Comparing contaminants of emerging concern and microbial signatures of wastewater treated by membrane bioreactor and oxidation ditch methods†
Received
26th September 2024
, Accepted 12th February 2025
First published on 25th March 2025
Abstract
Membrane bioreactors (MBRs) are an attractive option for upgrades to aging wastewater treatment infrastructure across the United States (U.S.) because they have a relatively small footprint and can handle various wastewater loading conditions. However, little is known regarding the efficiency with which MBRs can reduce contaminants of emerging concern (CECs) and microbial loads, or how they influence the microbiome of wastewater effluent. In 2022, we collected nine paired samples of primary treated effluent (PRI), secondary effluent treated by oxidation ditches (OXI), and secondary effluent treated by MBR from a facility in Minnesota, U.S. Samples were analyzed for CECs including 4 alkylphenol ethoxylates, 6 bisphenol analogues, 20 hormones, 110 pharmaceuticals, and 82 pesticides as well as heterotrophic bacteria counts (HPC) and microbiome communities. Except for pesticides, total sample concentrations of CEC classes in OXI and MBR effluents were similar and lower compared to PRI (p < 0.01). Pesticide concentrations were similar among all treatments. HPC was lower in MBR, compared to OXI, in 67% of samples. Despite limited differences in CEC signatures, differences in the microbiome between the two secondary treatments were observed. OXI showed more distinct differences in bacterial operational taxonomic unit (OTU) presence from PRI, compared to MBR. Relations between some contaminant groups (e.g., pharmaceuticals, bisphenols) and effluent microbiomes were observed, though most correlated with PRI effluent. Results from our study indicate that MBR is as effective as OXI at producing high-quality effluent and offers a viable alternative to conventional secondary treatment methods. This full-scale study provides data that helps fill knowledge gaps related to MBR performance outside of lab or benchtop experiments and direct comparison to OXI treatment.
Water impact
Membrane bioreactors are an attractive technology for wastewater treatment and can remove conventional pollutants such as nutrients. Results from this study indicate that membrane bioreactors provide similar benefits for removal of contaminants of emerging concern and microbial loads, when compared to more conventional oxidation ditches. This information can be useful for wastewater facility managers to make informed decisions when considering facility upgrades to aging infrastructure.
|
1. Introduction
In the United States (U.S.), an estimated 14
544 publicly owned wastewater treatment facilities (POTWs) provide treatment for over 250 million people.1 Mechanically aerated oxidation ditch technology (OXI) is most commonly used for secondary treatment, which follows primary treatment.2 OXI relies on aeration and turbulent mixing to enhance aerobic biological degradation of organic waste in a circular raceway.3 However, research has shown trace organic pollutants, including contaminants of emerging concern (CECs), are found in OXI-treated effluents.4–6 The presence of CECs in effluents is dependent on several factors including residence time during treatment and CEC physicochemical properties. While the microbial community in OXI systems influences removal efficacies,7 wastewater temperature strongly influences microbial activity8,9 and OXI performance.10,11 Further, removal efficiencies can be as high as 95% for some surfactants, while other CEC classes like pesticides have much lower removal rates.4 Although POTW are not designed to remove CECs, it would be beneficial to maximize removal to reduce potential impacts to receiving waters.
One secondary treatment technology developed over the last couple of decades for which interest is increasing is the membrane bioreactor (MBR). MBR is an advanced wastewater treatment technology that combines biological treatment with membrane filtration.12,13 It has gained popularity as an efficient and compact solution for wastewater treatment in various applications, including municipal and industrial settings. MBR systems incorporate a biological treatment process (i.e., activated sludge) using microorganisms to break down and consume organic pollutants in the wastewater. One distinguishing feature of MBR is the use of membrane filtration to separate solids from the treated water. The membrane, typically made of materials such as polyethylene or polypropylene, acts as a barrier that allows water to pass through while retaining solids, bacteria, and other contaminants. Membrane filtration has proven effective in the removal of bacterial and viral pathogens, protozoa, and also particulate matter which improves tertiary treatment performance.14 In some instances, the filtration step eliminates the need for secondary clarification.
MBR technology enhances treatment efficiency by providing high-quality effluents with low levels of suspended solids and pathogens.15 MBRs typically have a smaller footprint compared to conventional wastewater treatment plants, making them suitable for locations with limited space as is often the case in POTW renovation or expansion. Additionally, there can be various MBR configurations (e.g., aerobic, anaerobic), some of which may include an integrated approach to incorporate other processes such as reverse osmosis or nanofiltration. Aerobic MBR has shown higher removal of hydrophobic and hydrophilic CECs from effluent, compared to anaerobic systems,16,17 and integrated MBR systems have shown higher removal of some CECs, compared to non-integrated systems.18,19 However, anaerobic MBRs are more effective than aerobic systems at removing nitrogen-based trace organic compounds, such as amitriptyline, carbamazepine, and atrazine, as a consequence of anaerobic microorganisms facilitating nitrogen reduction.16,17,20
A major component of MBR systems is the microbial community, which influences overall treatment of the wastewater effluent. Typically, the microbial community of sludge influences that of the membrane biofilm, which in turn, influences MBR effluent.21 Recent studies and meta-analyses have identified core microbiome members that span wastewater and treatment types, including Proteobacteria, Firmicutes, and Bacteroidetes (e.g., ref. 22 and 23). Despite the identification of a core community across systems and effluents, MBR systems tend to have a more distinct community compared to influent and other treatment types.21,22 Furthermore, there is evidence that MBR microbial diversity may decrease with time,24 yet retain distinct communities.22 Decreased microbial diversity may stem from increased solid retention time, nutrient enrichment, or the availability of biodegradable organic material.25,26 Another factor influencing MBR sludge microbial diversity is immigration of species from the influent. However, evidence indicates that influent species immigration has less of an effect on the core microbial community compared to the rare taxa community, the latter of which can be an important reservoir for microbial diversity.27
Because a dense microbial population is maintained within MBR systems, removal of some CECs and treatment efficiency tends to be higher compared to other biological-based systems.15,28 Overall, Proteobacteria and Actinobacteriota have been documented as the dominant phyla in MBR sludge,29 and may be responsible for degradation of some more recalcitrant CECs. Higher abundance of Proteobacteria, specifically Burkholderiales, were observed in MBR systems that effectively removed carbamazepine and gemfibrozil.30 Additionally, relations between the abundance of the Proteobacteria genera, Rhodobacter spp., and antibiotic presence in wastewater influent have been observed.31 Enzymatic activity associated with Rhodobacter spp. is efficient in organic and inorganic pollutant degradation.32 Proteobacteria members, including ammonia-oxidizing bacteria, nitrite-oxidizing bacteria, and phosphorus accumulating organisms, are critical for nutrient removal.25,33,34 Members of the Acidobacteriota phylum are equipped with carbon metabolism genes which are important for polysaccharide and aromatic compound degradation.35,36 Bacteroidetes, α-Proteobacteria, and β-Proteobacteria,26 especially β-Proteobacteria ammonia oxidizers including Nitrosomonas spp. operational taxonomic units (OTUs), dominate MBR effluent.26,37
To date, few studies have taken an interdisciplinary approach to link CEC removal and microbial communities in effluents and compare those relations in MBR and OXI systems at a full-scale POTW. The objective of the current study was to compare effluent quality from MBR and OXI systems, with a focus on CECs. To reach our objective, we used an interdisciplinary approach that included quantification of select CECs, quantification of microbial loads, and characterization of the microbial community (i.e., microbiome) in primary-treated effluent, MBR-treated secondary effluent, and OXI-treated secondary effluent samples. The design of the POTW at which the study was conducted provided a unique opportunity to allow for direct side-by-side comparison of effluent that was treated by MBR and OXI methods.
2. Materials and methods
2.1. Site description
This study was conducted at the City of Hutchinson Wastewater Treatment Facility (Minnesota, U.S.). As of 2022 census estimates, the population of Hutchinson is about 14
700 (https://www.census.gov/quickfacts/fact/table/hutchinsoncityminnesotaMN). The facility is designed to treat an average of 3.67 million gallons per day (mgd) and receives wastewater from a mix of residential and industrial sources. In 2008, the facility underwent several upgrades, one of which was the addition of MBR as a secondary treatment. After incoming wastewater undergoes primary treatment to remove solids, the primary-treated effluent (PRI) is split equally into two secondary treatments: conventional oxidation ditches (OXI) and membrane bioreactor (MBR) (Fig. 1). The OXI method uses two aeration units with an average sludge age of 24 days and average flow of 2.5 mgd. MBR uses the ZeeWeed 500 ultrafiltration module (General Electric, Boston, Massachusetts, U.S.) with an average sludge age of 28 days and flow of 1.2 mgd. Pore size of the MBR is 0.04 μm. As part of routine maintenance, the MBR membranes are cleaned three times weekly with a 12.5% sodium hypochlorite solution and once per week with a 50% citric acid solution. After secondary treatment, both waste streams are disinfected with ultraviolet light prior to discharging into the South Fork of the Crow River.
 |
| Fig. 1 Simplified flow path of wastewater treatment processing at the study facility to depict the points at which samples were collected (denoted by red stars). | |
2.2. Effluent sample collection and analysis
Effluent samples were collected immediately downstream of each secondary treatment basin, prior to disinfection. A total of nine effluent samples were collected from April until September. One sample was collected in April, August, and September. During the time frame from May 17 to June 27, samples were collected once per week, coincident with an on-site fish exposure study.38 During the on-site fish exposure time periods, samples were collected directly from plumbing that fed the different effluent streams to exposure aquaria. The April, August, and September samples were collected by lowering a stainless-steel weighted bottle sampler fitted with the appropriate bottle into each effluent stream.
