Pathogen and indicator trends in southern Nevada wastewater during and after the COVID-19 pandemic†
Received
22nd July 2024
, Accepted 15th October 2024
First published on 13th December 2024
Abstract
Characterization of wastewater concentrations of human enteric pathogens and human fecal indicators provides valuable insights and data for use by regulators and other stakeholders when developing treatment criteria for water reuse applications, performing quantitative microbial risk assessments, or conducting microbial source tracking. Wastewater samples collected over three years during and after the COVID-19 pandemic were analyzed retrospectively (March 2020–September 2022) and prospectively (October 2022–December 2023) by qPCR for molecular markers of adenovirus, enterovirus, norovirus GI & GII, as well as the human fecal indicators pepper mild mottle virus, crAssphage, and HF183 (n = 1112). A sub-campaign was conducted, and wastewater samples were tested for the culturable enteric viruses adenovirus and enterovirus (n = 56) and the protozoan parasites Cryptosporidium and Giardia (n = 73) over one year (January–December 2023). All assays had high detection rates, ranging from 71% to 100%, and were fit to log-normal distributions. All molecular markers for enteric pathogens displayed seasonal and geographic variation, potentially explained by seasonal epidemiology of gastrointestinal illness, differing populations, and differing sample types. Additionally, the impact of Nevada-specific COVID-19 public health guidance (e.g., mask mandates, stay-at-home orders) on enteric pathogen concentrations was characterized, with significantly higher concentrations of molecular markers observed in “non-pandemic” conditions. This study provides high quality (i.e., high sensitivity, minimally censored, recovery adjusted) pathogen and indicator datasets with insights for use in academic, public health/epidemiological, and industry/regulatory applications.
Water impact
Wastewater samples collected over three years were analyzed for a variety of viral and protozoal pathogens and human fecal indicators to establish statistical distributions of concentrations. Pandemic conditions, seasonality, sample biobanking, and sample type all had various impacts on target concentrations with implications for use in risk assessments, regulatory rule setting, and in microbial source tracking applications.
|
1. Introduction
Understanding microbial constituents of wastewater and their variable concentrations provides valuable data and insights for diverse applications, including water reuse, wastewater-based epidemiology (WBE), quantitative microbial risk assessment (QMRA), microbial source tracking (MST), and more. Wastewater has gained renewed attention as a tool for understanding the spread and prevalence of disease within communities through wastewater surveillance and WBE, with a focus that now extends beyond SARS-CoV-2 to include other respiratory viruses,1 enteric pathogens,2–5 nosocomial fungal pathogens,6,7 sexually transmitted diseases,8–12 markers of antimicrobial resistance,13 high risk substances,14 and other emerging threats.1,8 One of these programs, WastewaterSCAN, represents one of the largest repositories of wastewater data across the United States, with a publicly available web interface to facilitate communication of wastewater data for public health applications.15 Characterizing the concentrations and variability of microbial constituents (including human fecal indicators) in raw wastewater is also particularly useful for identification and tracking of fecal contamination sources in environmental waters—an application known as MST.16,17
The integration of pathogen concentrations, exposure pathways, and treatment efficiency datasets can be used in QMRA studies for assessing risk from exposure to wastewater pathogens.18,19 Increasingly severe drought, particularly in the southwestern United States (U.S.), and global climate change have heightened awareness of recycled water as a valuable component of water resource portfolios. This includes indirect (IPR) or direct potable reuse (DPR). Robust pathogen concentration datasets in untreated or partially treated wastewater are necessary for determining overall treatment levels that are needed for safe implementation of potable reuse, and these datasets are instrumental in forming regulatory guidelines. Regulatory frameworks often emphasize worst-case assumptions (e.g., peak pathogen concentrations), resulting in potentially unsustainable capital and O&M costs for the resultant treatment paradigms. By better characterizing conditions and factors that lead to these worst-case scenarios, it is possible to respond to these conditions in near-real-time rather than resorting to excessive levels of advanced treatment aimed at mitigating low frequency, high consequence, and potentially site-specific events.
Reported concentrations of pathogens and human fecal indicators in wastewater are highly variable due to extensive external (e.g., geographic location, dilution, disease burden, socio-economic status20) and internal (e.g., quantification methods, intra-laboratory variation21) factors. Few studies have characterized the occurrence and variability of a wide set of human enteric pathogens and fecal indicators over extended periods of time in an effort to explain the drivers of these observed concentration ranges and variability.4,22–26 Notably, Water Research Foundation (WRF) project 4989, “Pathogen Monitoring in Untreated Wastewater”,27 set out to perform an extensive monitoring campaign and literature review to develop a combined dataset of pathogen concentrations in wastewater for use by California regulators in establishing log reduction value (LRV) targets for DPR. WRF 4989 identified two major limitations in wastewater pathogen occurrence studies that have hindered the application of wastewater data, particularly for regulatory purposes, though these limitations extend to applications beyond regulatory development. These limitations include the lack of sufficient analytical sensitivity, resulting in high levels of non-detects and non-quantifiable (i.e., highly censored) data, and the absence of appropriate recovery spike-ins and controls. Other limitations that impact large-scale wastewater studies include limited analytical scope or using only one enumeration method, such as viral cell culture, microscopy, or molecular methods, rather than combining multiple approaches. For understanding pathogen trends in wastewater on a broad scale, it is useful to integrate multiple methods to uncover findings that go beyond methods-related variability.
In this study, we leverage efforts related to a SARS-CoV-2 wastewater surveillance campaign in Southern Nevada to develop a comprehensive wastewater dataset of diverse pathogens and indicators. This dataset characterizes the concentrations of human enteric pathogens and human fecal indicators in raw wastewater over a three-year-long monitoring effort yielding over 1000 samples analyzed by qPCR and over 50 samples analyzed by both cell culture and microscopy methods. The sampling period spanned from 2020–2024, encompassing the peak impact of the COVID-19 pandemic. Herein, “pandemic” and “non-pandemic” conditions were delineated by Nevada's statewide COVID-19 Declaration of Emergency. The targets chosen reflect the potential use of these data for DPR regulatory development by including both molecular and culture-based enumeration of the enteric viruses adenovirus (AdV) and enterovirus (EnV), molecular data for norovirus (NoV) GI and GII, and microscopy-based enumeration of the protozoa Cryptosporidium and Giardia. Additionally, three human fecal indicator targets were included for MST and WBE applications: the RNA of pepper mild mottle virus (PMMoV), the DNA of Bacteroides phage crAssphage (Carjivirus communis28), and a DNA marker for human-specific Bacteroides (HF183). To ensure that our data meet high quality standards required for use in regulatory contexts, we implement extensive quality control following recommendations outlined by WRF 4989 and related publications29,30 and expand on them to develop additional quality control recommendations for future studies utilizing biobanked nucleic acid samples.
2. Methods
2.1 Study location
Wastewater samples were collected from the six major wastewater treatment plants (WWTPs) in the Las Vegas area in Southern Nevada, with samples representing eight different sewersheds of varying sizes (population and flow rate), sample type (composite and grab), and composition (demographics). Fig. 1 displays the geographic service area (sewershed) for each of the sampled facilities. The population served by these facilities is approximately 2.2 million residents across four incorporated cities (Las Vegas, North Las Vegas, Henderson, and Boulder City) and unincorporated Clark County, collectively representing 70% of the population of Nevada. In addition, facility 1 serves the Las Vegas Strip—a casino/resort entertainment corridor with ∼700
000 weekly visitors, the University of Nevada Las Vegas, with ∼28
000 students, and Harry Reid International Airport, which serves ∼55 million travelers annually.31
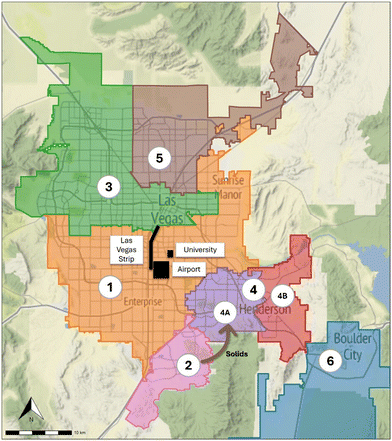 |
| Fig. 1 Map of the sewersheds (and their facility designations) sampled in this study. Facility 1 serves the Las Vegas Strip, a large international airport, and a university. Facility 4 was also subdivided into 4A and 4B via grab samples collected from individual sewer trunk lines entering the WWTP. Also, solids from facility 2 are transported to facility 4 via the 4A sewer trunk line. | |
2.2 Wastewater sampling
Wastewater was collected, processed, and stored as part of a SARS-CoV-2 wastewater surveillance program in Southern Nevada from March 2020 through December 2023.32,33 The surveillance program was initiated in March 2020 to provide data on the progression of the pandemic by measuring the quantity of SARS-CoV-2 genetic material in wastewater as a proxy for cases, but the samples collected have since provided an opportunity to measure additional pathogenic and human fecal indicator targets. A one-year monthly sub-campaign was conducted from January 2023 through December 2023, in which 100 mL of untreated wastewater was collected from the six WWTPs for Cryptosporidium and Giardia enumeration. Additionally, select samples during this sub-campaign were also analyzed by cell culture for detection and enumeration of viable AdV and EnV; this involved 1 L samples collected from the six WWTPs and two additional trunk line locations (facilities 4A and 4B). Table 1 provides a summary of the WWTPs, the respective sewersheds, and sample volumes, and Table S1 in the ESI† provides an inventory of total sample numbers by location and target/method.
Table 1 Summary of WWTP sampling locations and sample characteristics
Facility |
Population served |
Flow rate (mgd) |
Sample type and source |
Sample collection volume (mL) |
qPCR assays |
Protozoa assays |
Viral culture assays |
Facility 1 also serves ∼700 000 weekly visitors and ∼55 million airport travelers annually.
Facility 4 is the 24 hour composite of facility 4A (west trunk line) and facility 4B (east trunk line).
Facility 4A (and by default facility 4) receives solids and bypass flows from facility 2.
