainment. Future analyses should consider intersections amongst multiple community attributes using publicly available data. The American Community Survey, for instance, offers a breadth of demographic data at broader scales (e.g., census block group or tract).34 While the present study focuses on community transmission at the city-level, population representation should also be assessed at county and regional levels.12 For a well-balanced distribution of sampling nodes at broader geographic scales, optimization models can quantitatively consider parameters of interest, including the population served, spatial coverage, social vulnerability, and dissimilarity of wastewater signals.11,35 The methodology presented in the present study can also be applied to other diseases that have known or anticipated links to demographic factors. For example, higher morbidity is observed in young children for respiratory syncytial virus (RSV).36 In preparedness efforts, cities might develop a suite of sampling strategies in anticipation of varied disease transmission scenarios and high-risk groups.
A successful wastewater-based disease surveillance program should aim for equitable representation of vulnerable groups within the sampling design, while preserving the anonymity of the sampled populations. When sampling frameworks are designed appropriately, wastewater data can inform health interventions that address disparities faced by underserved communities. The probabilistic assignment approach demonstrated in this study offers a way to assess impacts of changes to sampling regimes at a sub-sewershed level, facilitating design amidst shifting priorities and variable conditions.
This study also demonstrates the utility of equity assessments in scenario planning for wastewater monitoring. Before a sampling effort is undertaken, the approach can be used to determine whether the sampling area includes zones with more vulnerable subgroup populations, and sampling zones can be adjusted to ensure collection at appropriate MHs. If a limited number of autosamplers can be deployed in an area, the procedure outlined in the study could be used to determine favorable locations to optimize a chosen measure (e.g., capture a larger proportion of the population over the age of 65, who are more vulnerable to serious illness from COVID-19). Sampling locations can then be chosen strategically while accounting for different urban contexts, such as sewer system layout, MH access points, and population density. Ultimately, using this approach can help stakeholders adapt their sampling strategies to the local landscape, supporting incorporation of equity-centered public health interventions.
Data availability
Data for this article, including the code used to generate the outputs analyzed in this study, are available at https://tinyurl.com/HealthEquityWBE.
Author contributions
Conceptualization, H. N. B. and A. M.; methodology, H. N. B., R. O., and C. W. B.; investigation, H. N. B., R. O., C. W. B., and A. M.; writing – original draft, A. M. and H. N. B.; writing – review and editing, H. N. B., R. O., C. W. B. and A. M.; funding acquisition, H. N. B.; resources, H. N. B; supervision, H. N. B.
Conflicts of interest
There are no conflicts to declare.
Acknowledgements
This research was conducted on land that has been the home of Patwin people for thousands of years. Today, there are three federally recognized Patwin tribes: Cachil DeHe Band of Wintun Indians of the Colusa Indian Community, Kletsel Dehe Wintun Nation, and Yocha Dehe Wintun Nation. We are honored and grateful for the stewardship of this land by the Patwin people over many centuries. Wastewater-based disease surveillance in Davis, CA and associated research was funded through Healthy Davis Together (HDT) and led by Professor Heather Bischel at the University of California, Davis. This research also received support from the UC Davis/UC Merced Wastewater Epidemiology Center of Excellence, which is funded by the NIH Rapid Acceleration of Diagnostics (RADx) initiative (Contract No. 75N92021C00012 to Ceres Nanosciences, Inc). We greatly appreciate the dedication of the many City of Davis staff who were critical to the implementation of wastewater surveillance in Davis during the pandemic. We also acknowledge the contributions of H. Safford to sampling design, coordinating sample collection, and data management, and of L. Rueda for lab analysis.
References
- R. H. Holm, N. Osborne Jelks, R. Schneider and T. Smith, Beyond COVID-19: Designing Inclusive Public Health Surveillance by Including Wastewater Monitoring, Health Equity, 2023, 7(1), 377–379, DOI:10.1089/heq.2022.0055.
- J. Heller, M. Givens, T. Yuen, S. Gould, M. Benkhalti and E. Bourcier,
et al., Advancing Efforts to Achieve Health Equity: Equity Metrics for Health Impact Assessment Practice, Int. J. Environ. Res. Public Health, 2014, 11, 11054–11064, DOI:10.3390/ijerph111111054.
- J. Mandelbaum, Advancing health equity by integrating intersectionality into epidemiological research: applications and challenges, J. Epidemiol. Community Health, 2020, 74(9), 761–762, DOI:10.1136/jech-2020-213847.