2.2.1. CEC analysis.
Effluent samples were analyzed for bisphenols and alkylphenols by SGS AXYS Laboratories (British Columbia, Canada) and pesticides, pharmaceuticals, and hormones at the U.S. Geological Survey National Water Quality Laboratory (Lakewood, Colorado, U.S.) (Table S1†). Whole-water effluent samples intended for bisphenol (n = 6) and alkylphenol (n = 4) analyses were collected in 1 L glass, amber bottles and analyzed using liquid chromatography coupled with tandem mass spectrometry (LC-MS/MS) and gas chromatography coupled with low resolution mass spectrometry, respectively. Samples for pesticide and pharmaceutical analyses were filtered through a 0.7 μm pore syringe filter into a 20 mL glass amber vial, per USGS standard operating procedures.39 Both pesticides (n = 82) and pharmaceuticals (n = 111) were analyzed using direct injection methods with LC-MS/MS.40,41 Whole-water effluent samples intended for hormone analysis were collected in 500 mL high density polyethylene bottles and analyzed for 20 hormones, including 2 sterols, using gas chromatography coupled with tandem mass spectrometry.42
To assess potential contamination associated with sample collection, sample processing, and matrix interference, 2 field blank, 2 field replicate, and 3 matrix spike samples were collected. Field blank samples were collected by pouring organic-free blank water directly into sample bottles for whole-water analyses and by filtering organic-free blank water through a syringe filter into samples bottles for pesticide and pharmaceutical analyses. Sequential field replicate samples were collected following collection methods of environmental samples. Matrix-spike samples were collected for pharmaceutical, pesticide, and hormone analyses, which were prepared by adding a solution containing known amounts of target contaminants to environmental samples upon receipt at the laboratory and analyzed accordingly.
2.2.2. Heterotrophic plate counts.
Whole-effluent samples were collected directly into 1 L sterile Nalgene polyethylene terephthalate copolyester and glycol-modified bottles. Samples were shipped overnight on wet ice to the USGS Michigan Bacteriological Research Laboratory (MIBaRL; Lansing, Michigan, U.S.) and processed within 24 hours of collection. Serial dilutions starting with 100 mL of wastewater sample were prepared with sterile 1× phosphate buffered saline (PBS) and filtered using aseptic technique and standard methods for membrane filtration43 for enumeration on Reasoner's 2A agar (R2A, heterotrophic plate count, HPC), according to manufacturer's instructions. Additional wastewater sample dilutions were made with sterile deionized water and added to IDEXX HPC assay (Westbrook, Maine, U.S.) to quantify heterotrophic bacteria load. IDEXX Quanti-Tray/2000 results were identified and enumerated according to the manufacturer's instructions. Final count data for the dilution series was calculated and reported according to the IDEXX Most Probable Number (MPN) Generator 1.4.4 (ref. 44) and the USGS National Water Quality Manual.45 Laboratory blank samples consisting of sterile 1× PBS (for R2A), and sterile deionized water (for IDEXX HPC) were processed with wastewater samples.
2.2.3. Microbial sequencing.
A total of 900 mL of each wastewater sample was filtered through a 0.2 μm pore size polycarbonate filter, aseptically transferred to a sterile microcentrifuge tube, and frozen at −20 °C for subsequent molecular analysis. Frozen filters were thawed, unfolded, and placed into a PowerWater Bead Tube (Qiagen; Germantown, Maryland, U.S.) for DNA extraction, and extracted using Qiagen DNeasy PowerWater Kit according to manufacturer's instructions. DNA concentrations were measured with a Quantus fluorometer (Promega; Madison, Wisconsin, U.S.).
Genomic DNA was delivered frozen to the Michigan State University Research Support Technology Facility Genomics Core (MSU RTSF; East Lansing, Michigan, U.S.) for 16S rRNA amplicon sequencing on the Illumina MiSeq sequencing platform (Illumina; San Diego, California, U.S.) for library preparation and sequencing. The V4 hypervariable region of the bacterial 16S rRNA gene was amplified with dual-indexed, Illumina-compatible primers (515F: GTGCCAGCMGCCGCGGTAA/806R: GGACTACHVGGGTWTCTAAT) following the protocol described by ref. 46, except that the index sequences were revised to unique dual index pairs. Following PCR, all amplified products were batch normalized using an Invitrogen (Carlsbad, California, U.S.) SequalPrep DNA Normalization plate; products recovered from this plate were pooled. Individual pools were concentrated and cleaned using a QIAquick Spin column (Qiagen) and AMPure XP magnetic beads (Beckman Coulter; Brea, California, U.S.). The pools were then verified and quantified using a combination of the Qubit dsDNA HS (ThermoFisher Scientific; Waltham, Massachusetts, U.S.), Agilent 4200 TapeStation High Sensitivity DNA1000 (Agilent; Santa Clara, California, U.S.), and Invitrogen Collibri Illumina Library Quantification qPCR assays (ThermoFisher Scientific; Waltham, Massachusetts, U.S.). Each pool was sequenced using an Illumina MiSeq v2 standard flow cell in a 2 × 250 base pair (bp) paired end format using a v2 500 cycle reagent cartridge. Custom sequencing and index primers complementary to the 515F/806R target-specific sequences were added to appropriate wells of the reagent cartridge. Base calling was done by Illumina Real Time Analysis (RTA) v1.18.54 and the output was demultiplexed and converted to FastQ format with Illumina Bcl2fastq v2.20.0.
2.3. Data analysis
CEC concentrations and microbial counts used in this analysis are available in a U.S. Geological Survey data release.47 Raw sequences and associated metadata and processing information are available in the National Center for Biotechnology Information Sequence Read Archive under Accession Number PRJNA1149818. Unless stated otherwise, analyses were conducted in R version 4.2.2.48 Treatment efficiency for removal of heterotrophic bacteria was calculated by dividing the difference between the influent and effluent concentrations by the concentration in influent and multiplying by 100.49
2.3.1. CECs.
Values reported as estimated values were used as reported by the laboratory. Typically, these are values that fall between the method detection limit and reporting limit so are associated with more uncertainty. In instances when the analyzing laboratory assigned a qualifier to a value indicating potential contamination, the value was removed from the dataset. Environmental data were compared to field blank sample results. In instances when environmental concentrations were <3 times the field blank concentration, the result was removed from the dataset. Three contaminants were detected one time in a field blank sample: caffeine (557 ng L−1), loperamide (4.72 ng L−1), and 17β-estradiol (0.15 ng L−1). A total of 1, 15, and 0 samples were re-censored for caffeine, loperamide, and 17β-estradiol, respectively.
After data screening was complete, statistical summaries including detection frequency, maximum concentration, total number of contaminants detected within CEC classes in each sample, and the total concentration of CEC classes in each sample were calculated. Percent removal of each contaminant was calculated for paired samples by dividing the difference between PRI and OXI or MBR concentrations by PRI concentration and multiplying by 100. For percent removal calculations, zero was substituted for censored (i.e., below the reporting level) results, which may overestimate percent removal.
Several approaches were used to assess differences among treatments in paired samples. First, the number of contaminants detected within each class and total sample concentration of each class were compared using a Kruskal-Wallis rank sum test, followed by a Dunn's Test with Holm correction for multiple comparisons,50 when appropriate. Next, for CECs that were detected at least one time in all three treatments (n = 47), concentrations among treatments were compared using a Wilcoxon signed-rank test with the cen_signedranktest function in NADA2.51 Lastly, percent removal of specific CECs was compared between OXI and MBR effluents using the Wilcoxon signed-rank test on paired samples.
2.3.2. Microbiome.
FastQ files were downloaded from MSU RTSF's genomics server and analyzed using the mothur v.1.42.1 software package.52,53 In summary, paired-end contigs were assembled, and any paired contigs (sequences) with an ambiguous base (defined as an N for the nucleotide base) or those outside of the expected read length (i.e., minimum of 225 bp and maximum of 275 bp) were removed from analysis. Sequences were aligned to the Silva Release 138 reference alignment54,55 and trimmed to align to the target V4 region. Unique sequences and their respective abundance were identified, and those sequences were clustered and then screened for chimeras using VSEARCH.56 Using mothur's Bayesian classifier, sequences were classified against the Silva Release 138 reference database,54,55 and any sequences classified as chloroplasts, mitochondria, unknown kingdom, Archaea, and Eukaryota removed from processing. The remaining sequences were clustered into OTUs based on 97% sequence similarity.
Using the mothur software, sample sequencing libraries were subsampled to 30
000 sequences, ensuring even sampling across samples for the calculation of alpha and beta diversity metrics. Alpha diversity metrics of Observed Species, Good's Coverage, inverse Simpson, and Shannon Index were calculated to produce sample-respective estimates for species richness, sequencing coverage, and diversity incorporating both species richness and species evenness. Phylip-formatted distance matrices for the Sorensen similarity coefficient based on observed species and Bray-Curtis indices were generated to calculate the dissimilarity between community membership and community structure, respectively.
Two-dimensional non-metric multidimensional scaling (NMDS) was used to visualize the Sorenson and Bray-Curtis distance matrix using the ggplot package.57 The ordiellipse function within the vegan package58 was used to plot ellipses on the NMDS ordinations. Analysis of molecular variance (AMOVA) and homogeneity of molecular variance (HOMOVA) were calculated using the Sorensen and Bray-Curtis distance matrices to determine whether clustering within the NMDS ordinations was statistically significant. Correlations of the relative abundance of each OTU with the NMDS axes were calculated to determine the influence of OTUs over NMDS ordination.
Additional statistical analyses were conducted using the NADA package (version 1.6–1.1)59 and dunn.test package (version 1.3.5).50 The nonparametric Kruskal-Wallis test was used to test for differences in HPC among treatments, followed by a Dunn's Test for multiple comparisons, when appropriate. Permutational Multivariate Analysis of Variance (PERMANOVA) using a Bray-Curtis distance matrix (adonis2 with 9999 permutations and pairwise.adonis2; vegan package) assessed the statistical significance between distance matrix partitions and treatment types. The envfit function in the vegan package was used to determine relations of measured contaminants on the NMDS ordination and clustering patterns of wastewater microbiomes. Using the vegan package, the Mantel test was performed to determine if there was a significant Spearman's correlation between the wastewater microbiome via the Bray-Curtis distance matrix and measured CEC or pharmaceutical group concentrations. The non-parametric Linear discriminant analysis Effect Size (LEfSe)60 was run to determine which OTUs contributed to statistically significant differences in relative abundances between microbiomes of the PRI, OXI, and MBR wastewater streams. Plots were constructed using tidyverse (version 1.3.1),61 ggplot2 (version 3.3.3),62 reshape2 (version 1.4.4),63 and RcolorBrewer (version 1.1–2) packages.
