DOI:
10.1039/D4GC05891G
(Paper)
Green Chem., 2025,
27, 5482-5497
Multi-scale computational screening and mechanistic insights of cyclic amines as solvents for improved lignocellulosic biomass processing†
Received
19th November 2024
, Accepted 27th February 2025
First published on 26th March 2025
Abstract
Lignocellulosic biomass is a promising feedstock for production of affordable fuels and chemicals from renewable resources. Effective solubilization and subsequent deconstruction of its cellulose, hemicellulose, and lignin fractions is essential for the viability of future biorefineries. This study used quantum chemistry-based equilibrium thermodynamics methods to evaluate the potential of 650 cyclic amines to solubilize cellulose, hemicellulose, and lignin. The activity coefficients of solvent - biopolymer interactions were predicted using the COSMO-RS (COnductor-like Screening MOdel for Real Solvents) method and used to identify cyclic amines that can efficiently dissolve and extract selective fractions of biopolymers during biomass pretreatment. Among the 650 cyclic amines, 1-piperazineethanmaine was predicted to be an effective solvent for extracting all three polymers and was experimentally shown to achieve the highest lignin removal (97.1%). Non-covalent interaction, reduced density gradient and quantum chemical calculations were performed to elucidate the dissolution mechanism of lignin, cellulose and hemicellulose and gain further molecular level insights into the interactions between the cyclic amines and biomass polymers that promote efficient solubilization and extraction. These analyses indicated that 1-piperazineethanmaine and 1-methylimidazole make noncovalent van der Waals, electrostatic interactions and hydrogen bonding with lignin, leading to enhanced lignin removal, while the strong intramolecular hydrogen bonding interactions in cellulose and hemicellulose result in weaker solvent-biopolymer interactions. Overall, the computational approach provided an efficient method for identifying cyclic amines tailored for optimal biomass pretreatment and resulted in the identification of a potential new class of solvents for effective biomass pretreatment.
Green foundation
Efficient biomass deconstruction remains a significant challenge for biorefineries. The potential of 650 cyclic amines to solubilize lignin, cellulose, and hemicellulose was assessed based on the activity coefficients of solvent - biopolymers predicted by COSMO-RS (COnductor-like Screening MOdel for Real Solvents).
The screening predicted that 1-piperazineethanmaine (PzEtN) was a very effective solvent for selective lignin extraction and promoted the highest lignin removal (97.1%) observed experimentally. Non-covalent interaction (NCI)-reduced density gradient (RDG) and quantum chemical calculations were conducted, indicating that PzEtN and 1-methylimidazole (MeIm) demonstrate robust electrostatic interactions and hydrogen bonding with lignin, which lead to enhanced lignin removal.
Overall, these computational techniques offer an efficient method for quickly identifying solvents tailored for optimal biomass pretreatment. Further research into other protic solvents capable of solubilizing lignocellulose would add to this work.
|
1. Introduction
The continued generation and use of liquid fuels and commodity chemicals requires development of alternative sources that are affordable and scalable. The most prevalent annual source of terrestrial carbon produced via photosynthesis is lignocellulosic biomass, and holistic utilization and conversion of lignocellulosic biomass into valuable chemicals and materials is essential for advancement of sustainable and economically viable biorefineries.1–4 A crucial part of this process is the efficient deconstruction of the biomass, which consists of cellulose, hemicellulose, and lignin tightly bound together. A multitude of chemical, physical, and biological pretreatment methods have been devised.5 Most prominent pretreatment methods are chemical-based, with a variety of solvents, such as alkali, dilute acids, organic solvents, ionic liquids (ILs), and deep eutectic solvents (DESs), being explored for biomass fractionation, delignification, and carbohydrate processing.6–8
The incorporation of pretreatment unit operations into lignocellulose conversion processes has demonstrated significant advantages in biomass fractionation and considerable benefits for subsequent processes.9 An optimal pretreatment solvent should fractionate diverse feedstock phenotypes (i.e., be feedstock agnostic), solubilize cellulose, hemicellulose, and lignin, and be recyclable, economical, and non-toxic to enzymes and microbial hosts.10 However, most of the documented pretreatment solvents exhibit certain deficiencies, including generation of inhibitory byproducts and the requirement for specific operational conditions. Amine-based pretreatment solvents have garnered significant attention in lignocellulose valorization due to their (a) high basicity and nucleophilicity that allows for the solubilization and fractionation of lignin, (b) miscibility/compatibility with water, and (c) ease of recovery based on their boiling point.11–14 The ester linkages between hemicellulose and lignin are selectively cleaved by amines through nucleophilic addition, leading to incorporation of nitrogen functional groups into the structure, thereby producing lignin with unique properties. Amines possess the potential to extract lignin with favorable characteristics and facilitate enzymatic hydrolysis of fractionated carbohydrates. Amine-based pretreatment, in conjunction with polymorphic transformation of cellulose, can markedly decrease the crystallinity index, thereby enhancing cellulose digestibility.12,15
Despite these advances, biomass pretreatment and lignocellulose fractionation remain a significant challenge, as the commonly used solvents typically have limited ability to solubilize all three biomass components. Furthermore, the pretreatment and fractionation step suffer from high cost, low solvent recyclability, negative environmental impacts, and a propensity to degrade useful biomolecules, rendering them unavailable for conversion to valuable fuels and chemicals.16 These limitations highlight the need for innovative solvent selection strategies for maximizing the efficacy of the solvent, while minimizing environmental impacts and toxicity to enzymes and microbial hosts. Given the extremely large number of potential solvents (e.g., ILs, DES, amines, and organic solvents), experimental screening is limited. Computational methods offer a promising solution to the challenge of identifying promising solvents from this huge pool of candidates. Multi-scale computational methods allow for an extensive screening of the unexplored solvent space, aiding in the discovery of efficient, non-obvious solvents based on their physicochemical properties, biomass’ component (or biopolymer) solubilities, and environmental, health, and safety (EHS) attributes.17–24
Amines, especially those with lower boiling points, offer several advantages over DESs and other ILs for biomass deconstruction, as amines can be easily recovered through distillation or vacuum evaporation, reducing energy consumption and improving process efficiency.25 Meanwhile, DESs and ILs often require more complex separation methods such as liquid–liquid extraction or solid–liquid separation due to their high melting points and strong hydrogen bonding.26,27,28 The lower viscosity of amines facilitates better mass transfer and penetration into biomass structures, overcoming the high viscosity often associated with several ILs and DESs that can impede efficient interaction with biomass. Additionally, amines are generally more cost-effective, being commercially available and less expensive than the DESs or ILs that require rigorous optimization and tailoring.
Recent work with amines12,14,29,30 as pretreatment solvents has established key advantages, including high solids loading, effective biomass fractionation and delignification, and ease of separation. These studies suggested a strong influence of the nucleophilicity and basicity on the pretreatment effectiveness, while also stressing the stability issues related to primary amines. Continuing our research efforts on amines as pretreatment solvents, herein we further explore the suitability of cyclic amines, an underutilized component of the amine toolbox, in lignocellulosic biomass processing. Cyclic amines, secondary or tertiary amines with one or more nitrogen atoms, are attractive due to their high nucleophilicity and basicity, higher stability, and availability (lower cost) compared to primary amines. This study provides a thorough computational screening of over 650 unexplored cyclic amine solvent candidates to identify specific solvents that can dissolve either a specific biomass component or all components simultaneously (see Scheme 1 for various classes of cyclic amines, including aromatic, employed in this study). Ultimately, to achieve a more profound mechanistic comprehension of the solvents’ role in biomass fraction dissolution, quantum chemical simulations were conducted to examine the interactions between the solvent and lignin, cellulose, and hemicellulose. The non-covalent interaction (NCI) and reduced density gradient (RDG) analyses were conducted to examine the strength and characteristics of hydrogen bonding in lignin and molecular solvents. This investigation revealed critical insights into lignin dissolution in cyclic amines, demonstrating that hydrogen bonding between the solvent and lignin is a primary factor influencing biomass breakdown. The computational findings were experimentally validated for two cyclic amines that were predicted to have high propensity to solubilize lignin. The COSMO-RS prediction, coupled with mechanistic insights into biopolymer dissolution, establishes a robust basis for swiftly discovering efficient solvents for biomass pretreatment and advancing economical lignocellulosic conversion technologies.
 |
| Scheme 1 Classification of identified solvents in different classes of amines, where R can be hydrogen or any variety of carbon-, oxygen-, nitrogen-, or halogen-based substituent. | |
2. Methods
2.1 Computational methods
COnductor-like Screening MOdel for Real Solvents (COSMO-RS) calculations were carried out to measure the capability of each cyclic amine to solubilize lignocellulosic biomass fractions based on the computed logarithmic activity coefficients. The ln(γ) values are often used as a quantitative descriptor for the dissolution power of a solvent. In the literature, ln(γ) has been reported as the dominating parameter in deciding the capability of a solvent, and has also been successfully employed in previous studies to predict the solubility of cellulose and lignin in solvents.12,31,32
The structural configurations of individual and complex molecules were initially constructed using the Turbomole software (TmoleX version 23) by incorporating the coordinates of lignin, cellulose, hemicellulose, PzEtN and MeIm from the literature or by drawing for complexes using the Turbomole program.33 It is important to clarify that TmoleX, by default, generates an initial geometry using RDKit's random distance geometry algorithm based on the Merck molecular force field (MMFF).34 Density functional theory (DFT) modeling was then used to optimize the various geometries and identify a low-energy conformer with no negative imaginary frequency. DFT modeling was carried out using the def2-TZVP basis set, achieving self-consistent field convergence at 1 × 10−6 Hartree within 500 cycles.