Grab influent samples were collected on 6/14/2021, 8/15/2022, 8/22/2022, and 8/29/2022 (otherwise composite).
|
1 |
872 009a |
100 |
Grab primary effluent |
10 000 |
NA |
1000 |
Grab influent |
NA |
100 |
NA |
2 |
86 330 |
5 |
Composite influent |
10 000 |
100 |
1000 |
3 |
757 418 |
42 |
Composite/grab influentd |
250 |
100 |
1000 |
4b |
N/Ab |
N/Ab |
Composite influent |
250 |
100 |
1000 |
4Ac |
133 977 |
15 |
Grab influent |
250 |
NA |
1000 |
4B |
114 532 |
6 |
Grab influent |
250 |
NA |
1000 |
5 |
255 008 |
20 |
Composite influent |
250 |
100 |
1000 |
6 |
16 399 |
0.8 |
Grab influent |
250 |
100 |
1000 |
2.3 Sample concentration and nucleic acid extraction
Wastewater samples were collected and concentrated for qPCR targets as previously described.32,33 For the viral targets, sample volumes (10 L vs. 250 mL), locations (influent vs. primary effluent), and types (composite vs. grab) were artifacts of the SARS-CoV-2 monitoring effort so they could not be changed for this study. In summary, 10 L samples were spiked with the recovery control bovine coronavirus (BCoV), amended with sodium polyphosphate, and concentrated using hollow fiber ultrafiltration (HFUF) (REXEED-25S, 30 kDa, Asahi Kasei Medical Co.) to approximately 100–200 mL. Then, the concentrates were centrifuged, the pelleted solids were discarded, and the supernatants were used for nucleic acid extraction. 250 mL samples were spiked with BCoV and concentrated with Centricon Plus-70 centrifugal filter units (100 kDA, EMD Millipore) to an approximate volume of 1 mL for subsequent nucleic acid extraction. Nucleic acid extraction was performed with PureLink Viral RNA/DNA Mini Kits (ThermoFisher Scientific) according to manufacturer's instructions. Several 10 L samples from early 2020 (n = 27) were concentrated with HFUF and Centricon units, but this combined process was eventually discontinued due to low BCoV recovery. Additional details are available in Table S2.†
2.4 Virus and fecal indicator qPCR analyses
Samples were separated into two categories – archived or fresh – depending on their collection and qPCR analysis time frame. Archived samples were originally collected between March 10, 2020, and September 26, 2022, and extracted nucleic acids from those samples were stored at −30 °C until they were re-analyzed for the new targets with a new cDNA synthesis in 2022. From October 3, 2022, to December 18, 2023, samples were considered fresh and were processed/analyzed within a week of collection using the same cDNA as the ongoing SARS-CoV-2 WBE campaign. Both fresh and archived nucleic acid extracts were subjected to one freeze–thaw prior to qPCR. Nucleic acid extracts were thawed at 4 °C, and cDNA was synthesized for RNA viral assays using the Maxima First Strand cDNA Synthesis Kit (ThermoFisher Scientific) according to manufacturer's instructions. Pathogenic virus assays included EnV,29,34 NoV GIA,29 NoV GIB,29,34 NoV GII,29,34 and AdV (targeting the enteric serotypes 40/41).29,35 Human fecal indicators, including pepper mild mottle virus (PMMoV),36 crAssphage (CP56),16 and HF183 (Bacteroides genus)37 were also quantified, alongside BCoV.32 Assays were run in triplicate on a CFX384 Touch Real-Time PCR Detection System or a CFX Opus 384 Real-Time PCR System (BIO-RAD Laboratories, Inc., Hercules, CA), and data were visualized with the CFX Maestro software. Reaction concentrations of primers and probes, as well as sequences, are listed in Table S3.† A standard curve was generated for every plate using gBlock Gene Fragments (Integrated DNA Technologies, Skokie, IL) (Table S4†). Starting quantities of each target were estimated from the instrument-provided Cq paired with a combined, study-wide standard curve (Text S1 and Fig. S1†), and then were divided by a sample-specific equivalent sample volume (ESV) (Table S2†) – original volume of sample assayed38 – to calculate the non-recovery-adjusted concentration (in log10 gene copies per liter) of each target in the original wastewater sample. Samples with target amplification in ≥2 replicates with an average Cq earlier than the limit of quantification (LoQ) (Table S5†) were considered detected and quantifiable. Samples with target amplification in ≥2 replicates with an average Cq later than the LoQ were considered detected but non-quantifiable, and samples with target amplification in ≤1 replicate were considered non-detects. Additional details for ESV calculations, recovery efficiencies, LoQs, and duplex assay optimization (Fig. S2, Text S2 and Table S6) are available in the ESI.†
2.5 Virus cell culture
A modified version of EPA Method 1615 (ref. 34) was used to concentrate and quantify enteric virus concentrations using cell culture.29 Approximately 108 plaque forming units (PFU) each of MS2 and phiX174 were added as matrix spikes to 1 L of wastewater. After a modified concentration/purification procedure involving PEG precipitation with chloroform extraction, a 10-fold dilution series was performed on the final sample concentrates, and aliquots were inoculated onto buffalo green monkey (BGM) kidney cells (provided by the USEPA) or human lung carcinoma epithelial cells (A549-CCL-185, purchased from ATCC, Manassas, Virginia) to enumerate culturable EnV and AdV, respectively. Ten sterile 25 cm2 canted neck polystyrene tissue culture flasks containing BGM cells and 10 flasks containing A549 cells were each inoculated with 0.1 mL of the target dilutions (100, 10−1, 10−2). The flasks were incubated at 36.5 ± 1 °C for 2 weeks in Dulbecco's modified Eagle's medium (DMEM) supplemented with antibiotics, antimycotics, and 2% fetal bovine serum (FBS). Cultures were examined microscopically for the appearance of cytopathogenic effects (CPE) daily for the first 3 days and then every 1–3 days for a total of 14 days. For both the EnV and AdV cell culture assay, second passages were completed for all flasks. The EPA MPN calculator39 was used to quantify the total culturable virus from both assays. A portion of the final sample concentrates were inoculated onto agar plates with E. coli CN-13 for phiX174 enumeration and with E. coli Famp for MS2 enumeration following a modified version of EPA Method 1602.40 Sample-specific recovery was determined as the average of MS2 and phiX174 recovery (mean = 34% ± 21%; min = 8%; max = 93%), with additional details available in Text S3.†
2.6
Cryptosporidium and Giardia
A modified version of EPA Method 1693 (ref. 29 and 41) was used to enumerate Giardia cysts and Cryptosporidium oocysts in samples collected monthly from six sewersheds from January 2023 to December 2023. First, a ColorSeed™ matrix spike (BioPoint USA, Pittsburgh, PA) containing approximately 100 fluorescently-labeled Giardia cysts and 100 fluorescently-labeled Cryptosporidium oocysts was added to each 100 mL sample. 100 mL was chosen over 1000 mL because the lower volume was found to generally contain sufficient organisms to avoid left-censored data, while also allowing for the entire pellet to be analyzed. Next, 5 mL of 20% Tween-80 was added, and the sample was mixed with a magnetic stir bar for 15 min. The sample was then centrifuged at 1500 × g for 15 min to concentrate the (oo)cysts into a pellet. The full pellet was purified using immunomagnetic separation (IMS) beads. 0.5 mL subsamples of pellets larger than 0.5 mL were added to each IMS tube for processing. No portions of pellets were discarded without analysis (i.e., the ESV was equal to the original sample volume). 0.25 mg kaolin was added to the tubes after adding the IMS beads and buffer. The (oo)cysts were dissociated from the beads by vortexing with hydrochloric acid (0.1 N). Two acid dissociations were completed per IMS tube, and both acid dissociations were plated onto a single slide well and left to dry overnight. Once dried, the slides were stained with 4′,6-diamidino-2-phenylindole (DAPI) and fluorescein isothiocyanate-labeled antibodies (FITC) and scanned with an epifluorescence microscope with FITC, DAPI, and Texas Red filter blocks to identify both spiked ColorSeed™ and native (oo)cysts. For each run, a method blank (MB), ongoing precision and recovery (OPR), and staining controls were used. The LoQ and LoD (limit of detection) were set to 1 (oo)cyst per 100 mL, as 100 mL was the volume analyzed. Recovery details are available in Text S3† (mean recovery = 55% ± 21% for Giardia and 31% ± 19% for Cryptosporidium).
2.7 Quality control and recovery efficiency
For molecular methods, an extraction control of nuclease-free water was included for each nucleic acid extraction, and a cDNA synthesis control for each cDNA synthesis step was also included. Three negative controls consisting of TE buffer (10 mM Tris/0.1 mM EDTA) or nuclease-free water were included as no-template controls for each qPCR run. CP56, HF183, BCoV, and PMMoV were considered quality control indicator markers for this study. Specifically, if ≥2 of these markers were non-detect in any archived nucleic acid extract, the corresponding frozen wastewater concentrate was re-extracted and analyzed. For virus culture methods, positive and negative controls were run with every sample batch. The positive controls consisted of poliovirus type 1 strain Sabin (EnV assay), human adenovirus 10 (AdV assay), MS2 (E. coli Famp assay), and phiX174 (E. coli CN-13 assay).
BCoV recovery was determined for every sample using one of several approaches, depending on the history of the sample. If BCoV recovery was ultimately determined to be <1%, the sample was excluded (n = 89, <8% of all samples). For ‘fresh’ samples, (i.e., non-archived and analyzed approximately within one week of collection), spiked BCoV was directly quantified in each sample to estimate recovery of molecular viral targets. Some samples collected in early 2020 were processed and analyzed before the BCoV spiking approach was implemented into the monitoring effort (n = 37). For these samples, recovery was set to 2%—the average observed recovery for the combined HFUF-Centricon method and consistent with Gerrity et al. (2021).32 For ‘archived’ samples several approaches to determining recovery were assessed.
The remainder of this section discusses the various approaches for assessing recovery and the potential effects of target degradation during long-term sample storage. By re-quantifying BCoV in archived nucleic acid extracts and comparing to the original recovery of any given sample, it is possible to not only account for original loss during sample processing but also for potential nucleic acid degradation and loss caused by several years of storage at −30 °C. The resulting correction factor (recovery×degradation) can then be applied to all assays in which BCoV can be quantified before and after storage. For samples in which BCoV was originally detected but then non-detect upon re-analysis of the archived extract, several recovery estimation methods were assessed: (1) substitution of an average BCoV recovery, (2) substitution of a PMMoV-derived degradation factor, and (3) supervised machine learning (SML). Data where BCoV recoveries were known (n = 610) were split randomly into two sets, one with 75% of the data and the other with the remaining 25%. The larger set (n = 458) was used to develop the averages, PMMoV degradation terms, and SML models. These models were then used to estimate the recovery for the smaller dataset (n = 152), and the differences between the estimated recovery values and the actual recovery values were evaluated using root mean square error (RMSE), R2, and mean absolute error (MAE) goodness-of-fit metrics.
Traditionally, recovery analyses involve only testing a subset of samples due to cost and labor constraints.21,42–45 The subset recoveries are then averaged and applied to all samples, or a distribution is fit.43 However, with qPCR applications, especially in wastewater matrices, recovery efficiencies vary widely across samples. Thus, applying a generalized average may not be appropriate, as this approach may contribute significant bias.42,43 To evaluate potential bias, we considered multiple methods for averaging BCoV recovery rates. The first approach was using the facility-specific average recovery determined from the re-quantification of BCoV after storage (R1). Another method was using the original facility-specific average of recovery from the original quantification of BCoV before storage (R2) or substituting the original sample-specific recovery values (R3). Additionally, we evaluated substituting an overall combined average recovery point-value determined from the re-quantification of BCoV after storage (R4) or an overall point-value average of original recovery values (R5) (Table S7†).
As with BCoV, PMMoV was quantified before and after sample archiving, with the difference in concentration theoretically accounting for losses during storage. In the SARS-CoV-2 wastewater surveillance campaign workflow, PMMoV was quantified using a SYBR-based qPCR assay for all samples collected March 2020–December 2023. A paired sub-analysis of fresh samples showed that the SYBR-based assay yielded significantly different concentrations compared to the probe-based qPCR assay used for analysis of archived samples, with the SYBR-based assay yielding higher concentrations on average (by ∼0.26
log10 gc L−1) (p < 0.0001, paired t-test on normally distributed log10 transformed data, n = 48). Accordingly, SYBR-based PMMoV concentrations were adjusted using multiple approaches before dividing to determine the degradation term (PM1, 2, and 3). First, SYBR-based PMMoV concentrations were converted to probe-based concentrations using a linear regression (Fig. S3†), and degradation was calculated as the probe-based concentration (post-storage value) divided by the normalized concentration (pre-storage value) (PM1). Second, the average difference in concentrations (0.26
log10 gc L−1) was subtracted from the SYBR-based concentrations to convert to probe-based concentrations (PM2), and the degradation factor was determined as above. Third, no adjustment for the different assays was applied, and the degradation factor was calculated with the probe-based concentrations (post-storage value) divided by the SYBR-based concentrations (pre-storage value) (PM3). PMMoV degradation factors over 100% (due to error in the assay correction factors) were set to 100%, indicating no degradation occurred.