- R. H. Holm, G. Pocock, M. A. Severson, V. C. Huber, T. Smith and L. M. McFadden, Using wastewater to overcome health disparities among rural residents, Geoforum, 2023, 144, 103816, DOI:10.1016/j.geoforum.2023.103816.
- CDC. Centers for Disease Control and Prevention, 2020, [cited 2023 Nov 4]. Healthcare Workers. Available from: https://www.cdc.gov/coronavirus/2019-ncov/hcp/clinical-care/underlyingconditions.html.
- COVID-19 Death Data and Resources - National Vital Statistics System [Internet], 2023, [cited 2023 Nov 4]. Available from: https://www.cdc.gov/nchs/nvss/covid-19.htm.
- K. J. G. Cheng, Y. Sun and S. M. Monnat, COVID-19 Death Rates Are Higher in Rural Counties With Larger Shares of Blacks and Hispanics, J. Rural Health, 2020, 36(4), 602–608, DOI:10.1111/jrh.12511.
- K. Kadonsky, C. Naughton, M. Susa, R. Olson, G. Singh and M. Daza-Torres,
et al., Expansion of wastewater-based disease surveillance to improve health equity in Californias Central Valley: sequential shifts in case-to-wastewater and hospitalization-to-wastewater ratios, Front. Public Health, 2023, 11 DOI:10.3389/fpubh.2023.1141097.
- CDC. Centers for Disease Control and Prevention, 2023, [cited 2023 Nov 5]. National Wastewater Surveillance System. Available from: https://www.cdc.gov/nwss/wastewater-surveillance.html.
-
Bureau UC, Census.gov. [cited 2024 Jan 29]. What are census blocks? Available from: https://www.census.gov/newsroom/blogs/random-samplings/2011/07/what-are-census-blocks.html.
- R. Yeager, R. H. Holm, K. Saurabh, J. L. Fuqua, D. Talley and A. Bhatnagar,
et al., Wastewater Sample Site Selection to Estimate Geographically Resolved Community Prevalence of COVID-19: A Sampling Protocol Perspective, GeoHealth, 2021, 5(7), e2021GH000420, DOI:10.1029/2021GH000420.
- C. Y. Medina, K. F. Kadonsky, F. A. Roman, A. Q. Tariqi, R. G. Sinclair and P. M. D'Aoust,
et al., The need of an environmental justice approach for wastewater based epidemiology for rural and disadvantaged communities: A review in California, Curr. Opin. Environ. Sci. Health, 2022, 27 DOI:10.1016/j.coesh.2022.100348.
-
National Academies of Sciences, Engineering, and Medicine, Wastewater-based Disease Surveillance for Public Health Action, The National Academies Press, Washington DC, 2023, DOI:10.17226/26767.
-
X. C. Hu, S. K. Reckling and A. Keshaviah, Assessing health equity in wastewater monitoring programs: Differences in the demographics and social vulnerability of sewered and unsewered populations across North Carolina, medRxiv, 2023, preprint, DOI:10.1101/2023.10.06.23296680.
- U.S. Census Bureau QuickFacts: Davis city, California [Internet]. [cited 2023 Oct 23]. Available from: https://www.census.gov/quickfacts/fact/table/daviscitycalifornia/PST045222.
- B. H. Pollock, C. L. Bergheimer, T. S. Nesbitt, T. Stoltz, S. R. Belafsky and K. C. Burtis,
et al., Healthy Davis Together: Creating a Model for Community Control of COVID-19, Am. J. Public Health, 2022, 112(8), 1142–1146, DOI:10.2105/AJPH.2022.306880.
- H. Safford, R. E. Zuniga-Montanez, M. Kim, X. Wu, L. Wei and J. Sharpnack,
et al., Wastewater-Based Epidemiology for COVID-19: Handling qPCR Nondetects and Comparing Spatially Granular Wastewater and Clinical Data Trends, ACS ES&T Water, 2022, 2(11), 2114–2124, DOI:10.1021/acsestwater.2c00053.
- M. L. Daza-Torres, J. C. Montesinos-López, M. Kim, R. Olson, C. W. Bess and L. Rueda,
et al., Model training periods impact estimation of COVID-19 incidence from wastewater viral loads, Sci. Total Environ., 2023, 858, 159680, DOI:10.1016/j.scitotenv.2022.159680.