3. Results & discussion
3.1. CEC presence in effluents and removal from secondary treatment
Overall, 130 CECs (58% of those analyzed) were detected among all samples with 119 (56%), 83 (39%), and 80 (38%) detected in PRI, OXI, and MBR effluents, respectively (Table S2†). A total of 18 pharmaceuticals, 1 pesticide (prometon), and a corrosion inhibitor were detected in all samples, indicating little removal of these CECs by the treatment processes. Generally, the compositions of CECs (i.e., presence and magnitude) measured in the effluent samples align with those previously reported for wastewater effluent around the world.64 More CECs were detected in all PRI samples, compared to OXI and MBR, and at higher concentrations (Fig. 2). Compared to PRI, OXI and MBR had significantly lower numbers and total concentrations of all classes except pesticides (Table S4†). Total sample pesticide concentrations were similar among all treatments. Poor pesticide removal from wastewater using biological methods, including MBR, has previously been documented in the literature (e.g., ref. 28 and 65). The only differences observed between OXI and MBR effluent concentrations were for the sterols; there were significantly fewer detections and lower concentrations in MBR compared to OXI. This was driven by 3-beta-coprostanol, which was present in 8 of 9 OXI samples but not present in any MBR samples. On an individual CEC basis, there was general agreement in concentration differences between PRI and the two secondary treated effluents (e.g., diphenhydramine was significantly lower in both OXI and MBR, compared to PRI) (Table S5†). Furthermore, there was only one significant difference in concentrations between OXI and MBR effluents; phenytoin concentrations were greater in MBR effluents, compared to OXI.
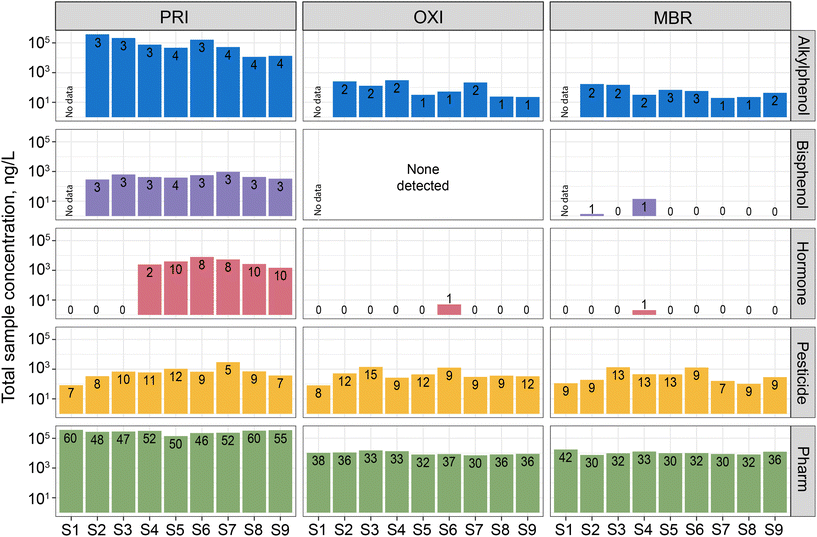 |
| Fig. 2 Sum of contaminant of concern concentrations detected within each sample, grouped by class. Numbers indicate the number of contaminants detected. Only classes represented by at least four contaminants are shown. PRI, primary-treated effluent; OXI, secondary effluent treated by oxidation ditches; MBR, secondary effluent treated by membrane bioreactor. | |
Percent removal of most CECs from PRI was generally consistent across sample dates. A total of 33 CECs (mostly pharmaceuticals) were 100% removed in all OXI and MBR samples, when more than one comparison could be made (Table S6†). High variability (mean ± >30%) in removal was observed for 52 CECs and often when high variability was observed for one of the secondary treatments, it was observed for both. In fact, percent removal was significantly different between OXI and MBR for only three CECs: fipronil, 3-beta-coprostanol, and cholesterol. Both 3-beta-coprostanol and cholesterol were fully removed from effluent when treated by MBR, but not OXI. Despite the apparent difference in removal, concentrations of both sterols in OXI were >95% lower, on average, compared to PRI. Slightly more removal of fipronil was observed from OXI treatment, compared to MBR, although percent removal for both treatments was negative. In fact, percent removal was negative for 15 CECs which is <10% of chemicals analyzed. This occurred when a CEC was detected in a secondary-treated effluent sample, but not the associated PRI sample. Although we did not use a Lagrangian sample design to track the fate of a distinct parcel of water through the two treatment streams, we have confidence in the observations regarding removal because the general patterns were relatively consistent across sample dates.
Similar removal rates of CECs were observed for MBR and OXI effluents, indicating that both processes provide similar benefits, in terms of CEC removal. It is important to note that both processes are operated similarly at the facility. For example, solid retention time is similar between MBR and OXI (28 and 24 days for MBR and OXI, respectively) so it might be expected that similar chemical transformation and degradation processes could occur. Increasing solid retention time can increase degradation of CECs that are not easily biodegraded.18,28
3.2. Bacteria loads in effluents and reduction from secondary treatments
HPC is a measure of the number of viable, culturable heterotrophic bacteria, or bacteria load, present in the environment and provides an understanding of water quality and water treatment efficacy. HPC ranging from 71 to 2.4 × 107 MPN/100 mL was detected in effluents (Fig. 3), with a significant difference among treatments (p = 2.2 × 10−3). Specifically, a significant difference was observed between the HPC detected in PRI and MBR (p = 7 × 10−3), with lower HPC in MBR. MBR effluents also exhibited the largest variation in bacteria loads among the treatments. MBR treatment incorporates membrane filtration which has proved more effective than conventional activated sludge treatment in removing organic and inorganic material,66 including viral coliphages and fecal indicator bacteria.67–69 Seasonal temperature fluctuations influence the viability of and functionality of critical nutrient-degrading microorganisms, such as ammonia-oxidizing bacteria, in conventional activated sludge more than MBR, which consequently reduces conventional activated sludge treatment efficiency.26,70 During our study, average maximum air temperatures ranged from 8.3 °C in April to 28 °C in June and July, with greater ranges in April and May. These temperature fluctuations could have influenced the treatment efficiency of OXI. Although seasonal fluctuations in microbiome communities have been reported, differences by year for a particular month may not be significant.71
 |
| Fig. 3 Heterotrophic plate counts (HPC) were significantly different among the three effluents with the highest HPC recovered in the primary treated effluent (PRI). Boxplots show the 25th to 75th percentiles with the median value indicated by the line within the box, whiskers extend to minimum and maximum values, and individual points indicate outliers. Navy blue dotted line indicates the limit of detection. MPN, most probable number; MBR, secondary effluent treated by membrane bioreactor; OXI, secondary effluent treated by oxidation ditches. | |
HPC measured in MBR and OXI effluent is similar to those concentrations reported from secondary effluents treated through either a conventional activated sludge process72,73 or primary sedimentation followed by an anaerobic–anoxic–oxic treatment.74 Both the MBR and OXI treatment have high removal efficacy rates of HPC with a 98.7% and 95.7% removal of HPC detected in PRI wastewater. These removal efficacy rates are 26–29% higher than those reported previously in ref. 72.
3.3. Wastewater microbiome among treatments
Within the PRI, OXI, and MBR effluent samples, a total of 2
406
614 sequences were binned at 97% similarity to 12
446 OTUs assigned to 44 bacterial phyla. Ten of those bacterial phyla and an unclassified bacteria group were considered dominant phyla as they each contributed over 1% of the total sequencing reads and collectively 93% of the sequence reads within the effluent microbiome (Fig. 4 and S1†).
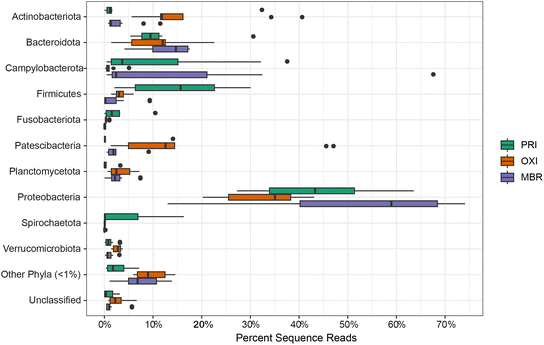 |
| Fig. 4 Bacteria phyla contributing to greater than 1% of sequence reads in the primary-treated effluent (PRI), secondary effluent treated by oxidation ditch (OXI), and secondary effluent treated by membrane bioreactor (MBR) microbiome sequencing library. Sequence reads were classified to an individual phylum if the read exceeded 97% sequence similarity. Phyla contributing less than 1% are grouped in the ‘other phyla’ category. The ‘unclassified’ category consists of sequence reads classified to the domain Bacteria, but not to an individual phylum. | |
Similar to results reported in other studies (e.g., ref. 8 and 26), Proteobacteria was the dominant phylum comprising approximately 44% of all sequencing reads across PRI, OXI, and MBR treatments and samples. In order of contribution within the effluent sequencing library, the remaining dominant phyla include Bacteroidota, Campilobacterota, Firmicutes, Actinobacteriota, Patescibacteria, Spirochaetota, Planctomycetota, Verrucomicrobiota, and Fusobacteriota. Several of these dominant phyla have been reported similarly in other studies assessing wastewater effluent microbiome.8,23,26,70,75–77 Proteobacteria species contribute to critical functional wastewater treatment roles involving hydrolysis,75 Proteobacteria and Actinobacteriota species participate in phosphorus removal,70,75,78 Proteobacteria and Firmicutes species are important for nitrogen removal,70,75,79 and Bacteroidota species are involved in molecular compound degradation and decomposition.76
These results expand on previous results by comparing the microbiomes of PRI effluent and effluent receiving secondary treatment by either OXI or MBR technologies. All the dominant phyla except for Bacteroidota, differ significantly in the percent contribution among the different effluent treatments (Table S7†). Of the 10 dominant phyla contributions, Firmicutes, Fusobacteriota, and Spirochaetota sequence reads are more prevalent in PRI than in either OXI or MBR. Actinobacteriota and Patescibacteria sequence reads are more abundant in the OXI microbiome than either the MBR or PRI microbiomes.