Cosmo files were generated using the BIOVIA COSMOtherm software (2023 edition), and σ-profiles were subsequently generated using the BP_TZVP_23.ctd parameterization to calculate the thermophysical properties. The resulting σ-profiles can be replicated by utilizing the identical software, basis set, and DFT parameterization, specifically the def2-TZVP basis and B3-LYP functional with DFT-D3(BJ) dispersion correction in TmoleX. We performed a search for conformations of biomolecules and amines using the Turbomole and BIOVIA COSMOconfX2023 program (version 23.0.0, BIOVIA, Germany), which automatically identifies conformers for subsequent COSMO-RS calculations. COSMO calculations in COSMOConf were performed using the BP-TZVP method and basis set. Fig. 1 shows the workflow of the proposed QM-based prediction methodology and latter experimental validation.
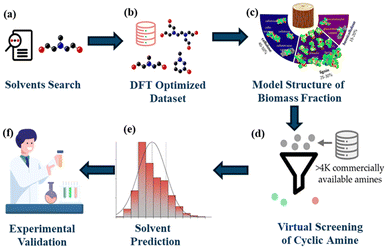 |
| Fig. 1 Workflow of the proposed methodology. (a) Data were collected from the literature on amines used as solvents; (b) the COSMO-RS model was applied to a diverse dataset containing 650 cyclic amine structures by first optimizing and generating the cosmo file; (c) the model structure of different biomass fractions; mainly cellulose, hemicellulose, and lignin were generated; (d) the activity coefficients of different biomass fractions were calculated for the different cyclic amines; (e) solvents were ranked based on the solute-solvent activity coefficient; and (f) solvents predicted to be the most effective were selected for further study and experimental testing. | |
The logarithmic activity coefficient, ln(γ), of component i is related to the chemical potential, as given in the following eqn (1):35,36
| 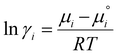 | (1) |
where,

is the chemical potential of the pure component
i, and
R and
T are the ideal gas constant and absolute temperature, respectively. Additional details on the methodology of COSMO-RS calculations in predicting the activity coefficients are provided elsewhere.
37–40
2.2 Quantum chemical calculations
In addition to COMO-RS calculations, quantum chemical (QC) calculations were performed to understand the mechanistic behavior of biomass fraction dissolution in the investigated cyclic amine solvents. For QC simulations, the structures of biopolymers - cyclic amine complexes were drawn in TmoleX. The geometries of the molecular systems were fully optimized at the specific def2-TZVP basis and B3-LYP functional with DFT-D3(BJ) dispersion correction in TmoleX, including different conformers.
From QC calculations, the interaction energy (ΔEInteraction Energy) is calculated by eqn (2):
|  | (2) |
where,
Ecomplex is the total energy of the complex system (
i.e., biopolymer + solvent) in kcal mol
−1.
Eindividual molecules are the energies of the individual biopolymer or protic solvent in kcal mol
−1. Furthermore, to examine the nature of intermolecular interactions in the biopolymer plus solvent systems, reduced density gradient non-covalent interactions (RDG-NCI) were analyzed using Multiwfn and VMD packages.
41,42
2.3 Modeling of representative biomass fractions
To predict the interaction of biomass components with cyclic amines as solvents, it is essential to first model these biomolecules with the continuum solvation model. The amounts of each component of the lignocellulosic biomass and the structure of the macromolecules may differ based on the biological source. Lignocellulosic biopolymers generally exhibit substantial molar weight (>150 kDa for cellulose, >30 kDa for hemicelluloses, and ∼2–15 kDa for lignin); thus, simulating their native structures is computationally challenging. To save computational time and complexity, the biomass fractions in this study were modeled separately as components of the original polymeric structure, as illustrated in Fig. 2. We modeled four structures as prospective representatives of the cellulose fraction, evaluated five structures for their capacity to represent the lignin fraction, and developed three model molecules for the hemicellulose fraction. The structures of all representative biomolecules (Fig. S1†) generated using the COSMO-RS model are included in the ESI† and were modeled at a quantum chemical level, as explained in the computational method of section 2.1.
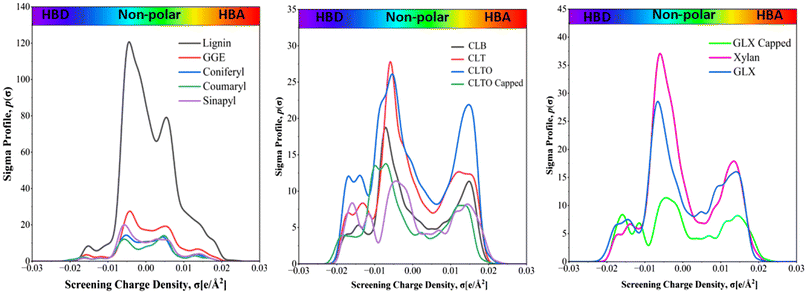 |
| Fig. 2 Sigma profiles of the representative biomolecules of biomass fractions: (a) lignin, (b) cellulose, and (c) hemicellulose calculated using the COSMO-RS model. | |
Cellulose.
Cellulose is composed of polymers of glucose monomers that are β-1-4-glycosidically connected and have a degree of polymerization of up to 10
000 units.43 These polymers are hydrogen bonded together to form crystalline cellulose. Cellulose is insoluble or only slightly soluble in the majority of common organic solvents44 because of its chemical structure and strong intra- and intermolecular hydrogen bonding. Although DMSO-tetrabutylammonium fluoride, N-methyl morpholine oxide (NMMO), and ILs are solvents that are known to be able to solubilize cellulose to a considerable degree, they are either toxic, thermally unstable, costly, and/or challenging to recycle.45 In the literature, various molecules of cellulose have been delineated. Casas et al. employed a single glucose monomer in the most basic scenario for the screening of ILs using the COMSO-RS model.31 Nevertheless, the solubilization properties of glucose are significantly different from those of cellulose. Chu et al. used glucose, cellobiose, cellotriose, and cellotetraose as typical molecules to predict the excess enthalpies of cellulose in ILs.46 The predicted excess enthalpies of cellobiose and cellotetraose had the strongest match with experimental solubility data in their investigation. A prevalent method for managing polymers using COSMO-RS involves doing quantum mechanical calculations on a computationally viable biopolymer fragment, followed by the truncation of its terminal groups. The entire polymer fragment undergoes geometric optimization, with mid-groups positioned as if integrated inside the polymer chain. The impact of the end-groups is typically minimal in the native polymer structure, necessitating their removal for solubility estimates. Both investigations emphasized the significance of intramolecular hydrogen bonding, which varies among different conformers of molecular cellulose. Yamin et al. asserts that hydrogen bonding is more accurately represented when two mid-monomers, as in cellotetraose, are considered rather than a single mid-monomer, as in a truncated cellotriose molecule.47 Utilizing this knowledge, we conducted a conformer search for all modeled biomolecules and evaluated cellobiose, cellotriose, cellotetraose, and a capped cellotetraose molecule for their efficacy as cellulose representations in interactions predictions.
Lignin.
Most lignin has a molar mass between 2500 and 15
000 Da and is an amorphous polymer composed of three main phenylpropanoid units, p-coumaryl, coniferyl, and sinapyl monolignols, bonded together via radical coupling reactions. Subunits of lignin include p-hydroxyphenyl (H), guaiacyl (G), and syringyl (S).48 The quantity of each subunit varies considerably based on the biomass source. Lignin derived from herbaceous biomass consists of all three subunits. Softwood lignin has mostly G units (>95%), while hardwood lignin predominantly consists of S and G units (the H content is typically <8%).49 The connections between the lignin subunits primarily consist of ether motifs and carbon–carbon bonds. The β-O-4 ether linkage is the predominant bond type joining monolignols and is also the most readily cleaved, but there are substantial quantities of other intricate connections that make lignin a challenging target for solubilization. Prior COSMO-RS investigations predominantly utilized monolignols as representatives of lignin.50 Casas et al. employed pinoresinol and guaiacyl glycerol-2-coniferyl ether with monolignols as typical lignin compounds.32 Gladden and coworkers employed S- and G-units linked through all principal linking motifs for solubility predictions in a different study.12,20 In this study, we modeled S, G, and H, together with dimers and trimers of S- and G-units linked by β-O-4 bonds. Furthermore, we simulated a conformer of a 2500 Da lignin fragment with DP = 26 for solubility predictions utilizing COSMO-RS.
Hemicellulose.