- M. A. Borchardt, A. B. Boehm, M. Salit, S. K. Spencer, K. R. Wigginton and R. T. Noble, The Environmental Microbiology Minimum Information (EMMI) Guidelines: qPCR and dPCR Quality and Reporting for Environmental Microbiology, Environ. Sci. Technol., 2021, 55(15), 10210–10223, DOI:10.1021/acs.est.1c01767.
- Ceres Nano [Internet]. [cited 2024 Feb 7]. Ceres - Protocols. Available from: https://www.ceresnano.com/protocols.
- Introduction to Digital PCR | Bio-Rad [Internet]. [cited 2023 Oct 24]. Available from: https://www.bio-rad.com/en-us/life-science/learning-center/introduction-to-digital-pcr.
- M. A. I. Juel, N. Stark, B. Nicolosi, J. Lontai, K. Lambirth and J. Schlueter,
et al., Performance evaluation of virus concentration methods for implementing SARS-CoV-2 wastewater based epidemiology emphasizing quick data turnaround, Sci. Total Environ., 2021, 801, 149656, DOI:10.1016/j.scitotenv.2021.149656.
-
C. Li, M. Bayati, S. Y. Hsu, H. Y. Hsieh, W. Lindsi and A. Belenchia, et al., Population Normalization in SARS-CoV-2 Wastewater-Based Epidemiology: Implications from Statewide Wastewater Monitoring in Missouri, medRxiv, 2022, preprint, DOI:10.1101/2022.09.08.22279459.
- CDC. Centers for Disease Control and Prevention. 2023 [cited 2023 Nov 4]. CDC Museum COVID-19 Timeline. Available from: https://www.cdc.gov/museum/timeline/covid19.html.
-
Bureau UC, Census.gov. [cited 2024 Jan 11]. Understanding Geographic Identifiers (GEOIDs). Available from: https://www.census.gov/programs-surveys/geography/guidance/geo-identifiers.html.
- Census Bureau Data [Internet]. [cited 2023 Nov 3]. Available from: https://data.census.gov/.
- Planning and Zoning | City of Davis, CA [Internet]. [cited 2023 Nov 13]. Available from: https://www.cityofdavis.org/city-hall/community-development-and-sustainability/planning-and-zoning.
-
Bureau UC, Census.gov. [cited 2023 Nov 20]. Increased Margins of Error in the 5-Year Estimates Containing Data Collected in 2020. Available from: https://www.census.gov/programs-surveys/acs/technical-documentation/user-notes/2022-04.html.
- COVID-19 Age, Race and Ethnicity Data [Internet]. [cited 2023 Nov 14]. Available from: https://www.cdph.ca.gov/Programs/CID/DCDC/Pages/COVID-19/Age-Race-Ethnicity.aspx.
- Spearman Rank - an overview | ScienceDirect Topics [Internet]. [cited 2024 Jan 29]. Available from: https://www.sciencedirect.com/topics/engineering/spearman-rank.
-
Chemistry LibreTexts, [Internet], 2013, [cited 2023 Dec 11]. Propagation of Error. Available from: https://chem.libretexts.org/Bookshelves/Analytical_Chemistry/Supplemental_Modules_(Analytical_Chemistry)/Quantifying_Nature/Significant_Digits/Propagation_of_Error.
-
Bureau UC, Census.gov. [cited 2024 Sep 16]. Detailed Coverage Estimates for the 2020 Census Released Today. Available from: https://www.census.gov/library/stories/2022/03/who-was-undercounted-overcounted-in-2020-census.html.
- Disclosure Avoidance and the 2020 Census: How the TopDown Algorithm Works.
-
Bureau UC, Census.gov. [cited 2024 Feb 19]. American Community Survey 5-Year Data (2009-2022). Available from: https://www.census.gov/data/developers/data-sets/acs-5year.html.
-
M. L. Daza-Torres, J. C. Montesinos-López, C. Herrera, Y. E. García, C. C. Naughton, H. N. Bischel and M. Nuño, Optimizing Spatial Distribution of Wastewater-Based Disease Surveillance to Advance Health Equity, medRxiv, 2024, preprint, DOI:10.1101/2024.05.02.24306777v1.
- H. Brenes-Chacon, M. Eisner, S. Acero-Bedoya, O. Ramilo and A. Mejias, Age-specific predictors of disease severity in children with respiratory syncytial virus infection beyond infancy and through the first 5 years of age, Pediatr. Allergy Immunol., 2024, 35(2), e14083, DOI:10.1111/pai.14083.
|
This journal is © The Royal Society of Chemistry 2025 |
Click here to see how this site uses Cookies. View our privacy policy here.