The most prevalent OTUs (Fig. S2†) within the effluent microbiome are classified within the dominant phyla of Proteobacteria, Bacteroidota, Campilobacterota, Firmicutes, Actinobacteriota, and Spirochaeta. The prevalent OTUs are variable between the PRI, OXI, and MBR microbiomes with only a Comamonadaceae and Mycobacterium OTU found to contribute abundant sequencing reads among all three wastewater treatment types. Comamonadaceae species' Comamonas denitrificans is frequently associated with wastewater treatment as an efficient denitrifier80 and the presence and relative abundance of similar Comamonadaceae OTUs among the PRI, OXI, and MBR microbiomes is unsurprising. The microbial community associated with oxidation ditch treatment influences pollutant removal efficiency.7 The OXI dominant OTUs of both Comamonadaceae and Saprospiraceae were also present and dominant members of the bacteria assemblage recovered from activated sludge in an oxidation ditch8 and are critical for degradation of aromatic compounds81 and denitrification,82 and protein hydrolysis,83 respectively.
Dominant members of the MBR microbiome include Flavobacterium, Nitrosomonas, Nitrospira, and Acinetobacter, which are associated with denitrification and nitrite reduction,84 ammonia oxidation and nitrite oxidation in nitrogen removal,8 and pollutant removal.85,86 Found in all three effluent streams, Comamonadaceae OTUs have been identified as poly(3-hydroxybutyrate-co-3-hydroxyvalerate) (PHBV)-degrading denitrifying bacteria87 and critical wastewater denitrifiers removing nitrogen.88 Additionally, Acinetobacter spp. are known to degrade aromatic hydrocarbons,89Flavobacterium spp. are associated with degradation of polycyclic aromatic hydrocarbons90 and industrial dyes,91 and Rhodobacter spp. are effective denitrifiers aiding in nitrogen removal,92 removal of heavy metals including cadmium and zinc,93 and have been shown to degrade organophosphorus pesticides.94 Further, Mycobacterium spp. OTUs which were prevalent in our wastewater microbiomes and globally associated with wastewater effluent95 can degrade aromatic hydrocarbons96,97 and steroids, including cholesterol,98 suggesting an important role in treating human waste. As psychrophilic bacteria, Flavobacterium and Acinetobacter grow best when wastewater temperature remains under 15 °C.8,84 Similarly, lower wastewater temperatures promote growth and survival of ammonia-oxidizing bacteria (i.e. Nitrosomonas) and nitrite-oxidizing bacteria (i.e. Nitrospira), as well as relative abundance8 and diversity.99
Several of the abundant OTUs present in the PRI, OXI, and MBR microbiomes are potential bacterial pathogens. Potential pathogens including Arcobacter, Acinetobacter, Treponema, Mycobacterium, Pseudomonas, and Leptotrichia OTUs are all present as dominant OTUs within the PRI microbiome; however, only Acinetobacter, Mycobacterium, and Arcobacter OTUs remain in either the OXI or MBR microbiome. The removal and reduction of some of these potentially pathogenic OTUs with OXI or MBR treatment as compared to PRI, suggests that these secondary treatment methods are effective in removing and reducing some pathogenic bacteria. Although wastewater treatment effectively reduces and removes several potential pathogens, others can persist in the effluent prior to disinfection (e.g., ref. 100 and 101). For instance, the relative abundance of Legionella increased from the PRI to OXI treatment. Although increased relative abundance does not necessarily indicate increased growth, previous studies have found an increase of Legionella cells up to two orders of magnitude between influent and effluent.19,102 It is important to remember that all sampling for this study was conducted prior to effluent disinfection in the plant before discharge to receiving waters. The relative increase of any potentially pathogenetic bacteria OTUs in OXI or MBR effluents does not represent an increase of discharge of these bacteria to surface waters.
The microbiome of OXI effluent had the highest OTU richness and diversity, as measured by the inverse Simpson Index and non-parametric Shannon Index (Fig. 5). Good's coverage is a measure of sequencing coverage and the PRI, MBR, and OXI microbiomes' coverage metrics ranged between 0.98 and 1.00 (median = 0.99) indicating high sequencing coverage at a sequencing depth of 30
000 reads. The high coverage suggests that our microbiome analysis represents a comprehensive analysis of the PRI, MBR, and OXI effluent microbiomes. The microbiomes of the different treatments were significantly different in the observed OTUs, or richness (p = 0.004), non-parametric Shannon diversity (p = 0.0002), and inverse Simpson diversity (p = 0.001). Specifically, the OXI microbiome was richer (p = 0.002) and more diverse (Shannon p = 0.0001 and inverse Simpson p = 0.0002) than the PRI effluent microbiome. Comparatively, these results suggest decreased species richness and diversity in MBR, compared to OXI, which has been theorized to be influenced by the solid retention time, proportion of nutrients to bacteria, or the availability of organic degradates.25,26 In fact, distinct MBR communities have been observed compared to influent and other treatment effluents.103 Mature MBR biofilms (collected more than five hours of operation after cleaning or installation of a new membrane) were found to be less diverse, compared to early MBR and activated sludge microbial communities.24 Furthermore, the differences were largely attributed to the presence of unique OTUs. The results from our study align with those observed by ref. 24, given the fact that our sampling would have been conducted when mature biofilms were present and influencing effluent microbial communities.
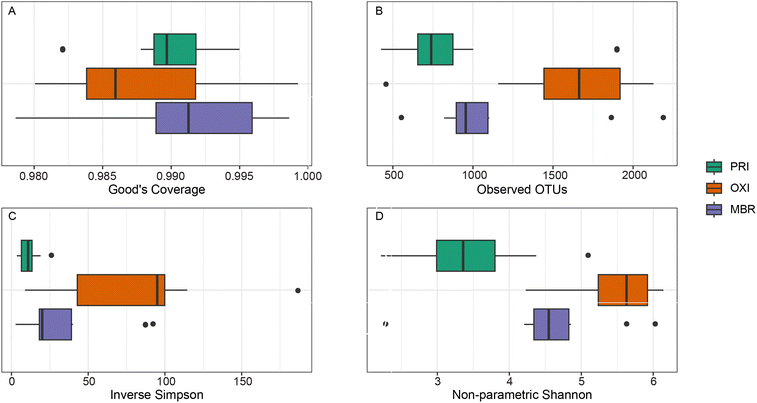 |
| Fig. 5 Alpha diversity metrics of primary-treated effluent (PRI), secondary effluent treated by membrane bioreactor (MBR), and secondary effluent treated by oxidation ditches (OXI): (A) Good’s coverage, (B), observed operational taxonomic units (OTUS), (C) inverse Simpson index, and (D) non-parametric Shannon index. | |
NMDS ordination illustrates separate clustering of the microbiome membership and structure among effluents, as defined by the Sorensen (Fig. 6A) and Bray-Curtis (Fig. 6B) distance matrices. One PRI sample (09072022) was an outlier, clustering with the OXI samples in both ordinations. Observed separation for all effluent types was significant (p < 0.001). The PRI microbiome membership is significantly more variable than that of the OXI (p < 0.001) and MBR microbiome (p = 0.006) (Fig. 6A). Considered collectively, the PRI microbiome membership and structure is significantly more variable than that of the OXI microbiome (p < 0.001, Fig. 6B). Analysis of variance indicates that wastewater microbiomes were significantly different (p = 1 × 10−4) with differences existing between the pairs of MBR-OXI, MBR-PRI, and OXI-PRI (p = 0.01). Treatment type explains 26% of variation in the microbiome of the different treatments.
 |
| Fig. 6 Non-metric multidimensional scaling (NMDS) ordinations of the Sorensen (presence/absence; A) and Bray-Curtis (abundance; B) distance matrices calculated from the wastewater microbiome membership (A) and structure (B) of the primary-treated effluent (PRI), secondary effluent treated by membrane bioreactor (MBR), and secondary effluent treated by oxidation ditches (OXI). | |
LEfSe analysis indicated that the linear discriminant analysis score was significantly different among 733 OTUs found in the microbiomes of the different effluent types (Fig. 7). Of those 733 OTUs, 74, 406, and 253 were more prevalent in the PRI, OXI, and MBR effluent microbiomes, respectively (Table S8†). The 406 OTUs significantly more prevalent in OXI effluent samples contributed to the OTU richness and likely influenced the elevated diversity metrics observed for OXI. The 253 OTUs more prevalent in the MBR effluent also elevated observed diversity above the value calculated for PRI effluent. Several taxonomic groups, including Polaromonas, Rhodopseudomonas, Nitrospira, Mycobacterium, Arcobacter, Legionella, and Sphingomonadaceae, had multiple OTUs that were significantly elevated in the microbiome of more than one treatment type. Although these OTUs were assigned to a similar genus, they were different enough to possibly indicate a different species or strain. Several of these OTUs that were significantly different among treatment types were also dominant OTUs (Fig. S2†) and have known associations with wastewater treatment, nitrogen and phosphorus cycling and removal, and pollutant treatment.
 |
| Fig. 7 Linear discriminant analysis (LDA) scores indicate specific operational taxonomic units (OTUs) that were significantly more prevalent in the microbiome of secondary effluent treated by membrane bioreactor (MBR), secondary effluent treated by oxidation ditches (OXI), or primary-treated effluent (PRI). Only scores of the top 50 OTUs are shown, the remaining significant LDA scores are listed in ESI† Table S8. LDA scores were calculated using non-parametric linear discriminant analysis effect size (LEfSe).60 LDA scores were considered significant if p < 0.05. OTUs are labelled with a “1” or “2” if more than one OTU was classified to a specific consensus taxonomy at over 97% similarity. ANP-Rhizobium = allorhizobium–neorhizobium–pararhizobium–rhizobium; un = unclassified; uc = uncultured. | |
The presence of particular CECs may influence the microbiome of effluents and our results indicate that some CEC groups were related to particular treatments. We observed significant relations between microbiome community structure and the concentration of all CEC groups, except hormones (Table 1), indicating that the CECs detected in the PRI, OXI, and MBR effluent streams significantly influenced differences in the respective effluent microbiomes. Pharmaceuticals and bisphenols had the highest relation (r = 0.46, p < 0.05) to relative abundance of the effluent microbiomes. Some microbial members (e.g., Achromobacter, Cupriavidus) have been shown to increase with increasing pharmaceutical concentrations, while other decrease (e.g., Comamonas).104 Because the pharmaceutical group reflects a diverse set of contaminants, we also explored the relation between types of pharmaceuticals and the effluent microbiomes. Out of 20 pharmaceutical groups, 6 had significant but weak correlations (r < 0.25, p < 0.05) to the relative abundance of effluent microbiome OTUs (Tables 1 and S9†). Other factors that can influence the effluent microbiome include environmental variables such as season and pH. Although these variables may have played a role in the microbiome communities at large, considering that the effluent samples were collected during one part of the year (i.e., little temperature fluctuation) and the secondary effluents were treated similarly (i.e., similar sludge retention times), we hypothesize that the presence of CECs contributed more to the observed differences between treatments.