Hemicelluloses are a diverse class of polysaccharides found in plant cell walls. Hemicellulose primarily contains xylose as a sugar, with smaller amounts of mannose, galactose, arabinose, and rhamnose present as well. An acetyl group or uronic acid is another typical chain addition.51,52 The degrees of polymerization are typically between 50 and 300 units. The amorphous and hydrophilic hemicellulose dissolves quite easily in water at high temperatures (>150 °C). An exception to this is the lignin-carbohydrate complex (LCC) that exists in biomass when cellulose and hemicellulose are bound to lignin. Benzyl ether, ester, and phenyl glycosidic linkages are by far the most prevalent types of lignin-carbohydrate interactions. Due to its strong bonding, the LCC can partially degrade in alkaline environments and hinder the enzymatic hydrolysis of biomass.53 A combination of xylose and glucose monomers was used to represent hemicellulose in recent research studies.18,54 The hemicellulose fraction is represented in this investigation by glucuronoxylan, xylan, and capped glucuronoxylan, which are primarily found in dicots.
2.4 Environmental health and safety and boiling point
The physicochemical properties and hazards data for the studied compounds were obtained from established chemical databases. Boiling point data were retrieved from ChemSpider, a free chemical structure database maintained by the Royal Society of Chemistry. For compounds with multiple reported boiling points, only values at standard pressure (1 atm) were considered, and these values were averaged to obtain a single representative boiling point for each compound. Environmental, health, and safety (EHS) hazard classifications were extracted from PubChem, the open chemistry database of the National Institutes of Health. The hazard categories collected include acute toxicity, corrosivity, environmental hazards, flammability, general health hazards, and irritant properties. These classifications are based on the Globally Harmonized System of Classification and Labeling of Chemicals (GHS). Binary indicators were used to denote the presence of each hazard classification for each compound in the dataset.
2.5 Experimental methodology
Materials.
Sorghum (Sorghum bicolor) biomass was used throughout this work, and was donated from Idaho National Laboratories (Idaho Falls, USA). The biomass was dried at 40 °C for 24 h prior to use, and subsequently screened through a 2 mm screen (Thomas-Wiley Model 4, NJ, USA). The biomass was stored in a cool, dry place in a leak- and air-resistant container to avoid moisture until further use. Cyclic amines were purchased from Sigma Aldrich (St Louis, MO, USA), including 1-piperazineethanamine (1-(2-aminoethyl) piperazine) (99% purity) and 1-methylimidazole (≥99% purity). Additionally, sugar glucose (≥99.5%), xylose (≥99%), sodium hydroxide, acetic acid (glacial), lignin (alkali, 96% purity), acetyl bromide (99% purity), and ethanol (≥99%) were procured from Sigma-Aldrich, as well as sulphuric acid (72% and 95–98%) from VWR for high-performance liquid chromatography (HPLC) analysis.
Pretreatment of sorghum biomass.
In a typical experiment, 0.9 g of the biomass was mixed with 5.1 g of solvent, loaded into a glass pressure tube (30 mL, Ace Glass Inc., Vineland, NJ, USA), and mixed well prior to the experiments. The pretreatment experiments were performed at 140 °C for 3 h of reaction time at a solid loading of 15 wt%. The pretreatment of biomass sorghum was carried out using a two-step process that involves early separation (or washing) to remove the solvent after pretreatment. Post-pretreatment, some amount of ethanol and water mixture (1
:
1) was added to the slurry to transfer the contents to a 50 mL Falcon tube, which were then centrifuged at 4000 rpm to separate solids from liquids. Subsequently, the recovered solid was further washed with the same mixture to remove any residual cyclic amines. Finally, the recovered solid fractions were lyophilized before performing compositional analysis on the pretreated solids. All the experiments were performed in duplicate, and the average values were reported here. The solid recovery (%SR) after pretreatment was calculated based on eqn (3): |  | (3) |
Compositional analysis.
The compositional analysis of pretreated and untreated biomass sorghum was performed to determine the glucan and xylan content, following the modified two-step acid hydrolysis procedure previously described by NREL.55 In summary, 10 mg of the dry biomass was used, to which 100 μL of 72% w/w H2SO4 was added and incubated at 30 °C for 1 h. Subsequently, secondary hydrolysis was performed for all of the samples at 4% w/w H2SO4 by diluting the acid in the samples using ultra-pure water at 121 °C for 1 h. After the two-step acid hydrolysis, the hydrolysates were filtered using 0.45 μm filter plates (Pall AcroPrep). Additionally, monomeric sugars (glucose and xylose) were determined by HPLC using an Agilent 1200 (Santa Clara, CA, USA) series instrument equipped with a refractive index detector and Bio-Rad Aminex HPX-87H column (Bio-Rad, Richmond, CA, USA), coupled with a guard column assembly. Product separation was obtained at 60 °C with 4 mM H2SO4 as a mobile phase at a flow rate of 0.6 mL min−1.
For the lignin content, the acetyl bromide-based lignin assay method was employed, as reported previously. 10 mg of untreated or pretreated biomass was weighed in a 2 mL glass screwcap vial. 1 mL 25%(v/v) acetyl bromide in glacial acetic acid was added to the vials containing biomass samples, and incubated at 50 °C for 2 h with constant stirring at 300 rpm. After digestion, the sample was quickly cooled in an ice bath and then mixed with 60 μl of an acetic acid
:
2 M NaOH
:
5 M hydroxylamine mixture (48
:
10
:
2) and 200 μL glacial acetic acid sufficient for complete solubilization of the lignin extract. Following centrifugation (3000 rpm for 5 min), the absorbance of the supernatant was measured at 280 nm. A standard curve was generated with alkali lignin (TCI America) subjected to identical treatment with acetyl bromide in acetic acid (Caution: acetyl bromide must be operated in a fume hood).56,57
Structural characterization using P-XRD.
The powder X-ray diffraction (P-XRD) data were collected on a PANalytical Empyrean X-ray diffractometer equipped with a PIXcel3D detector, and operated at 40 kV and 30 mA using Cu Kα radiation (λ = 1.5418 Å). The diffraction patterns were collected in the 2θ range of 4°–40° with a step size of 0.026° and an exposure time of 30 s. The crystallinity index was also calculated using eqn (4), using the ratio of the height of the 002 peak (I002) and the height of the minimum (Iam) between the 002 and the 101 peaks.58,59 |  | (4) |
where, I002 is the intensity of the crystalline plane (002), and Iam is the minimum between (002) and (101) peaks and is at about 18°.
3. Results and discussion
3.1 Sigma profile of lignocellulosic biomass
The sigma (σ)-profiles of biomolecules provide information regarding their polarity, acidity, and basicity.38 The sigma profiles of the model compounds of biomass fractions (Fig. 2) were plotted and analyzed to determine the physicochemical properties of biomolecules. These profiles represent the probability p(σ) of a molecular surface segment having a specific screening charge density (SCD). The SCD refers to the distribution of charges on a molecule's surface, representing how the molecule interacts with its surrounding environment by creating a screening charge, essentially as a measure of the molecule's polarity at different points on its surface. The interaction energies with other liquid-phase surface segments are determined by the screening charge density σ(e Å−2). The chemical potential and all subsequent thermodynamic predictions in the COSMO-RS model theory are derived from the σ-profiles. The σ-profiles can be categorized as hydrogen bond donor (HBD) (−0.03 to −0.01 e Å−2), neutral (−0.01 to 0.01 e Å−2), and hydrogen bond acceptor (HBA) (0.01 to 0.03 e Å−2) regions.
As shown in Fig. 2(a), all lignin samples exhibit two peaks at −0.006 (the highest peak) and 0.005 e Å−2, resulting from the slightly electropositive hydrogen atoms of aromatic rings and carbon atoms, respectively. Polymeric lignin has the largest peak at −0.006 e Å−2 due to the aromatic ring's face. Oxygen atoms in β-O-4 bonds and unbound hydroxy groups cause the HBA behavior, as depicted in Fig. 2(a) for all lignin samples. The hydrogen atoms of the unbound hydroxy groups cause a tiny peak in the HBD area. Thus, inter- and intramolecular hydrogen bonding is feasible, albeit less intense than with cellulose and hemicellulose. Lignin has broader neutral area peaks compared to cellulose and hemicellulose in its σ-profiles, suggesting different solubilization characteristics. All cellulose representatives (see Fig. 2(b)) exhibit strong HBA- and HBD-behavior, with peaks at 0.017 and −0.018 e Å−2, respectively. There are opportunities for hydrogen bonds with possible solvents, and intramolecular hydrogen bonding is also possible. Additionally, cellulose exhibits a peak at −0.007 e Å−2 due to sugar carbons in the neutral area. The peak height increases with chain length, notably in the neutral zone, indicating decreased solubility for polar solvents. Capped cellotetraose exclusively uses cellobiose as a repeating unit by inactivating the terminal ends. The repeating units of polymers are deactivated as they represent similar behavior, and the handling of large polymers is computationally expensive. A solvent that dissolves cellulose should establish strong intermolecular hydrogen bonds and have neutral screening charges. Hemicellulose representatives (Fig. 2(c)) exhibit robust hydrogen bond formation due to their comparable σ-profiles to cellulose. Table S3† shows the model structures for the different biopolymers used in the present study.