Calculating a correction term using PMMoV concentrations before and after archiving only accounts for losses due to degradation and the cDNA synthesis step for archived samples. To simultaneously correct for degradation via the PMMoV-derived correction factors and losses during initial processing, each iteration of the averaging approach (R1–R5) was also multiplied by each iteration of the PMMoV correction term (PM1–PM3) in separate models (R* × P*).
Recovery efficiency appears to be a non-independent adjustment factor, meaning that recovery can be correlated or have interdependent relationships with concentrations of other targets, water quality parameters such as total dissolved solids (TDS),43 and sample handling procedures. For this reason, we also attempted to use SML to estimate recovery using metadata and target concentrations, including temperature, storage time, facility, concentration method, detection status for each assay and sample, and non-recovery-corrected concentrations of the markers. SML was conducted using the cubist model,46 which is a form of decision tree modeling, from the caret package in R.47 The least important variables were identified with the varImp function and omitted to determine whether equal or greater accuracy could be achieved in equal or less computation time. The most accurate model was then tested across a wider range of hyperparameter settings. Additional information on the SML model, including all variables considered, the most important variables identified, and algorithm information, are available in Text S4.† Each individual method (i.e., SML, R* × P*, R1–R4, and PM1–3) was evaluated to determine the best fit to the known data.
2.8 Statistical analysis
Recovery-corrected results were log10 transformed before statistical analysis was performed. Statistics were performed in R (version 4.3.2). The package fitdistrplus48 was used to estimate the mean, standard deviation, and distribution of marker concentrations. For the “NoV GI Sum” distribution, concentrations of both GIA and GIB were added if both were detected. A detect for either GIA or GIB and a non-detect for the other still counted as a detect for GI, although only the value of the detected genotype was used for the NoV GI Sum distribution. For setting upper limits for left-censored data, the higher limits of detection and quantification (GIA) were used. For comparisons between groups of censored data, the Kruskal–Wallis test followed by Dunn's post hoc test with Bonferroni adjustment for multiple comparisons was used. For comparisons between groups of non-censored data, individual tests are indicated in the text. As these are ranked tests, they are agnostic to detection status. The lowest rank was assigned to non-detects, and the second-lowest rank was applied to <LoQs. Boxplots are in the style of Tukey,49 with the median as the center line, the box limits representing the first and third quartiles, and the whiskers extending ±1.5 times the interquartile range (IQR).
3. Results & discussion
3.1 Recovery correction
The original BCoV spike underwent degradation during storage, resulting in lower detection rates in archived (73.6%) vs. fresh (100%) samples. Table 2 shows the goodness-of-fit metrics for each assessed method for assigning recovery to the ∼19% of the overall dataset with non-detects or <LoQ values for BCoV re-quantification.
Table 2 Root mean square error (RMSE), R2, and mean absolute error (MAE) for each recovery estimation method ordered by RMSE from lowest to highest. The full method names and descriptions are provided in Table S7†
Methoda |
RMSE |
R
2
|
MAE |
Method names and descriptions are available in Table S7.† “R” methods are BCoV recovery corrections, “PM” methods are PMMoV degradation corrections, “×” indicates multiplication of two corrections, and SML is supervised machine learning.
Chosen for final pathogen distribution comparison (Text S4†).
Sample-specific original recovery.
|
SMLb |
0.158 |
0.863 |
0.096 |
R2 × PM1b |
0.199 |
0.783 |
0.145 |
R1 × PM1 |
0.216 |
0.745 |
0.141 |
R3 × PM2 |
0.221 |
0.732 |
0.158 |
R5 × PM1 |
0.223 |
0.727 |
0.158 |
R2 × PM2 |
0.228 |
0.714 |
0.177 |
R1 |
0.229 |
0.712 |
0.171 |
R3 × PM3 |
0.237 |
0.690 |
0.153 |
R1 × PM2 |
0.247 |
0.664 |
0.167 |
R2 |
0.248 |
0.662 |
0.212 |
R4 × PM1 |
0.248 |
0.661 |
0.158 |
R3 × PM1 |
0.254 |
0.646 |
0.169 |
R2 × PM3 |
0.262 |
0.622 |
0.171 |
R5 × PM2 |
0.263 |
0.619 |
0.206 |
R5 |
0.264 |
0.616 |
0.243 |
R4 × PM2 |
0.278 |
0.576 |
0.189 |
R4 |
0.279 |
0.572 |
0.200 |
R5 × PM3 |
0.285 |
0.552 |
0.188 |
R3b,c |
0.287 |
0.547 |
0.210 |
R1 × PM3 |
0.288 |
0.545 |
0.181 |
R4 × PM3 |
0.306 |
0.485 |
0.194 |
PM1 |
0.550 |
−0.660 |
0.452 |
PM3 |
0.651 |
−1.328 |
0.567 |
PM2 |
0.725 |
−1.892 |
0.668 |
The recovery estimation method with the lowest RMSE, lowest MAE, and highest R2 was SML using the cubist model. The RMSE was 0.158, which suggests that SML predicts recovery on average within 15.8% of the measured recovery value in the test set. The second lowest RMSE was R2 × PM1 (average of 0.199), referring to the substitution model where the facility-specific average recovery determined from the original BCoV quantification was multiplied by PMMoV degradation determined after correcting SYBR-based concentrations with linear regression. A subsequent analysis of the impact of recovery estimation method on final target concentration distributions revealed no significant difference (p > 0.95) between using the R2 × PM1 and SML methods, but that neglecting to account for degradation (R3, original recovery values alone), yielded significantly lower overall concentrations (Text S5 and Table S8†). This is expected since concentrations can decrease due to degradation, and original recovery alone would not account for that change, thereby yielding artificially low concentrations. Since the difference between the R2 × PM1 and SML approaches was statistically indistinguishable (all markers p > 0.95; Table S8†) and because SML is less intuitive and more computationally intensive, R2 × PM1 was chosen for calculating recovery for samples with BCoV results that were non-detect or <LoQ upon re-analysis.
Archived biobanks face challenges with nucleic acid degradation of targets due to the effects of one or more freeze–thaws, overall duration of storage, and storage temperature. This is evident here in lower detection rates in archived samples vs. fresh samples (AdV: 66% vs. 93%, EnV: 46% vs. 87%, NoV GI: 57% vs. 100%, NoV GII: 68% vs. 99%). Whenever possible, these impacts must be accounted for to avoid underestimating target presence and concentrations, which occurs when sample degradation has occurred but is not considered or quantified. Ideally, controls should be spiked into each sample and quantified before and after storage. In cases where this is not possible, we recommend assessing several different recovery or degradation estimation factors. SML provided the most accurate estimate for recovery in this study. However, not all biobank studies have access to the volume of data, including metadata, that were used to train the model. Using the advanced machine learning method was shown to not significantly impact concentration distributions compared to a simpler, average-based approach using original facility averages multiplied by a PMMoV degradation factor, though SML might be more useful if there was more extensive metadata available, such as water quality parameters. While machine learning might not be necessary when recovery can be estimated more directly, it could be useful in cases with extensive metadata, and some form of critical assessment is essential to determine the best method for estimating recoveries in the absence of consistent recovery data. Critical to all these methods is the assumption of using a spike-in for individual samples, which should be considered a high priority for wastewater studies.
3.2 Summary statistics & facility comparisons
3.2.1 Viral pathogen targets.
Norovirus.
NoV GI was observed at lower detection rates but had higher maximum concentrations across all facilities (overall max of 9.46
log10 gc L−1) compared to NoV GII (Table 3 and Fig. 2). Despite lower detection rates for GI (80%) compared to GII (90%), the fitted distribution mean for GI was higher (6.48
log10 gc L−1) than for GII (6.15
log10 gc L−1). Facility 3 had the highest concentrations of GI and GII and was significantly higher than facilities 1, 2, and 6 for GI and facilities 1, 2, 4A, 4B, and 6 for GII (p < 0.05) (Fig. S4†). The four highest GI concentrations were all greater than 9.17
log10 gc L−1, which is the concentration used as the basis for California's DPR regulatory rule setting,50,51 and the top three occurred in samples collected from facility 6, with the fourth occurring in facility 4B. Facility 6 and 4B were represented by grab samples, and facility 6 is also the smallest sewershed in the study (only ∼16
000 people), so it is possible that these particular grab samples constituted plugs of wastewater containing contributions from ‘supershedders’. In fact, the top two GI concentrations (9.46
log10 gc L−1 and 9.38
log10 gc L−1) both occurred in facility 6 in subsequent weeks in March 2023. The 9.17
log10 gc L−1 concentration utilized by California was observed in a grab sample from a very small facility in France (290 m3 per day or 0.08 mgd) serving approximately 1200 inhabitants during a known NoV GI outbreak.52 Given the small sewershed sizes for facility 6 in this study and the facility in France, coupled with their similar per capita wastewater generation rates, we hypothesize that the hydraulic characteristics of small systems (i.e., less dilution and dispersion) may result in higher observed pathogen peaks, especially during outbreaks. We detected 3 peak (≥9.17
log10gc L−1) samples from facility 6 across 120 samples, for a similar peak frequency as the facility in France (1 out of 28 collected samples).
Table 3 Summary statistics for all targets (recovery corrected). Corresponding non-recovery-corrected data are available in Table S9†
Parameter |
Crypto
|
Giardia
|
AdV (cult.) |
EnV (cult.) |
AdV (mol.) |
EnV (mol.) |
NoV GIA (mol.) |
NoV GIB (mol.) |
NoV GI sum |
NoV GII (mol.) |
CP56 (mol.) |
HF183 (mol.) |
PMMoV (probe) |
PMMoV (SYBR) |
Mean and standard deviation are of samples with detected target only.
Minimum is the lowest measured concentration above the LoQ.
Recovery mean and range.
Distribution fit to censored data using ‘fitdistcens’ with MLE or non-censored data with ‘fitdist’. Non-detect values were considered left-censored, and <LoQ values were considered interval-censored between the LoD and LoQ. Distributions are normal distributions of log10-transformed data, with mean and standard deviation reported in log10 target per L.
Average of MS2 & phiX174 recoveries.