Table 1 Relations between total contaminant concentrations, by group, and calculated Bray-Curtis distance matrix. Significant Spearman correlations are indicated in bold text (p < 0.05). For pharmaceutical groups, only significant correlations are listed. Results for all pharmaceutical groups are listed in Table S9.† Pharms = pharmaceuticals; Pest = pesticides; Horm = hormones; Corr = corrosion inhibitor; Alkyl = alkylphenols; Bisph = bisphenols; All = all groups
|
Spearman corelation coefficient |
Contaminant groups |
Pharms |
0.46 |
Pest |
0.11 |
Horm |
0.01 |
Sterols |
0.28 |
Corr |
0.13 |
Alkyl |
0.39 |
Bisph |
0.46 |
All |
0.44 |
Pharmaceutical groups |
Antacid |
0.21 |
Antidiabetic |
0.15 |
Antihistamine |
0.17 |
Beta blocker |
0.15 |
Calcium channel blocker |
0.19 |
Stimulant |
0.19 |
CEC concentrations were significantly associated with the separation of the PRI effluent microbiome composition and structure from that of the OXI and MBR effluent microbiomes (Fig. 8). Similarly, when considering pharmaceutical class, antacids, beta blockers, antidiabetic, antihistamine, antidepressant, anticonvulsant, and bronchodilator concentrations influenced the difference in microbiome membership and structure between the PRI effluent and that of the OXI and MBR effluents. When assessing the influence of these same pharmaceuticals on the presence/absence of microbiome membership, concentrations influenced significant spatial separation of OXI and MBR effluent microbiomes, and among PRI effluent microbiome membership (Fig. 8). The OXI microbiome is richer and more diverse than the PRI and MBR microbiomes, and specific pharmaceutical concentrations may select or enrich the growth of some OTUs recovered from the OXI microbiome. For instance, there was a significantly higher prevalence of a Rhodopseudomonas spp. in effluent microbiomes with pharmaceutical concentrations higher than 3 × 105 ng L−1. Rhodopseuduomonas have been frequently used for degradation of wastewater chemicals,105 including heavy oil,106 hormones,107 and pharmaceuticals.108–110 Although a majority of pharmaceuticals were detected at similar frequencies and concentrations in both the OXI and MBR effluents, five were detected only in OXI but not in MBR (Table S2†) at least once. On the contrary, only two pharmaceuticals were detected in MBR but not OXI. However, these few differences could be attributed to the sample design more than the microbial communities of the secondary treatments.
 |
| Fig. 8 Non-metric multidimensional scaling (NMDS) ordinations using Bray-Curtis distance (A and B) and Sorensen (C and D) measures fitted with significant contaminant vectors (gray lines). Plots are fitted with vectors calculated using total contaminant group concentrations (A and C) and pharmaceutical group concentrations (B and D). Vectors were determined using 999 permutations and significance determined when p < 0.05. The vector lengths are scaled by correlation to the strength of the predictors (vectors). Colors of points indicate effluent treatment type: primary-treated effluent (PRI), secondary effluent treated by oxidation ditches (OXI), and secondary effluent treated by membrane bioreactor (MBR). Abbreviations are as follows: STE = sterols, PHA = pharmaceuticals, ALK = alkylphenols, BIS = bisphenols, HOR = hormones, ACI = antacid, BB = beta blocker, DIA = antidiabetic, HIS = antihistamine, DEP = antidepressant, CON = anticonvulsant, BRO = bronchodilator. | |
Wastewater is an optimal medium for microorganisms,111 and primary and secondary treatment does not aim to reduce or eliminate microorganisms. However, the associated processes and environmental conditions maintained in these treatment systems select for certain microorganisms or bacterial groups and influence the microbiome composition112 as does biological interaction between the groups of microorganisms.70 For example, previous work has documented different bacterial communities, especially associated with nitrogen cycling, between MBR and conventional activated sludge systems.26 Differences may be attributed to the design of treatment systems or operating conditions associated with sludge residence time or flow.26,37
In our study, microbiome richness and diversity in the PRI, OXI, and MBR effluent streams appear to be influenced by differences in the OXI and MBR treatment processes, producing significant differences between the treatments (Fig. 5 and 6). These different microbiomes are an emergent property of the biological, chemical, and physical interactions within each treatment process, and the outcomes from these interactions appear to influence the chemical composition of the effluents (Table 1, Fig. 8). Previous work has observed that the removal efficiency of pollutants is tied to microbial community composition (e.g., ref. 7), and several of the dominant OTUs observed in our study are associated with pollutant removal (Table S8, Fig. S2†).
Conclusions
This study assessed the removal of select CECs, removal of bacterial loads, and structure of microbial communities in primary-treated effluent (PRI), secondary effluent treated by oxidation ditches (OXI), and secondary effluent treated by membrane bioreactor (MBR) by taking advantage of a full-scale system that splits primary-treated effluent between the two secondary treatments. Fewer CECs, and lower concentrations, were detected in OXI and MBR. We observed similar treatment efficiencies between OXI and MBR secondary treatments, indicating that MBR may be a useful option to consider when planning POTW upgrades. However, additional full-scale studies could provide confidence that MBR is a useful option in various scenarios. For example, our study represents treatment at one facility within a particular climate. Comparison of multiple POTWs that vary in geographic location (and climate) and comparison across seasons would be valuable. Additionally, we did not consider microbial-mediated process or function (i.e., nutrient cycling, biodegradation, antimicrobial resistance). Consideration of how secondary treatment processes enhance these processes would provide useful information to inform wastewater treatment in the future.
Data availability
Chemical data for this article are available in a U.S. Geological Survey data release at https://doi.org/10.5066/P901VGAX. Raw sequences, metadata, and processing information are available in the National Center for Biotechnology Information Sequence Read Archive under Accession Number PRJNA1149818.
Disclaimer
Any use of trade, firm, or product names is for descriptive purposes only and does not imply endorsement by the U.S. Government.
Author contributions
Conceptualization – CEG, SME, RLK, HLS. Data curation – CEG, SME, CHC. Formal analysis – CEG, SME. Funding acquisition – SME, RLK, HLS. Investigation – SME, RLK, CHC, HLS. Project administration – SME, RLK, HLS. Resources – CEG, CHC, HLS. Supervision – SME, RLK, HLS. Visualization – CEG, SME. Writing – original draft – CEG, SME, RLK. Writing – review & editing – CEG, SME, RLK, CHC, HLS.
Conflicts of interest
There are no conflicts to declare.
Acknowledgements
Funding for this study was provided by the Minnesota Environment and Natural Resources Trust Fund as recommended by the Legislative-Citizen Commission on Minnesota Resources (grant M.L. 2021, First Special Session, Chp. 6, Art. 6, Sec. 2, Subd. 04f), Minnesota Pollution Control Agency, and U.S. Geological Survey Cooperative Matching Funds. We thank the City of Hutchinson Wastewater Treatment Facility staff for their support and assistance with the study design and access to the facility. We also thank Ben Adolphson (USGS), Alissa VanDenBoom (St. Cloud State University), and Satomi Kohno (USGS) for their efforts in the field and assistance with sample collection.
References
-
(USEPA) U.S. Environmental Protection Agencyoo, Clean watersheds needs survey 2022, Report to Congress EPA-832-R-24-002, U.S. Environmental Protection Agency, Washington, DC, 2024, [Online], Available: https://www.epa.gov/system/files/documents/2024-05/2022-cwns-report-to-congress.pdf Search PubMed
.
- K. Noguera-Oviedo and D. S. Aga, Lessons learned from more than two decades of research on emerging contaminants in the environment, J. Hazard. Mater., 2016, 316, 242–251, DOI:10.1016/j.jhazmat.2016.04.058
.
- E. Butler, Y.-T. Hung, M. Suleiman Al Ahmad, R. Y.-L. Yeh, R. L.-H. Liu and Y. Fu, Oxidation pond for municipal wastewater treatment, Appl. Water Sci., 2017, 7(1), 31–51, DOI:10.1007/s13201-015-0285-z
.
- M. B. Ahmed, J. L. Zhou, H. H. Ngo, W. Guo, N. S. Thomaidis and J. Xu, Progress in the biological and chemical treatment technologies for emerging contaminant removal from wastewater: A critical review, J. Hazard. Mater., 2017, 323, 274–298, DOI:10.1016/j.jhazmat.2016.04.045
.
- Z. Li,
et al., Occurrence and ecological risk assessment of disinfection byproducts from chlorination of wastewater effluents in East China, Water Res., 2019, 157, 247–257, DOI:10.1016/j.watres.2019.03.072
.