To assess how COSMO-RS predictions correlate with experimental data, different lignin solubility prediction models (linear and non-linear) were developed for the amines with different physical properties including the activity coefficient, and reported an excellent correlation (R2 = 0.98) with the screening result.12 Another study also reported that the R-square of the longer chain lignin polymer and trimer (R2 = 0.88) were higher among the investigated lignin model structures when correlated with the experimental lignin solubility in ILs.19 Koenig-Mattern and coworkers also compared the experimental solubility for different types of lignin with COSMO-RS prediction results by linear regression analysis for monolignols, dimers, and trimers.60 They found that the average of all monolignols, dimers, trimers exhibited a correlation coefficient of R2 = 0.70. Overall, the comparison of experimental solubility data with COSMO-RS predictions for lignin and cellulose highlighted the importance of identifying meaningful representative molecules, and confirmed the suitability of COSMO-RS for qualitative solvent comparison for lignocellulose processing.61
3.2 Comprehensive dissolution of lignin, cellulose and hemicellulose
Solvent selection for the comprehensive dissolution of cellulose, lignin, and hemicellulose was a challenging task due to the complex nature of biomolecules. Fig. 3 shows the ln(γ) values for all three fractions of biomass in the 650 cyclic amines at 140 °C and 1 atm pressure. The more negative value of ln(γ) indicates the higher solubility, signifying that the solute is undergoing a positive interaction with the solvent. The values reported in this work are calculated at infinite dilution, unless otherwise specified. The solvents having the more negative value of ln(γ) for all three fractions of biomass are predicted as optimal solvents for the combined dissolution of lignin, cellulose, and hemicellulose. There are several solvents that were identified as optimal solvents for the improved dissolution of lignin, cellulose and hemicellulose fraction of biomass. Table S1† shows the activity coefficients for the biopolymers calculated using the COSMO-RS model at 140 °C and 1 atm pressure.
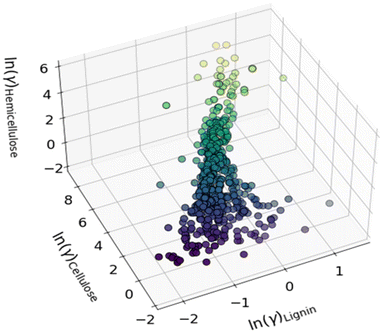 |
| Fig. 3 Comprehensive interaction of lignin, cellulose, and hemicellulose fractions with solvents at 140 °C and 1 atm pressure. The color represents the activity coefficients values from most negative (dark blue) to least negative (yellow). | |
Multiple research groups, including ours, have successfully established the suitability of COSMO-RS models in predicting the interactions between biopolymers (i.e., biomass fragments) and solvents (ILs, amines, etc.,) using activity coefficients (ln(γ)) and other physical properties.19,60,62,63 In addition, earlier studies showed that higher dissolution of plastic polymers, pharmaceuticals, and asphaltene in ILs and DESs was associated with more negative activity coefficients computed using COSMO-RS.35,64,65 The results discussed in this study align with previous studies, indicating that the calculated ln(γ) is a good predictor of a cyclic amine solvent's ability to solubilize components of lignocellulosic biomass, with more negative ln(γ) corresponding to increased solubility.
In addition to the activity coefficient, the solvent screening process incorporated criteria for both physical properties and safety considerations. The boiling point range was constrained to 180 °C–300 °C, with the lower limit ensuring thermal stability during biomass processing, and the upper limit facilitating energy-efficient solvent recovery and recycling. Environmental, health, and safety (EHS) classifications were evaluated using the Globally Harmonized System (GHS) framework, which categorizes hazards into six primary types: acute toxicity, corrosivity, environmental hazards, flammability, general health hazards, and irritant properties. Solvents were assessed based on their hazard's profiles, with preference given to candidates showing fewer hazard classifications. While the complete absence of hazard markers was ideal, solvents with up to four hazard classifications were considered acceptable if they suggested superior biomass processing capabilities. Solvents exhibiting all six hazardous categories were automatically excluded from consideration. This screening approach to solvent selection ensured that candidates not only met the technical requirements for effective biomass processing, but also aligned with the practical safety and handling considerations necessary for potential industrial implementation. However, one limitation is that we are unable to screen for side reactions that would create hazardous chemicals during processing. Such products would ultimately have to be experimentally screened for, as their development depends on the processing conditions.
3.3 Best solvents for cellulose and lignin
The solvent selection for joint dissolution of cellulose and lignin was challenging due to the entirely different behavior of lignin and cellulose. Fig. 4 shows the joint dissolution of lignin and cellulose for the cyclic amine's interactions at 140 °C and 1 atm pressure. The solvents having the more negative value of ln(γ) for lignin and cellulose are considered as the optimal solvents for combined dissolution. Fig. 4 is divided into four parts depending on the ln(γ) values for lignin and cellulose, which aids in identifying the solvents relative to their interaction with lignin and cellulose. Based on the plot data, some of the solvents were identified as the best solvents for the joint dissolution of lignin and cellulose, which were then tested experimentally. To further understand the behavior of hemicellulose and cellulose with cyclic amine solvents, we plotted the lignin vs. hemicellulose and cellulose vs. hemicellulose at the same operating conditions, which are shown in Fig. S1 and S2† respectively. The cellulose vs. hemicellulose plot shows a linear relationship indicating similar behaviors of the monomeric sugar units towards the solvents.
 |
| Fig. 4 Combined interaction plot for cellulose and lignin with the cyclic amine at 140 °C and 1 atm pressure. The color represents the activity coefficient values from most negative (dark blue) to least negative (yellow). | |
3.4 Selective lignin extraction using cyclic amines as the solvent
Multiple efforts have been made to identify appropriate solvents that can solubilize the lignin in the lignocellulosic biomass to enable the efficient processing of cellulose. Ideally, an appropriate solvent would selectively dissolve lignin and not interact with the holocellulosic component of the lignocellulosic biomass. Consequently, for this argument, we designated the best point as low-cellulose, high-lignin solubility solvents. Solvents preferentially extract lignin, leaving cellulose as a readily separable solid, consistent with typical fractionation methods. A substantial array of known solvents facilitates an understanding of the structural patterns responsible for the elevated lignin solubilities. We identified numerous structural similarities among the most promising solvent options from the list that aid in tuning solvents for lignin solvation. The detected solvents are categorized into the following principal classes: azines (including pyridines, pyrazines, pyrimidines, pyridazines, and triazines), and oxazolines. These solvents may possess superior solubility and more favorable EHS features, as shown for the top 30 candidates selected on the basis of selective lignin extraction in Table S2.†Fig. 5 shows the top 30 cyclic amines as solvents for this purpose based on the activity coefficients calculated at 140 °C and 1 atm pressure.
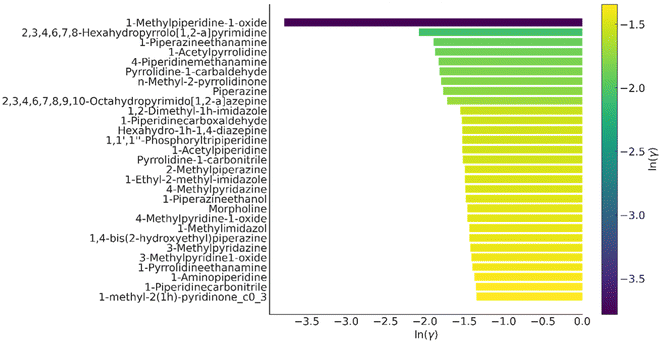 |
| Fig. 5 List of identified solvents selected for selective lignin extraction at 140 °C and 1 atm pressure with the identified class of cyclic amines, as depicted in Scheme 1. The scale color bar represents the activity coefficients values from the most negative (dark blue) to the least negative (yellow). | |
Aniline and pyridine were recently reported as solvents for lignin dissolution, all of which were rediscovered by the presented solvent screening framework.66 In addition to the already established lignin solvents such as pyridine, we discovered commercially available azoles such as thiazole or isoxazole. Thiazole is only slightly toxic (LD50 oral rat: 938 mg kg−1), while toxicity data for isoxazole is not available. Further aromatic N-heterocycles were designed, including triazines, diazines, pyridines, bicyclic compounds, and aromatic compounds. Common side chain motifs were methoxy-, alkyl-, and NH2-groups. Pyridines and many diazines have benign EHS properties, and are readily commercially available. Most triazines are solid at room temperature, limiting their applicability for lignin upgrading. During the solvent design, functional groups associated with low lignin solubilities (e.g., alkanes) were gradually replaced by functional groups associated with higher lignin solubilities (e.g., aromatic N-atoms), leading to a gradually increasing mean lignin solubility of the population. We were unable to find any experimental data on lignocellulose processing using 1-methylpiperidine-1-oxide, and it was not commercially available, although it was one of the best predicted solvents for the dissolution of cellulose and lignin. Despite its slightly higher price and lower thermal stability, 1-methylpiperidine-1-oxide is structurally similar to the solvent NMMO, which is known to have excellent cellulose dissolving properties.67 Similarly, according to the activity coefficients, 1-piperazinethanamine (PzEtN) and 1-methylimidazole (MeIm) were predicted to be better solvents for selective lignin extraction. However, ln(γ) for PzEtN for cellulose, indicating a lower dissolution capacity. Following this selection process, the cyclic amines MeIm and PzEtN were selected for experimental validation via pretreatment of sorghum biomass. Experimental findings in section 3.8 validate the prediction result, emphasizing the uniqueness of our results alignment as PzEtN showed the exceptionally higher lignin removal (97.1%) and lower cellulose dissolution. These predictions provide guidance for innovative QM based predictive framework utilizing newly identified solvents from the novel class of solvents, such as cyclic amine.