Recovery separated by concentration method is available in Table S4.†
|
Number of samples (#) |
73 |
73 |
56 |
56 |
1107 |
1112 |
1112 |
1112 |
1112 |
1112 |
1112 |
1112 |
807 |
1108 |
Detection frequency (%) |
81% |
100% |
96% |
96% |
84% |
82% |
71% |
77% |
80% |
90% |
100% |
99% |
100% |
100% |
Meana (log10 target per L) |
2.18 |
3.73 |
3.29 |
3.77 |
6.53 |
5.92 |
6.71 |
6.65 |
6.91 |
6.41 |
9.19 |
8.04 |
9.30 |
9.07 |
St. dev.a (log10 target per L) |
0.47 |
0.40 |
0.75 |
0.88 |
1.06 |
0.67 |
0.83 |
0.88 |
0.90 |
0.84 |
0.70 |
1.43 |
0.53 |
0.49 |
Minb (log10 target per L) |
1.22 |
2.52 |
1.74 |
1.17 |
4.08 |
4.53 |
4.88 |
5.17 |
5.21 |
4.36 |
5.82 |
4.53 |
6.71 |
6.66 |
Max (log10 target per L) |
3.31 |
4.69 |
5.30 |
5.76 |
9.25 |
8.15 |
9.27 |
9.31 |
9.46 |
8.57 |
11.54 |
11.45 |
11.14 |
10.68 |
Recoveryc (%) |
31% (3–91) |
55% (3–90) |
34% (8–93)e |
23% (1–100)f |
31% (1–100) |
Fitted distributiond |
μ = 2.04 |
μ = 3.73 |
μ = 3.24 |
μ = 3.70 |
μ = 6.23 |
μ = 5.45 |
μ = 6.26 |
μ = 5.99 |
μ = 6.48 |
μ = 6.15 |
μ = 9.18 |
μ = 7.99 |
μ = 8.80 |
μ = 9.07 |
σ = 0.54 |
σ = 0.40 |
σ = 0.78 |
σ = 0.93 |
σ = 1.17 |
σ = 1.1 |
σ = 1.03 |
σ = 1.43 |
σ = 1.17 |
σ = 1.11 |
σ = 0.71 |
σ = 1.47 |
σ = 0.61 |
σ = 0.50 |
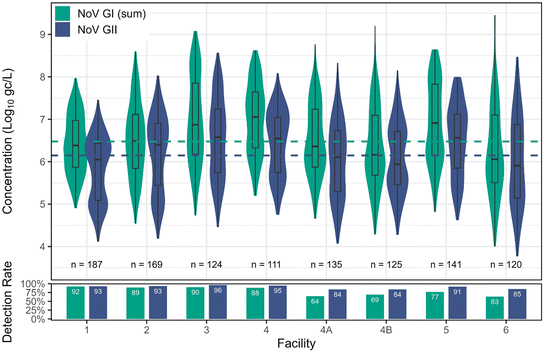 |
| Fig. 2 Distributions of concentrations of NoV GI (sum of GIA and GIB) and GII across facilities, along with corresponding detection rates. Censored data estimated for visualization using regression on order statistics (ROS) in NADA.53 Dashed lines indicate the overall combined fitted distribution means, taking into account left-censored data. | |
In composite samples or samples taken further into the treatment train (e.g., after primary clarification at facility 1), there is a peak “averaging” effect.54 This is useful when the goal is to evaluate wastewater that is representative of the overall population, for instance in WBE applications. Grab samples taken from influent wastewater are more likely to capture non-representative (e.g. non-dispersed or single-sourced) plugs of wastewater, but these samples are useful when assessing wastewater pathogen concentrations from a hydraulic perspective (e.g., when examining treatment train performance and its tolerance to spikes). Therefore, the grab samples resulting in NoV GI spikes are important additions to the dataset, highlighting how small systems may be more susceptible to concentration extremes.
It should be noted that while these concentrations appear to be outliers in Fig. 2, these values do not actually fall in that category when censored data are included in the outlier determination, and this applies to all apparent outliers in the boxplots. To verify that these points are not true outliers, we removed the four values >9.17
log10 gc L−1 and re-fit the distribution. The exceedance probability for 9.17
log10 gc L−1 had only a slight change between the high-points-included distribution (1.0%) vs. the high-points-excluded distribution (0.85%), and there was no statistically significant difference between the two distributions (Kolmogorov–Smirnov, p = 0.24).
Enterovirus.
EnV was primarily enumerated with qPCR (n = 1112), with a subset enumerated via cell culture (n = 56). EnV had an 82% detection rate via qPCR, with a distribution mean concentration of 5.45
log10 gc L−1. Concentrations of infectious EnV were lower, with an overall detection rate of 96% and distribution mean concentration of 3.70
log10 MPN L−1. Fig. 3 shows EnV concentrations, mean distribution across facilities, and detection rates. Facility 3 had the highest mean concentrations of EnV for both qPCR and cell culture. For qPCR, facility 3 was significantly higher than facilities 1, 2, 4A, 4B, and 6 (p < 0.05), and for culture methods, facility 3 was significantly higher than facilities 4A and 6 (p < 0.05).
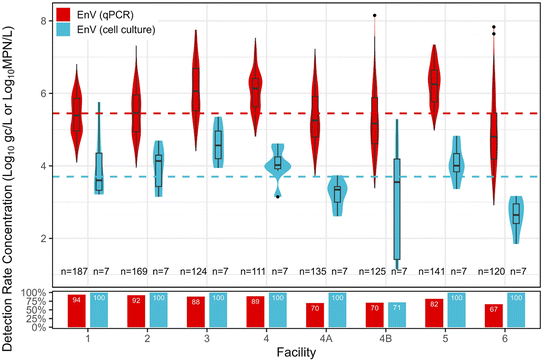 |
| Fig. 3 Distributions of EnV across facilities, along with corresponding detection rates. Censored data estimated for visualization using ROS. Dashed lines indicate the overall combined fitted distribution means, taking into account left-censored data. | |
Adenovirus.
AdV was also primarily quantified with qPCR (n = 1107), with a subset enumerated via cell culture (n = 56). Overall qPCR detection rate was 84%, falling between EnV and NoV GII in increasing detection rates. The distribution mean concentration by qPCR was 6.23
log10 gc L−1. For cell culture, AdV had a 96% detection rate, with a distribution mean concentration of 3.24
log10 MPN L−1. No significant differences in culture-based concentrations were observed among facilities, but similar to EnV, facility 3 had the highest concentration of AdV via qPCR, and was significantly higher than facilities 1, 2, 4A, 4B, and 6 (p < 0.05) (Fig. 4).
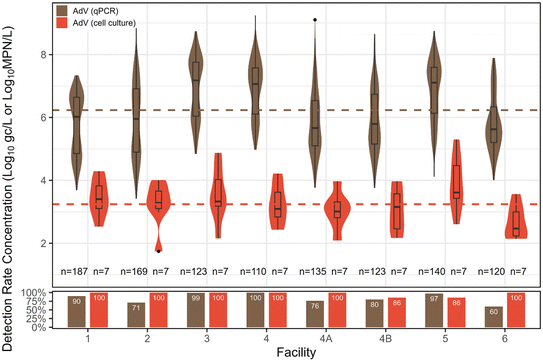 |
| Fig. 4 Distributions of AdV across facilities, along with corresponding detection rates. Censored data estimated for visualization using ROS. Dashed lines indicate the overall combined fitted distribution means, taking into account left-censored data. | |
Gene copy to infectious unit (GC
:
IU) ratios.
The subset of data analyzed by both qPCR and cell culture methods (n = 56) was used to develop distributions of GC
:
IU ratios for AdV and EnV (Fig. 5). GC
:
IU ratios varied widely across all samples and between facilities (Fig. S5†). GC
:
IU ratios ranged between 19
:
1 and 246
000
:
1 for AdV and between 1
:
1 and 54
400
:
1 for EnV for detectable data. These ranges (∼5
log10 and ∼4
log10, respectively) are consistent with Pecson et al. (2022),29 which utilized very similar methods. Notably, both the ratios observed here and in Pecson et al. (2022) were lower than those observed in a study utilizing similar methods on wastewater from San Diego, CA, where ratios for EnV ranged from 4.5–8
log10 GC
:
IU.55
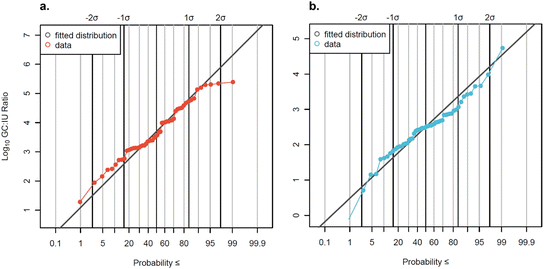 |
| Fig. 5 Measured GC : IU ratios for (a) AdV and (b) EnV plotted with the fitted log10 normal distributions (AdV: μ = 3.67, σ = 1.12; EnV: μ = 2.45, σ = 0.84). Non-detects are omitted from the plots but were used in the censored distribution fitting. Solid black vertical lines represent standard deviations from the 50th percentile. | |
Data were slightly censored (7% for AdV and 13% for EnV) so log10-transformed GC
:
IU ratios were fit to a censored normal distribution. The distribution mean GC
:
IU ratio for AdV was 3.67
log10, corresponding to a GC
:
IU ratio of 4699
:
1, and the mean GC
:
IU ratio for EnV was 2.45
log10, corresponding to a GC
:
IU ratio of 280
:
1. A recent study56 suggested that the cell culture method for enumerating EnV utilized here may not be optimal for detection of all infectious EnV in wastewater, and so subsequent QMRAs57 increased measured EnV culture concentrations by an assumed factor of 10 to correct for potential undercounting of virus in a viable-but-non-culturable (VBNC) state. This recently suggested correction factor, if applied to this study's reported EnV concentrations in future QMRAs, would bring the average EnV concentration by cell culture closer to the observed average concentration via qPCR (4.70
log10 MPN L−1 compared to 5.45
log10 gc L−1), and the distribution mean GC
:
IU ratio to only 28
:
1. Although molecular methods measured higher concentrations, detection rates were lower for gene copies compared to infectious units (84% vs. 96% for AdV, 82% vs. 96% for EnV). Two factors may explain this discrepancy: differences in ESV and storage degradation. Culture methods had higher ESVs (∼200 mL, Text S3†) compared to molecular methods (∼1 mL), increasing detection likelihood. Additionally, archived samples may have degraded, causing concentrations near the detection limit to fall below it. Notably, in paired samples measured by both methods, the molecular detection rate was greater than or equal to the culture detection rate (96% for AdV and 100% for EnV).
3.2.2 Protozoa targets.
Cryptosporidium oocysts were detected in 81% of samples, with most non-detects occurring in facility 6. The distribution mean concentration across all facilities was 2.04
log10 oocysts per L. Cryptosporidium's highest mean concentration occurred in facility 5, although the differences between facility 5 and other facilities were not statistically significant (p values ranging between 0.1 and 1 for each facility comparison). Cryptosporidium also exhibited spikes, with the maximum concentration (3.31
log10 oocysts per L) occurring in facility 4. A Kruskal–Wallis test indicated a slightly significant difference (p = 0.01) between facilities, but Dunn's post hoc analysis revealed no individual significant differences between facilities. Giardia was found in every sample (100% detection rate), with a mean concentration of 3.73
log10 cysts per L across all facilities. Giardia concentrations varied slightly between facilities (one-way ANOVA; p = 0.00155) (Fig. 6), with two spikes in facility 6 (4.66
log10 cysts per L and 4.69
log10 cysts per L) on separate sampling dates, causing facility 6 to have the widest range of concentrations and highlighting again the impact of grab sampling from small systems. However, facility 1 had the highest mean concentration, with significant differences compared to facilities 2, 4, and 6 (ANOVA, Tukey post hoc; p < 0.05).