- R. A. Molé,
et al., Correlating effluent concentrations and bench-scale experiments to assess the transformation of endocrine active compounds in wastewater by UV or chlorination disinfection, Chemosphere, 2019, 226, 565–575, DOI:10.1016/j.chemosphere.2019.03.145
.
- Y. Miura, M. N. Hiraiwa, T. Ito, T. Itonaga, Y. Watanabe and S. Okabe, Bacterial community structures in MBRs treating municipal wastewater: Relationship between community stability and reactor performance, Water Res., 2007, 41(3), 627–637, DOI:10.1016/j.watres.2006.11.005
.
- Y. Luo, J. Yao, X. Wang, M. Zheng, D. Guo and Y. Chen, Efficient municipal wastewater treatment by oxidation ditch process at low temperature: Bacterial community structure in activated sludge, Sci. Total Environ., 2020, 703, 135031, DOI:10.1016/j.scitotenv.2019.135031
.
- N. Morales, Á. Val Del Río, J. R. Vázquez-Padín, R. Méndez, J. L. Campos and A. Mosquera-Corral, The granular biomass properties and the acclimation period affect the partial nitritation/anammox process stability at a low temperature and ammonium concentration, Process Biochem., 2016, 51(12), 2134–2142, DOI:10.1016/j.procbio.2016.08.029
.
- R. Salvetti, A. Azzellino, R. Canziani and L. Bonomo, Effects of temperature on tertiary nitrification in moving-bed biofilm reactors, Water Res., 2006, 40(15), 2981–2993, DOI:10.1016/j.watres.2006.05.013
.
- H. Zhou, X. Li, G. Xu and H. Yu, Overview of strategies for enhanced treatment of municipal/domestic wastewater at low temperature, Sci. Total Environ., 2018, 643, 225–237, DOI:10.1016/j.scitotenv.2018.06.100
.
- O. Iorhemen, R. Hamza and J. Tay, Membrane bioreactor (MBR) technology for wastewater treatment and reclamation: membrane fouling, Membranes, 2016, 6(2), 33, DOI:10.3390/membranes6020033
.
- N. S. A. Mutamim, Z. Z. Noor, M. A. A. Hassan, A. Yuniarto and G. Olsson, Membrane bioreactor: Applications and limitations in treating high strength industrial wastewater, Chem. Eng. J., 2013, 225, 109–119, DOI:10.1016/j.cej.2013.02.131
.
- J. Ottoson, A. Hansen, B. Björlenius, H. Norder and T. A. Stenström, Removal of viruses, parasitic protozoa and microbial indicators in conventional and membrane processes in a wastewater pilot plant, Water Res., 2006, 40(7), 1449–1457, DOI:10.1016/j.watres.2006.01.039
.
- A. T. Besha, A. Y. Gebreyohannes, R. A. Tufa, D. N. Bekele, E. Curcio and L. Giorno, Removal of emerging micropollutants by activated sludge process and membrane bioreactors and the effects of micropollutants on membrane fouling: A review, J. Environ. Chem. Eng., 2017, 5(3), 2395–2414, DOI:10.1016/j.jece.2017.04.027
.
- W. Liu, X. Song, N. Huda, M. Xie, G. Li and W. Luo, Comparison between aerobic and anaerobic membrane bioreactors for trace organic contaminant removal in wastewater treatment, Environ. Technol. Innovation, 2020, 17, 100564, DOI:10.1016/j.eti.2019.100564
.
- H. V. Phan,
et al., Nutrient and trace organic contaminant removal from wastewater of a resort town: Comparison between a pilot and a full scale membrane bioreactor, Int. Biodeterior. Biodegrad., 2015, 102, 40–48, DOI:10.1016/j.ibiod.2015.02.010
.
- X. Ma, G. Shan, M. Chen, J. Zhao and L. Zhu, Riverine inputs and source tracing of perfluoroalkyl substances (PFASs) in Taihu Lake, China, Sci. Total Environ., 2018, 612, 18–25, DOI:10.1016/j.scitotenv.2017.08.235
.
- J. Ma, R. Dai, M. Chen, S. J. Khan and Z. Wang, Applications of membrane bioreactors for water reclamation: Micropollutant removal, mechanisms and perspectives, Bioresour. Technol., 2018, 269, 532–543, DOI:10.1016/j.biortech.2018.08.121
.
- K. C. Wijekoon, J. A. McDonald, S. J. Khan, F. I. Hai, W. E. Price and L. D. Nghiem, Development of a predictive framework to assess the removal of trace organic chemicals by anaerobic membrane bioreactor, Bioresour. Technol., 2015, 189, 391–398, DOI:10.1016/j.biortech.2015.04.034
.
- Z. U. Rehman, L. Fortunato, T. Cheng and T. Leiknes, Metagenomic analysis of sludge and early-stage biofilm communities of a submerged membrane bioreactor, Sci. Total Environ., 2020, 701, 134682, DOI:10.1016/j.scitotenv.2019.134682
.
- J. Wijaya and S. Oh, Machine learning reveals the complex ecological interplay of microbiome in a full-scale membrane bioreactor wastewater treatment plant, Environ. Res., 2023, 222, 115366, DOI:10.1016/j.envres.2023.115366
.
- M. M. Palanisamy, M. M. Lebbai and M. V. Ratnam, Treatment of Municipal Wastewater in a Fixed Aerated Bed: Use of Natural Fibrous Materials, Int. J. Biomater., 2022, 2022, 4839913, DOI:10.1155/2022/4839913
.
- G. K. Matar, S. Bagchi, K. Zhang, D. B. Oerther and E. Saikaly, Membrane biofilm communities in full-scale membrane bioreactors are not randomly assembled and consist of a core microbiome, Water Res., 2017, 123, 124–133, DOI:10.1016/j.watres.2017.06.052
.
- M. Hu, X. Wang, X. Wen and Y. Xia, Microbial community structures in different wastewater treatment plants as revealed by 454-pyrosequencing analysis, Bioresour. Technol., 2012, 117, 72–79, DOI:10.1016/j.biortech.2012.04.061
.
- C.-Y. Wan, H. De Wever, L. Diels, C. Thoeye, J.-B. Liang and L.-N. Huang, Biodiversity and population dynamics of microorganisms in a full-scale membrane bioreactor for municipal wastewater treatment, Water Res., 2011, 45(3), 1129–1138, DOI:10.1016/j.watres.2010.11.008
.
- R. Xu,
et al., The positive roles of influent species immigration in mitigating membrane fouling in membrane bioreactors treating municipal wastewater, Water Res., 2023, 235, 119907, DOI:10.1016/j.watres.2023.119907
.
- L. Goswami, R. Vinoth Kumar, S. N. Borah, N. Arul Manikandan, K. Pakshirajan and G. Pugazhenthi, Membrane bioreactor and integrated membrane bioreactor systems for micropollutant removal from wastewater: A review, J. Water Process Eng., 2018, 26, 314–328, DOI:10.1016/j.jwpe.2018.10.024
.
- L. N. Nguyen,
et al., Genome sequencing as a new window into the microbial community of membrane bioreactors – A critical review, Sci. Total Environ., 2020, 704, 135279, DOI:10.1016/j.scitotenv.2019.135279
.
- H. V. Phan, F. I. Hai, R. Zhang, J. Kang, W. E. Price and L. D. Nghiem, Bacterial community dynamics in an anoxic-aerobic membrane bioreactor – Impact on nutrient and trace organic contaminant removal, Int. Biodeterior. Biodegrad., 2016, 109, 61–72, DOI:10.1016/j.ibiod.2016.01.002
.
- Q. Wen, L. Yang, Y. Zhao, L. Huang and Z. Chen, Insight into effects of antibiotics on reactor performance and evolutions of antibiotic resistance genes and microbial community in a membrane reactor, Chemosphere, 2018, 197, 420–429, DOI:10.1016/j.chemosphere.2018.01.067
.
- A. S. Oberoi, L. Philip and S. M. Bhallamudi, Biodegradation
of various aromatic compounds by enriched bacterial Cultures: Part A – monocyclic and polycyclic aromatic hydrocarbons, Appl. Biochem. Biotechnol., 2015, 176(7), 1870–1888, DOI:10.1007/s12010-015-1684-1
.
- H. V. Phan,
et al., Simultaneous nitrification/denitrification and trace organic contaminant (TrOC) removal by an anoxic–aerobic membrane bioreactor (MBR), Bioresour. Technol., 2014, 165, 96–104, DOI:10.1016/j.biortech.2014.03.094
.
- L. Ye, M.-F. Shao, T. Zhang, A. H. Y. Tong and S. Lok, Analysis of the bacterial community in a laboratory-scale nitrification reactor and a wastewater treatment plant by 454-pyrosequencing, Water Res., 2011, 45(15), 4390–4398, DOI:10.1016/j.watres.2011.05.028
.
- E. R. Hester,
et al., Linking nitrogen load to the structure and function of wetland soil and rhizosphere microbial communities, mSystems, 2018, 3(1), e00214–e00217, DOI:10.1128/mSystems.00214-17
.
- P. H. Janssen, S. Yates, B. E. Grinton, M. Taylor and M. Sait, Improved culturability of soil bacteria and isolation in pure culture of novel members of the divisions Acidobacteria, Actinobacteria, Proteobacteria, and Verrucomicrobia, Appl. Environ. Microbiol., 2002, 68(5), 2391–2396, DOI:10.1128/AEM.68.5.2391-2396.2002
.
-
R. Manser, Population dynamics and kinetics of nitrifying bacteria in membrane and conventional activated sludge plants, Doctoral dissertation, Swiss Federal Institute of Technology Zurich, 2005, DOI:10.3929/ETHZ-A-005083976
.
- C. H. Christen,
et al., A comprehensive assessment of membrane bioreactor contaminant removal efficacy through analytical chemistry, fish exposures, and microbiome characterization, Environ. Toxicol. Chem., 2025, vgaf027, DOI:10.1093/etojnl/vgaf027
.
-
F. D. Wilde, M. W. Sandstrom and S. C. Skrobialowski, Selection of equipment for water sampling (ver. 3.1), U.S. Geological Survey Techniques of Water Resources Investigations book 9, chaA2, 2014, DOI:10.3133/twri09A2
.