3.5 Sigma potential insights of biopolymers and cyclic amines interactions
The sigma potentials (μ(σ)) of the biopolymers cellulose, hemicellulose, lignin and the cyclic amines MeIm and PzEtN were calculated to understand the affinity of solvents for the surface polarity of different biomass fractions. The σ-potentials are divided into three main types: non-polar (0.01 e Å−2 < σ > +0.01 e Å−2), H-bond acceptor (σ < −0.01 e Å−2), and H-bond donor (σ > +0.01 e Å−2) regions (see Fig. 6). The sigma potentials, μ(σ), of the Lignin dimer (GGE), Cellobiose (CLB), and Glucuronoxylan (GLX) surrogate compounds are negative in both the negative and positive charge density regions (σ < −0.01 e Å−2 and σ > + 0.01 e Å−2), indicating that biopolymers tend to interact with both negative and positive polar surfaces of solvent molecules (i.e., H-bond donors and acceptors in the solvent). On the negative screening charge densities side (σ > −0.01 e Å−2), the σ-potential value of PzEtN (−2.0 kcal mol−1 Å2) is more negative than the MeIm σ-potential (−1.4 kcal mol−1 Å2), indicating that the former has more affinity to interact with the positive surface charge density of biopolymers (i.e., H-bond donors) and have high bond basicity, both of which would predict higher lignin solubility compared to cellulose and hemicellulose. In contrast, the σ-potential values of MeIm and PzEtN are positive (0.3–0.4 kcal mol−1 Å2) in the region of positive screening charge densities (σ > +0.01 e Å−2), which reflects that MeIm and PzEtN lack electron donor surfaces. Thus, the intramolecular interactions in MeIm and PzEtN are very weak, which would enable stronger interaction with the biopolymers. PzEtN has a higher tendency to interact with negatively charged surfaces, thereby forming stronger electrostatic and hydrogen bonding with lignin compared to MeIm. As a result, the logarithmic activity coefficient for PzEtN with lignin was higher than MeIm. PzEtN has shown a very weak interaction with CLB and GLX due to their existing strong intramolecular hydrogen bonding.
 |
| Fig. 6 COSMO-RS predicted sigma potentials for GGE, CLB, and GLX and the cyclic amines MeIM and PzEtN. | |
3.6 Understanding the mechanistic behavior of lignocellulosic biomass dissolution
Quantum chemical (QC) simulations were performed to study how the cyclic amines as solvents interact with cellulose, hemicellulose, and lignin, and to learn which interactions are critical for lignocellulosic biomass solvation. We selected guaiacyl glycerol-β-guaiacyl ether (GGE), a representative lignin dimer with the most common β-O-4 monomer–monomer linkage, as our model molecule for lignin because performing QC calculations with structures of large macromolecules like polymeric lignin is computationally expensive. Similarly, we used cellobiose (CLB) as the model compound for cellulose and glucuronoxylan (GLX) as hemicellulose to understand the solvation mechanism insights for different biomass fractions. The investigation of these (CLB, GLX, and GGE) biopolymer/amine systems becomes more complicated due to their ability to adopt multiple conformations. The Boltzmann distribution states that the most abundant distribution is characterized by the conformers having the lowest energy, so the lowest energy conformer structures of the different biomass fractions were simulated with the two cyclic amines as solvents, PzEtN and MeIm, and used to gain mechanistic insights into biomass solvation. The biopolymer-solvent clusters and their relative interaction energies are provided in Fig. 7. The lignin dimer (GGE) in PzEtN had the highest interaction energy compared to cellulose and hemicellulose. MeIm had ∼5 times lower interaction energy with GGE compared to PzEtN. The interaction energy of GLX and CLB with PzEtN was ∼10 times higher compared to MeIm, indicating that PzEtN interacts more strongly with all three biomass components. All optimized conformers showed that the interaction site for the α-OH, γ-OH, and phenol-OH groups of GGE form strong H-bonds with both cyclic amine solvents. Similarly, the amine functional group interacted with the hydroxyl group present in CLB, GLX and makes robust H-bonding. However, cellulose and glucuronoxylan possess a strong intramolecular hydrogen bonding as depicted by NCI-RDG and sigma potential. As a result, it becomes difficult for the solvents to penetrate and reduce the crystallinity as corroborated by experimental findings in PXRD analysis. In addition, NCI-RDG analysis was performed for the most stable optimized conformers to dissect the non-covalent interactions responsible for solvation of the biopolymers in MeIm and PzEtN.
 |
| Fig. 7 Optimized structures of GGE, CLB, and GLX with cyclic amines PzEtN and MeIm, along with the respective interaction energy of each complex. The dotted lines represent the H-bonds and the respective bond lengths in Angstroms (Å). C is represented by gray, O by red, N by blue, and H by white in the designated color scheme. | |
3.7 Reduced density gradient (RDG) analysis of biopolymers-amine solvents
Reduced Density Gradient (RDG) analysis was conducted to assess the strength of the non-covalent interactions (NCIs) between the CLB, GGE, GLX, and solvents, namely PzEtN and MeIm. This method can be used to visualize the different interaction energy contributions responsible for the dissolution of the different biomass fractions. The concept of RDG analysis is articulated using eqn (5), which focuses on the study of electron density in distribution areas characterized by both low electron density (ρ) and its low gradient values.68,69 | 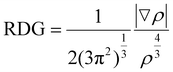 | (5) |
This equation sets the basis for NCI analysis, which interprets spikes in RDG plots as indicators of various weak non-covalent interactions. Utilizing RDG for NCI analysis can explain and quantify multiple weak interactions, such as H-bonds, vdW, and London dispersion interactions. The RDG scatter graph plotted against the electron density modified by the sign of the second eigenvalue of the electron density [sign(λ2)ρ] shows a spectrum of weak interactions. These non-covalent interactions are depicted through RDG isosurfaces and scatter plots, which display changes from positive to negative [sign(λ2)ρ] values for different compounds, as shown in Fig. 8a–f at using the def2-TZVP level of theory with D3BJ dispersion correction. Different colored peaks indicate different types of interactions. Blue areas (λ2 < 0) are linked with strong, attractive forces like hydrogen/halogen bonds and electrostatic interactions, while red areas (λ2 > 0) signify strong steric repulsion from nonbonded overlap. The intermediary or transition areas (λ2 ≅ 0) are depicted in green and track van der Waals interactions, such as dispersion forces and dipole–dipole interactions.
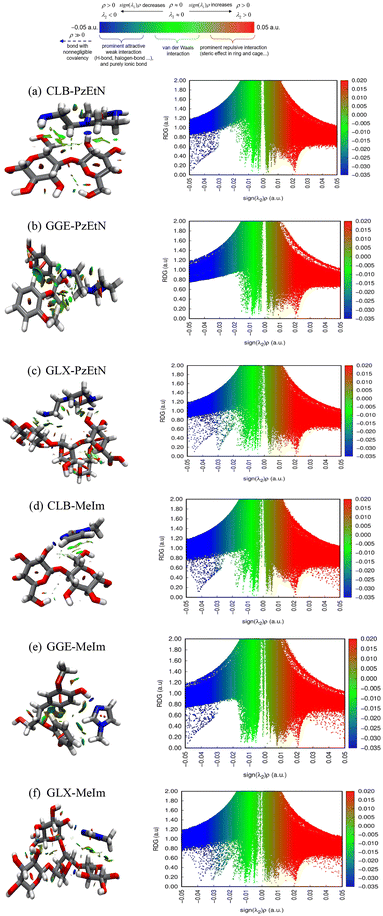 |
| Fig. 8 RDG scatter and NCI plots for 1-piperazineethanamine (PzEtN) and 1-methylimidazole (MeIm) solvents with GGE, CLB, and GLX. The RDG/NCI plots are colored on a blue-green-red scale according to the values of sign(λ2)ρ(r), ranging between −0.045 and 0.025. Red indicates steric repulsion, green indicates vdW interaction, and blue indicates strong attractive interactions. | |
The two cyclic amine solvents PzEtN and MeIm were explored in detail using this analysis (see Fig. 8a–f). Scanning across sign(λ2)ρ(r) from positive to negative values, there are several spikes in the RDG scatter plot that corresponds to the steric repulsion (red), van der Waal (green) interaction, and hydrogen bonding (blue). In Fig. 8a–c, the interactions are visualized for PzEtN interaction with CLB, GGE, and GLX, respectively. In general, if the RDG scatters in the range of ±0.005, weak interactions such as dispersion force exist; if the RDG scatters in the range of >0.005, strong repulsive interactions exist, such as steric hindrance in aromatic rings; and if the RDG scatters in the range <0.005, strong attractive interactions exist, such as hydrogen bonds. Examination of the NCI plots shows that the PzEtN have spikes in the negative region of sign(λ2)ρ(r). The reason for the extra, blue-colored spikes in CLB and GLX compared to GGE with PzEtN in the range of −0.05–0.03 was interpreted as being due to intramolecular hydrogen bonding between the complex structure of cellulose and hemicellulose. Fig. 8b shows the NCI-RDG plot of PzEtN-GGE. It shows that the minimal repulsive interaction compared to MeIm and no such intramolecular hydrogen bonding exists. PzEtN interacts with GGE from the primary amine and interacts with the β-O-4 bond of lignin. On the other hand, in the attractive region, multiple spikes are observed for biopolymer fractions-cyclic amine interactions, which are consistent with the geometrical analysis either from sigma potential or interaction energy. In addition, certain repulsive interaction peaks in the range of 0.03–0.05 (red) (Fig. 8d–f) are more prevalent in the MeIm-biopolymers system, which can be attributed to the repulsive interaction of aromatic rings and is predicted to result in lower dissolution of biomass.