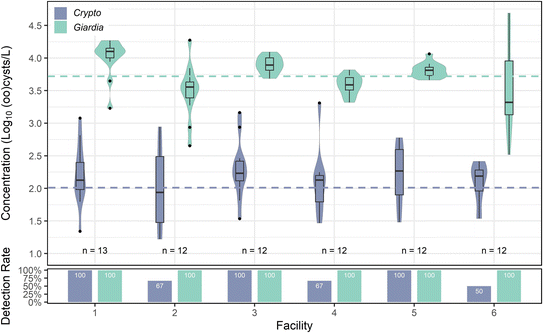 |
| Fig. 6 Distributions of Cryptosporidium and Giardia across facilities, along with corresponding detection rates. Censored data estimated for visualization using ROS. Dashed lines indicate the overall combined fitted distribution means, taking into account left-censored data. For Giardia, the fitted distribution mean is equivalent to the overall mean as the overall detection rate was 100%. Facility 1 has one additional datapoint due to a singular sample taken at the commencement of the study for methods optimization. | |
3.3 Distribution of pathogen concentrations
The Shapiro–Wilk test was used to test normality of pathogen datasets before fitting them to distributions. Log10-transformed concentrations of EnV (culture), AdV (culture), Cryptosporidium, Giardia, and EnV (molecular) were all normally distributed (p > 0.05), while all other molecular assays were not. The normal distribution on log10-transformed concentrations was still chosen for fitting for all targets because (1) there are no direct normality tests currently available for left- and interval-censored data; (2) the log10 normal distribution outperformed other commonly used environmental distributions (gamma, loge-normal, and Weibull) in terms of higher probability plot correlation coefficient (PCPP) goodness-of-fit values,58 indicating a superior fit; and (3) it is widely established in the literature to describe pathogen data in wastewater as log-normally distributed30 (either log10-normal [normal distribution of log10-transformed values] or loge-normal). Because of the non-normally distributed majority of the data, and due to censoring, the Kruskal–Wallis non-parametric rank-based test or the non-parametric Mann–Whitney tests were employed for censored data. Non-censored data or normally-distributed data comparisons have tests individually noted with the p-values. Pathogen distributions can be seen in Fig. 7, with both observed data and fitted distribution shown. Human fecal indicator distributions are available in Fig. S6.†
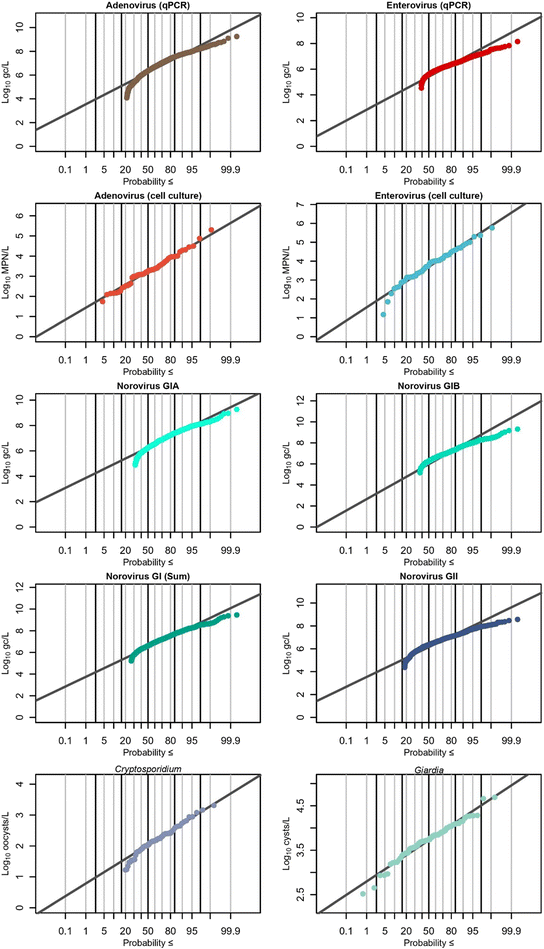 |
| Fig. 7 Probability plots of fitted normal distributions (gray) and observed data (colors) for log10-transformed pathogen concentrations. <LoQ and non-detect data are not plotted but included in the calculation of the percentiles (exceedance probabilities). Solid black vertical lines represent standard deviations from the 50th percentile. | |
3.3.1 Comparison to previously published datasets.
WRF 4989 and a subsequent publication by Darby et al. (2023)30 performed a literature review of high quality pathogen wastewater data (as defined by various minimum criteria in data relevance, method quality, compatibility with QMRA, large sample size, and temporal distributions) with the goal of creating combined distributions for use in DPR regulatory development. Their meta-analysis distribution as well as their project-specific distribution is reproduced in Table 4 for comparison and contextualization. Compared to their results, Southern Nevada pathogen wastewater concentrations were generally higher, within 1
log10 per L for most targets, but notably over 2
log10 gc L−1 higher in the case of all NoV targets, and 1.9
log10 gc L−1 higher for AdV (qPCR) compared to the California dataset. Additionally, there was greater variability (as measured by standard deviation) for all molecular targets except AdV (1.6 vs. 1.2) in the Southern Nevada dataset. This variability may be driven by the large sample size paired with censored data, encompassing over three years of weekly and monthly sampling, as well as encompassing both pandemic conditions and ‘normal’ conditions. Nearly identical methods were employed between this study and WRF 4989, so while the differences are unlikely to be methods driven, there still could be differences due to archiving, recovery adjustment methods, and inherent sampling differences. Future research can use this study and the unprecedented volume of biobanks of wastewater data from other COVID-19 wastewater surveillance programs, which will result in an increase in published pathogen datasets, to create a new combined distribution.
Table 4 Comparison to WRF 4989 pathogen distributions
Pathogen |
Meta-analysisa |
Californiaa |
Current study |
Differencec |
Meanb |
St. dev.b |
Meanb |
St. dev.b |
Meanb |
St. dev.b |
Δ |
Data reproduced here from WRF 4989.
Mean and standard deviation are of a normal distribution of log10-transformed concentrations in units of target per L.
Δ = current study − literature (i.e., meta-analysis or California). For markers with no meta-analysis data, the California data are used.
|
Cryptosporidium microscopy |
1.9 |
0.6 |
1.7 |
0.4 |
2.0 |
0.5 |
+0.1 |
Giardia microscopy |
4.0 |
0.4 |
4.0 |
0.4 |
3.7 |
0.4 |
−0.3 |
Enterovirus culture |
3.2 |
1.0 |
3.2 |
1.0 |
3.7 |
0.9 |
+0.5 |
Adenovirus culture |
— |
— |
2.8 |
1.0 |
3.2 |
0.8 |
+0.4 |
Enterovirus molecular |
5.1 |
1.1 |
4.9 |
0.8 |
5.5 |
1.1 |
+0.4 |
Adenovirus molecular |
— |
— |
4.3 |
1.6 |
6.2 |
1.2 |
+1.9 |
Norovirus GIA molecular |
— |
— |
3.8 |
1.0 |
6.3 |
1.0 |
+2.5 |
Norovirus GIB molecular |
— |
— |
3.6 |
1.0 |
6.0 |
1.4 |
+2.4 |
Norovirus GI sum |
— |
— |
— |
— |
6.5 |
1.2 |
— |
Norovirus GII molecular |
— |
— |
4.0 |
0.2 |
6.2 |
1.1 |
+2.2 |
3.4 Geographic and temporal trends
3.4.1 Seasonality.
Significant seasonal variation was observed for all qPCR viral pathogen targets but not culture-based AdV and EnV, Cryptosporidium, nor Giardia, potentially due to the smaller datasets for the latter targets (e.g., n ≈ 18 per season for Cryptosporidium and Giardia vs. n ≈ 300 per season for molecular targets). All NoV GI targets (GIA, GIB, and sum) displayed significantly higher concentrations in the winter over all other seasons. NoV GII was significantly higher in winter than summer and fall (p < 0.05) and higher in fall than in summer (p = 0.03). The observed higher concentrations of NoV GI and GII in winter align with seasonal patterns of norovirus outbreaks across the United States.59 This pattern has been similarly observed in other non-equatorial areas of the world in both clinical cases60 and wastewater monitoring.61 Worldwide, NoV GII tends to be the predominant genogroup in both clinical cases/outbreaks during winter and spring (peak seasons) and in off-seasons (summer/fall).62–69 This is reflected in higher detection of Nov GII in wastewater globally,68,70–72 as well as in this study. Interestingly, NoV GI had higher concentrations in this study, although it is rarely determined to be the causative agent of outbreaks or clinical cases, regardless of season, despite its prevalence in wastewater throughout the world and in this study.67,68,73,74 This is corroborated by Southern Nevada public health data, which suggests endemic circulation of NoV GII, and more sporadic detection NoV GI in clinical samples.75 The high concentrations of NoV GI in wastewater combined with low detection in clinical data/outbreaks could indicate underreported mild or asymptomatic illness.68,71,74
AdV and EnV did not display as substantial differences. AdV was slightly, but significantly, higher in the winter than in summer (p = 0.02); no other comparisons were statistically significant. Seasonality trends of gastrointestinal AdV are varied, largely depending on the particular location or timeframe.76,77 AdV gastroenteritis infections do not usually display strong seasonal patterns; instead, they generally peak sporadically throughout the year.78 For EnV, concentrations were higher in the fall than in spring or summer (p < 0.05). Though EnV gastroenteritis infections are sometimes associated with summertime illness,79 seasonality of EnV will largely depend on which EnV species are circulating within the population, as different enteroviruses have shown different seasonality in wastewater monitoring data.80
3.4.2 Effect of the COVID-19 pandemic.
The hypothesized effect of the COVID-19 pandemic on wastewater concentrations of enteric viruses (i.e., lower transmission of gastrointestinal pathogens due to disease control measures) was one reason for the California Division of Drinking Water's decision not to use the California-specific wastewater pathogen dataset developed by WRF 4989 (Table 4) as the basis for DPR regulations.50 Here, sufficient data is available to test the hypothesis using molecular data for AdV, EnV, NoV GI, and NoV GII in Southern Nevada wastewater. Two approaches were taken. First, the pandemic was divided into phases representing Nevada-specific guidelines,81 and concentrations of pathogens were compared between phases. Pandemic phases are characterized by disease control measures such as mandated masking, state shutdowns, and stay-at-home orders (Table 5). Second, the data were divided into “pandemic” (state stay-at home order of March 18, 2020, through the end of the statewide Declaration of Emergency on May 20, 2022) and “normal” conditions (pre-March 18, 2020 and post-May 20, 2022); pathogen concentrations were compared between these two sets. All viral pathogens were significantly higher (between 0.97 to 2.24
log10 higher) in samples collected during normal conditions, suggesting that gastrointestinal disease circulation was lower during the pandemic (Fig. 8 and S8†). Concentrations of all viral molecular markers were higher after the end of the state of emergency than all other individual pandemic phases, with the following exceptions. There was no significant difference between the phase after the end of the state of emergency and the end of the second mask mandate for NoV GII and AdV. Otherwise, all other phases exhibited significantly lower concentrations than after the end of the state of emergency (Fig. S7 and S8†).