-
E. T. Furlong, M. C. Noriega, C. J. Kanagy, L. K. Kanagy, L. J. Coffey and M. R. Burkhardt, Determination of human-use pharmaceuticals in filtered water by direct aqueous injection-high-performance liquid chromatography/tandem mass spectrometry, U.S. Geological Survey, Techniques and Methods book 5, chaB10, 2014, [Online], Available: DOI:10.3133/tm5B10
.
-
M. W. Sandstrom, L. K. Kanagy, C. A. Anderson and C. J. Kanagy, Determination of pesticides and pesticide degradates in filtered water by direct aqueous-injection liquid chromatograhpy-tandem mass spectrometry, U.S. Geological Survey, Techniques and Methods book 5, chaB11, 2015, [Online], Available: DOI:10.3133/tm5B11
.
-
W. T. Foreman, J. L. Gray, R. C. ReVello, C. E. Lindley, S. A. Losche and L. B. Barber, Determination of steroid hormones and related compounds in filtered and unfiltered water by solid-phase extraction, derivatization, and gas chromatography with tandem mass spectrometry, U.S. Geological Survey Techniques and Methods book 5, chaB9, 2012, [Online], Available: https://pubs.usgs.gov/tm/5b9/TM5-B9.pdf Search PubMed
.
-
W. C. Lipps, T. E. Baxter and E. Braun-Howland, 9215 heterotrophic plate count, in Standard Methods for the Examination of Water and Wastewater, American Public Health Association, 2017, DOI:10.2105/SMWW.2882.188
.
-
IDEXX Laboratories, IDEXX MPN Generator, 2022, 1.4.4 Search PubMed
.
-
D. N. Myers and M. A. Sylvester, Chapter A7, Section 7.1, Fecal indicator bacteria, 2014, DOI:10.3133/twri09A7.1
.
- J. J. Kozich, S. L. Westcott, N. T. Baxter, S. K. Highlander and D. Schloss, Development of a dual-index sequencing strategy and curation pipeline for analyzing amplicon sequence data on the MiSeq illumina sequencing platform, Appl. Environ. Microbiol., 2013, 79(17), 5112–5120, DOI:10.1128/AEM.01043-13
.
-
S. M. Elliott, C. E. Givens, R. L. Kiesling, C. H. Christen, S. Kohno and H. L. Schoenfuss, Data collected from wastewater effluent sampling and on-site fish exposures: trace organic contaminant concentrations baceria concentrations, and fish responses - Hutchinson, Minnesota, 2022, U.S. Geological survey data release, 2025, DOI:10.5066/P901VGAX.
-
R Core Team, R: A language and environment for statistical computing, R. R Foundation for Statistical Computing, Vienna, Austria, 2022, [Online], Available: https://www.R-project.org/ Search PubMed
.
- I. Papajová,
et al., Effect of Wastewater Treatment on Bacterial Community, Antibiotic-Resistant Bacteria and Endoparasites, Int. J. Environ. Res. Public Health, 2022, 19(5), 2750, DOI:10.3390/ijerph19052750
.
-
A. Dinno, Dunn's test of multiple comparisons using rank sums, R
package, 2017, Available: https://CRAN.R-project.org/package=dunn.test Search PubMed
.
-
P. Julian and D. Helsel, NADA2: Data analysis for censored environmental data, R package, 2021, Available: https://github.com/SwampThingPaul/NADA2 Search PubMed
.
- D. Schloss,
et al., Introducing mothur: Open-source, platform-independent, community-supported software for describing and comparing microbial communities, Appl. Environ. Microbiol., 2009, 75(23), 7537–7541, DOI:10.1128/AEM.01541-09
.
- D. Schloss and S. L. Westcott, Assessing and improving methods used in operational taxonomic unit-based approaches for 16S rRNA gene sequence analysis, Appl. Environ. Microbiol., 2011, 77(10), 3219–3226, DOI:10.1128/AEM.02810-10
.
- C. Quast,
et al., The SILVA ribosomal RNA gene database project: improved data processing and web-based tools, Nucleic Acids Res., 2012, 41(D1), D590–D596, DOI:10.1093/nar/gks1219
.
- P. Yilmaz,
et al., The SILVA and ‘All-species Living Tree Project (LTP)’ taxonomic frameworks, Nucleic Acids Res., 2014, 42(D1), D643–D648, DOI:10.1093/nar/gkt1209
.
- T. Rognes, T. Flouri, B. Nichols, C. Quince and F. Mahé, VSEARCH: a versatile open source tool for metagenomics, PeerJ, 2016, 4, e2584, DOI:10.7717/peerj.2584
.
-
H. Wickham, ggplot2: Elegant Graphics for Data Analysis, Springer-Verlag, New York, 2016. [Online]. Available: https://ggplot2.tidyverse.org Search PubMed
.
-
J. Oksanen, et al., vegan: Community Ecology Package, May 21, 2024, Accessed: May 29, 2024, [Online], Available: https://cran.r-project.org/web/packages/vegan/index.html Search PubMed
.
-
L. Lopaka, Nondetects and data analysis for environmental data, R, [Online], 2020, Available: https://cran.r-project.org/web/packages/NADA/index.html Search PubMed
.
- N. Segata,
et al., Metagenomic biomarker discovery and explanation, Genome Biol., 2011, 12(6), R60, DOI:10.1186/gb-2011-12-6-r60
.
- H. Wickham,
et al., Welcome to the Tidyverse, J. Open Source Softw., 2019, 4(43), 1686, DOI:10.21105/joss.01686
.
-
H. Wickham, ggplot2: Elegant Graphics for Data Analysis, Springer New York, New York, NY, 2009. DOI:10.1007/978-0-387-98141-3
.
- H. Wickham, Reshaping dat with the reshape package, J. Stat. Softw., 2007, 21(12), 1–20, DOI:10.18367/jss.v021.i12
.
- N. H. Tran, M. Reinhard and K. Y.-H. Gin, Occurrence and fate of emerging contaminants in municipal wastewater treatment plants from different geographical regions-a review, Water Res., 2018, 133, 182–207, DOI:10.1016/j.watres.2017.12.029
.
- M. Köck-Schulmeyer, M. Villagrasa, M. López de Alda, R. Céspedes-Sánchez, F. Ventura and D. Barceló, Occurrence and behavior of pesticides in wastewater treatment plants and their environmental impact, Sci. Total Environ., 2013, 458–460, 466–476, DOI:10.1016/j.scitotenv.2013.04.010
.
- S. Al-Asheh, M. Bagheri and A. Aidan, Membrane bioreactor for wastewater treatment: A review, Case Stud. Chem. Environ. Eng., 2021, 4, 100109, DOI:10.1016/j.cscee.2021.100109
.
- S. Bonetta, C. Pignata, E. Gasparro, L. Richiardi, S. Bonetta and E. Carraro, Impact of wastewater treatment plants on microbiological contamination for evaluating the risks of wastewater reuse, Environ. Sci. Eur., 2022, 34, 20, DOI:10.1186/s12302-022-00597-0
.
-
A. R. Vagadia, Comparison of bacterial and viral reduction across different wastewater treatment processes, Master's of Science, University of South Florida, 2018, [Online], Available: https://digitalcommons.usf.edu/etd/7586 Search PubMed
.
-
M. E. Verbyla, M. Von Sperling and Y. Maiga, Waste Stabilization Ponds, in Water and Sanitation for the 21st Century: Health and Microbiological Aspects of Excreta and Wastewater Management (Global Water Pathogen Project), Michigan State University, 2019, DOI:10.14321/waterpathogens.65.
- A. Cydzik-Kwiatkowska and M. Zielińska, Bacterial communities in full-scale wastewater treatment systems, World J. Microbiol. Biotechnol., 2016, 32(4), 66, DOI:10.1007/s11274-016-2012-9
.
- M. De Celis,
et al., Tuning up microbiome analysis to monitor WWTPs' biological reactors functioning, Sci. Rep., 2020, 10(1), 4079, DOI:10.1038/s41598-020-61092-1
.
- I. Howard, E. Espigares, P. Lardelli, J. L. Martín and M. Espigares, Evaluation of microbiological and physicochemical indicators for wastewater treatment, Environ. Toxicol., 2004, 19(3), 241–249, DOI:10.1002/tox.20016
.
- B. Xie, J. Gu and J. Lu, Surface properties of bacteria from activated sludge in relation to bioflocculation, J. Environ. Sci., 2010, 22(12), 1840–1845, DOI:10.1016/S1001-0742(09)60329-6
.
- J. J. Huang,
et al., Monitoring and evaluation of antibiotic-resistant bacteria at a municipal wastewater treatment plant in China, Environ. Int., 2012, 42(1), 31–36, DOI:10.1016/j.envint.2011.03.001
.
- Y. Chen,
et al., Occurrence, removal and environmental risk assessment of pharmaceuticals and personal care products in rural wastewater treatment wetlands, Sci. Total Environ., 2016, 566–567, 1660–1669, DOI:10.1016/j.scitotenv.2016.06.069
.
- C. Kragelund,
et al., Identity, abundance and ecophysiology of filamentous bacteria belonging to the Bacteroidetes present in activated sludge plants, Microbiology, 2008, 154(3), 886–894, DOI:10.1099/mic.0.2007/011684-0
.
- H. Qin, B. Ji, S. Zhang and Z. Kong, Study on the bacterial and archaeal community structure and diversity of activated sludge from three wastewater treatment plants, Mar. Pollut. Bull., 2018, 135, 801–807, DOI:10.1016/j.marpolbul.2018.08.010
.
- R. J. Seviour, C. Kragelund, Y. Kong, K. Eales, J. L. Nielsen and H. Nielsen, Ecophysiology of the Actinobacteria in activated sludge systems, Antonie van Leeuwenhoek, 2008, 94(1), 21–33, DOI:10.1007/s10482-008-9226-2
.