3.8 Experimental validation: pretreatment with cyclic amines
As described, two cyclic amines with different predicted biomass solubilization properties, low cost, commercial availability, EHS, and boiling point MeIm and PzEtN, were selected to experimentally validate the results from the computational screening. To do so, pretreatment of sorghum biomass was performed, and the material obtained after the reaction was recovered and analyzed by measuring the lignin, glucan, and xylan removal. Solids recovery after pretreatment was 66.8% for MeIm and 48.8% for PzEtN, indicating a stronger biomass solubilization effect by the latter under the tested conditions (15 wt% solids, 140 °C, 3 h). The residual biomass composition varied significantly between treatments in terms of lignin and cellulose content. When using PzEtN, we observed almost complete lignin removal (97.1%) with lower glucan losses (24.7%) and a slightly higher xylan removal (40.9%) (Table 1). Pretreatment with MeIm resulted in significantly lower lignin removal (57.5%) and higher cellulose losses (51.1%). This aligns with the expected interactions between amine solvents and lignocellulosic biopolymers. Furthermore, the near-complete lignin removal in the case of PzEtN results in a biomass with a more fragmented and porous texture when compared to a granular texture for the biomass pretreated with MeIm (Fig. S3†). Since lignin extraction coincides with the removal of hemicellulose and cellulose, these results are consistent with typical behavior of biomass deconstruction. In a practical biorefinery setting, the extracted polysaccharides would be reintroduced into the saccharification reaction after solvent recovery.
Table 1 Compositional analysis of sorghum biomass before and after pretreatment with two cyclic amines
|
Solid recovery (%) |
Composition (%) |
Removal (%) |
Glucan |
Xylan |
Lignin |
Glucan |
Xylan |
Lignin |
Untreated |
|
39.1 ± 1.8 |
15.3 ± 0.1 |
28.4 ± 0.1 |
|
|
|
1-Methyl imidazole (MeIm) |
66.8 ± 1.6 |
28.5 ± 2.4 |
16.1 ± 1.3 |
17.9 ± 0.1 |
51.1 ± 2.0 |
29.8 ± 1.1 |
57.8 ± 0.1 |
1-Piperazineethanamine (PzEtN) |
48.8 ± 4.9 |
43.9 ± 1.2 |
18.6 ± 1.9 |
1.7 ± 0.1 |
24.7 ± 1.1 |
40.9 ± 1.6 |
97.1 ± 0.1 |
We also investigated the structural changes that occurred to cellulose after pretreatment with the cyclic amines using powder X-ray diffraction. Specifically, we aimed to identify the cellulose polymorphs and the ratio of crystalline to amorphous components in the lignocellulosic biomass since conversion of crystalline cellulose to a more amorphous form is known to increase the efficiency of saccharification and yields of fermentable sugars.21,22,70 Furthermore, a change in the crystallinity index (CrI) is an indication of structural changes and depends on (1) the amount of crystalline cellulose dissolved and precipitated during biomass pretreatment and (2) the loss of non-crystalline components during washing of the pretreatment solvent.71 The diffraction patterns observed in untreated sorghum correspond to the cellulose I polymorph, which is commonly associated with native cellulose found in untreated lignocellulosic biomass, with a CrI of 64.3%. When MeIm was used to pretreat the biomass, a lower CrI of 58.1% was obtained. Pretreatment with PzEtN resulted in a CrI of 63.6%. Fig. 9 shows the X-ray diffraction patterns of sorghum biomass before and after pretreatment with their CrI.
 |
| Fig. 9 X-ray diffraction patterns of sorghum biomass before and after pretreatment with the respective percentage of crystallinity index. | |
The contrasting effects of MeIm and PzEtN on lignin and cellulose reflect the importance of pretreatment choice for optimizing downstream processes. Pretreatments that decrease both lignin content and cellulose crystallinity tend to enhance enzyme accessibility, promoting more efficient cellulose hydrolysis. For instance, the moderate reduction in CrI and lignin content in the MeIm-treated sample depicts partial disruption of the cellulose structure due to pretreatment and result in broader and less intense peaks, which indicates a shift towards a more amorphous structure with granular and moderately broken-down texture (Fig. S3†).72,73 In contrast, for PzEtN pretreatment, despite significant lignin removal (97.1%), the CrI remains close to that of untreated sorghum biomass. It suggests pretreatment effectively removes amorphous lignin (and hemicellulose to some extent) but does not significantly impact cellulose crystallinity. The sharpness of peak at 22° supports this interpretation, as the cellulose microfibrils remain largely intact. This corresponds to the loose, disrupted texture seen in the image indicating a delignified yet crystalline cellulose structure (Fig. S3†). This outcome underscores the importance of balancing lignin removal with partial cellulose amorphization to maximize the saccharification yield.70,74
4. Conclusion
The current work demonstrated an effective framework for (1) discovering and predicting high-performing solvents for the deconstruction of different lignocellulosic biomass fractions, and (2) understanding the mechanistic factors that control the biopolymer dissolution capacity of cyclic amines as biomass solvents. The study established a quantum mechanics-based solvent screening framework for the improved processing of lignocellulosic biomass, which was then experimentally verified. The computational approach employed rigorously evaluated representative biomolecules to predict the activity coefficient of biomass fractions using the COSMO-RS model and sequentially it was later verified experimentally using 1-piperazineethanamine (PzEtN) and 1-methylimidazole (MeIm). Lignin extraction for two previously unexplored cyclic amines was much higher (97.1%) when compared to other reported solvents. The lignin dimer GGE and cyclic amine PzEtN showed the highest interaction energy compared to cellulose and hemicellulose, resulting in highest lignin removal. The MeIm showed ∼5 times lower interaction energy with GGE compared to PzEtN, resulting in lower lignin removal. The QC methods presented aid in identifying the functional groups that determine biopolymer solvation, such as azines and oxazolines, and they can be tuned easily by replacing degree of branching, functional group, and ring structures. The higher lignin removal by PzEtN may be more advantageous in biorefinery approaches requiring almost complete delignification, although additional steps may be necessary to address the retained cellulose crystallinity. As a future perspective, the feasibility and sustainability of the identified solvents in biorefinery processes, fractionation and downstream processing, including detailed toxicity and hazard analysis should be assessed. To incorporate the identified solvents into a particular biorefinery process, it is necessary to conduct analyses of their stability, recovery, recycling, cost, and product selectivity.
Data availability
The data associated with this study will be made available upon reasonable request to the corresponding authors.
Conflicts of interest
BAS has a financial interest in Illium Technologies, Caribou Biofuels, and Erg Bio. All other authors declare the absence of any commercial or financial relationships that could be construed as a potential conflict of interest.
Acknowledgements
The work conducted at the Joint BioEnergy Institute was supported by the U.S. Department of Energy, Office of Science, Biological and Environmental Research Program, through contract DE-AC02-05CH11231 between Lawrence Berkeley National Laboratory and the U.S. Department of Energy. The United States Government retains and the publisher, by accepting the article for publication, acknowledges that the United States Government retains a nonexclusive, paid-up, irrevocable, worldwide license to publish or reproduce the published form of this manuscript, or allow others to do so, for United States Government purposes. Any subjective views or opinions that might be expressed in the paper do not necessarily represent the views of the U.S. Department of Energy or the United States Government. Sandia National Laboratories is a multi-mission laboratory managed and operated by National Technology and Engineering Solutions of Sandia, LLC, a wholly owned subsidiary of Honeywell International Inc., for the U.S. DOE's National Nuclear Security Administration under contract DE-NA0003525.
References
- Z. Usmani, M. Sharma, A. K. Awasthi, T. Lukk, M. G. Tuohy and L. Gong,
et al., Lignocellulosic biorefineries: the current state of challenges and strategies for efficient commercialization, Renewable Sustainable Energy Rev., 2021, 148, 111258 CrossRef CAS.
- N. Singh, R. R. Singhania, P. S. Nigam, C.-D. Dong, A. K. Patel and M. Puri, Global status of lignocellulosic biorefinery: Challenges and perspectives, Bioresour. Technol., 2022, 344, 126415 CrossRef CAS PubMed.
- G. De Bhowmick, A. K. Sarmah and R. Sen, Lignocellulosic biorefinery as a model for sustainable development of biofuels and value added products, Bioresour. Technol., 2018, 247, 1144–1154 CrossRef CAS PubMed.
- B. Long, F. Zhang, S. Y. Dai, M. Foston, Y. J. Tang and J. S. Yuan, Engineering strategies to optimize lignocellulosic biorefineries, Nat. Rev. Bioeng., 2024, 1–15 Search PubMed.