Table 5 Pandemic phase characteristics
Milestone |
Sampling starts |
Stay-at-home directive |
State reopening |
State pause |
End of mask mandate |
Mask mandate 2 |
End of mask mandate 2 |
End of state of emergency |
Masking requirements reinstituted on 06/24/2020.
|
Start date |
3/10/2020 |
3/18/2020 |
5/9/2020 |
11/22/2020 |
6/1/2021 |
7/27/2021 |
2/10/2022 |
5/20/2022 |
Masking requirements |
No |
No |
Yesa |
Yes |
No |
Yes |
No |
No |
Social distancing measures |
No |
Yes (lockdown) |
Yes |
Yes |
Reopening at 100% capacity |
No |
No |
No |
Public school status |
In-person |
Remote |
Remote |
Remote/hybrid |
Hybrid/summer break |
Summer break/in-person |
In-person |
In-person |
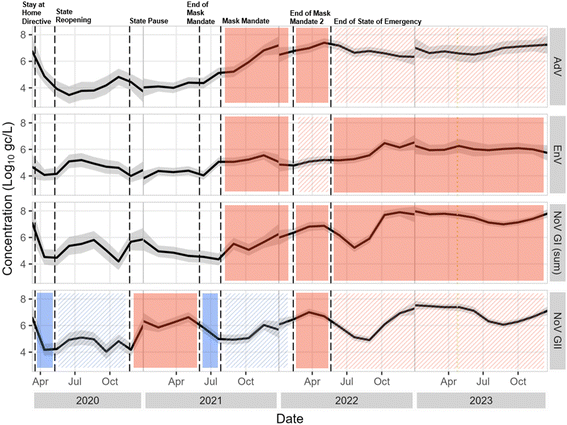 |
| Fig. 8 Locally estimated scatterplot smoothed (LOESS, a nonparametric method for smoothing82) concentrations with 95 percent confidence interval plotted over time and separated by pathogen. For plotting purposes only, <LoQ and non-detect data were set to the LoQ/ . Statistical significance as measured by the Kruskal–Wallis test and post hoc testing is indicated with solid shading, with solid shade of red indicating a significant increase from the previous phase and a solid shade of blue indicating a significant decrease from the previous phase. Hatching indicates no significant difference from the previous phase, and color of the hatching matches the previous shade. Concentrations are not smoothed across calendar year borders. | |
An argument can be made that for application of these data, distinction should be made between pandemic conditions and normal conditions, with the normal-condition distribution providing more conservative (i.e., higher) pathogen concentrations for use in QMRA. Alternatively, the full dataset provides a larger range of potential wastewater conditions, incorporating variability that could be useful for estimates of risk across broad scenarios. Separate pandemic-condition and normal-condition distribution fittings are available in Table S10.†
3.5 Human fecal indicators
Of the three indicators assessed (crAssphage, HF183, and PMMoV), crAssphage had the highest concentrations (distribution mean concentration = 9.18
log10 gc L−1), followed by PMMoV (distribution mean concentration = 8.80
log10 gc L−1) and HF183 (distribution mean concentration = 7.99
log10 gc L−1). SYBR-based PMMoV data are not included in these analyses.
In Southern Nevada, human fecal indicator concentrations in wastewater should be fairly constant due to few and theoretically stable non-human contributions to the non-combined sewer system. However, we observed human fecal indicator changes between facilities, pandemic phases, and slight seasonal variation. PMMoV and crAssphage exhibited lower variation in wastewater than the viral pathogens measured by qPCR (Fig. 9). Bacterial indicator HF183 had an unexpected drop in concentration in October 2022 before recovering to previous levels in 2023, which is curious as methods were consistent for the duration of the study, viral fecal indicators remained constant, and the drop off was observed in all facilities. This drop-off impacted the standard deviation, 1.47
log10 gc L−1, the highest observed across all targets. We note that the wastewater concentration methods involve removing the solids fraction, which may contain a significant portion of bacteria due to their larger size. Therefore, these methods may not be optimal for bacterial markers. Seasonal trends were not observed for crAssphage (p = 0.09). For PMMoV and HF183, there were higher concentrations observed in summer compared to all other seasons (p < 0.0001). All fecal indicators displayed significant variation between facilities, with the most obvious trend being that facility 1 had significantly lower concentrations (p < 0.005) than all other facilities, with the only exception being PMMoV in facilities 1 and 2 not being statistically distinguishable (p = 1). The facility 1 sample is of primary effluent (influent after undergoing a settling step), so fecal material may have been somewhat removed. Moreover, due to the grab nature of the primary effluent, representing influent arriving at ∼5:00–6:00 am, there may be a diurnal effect leading to lower human fecal inputs.32
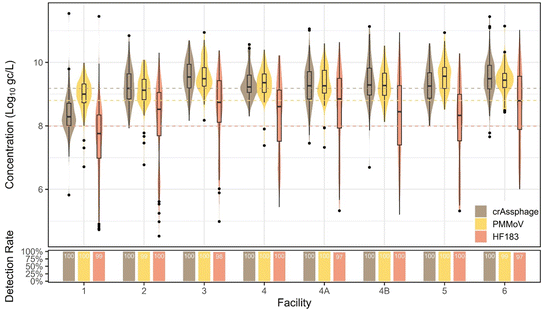 |
| Fig. 9 Human fecal indicator quantifiable concentrations and detection rates plotted with the dashed lines representing the overall combined distribution means. For crAssphage, the fitted distribution mean is equivalent to the overall mean as the overall detection rate was 100%. | |
Interestingly, there were significant changes between individual pandemic phases for all indicators. For instance, crAssphage increased somewhat during the state reopening phase (p = 0.01), stayed high and constant, and then significantly decreased when the second mask mandate was issued (p = 0.007), potentially due to changes in commuting behaviors, tourism, or other factors. PMMoV did not follow this pattern, however, with a significant increase during the state reopening phase (p = 0.04), increasing again during the state pause (p = 0.01), decreasing at the end of the mask mandate (p = 0.03), constant through the second mask mandate, and a final significant decrease from the end of the second mask mandate into the end of the state of emergency phase (p < 0.0001). When divided into pandemic and normal phases, crAssphage showed no significant difference between phases (p = 0.71, Mann–Whitney), whereas PMMoV was significantly higher (p < 0.0001, Mann–Whitney) in normal/non-pandemic phases and HF183 was significantly higher (p < 0.0001, Mann–Whitney) in pandemic phases.
Ultimately, due to the high variability of HF183 and the potential non-human sources of PMMoV (e.g., food preparation and food waste disposal down drains), our data suggest that crAssphage is the best performing molecular fecal indicator for MST, or potentially for future data normalization approaches, at least specifically in the studied watershed.
4. Conclusions
For future work, clinical datasets as well as alternative datasets (e.g., consumer purchases of medications) can validate WBE of enteric pathogens, which could increase public health practitioners' confidence in the use of wastewater for gastrointestinal disease surveillance, as clinical data are known to underestimate the true prevalence of gastrointestinal illness.83 Also, QMRA sensitivity analyses should consider some of the factors found to be significant in this study, including sewershed-specific characteristics, seasonality or overall time-dependence of concentrations (e.g., extended peak concentrations during outbreaks), and larger disruptions to social behavior such as pandemic phases and associated policies.
Also, here we characterize microbial constituents (excluding Giardia and Cryptosporidium) in the liquid portion of wastewater, although we recognize that enteric pathogens and fecal indicators exist in both liquid and solid phases.4,84 The WastewaterSCAN program, initially developed to monitor SARS-CoV-2 in wastewater, has now expanded to include other targets, including enteric pathogens. However, it solely focuses on pathogen concentrations in the solid phase of wastewater, which presents certain limitations for environmental applications, wastewater treatment optimization, and regulatory decision making. While its current form is validated, optimized, and highly useful for public health applications, the dataset's utility can be significantly enhanced in future research by incorporating methodologies to convert between solid and liquid phase concentrations using partitioning coefficients. For instance, research could include back-calculating overall influent concentrations for water reuse LRV development, and also infection estimates for WBE applications. Characterizing partitioning coefficients for a growing list of viruses and pairing these coefficients with total suspended solids data could facilitate translation of reported gene copies per gram of solids to overall gene copies per liter of wastewater. This approach could allow future research to compare pathogen wastewater dynamics in Southern Nevada to the United States in general, allowing for a more comprehensive utilization of the WastewaterSCAN data, enhancing its applicability across various multidisciplinary fields.
Major products of our study include robust fitted distributions for culture-based enteric viruses, qPCR-based enteric viruses, protozoan pathogens, and human fecal indicators. Additionally, we developed methods for recovery estimation when degradation of biobank samples may be a concern, and we established GC
:
IU ratio distributions. These ratios are critical parameters for QMRAs when converting molecular data, which includes both non-infectious and infectious genetic material, into infectious units. Our analyses of wastewater concentrations of enteric viruses during the COVID-19 pandemic supports the hypothesis that concentrations of enteric pathogens were significantly lower in some wastewater systems during pandemic conditions. This decrease is potentially due to reduced spread of gastrointestinal illnesses during social distancing and other pandemic response measures. Our study confirmed that enteric viruses, as measured by molecular methods, exhibited seasonal variation, with norovirus GI and GII following well documented trends in the literature. Furthermore, our findings indicated that fresh samples had fewer non-detects compared to archived samples, suggesting that storage conditions impact nucleic acid integrity. Therefore, incorporating appropriate storage and degradation controls are crucial for studies of biobanked nucleic acid extracts.
Data availability
Data for this article have been included as a part of the ESI.†
Conflicts of interest
The authors declare no competing conflicts of interest.
Acknowledgements
This study was supported by Water Research Foundation (WRF) project 5197, funded under Assistance Agreement No. 84046201-0, CFDA 66.511, which was awarded by the U.S. Environmental Protection Agency (EPA) to WRF. This study was also supported by the WaterSMART Applied Sciences program through the U.S. Bureau of Reclamation (Grant No. R22AP00236) and by the U.S. Centers for Disease Control and Prevention (CDC) (Grant No. NH75OT000057-01-00). This publication has not been formally reviewed by WRF, EPA, USBR, nor CDC, so the views expressed here are solely those of the authors and do not necessarily reflect the views of these agencies. Also, this work would not be possible without the continued support of the collaborating wastewater agencies.
References
-
M. J. Tisza, B. Hanson, J. R. Clark, L. Wang, K. Payne, M. C. Ross, K. D. Mena, A. Gitter, S. J. Javornik Cregeen and J. J. Cormier, Virome Sequencing Identifies H5N1 Avian Influenza in Wastewater from Nine Cities, medRxiv, 2024, preprint, DOI:10.1101/2024.05.10.24307179.
- K. Yuan, G. Huang, L. Wang, T. Wang, W. Liu, H. Jiang and A. C. Yang, Predicting norovirus in the United States using Google Trends: infodemiology study, J. Med. Internet Res., 2021, 23(9), e24554, DOI:10.2196/24554.
- A. B. Boehm, M. K. Wolfe, B. J. White, B. Hughes, D. Duong, N. Banaei and A. Bidwell, Human norovirus (HuNoV) GII RNA in wastewater solids at 145 United States wastewater treatment plants: comparison to positivity rates of clinical specimens and modeled estimates of HuNoV GII shedders, J. Exposure Sci. Environ. Epidemiol., 2023, 1–8, DOI:10.1038/s41370-023-00592-4.
- B. Boehm Alexandria, B. Shelden, D. Duong, N. Banaei, J. White Bradley and K. Wolfe Marlene, A retrospective longitudinal study of adenovirus group F, norovirus GI and GII, rotavirus, and enterovirus nucleic acids in wastewater solids at two wastewater treatment plants: solid-liquid partitioning and relation to clinical testing data, mSphere, 2024, 9(3), e00736-23, DOI:10.1128/msphere.00736-23.
- M. L. Ammerman, S. Mullapudi, J. Gilbert, K. Figueroa, F. de Paula Nogueira Cruz, K. M. Bakker, M. C. Eisenberg, B. Foxman and K. R. Wigginton, Norovirus GII wastewater monitoring for epidemiological surveillance, PLOS Water, 2024, 3(1), e0000198, DOI:10.1371/journal.pwat.0000198.
- C. Barber, K. Crank, K. Papp, G. K. Innes, B. W. Schmitz, J. Chavez, A. Rossi and D. Gerrity, Community-scale wastewater surveillance of Candida auris during an ongoing outbreak in Southern Nevada, Environ. Sci. Technol., 2023, 57(4), 1755–1763, DOI:10.1021/acs.est.2c07763.
- K. Babler, M. Sharkey, S. Arenas, A. Amirali, C. Beaver, S. Comerford, K. Goodman, G. Grills, M. Holung, E. Kobetz, J. Laine, W. Lamar, C. Mason, D. Pronty, B. Reding, S. Schürer, N. Schaefer Solle, M. Stevenson, D. Vidović, H. Solo-Gabriele and B. Shukla, Detection of the clinically persistent, pathogenic yeast spp. Candida auris from hospital and municipal wastewater in Miami-Dade County, Florida, Sci. Total Environ., 2023, 898, 165459, DOI:10.1016/j.scitotenv.2023.165459.