- H. Liu, Z. Hu, Y. Zhang, J. Zhang, H. Xie and S. Liang, Microbial nitrogen removal of ammonia wastewater in poly (butylenes succinate)-based constructed wetland: effect of dissolved oxygen, Appl. Microbiol. Biotechnol., 2018, 102(21), 9389–9398, DOI:10.1007/s00253-018-9386-6
.
- D. Numberger,
et al., Characterization of bacterial communities in wastewater with enhanced taxonomic resolution by full-length 16S rRNA sequencing, Sci. Rep., 2019, 9(1), 9673, DOI:10.1038/s41598-019-46015-z
.
- M. Höckenreiner, H. Neugebauer and L. Elango, Ex situ bioremediation method for the treatment of groundwater contaminated with PAHs, Int. J. Environ. Sci. Technol., 2015, 12(1), 285–296, DOI:10.1007/s13762-013-0427-5
.
- D. Xu, S. Liu, Q. Chen and J. Ni, Microbial community compositions in different functional zones of Carrousel oxidation ditch system for domestic wastewater treatment, AMB Express, 2017, 7, 40, DOI:10.1186/s13568-017-0336-y
.
- Y. Xia, Y. Kong, T. R. Thomsen and H. Nielsen, Identification and ecophysiological characterization of epiphytic protein-hydrolyzing Saprospiraceae (‘Candidatus epiflobacter’ spp.) in activated sludge, Appl. Environ. Microbiol., 2008, 74(7), 2229–2238, DOI:10.1128/AEM.02502–07
.
- E. Broman,
et al., Low temperature, autotrophic microbial denitrification using thiosulfate or thiocyanate as electron donor, Biodegradation, 2017, 28(4), 287–301, DOI:10.1007/s10532-017-9796-7
.
- A. Gonzalez-Martinez, B. Muñoz-Palazon, P. Maza-Márquez, A. Rodriguez-Sanchez, J. Gonzalez-Lopez and R. Vahala, Performance and microbial community structure of a polar Arctic Circle aerobic granular sludge system operating at low temperature, Bioresour. Technol., 2018, 256, 22–29, DOI:10.1016/j.biortech.2018.01.147
.
- R. Mortazavi, S. Attiya and A. Ariya, Arctic microbial and next-generation sequencing approach for bacteria in snow and frost flowers: Selected identification, abundance and freezing nucleation, Atmos. Chem. Phys., 2015, 15(11), 6183–6204, DOI:10.5194/acp-15-6183-2015
.
- S. T. Khan, Y. Horiba, M. Yamamoto and A. Hiraishi, Members of the family Comamonadaceae as primary Poly(3-Hydroxybutyrate-co-3-Hydroxyvalerate)-degrading denitrifiers in activated sludge as revealed by a polyphasic approach, Appl. Environ. Microbiol., 2002, 68(7), 3206–3214, DOI:10.1128/AEM.68.7.3206-3214.2002
.
- S. Rahimi, O. Modin and I. Mijakovic, Technologies for biological removal and recovery of nitrogen from wastewater, Biotechnol. Adv., 2020, 43, 107570, DOI:10.1016/j.biotechadv.2020.107570
.
- R. Simarro, N. González, L. F. Bautista and M. C. Molina, Assessment of the efficiency of in situ bioremediation techniques in a creosote polluted soil: Change in bacterial community, J. Hazard. Mater., 2013, 262, 158–167, DOI:10.1016/j.jhazmat.2013.08.025
.
- Y. Patel and A. Gupte, Evaluation of bioremediation potential of isolated bacterial culture YPAG-9 (Pseudomonas aeruginosa) for decolorization of sulfonated di-azodye reactive red HE8B under optimized culture conditions, Int. J. Curr. Microbiol. Appl. Sci., 2016, 5(8), 258–272, DOI:10.20546/ijcmas.2016.508.027
.
-
P. Sivaperumal, K. Kamala and R. Rajaram, Bioremediation of industrial waste through enzyme producing marine microorganisms, in Advances in Food and Nutrition Research, Elsevier, 2017, vol. 80, pp. 165–179, DOI:10.1016/bs.afnr.2016.10.006
.
- M. Zielińska, P. Rusanowska, J. Jarząbek and J. L. Nielsen, Community dynamics of denitrifying bacteria in full-scale wastewater treatment plants, Environ. Technol., 2016, 37(18), 2358–2367, DOI:10.1080/09593330.2016.1150350
.
- X. Li, W. Peng, Y. Jia, L. Lu and W. Fan, Removal of cadmium and zinc from contaminated wastewater using Rhodobacter sphaeroides, Water Sci. Technol., 2017, 75(11), 2489–2498, DOI:10.2166/wst.2016.608
.
- P. Wu,
et al., The organophosphorus pesticides in soil was degradated by Rhodobacter sphaeroides after wastewater treatment, Biochem. Eng. J., 2019, 141, 247–251, DOI:10.1016/j.bej.2018.07.019
.
- T. Zhang, M.-F. Shao and L. Ye, 454 Pyrosequencing reveals bacterial diversity of activated sludge from 14 sewage treatment plants, ISME J., 2012, 6(6), 1137–1147, DOI:10.1038/ismej.2011.188
.
- B. W. Bogan and W. R. Sullivan, Physicochemical soil parameters affecting sequestration and mycobacterial biodegradation of polycyclic aromatic hydrocarbons in soil, Chemosphere, 2003, 52(10), 1717–1726, DOI:10.1016/S0045-6535(03)00455-7
.
- M. A. Heitkamp and C. E. Cerniglia, Polycyclic aromatic hydrocarbon degradation by a Mycobacterium sin microcosms containing sediment and water from a pristine ecosystem, Appl. Environ. Microbiol., 1989, 55(8), 1968–1973, DOI:10.1128/aem.55.8.1968-1973.1989
.
- F. Guo, T. Zhang, B. Li, Z. Wang, F. Ju and Y. Liang, Mycobacterial species and their contribution to cholesterol degradation in wastewater treatment plants, Sci. Rep., 2019, 9(1), 836, DOI:10.1038/s41598-018-37332-w
.
- S. Siripong and B. E. Rittmann, Diversity study of nitrifying bacteria in full-scale municipal wastewater treatment plants, Water Res., 2007, 41(5), 1110–1120, DOI:10.1016/j.watres.2006.11.050
.
- I. Marín, P. Goñi, A. M. Lasheras and M. Ormad, Efficiency of a Spanish wastewater treatment plant for removal potentially pathogens: Characterization of bacteria and protozoa along water and sludge treatment lines, Ecol. Eng., 2015, 74, 28–32, DOI:10.1016/j.ecoleng.2014.09.027
.
- R. Mosteo, M. Ormad, P. Goñi, J. Rodríguez-Chueca, A. García and A. Clavel, Identification of pathogen bacteria and protozoa in treated urban wastewaters discharged in the Ebro River (Spain): Water reuse possibilities, Water Sci. Technol., 2013, 68(3), 575–583, DOI:10.2166/wst.2013.201
.
- K. Timraz, Y. Xiong, H. A. Qarni and Y. Hong, Removal of bacterial cells, antibiotic resistance genes and integrase genes by on-site hospital wastewater treatment plants: Surveillance of treated hospital effluent quality, Environ. Sci.:Water Res. Technol., 2017, 3(2), 293–303, 10.1039/c6ew00322b
.
- J. Wijaya and S. Oh, Machine learning reveals the complex ecological interplay of microbiome in a full-scale membrane bioreactor wastewater treatment plant, Environ. Res., 2023, 222, 115366, DOI:10.1016/j.envres.2023.115366
.
- M. Suleiman, F. Demaria, C. Zimmardi, B. A. Kolvenbach and F.-X. Corvini, Analyzing microbial communities and their biodegradation of multiple pharmaceuticals in membrane bioreactors, Appl. Microbiol. Biotechnol., 2023, 107(17), 5545–5554, DOI:10.1007/s00253-023-12677-z
.
- M. Li, P. Ning, Y. Sun, J. Luo and J. Yang, Characteristics and application of rhodopseudomonas palustris as a microbial cell factory, Front. Bioeng. Biotechnol., 2022, 10, 897003, DOI:10.3389/fbioe.2022.897003
.
- Y. Sun, X. Li, H. Xie and G. Liu, Removal of pollutants and accumulation of high-value cell inclusions in heavy oil refinery wastewater treatment system using Rhodopseudomonas and Pseudomonas: Effects of light intensity, Chem. Eng. J., 2022, 430, 132586, DOI:10.1016/j.cej.2021.132586
.
- K. Chojnacka,
et al., Biodegradation of pharmaceuticals in photobioreactors – a systematic literature review, Bioengineered, 2022, 13(2), 4537–4556, DOI:10.1080/21655979.2022.2036906
.
- M. U. Devi and B. Rajani, Treatment of pharmaceutical effluent using photosynthetic purple nonsulfur bacterium, World J. Pharm. Res., 2015, 4(7), 1482–1488 CAS
.
- E. Navrozidou, N. Remmas, P. Melidis, D. G. Karpouzas, G. Tsiamis and S. Ntougias, Biodegradation potential and diversity of diclofenac-degrading microbiota in an immobilized cell biofilter, Processes, 2019, 7(9), 554, DOI:10.3390/pr7090554
.
- J. Sun,
et al., Enhanced removal of veterinary antibiotic from wastewater by photoelectroactive biofilm of purple anoxygenic phototroph through photosynthetic electron uptake, Sci. Total Environ., 2020, 713, 136605, DOI:10.1016/j.scitotenv.2020.136605
.
- N. Abdel-Raouf, A. A. Al-Homaidan and I. B. M. Ibraheem, Microalgae and wastewater treatment, Saudi J. Biol. Sci., 2012, 19(3), 257–275, DOI:10.1016/j.sjbs.2012.04.005
.
- F. Ju, B. Li, L. Ma, Y. Wang, D. Huang and T. Zhang, Antibiotic resistance genes and human bacterial pathogens: Co-occurrence, removal, and enrichment in municipal sewage sludge digesters, Water Res., 2016, 91, 1–10, DOI:10.1016/j.watres.2015.11.071
.
|
This journal is © The Royal Society of Chemistry 2025 |
Click here to see how this site uses Cookies. View our privacy policy here.