- L. da Costa Sousa, S. P. Chundawat, V. Balan and B. E. Dale, ‘Cradle-to-grave'assessment of existing lignocellulose pretreatment technologies, Curr. Opin. Biotechnol., 2009, 20(3), 339–347 CrossRef PubMed.
- B. Kumar, N. Bhardwaj, K. Agrawal, V. Chaturvedi and P. Verma, Current perspective on pretreatment technologies using lignocellulosic biomass: An emerging biorefinery concept, Fuel Process. Technol., 2020, 199, 106244 CrossRef CAS.
- P. Kumar, D. M. Barrett, M. J. Delwiche and P. Stroeve, Methods for pretreatment of lignocellulosic biomass for efficient hydrolysis and biofuel production, Ind. Eng. Chem. Res., 2009, 48(8), 3713–3729 CrossRef CAS.
- M. Galbe and O. Wallberg, Pretreatment for biorefineries: a review of common methods for efficient utilisation of lignocellulosic materials, Biotechnol. Biofuels, 2019, 12(1), 294 CrossRef PubMed.
- S. Singh, G. Cheng, N. Sathitsuksanoh, D. Wu, P. Varanasi and A. George,
et al., Comparison of different biomass pretreatment techniques and their impact on chemistry and structure, Front. Energy Res., 2015, 2, 62 Search PubMed.
- K. H. Kim and C. G. Yoo, Challenges and perspective of recent biomass pretreatment solvents, Front. Chem. Eng., 2021, 3, 785709 CrossRef.
- L. Xu, J. Zhang, Q.-J. Zong, L. Wang, T. Xu and J. Gong,
et al., High-solid ethylenediamine pretreatment to fractionate new lignin streams from lignocellulosic biomass, Chem. Eng. J., 2022, 427, 130962 CrossRef CAS.
- E. C. Achinivu, M. Mohan, H. Choudhary, L. Das, K. Huang and H. D. Magurudeniya,
et al., A predictive toolset for the identification of effective lignocellulosic pretreatment solvents: a case study of solvents tailored for lignin extraction, Green Chem., 2021, 23(18), 7269–7289 RSC.
- L. Qin, W.-C. Li, J.-Q. Zhu, J.-N. Liang, B.-Z. Li and Y.-J. Yuan, Ethylenediamine pretreatment changes cellulose allomorph and lignin structure of lignocellulose at ambient pressure, Biotechnol. Biofuels, 2015, 8, 1–15 CrossRef PubMed.
- L. Xu, M. Cao, J. Zhou, Y. Pang, Z. Li and D. Yang,
et al., Aqueous amine enables sustainable monosaccharide, monophenol, and pyridine base coproduction in lignocellulosic biorefineries, Nat. Commun., 2024, 15(1), 734 CrossRef CAS PubMed.
- S. P. Chundawat, G. Bellesia, N. Uppugundla, L. da Costa Sousa, D. Gao and A. M. Cheh,
et al., Restructuring the crystalline cellulose hydrogen bond network enhances its depolymerization rate, J. Am. Chem. Soc., 2011, 133(29), 11163–11174 CrossRef CAS PubMed.
- L. Shuai and J. Luterbacher, Organic solvent effects in biomass conversion reactions, ChemSusChem, 2016, 9(2), 133–155 CrossRef CAS PubMed.
- M. Mohan, N. Kumar, V. V. Goud, B. A. Simmons, K. L. Sale and J. M. Gladden,
et al., Effect of cosolvent on the solubility of glucose in ionic liquids: Experimental and molecular dynamics simulations, Fluid Phase Equilib., 2022, 562, 113559 CrossRef CAS.
- M. Mohan, P. Viswanath, T. Banerjee and V. V. Goud, Multiscale modelling strategies and experimental insights for the solvation of cellulose and hemicellulose in ionic liquids, Mol. Phys., 2018, 116(15–16), 2108–2128 CrossRef CAS.
- M. Mohan, B. A. Simmons, K. L. Sale and S. Singh, Multiscale molecular simulations for the solvation of lignin in ionic liquids, Sci. Rep., 2023, 13(1), 271 CAS.
- M. Mohan, K. Huang, V. R. Pidatala, B. A. Simmons, S. Singh and K. L. Sale,
et al., Prediction of solubility parameters of lignin and ionic liquids using multi-resolution simulation approaches, Green Chem., 2022, 24(3), 1165–1176 CAS.
- H. Liu, K. L. Sale, B. A. Simmons and S. Singh, Molecular dynamics study of polysaccharides in binary solvent mixtures of an ionic liquid and water, J. Phys. Chem. B, 2011, 115(34), 10251–10258 CrossRef CAS PubMed.
- H. Liu, G. Cheng, M. Kent, V. Stavila, B. A. Simmons and K. L. Sale,
et al., Simulations reveal conformational changes of methylhydroxyl groups during dissolution of cellulose Iβ in ionic liquid 1-ethyl-3-methylimidazolium acetate, J. Phys. Chem. B, 2012, 116(28), 8131–8138 CAS.
- B. R. Taylor, N. Kumar, D. K. Mishra, B. A. Simmons, H. Choudhary and K. L. Sale, Computational Advances in Ionic Liquid Applications for Green Chemistry: A Critical Review of Lignin Processing and Machine Learning Approaches, Molecules, 2024, 29(21), 5073 CAS.
- N. Kumar, P. K. Naik and T. Banerjee, Molecular Dynamic Insights into the Distinct Solvation Structures of Aromatic and Aliphatic Compounds in Monoethanolamine-Based Deep Eutectic Solvents, J. Phys. Chem. B, 2022, 126(26), 4925–4938 CAS.
- W. Li, N. Sun, B. Stoner, X. Jiang, X. Lu and R. D. Rogers, Rapid dissolution of lignocellulosic biomass in ionic liquids using temperatures above the glass transition of lignin, Green Chem., 2011, 13(8), 2038–2047 CAS.
- N. Kumar and T. Banerjee, Dearomatization insights with phosphonium-based deep eutectic solvent: Liquid–liquid equilibria experiments and predictions, J. Chem. Eng. Data, 2021, 66(9), 3432–3442 CAS.
- N. Kumar and T. Banerjee, Molecular Mechanism and Solubility Performance Evaluation for Separation of Benzothiophene and Model Diesel Compounds through Deep Eutectic Solvents as Extractants, Ind. Eng. Chem. Res., 2022, 61(3), 1464–1474 CAS.
- E. L. Smith, A. P. Abbott and K. S. Ryder, Deep eutectic solvents (DESs) and their applications, Chem. Rev., 2014, 114(21), 11060–11082 CAS.
- E. C. Achinivu, S. Frank, N. R. Baral, L. Das, M. Mohan and P. Otoupal,
et al., Alkanolamines as dual functional solvents for biomass deconstruction and bioenergy production, Green Chem., 2021, 23(21), 8611–8631 CAS.
- S. Ntakirutimana, T. Xu, H. Liu, J.-Q. Cui, Q.-J. Zong and Z.-H. Liu,
et al., Amine-based pretreatments for lignocellulose fractionation and lignin valorization: a review, Green Chem., 2022, 24(14), 5460–5478 CAS.
- A. Casas, J. Palomar, M. V. Alonso, M. Oliet, S. Omar and F. Rodriguez, Comparison of lignin and cellulose solubilities in ionic liquids by COSMO-RS analysis and experimental validation, Ind. Crops Prod., 2012, 37(1), 155–163 CrossRef CAS.
- A. Casas, S. Omar, J. Palomar, M. Oliet, M. V. Alonso and F. Rodriguez, Relation between differential solubility of cellulose and lignin in ionic liquids and activity coefficients, RSC Adv., 2013, 3(10), 3453–3460 RSC.
- C. Steffen, K. Thomas, U. Huniar, A. Hellweg, O. Rubner and A. Schroer, TmoleX—a graphical user interface for TURBOMOLE, J. Comput. Chem., 2010, 31(16), 2967–2970 CrossRef CAS PubMed.
- P. Tosco, N. Stiefl and G. Landrum, Bringing the MMFF force field to the RDKit: implementation and validation, J. Cheminf., 2014, 6, 1–4 Search PubMed.
- N. Kumar, M. Mohan, J. C. Smith, B. A. Simmons, S. Singh and T. Banerjee, Inhibition of asphaltene aggregation using deep eutectic solvents: COSMO-RS calculations and experimental validation, J. Mol. Liq., 2024, 400, 124471 CrossRef CAS.
- K. A. Kurnia, P. SoP and C. JoA, Evaluation of the conductor-like screening model for real solvents for the prediction of the water activity coefficient at infinite dilution in ionic liquids, Ind. Eng. Chem. Res., 2014, 53(31), 12466–12475 CrossRef CAS.
- A. Klamt, Conductor-like screening model for real solvents: a new approach to the quantitative calculation of solvation phenomena, J. Phys. Chem., 1995, 99(7), 2224–2235 CrossRef CAS.
- A. Klamt and F. Eckert, COSMO-RS: a novel and efficient method for the a priori prediction of thermophysical data of liquids, Fluid Phase Equilib., 2000, 172(1), 43–72 CrossRef CAS.
- F. Eckert and A. Klamt, Fast solvent screening via quantum chemistry: COSMO–RS approach, AIChE J., 2002, 48(2), 369–385 CrossRef CAS.