- M. K. Wolfe, A. T. Yu, D. Duong, M. S. Rane, B. Hughes, V. Chan-Herur, M. Donnelly, S. Chai, B. J. White and D. J. Vugia, Use of wastewater for mpox outbreak surveillance in California, N. Engl. J. Med., 2023, 388(6), 570–572, DOI:10.1056/NEJMc2213882.
- S. P. Sherchan, T. Solomon, O. Idris, D. Nwaubani and O. Thakali, Wastewater surveillance of Mpox virus in Baltimore, Sci. Total Environ., 2023, 891, 164414, DOI:10.1016/j.scitotenv.2023.164414.
-
J. Oghuan, C. Chavarria, S. R. Vanderwal, A. Gitter, A. A. Ojaruega, C. Monserrat, C. X. Bauer, E. L. Brown, S. J. Cregeen and J. Deegan, Wastewater surveillance suggests unreported Mpox cases in a low-prevalence area, MedRxiv, 2023, preprint, DOI:10.1101/2023.05.28.23290658.
- E. M. Mejia, N. A. Hizon, C. E. Dueck, R. Lidder, J. Daigle, Q. Wonitowy, N. G. Medina, U. P. Mohammed, G. W. Cox and D. Safronetz, Detection of mpox virus in wastewater provides forewarning of clinical cases in Canadian cities, Sci. Total Environ., 2024, 933, 173108, DOI:10.1016/j.scitotenv.2024.173108.
-
J. E. Chin Quee, Using Wastewater-Based Epidemiology to Study Chlamydia Occurrence on a College Campus, Honors Undergraduate Thesis, University of Central Florida, 2023.
- A. Pruden, P. J. Vikesland, B. C. Davis and A. M. de Roda Husman, Seizing the moment: now is the time for integrated global surveillance of antimicrobial resistance in wastewater environments, Curr. Opin. Microbiol., 2021, 64, 91–99, DOI:10.1016/j.mib.2021.09.013.
- D. Gerrity, K. Crank, E. C. Oh, O. Quinones, R. A. Trenholm and B. J. Vanderford, Wastewater surveillance of high risk substances in Southern Nevada: Sucralose normalization to translate data for potential public health action, Sci. Total Environ., 2024, 908, 168369, DOI:10.1016/j.scitotenv.2023.168369.
- WastewaterSCAN WastewaterSCAN, https://www.wastewaterscan.org/en/about (06/20/2024).
- E. Stachler, C. Kelty, M. Sivaganesan, X. Li, K. Bibby and O. C. Shanks, Quantitative CrAssphage PCR assays for human fecal pollution measurement, Environ. Sci. Technol., 2017, 51(16), 9146–9154, DOI:10.1021/acs.est.7b02703.
- K. Crank, X. Li, D. North, G. B. Ferraro, M. Iaconelli, P. Mancini, G. La Rosa and K. Bibby, CrAssphage abundance and correlation with molecular viral markers in Italian wastewater, Water Res., 2020, 184, 116161, DOI:10.1016/j.watres.2020.116161.
- K. Crank, S. Petersen and K. Bibby, Quantitative microbial risk assessment of swimming in sewage impacted waters using CrAssphage and pepper mild mottle virus in a customizable model, Environ. Sci. Technol. Lett., 2019, 6(10), 571–577, DOI:10.1021/acs.estlett.9b00468.
- W. C. Jennings, E. Gálvez-Arango, A. L. Prieto and A. B. Boehm, CrAssphage for fecal source tracking in Chile: covariation with norovirus, HF183, and bacterial indicators, Water Res.: X, 2020, 9, 100071, DOI:10.1016/j.wroa.2020.100071.
- N. Sims and B. Kasprzyk-Hordern, Future perspectives of wastewater-based epidemiology: monitoring infectious disease spread and resistance to the community level, Environ. Int., 2020, 139, 105689, DOI:10.1016/j.envint.2020.105689.
- B. M. Pecson, E. Darby, C. N. Haas, Y. M. Amha, M. Bartolo, R. Danielson, Y. Dearborn, G. Di Giovanni, C. Ferguson and S. Fevig, Reproducibility and sensitivity of 36 methods to quantify the SARS-CoV-2 genetic signal in raw wastewater: findings from an interlaboratory methods evaluation in the US, Environ. Sci.: Water Res. Technol., 2021, 7(3), 504–520, 10.1039/D0EW00946F.
- H. Katayama, E. Haramoto, K. Oguma, H. Yamashita, A. Tajima, H. Nakajima and S. Ohgaki, One-year monthly quantitative survey of noroviruses, enteroviruses, and adenoviruses in wastewater collected from six plants in Japan, Water Res., 2008, 42(6–7), 1441–1448, DOI:10.1016/j.watres.2007.10.029.
-
J. B. Rose, Reduction of pathogens, indicator bacteria, and alternative indicators by wastewater treatment and reclamation processes, IWA Publishing, 2005 Search PubMed.
- M. Kitajima, B. C. Iker, I. L. Pepper and C. P. Gerba, Relative abundance and treatment reduction of viruses during wastewater treatment processes—identification of potential viral indicators, Sci. Total Environ., 2014, 488, 290–296, DOI:10.1016/j.scitotenv.2014.04.087.
- G. Sedmak, D. Bina, J. MacDonald and L. Couillard, Nine-year study of the occurrence of culturable viruses in source water for two drinking water treatment plants and the influent and effluent of a wastewater treatment plant in Milwaukee, Wisconsin (August 1994 through July 2003), Appl. Environ. Microbiol., 2005, 71(2), 1042–1050, DOI:10.1128/AEM.71.2.1042-1050.2005.
- F. J. Simmons and I. Xagoraraki, Release of infectious human enteric viruses by full-scale wastewater utilities, Water Res., 2011, 45(12), 3590–3598, DOI:10.1016/j.watres.2011.04.001.
-
B. M. Pecson, E. Darby, G. Di Giovanni, M. Leddy, K. Nelson, C. Rock, T. Slifko, W. Jakubowski and A. Olivieri, WRF 4989: Pathogen Monitoring in Untreated Wastewater, 2021 Search PubMed.
-
D. T. Schmidtke, A. S. Hickey, I. Liachko, G. Sherlock and A. S. Bhatt, Analysis and culturing of the prototypic crAssphage reveals a phage-plasmid lifestyle, bioRxiv, 2024, preprint, DOI:10.1101/2024.03.20.585998.
- B. M. Pecson, E. Darby, R. Danielson, Y. Dearborn, G. Di Giovanni, W. Jakubowski, M. Leddy, G. Lukasik, B. Mull and K. L. Nelson, Distributions of waterborne pathogens in raw wastewater based on a 14-month, multi-site monitoring campaign, Water Res., 2022, 213, 118170, DOI:10.1016/j.watres.2022.118170.
- E. Darby, A. Olivieri, C. Haas, G. Di Giovanni, W. Jakubowski, M. Leddy, K. L. Nelson, C. Rock, T. Slifko and B. M. Pecson, Identifying and aggregating high-quality pathogen data: a new approach for potable reuse regulatory development, Environ. Sci.: Water Res. Technol., 2023, 9(6), 1646–1653, 10.1039/D3EW00131H.
- Las Vegas Convention and Visitors Authority LVCVA executive summary of southern Nevada tourism indicators, https://www.lvcva.com/research/visitor-statistics/ (06/24/2024).
- D. Gerrity, K. Papp, M. Stoker, A. Sims and W. Frehner, Early-pandemic wastewater surveillance of SARS-CoV-2 in Southern Nevada: Methodology, occurrence, and incidence/prevalence considerations, Water Res.: X, 2021, 10, 100086, DOI:10.1016/j.wroa.2020.100086.
- V. Vo, R. L. Tillett, K. Papp, S. Shen, R. Gu, A. Gorzalski, D. Siao, R. Markland, C.-L. Chang, H. Baker, J. Chen, M. Schiller, W. Q. Betancourt, E. Buttery, M. Pandori, M. A. Picker, D. Gerrity and E. C. Oh, Use of wastewater surveillance for early detection of Alpha and Epsilon SARS-CoV-2 variants of concern and estimation of overall COVID-19 infection burden, Sci. Total Environ., 2022, 835, 155410, DOI:10.1016/j.scitotenv.2022.155410.
-
USEPA, Method 1615: Measurement of enterovirus and norovirus occurrence in water by culture and RT-qPCR, 2012, pp. 1–91 Search PubMed.
- G. Ko, N. Jothikumar, V. R. Hill and M. D. Sobsey, Rapid detection of infectious adenoviruses by mRNA real-time RT-PCR, J. Virol. Methods, 2005, 127(2), 148–153, DOI:10.1016/j.jviromet.2005.02.017.
- E. Haramoto, M. Kitajima, N. Kishida, Y. Konno, H. Katayama, M. Asami and M. Akiba, Occurrence of pepper mild mottle virus in drinking water sources in Japan, Appl. Environ. Microbiol., 2013, 79(23), 7413–7418, DOI:10.1128/AEM.02354-13.
- R. A. Haugland, M. Varma, M. Sivaganesan, C. Kelty, L. Peed and O. C. Shanks, Evaluation of genetic markers from the 16S rRNA gene V2 region for use in quantitative detection of selected Bacteroidales species and human fecal waste by qPCR, Syst. Appl. Microbiol., 2010, 33(6), 348–357, DOI:10.1016/j.syapm.2010.06.001.
- K. Crank, K. Papp, C. Barber, P. Wang, A. Bivins and D. Gerrity, Correspondence on “The Environmental Microbiology Minimum Information (EMMI) Guidelines: qPCR and dPCR quality and reporting for environmental microbiology”, Environ. Sci. Technol., 2023, 57(48), 20448–20449, DOI:10.1021/acs.est.3c07968.
-
USEPA, Most Probable Number (MPN) Calculator.
-
USEPA, Method 1602: Male-specific (F+) and Somatic Coliphage in Water by Single Agar Layer (SAL) Procedure, Washington, DC, 2001 Search PubMed.
-
USEPA, Method 1693: Cryptosporidium and Giardia in Disinfected Wastewater by Concentration/IMS/IFA, Fed. Register, 2014.
- M. A. Borchardt, A. B. Boehm, M. Salit, S. K. Spencer, K. R. Wigginton and R. T. Noble, The environmental microbiology minimum information (EMMI) guidelines: qPCR and dPCR quality and reporting for environmental microbiology, Environ. Sci. Technol., 2021, 55(15), 10210–10223, DOI:10.1021/acs.est.1c01767.
- P. Schmidt, M. Emelko and M. Thompson, Analytical recovery of protozoan enumeration methods: Have drinking water QMRA models corrected or created bias?, Water Res., 2013, 47(7), 2399–2408, DOI:10.1016/j.watres.2013.02.001.
- S. Petterson, R. Grøndahl-Rosado, V. Nilsen, M. Myrmel and L. J. Robertson, Variability in the recovery of a virus concentration procedure in water: implications for QMRA, Water Res., 2015, 87, 79–86, DOI:10.1016/j.watres.2015.09.006.
- J. Van Heerden, M. Ehlers, J. Vivier and W. Grabow, Risk assessment of adenoviruses detected in treated drinking water and recreational water, J. Appl. Microbiol., 2005, 99(4), 926–933, DOI:10.1111/j.1365-2672.2005.02650.x.
-
J. R. Quinlan, Learning With Continuous Classes, 1992 Search PubMed.
-
M. Kuhn, S. Weston, C. Keefer and N. Coulter, Cubist models for regression, R package Vignette R package version 0.0, 2012, vol. 18, p. 480 Search PubMed.
- M. L. Delignette-Muller and C. Dutang, fitdistrplus: An R Package for Fitting Distributions, J. Stat. Softw., 2019, 64(4), 1–34 Search PubMed.
-
H. Wickham, ggplot2: Elegant Graphics for Data Analysis, Springer-Verlag New York, 2016 Search PubMed.
-
CADDW, Division of Drinking Water Response to “Expert Panel Preliminary Findings, Recommendations, and Comments on Draft DPR Criteria (dated August 17, 2021)” in the Memorandum of Findings submitted by NWRI dated March 14, 2022, 2022, p. 22 Search PubMed.
- S. E. Eftim, T. Hong, J. Soller, A. Boehm, I. Warren, A. Ichida and S. P. Nappier, Occurrence of norovirus in raw sewage – A systematic literature review and meta-analysis, Water Res., 2017, 111, 366–374, DOI:10.1016/j.watres.2017.01.017.
- A. K. da Silva, J.-C. Le Saux, S. Parnaudeau, M. Pommepuy, M. Elimelech and F. S. Le Guyader, Evaluation of removal of noroviruses during wastewater treatment, using real-time reverse transcription-PCR: different behaviors of genogroups I and II, Appl. Environ. Microbiol., 2007, 73(24), 7891–7897, DOI:10.1128/AEM.01428-07.
-
L. Lee, NADA: Nondetects and Data Analysis for Environmental Data, R package version 11, 2020 Search PubMed.
- D. Gerrity, K. Papp and B. M. Pecson, Pathogen peak “averaging” in potable reuse systems: lessons learned from wastewater surveillance of SARS-CoV-2, ACS ES&T Water, 2022, 2(11), 1863–1870, DOI:10.1021/acsestwater.1c00378.
-
Trussell Technologies, Pathogen Monitoring Study at the North City Water Reclamation Plant, 2017 Search PubMed.
- C. P. Gerba and W. Q. Betancourt, Assessing the Occurrence of Waterborne Viruses in Reuse Systems: Analytical Limits and Needs, Pathogens, 2019, 8(3), 107, DOI:10.3390/pathogens8030107.
- D. Gerrity, K. Crank, E. Steinle-Darling and B. M. Pecson, Establishing pathogen log reduction value targets for direct potable reuse in the United States, AWWA Water Sci., 2023, 5(5), e1353, DOI:10.1002/aws2.1353.
-
S. Millard, EnvStats: An R Package for Environmental Statistics, Springer, New York, 2013 Search PubMed.
- A. K. Kambhampati, L. Calderwood, M. E. Wikswo, L. Barclay, C. P. Mattison, N. Balachandran, J. Vinjé, A. J. Hall and S. A. Mirza, Spatiotemporal Trends in Norovirus Outbreaks in the United States, 2009–2019, Clin. Infect. Dis., 2022, 76(4), 667–673, DOI:10.1093/cid/ciac627.
- S. M. Ahmed, B. A. Lopman and K. Levy, A Systematic Review and Meta-Analysis of the Global Seasonality of Norovirus, PLoS One, 2013, 8(10), e75922, DOI:10.1371/journal.pone.0075922.
- Y. Huang, N. Zhou, S. Zhang, Y. Yi, Y. Han, M. Liu, Y. Han, N. Shi, L. Yang and Q. Wang, Norovirus detection in wastewater and its correlation with human gastroenteritis: a systematic review and meta-analysis, Environ. Sci. Pollut. Res., 2022, 29(16), 22829–22842, DOI:10.1007/s11356-021-18202-x.
- J. A. M. Siqueira, A. d. C. Linhares, T. C. N. de Carvalho, G. C. Aragao, D. d. S. Oliveira, M. C. Dos Santos, M. S. de Sousa, M. C. A. Justino, J. D. A. P. Mascarenhas and Y. B. Gabbay, Norovirus infection in children admitted to hospital for acute gastroenteritis in Belém, Pará, Northern Brazil, J. Med. Virol., 2013, 85(4), 737–744, DOI:10.1002/jmv.23506.
- M. Hellmér, N. Paxéus, L. Magnius, L. Enache, B. Arnholm, A. Johansson, T. Bergström and H. Norder, Detection of pathogenic viruses in sewage provided early warnings of hepatitis A virus and norovirus outbreaks, Appl. Environ. Microbiol., 2014, 80(21), 6771–6781, DOI:10.1128/AEM.01981-14.
- A. J. Hall, M. E. Wikswo, K. Pringle, L. H. Gould and U. D. Parashar, Vital signs: foodborne norovirus outbreaks—United States, 2009–2012, Morb. Mortal. Wkly. Rep., 2014, 63(22), 491–495 Search PubMed.
- M. Victoria, L. Tort, A. Lizasoain, M. García, M. Castells, M. Berois, M. Divizia, J. Leite, M. Miagostovich and J. Cristina, Norovirus molecular detection in Uruguayan sewage samples reveals a high genetic diversity and GII. 4 variant replacement along time, J. Appl. Microbiol., 2016, 120(5), 1427–1435, DOI:10.1111/jam.13058.
- S. Kazama, T. Miura, Y. Masago, Y. Konta, K. Tohma, T. Manaka, X. Liu, D. Nakayama, T. Tanno and M. Saito, Environmental surveillance of norovirus genogroups I and II for sensitive detection of epidemic variants, Appl. Environ. Microbiol., 2017, 83(9), e03406–e03416, DOI:10.1128/AEM.03406-16.
- T. M. Fumian, J. M. Fioretti, J. H. Lun, I. A. L. dos Santos, P. A. White and M. P. Miagostovich, Detection of norovirus epidemic genotypes in raw sewage using next generation sequencing, Environ. Int., 2019, 123, 282–291, DOI:10.1016/j.envint.2018.11.054.
- J. Lu, J. Peng, L. Fang, L. Zeng, H. Lin, Q. Xiong, Z. Liu, H. Jiang, C. Zhang, L. Yi, T. Song, C. Ke, C. Li, B. Ke, G. He, G. Zhu, J. He, L. Sun, H. Li and H. Zheng, Capturing noroviruses circulating in the population: sewage surveillance in Guangdong, China (2013–2018), Water Res., 2021, 196, 116990, DOI:10.1016/j.watres.2021.116990.
- D. Chen, Q. Shao, X. Ru, S. Chen, D. Cheng and Q. Ye, Epidemiological and genetic characteristics of norovirus in Hangzhou, China, in the postepidemic era, J. Clin. Virol., 2024, 172, 105679, DOI:10.1016/j.jcv.2024.105679.
- N. Montazeri, D. Goettert, C. Achberger Eric, N. Johnson Crystal, W. Prinyawiwatkul and E. Janes Marlene, Pathogenic Enteric Viruses and Microbial Indicators during Secondary Treatment of Municipal Wastewater, Appl. Environ. Microbiol., 2015, 81(18), 6436–6445, DOI:10.1128/AEM.01218-15.
- M. Kitajima, E. Haramoto, C. Phanuwan, H. Katayama and H. Furumai, Molecular detection and genotyping of human noroviruses in influent and effluent water at a wastewater treatment plant in Japan, J. Appl. Microbiol., 2012, 112(3), 605–613, DOI:10.1111/j.1365-2672.2012.05231.x.
- C. J. A. Campos, J. Avant, J. Lowther, D. Till and D. N. Lees, Human norovirus in untreated sewage and effluents from primary, secondary and tertiary treatment processes, Water Res., 2016, 103, 224–232, DOI:10.1016/j.watres.2016.07.045.
- L. D. Bruggink, O. Oluwatoyin, R. Sameer, K. J. Witlox and J. A. Marshall, Molecular and epidemiological features of gastroenteritis outbreaks involving genogroup I norovirus in Victoria, Australia, 2002–2010, J. Med. Virol., 2012, 84(9), 1437–1448, DOI:10.1002/jmv.23342.
- D. M. Teixeira, P. K. de Pontes Spada, L. L. C. de Sá Morais, T. M. Fumian, I. C. G. de Lima, D. de Souza Oliveira, R. da Silva Bandeira, T. C. M. Gurjão, M. S. de Sousa, J. D. A. P. Mascarenhas and Y. B. Gabbay, Norovirus genogroups I and II in environmental water samples from Belém city, Northern Brazil, J. Water Health, 2016, 15(1), 163–174, DOI:10.2166/wh.2016.275.
-
H.-Y. Kan, in Norovirus GI and GII seasonality, ed. K. Crank, 2024 Search PubMed.
- R. Cooper, R. Hallett, A. Tullo and P. Klapper, The epidemiology of adenovirus infections in Greater Manchester, UK 1982–96, Epidemiol. Infect., 2000, 125(2), 333–345 CrossRef CAS PubMed.
- G. Guga, S. Elwood, C. Kimathi, G. Kang, M. N. Kosek, A. A. Lima, P. O. Bessong, A. Samie, R. Haque and J. P. Leite, Burden, clinical characteristics, risk factors, and seasonality of adenovirus 40/41 diarrhea in children in eight low-resource settings, Open Forum Infect. Dis., 2022, ofac241, DOI:10.1093/ofid/ofac241.
-
O. Ruuskanen, J. P. Metcalf, O. Meurman and G. Akusjärvi, Adenoviruses, Clinical Virology, 2009, ch. 26, pp. 559–580, DOI:10.1128/9781555815981.ch26.
- M. Pons-Salort, M. S. Oberste, M. A. Pallansch, G. R. Abedi, S. Takahashi, B. T. Grenfell and N. C. Grassly, The seasonality of nonpolio enteroviruses in the United States: Patterns and drivers, Proc. Natl. Acad. Sci. U. S. A., 2018, 115(12), 3078–3083, DOI:10.1073/pnas.1721159115.
- N. E. Brinkman, G. S. Fout and S. P. Keely, Retrospective Surveillance of Wastewater To Examine Seasonal Dynamics of Enterovirus Infections, mSphere, 2017, 2(3) DOI:10.1128/msphere.00099-17.
-
Wikipedia contributors, COVID-19 pandemic in Nevada, in Wikipedia, The Free Encyclopedia, 2024 Search PubMed.
- W. S. Cleveland, Robust Locally Weighted Regression and Smoothing Scatterplots, J. Am. Stat. Assoc., 1979, 74(368), 829–836 CrossRef.
- C. L. Gibbons, M.-J. J. Mangen, D. Plass, A. H. Havelaar, R. J. Brooke, P. Kramarz, K. L. Peterson, A. L. Stuurman, A. Cassini and E. M. Fèvre, Measuring underreporting and under-ascertainment in infectious disease datasets: a comparison of methods, BMC Public Health, 2014, 14, 1–17, DOI:10.1186/1471-2458-14-147.
- M. K. Wolfe, A. Archana, D. Catoe, M. M. Coffman, S. Dorevich, K. E. Graham, S. Kim, L. M. Grijalva, L. Roldan-Hernandez and A. I. Silverman, Scaling of SARS-CoV-2 RNA in settled solids from multiple wastewater treatment plants to compare incidence rates of laboratory-confirmed COVID-19 in their sewersheds, Environ. Sci. Technol. Lett., 2021, 8(5), 398–404, DOI:10.1021/acs.estlett.1c00184.
|
This journal is © The Royal Society of Chemistry 2025 |
Click here to see how this site uses Cookies. View our privacy policy here.