- J. Reinisch, A. Klamt, F. Eckert and M. Diedenhofen, Prediction of the temperature dependence of a polyether–water mixture using COSMOtherm, Fluid Phase Equilib., 2011, 310(1–2), 7–10 CrossRef CAS.
- W. Humphrey, A. Dalke and K. Schulten, VMD: visual molecular dynamics, J. Mol. Graphics, 1996, 14(1), 33–38 CrossRef CAS PubMed.
- T. Lu and F. Chen, Multiwfn: A multifunctional wavefunction analyzer, J. Comput. Chem., 2012, 33(5), 580–592 CrossRef CAS PubMed.
- D. Klemm, B. Heublein, H. P. Fink and A. Bohn, Cellulose: fascinating biopolymer and sustainable raw material, Angew. Chem., Int. Ed., 2005, 44(22), 3358–3393 CrossRef CAS PubMed.
- C. Olsson and G. Westman, Direct dissolution of cellulose: background, means and applications, Cellul.: Fundam. Aspects, 2013, 10, 52144 Search PubMed.
- S. Köhler and T. Heinze, New solvents for cellulose: dimethyl sulfoxide/ammonium fluorides, Macromol. Biosci., 2007, 7(3), 307–314 CrossRef PubMed.
- Y. Chu, X. Zhang, M. Hillestad and X. He, Computational prediction of cellulose solubilities in ionic liquids based on COSMO-RS, Fluid Phase Equilib., 2018, 475, 25–36 CrossRef CAS.
-
P. Yamin, A. Bardow, K. O. Leonhard and I. Smirnova, COSMO-RS-based methods for improved modelling of complex chemical systems: Lehrstuhl für Technische Thermodynamik und Institut für Thermodynamik, 2019 Search PubMed.
- A. Tolbert, H. Akinosho, R. Khunsupat, A. K. Naskar and A. J. Ragauskas, Characterization and analysis of the molecular weight of lignin for biorefining studies, Biofuels, Bioprod. Biorefin., 2014, 8(6), 836–856 CrossRef CAS.
- R. Vanholme, K. Morreel, C. Darrah, P. Oyarce, J. H. Grabber and J. Ralph,
et al., Metabolic engineering of novel lignin in biomass crops, New Phytol., 2012, 196(4), 978–1000 CrossRef CAS PubMed.
- C. Balaji, T. Banerjee and V. V. Goud, COSMO-RS based predictions for the extraction of lignin from lignocellulosic biomass using ionic liquids: Effect of cation and anion combination, J. Solution Chem., 2012, 41, 1610–1630 CrossRef CAS.
- P. Sannigrahi, A. J. Ragauskas and G. A. Tuskan, Poplar as a feedstock for biofuels: a review of compositional characteristics, Biofuels, Bioprod. Biorefin., 2010, 4(2), 209–226 CrossRef CAS.
- H. V. Scheller and P. Ulvskov, Hemicelluloses, Annu. Rev. Plant Biol., 2010, 61(1), 263–289 CrossRef CAS PubMed.
- D. Tarasov, M. Leitch and P. Fatehi, Lignin–carbohydrate complexes: properties, applications,
analyses, and methods of extraction: a review, Biotechnol. Biofuels, 2018, 11, 1–28 CrossRef PubMed.
- M. Mohan, C. Balaji, V. V. Goud and T. Banerjee, Thermodynamic Insights in the Separation of Cellulose/Hemicellulose Components from Lignocellulosic Biomass Using Ionic Liquids, J. Solution Chem., 2015, 44(3–4), 538–557 CrossRef CAS.
-
A. Sluiter, B. Hames, R. Ruiz, C. Scarlata, J. Sluiter and D. Templeton, et al., Determination of structural carbohydrates and lignin in biomass. Technical Report NREL/TP-510-42618, National Renewable Energy Laboratory, 2008 Search PubMed.
- A. Yao, H. Choudhary, M. Mohan, A. Rodriguez, H. Magurudeniya and J. G. Pelton,
et al., Can multiple ions in an ionic liquid improve the biomass pretreatment efficacy?, ACS Sustainable Chem. Eng., 2021, 9(12), 4371–4376 CrossRef CAS.
- R. S. Fukushima, M. S. Kerley, M. H. Ramos, J. H. Porter and R. L. Kallenbach, Comparison of acetyl bromide lignin with acid detergent lignin and Klason lignin and correlation with in vitro forage degradability, Anim. Feed Sci. Technol., 2015, 201, 25–37 CrossRef CAS.
- L. Segal, J. J. Creely, A. Martin Jr and C. Conrad, An empirical method for estimating the degree of crystallinity of native cellulose using the X-ray diffractometer, Text. Res. J., 1959, 29(10), 786–794 CrossRef CAS.
- S. Park, J. O. Baker, M. E. Himmel, P. A. Parilla and D. K. Johnson, Cellulose crystallinity index: measurement techniques and their impact on interpreting cellulase performance, Biotechnol. Biofuels, 2010, 3, 1–10 CrossRef PubMed.
- L. Koenig-Mattern, A. O. Komarova, A. Ghosh, S. Linke, L. K. Rihko-Struckmann and J. Luterbacher,
et al., High-throughput computational solvent screening for lignocellulosic biomass processing, Chem. Eng. J., 2023, 452, 139476 CrossRef CAS.
- J. Heng, Z. Zhang, E. Proctor, M. Tyufekchiev, N. A. Deskins and M. T. Timko, Cellobiose as a model carbohydrate for predicting solubilities in nonaqueous solvents, Ind. Eng. Chem. Res., 2021, 60(4), 1859–1871 CrossRef CAS.
- J. Iqbal, N. Muhammad, A. Rahim, A. S. Khan, Z. Ullah and G. Gonfa,
et al., COSMO-RS predictions, hydrogen bond basicity values and experimental evaluation of amino acid-based ionic liquids for lignocellulosic biomass dissolution, J. Mol. Liq., 2019, 273, 215–221 CrossRef CAS.
- J. P. Wojeicchowski, A. M. Ferreira, T. Okura, M. Pinheiro Rolemberg, M. R. Mafra and J. A. Coutinho, Using COSMO-RS to predict hansen solubility parameters, Ind. Eng. Chem. Res., 2022, 61(42), 15631–15638 CrossRef CAS.
- M. Mohan, J. D. Keasling, B. A. Simmons and S. Singh, In silico COSMO-RS predictive screening of ionic liquids for the dissolution of plastic, Green Chem., 2022, 24(10), 4140–4152 RSC.
- M. Klajmon, Purely predicting the pharmaceutical solubility: What to expect from PC-SAFT and COSMO-RS?, Mol. Pharm., 2022, 19(11), 4212–4232 CrossRef CAS PubMed.
- J. Sameni, S. Krigstin and M. Sain, Solubility of lignin and acetylated lignin in organic solvents, BioResources, 2017, 12(1), 1548–1565 CrossRef CAS.
-
R. Wikandari, R. Millati and M. J. Taherzadeh, Pretreatment of lignocelluloses with solvent N-methylmorpholine N-oxide. Biomass fractionation technologies for a lignocellulosic feedstock based biorefinery, Elsevier, 2016, pp. 255–280 Search PubMed.
- E. R. Johnson, S. Keinan, P. Mori-Sánchez, J. Contreras-García, A. J. Cohen and W. Yang, Revealing noncovalent interactions, J. Am. Chem. Soc., 2010, 132(18), 6498–6506 CrossRef CAS PubMed.
- B. A. Marekha, O. N. Kalugin and A. Idrissi, Non-covalent interactions in ionic liquid ion pairs and ion pair dimers: a quantum chemical calculation analysis, Phys. Chem. Chem. Phys., 2015, 17(26), 16846–16857 RSC.
- G. Cheng, P. Varanasi, R. Arora, V. Stavila, B. A. Simmons and M. S. Kent,
et al., Impact of ionic liquid pretreatment conditions on cellulose crystalline structure using 1-ethyl-3-methylimidazolium acetate, J. Phys. Chem. B, 2012, 116(33), 10049–10054 CrossRef CAS PubMed.
- H. Choudhary, B. A. Simmons and J. M. Gladden, Comparative study on the pretreatment of aspen and maple with 1-ethyl-3-methylimidazolium acetate and cholinium lysinate, Front Energy Res., 2022, 10, 868181 CrossRef.
- A. D. French and M. Santiago Cintrón, Cellulose polymorphy, crystallite size, and the Segal Crystallinity Index, Cellulose, 2013, 20, 583–588 CrossRef CAS.
- Y. Pu, F. Hu, F. Huang, B. H. Davison and A. J. Ragauskas, Assessing the molecular structure basis for biomass recalcitrance during dilute acid and hydrothermal pretreatments, Biotechnol. Biofuels, 2013, 6, 1–13 CrossRef PubMed.
- M. Hall, P. Bansal, J. H. Lee, M. J. Realff and A. S. Bommarius, Cellulose crystallinity–a key predictor of the enzymatic hydrolysis rate, FEBS J., 2010, 277(6), 1571–1582 CrossRef CAS PubMed.
|
This journal is © The Royal Society of Chemistry 2025 |
Click here to see how this site uses Cookies. View our privacy policy here.